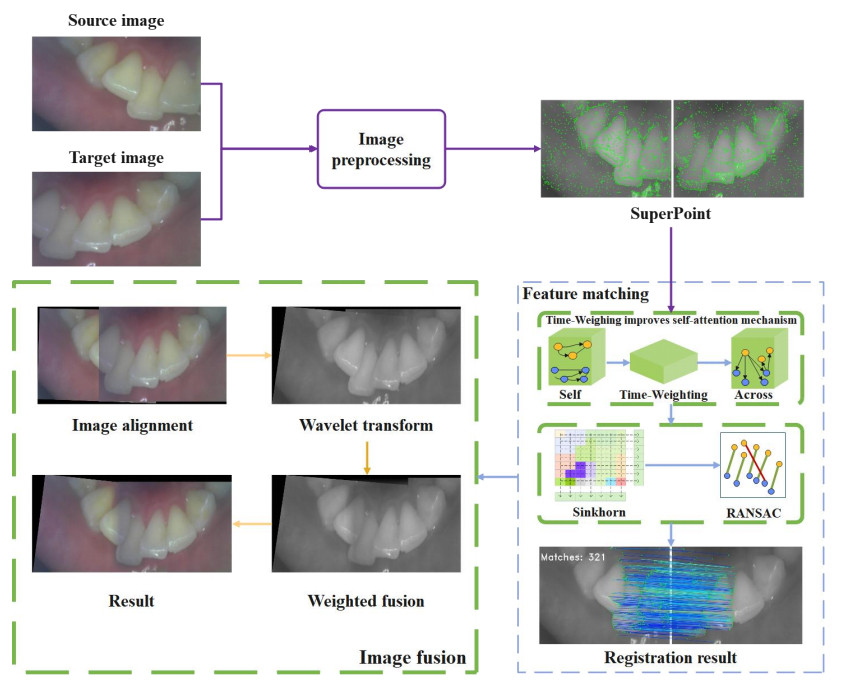
To address the challenges of repetitive and low-texture features in intraoral endoscopic images, a novel methodology for stitching panoramic half jaw images of the oral cavity is proposed. Initially, an enhanced self-attention mechanism guided by Time-Weighting concepts is employed to augment the clustering potential of feature points, thereby increasing the number of matched features. Subsequently, a combination of the Sinkhorn algorithm and Random Sample Consensus (RANSAC) is utilized to maximize the count of matched feature pairs, accurately remove outliers and minimize error. Last, to address the unique spatial alignment among intraoral endoscopic images, a wavelet transform and weighted fusion algorithm based on dental arch arrangement in intraoral endoscopic images have been developed, specifically for use in the fusion stage of intraoral endoscopic images. This enables the local oral images to be precisely positioned along the dental arch, and seamless stitching is achieved through wavelet transformation and a gradual weighted fusion technique. Experimental results demonstrate that this method yields promising outcomes in panoramic stitching tasks for intraoral endoscopic images, achieving a matching accuracy of 84.6% and a recall rate of 78.4% in a dataset with an average overlap of 35%. A novel solution for panoramic stitching of intraoral endoscopic images is provided by this method.
Citation: Tian Ma, Boyang Meng, Jiayi Yang, Nana Gou, Weilu Shi. A half jaw panoramic stitching method of intraoral endoscopy images based on dental arch arrangement[J]. Mathematical Biosciences and Engineering, 2024, 21(1): 494-522. doi: 10.3934/mbe.2024022
[1] | Ning He, Hongmei Jin, Hong'an Li, Zhanli Li . A global optimization generation method of stitching dental panorama with anti-perspective transformation. Mathematical Biosciences and Engineering, 2023, 20(9): 17356-17383. doi: 10.3934/mbe.2023772 |
[2] | Duolin Sun, Jianqing Wang, Zhaoyu Zuo, Yixiong Jia, Yimou Wang . STS-TransUNet: Semi-supervised Tooth Segmentation Transformer U-Net for dental panoramic image. Mathematical Biosciences and Engineering, 2024, 21(2): 2366-2384. doi: 10.3934/mbe.2024104 |
[3] | Shuai Cao, Biao Song . Visual attentional-driven deep learning method for flower recognition. Mathematical Biosciences and Engineering, 2021, 18(3): 1981-1991. doi: 10.3934/mbe.2021103 |
[4] | Fang Zhu, Wei Liu . A novel medical image fusion method based on multi-scale shearing rolling weighted guided image filter. Mathematical Biosciences and Engineering, 2023, 20(8): 15374-15406. doi: 10.3934/mbe.2023687 |
[5] | Xiao Zou, Jintao Zhai, Shengyou Qian, Ang Li, Feng Tian, Xiaofei Cao, Runmin Wang . Improved breast ultrasound tumor classification using dual-input CNN with GAP-guided attention loss. Mathematical Biosciences and Engineering, 2023, 20(8): 15244-15264. doi: 10.3934/mbe.2023682 |
[6] | Zhijing Xu, Jingjing Su, Kan Huang . A-RetinaNet: A novel RetinaNet with an asymmetric attention fusion mechanism for dim and small drone detection in infrared images. Mathematical Biosciences and Engineering, 2023, 20(4): 6630-6651. doi: 10.3934/mbe.2023285 |
[7] | Yong Tian, Tian Zhang, Qingchao Zhang, Yong Li, Zhaodong Wang . Feature fusion–based preprocessing for steel plate surface defect recognition. Mathematical Biosciences and Engineering, 2020, 17(5): 5672-5685. doi: 10.3934/mbe.2020305 |
[8] | Ziyue Wang, Junjun Guo . Self-adaptive attention fusion for multimodal aspect-based sentiment analysis. Mathematical Biosciences and Engineering, 2024, 21(1): 1305-1320. doi: 10.3934/mbe.2024056 |
[9] | Xi Lu, Xuedong Zhu . Automatic segmentation of breast cancer histological images based on dual-path feature extraction network. Mathematical Biosciences and Engineering, 2022, 19(11): 11137-11153. doi: 10.3934/mbe.2022519 |
[10] | Hui Yao, Yuhan Wu, Shuo Liu, Yanhao Liu, Hua Xie . A pavement crack synthesis method based on conditional generative adversarial networks. Mathematical Biosciences and Engineering, 2024, 21(1): 903-923. doi: 10.3934/mbe.2024038 |
To address the challenges of repetitive and low-texture features in intraoral endoscopic images, a novel methodology for stitching panoramic half jaw images of the oral cavity is proposed. Initially, an enhanced self-attention mechanism guided by Time-Weighting concepts is employed to augment the clustering potential of feature points, thereby increasing the number of matched features. Subsequently, a combination of the Sinkhorn algorithm and Random Sample Consensus (RANSAC) is utilized to maximize the count of matched feature pairs, accurately remove outliers and minimize error. Last, to address the unique spatial alignment among intraoral endoscopic images, a wavelet transform and weighted fusion algorithm based on dental arch arrangement in intraoral endoscopic images have been developed, specifically for use in the fusion stage of intraoral endoscopic images. This enables the local oral images to be precisely positioned along the dental arch, and seamless stitching is achieved through wavelet transformation and a gradual weighted fusion technique. Experimental results demonstrate that this method yields promising outcomes in panoramic stitching tasks for intraoral endoscopic images, achieving a matching accuracy of 84.6% and a recall rate of 78.4% in a dataset with an average overlap of 35%. A novel solution for panoramic stitching of intraoral endoscopic images is provided by this method.
