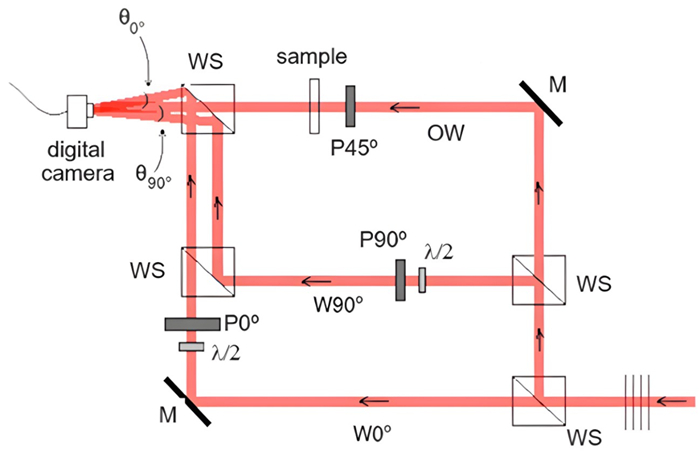
Methamphetamine (meth) addiction is a significant social and public health problem worldwide. The relapse rate of meth abstainers is significantly high, but the underlying physiological mechanisms are unclear. Therefore, in this study, we performed resting-state functional magnetic resonance imaging (rs-fMRI) analysis to detect differences in the spontaneous neural activity between the meth abstainers and the healthy controls, and identify the physiological mechanisms underlying the high relapse rate among the meth abstainers. The fluctuations and time variations in the blood oxygenation level-dependent (BOLD) signal of the local brain activity was analyzed from the pre-processed rs-fMRI data of 11 meth abstainers and 11 healthy controls and estimated the amplitude of low-frequency fluctuations (ALFF) and the dynamic ALFF (dALFF). In comparison with the healthy controls, meth abstainers showed higher ALFF in the anterior central gyrus, posterior central gyrus, trigonal-inferior frontal gyrus, middle temporal gyrus, dorsolateral superior frontal gyrus, and the insula, and reduced ALFF in the paracentral lobule and middle occipital gyrus. Furthermore, the meth abstainers showed significantly reduced dALFF in the supplementary motor area, orbital inferior frontal gyrus, middle frontal gyrus, medial superior frontal gyrus, middle occipital gyrus, insula, middle temporal gyrus, anterior central gyrus, and the cerebellum compared to the healthy controls (P<0.05). These data showed abnormal spontaneous neural activity in several brain regions related to the cognitive, executive, and other social functions in the meth abstainers and potentially represent the underlying physiological mechanisms that are responsible for the high relapse rate. In conclusion, a combination of ALFF and dALFF analytical methods can be used to estimate abnormal spontaneous brain activity in the meth abstainers and make a more reasonable explanation for the high relapse rate of meth abstainers.
Citation: Guixiang Liang, Xiang Li, Hang Yuan, Min Sun, Sijun Qin, Benzheng Wei. Abnormal static and dynamic amplitude of low-frequency fluctuations in multiple brain regions of methamphetamine abstainers[J]. Mathematical Biosciences and Engineering, 2023, 20(7): 13318-13333. doi: 10.3934/mbe.2023593
[1] | Katsumasa Satoh, Yasuhide Tsuji . A study on photonic crystal slab waveguide with absolute photonic band gap. AIMS Materials Science, 2018, 5(1): 116-126. doi: 10.3934/matersci.2018.1.116 |
[2] | M.M. Reza Mousavi, Masoud D. Champiri, Mohammad S. Joshaghani, Shahin Sajjadi . A kinematic measurement for ductile and brittle failure of materials using digital image correlation. AIMS Materials Science, 2016, 3(4): 1759-1772. doi: 10.3934/matersci.2016.4.1759 |
[3] | Grujicic Mica, Galgalikar Rohan, Ramaswami S., Snipes Jennifer S. . Derivation, parameterization and validation of a creep deformation/rupture material constitutive model for SiC/SiC ceramic-matrix composites (CMCs). AIMS Materials Science, 2016, 3(2): 591-619. doi: 10.3934/matersci.2016.2.591 |
[4] | M.A.R. Loja, André Carvalho, Ines C.J. Barbosa . A study on the static behavior of functionally graded I-shaped beams. AIMS Materials Science, 2024, 11(1): 28-57. doi: 10.3934/matersci.2024002 |
[5] | Ahmed M. Abood, Haider Khazal, Abdulkareem F. Hassan . On the determination of first-mode stress intensity factors and T-stress in a continuous functionally graded beam using digital image correlation method. AIMS Materials Science, 2022, 9(1): 56-70. doi: 10.3934/matersci.2022004 |
