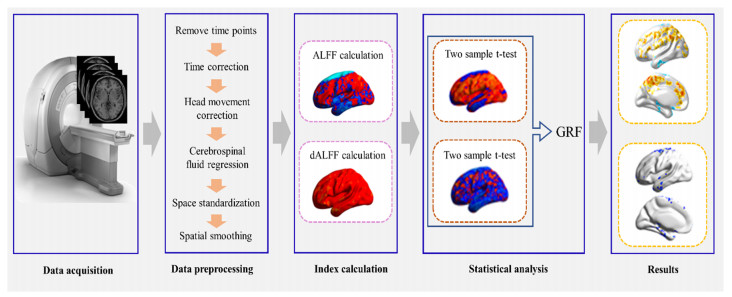
Methamphetamine (meth) addiction is a significant social and public health problem worldwide. The relapse rate of meth abstainers is significantly high, but the underlying physiological mechanisms are unclear. Therefore, in this study, we performed resting-state functional magnetic resonance imaging (rs-fMRI) analysis to detect differences in the spontaneous neural activity between the meth abstainers and the healthy controls, and identify the physiological mechanisms underlying the high relapse rate among the meth abstainers. The fluctuations and time variations in the blood oxygenation level-dependent (BOLD) signal of the local brain activity was analyzed from the pre-processed rs-fMRI data of 11 meth abstainers and 11 healthy controls and estimated the amplitude of low-frequency fluctuations (ALFF) and the dynamic ALFF (dALFF). In comparison with the healthy controls, meth abstainers showed higher ALFF in the anterior central gyrus, posterior central gyrus, trigonal-inferior frontal gyrus, middle temporal gyrus, dorsolateral superior frontal gyrus, and the insula, and reduced ALFF in the paracentral lobule and middle occipital gyrus. Furthermore, the meth abstainers showed significantly reduced dALFF in the supplementary motor area, orbital inferior frontal gyrus, middle frontal gyrus, medial superior frontal gyrus, middle occipital gyrus, insula, middle temporal gyrus, anterior central gyrus, and the cerebellum compared to the healthy controls (P<0.05). These data showed abnormal spontaneous neural activity in several brain regions related to the cognitive, executive, and other social functions in the meth abstainers and potentially represent the underlying physiological mechanisms that are responsible for the high relapse rate. In conclusion, a combination of ALFF and dALFF analytical methods can be used to estimate abnormal spontaneous brain activity in the meth abstainers and make a more reasonable explanation for the high relapse rate of meth abstainers.
Citation: Guixiang Liang, Xiang Li, Hang Yuan, Min Sun, Sijun Qin, Benzheng Wei. Abnormal static and dynamic amplitude of low-frequency fluctuations in multiple brain regions of methamphetamine abstainers[J]. Mathematical Biosciences and Engineering, 2023, 20(7): 13318-13333. doi: 10.3934/mbe.2023593
[1] | Ying Chen, Lele Wang, Zhixin Li, Yibin Tang, Zhan Huan . Unveiling critical ADHD biomarkers in limbic system and cerebellum using a binary hypothesis testing approach. Mathematical Biosciences and Engineering, 2024, 21(4): 5803-5825. doi: 10.3934/mbe.2024256 |
[2] | Enas Abdulhay, Maha Alafeef, Hikmat Hadoush, V. Venkataraman, N. Arunkumar . EMD-based analysis of complexity with dissociated EEG amplitude and frequency information: a data-driven robust tool -for Autism diagnosis- compared to multi-scale entropy approach. Mathematical Biosciences and Engineering, 2022, 19(5): 5031-5054. doi: 10.3934/mbe.2022235 |
[3] | Xiang Li, Jinyu Cong, Kunmeng Liu, Pingping Wang, Min Sun, Benzheng Wei . Aberrant intrinsic functional brain topology in methamphetamine-dependent individuals after six-months of abstinence. Mathematical Biosciences and Engineering, 2023, 20(11): 19565-19583. doi: 10.3934/mbe.2023867 |
[4] | Zhenquan Zhang, Junhao Liang, Zihao Wang, Jiajun Zhang, Tianshou Zhou . Modeling stochastic gene expression: From Markov to non-Markov models. Mathematical Biosciences and Engineering, 2020, 17(5): 5304-5325. doi: 10.3934/mbe.2020287 |
[5] | Hao Wang, Yang Kuang . Alternative models for cyclic lemming dynamics. Mathematical Biosciences and Engineering, 2007, 4(1): 85-99. doi: 10.3934/mbe.2007.4.85 |
[6] | Yi Yang, Lirong Liu, Changcheng Xiang, Wenjie Qin . Switching dynamics analysis of forest-pest model describing effects of external periodic disturbance. Mathematical Biosciences and Engineering, 2020, 17(4): 4328-4347. doi: 10.3934/mbe.2020239 |
[7] | Sven Blankenburg, Benjamin Lindner . The effect of positive interspike interval correlations on neuronal information transmission. Mathematical Biosciences and Engineering, 2016, 13(3): 461-481. doi: 10.3934/mbe.2016001 |
[8] | LanJiang Luo, Haihong Liu, Fang Yan . Dynamic behavior of P53-Mdm2-Wip1 gene regulatory network under the influence of time delay and noise. Mathematical Biosciences and Engineering, 2023, 20(2): 2321-2347. doi: 10.3934/mbe.2023109 |
[9] | Zhibo Liu, Yu Yuan, Ling Yu, Yingjie Li, Jiyou Fei . A novel threshold segmentation instantaneous frequency calculation approach for fault diagnosis. Mathematical Biosciences and Engineering, 2020, 17(5): 5395-5413. doi: 10.3934/mbe.2020291 |
[10] | Mingju Chen, Hongyang Li, Hongming Peng, Xingzhong Xiong, Ning Long . HPCDNet: Hybrid position coding and dual-frquency domain transform network for low-light image enhancement. Mathematical Biosciences and Engineering, 2024, 21(2): 1917-1937. doi: 10.3934/mbe.2024085 |
Methamphetamine (meth) addiction is a significant social and public health problem worldwide. The relapse rate of meth abstainers is significantly high, but the underlying physiological mechanisms are unclear. Therefore, in this study, we performed resting-state functional magnetic resonance imaging (rs-fMRI) analysis to detect differences in the spontaneous neural activity between the meth abstainers and the healthy controls, and identify the physiological mechanisms underlying the high relapse rate among the meth abstainers. The fluctuations and time variations in the blood oxygenation level-dependent (BOLD) signal of the local brain activity was analyzed from the pre-processed rs-fMRI data of 11 meth abstainers and 11 healthy controls and estimated the amplitude of low-frequency fluctuations (ALFF) and the dynamic ALFF (dALFF). In comparison with the healthy controls, meth abstainers showed higher ALFF in the anterior central gyrus, posterior central gyrus, trigonal-inferior frontal gyrus, middle temporal gyrus, dorsolateral superior frontal gyrus, and the insula, and reduced ALFF in the paracentral lobule and middle occipital gyrus. Furthermore, the meth abstainers showed significantly reduced dALFF in the supplementary motor area, orbital inferior frontal gyrus, middle frontal gyrus, medial superior frontal gyrus, middle occipital gyrus, insula, middle temporal gyrus, anterior central gyrus, and the cerebellum compared to the healthy controls (P<0.05). These data showed abnormal spontaneous neural activity in several brain regions related to the cognitive, executive, and other social functions in the meth abstainers and potentially represent the underlying physiological mechanisms that are responsible for the high relapse rate. In conclusion, a combination of ALFF and dALFF analytical methods can be used to estimate abnormal spontaneous brain activity in the meth abstainers and make a more reasonable explanation for the high relapse rate of meth abstainers.