As living standards improve, people are placing increasing emphasis on dental health. With the rising number of patients, clinicians face the cumbersome task of image interpretation, which undermines the efficiency of healthcare services. In recent years, intelligent diagnostic systems based on intraoral endoscopy have emerged as vital adjunct tools for oral treatment, capable of conducting dental lesion image segmentation and preliminary diagnosis [1]. However, due to the confined space within the oral cavity and the limited field of view of the endoscope, each capture yields only fragmentary images of a few teeth, making it difficult to provide a continuous, comprehensive diagnostic assessment of the entire jaw. To address this issue, panoramic stitching of half jaw images has become a key.
Compared to other images, intraoral endoscopic images present unique challenges due to their short focal length, limited shooting range and the similar structure of teeth. Furthermore, these images are often affected by variables such as oral cavity structure, tongue and saliva, which can result in uneven lighting or occlusions, manifesting in repetitive and weak textural features. These factors complicate the stitching of intraoral endoscopic images, leading to issues such as a scarcity of feature points and low accuracy in feature matching. Moreover, the spatial relationship between intraoral Endoscopic images generally not horizontal or vertical but instead conforms to the curvature of the dental arch. Therefore, specialized treatment is required during the image fusion stage to accommodate this curvilinear spatial arrangement. Such treatment enables accurate capture of the curved tooth structures. Presently, methods based on local features like SURF [2] and ORB [3] are widely applied in the image stitching domain [4,5]. However, when applied to intraoral endoscopic image stitching, these methods are prone to false detections and mismatches due to similarities in tooth shape and the deformable nature of oral soft tissues. Additionally, traditional panoramic image fusion techniques are ill-suited for the specific alignment of intraoral endoscopic images, leading to issues like noticeable double exposures and artifacts.
In summary, we introduce a method for panoramic stitching of semi-mandibular intraoral endoscopic images to address these unique image characteristics and alignment challenges. The main contributions are as follows:
1) By integrating the concept of Time-weighting [6], the attention mechanism has been enhanced, effectively increasing the quantity of feature-matching pairs. Coupled with the Sinkhorn [7] and RANSAC [8] algorithms, this results in heightened matching accuracy and reduced error rates.
2) We propose a wavelet transform and weighted fusion algorithm based on dental arch arrangement intraoral endoscopic images, resolving the applicability issues of intraoral endoscopic image arrangement and facilitating seamless fusion of these images.
3) An intraoral endoscopic image stitching dataset, termed as Intraoral Camera Panorama Album (ICPA), has been constructed. This dataset features image pairs with smaller overlapping regions, averaging around 35%.
Image stitching is the process of merging multiple partially overlapping images into a larger composite image, involving steps such as feature detection, feature matching and image fusion. In the domain of endoscopic medical image stitching [9], early research primarily utilized frequency-domain correlation algorithms and maximum mutual information methods. For example, Y.Hernandez-Mier [10] proposed an automated stitching algorithm specifically designed for 2D cystoscopic sequence images, demonstrating robustness against blurring, variable lighting and non-uniform radial distortions. This algorithm also exploited cancer autofluorescence within the images to detect cancerous lesions. Bergen et al. [11] employed graph-based techniques for stitching cystoscopic video frames, identifying coherent subgraphs from framework graphs to stitch local patches into larger composites. In intestinal endoscopic image stitching, Igarashi et al. [12,13] and Ishii et al. [14] utilized the "shape-from-shading" technique to generate open panoramic images of tubular organs, such as male urethrae, pig colons and human colons. They assumed the organs to be cylindrical and that the light axis was perfectly aligned with the cylindrical axis, generating panoramas from circles extracted around the image center during constant endoscope retraction. In 2002, Can et al. [15] presented mosaics generated from images of the human retina acquired with a fundus microscope. They explicitly exploited vascular structures to register pairs of images and used a quadric surface model to represent the retina. Their work is based on earlier experiments by Becker et al. carried out in 1998 [16]. In 2013, Yi et al. [17] presented real-time visualization technology for capsule endoscopic videos based on gastrointestinal tract unfolding panoramas. However, their approach was solely reliant on homographic descriptions of inter-frame transformations, leading to issues of ghosting and artifacts in the stitched result. Schuster et al. [18] have successfully applied general-purpose stitching software to laryngoscopic image sequences and presented panorama images of the larynx for documentation purposes.
Research and literature on stitching images in intraoral endoscopy are relatively sparse. In 2018, Ruiqing He proposed a teeth occlusal surface panoramic image stitching technique based on local optimization algorithms [19]. This method utilized adaptive SIFT for the stitching process but required image acquisition from devices equipped with a shooting track, making it computationally intensive and time-consuming. In the same year, he also presented a modification of the previous method, introducing a teeth buccal side panoramic image stitching technique based on local optimization algorithms [20]. This updated method employed bundle adjustment to calculate adjacent transformation matrices, thus enhancing the quality of the stitching. However, the time consumption issue persisted due to the continued use of SIFT. Additionally, the requirement for specialized image acquisition equipment with shooting tracks limits the method's universality.
The realm of image stitching has garnered substantial attention in recent research endeavors. A View-Free Image Stitching Network (VFISNet) was proposed by Lang Nie and co-authors [21], which employs deep learning to estimate homography matrices based on global homography, thus enabling effective image stitching. This method successfully mitigates the poor generalizability of previous learning algorithms in scenarios involving flexible views. However, its effectiveness diminishes in the presence of sparse feature points and abundant repetitive textures within images. Subsequently, Lang Nie and associates [22] advanced an Unsupervised Deep Image Stitching (UDIS) technique, specifically designed to enhance the accuracy of homography-based registrations in images featuring large disparities by reconstructing the stitching features. However, its utility is restricted to specific natural scenes endowed with sufficient geometric complexities. Contributing further, Daniel DeTone and collaborators [23] devised the SuperPoint network for feature detection and description in images, which detects a broader spectrum of interest points relative to conventional methods. Moreover, Sarlin and others [24] introduced the SuperGlue methodology, which incorporates graph neural networks and attention mechanisms to address the optimization of feature point assignments. Xiangyang Xu and colleagues [25] also formulated an image stitching method that integrates both global and local features, thus overcoming challenges of large disparities and high-resolution needs.
In summary, methods for stitching intraoral endoscopic images require the ability to identify as many feature points and matching pairs as possible while maintaining accuracy, especially in cases of repetitive and low textures. SuperPoint and SuperGlue demonstrate high performance in both feature detection count and accuracy when applied to intraoral endoscopic images. Therefore, we employ SuperPoint for the task of feature detection and borrows and refines the attention mechanism concept from SuperGlue for feature matching. Subsequently, we utilize a wavelet transform weighted fusion approach based on dental arch alignment to achieve panoramic image stitching of intraoral endoscopy.
As illustrated in Figure 1, the overall stitching workflow of the proposed method is delineated, with the green dashed section representing the primary innovations of this paper. Initially, preprocessing steps are applied to the intraoral endoscopic images slated for stitching. These include lighting compensation, resizing and grayscale conversion to counteract issues related to point light source imaging and significant lighting variations. Considering the recurrent and low-texture characteristics often found in intraoral endoscopic images, we employ the SuperPoint deep learning methodology for feature extraction. In addition, we design a feature-matching network that incorporates Time-weighting concepts and iteratively improves upon self-attention mechanisms for more effective feature aggregation. Subsequently, a combination of Sinkhorn and RANSAC algorithms is utilized to ascertain mutually matching feature points between images intended for stitching, thus deriving the homography matrices. Finally, due to the typical arc-shaped arrangement in intraoral endoscopic images, we propose a wavelet-transform-based weighted fusion algorithm aligned with dental arch configurations. This algorithm initially preprocesses image pairs for alignment and utilizes wavelet transformation for image fusion. Moreover, a fade-in, fade-out weighted fusion strategy is deployed for seamless stitching.