[6] | G. Sri Harsha, P. Poluraju, Veerendrakumar C. Khed . Computation of shear strength equation for shear deformation of reinforced concrete deep beams using finite element method. AIMS Materials Science, 2021, 8(1): 42-61. doi: 10.3934/matersci.2021004 |
[7] | Mohammed Y. Abdellah, Hamzah Alharthi, Mohamed K. Hassan, Ahmed F. Mohamed . Effect of specimen size on natural vibration of open hole copper/glass-reinforced epoxy laminate composites. AIMS Materials Science, 2020, 7(4): 499-517. doi: 10.3934/matersci.2020.4.499 |
[8] | Ivan Jandrlić, Stoja Rešković, Dušan Ćurčija, Ladislav Lazić, Tin Brlić . Modeling of stress distribution on the basis of the measured values of strain and temperature changes. AIMS Materials Science, 2019, 6(4): 601-609. doi: 10.3934/matersci.2019.4.601 |
[9] | Sujith Bobba, Z. Leman, E.S. Zainudin, S.M. Sapuan . Hoop tensile strength behaviour between different thicknesses E-glass and S-glass FRP rings. AIMS Materials Science, 2019, 6(3): 315-327. doi: 10.3934/matersci.2019.3.315 |
[10] | Bandar Abdullah Aloyaydi, Subbarayan Sivasankaran, Hany Rizk Ammar . Influence of infill density on microstructure and flexural behavior of 3D printed PLA thermoplastic parts processed by fusion deposition modeling. AIMS Materials Science, 2019, 6(6): 1033-1048. doi: 10.3934/matersci.2019.6.1033 |
Methamphetamine (meth) addiction is a significant social and public health problem worldwide. The relapse rate of meth abstainers is significantly high, but the underlying physiological mechanisms are unclear. Therefore, in this study, we performed resting-state functional magnetic resonance imaging (rs-fMRI) analysis to detect differences in the spontaneous neural activity between the meth abstainers and the healthy controls, and identify the physiological mechanisms underlying the high relapse rate among the meth abstainers. The fluctuations and time variations in the blood oxygenation level-dependent (BOLD) signal of the local brain activity was analyzed from the pre-processed rs-fMRI data of 11 meth abstainers and 11 healthy controls and estimated the amplitude of low-frequency fluctuations (ALFF) and the dynamic ALFF (dALFF). In comparison with the healthy controls, meth abstainers showed higher ALFF in the anterior central gyrus, posterior central gyrus, trigonal-inferior frontal gyrus, middle temporal gyrus, dorsolateral superior frontal gyrus, and the insula, and reduced ALFF in the paracentral lobule and middle occipital gyrus. Furthermore, the meth abstainers showed significantly reduced dALFF in the supplementary motor area, orbital inferior frontal gyrus, middle frontal gyrus, medial superior frontal gyrus, middle occipital gyrus, insula, middle temporal gyrus, anterior central gyrus, and the cerebellum compared to the healthy controls (P<0.05). These data showed abnormal spontaneous neural activity in several brain regions related to the cognitive, executive, and other social functions in the meth abstainers and potentially represent the underlying physiological mechanisms that are responsible for the high relapse rate. In conclusion, a combination of ALFF and dALFF analytical methods can be used to estimate abnormal spontaneous brain activity in the meth abstainers and make a more reasonable explanation for the high relapse rate of meth abstainers.