Methamphetamine, also known as meth, is a strong stimulant of the central nervous system and is a predominant recreational drug worldwide [1,2]. Long-term abuse of meth causes abnormal changes in the brain structure and functions, thereby inducing psychiatric symptoms and cognitive deficits that are associated with mental disorders [3]. The long term meth abuse is also associated with increased likelihood of premature death from unnatural causes [4]. In recent years, the number of meth abusers has significantly increased globally. According to the statistics from the United Nations Office on Drugs and Crime (UNODC) in 2022, nearly 284 million individuals were reported to have used drugs in 2020 worldwide, a 26 percent increase over the previous decade, nearly 34 million individuals have used meth [5]. In 2021, meth abusers represented 53.1 percent of all drug addicts in China and were considered as a significant threat to public health [6]. A significant problem of drug addiction treatment is that a large percentage of meth abstainers easily relapse into addiction again. However, the underlying relapse mechanisms of addiction are still unclear because effective analytical methods have not been used in the existing studies.
Resting-state functional magnetic resonance imaging (rs-fMRI) is an effective non-invasive brain imaging method commonly used to analyze pathological changes in spontaneous neural activity in meth abstainers [7]. Currently, several fMRI analytical methods are available to estimate the spontaneous brain activity in the study subjects. The most commonly used method to analyze the spontaneous neuronal activity of the brain regions is the amplitude of low-frequency fluctuations (ALFF), which estimates the blood oxygenation level-dependent (BOLD) signal intensity as a read-out of the spontaneous activity in the brain region. Du and colleagues used ALFF to analyze the brain functional activities of the meth abusers and reported that the right middle frontal gyrus was a potential biomarker to identify the effects of abstinence during therapeutic intervention in the meth abusers [8]. Gong and colleagues used ALFF to investigate systemic dysfunction in the meth abstainers and reported that in comparison with the healthy control group, the intensity of ALFF increased in the right precuneus and decreased in the left posterior cingulate cortex of the meth abusers [9]. However, changes in the BOLD signal intensity related to the spontaneous activity in the brain regions is dynamic and varies with time in accordance with behavioral patterns [10]. Nevertheless, the ALFF method assumes that the brain signal is static and does not analyze the dynamic changes in the spontaneous neural activity of the brain, which is required to highlight the abnormalities in the brain activity and functions of the meth abstainers.
Dynamic ALFF (dALFF) is a novel method to analyze the temporal changes in the local brain activity [11]. The dALFF has been used to investigate the pathological changes in the dynamic local brain activity of patients with mental diseases such as depression, anxiety disorder, and schizophrenia [12,13,14]. In this study, we combined the ALFF and dALFF methods to investigate the static and the dynamic spontaneous neural activity in the brains of meth abstainers. Furthermore, we analyzed the relationship between the abnormal spontaneous neural activity and the high relapse rate in the meth abstainers.
To investigate the differences in spontaneous brain activity between meth abstainers and healthy controls, fMRI data were collected from recruited volunteers and the collected data were preprocessed. Then ALFF and dALFF index calculations were performed separately, and statistical analysis of ALFF and dALFF results were done for meth abstainers as well as healthy controls, and finally the differential brain areas of meth abstainers and healthy controls were derived. The flowchart of the study is shown in Figure 1.
This study recruited 11 male meth abstainers that had completed voluntary detoxification from the Shandong Detoxification Monitoring and Treatment Institute. The inclusion criteria were as follows: (i) right-handedness; (ii) without any previous addiction history other than meth addiction history; (iii) without any previous history of mental disorders or brain trauma; (iv) negative urine test after detoxification treatment for more than 14 days (out of the physiological detoxification period); and (v) without any medication intake for two weeks before data collection to avoid any adverse effects on the experimental results. The exclusion criteria were as follows: (i) subjects with fMRI contraindications; (ii) subjects with severe physical diseases.
This study recruited 11 healthy male volunteers from the local community without any significant differences in age, sex, and education level compared with the meth abstainers (P>0.05). The inclusion criteria were as follows: (i) right-handedness; (ii) without any previous history of alcohol or drug dependence; and (iii) without any previous history of mental disorders and brain trauma. The exclusion criteria were as follows: (i) patients with fMRI contraindication and (ii) subjects with severe physical diseases.
This study was approved by the Ethics Committee of the Affiliated Hospital of Shandong University of Traditional Chinese Medicine (Ethics No. 078 of 2018 Clinical Research Application). The signed informed consent forms were obtained from all the participants after they were informed about the whole study process before MRI scanning.
The fMRI was performed using the Philips Ingenia 3.0 T MRI scanner equipped with an eight-channel head coil. During the acquisition, the subjects laid on their backs and were requested to keep their eyes open and their heads fixed. Their heads were fixed with foam pads and the ears were plugged with sponge to prevent noise. Functional images were acquired using the gradient-echo planar imaging sequence with the following scanning parameters: TR = 2000 ms, TE = 30 ms, flip angle = 90°, field of view = 220 × 220 mm2, number of layers = 33, layer thickness = 3 mm, and matrix = 64 × 64. Data was acquired for 150 time points. The gradient echo sequence was also used to obtain the high-resolution structural images with the following scanning parameters: rotation angle = 90°; TR = 2300 ms; TE = 3.01 ms; matrix = 256 × 256; thickness = 1 mm.