First, it is imperative to standardize the dimensions of the input images and perform lighting compensation to ensure that significant discrepancies in lighting intensity across image pairs do not adversely impact the visual perception of the stitched image. Subsequently, we employ a pre-trained SuperPoint network for feature detection. The SuperPoint network incorporates a strategy known as Homographic Adaptation to enhance the detection rate of feature points and their adaptability across different scenarios. Consequently, when confronted with large areas of repetitive textures and low-texture environments, SuperPoint is capable of detecting a greater number of features with higher accuracy compared to traditional feature detection methods.
This phase consists of two main components: the attention-based Graph Neural Network (GNN) section and the matching section. In the GNN section, feature aggregation is iteratively performed through Time-Weighting improved self-attention and cross-attention mechanisms, culminating in the generation of matching descriptors akin to feature descriptors. The matching section takes the output from the GNN as input and establishes an allocation matrix. It then employs the Sinkhorn algorithm in conjunction with the RANSAC method to identify correspondingly matched feature point pairs.
(1) MLP encoder
The attention GNN part is shown in Figure 2, For the i -th feature point of the image A to be spliced, it is represented by pAi, The feature descriptor is represented as dAi, The same method is used for image B. Initially, the feature points of both images are enhanced for unique matching characteristics via a Multilayer Perceptron (MLP) encoder. Subsequently, the concept of Time-Weighting is employed to improve the self-attention mechanism. Feature points and descriptors are then cyclically iterated through self-attention and cross-attention processes to aggregate image features, ultimately yielding matching descriptors analogous to traditional feature descriptors.
Both the location and the descriptor of each feature point contribute to heightened specificity in feature matching. Therefore, the initial representation (0)xi of each feature point combines the position and the descriptor as illustrated in Eq (1).
xi=di+MLPenc(pi) | (1) |
Among them, pi represents the i -th feature, the descriptor is represented by di, MLPenc represents a Multilayer Perceptron (MLP) employed for dimensionality elevation of low-level features, effectively coupling visual appearance with feature point location. The architecture of this encoder facilitates subsequent attention mechanisms to fully consider both the appearance and positional similarity of the features.
(2) Time-Weighting improves attention mechanism
For a given individual image, each node within its graph corresponds to each feature point in the image. The graph consists of two types of undirected edges: one type "s "Intra-image edg"s, " also known as self-edges, which connect feature points within the same image. The other type "s "Inter-image edg"s, " or cross-edges, which link feature points from the graph to all feature points in another image, thereby constituting that particular edge. Among them, self-edge uses self-attention, and cross edge uses cross-attention. Aggregating self-attention and cross-attention to obtain mε→i, as shown in Eq (2).
mε→i=∑αijvj | (2) |
The attention weight αij is the softmax of the similarity between the query and retrieved object key values, as shown in Eq (3):
αij=Softmax(qTikj) | (3) |
In Eq (2), let the feature point i to be queried be located on the query image Q, and all source feature points j be located on the source image S. For qi and key ki, the value vj can be written in the form of Eq (4):
qi=W(ℓ)1xQi+b1 and [kjvj]=[W2W3](ℓ)xSi+[b2b3] | (4) |
Each layer ℓ has its corresponding set of projection parameters W, which are shared by all feature points. qi represents a feature point i on the query image. ki, kj, vi and vj are representations of a transformed feature point j.αij signifies the similarity between the two features; a higher value indicates greater similarity. Subsequently, this similarity measure is utilized to weight-sum vj, resulting in mε→i, which is termed as feature aggregation.
According to the idea of time-weighting, each point is weighted after each softmax. As shown in Eq (5):
αij=αij∗ωij | (5) |
ωij represents the Time-Weighting factor. The weight is relatively low in non-overlapping areas along the image's edges. Conversely, the weight is higher in the image's central region and the overlapping areas. Time-Weighting is employed as a component of the relative position embedding in text recognition. Incorporating Time-Weighting into the self-attention mechanism is motivated by two considerations.
First, during the process of stitching intraoral endoscopic images, the contributions for different regions, such as teeth and tongue, ought to vary.
Second, for peripheral information with a comparatively low data density, the overall self-attention weight should be reduced.
In self-attention, edges within a single image are aggregated to better focus on all distinctive points, unrestricted by their neighboring positional features. In contrast, cross-attention serves to match features between two images that share similar appearances.
After L iterations of self/cross-attention, the output of the attention-based Graph Neural Network (GNN) for image A can be represented as shown in Equation (6).
fAi=WxAi+b,∀i∈A | (6) |
fAi can be interpreted as the matching descriptor for the ith feature point of Image A, analogous to a feature descriptor. This is specifically designed for feature matching purposes. A similar formulation applies to Image B.
The visualization of the aforementioned process is illustrated in Figure 3. In self-attention, edges within a single image are aggregated to heighten focus on all unique points without being limited by neighboring positional features. Conversely, cross-attention is employed to match features between two visually similar images. Analogous to how humans perform feature matching—by tentatively filtering key matching points through iterative scrutiny between two images—the model aims to simulate this human-like approach. The core idea is to leverage Graph Neural Networks (GNNs) based on attention mechanisms to replicate this process, thereby actively seeking context to enhance feature-point specificity and exclude anomalous matches.
(1) Assignment matrix
In the matching section, the objective is to construct an assignment matrix Pto determine the pairs of matched features, as outlined in Figure 4. Initially, the inner products of fAi and fBj obtained from the GNN steps are calculated to yield scores Sij, which are then organized into a score matrix S. An "unmatched" channel is incorporated to form ¯S. Subsequently, the Sinkhorn algorithm, in conjunction with the RANSAC algorithm, is employed to identify and refine feature matches, excluding erroneous matches during each iteration. The ultimate goal is to derive an optimal assignment matrix P, achieved by calculating a score matrix S∈Rm×n that represents potential matches. The optimization of P is accomplished by maximizing the aggregate score∑ijSi,jPi,j, According to the matrix information, the set mAB of feature point pairs matching the image A and B is obtained.
As demonstrated in Figure 5, the yellow rectangles and circles represent the reference image A and its M corresponding feature points within a pair of images to be stitched, while the blue rectangles and circles represent the target image B and its N feature points. Each row of the assignment matrix P represents the potential N matches for a particular feature point originating from the reference image A to the target image B.
In the reference image A, there are three feature points, whereas the target image B has four. Consequently, the dimensions of the assignment matrix P would be 3×4. From the first row of matrix P, as shown in Figure 5, the maximum value is 0.6, indicating a match between the first feature point in reference image A and the second feature point in target image B. Likewise, in the first column of P, the highest value is 0.5, signifying a match between the first feature in B and the second feature in A. It is worth mentioning that this assignment matrix P is not fully distributed. In an ideal scenario, the sum of each row or column in P should be equal to 1. This " ideal scenario" assumes that all features in both images A and B have corresponding matches; however, real-world conditions such as occlusions, changes in viewpoint, or noise may prevent such perfect matching.
As illustrated in Figure 6, for the third column of matrix P, which corresponds to the third feature in the target image B, no matching feature is identified. Hence, the sum of the third column is less than 1. The subsequent aim is to compute and construct an optimal assignment matrix P.
(2) Sinkhorn combines RANSAC algorithm to improve accuracy
In the matching section, the inner product of fAi and fBj, obtained through GNN aggregation, is first calculated to yield the score Sij, as shown in Eq (7).