Transparent photoelastic materials represent an exciting alternative to the experimental study of stress and strain distributions induced in solids by carges. When subjected to carges, these materials present the double refraction phenomenon, or birefringence, changing the polarization state of the transmitted light through the solids, which can be used to analyze the stress distribution [1]. The effect of double refraction, first described by Bartholinus [2,3,4] and related with the stress state by Brewster in the early 19th century [5], advanced throughout the 20th century with a non-destructive set of techniques and methods which associates the study of material stresses with optics, the photoelasticity [6,7,8]. Since the pioneering work of Coker and Filon [9,10,11], photoelasticity became a fundamental base for determining stress and strain distributions in photoelastic materials. Thus, great interest was generated in several fields, such as Engineering and Odontology [12,13,14], which validated and contributed to developing the theoretical method of finite elements [15,16].
Despite advances, most studies are qualitative or indirectly quantitative [13,14] due to the difficulties in obtaining direct optical information. Improvements in qualitative data and quantitative analysis methods are needed so that non-destructive, fast, and reliable optical methods become a reference for determining stress distribution in materials. Although holographic techniques have advanced significantly in recent years, the various works have invested very little in the stresses distribution analysis in photoelasticity using holography. The dynamics of holography allow the results to be more precise, as they are based on optical properties such as intensity, phase, refractive index, etc., which are provided directly or almost directly, thus offering a great perspective in the more quantitative treatment of problems involving elasticity mechanics [17].
We present an alternative approach to determine the stress distribution profile through a non-destructive procedure based on digital holography (DH) [18,19], allowing us to obtain quantitative intensity and phase information from light transmitted through a photoelastic material. The DH produces remarkably accurate results when combined with appropriate statistical processing of optical data, facilitating the quantitative treatment of the specific problem as outlined in the proposed methods [20]. An off-axis holographic setup was used to obtain two cross-holograms with two orthogonally polarized reference waves and a birefringent system with photoelastic samples under static loads [21,22,23,24,25]. After digitally reconstructed with DH, the received data generates the phase differences used to calculate the distributions of elastic stresses. The validation of the method was carried out with the methods finite elements and RGB (red, green, and blue) photoelasticity [14,16,26,27].
Four standard rectangular blocks, composed of mixtures of epoxy resin solutions, were prepared according to the traditional procedures [28] of the photoelastic technique, constituting the samples used in this work. The preparation of the samples involved two stages: making the silicone molds, from curing in a liquid solution and catalyst, and the photoelastic samples, from curing, in silicone molds, a liquid solution of epoxy resin and hardener. Details are presented in the work [17]. For the determinations of the mechanical and holographic parameters, two samples with different thicknesses were made, one more flexible and one less flexible. Two other samples with different thicknesses, one more and one less flexible, were also made to determine the stress distributions. Details of the procedures are presented in [17]. The more or less flexible samples were intended to help verify the order of magnitude of the stress-optical coefficient (C) and to provide a greater range of comparison with the photoelastic methodology.
The utilized holographic technique is shown in Figure 1.
A laser light source (1) was used to generate three independent waves: one object wave (OW), with the direction of polarization at 45º concerning two orthogonal reference waves, one with the direction of polarization at 0º (W0º), and another with the direction of polarization at 90º (W90º). Two distinct holograms were produced from the resulting interference patterns among OW, W0º, and W90º, propagating with different angles to the digital camera, as shown in Figure 1: θ(0º) between the OW and W0º, and θ (90º) between OW and W90º, as limited by the N-quest Theorem [29,30,31]. Two sets of holograms were recorded from each sample for compression and decompression in the birefringent system. The compression occurs by the progressive addition of load on the samples, and the decompression occurs by progressive removal of these loads.
The photoelastic images were obtained by blocking both reference waves, removing the wave splitter (WS), near the digital camera, and exchanging the polarizer, P45º, of the object wave for two polarizers with orthogonal polarizations, one before and another after the photoelastic sample. Figure 2 presents an experimental configuration scheme used in photoelasticity.