The fMRI data was preprocessed using the Data Processing and Analysis for Brain Imaging (DPABI, http://rfmri.org/dpabi) [15] software based on MATLAB R2019b platform. Data for the first 10 time points were removed to ensure that MRI scanner signal had stabilized. When collecting data, the machine was scanned layer by layer, and there was a time difference between different layer acquisitions. The purpose of time layer correction was to adjust the data as if they were scanned instantaneously at the same moment. Head movement correction was performed to align the images at each point in time, eliminating to some extent the effect of voxel misalignment caused by head movement, subjects with head movement ≥ 3 mm or rotation angles ≥ 3° were removed from the analysis. Regression of the cerebrospinal fluid, white matter signal, and the Friston 24 head motion parameters was performed. Space standardization (matching function image to the EPI template) was performed and the MRI scan image was resampled to 3 mm × 3 mm × 3 mm. Spatial smoothing was performed to improve the signal-to-noise ratio.
ALFF was calculated using the DPABI software. The preprocessed fMRI data was filtered using a time band-pass filter (0.01 < f < 0.08 Hz) to reduce the low-frequency drift and the high-frequency respiratory and cardiac noise. The time series of each voxel was transformed into the frequency domain to obtain the power spectrum. The power of a given frequency is proportional to the square of the amplitude for the frequency component. Therefore, the square root of the signal power spectrum in the given range is calculated and the root mean square of 0.01~0.08 Hz is obtained for each voxel and denoted as previously described [16,17] using the following equations:
g(t)=∑Nk=1[akcos(2πfkt)+bksin(2πfkt)] | (1) |
ALFF=∑K:fk∈[0.01,0.1]√ak2(f)+bk2(f)N | (2) |
where t represents the time, ak and bk represent the amplitudes, g(t) represents the power spectrum.
The DynamicBC toolbox (https://www.nitrc.org/projects/dynamicbc) was used to calculate the dALFF. The toolkit used a sliding window to describe the time dynamics. The setting of the window length was critical to the results of the dynamic analysis. Robust estimation of the dynamic changes was affected if the window length was too small, and dynamic activities were not detected if the window lengths were too long. Therefore, the window length was set at 50 TR and the coverage rate was set at 0.6 as described in previous studies [18,19]. The complete time series of each subject was divided into windows with a specific length of time. Then the dALFF value in each window was calculated to generate a set of dALFF maps for each subject. Subsequently, variance was calculated to evaluate the variability of dALFF. Finally, for each participant, the dALFF variability of each voxel was further converted to z-scores.
The differences between the ALFF and the dALFF estimates for the meth abstainers and the healthy controls were compared using the two-sample t-test with the DPABI software. Statistical significance was defined by voxel-level P<0.001, cluster level P<0.05 (two-tailed) after the estimates were corrected using the Gaussian random field (GRF).
To validate the dALFF results found in this study, the influence of the sliding window length on the study results was verified by analyzing the dALFF when the window length was set at 20 TR and 80 TR.
Our study included 22 adult male subjects including 11 meth abstainers and 11 healthy controls. There were no significant differences in age (P=0.375) and education level (P=0.576) between the two groups. Table 1 shows the demographics and clinical data of the study subjects belonging to the meth abstainer group and the healthy control group.
Characteristics | MA* (n = 11) | HC** (n = 11) | P-value |
Age (y*****) | 29.4 ± 4.2 | 30.8 ± 3.2 | 0.375 |
Education (y) | 12.7 ± 2.4 | 13.4 ± 2.2 | 0.576 |
Amount of drug use (g/d***) | 0.36 ± 0.31 | -****** | - |
Duration of drug use (m****) | 48.9 ± 13.3 | - | - |
Abstinence (m) | 5.1 ± 1.5 | - | - |
Note: *: meth abstainers; **: healthy controls; ***: day; ****: month; *****: year; ******: represents no data. |
ALFF was significantly increased in the right precentral gyrus, the left postcentral gyrus, the right triangular part of the inferior frontal gyrus, the left dorsolateral superior frontal gyrus, the left middle temporal gyrus and the left insula and decreased in the left paracentral lobule and the right middle occipital gyrus regions of the meth abstainers compared to the healthy controls. The differences in the ALFF values between the meth abstainers and the healthy controls are shown in Table 2 and Figure 2. The brain regions with abnormal ALFF values in different coordinates are shown in Figure 3. The brain regions with the highest and the lowest ALFF in the meth abstainers compared to the healthy controls are shown in Figure 4A, B.
Brain regions | MNI* | Cluster size | Peak t | P-value |
X Y Z | ||||
Precentral_R | 54 6 39 | 5056 | 12.5621 | 0.00049 |
Postcentral_L | -39 -21 54 | 900 | 11.0992 | 0.00061 |
Paracentral_Lobule_L | -15 -30 78 | 758 | -3.74902 | 0.01264 |
Frontal_Sup_L | -27 51 24 | 23 | 5.99581 | 0.01678 |
Frontal_Inf_Tri_R | 45 36 27 | 61 | 8.60305 | 0.00809 |
Temporal_Mid_L | -57 -57 0 | 23 | 5.90803 | 0.04381 |
Occipital_Mid_R | 39 -87 24 | 17 | -3.77159 | 0.01467 |
Insula_L | -30 15 -18 | 21 | 7.1495 | 0.00381 |
*Note: Montreal Neurological Institute, Montreal Institute. |
The dALFF values were significantly decreased in the right supplementary motor area, the right orbital part of inferior, the left middle frontal gyrus, the left medial superior frontal gyrus, the left middle occipital gyrus, the right insula, the right middle temporal gyrus, the left precentral gyrus, and the cerebellum of the meth abstainers compared to the normal controls (Table 3 and Figure 5). The brain regions with abnormal dALFF values in different coordinates are shown in Figure 6. The brain regions with lowest dALFF values in the meth abstainers compared to the healthy controls are shown in Figure 4C.