Sij=<fAi,fBj>,∀(i,j)∈A×B | (7) |
Moreover, a specialized "unmatched" channel is introduced in the final column or row of the score matrix, denoted as S, to create an augmented matrix ¯S. This addition aims to address instances where no feature points are identifiable, serving as a mechanism to eliminate erroneous matches.
Feature points from the reference image A are either mapped to corresponding feature points in the target image B or relegated to a designated "unmatched" channel. Under this framework, each "unmatched" is associated with N or M potential matches. Accordingly, the constraints imposed on the assignment matrix are articulated in Eq (8) and (9).
¯P1N+1=a,¯P1M+1=b | (8) |
a=[1TM,N]T,b=[1TN,M]T | (9) |
The variable a denotes the anticipated count of feature matches from the reference image A, including its dedicated "unmatched" channel. Conventionally, each feature point within image A aligns with a solitary corresponding point in target image B. However, the feature points that fall into the "unmatched" channel from image A may potentially align with any feature point in image B, thereby introducing N potential matches. Consequently, we havea=[1TM,N]T. The same goes for b.
As delineated in Algorithm Table 1, we leverage an integrative approach utilizing both Sinkhorn and RANSAC algorithms to maximize our scoring metric. The Sinkhorn algorithm is traditionally deployed for optimal transport issues. In our setup, Eq (8) and (9) act as specialized cost functions, or more precisely, as their negations. While ¯S in classical optimal transport scenarios serves as a cost matrix, in this context, it represents the cosine similarity between matching descriptors. Consequently, the objective diverges from minimizing cost to maximizing descriptor similarity, as indicated by the maximization operation in Eq (8). Various parameters are set: A regularization term λ at 1, confidence ξ at 0.995, error threshold ι at 10, inlier proportion ω and a minimum sample count m of 4 for computing model H. By purging outliers, the method achieves a marked improvement in registration precision and minimizes errors.
Algorithm 1 Integration of the Sinkhorn with the RANSAC |
Inputs: Cosine similarity matrix of matching descriptors ¯S. The length and width of the matrix n and m, anticipated count of feature matches a and b, regularization term λ, confidence ξ, error threshold ι |
Output: Feature point matching pairs removing external points P
1: Initialize assignment matrix:¯P=expλ¯S; 2: while ¯P does not converge do //Determine whether the Sinkhorn algorithm converges 3: i→m; 4: ¯Pij÷∑mj=0¯Pij×ai; 5: j→n 6: ¯Pij÷∑ni=0¯Pij×bj; 7: end while 8: while the number of iterations is less than K do //Ransac algorithm removes outliers 9: ¯Mij=random(¯Pi0,¯P0j)m; 10: H=FindHomography(¯Mij); 11: Error=¯Pi0×H−¯P0j; 12: K=log1−ξlog(1−ωm); 13: if P=Error<ι then // When the error is less than the set threshold, it ends 14: break; 15: end if 16: end while 17: return P |
Standard approaches to panoramic image stitching usually necessitate that the images align in a horizontal or vertical sequence. However, intraoral endoscopic images inherently correspond to the curvature of the dental arch, necessitating specialized methods during the image fusion stage. As depicted in Figure 7, we have formulated a wavelet transform and weighted fusion algorithm based on dental arch arrangement intraoral endoscopic images specifically crafted for images arrayed along the dental arch, which alleviates issues arising from suboptimal or incorrect stitching when the images are not horizontally or vertically aligned.
Let Is stand for the collection of images awaiting stitching, and Hij signify the homography matrix that corresponds to the source image Ii and its target image Ij. In the preprocessing phase of the images, a unique identifier, denoted as T and constrained within the interval (0, 1), is assigned to each image. Specifically, Ti serves as the identifier for image Ii.
As delineated in Algorithm Table 2, the initial procedure is to obtain the source image's bias matrix. The technique involves transforming the corner points of the source image through dot multiplication with the homography matrix. Following this transformation, the smallest values of the x and y coordinates of these transformed corners serve as the bias offset. The resultant bias matrix, termed as biasmatrix, is elaborated upon in Eq (10) and (11).
Algorithm 2 Wavelet transform and weighted fusion algorithm based on dental arch arrangement intraoral endoscopic images |
Inputs: Images set I1,I2....In, Source image Ii and target image Ij, mutually matched feature points Pi,Pj and position markers Ti and Tj, weighted matrix width θ |
Output: Stitching result image Iresult
1: bias=0; 2: for i=1→n−1 do 3: j=i+1; 4: if Ti==Tj==1 then // Determine whether it is the left half of the jaw 5: Hij=FindHomography(Pi,Pj); 6: bias=|min(xi,yi)|; 7: biasmatrix=[[1,0,bias[0]],[0,1,bias[1]],[0,0,1]]; // Build bias matrix 8: Ileft=biasmatrix∗Hij∗Ii; 9: hj,wj=Ij.shape(); 10: Ileft[bias[0]:bias[0]+hj][bias[1]:bias[1]+wj]=Ij; 11: waveletfusion(); // Perform wavelet fusion 12: Hweight=getweightmatrix(θ,bias); // Create weighted matrix 13: Ileft=Ileft∗Hweight+Ij∗(1−Hweight); // Weighted fusion processing seams 14: end if 15: if Ti==Tj==0 then 16: Get Iright in the same way 17: end if |
18: end for
19: Similarly, merge Ileft and Iright into Iresult 20: return Iresult |
bias=min((xi,yi)H) | (10) |
biasmatrix=[10bias[0]01bias[1]001] | (11) |
Here, (xi,yi) refers to the coordinates of the four corners of the source imageIi, where i is an integer between 0 and 3. The final homography matrix for the source image is obtained by matrix multiplication between the bias matrix and the original homography matrix. This leads to the transformed coordinates (xout,yout)=HijbiasmatrixIi for the resultant source image Iout.
After the transformation, we use image labels Ti and Tj to ascertain whether the set of images to be stitched pertains to the left or right half jaw teeth. Concretely, when Ti=Tj=1, the set of images correspond to the left half jaw teeth and their area of overlap is in the upper-left corner of the target image. Based on the coordinates post-transformation, image pairs are meticulously aligned. The aligned images are then fused using a wavelet transformation fusion technique. To ensure the seamlessness of the stitched images, a weighted fusion strategy is executed, featuring a fade-in, fade-out weighted matrix.
We conducted the experiments on a hardware setup featuring a 64-bit Windows 10 operating system and an AMD Ryzen 9 5900HX with Radeon Graphics, clocked at 3.30 GHz. The programming is built on PyCharm, which is seamlessly integrated with Anaconda and running in a Python 3.6 environment. The implementation leverages libraries like PyTorch and OpenCV-Python. The set parameters are as follows: A SuperPoint detection threshold of 0.007, attention iteration L fixed at 16, Time-Weighting with default settings, a RANSAC regularization term λ set to 1, a confidence value ξ of 0.995, an error cutoff ι at 10, a minimum sample size m of 4 and a weighted matrix width θ marked at 50. The hyperparameter settings employed in the proposed method of this paper are as follows: The learning rate is set at 0.0001, the Batch Size at 64 and the number of Epochs at 150. The model features 102 layers in the hidden layer, with ReLu as the activation function. The Sinkhorn iteration count is set to 150. For the convolutional layer, the kernel size is configured to (1, ) and the stride to (1, ). Batch normalization momentum is set at 0.1, with epsilon at 0.00001, and the Stochastic Gradient Descent (SGD) is used as the optimizer. Regularization employs Dropout with a dropout rate of 38.1%, and the weights are initialized randomly. These parameter settings are based on recommended values from relevant literature and best practices in existing research. Parameter settings for comparison methods follow either default configurations or recommendations cited in relevant studies.