The optical information obtained through photoelasticity is related to the difference between the stresses considered in the components longitudinal (σ∥) and transverse (σ⊥) to the applied load, defined by Eq 1, as given by the stress-optic law [6,17,28]:
n∥−n⊥=C(σ∥−σ⊥) | (1) |
where C is the stress-optical coefficient, and (n∥−n⊥) is the difference between the refraction indexes in the components longitudinal (n∥) and transverse (n⊥) to the effort. For a material with thickness e, the refractive index difference is also associated with the phase difference Δϕ, so Eq 1 can be rewritten as Eq 2:
(σ∥−σ⊥)=fσNe | (2) |
where N=Δϕ2π is defined as the relative retardation, fσ=λC is the fringe value that indicates the degree of rigidity of the material, and λ is the wavelength of the light source. Using the matrix of stress-strain (σ−ε) of the material in the stress state plane [26], the difference between the stresses in the orthogonal components, defined by Eq 3, is [17]:
[σ⊥σ∥]=E1−ν2[1νν1][ε⊥ε∥]⇒(σ∥−σ⊥)=E1+ν(ε∥−ε⊥) | (3) |
E is the mechanical elasticity modulus, and ν is the Poisson's coefficient. Therefore, comparing Eqs 2 and 3, with N=(ε∥−ε⊥), the material fringes value can be determined by their intrinsic properties through Eq 4 [17]:
fσ=eE1+ν=λC(λ) | (4) |
The holographic method was empirically inferred and correlated with the stress-optic law. The stress difference (σ∥−σ⊥) occurs in the plane normal to the passage of light [26]. Due to angular displacements, the shear stresses were not considered to limit the boundary conditions and obtain the desired equation. In analogy with photoelasticity, the stress-strain matrix for the holographic parameters is given by Eq 5 [17]:
[σ⊥σ∥]=Ea(1−ν2)[1νν1][aεH⊥aεH∥]⇒(σ∥−σ⊥)holographic=E(1+ν)ℵ | (5) |
considering, by Eq 6, that
E=aE | (6) |
E is defined as the holographic elasticity modulus, and a is a dimensionless constant that relates the holography elasticity with the mechanical elasticity. εH=1aε is defined as the relative holographic deformation and ℵ=(ϕ∥−ϕ⊥)holographic2π=(εH∥−εH⊥) as the relative holographic retardation. Thus, the holographic dispersion can be written as the Eq 7 [17]:
H(λ)≡λfσ=a(1+ν)eEλ | (7) |
where, by Eq 8,
![]() |
(8) |
and fσ are the fringe values obtained in holography and photoelasticity, respectively. The photoelastic fringe value is related to the wavelength of light (λ) and the photoelastic stress-optical coefficient (C). Then, analogously to what occurs with photoelasticity, there comes the holographic dispersion term, H(λ), an intrinsic property of the material whose value depends on the light wavelength, resultant from the relation between the component differences in refractive indexes, (n∥−n⊥)holographic and the plane stresses (σ∥−σ⊥)holographic. For a given wavelength, the stress-holographic law is given by Eq 9 [17]:
(n∥−n⊥)holographic=H(σ∥−σ⊥)holographic | (9) |
We have experimentally confirmed these equations in [21,22].
The off-axis configuration separates the diffraction orders during the digital reconstruction performed with FTM's fresnel transform method [17,32,33]. The image field (ψmn) and phase (ϕmn) were calculated using Eqs 10 and 11, and the corresponding maps were reconstructed.
ψmn=eikz∙eikzλ2(n2N2Δξ2+m2M2Δη2)izλ∙F[Δkξ,Δkη] | (10) |
ϕmn=arctg{Im[ψmn]Re[ψmn]} | (11) |
where F[Δkξ,Δkη]=F[IH(ξ,η)∙ψR(ξ,η)∙eπizλ[(n∙Δξ)2+(m∙Δη)2]] is the Fourier Transform of the discretized field, Δkξ=−kλNΔξ, Δkη=−kλMΔη, Δξ=zλNΔh, Δη=zλMΔv, Δh and Δv are the horizontal and vertical pixel dimensions, respectively. All the phase maps were demodulated with the Volkov Method [34].
The calibration of the setup followed the work of Colomb et al. [24] and was carried out using a quarter-wave plate as a sample. Two-phase maps reconstructed by FTM, one for each polarization, were subtracted to obtain the maps of phase differences in the function of the angle of orientation of the quarter-wave plate. The general expression for the phase difference (Δφ) as a function of the orientation of the quarter-wave, by Eq 12:
Δϕ=arctan[sin(2δ)cos2(2δ)] | (12) |
The process to obtain the demodulated phase maps for compression and decompression. The area selected (the rectangle on the hologram) of the hologram was processed with the FTM to obtain the frequency spectrum [17]. The chosen area (the rectangle on the frequency spectrum) of the frequency spectrum was obtained from the modulated phase map, and the Volkov method [34] was obtained from the demodulated phase map. The mean phase was determined from the phases of the pixels in the area selected from the demodulated phase map. To reduce the noise, in each phase map, a region of the phase map with no object was chosen, and the mean phase value of this region was subtracted from the phase map [17].