Brain regions | MNI | Cluster size | Peak t | P-value |
X Y Z | ||||
Cerebelum_Crus2_R | 45 -78 -39 | 112 | -3.55195 | 0.01553 |
Supp_Motor_Area_R | 12 -6 75 | 151 | -3.55518 | 0.00902 |
Frontal_Inf_Orb_R | 51 30 -15 | 27 | -3.55345 | 0.00023 |
Frontal_Mid_L | -39 54 18 | 21 | -3.55442 | 0.00285 |
Frontal_Sup_Medial_L | 0 54 36 | 29 | -3.55188 | 0.00019 |
Occipital_Mid_L | -39 -87 18 | 25 | -3.58389 | 0.00172 |
Cerebelum_6_R | 21 -72 -15 | 22 | -3.57556 | 0.00169 |
Insula_R | 39 -18 0 | 15 | -3.56448 | 0.01921 |
Temporal_Mid_R | 69 -39 -12 | 14 | -3.55848 | 0.00889 |
Precentral_L | -57 12 33 | 12 | -3.57966 | 0.01386 |
The dALFF values were validated by comparing different sliding window lengths (20 TR and 80 TR). The results showed that the abnormal brain regions with the sliding window length set at 50TR were also significantly different with the sliding window length set at 20 TR and 80 TR. Figure 7 shows the difference of abnormal brain regions when the length of sliding window is set to 20TR and 80 TR In addition, we also studied the differences in brain regions between meth abstainers and healthy controls when the sliding window length was set to 40 TR and 60 TR, and the results were similar to those above. However, when the window was set to 50 TR, the differential brain clusters were larger. A previous study by Liao et al. similarly found that the spatial pattern of temporal variability of dALFF was highly reproducible across window lengths [20].
Several studies have analyzed resting state brain activity of the meth abstainers using fMRI, but very few studies have analyzed dynamic changes in the spontaneous neural activity of the meth abstainers. In our study, dynamic and static spontaneous neural activity of the meth abstainers was analyzed using the dALFF and the ALFF methods to determine the physiological mechanisms underlying the high relapse rate of the meth abstainers. ALFF analyzes the neural activity in the brain of meth abstainers from a static perspective, reflecting the intensity of regional neural activity in meth abstainers, while dALFF uses a sliding window approach to explore the characteristics of temporal changes in regional neural activity in meth abstainers at rest. Smaller dALFF values in meth abstainers indicate reduced fluctuations in spontaneous neural activity, also known as temporal variability, in the brains of meth abstainers compared with normal controls.
ALFF values were significantly increased in the precentral gyrus, postcentral gyrus, triangular part of the inferior frontal gyrus and the dorsolateral superior frontal gyrus, and decreased in the paracentral lobule of the meth abstainers compared to the healthy controls. Furthermore, dALFF values were significantly reduced in the supplementary motor area, orbital part of inferior frontal gyrus, middle frontal gyrus, medial superior frontal gyrus, and the precentral gyrus of the meth abstainers compared to the healthy controls. All these regions were located in the frontal lobe of the brain, which plays a vital role in the cognitive, executive, and control functions of the human body. The frontal lobe controls majority of the neuropsychological functions and is responsible for the advanced cognitive activities in humans [21]. The executive functions of the frontal lobe include attention, reasoning, judgement, problem solving, emotional regulation, impulse control, choosing between good and destructive behaviors, and general awareness of one's actions in relation to those of others around. The frontal lobe is also associated with long-term memory and emotional memory of the limbic system. Meth abuse causes structural and functional damage to the frontal lobes including the prefrontal cortex [22]. The main manifestations of frontal lobe damage are personality changes, impulsivity, inability to control emotions, reduced social activity, creativity, and problem-solving skills [23]. The abnormal regions of the frontal lobe in the brain of meth abstainers showed increased ALFF and decreased dALFF values. This suggested that the frontal lobes of the meth abusers were abnormally active and unstable. Therefore, we speculate that the abnormal spontaneous neural activity in the frontal cortex of the meth abstainers impairs their cognition, control, and executive ability, and may be responsible for the high relapse rate.
The middle temporal gyrus is a region of the brain that is critical for encoding and retrieving emotional episodic memory, including emotions, learning memory, and social cognition [24]. The middle temporal gyrus is in the temporal lobe, a brain region related to hearing and memory. The temporal lobe is mainly responsible for receiving and processing auditory information and is also associated with memory and emotions. It is the olfactory, speech, and hearing center of the human body. The main functions of the temporal lobe include processing the sensory input, participation in the primary auditory perception, advanced visual processing of complex stimuli, language recognition, visual and linguistic semantic processing, visual memory, and memory storage [25]. In the meth abstainers, the middle temporal gyrus showed increased ALFF and decreased dALFF. The interactions between neural elements have been shown to be dynamic, allowing for flexible, time-varying patterns of information flow [26]. This suggested increased frequency and decreased time variability of spontaneous fluctuations in the neural activity of the middle temporal gyrus. Therefore, the high recurrence rate of meth abstainers may be related to the abnormal spontaneous neural activity in the middle temporal gyrus that adversely affects episodic memory and social cognition.
The middle occipital gyrus is in the occipital lobe, which forms the core of the visual cortex and plays an essential role in reading, word recognition, and processing of visual information. In the meth abstainers, the occipital gyrus showed decreased ALFF and dALFF values. This suggested weakened frequency and temporal variability in the spontaneous neural fluctuations of the occipital gyrus in the meth abstainers. Long-term abuse of meth is associated with significant decline in visual memory decline and may be related to aberrant functional changes in the middle occipital gyrus [27]. We also observed decreased dALFF in the insula of the meth abstainers. The insula is a vital node of the right frontoparietal network and is involved in executive functions such as attention allocation, working memory, and performance monitoring and planning [28]. Insula is also a critical region of the brain that is involved in the addiction circuit. Furthermore, glucose metabolism is significantly reduced in the insula of the meth abstainers [29], and may represent a pathological basis for abnormal function of the insula.