In this paper, we establish a specialized intraoral camera dataset, named ICPA, for capturing localized image samples of lower jaw teeth using an A3M model intraoral endoscope. As illustrated in Figure 8, the endoscope lens employed in this dataset features a diameter of 1.2 cm and a viewing angle of 60 degrees. To maximize the richness of the captured image content, a focal length of approximately 1.5 cm is maintained. Utilizing a stationary camera setup, the ICPA dataset captures images of both the upper and lower jaws, accumulating a total of roughly 400 adjacent image pairs across 16 mouths, all collected from real oral environment. For a set of 10 half-jaw images, we consider that there are 9 pairs of adjacent images with individual image dimensions being 480×640 pixels. The feature points of the images in the data set range from approximately 400 to 600, and the pairs of matching feature points range from approximately 60 to 180 pairs. The sample size of the data set is shown in Table 1. The images encompass common oral features such as crowded dentition, mandibular deviation, sparse tooth arrangement and dental malformations like prognathism, as well as prevalent oral diseases including dental caries, plaque, mouth ulcers and gingival bleeding. During the model training phase, we augmented the 400-pair dataset using techniques like rotation, brightness adjustment and random noise addition, expanding the data to approximately 1600 pairs. Concerning image overlap rate, a lower overlap rate can challenge the algorithm's ability to precisely match feature points, impacting the stitching's accuracy and overall quality. Conversely, a higher overlap rate, while providing more matching points and enhancing stitching precision, also increases the data collection time and cost. In practical dental diagnostics, due to the necessity of processing a large volume of cases rapidly, diagnostic images typically have a lower overlap rate. To emulate this reality, our study maintained an overlap rate of about 35% for image pairs, with an average of 10 images per half-jaw and a minimum overlap area of 25%, averaging around 35%. This setup ensures that the dataset accurately reflects the image processing requirements of real clinical diagnosis and enhances the feasibility and applicability of our research findings in future practical diagnostic applications.
Average number of feature points in the data set | The average number of feature point pairs that match each other | Average number of unmatched feature point pairs | Proportion of feature point pairs that match each other |
486 | 160 | 326 | 32% |
Moreover, excessive exposure in images compromises the clarity of edges and finer details. As depicted in Figure 9, the histogram of a well-exposed dental image maintains a balanced distribution, whereas in an overexposed version, the predominance of high-luminance pixels skews the grayscale histogram to the right. To rectify this imbalance, we implement the ACE (Automatic Color Equalization) algorithm to harmonize the color profile of the dataset. This method not only adjusts the brightness, hue and contrast of images but also takes into account local and nonlinear characteristics, aligning with the Gray World Theory and White Patch Assumption frameworks. A comparative analysis of the dataset pre- and post-ACE algorithm application is presented in Figure 10.
As delineated in Eq (12) to (14), the principal metrics for evaluating feature matching encompass the Matching Score (Ms), Precision (P) and Recall (R). In this context, n and m represent the number of feature points in the two images to be matched. The min(n,m) denotes the smaller value between n and m. TP (True Positives) refers to the feature point pairs that are correctly matched. Conversely, FP (False Positives) signifies the feature point pairs that are incorrectly matched. Lastly, FN (False Negatives) pertains to the feature point pairs that should have been matched but were not. TN (True Negatives) represents pairs of feature points that are considered not to match each other.
Ms=TP+FPmin(n,m) | (12) |
P=TPTP+FP | (13) |
R=TPTP+FN | (14) |
Correctly matched point pairs are identified based on annotated feature-matching pairs in the dataset, used to ascertain the ground-truth homography matrixH. Utilizing H, feature points are projected into the coordinate space of a corresponding image, where distances to another set of feature points are computed. A pair of feature points with the minimum distance is deemed to be a correct match. Given the potential for errors in manual annotation, a distance threshold γ is established, set at 5 in this study, constraining the distance between correctly matched feature point pairs to be within a 5-pixel range.
We evaluate five comparative algorithms: ORB, GMS [26], PointCN [27], OANet [28] and SuperGlue. Experimental trials were undertaken on a test set, and the average results were computed. Data from Table 1 illustrates that the methodology proposed herein outstripped competing approaches, with improvements of 4.5%, 6.3% and 10.6% in Ms, P and R metrics, respectively. The novel approach amalgamates self-attention with cross-attention to elevate feature point matching specificity and escalate the chances of match success. Additionally, outlier elimination is achieved in each Sinkhorn iteration through the application of the RANSAC algorithm, ensuring the accuracy of feature point matching and consequently yielding a higher recall rate vis-à-vis other methods.
When considering matching methodologies that leverage homography estimation, the yardstick for assessment is the Frobenius norm. The Frobenius norm of an arbitrary matrix A can be computed as illustrated in Eq (15).
||A||F=√∑mi=1∑nj=1|aij|2 | (15) |
Herein, ||A||F stands for the Frobenius norm of matrixA. The variables m and n correspond to the number of rows and columns in the matrix, respectively, while aij denotes the element located at the i -th row and j -th column. In the evaluation of discrepancies between two homography matrices, the Frobenius norm serves as a widely-accepted metric. The experimental protocol involves computing the Label homography matrix using labeled feature point pairs and then calculating the absolute difference between its Frobenius norm and that of the estimated homography matrix. A lower value suggests a higher degree of similarity between the estimated and Label homography matrices.
The methods employed for comparative analysis are HomographyNet[29], VFISNet and UDIS. As evidenced by Table 2, our technique demonstrates a 31% reduction in the Frobenius norm difference, thereby drawing us closer to the Label. It is noteworthy that higher accuracy and recall rates, under the condition of a consistent Label, lead to a homography matrix that is increasingly congruent with the Label's homography matrix. Hence, the homography matrix derived from our proposed method exhibits a closer alignment with the Label.
method type | Methods | Ms | P(%) | R(%) | Difference in absolute value of ||A||F |
Matching based on feature points | ORB | 11.9 | 10.8 | 2.6 | / |
GMS | 10.7 | 33.0 | 7.8 | ||
PointCN | 19.5 | 56.2 | 23.6 | ||
OANet | 23.8 | 65.0 | 40.9 | ||
SuperGlue | 31.4 | 78.3 | 67.8 | ||
Ours | 35.9 | 84.6 | 78.4 | ||
Based on homography matrix estimation | HomographyNet | / | 113.68 | ||
VFISNet | 84.47 | ||||
UDIS | 72.59 | ||||
Ours | 49.60 |
Considering the imbalance present in the dataset, this method incorporates the G-mean and Precision-Recall Area Under Curve (PR-AUC) metrics to provide a more nuanced evaluation. G-mean as delineated in Eq (16). The PR-AUC represents the area under the Precision-Recall (PR) curve, which illustrates the relationship between Precision and Recall for the model at various thresholds. In comparison to other metrics, the PR-AUC serves as a more valuable performance indicator, particularly when dealing with imbalanced datasets. Specific indicators are shown in Table 3.
G−mean=√TPTP+FN×TNFP+TN | (16) |
Methods | G-mean | PR-AUC |
PointCN | 0.445 | 0.484 |
OANet | 0.581 | 0.560 |
SuperGlue | 0.749 | 0.766 |
Ours | 0.832 | 0.813 |
To assess the comparative advantages of our proposed methodology over existing techniques, we conducted experiments using distinctively featured images sourced from the ICPA database, as delineated in Figure 11. The experimental setup comprises three specific groups: The left molar region (inclusive of the third, second and first molars), the right molar region and the anterior incisor region (which includes lateral incisors, central incisors and canines).