From the vertical phase maps of each stress applied, the load, σi, was calculated in the vertical holographic deformations, εHv−i, in both processes: compression and decompression. In the same way, with the phase values of the horizontal phase maps, the horizontal holographic deformations, εHh−i, were calculated. The mean value ⟨E⟩ was calculated from various values E by fitting the linear function, by Eq 13:
σi=⟨E⟩εHv−i | (13) |
The mean value of the Poisson's coefficient, ⟨ν⟩, was calculated from various values ν by fitting the linear function, by Eq 14:
εHh−i=⟨ν⟩εHv−i | (14) |
In both cases, the Least Squares Method [34] was utilized.
For each horizontal line j, the phase differences, (ϕv−ϕh)j, were obtained and, from the result, the relative retardation, ℵj=(ϕv−ϕh)j/2π, was calculated between the dark fringes. These results, associated with the holographic parameters ⟨E⟩ and⟨ν⟩, allowed us to find the stress differences (σv−σh)j−holographic using Eq 5. The graphic (σv−σh)j−holographic as a function of the number of pixels produced the distributions of stress differences in the selected region.
A dimensionless constant parameter from Eq 6 gives the relation between holographic and mechanical elasticity. According to Eq 4, using the photoelastic images, mechanical parameters, ⟨E⟩ and⟨ν⟩, and the thicknesses, e, it is possible to calculate the photoelastic fringes, fσ, and the photoelastic dispersions, C(λ). Using Eq 8, the holographic parameters, E and ν, the thickness e, and the constant a, the holographic fringes, , can be calculated using the phase maps. Using Eq 7 and the wavelength, λ, the holographic dispersion, H(λ), can be calculated.
The mechanical parameters were measured with samples sized 4.15, 2.25, and 1.03 cm, and the stress distribution was tested in samples sized 4.15, 2.25, and 4.90 cm, both in holography and photoelasticity.
Each technique was applied to only one block from each pair of standard blocks, which were prepared with identical flexibilities and labeled as −F low flexibility and +F high flexibility. Stresses were applied to the top surface of the sample blocks via a loading device, as illustrated in Figure 1.
The modified Mach-Zehnder interferometer apparatus was built with a He-Ne laser (632.8 nm CW, 20 mW, model 1135PUniphase). The photoelastic images and hologram registers were captured with a Thorlabs digital camera, model DCC1240C-HQ color, CMOS sensor, 1280 × 1024 pixels, size pixel 5.3 μm (square).
The calibration process of the holographic system generated an experimental distribution that, when compared to a theoretical curve, Figure 3, showed the reliability of the system [24].
The continuous line represents the theoretical curve, Eq 12, and the circles of the experimental distribution. The distribution of the point around the curve indicates that the adjustment of the polarization of waves is correct.
Two sets of holograms were recorded for each sample for compression and decompression. The stresses, applied to the upper central phase of the sample, ranged from 0.3 to 1.5 MPa. In holography, the mean phases were obtained from the statistics of ten selected areas in each demodulated phase map. The final mean was calculated using the values of compression and decompression.
The photoelasticity RGB method, in transmission mode, was associated with the finite elements method to determine the distributions of the stress differences in the same selected regions as those used with the holographic method. These results were used to evaluate the proposed method by comparing holography and photoelasticity by stress-optic and stress-holographic law, given by Eqs 1 and 9, respectively.
All the fitted functions used the least square method [27]. A third-degree polynomial function was fitted to the points, but all the results showed that they were linear functions.
Figure 4 presents the graphics of the experimental values of the σ versus εv for the +F sample under compression and decompression. This graphic is used to determine the mechanical and holographic modulus of elasticity.