Cerebellum is an integral part of the brain that coordinates the voluntary movements in the human body, regulates muscle tension, maintains body balance, and regulates cognitive activities and emotions [30]. Meth abuse induces neuronal apoptosis in the cerebellum and promotes pathological changes in the cerebellar structure that cause significant reduction in the cerebellar gray matter volume [31]. A previous study showed that the functional connectivity between the cerebellum and other cerebral functional networks such as the default mode, affective limbic, and sensorimotor networks were disrupted in the meth abstainers [32]. Furthermore, another study showed that meth abuse increased neural connectivity from both the thalamus and cerebellum to the sensorimotor and middle temporal gyrus networks, but the connectivity was reduced between the sensorimotor and the middle temporal gyrus networks [33]. Moreover, cerebellum also regulates cognitive emotions, attentional processing, and decision-making, all of which are deregulated in the drug abuse patients [34]. Our study showed reduced dALFF in most regions of the cerebellum. This suggested that the cerebellar injury caused by meth abuse adversely affected the normal structure and functions of the cerebellum. This provides a functional basis for the decreased emotional regulation in the meth abstainers.
In this study, we used a combination of ALFF and dALFF methods to analyze the fluctuation frequency of the spontaneous neural activity and changes in the time variability in different regions of the brain in the meth abstainers. However, our study has the following limitations: 1) The meth abstainers recruited in this study were all males. A previous study demonstrated gender-based differences in the user behavior of the meth abstainers [35]. However, there is no data regarding changes in the spontaneous neural activity in different regions of the brain of the female meth abstainers. 2) The sample size of this study was small, and all the study subjects were from a single center. This may have resulted in bias and affected the statistical results. Therefore, a multi-center large cohort study is required to validate our experimental results. 3) Optical coherence tomography (OCT) plays an important role in clinical medicine with its high resolution, high sensitivity, and high penetration depth. OCT can provide both functional and structural information about the brain [36,37,38,39], and in the future, it can be combined with fMRI to provide a variety of complementary information about brain function relevant to the study of relapse mechanisms in meth abstainers. 4) In recent years, an increasing number of researchers have used machine learning techniques to predict people at risk for addiction [40], and in the future, machine learning strategies could be used to model meth use and predict relapse rates among meth abstainers. 5) Deep learning has led to remarkable achievements in many research areas such as data mining, natural language processing and computer vision [41]. Deep learning can be used in the future to make further contributions to explore abnormalities in spontaneous neural activity in the brain of meth abstainers.
This study used combined analysis of ALFF and dALFF to determine changes in the frequency and time variability of the static and dynamic spontaneous neural activity in the meth abstainers. Abnormal spontaneous neural activity was mainly detected in the cerebellum, frontal lobe, temporal lobe, occipital lobe, and other brain regions, which control body balance, cognition, emotions, and execution. This suggested that combined analysis of the static and dynamic indicators can detect abnormal spontaneous neural activity in different brain regions of the meth abstainers, and can be used for identifying relapsing meth abstainers for clinical intervention. In conclusion, the ALFF and dALFF analytical methods demonstrate significant alterations in the spontaneous neural activity of the meth abstainers and provide a deeper understanding of the relapse mechanism in the meth abstainers that can be used to specifically guide clinical therapeutic strategy of the meth abstainers with higher risk of relapse.
This research was supported by funds from the National Nature Science Foundation of China (Grant No. 61872225), the Natural Science Foundation of Shandong Province (Grant Nos. ZR2019ZD04, ZR2020ZD44, ZR2020KF013 and ZR2020QF043), Introduction and Cultivation Program for Young Creative Talents in Colleges and Universities of Shandong Province (Grant No. 2019-173), Demonstration Projects of Science and Technology for the People of Qingdao City (Grant No. 23-2-8-smjk-2-nsh), and the Special fund of Qilu Health and Health Leading Talents Training Project.
Guixiang Liang and Xiang Li were responsible for research design and manuscript writing; Min Sun was responsible for data collection; Hang Yuan generated all the charts; Sijun Qin performed document retrieval; Benzheng Wei was responsible for research review and supervision. All the authors read and approved the final version of the manuscript.
The authors declare there is no conflict of interest.
[1] |