Figure 12 provides a comparative analysis of matching outcomes across diverse regions between our proposed technique and extant algorithms. In the inaugural column, algorithms like GMS, PointCN and OANet are observed to perform matches based on the reflections generated by saliva. Our method efficaciously eradicates a substantial number of such feature-point pairs prone to reflective matching. Given that soft tissues such as saliva and the tongue are susceptible to morphological changes during image capture, matches based on reflections usually exhibit diminished confidence levels. In the subsequent two columns, apparent mismatches are also discernible in other techniques. Hence, our approach is proficient at identifying a greater number of credible feature-point matches while effectively filtering out erroneous ones, thereby minimizing error rates.
To authenticate the algorithm's utility, we conducted experiments on three data sets from Figure 11, incorporating a total of 10 variations that encompass translational shifts, rotational adjustments, perspective transformations and variations in overlap rates. Figure 13 illustrates the test dataset, and Figure 14 delineates the matching precision of our proposed method in comparison with existing algorithms like GMS, PointCN, OANet and SuperGlue across mandibular teeth images taken at 10 divergent angles. Remarkably, our method outperforms the other algorithms, achieving an average accuracy rate exceeding 80%.
In Figure 14, an analysis of data from Groups 2 to 5 shows that all examined algorithms maintain a consistent level of matching accuracy under translational variations. However, the data from Groups 6 and 7 reveal a marked reduction in performance for GMS and PointCN when subjected to rotational transformations. Furthermore, in Groups 8 through 10, all three algorithms—GMS, PointCN and OANet—suffer from decreased accuracy. SuperGlue fares poorly in feature-sparse regions like the left (or right) molars but exhibits stable performance in feature-rich zones like the anterior incisors. Remarkably, the method proposed in this study demonstrates stable matching accuracy across different feature compositions.
In the phase dedicated to image fusion, we address a core limitation: Conventional panoramic image stitching demands either a vertical or horizontal positional relationship between images, a constraint not well-suited for intraoral endoscopic image pairs. The principal metrics evaluated are the mean gradient and standard deviation. Higher values in these metrics translate to better preservation of image details and smoother, more natural transitions in the fused image. As evidenced in Table 4, the methodology we propose achieves optimal levels in both these key metrics. This approach employs wavelet transformations for the fusion process and uses a weighted, fade-in, fade-out technique to seamlessly blend image seams. Consequently, relative to traditional approaches, our method yields superior fusion outcomes, preserving a greater extent of image details and facilitating smoother, more natural transitions.
Fusion methods | Average gradient | Standard deviation |
Based on maximum value | 6.65 | 58.71 |
Based on minimum value | 4.73 | 49.15 |
Average weighted | 7.45 | 50.29 |
Laplacian pyramid | 9.332 | 51.36 |
Wavelet transform fusion | 9.66 | 53.75 |
Ours | 11.23 | 58.78 |
In the ablation experiment, we chiefly examine the impact on the quality of image stitching involving either two or multiple images. The reliability of feature point pairs is gauged by their confidence scores, with higher scores indicating a greater likelihood of correct matching. These confidence levels are color-coded, ranging from blue for high confidence to red for low, with intermediary values represented by green and yellow. To enhance visual clarity, a lower mean confidence score will result in a reduced peak value, causing the overall image to take on more yellow or red tones. As indicated in Figure 15, the removal of the Time-Weighting-enhanced self-attention mechanism precipitates a decline in the ability to effectively aggregate feature points, and a corresponding reduction in matched feature point pairs. With the implementation of Time-Weighting, there is an approximate 16% boost in the quantity of feature point pairs. Conversely, the absence of the RANSAC algorithm leads to generally lower confidence scores for feature point pairs, manifesting in conspicuously red and yellow connecting lines.
As illustrated in Figure 16, we employ the same methodology for representing confidence levels in the context of multi-image stitching. Our results reveal that the proposed approach significantly improves the stitching outcome, augmenting the quantity of feature point pairs by an estimated 20%. While integrating the RANSAC method leads to a marginal reduction in the number of feature point pairs, it simultaneously enhances the overall confidence level of the matches.
As depicted in Figure 17, we present examples of semi-jaw data captured from an intraoral endoscope, consisting of three distinct dental photo sets: 10 images in set A, 8 in set A and 12 in set C. The image-capturing process is prone to variations in perspective due to camera shake when held by hand, leading to inconsistent overlap areas and positions between images. Current stitching algorithms generally focus on dual-image stitching, progressing step-by-step to create a panoramic image through iterative dual-image combinations. However, this approach is fraught with challenges, including the accumulation of deformation errors during the multi-image stitching, resulting in incomplete oral panoramic imagery in some instances.
As delineated in Figure 18, we employed a comparative analysis featuring ORB, GMS, PointCN, OANet, SuperGlue and our proposed algorithm. Blue rectangles highlight areas of misalignment and ghosting artifacts; green rectangles point out conspicuous distortions; while red rectangles signify incorrect stitching outcomes. ORB, GMS and PointCN tend to yield flawed results, including misalignments that prevent the creation of a complete stitched image. OANet and SuperGlue, on the other hand, do produce panoramic images but suffer from varying degrees of distortion and errors. According to the metrics compiled in Table 5, our proposed approach achieves optimal results in terms of both average feature matching and accuracy. In contrast, both ORB and GMS fail to correctly stitch over half of the total image set, and PointCN often yields incomplete panoramic images due to cumulative errors. OANet and SuperGlue manage to generate panoramas, albeit with diminished accuracy.
methods | incorrect stitching | ghosting artifacts | conspicuous distortions | Generate panorama | successfully stitched images (%) | average feature matching pair | average accuracy (%) |
ORB | √ | √ | √ | × | 20 | 16.3 | 10.1 |
GMS | √ | √ | √ | × | 26.6 | 26.4 | 23.1 |
PointCN | √ | √ | √ | × | 43.3 | 43.7 | 59.7 |
OANet | √ | √ | √ | 100 | 62.2 | 63.1 | |
SuperGlue | √ | √ | 100 | 89.5 | 79.3 | ||
Ours | √ | 100 | 108.4 | 86.2 |
As depicted in Figure 19, the proposed method begins the stitching process with images labeled starting with "a", representing the left side of the dental arch. The approach employs a two-sided sequential stitching strategy: First from one side and then the other, culminating in a fusion of these left and right stitched images. The end result accomplishes a comprehensive semi-jaw panoramic view of the teeth, meticulously preserving the content details from the original images. Moreover, each stitched image exhibits a distortion level that is within an acceptable range, devoid of discernible ghosting or artifacts.
In this paper, we study the splicing problem of intraoral endoscopic images and explore the impact of Time-Weighting combined with the attention mechanism on the number of feature point matches. In addition, the feature point pair matching mechanism of the Sinkhorn and RANSAC combination is clarified. To accomplish seamless Stitching of intraoral endoscopic visuals, a wavelet transform and weighted fusion algorithm based on dental arch alignment intraoral endoscopic images was designed. Experimental results show that the integration of Time-Weighting and attention mechanisms substantially augments the volume of feature point matches, whereas the accuracy of feature point pair matching can be improved by the combination of Sinkhorn and RANSAC. The algorithm this paper introduce excels in both quantitative metrics and visual aesthetics.