B. R. Lee, S. J. Sung, K. H. Hur, S. E. Kim, S. X. Ma, S. K. Kim, et al., Korean Red Ginseng inhibits methamphetamine addictive behaviors by regulating dopaminergic and NMDAergic system in rodents, J. Ginseng Res., 46 (2022), 147–155. https://doi.org/10.1016/j.jgr.2021.05.007 doi: 10.1016/j.jgr.2021.05.007
![]() |
[2] |
D. M. Stoneberg, R. K. Shukla, M. B. Magness, Global methamphetamine trends: an evolving problem, Int. Crim. Justice Rev., 28 (2018), 136–161. https://doi.org/10.1177/1057567717730104 doi: 10.1177/1057567717730104
![]() |
[3] |
L. Xu, L. Li, Q. Chen, Y. Huang, X. Chen, D. Qiao, The role of non-coding RNAs in methamphetamine-induced neurotoxicity, Cell. Mol. Neurobiol., 2023 (2023), 1–22. https://doi.org/10.1007/s10571-023-01323-x doi: 10.1007/s10571-023-01323-x
![]() |
[4] |
C. J. Kuo, Y. T. Liao, W. J. Chen, S. Y. Tsai, S. K. Lin, C. C. Chen, Causes of death of patients with methamphetamine dependence: a record‐linkage study, Drug Alcohol. Rev., 30 (2011), 621–628. https://doi.org/10.1111/j.1465-3362.2010.00255.x doi: 10.1111/j.1465-3362.2010.00255.x
![]() |
[5] | World Drug Report 2022, UN Office on Drugs and Crime, 2022. Available from: https://www.unodc.org/unodc/data-and-analysis/world-drug-report-2022.html. |
[6] |
P. Jiang, J. Y. Sun, X. B. Zhou, L. Lu, L. Li, X. Q. Huang, et al., Functional connectivity abnormalities underlying mood disturbances in male abstinent methamphetamine abusers, Hum. Brain Mapp., 42 (2021), 3366–3378. https://doi.org/10.1002/hbm.25439 doi: 10.1002/hbm.25439
![]() |
[7] |
P. J. McCarty, A. R. Pines, B. L. Sussman, S. N. Wyckoff, A. Jensen, R. Bunch, et al., Resting state functional magnetic resonance imaging elucidates neurotransmitter deficiency in autism spectrum disorder, J. Pers. Med., 11 (2021), https://doi.org/10.3390/jpm11100969 doi: 10.3390/jpm11100969
![]() |
[8] |
Y. Y. Du, W. H. Yang, J. Zhang, J. Liu, Changes in ALFF and ReHo values in methamphetamine abstinent individuals based on the Harvard-Oxford atlas: A longitudinal resting-state fMRI study, Addict. Biol., 27 (2022), e13080. https://doi.org/10.1111/adb.13080 doi: 10.1111/adb.13080
![]() |
[9] |
M. Q. Gong, Y. X. Shen, W. B. Liang, Z. Zhang, C. X. He, M. W. Lou, et al., Impairments in the default mode and executive networks in methamphetamine usersduring short-term abstinence, Int. J. Gen. Med., 15 (2022), 6073–6084. https://doi.org/10.2147/ijgm.S369571 doi: 10.2147/ijgm.S369571
![]() |
[10] |
O. Sporns, The non-random brain: efficiency, economy, and complex dynamics, Front. Comput. Neurosci., 5 (2011), 5. https://doi.org/10.3389/fncom.2011.00005 doi: 10.3389/fncom.2011.00005
![]() |
[11] |
W. Liao, G. R. Wu, Q. Xu, G. J. Ji, Z. Q. Zhang, Y. F. Zang, et al., DynamicBC: a MATLAB toolbox for dynamic brain connectome analysis, Brain Connect., 4 (2014), 780–790. https://doi.org/10.1089/brain.2014.0253 doi: 10.1089/brain.2014.0253
![]() |
[12] |
Q. Cui, W. Sheng, Y. Y. Chen, Y. J. Pang, F. M. Lu, Q. Tang, et al., Dynamic changes of amplitude of low-frequency fluctuations in patients with generalized anxiety disorder, Hum. Brain Mapp., 41 (2020), 1667–1676. https://doi.org/10.1002/hbm.24902 doi: 10.1002/hbm.24902
![]() |
[13] |
J. Li, X. J. Duan, Q. Cui, H. F. Chen, W. Liao, More than just statics: temporal dynamics of intrinsic brain activity predicts the suicidal ideation in depressed patients, Psychol. Med., 49 (2019), 852–860. https://doi.org/10.1017/s0033291718001502 doi: 10.1017/s0033291718001502
![]() |
[14] |
Z. Fu, A. Iraji, J. A. Turner, J. Sui, R. Miller, G. D. Pearlson, et al., Dynamic state with covarying brain activity-connectivity: On the pathophysiology of schizophrenia, Neuroimage, 224 (2021), 117385. https://doi.org/10.1016/j.neuroimage.2020.117385 doi: 10.1016/j.neuroimage.2020.117385
![]() |
[15] |
C. G. Yan, X. D. Wang, X. N. Zuo, Y. F. Zang, DPABI: data processing & analysis for (resting-state) brain imaging, Neuroinformatics, 14 (2016), 339–351. https://doi.org/10.1007/s12021-016-9299-4 doi: 10.1007/s12021-016-9299-4
![]() |
[16] |
J. J. Wang, X. Chen, S. K. Sah, C. Zeng, Y. M. Li, N. Li, et al., Amplitude of low-frequency fluctuation (ALFF) and fractional ALFF in migraine patients: a resting-state functional MRI study, Clin. Radiol., 71 (2016), 558–564. https://doi.org/10.1016/j.crad.2016.03.004 doi: 10.1016/j.crad.2016.03.004
![]() |
[17] |
H. Yuan, X. H. Yu, X. Li, S. J. Qin, G. X. Liang, T. Y. Bai, et al., Research on resting spontaneous brain activity and functional connectivity of acupuncture at uterine acupoints, Digital Chin. Med., 5 (2022), 59–67. https://doi.org/10.1016/j.dcmed.2022.03.006 doi: 10.1016/j.dcmed.2022.03.006
![]() |
[18] |
R. Li, W. Liao, Y. Y. Yu, H. Chen, X. N. Guo, Y. L. Tang, et al., Differential patterns of dynamic functional connectivity variability of striato-cortical circuitry in children with benign epilepsy with centrotemporal spikes, Hum. Brain Mapp., 39 (2018), 1207–1217. https://doi.org/10.1002/hbm.23910 doi: 10.1002/hbm.23910
![]() |
[19] |
Q. Li, X. H. Cao, S. Liu, Z. X. Li, Y. F. Wang, L. Cheng, et al., Dynamic alterations of amplitude of low-frequency fluctuations in patients with drug-naive first-episode early onset schizophrenia, Front. Neurosci., 14 (2020), 901. https://doi.org/10.3389/fnins.2020.00901 doi: 10.3389/fnins.2020.00901
![]() |
[20] |
W. Liao, J. Li, G. J. Ji, G. R. Wu, Z. Long, Q. Xu, et al., Endless fluctuations: temporal dynamics of the amplitude of low frequency fluctuations, IEEE Trans. Med. Imaging, 38 (2019), 2523–2532. https://doi.org/10.1109/TMI.2019.2904555 doi: 10.1109/TMI.2019.2904555
![]() |
[21] | A. Ardila, Executive functions brain functional system, in Dysexecutive syndromes: Clinical and experimental perspectives, Springer Press, (2019), 29–41. https://doi.org/10.1007/978-3-030-25077-5_2 |
[22] |
M. Gong, W. Liang, C. He, Y. Shen, Z. Zhang, M. Lou, et al., Neuroimaging mechanisms in short-term heroin-and methamphetamine-abstinent users: Similarities and differences, Neurosci. Lett., 796 (2023), 137057. https://doi.org/10.1016/j.neulet.2023.137057 doi: 10.1016/j.neulet.2023.137057
![]() |
[23] | J. G. Scott, M. R. Schoenberg, Frontal lobe/executive functioning, in The little black book of neuropsychology: A syndrome-based approach, Springer Press, (2010) 219–248. https://doi.org/10.1007/978-0-387-76978-3_10 |
[24] |
W. Sato, M. Toichi, S. Uono, T. Kochiyama, Impaired social brain network for processing dynamic facial expressions in autism spectrum disorders, BMC Neurosci., 13 (2012), 1–17. https://doi.org/10.1186/1471-2202-13-99 doi: 10.1186/1471-2202-13-99
![]() |
[25] |
W. Xie, J. I. Chapeton, S. Bhasin, C. Zawora, J. H. Wittig Jr, S. K. Inati, et al., The medial temporal lobe supports the quality of visual short-term memory representation, Nat. Hum. Behav., 7 (2023), 627–641. https://doi.org/10.1038/s41562-023-01529-5 doi: 10.1038/s41562-023-01529-5
![]() |
[26] |
A. Avena-Koenigsberger, B. Misic, O. Sporns, Communication dynamics in complex brain networks, Nat. Rev. Neurosci., 19 (2018), 17–33. https://doi.org/10.1038/nrn.2017.149 doi: 10.1038/nrn.2017.149
![]() |
[27] |
Y. Li, L. Liu, E. Wang, H. Zhang, S. Dou, L. Tong, et al., Abnormal neural network of primary insomnia: evidence from spatial working memory task fMRI, Eur. Neurol., 75 (2016), 48–57. https://doi.org/10.1159/000443372 doi: 10.1159/000443372
![]() |
[28] |
T. P. Zanto, A. Gazzaley, Fronto-parietal network: flexible hub of cognitive control, Trends Cognit. Sci., 17 (2013), 602–603. https://doi.org/10.1016/j.tics.2013.10.001 doi: 10.1016/j.tics.2013.10.001
![]() |
[29] |
D. Vuletic, P. Dupont, F. Robertson, J. Warwick, J. R. Zeevaart, D. J. Stein, Methamphetamine dependence with and without psychotic symptoms: A multi-modal brain imaging study, Neuroimage Clin., 20 (2018), 1157–1162. https://doi.org/10.1016/j.nicl.2018.10.023 doi: 10.1016/j.nicl.2018.10.023
![]() |
[30] |
U. Wolf, M. J. Rapoport, T. A. Schweizer, Evaluating the affective component of the cerebellar cognitive affective syndrome, J. Neuropsychiatry Clin. Neurosci., 21 (2009), 245–253. https://doi.org/10.1176/jnp.2009.21.3.245 doi: 10.1176/jnp.2009.21.3.245
![]() |
[31] |
E. A. Moulton, I. Elman, L. R. Becerra, R. Z. Goldstein, D. Borsook, The cerebellum and addiction: insights gained from neuroimaging research, Addict. Biol., 19 (2014), 317–331. https://doi.org/10.1111/adb.12101 doi: 10.1111/adb.12101
![]() |
[32] |
X. T. Li, H. Su, N. Zhong, T. Z. Chen, J. Du, K. Xiao, et al., Aberrant resting-state cerebellar-cerebral functional connectivity in methamphetamine-dependent individuals after six months abstinence, Front. Psychiatry, 11 (2020), 191. https://doi.org/10.3389/fpsyt.2020.00191 doi: 10.3389/fpsyt.2020.00191
![]() |
[33] |
M. Malina, S. Keedy, J. Weafer, K. Van Hedger, H. de Wit, Effects of methamphetamine on within-and between-network connectivity in healthy adults, Cereb. Cortex, 2 (2021), tgab063. https://doi.org/10.1093/texcom/tgab063 doi: 10.1093/texcom/tgab063
![]() |
[34] | A. Sathyanesan, J. Zhou, J. Scafidi, D. H. Heck, R. V. Sillitoe, V. Gallo, Emerging connections between cerebellar development, behaviour and complex brain disorders, Nat. Rev. Neurosci., 20 (2019), 298–313. https://doi.org/10.1038/s41583-019-0152-2 |
[35] | E. A. Evans, C. E. Grella, D. M. Upchurch, Gender differences in the effects of childhood adversity on alcohol, drug, and polysubstance-related disorders, Social Psychiatry Psychiatr. Epidemiol., 52 (2017), 901–912. https://doi.org/10.1007/s00127-017-1355-3 |
[36] |
K. Ratheesh, L. Seah, V. Murukeshan, Spectral phase-based automatic calibration scheme for swept source-based optical coherence tomography systems, Phys. Med. Biol., 61 (2016), 7652. https://doi.org/10.1088/0031-9155/61/21/7652 doi: 10.1088/0031-9155/61/21/7652
![]() |
[37] |
R. K. Meleppat, K. E. Ronning, S. J. Karlen, M. E. Burns, E. N. Pugh, R. J. Zawadzki, In vivo multimodal retinal imaging of disease-related pigmentary changes in retinal pigment epithelium, Sci. Rep., 11 (2021), 1–14. https://doi.org/10.1038/s41598-021-95320-z doi: 10.1038/s41598-021-95320-z
![]() |
[38] |
R. K. Meleppat, C. R. Fortenbach, Y. Jian, E. S. Martinez, K. Wagner, B. S. Modjtahedi, et al., In vivo imaging of retinal and choroidal morphology and vascular plexuses of vertebrates using swept-source optical coherence tomography, Transl. Vision Sci. Technol., 11 (2022), 11. https://doi.org/10.1167/tvst.11.8.11 doi: 10.1167/tvst.11.8.11
![]() |
[39] |