The proposed method currently has the following limitations: 1) Due to the use of point light sources in intraoral endoscopes, the images captured often exhibit uneven brightness, with higher luminance at the center and lower at the edges. This results in an unnatural brightness transition at the seams post-stitching. 2) The process of stitching involves distortion and stretching of the source images, leading to irregular boundaries in the final composite, which are not in line with typical visual perceptions and display modes of imaging devices.
Future research directions include: 1) Developing a global brightness optimization method [30] specifically for panoramic image stitching to ensure a natural luminance transition and mitigate abrupt brightness changes. 2) Devising a method to rectify the stitched panoramic intraoral endoscopic images into a rectangular format, meeting the visual expectations of humans and the display formats of imaging devices.
Furthermore, the dataset constructed by the proposed method is currently not extensive and fails to cover all possible oral features, such as periodontitis and dental cancer. Therefore, future work involves expanding the dataset, and collaborating with dental hospitals and other medical institutions to increase the diversity and quantity of samples. This will ensure a more comprehensive coverage of potential features in intraoral endoscopic images, enhancing the representativeness of the dataset and theesearchh.
The authors declare that they have not used Artificial Intelligence (AI) tools in the creation of this article.
This work was supported by the Shaanxi Natural Science Fundamental Research Program Project (No. 2022JM-508), and in part by the National Natural Science Foundation of China (Grant No. 62101432).
The authors declare there are no conflicts of interest.
[1] |
M. Tian, X. Zhou, J. Yang, B. Meng, J. Qian, J. Zhang, et al., Dental lesion segmentation using an improved ICNet network with attention, Micromachines, 13 (2022), 1920. https://doi.org/10.3390/mi13111920 doi: 10.3390/mi13111920
![]() |
[2] |
H. Bay, A. Ess, T. Tuytelaars, L. V. Gool, Speeded-up Robust Features (SURF), Comput. Vision Image Understand., 110 (2008), 346–359. https://doi.org/10.1016/j.cviu.2007.09.014 doi: 10.1016/j.cviu.2007.09.014
![]() |
[3] | E. Rublee, V. Rabaud, K. Konolige, G. Bradski, ORB: An efficient alternative to SIFT or SURF. 2011 International Conference on Computer Vision (ICCV), (2011), 2564–2571. https://doi.org/10.1109/ICCV.2011.6126544 |
[4] | L. Zhu, Y. Wang, B. Zhao, X. Zhang, A fast image stitching algorithm based on improved SURF, in 2014 Tenth International Conference on Computational Intelligence and Security (CIS'2014), (2014), 171–175. https://doi.org/10.1109/CIS.2014.67 |
[5] | Z. Y. Zhang, L. X. Wang, W. F. Zheng, L. R. Yin, R. R. Hu, B. Yang, Endoscope image mosaic based on pyramid ORB, Biomed. Signal Process. Control, 71 (2022). https://doi.org/10.1016/j.bspc.2021.103261 |
[6] | S. Peter, J. Uszkoreit, A. Vaswani, Self-attention with relative position representations, in North American Chapter of the Association for Computational Linguistics (NAACL2018), (2018). https://doi.org/10.48550/arXiv.1803.02155 |
[7] |
P. Gabriel, M. Cuturi, Computational optimal transport, Found. Trends Mach. Learn, 11 (2018), 355–607. https://doi.org/10.48550/arXiv.1803.00567 doi: 10.48550/arXiv.1803.00567
![]() |
[8] |
R. Raguram, O. Chum, M. Pollefeys, J. Matas, J. -M. Frahm, USAC: A universal framework for random sample consensus, IEEE Transact. Pattern Anal. Mach. Intell., 35 (2013), 2022–2038. https://doi.org/10.1109/TPAMI.2012.257 doi: 10.1109/TPAMI.2012.257
![]() |
[9] |
T. Bergen, T. Wittenberg, Stitching and surface reconstruction from endoscopic image sequences: A review of applications and methods, IEEE J. Biomed. Health Inform., 20 (2016), 304–321. https://doi.org/10.1109/JBHI.2014.2384134 doi: 10.1109/JBHI.2014.2384134
![]() |
[10] |
Y. H. Mier, W. Blondel, C. Daul, D. Wolf, G. B. Heckly, 2D panoramas from Cystoscopic image sequences and potential application to fluorescence imaging, IFAC Proceed. Volumes, 39 (2006), 291–296. https://doi.org/10.3182/20060920-3-FR-2912.00054 doi: 10.3182/20060920-3-FR-2912.00054
![]() |
[11] | T. Bergen, T. Wittenberg, C. Münzenmayer, C. Chen, G. D. Hager, A graph-based approach for local and global panorama imaging in cystoscopy, in Medical Imaging 2013: Image-Guided Procedures, Robotic Interventions, and Modeling (MI2013), 8671 (2013). https://doi.org/10.1117/12.2008174 |
[12] |
T. Ishii, Computer-based endoscopic image-processing technology for endourology and laparoscopic surgery, Int. J. Urol., 16 (2009), 533–543. https://doi.org/10.1111/j.1442-2042.2009.02258.x doi: 10.1111/j.1442-2042.2009.02258.x
![]() |
[13] |
T. Ishii, Three-dimensional image processing system for the ureter and urethra using endoscopic video, J. Endoutol., 22 (2008), 1569–1572. https://doi.org/10.1089/end.2008.0150 doi: 10.1089/end.2008.0150
![]() |
[14] |
T. Ishii, S. Zenbutsu, T. Nakaguchi, M. Sekine, Y. Naya, T. Igarashi, Novel points of view for endoscopy: Panoramized intraluminal opened image and 3D shape reconstruction, J. Med. Imag. Health Inform., 1 (2011), 13–20. https://doi.org/10.1166/jmihi.2011.1002 doi: 10.1166/jmihi.2011.1002
![]() |
[15] |
A. Can, C. V. Stewart, B. Roysam, H. L. Tanenbaum, A feature-based, robust, hierarchical algorithm for registering pairs of images of the curved human retina, IEEE Transact. Pattern Anal. Mach. Intell., 24 (2002), 347–364. https://doi.org/10.1109/34.990136 doi: 10.1109/34.990136
![]() |
[16] | D. E. Becker, A. Can, J. N. Turner, H. L. Tanenbaum, B. Roysam, Image processing algorithms for retinal montage synthesis, mapping, and real-time location determination, IEEE Transact. Biomed. Eng., 45 (1998), 105–118. https://doi.org/10.1109/10.650362 |
[17] | S. Yi, J. Xie, P. Mui, J. A. Leighton, Achieving real-time capsule endoscopy (CE) video visualization through panoramic imaging, in Real-Time Image and Video Processing 2013(JRTIP), 8656 (2013). https://doi.org/10.1117/12.2005243 |
[18] |
M. Schuster, T. Bergen, M. Reiter, C. Münzenmayer, S. Friedl, T. Wittenberg, Laryngoscopic Image Stitching for View Enhancement and Documentation—First Experiences, Biomedical Engineering/Biomedizinische Technik (BMT), 57 (2012), 704–707. https://doi.org/10.1515/bmt-2012-4471 doi: 10.1515/bmt-2012-4471