R. K. Meleppat, C. Shearwood, S. L. Keey, M. V. Matham, Quantitative optical coherence microscopy for the in situ investigation of the biofilm, J. Biomed. Opt., 21 (2016), 127002–127002. http://dx.doi.org/10.1117/1.JBO.21.12.127002 doi: 10.1117/1.JBO.21.12.127002
![]() |
[40] | W. Abada, A. Bouramoul, Using machinelearning techniques to predict people at-risk for drug addiction: A Bayesian-Based Model, in 2022 4th International Conference on Pattern Analysis and Intelligent Systems (PAIS), IEEE, (2022), 1–7. https://doi.org/10.1109/PAIS56586.2022.9946914 |
[41] |
H. Chen, C. Li, G. Wang, X. Li, M. M. Rahaman, H. Sun, et al., GasHis-Transformer: A multi-scale visual transformer approach for gastric histopathological image detection, Pattern Recognit., 130 (2022), 108827. https://doi.org/10.1016/j.patcog.2022.108827 doi: 10.1016/j.patcog.2022.108827
![]() |
1. | Maximilian Fascher, Sandra Nowaczynski, Markus Muehlhan, Substance use disorders are characterised by increased voxel-wise intrinsic measures in sensorimotor cortices: An ALE meta-analysis, 2024, 162, 01497634, 105712, 10.1016/j.neubiorev.2024.105712 | |
2. | Yanan Zhou, Winson Fu Zun Yang, Qiuxia Wu, Yuejiao Ma, Jun Zhou, Honghong Ren, Yuzhu Hao, Manyun Li, Yunfei Wang, Pu Peng, Ning Yuan, Yifan Xiong, Yizhuo Wang, Qianjin Wang, Tieqiao Liu, Altered spontaneous neurological activity in methamphetamine use disorders and its association with cognitive function, 2024, 94, 18762018, 103936, 10.1016/j.ajp.2024.103936 | |
3. | Xiang Li, Jinyu Cong, Kunmeng Liu, Pingping Wang, Min Sun, Benzheng Wei, Aberrant intrinsic functional brain topology in methamphetamine-dependent individuals after six-months of abstinence, 2023, 20, 1551-0018, 19565, 10.3934/mbe.2023867 | |
4. | Jiaxue Sun, Yi Lu, Deshenyue Kong, Wenhua Lin, Jinze Du, Guangqing Wang, Xingfeng Ma, Congbin Li, Kunhua Wang, Mei Zhu, Yu Xu, Cerebral blood flow in the paracentral lobule is associated with poor subjective sleep quality among patients with a history of methadone maintenance treatment, 2024, 15, 1664-2295, 10.3389/fneur.2024.1400810 |
Characteristics | MA* (n = 11) | HC** (n = 11) | P-value |
Age (y*****) | 29.4 ± 4.2 | 30.8 ± 3.2 | 0.375 |
Education (y) | 12.7 ± 2.4 | 13.4 ± 2.2 | 0.576 |
Amount of drug use (g/d***) | 0.36 ± 0.31 | -****** | - |
Duration of drug use (m****) | 48.9 ± 13.3 | - | - |
Abstinence (m) | 5.1 ± 1.5 | - | - |
Note: *: meth abstainers; **: healthy controls; ***: day; ****: month; *****: year; ******: represents no data. |
Brain regions | MNI* | Cluster size | Peak t | P-value |
X Y Z | ||||
Precentral_R | 54 6 39 | 5056 | 12.5621 | 0.00049 |
Postcentral_L | -39 -21 54 | 900 | 11.0992 | 0.00061 |
Paracentral_Lobule_L | -15 -30 78 | 758 | -3.74902 | 0.01264 |
Frontal_Sup_L | -27 51 24 | 23 | 5.99581 | 0.01678 |
Frontal_Inf_Tri_R | 45 36 27 | 61 | 8.60305 | 0.00809 |
Temporal_Mid_L | -57 -57 0 | 23 | 5.90803 | 0.04381 |
Occipital_Mid_R | 39 -87 24 | 17 | -3.77159 | 0.01467 |
Insula_L | -30 15 -18 | 21 | 7.1495 | 0.00381 |
*Note: Montreal Neurological Institute, Montreal Institute. |
Brain regions | MNI | Cluster size | Peak t | P-value |
X Y Z | ||||
Cerebelum_Crus2_R | 45 -78 -39 | 112 | -3.55195 | 0.01553 |
Supp_Motor_Area_R | 12 -6 75 | 151 | -3.55518 | 0.00902 |
Frontal_Inf_Orb_R | 51 30 -15 | 27 | -3.55345 | 0.00023 |
Frontal_Mid_L | -39 54 18 | 21 | -3.55442 | 0.00285 |
Frontal_Sup_Medial_L | 0 54 36 | 29 | -3.55188 | 0.00019 |
Occipital_Mid_L | -39 -87 18 | 25 | -3.58389 | 0.00172 |
Cerebelum_6_R | 21 -72 -15 | 22 | -3.57556 | 0.00169 |
Insula_R | 39 -18 0 | 15 | -3.56448 | 0.01921 |
Temporal_Mid_R | 69 -39 -12 | 14 | -3.55848 | 0.00889 |
Precentral_L | -57 12 33 | 12 | -3.57966 | 0.01386 |
Characteristics | MA* (n = 11) | HC** (n = 11) | P-value |
Age (y*****) | 29.4 ± 4.2 | 30.8 ± 3.2 | 0.375 |
Education (y) | 12.7 ± 2.4 | 13.4 ± 2.2 | 0.576 |
Amount of drug use (g/d***) | 0.36 ± 0.31 | -****** | - |
Duration of drug use (m****) | 48.9 ± 13.3 | - | - |
Abstinence (m) | 5.1 ± 1.5 | - | - |
Note: *: meth abstainers; **: healthy controls; ***: day; ****: month; *****: year; ******: represents no data. |
Brain regions | MNI* | Cluster size | Peak t | P-value |
X Y Z | ||||
Precentral_R | 54 6 39 | 5056 | 12.5621 | 0.00049 |
Postcentral_L | -39 -21 54 | 900 | 11.0992 | 0.00061 |
Paracentral_Lobule_L | -15 -30 78 | 758 | -3.74902 | 0.01264 |
Frontal_Sup_L | -27 51 24 | 23 | 5.99581 | 0.01678 |
Frontal_Inf_Tri_R | 45 36 27 | 61 | 8.60305 | 0.00809 |
Temporal_Mid_L | -57 -57 0 | 23 | 5.90803 | 0.04381 |
Occipital_Mid_R | 39 -87 24 | 17 | -3.77159 | 0.01467 |
Insula_L | -30 15 -18 | 21 | 7.1495 | 0.00381 |
*Note: Montreal Neurological Institute, Montreal Institute. |
Brain regions | MNI | Cluster size | Peak t | P-value |
X Y Z | ||||
Cerebelum_Crus2_R | 45 -78 -39 | 112 | -3.55195 | 0.01553 |
Supp_Motor_Area_R | 12 -6 75 | 151 | -3.55518 | 0.00902 |
Frontal_Inf_Orb_R | 51 30 -15 | 27 | -3.55345 | 0.00023 |
Frontal_Mid_L | -39 54 18 | 21 | -3.55442 | 0.00285 |
Frontal_Sup_Medial_L | 0 54 36 | 29 | -3.55188 | 0.00019 |
Occipital_Mid_L | -39 -87 18 | 25 | -3.58389 | 0.00172 |
Cerebelum_6_R | 21 -72 -15 | 22 | -3.57556 | 0.00169 |
Insula_R | 39 -18 0 | 15 | -3.56448 | 0.01921 |
Temporal_Mid_R | 69 -39 -12 | 14 | -3.55848 | 0.00889 |
Precentral_L | -57 12 33 | 12 | -3.57966 | 0.01386 |