![]() |
[19] | H. R. Qing, G. L. Qing, X. Wen, L. Jun, Y. Hai, Y. Jun, et al., A teeth occlusal surface panoramic image stitching technique based on local optimization algorithms, China, patent, CN, CN201810428969.7, 2018, September 28. |
[20] | H. R. Qing, G. L. Qing, X. Wen, L. Jun, Y. Hai, Y. Jun, et al., A teeth buccal side panoramic image stitching technique based on local optimization algorithms, China, patent, CN, CN201810435287.9, 2018, October 2. |
[21] |
L. Nie, L. Y. Chun, K. Liao, L. Q. Mei, Z. Yao, A view-free image stitching network based on global homography, J. Visual Commun. Image Represent., 73 (2020), 102950. https://doi.org/10.1016/j.jvcir.2020.102950 doi: 10.1016/j.jvcir.2020.102950
![]() |
[22] |
L. Nie, C. Lin, K. Liao, S. Liu, Y. Zhao, Unsupervised deep image stitching: Reconstructing stitched features to images, IEEE Transact. Image Process., 30 (2021), 6184–6197. https://doi.org/10.1109/TIP.2021.3092828 doi: 10.1109/TIP.2021.3092828
![]() |
[23] | D. D. Tone, D. T. Malisiewicz, A. Rabinovich, SuperPoint: Self-supervised interest point detection and description, in 2018 IEEE/CVF Conference on Computer Vision and Pattern Recognition Workshops (CVPRW), (2017), 33712. https://doi.org/10.48550/arXiv.1712.07629 |
[24] | P. E. Sarlin, D. D. Tone, T. Malisiewicz, A. Rabinovich, SuperGlue: Learning feature matching with graph neural networks, in 2020 IEEE/CVF Conference on Computer Vision and Pattern Recognition (CVPR), (2019), 4937–4946. https://doi.org/10.48550/arXiv.1911.11763 |
[25] |
X. X. Yang, Y. S. Shan, W. Jun, D. Y. Ping, An image stitching method that integrates both global and local features, J. Beijing Institute Technol., 42 (2022), 9. https://doi.org/10.15918/j.tbit1001-0645.2021.093 doi: 10.15918/j.tbit1001-0645.2021.093
![]() |
[26] | J. Bian, W. Y. Lin, Y. Matsushita, S. K. Yeung, T. D. Nguyen, M. M. Cheng, GMS: Grid-based motion statistics for fast, ultra-robust feature correspondence, in 2017 IEEE Conference on Computer Vision and Pattern Recognition (CVPR), (2017), 2828–2837. https://doi.org/10.1109/CVPR.2017.302 |
[27] | K. M. Yi, E. Trulls, Y. Ono, V. Lepetit, M. Salzmann, P. Fua, Learning to find good correspondences, in 2018 IEEE/CVF Conference on Computer Vision and Pattern Recognition (CVPR), (2018), 2666–2674. https://doi.org/10.1109/CVPR.2018.00282 |
[28] | J. Zhang, D. Sun, Z. Luo, A. Yao, L. Zhou, T. Shen, et al., Learning two-view correspondences and geometry using order-aware network, in 2019 IEEE/CVF International Conference on Computer Vision (ICCV), (2019), 5844–5853. https://doi.org/10.1109/ICCV.2019.00594 |
[29] | D. D. Tone, T. Malisiewicz, A. Rabinovich, Deep image homography estimation, (2016), arXiv, abs/1606.03798. https://doi.org/10.48550/arXiv.1606.03798 |
[30] |
T. Ma, C. Fu, J. Yang, J. Zhang, C. Yang, RF-Net: Unsupervised low-light image enhancement based on retinex and exposure fusion, Comput. Mater. Cont., 77 (2023), 1103–1122. https://doi.org/10.32604/cmc.2023.042416 doi: 10.32604/cmc.2023.042416
![]() |
1. | James C. L. Chow, Computational physics and imaging in medicine, 2025, 22, 1551-0018, 106, 10.3934/mbe.2025005 |
Average number of feature points in the data set | The average number of feature point pairs that match each other | Average number of unmatched feature point pairs | Proportion of feature point pairs that match each other |
486 | 160 | 326 | 32% |
method type | Methods | Ms | P(%) | R(%) | Difference in absolute value of ||A||F |
Matching based on feature points | ORB | 11.9 | 10.8 | 2.6 | / |
GMS | 10.7 | 33.0 | 7.8 | ||
PointCN | 19.5 | 56.2 | 23.6 | ||
OANet | 23.8 | 65.0 | 40.9 | ||
SuperGlue | 31.4 | 78.3 | 67.8 | ||
Ours | 35.9 | 84.6 | 78.4 | ||
Based on homography matrix estimation | HomographyNet | / | 113.68 | ||
VFISNet | 84.47 | ||||
UDIS | 72.59 | ||||
Ours | 49.60 |
Methods | G-mean | PR-AUC |
PointCN | 0.445 | 0.484 |
OANet | 0.581 | 0.560 |
SuperGlue | 0.749 | 0.766 |
Ours | 0.832 | 0.813 |
Fusion methods | Average gradient | Standard deviation |
Based on maximum value | 6.65 | 58.71 |
Based on minimum value | 4.73 | 49.15 |
Average weighted | 7.45 | 50.29 |
Laplacian pyramid | 9.332 | 51.36 |
Wavelet transform fusion | 9.66 | 53.75 |
Ours | 11.23 | 58.78 |
methods | incorrect stitching | ghosting artifacts | conspicuous distortions | Generate panorama | successfully stitched images (%) | average feature matching pair | average accuracy (%) |
ORB | √ | √ | √ | × | 20 | 16.3 | 10.1 |
GMS | √ | √ | √ | × | 26.6 | 26.4 | 23.1 |
PointCN | √ | √ | √ | × | 43.3 | 43.7 | 59.7 |
OANet | √ | √ | √ | 100 | 62.2 | 63.1 | |
SuperGlue | √ | √ | 100 | 89.5 | 79.3 | ||
Ours | √ | 100 | 108.4 | 86.2 |
Average number of feature points in the data set | The average number of feature point pairs that match each other | Average number of unmatched feature point pairs | Proportion of feature point pairs that match each other |
486 | 160 | 326 | 32% |
method type | Methods | Ms | P(%) | R(%) | Difference in absolute value of ||A||F |
Matching based on feature points | ORB | 11.9 | 10.8 | 2.6 | / |
GMS | 10.7 | 33.0 | 7.8 | ||
PointCN | 19.5 | 56.2 | 23.6 | ||
OANet | 23.8 | 65.0 | 40.9 | ||
SuperGlue | 31.4 | 78.3 | 67.8 | ||
Ours | 35.9 | 84.6 | 78.4 | ||
Based on homography matrix estimation | HomographyNet | / | 113.68 | ||
VFISNet | 84.47 | ||||
UDIS | 72.59 | ||||
Ours | 49.60 |
Methods | G-mean | PR-AUC |
PointCN | 0.445 | 0.484 |
OANet | 0.581 | 0.560 |
SuperGlue | 0.749 | 0.766 |
Ours | 0.832 | 0.813 |
Fusion methods | Average gradient | Standard deviation |
Based on maximum value | 6.65 | 58.71 |
Based on minimum value | 4.73 | 49.15 |
Average weighted | 7.45 | 50.29 |
Laplacian pyramid | 9.332 | 51.36 |
Wavelet transform fusion | 9.66 | 53.75 |
Ours | 11.23 | 58.78 |
methods | incorrect stitching | ghosting artifacts | conspicuous distortions | Generate panorama | successfully stitched images (%) | average feature matching pair | average accuracy (%) |
ORB | √ | √ | √ | × | 20 | 16.3 | 10.1 |
GMS | √ | √ | √ | × | 26.6 | 26.4 | 23.1 |
PointCN | √ | √ | √ | × | 43.3 | 43.7 | 59.7 |
OANet | √ | √ | √ | 100 | 62.2 | 63.1 | |
SuperGlue | √ | √ | 100 | 89.5 | 79.3 | ||
Ours | √ | 100 | 108.4 | 86.2 |