Figure 5 presents the graphics of the experimental values of σ versus εHv for the +F samples under compression and decompression, and they are used to obtain the Holographic and Mechanical modulus of elasticity.
Similar graphs were made for the −F samples. Table 1 summarizes the holographic and mechanical modules of elasticity. The values are similar for the samples with different flexibilities in analysis. However, the rate E/E differed for each method (holographic and mechanical). Considering the uncertainties, the values of ⟨E/E⟩ are practically equal for all samples in both flexibilities.
−F sample (modulus of elasticity) | +F sample (modulus of elasticity) | |
Holography (MPa) | 1.589 ± 0.032 | 1.320 ± 0.024 |
Mechanical elasticity (10 MPa) | 3.35 ± 0.16 | 2.86 ± 0.11 |
⟨a⟩=⟨E/E⟩(10−2) | 4.75 ± 0.26 | 4.62 ± 0.20 |
It was verified, in all graphics, using polynomials up to the third degree, that the best-fit function was a first-degree polynomial, related function, agreeing with Robert Hooke's theory of elasticity for the elastic regime.
Figure 6 presents the values of the εh(transverse) versus εv (longitudinal), used to calculate the mechanical Poisson's coefficient under the compression and decompression processes, using samples +F.
Figure 7 presents the values of the εHh(transverse)versus εHv(longitudinal) used to determine the holographic Poisson's coefficient under the compression and decompression processes, using samples +F to determine the stress distribution.
Similar graphs were made for the −F samples. Table 2 summarizes the Poisson's coefficients: holographic and mechanical. Considering the uncertainties, the Poisson's coefficients ν are practically equal for the sample with the same flexibility.
−F sample [Poisson's coefficient (10−1)] | +F sample [Poisson's coefficient (10−1)] | |
Holography | 3.723 ± 0.022 | 3.735 ± 0.025 |
Mechanical elasticity | 3.822 ± 0.095 | 3.90 ± 0.11 |
With these parameters, it was possible to determine the mean stress-optical coefficients in photoelasticity and holography, whose values are presented in Table 3.
−F sample [stress-optical coefficient (10−12 m2/N)] |
+F sample [stress-optical coefficient (10−12 m2/N)] |
|
Holography | 3.921 ± 0.08 | 4.442 ± 0.09 |
Mechanical elasticity | 3.95 ± 0.21 | 4.50 ± 0.22 |
The difference between the values of the samples with the same flexibility is due to the dependence of the stress-optical coefficient with sample thickness, e. However, for the same flexibility, the values agree both in holography and in photoelasticity. An important observation is that in holography, the precision of the results is better since the values are of the order of 10−6 N/m2.
The graphics in Figure 8 show the distribution of stress difference over the vertical lines between two dark fringes obtained using the holographic method (), photoelasticity RGB (+), and analytical method (
). The load, in mass, applied was 600 g for all samples.
The behavior of curves in graphics exhibits similarities, indicating that the theoretical model of the proposed method is correct. The +F samples are less rigid and suffer more significant vertical deformation, reducing the modulus of elasticity under the same load. This behavior is observed graphically due to the smaller and more scattered peaks about the −F sample distribution.
In photoelasticity, the intensity of the images of the fringes pattern depends on the characteristics of the experimental configuration: transmittance of the materials, anisotropic behavior level, spectral radiation distribution of the light source (white light), the conversion factor of the light-signal control and electric-signal control, and the temporary birefringence effect. In digital holography, these effects are less relevant due to the subtractions performed in the numerical reconstruction process, which eliminate much of this noise, and also due to the optical retardations obtained directly from the phase differences maps.
We present an alternative method to obtain the stress differences in photoelastic materials, as verified by the experiments. The similar results for the modulus of elasticity obtained through the different methods (photoelasticity, mechanical, and holographical) allowed the assumption of an analogy between Hooke's law and holography. The photoelastic and holographic dispersions are equal, allowing the establishment of the stress-holographic law in analogy with the stress-optic law. The experimental stress distributions in holography presented the same behavior as the analytic and photoelastic distributions. The results of stress distributions in holography were more accurate than in photoelasticity when compared to the theoretical results. Thus, the proposed method presents efficiency and independence in procedures since it uses only the extracted parameters obtained directly from the phase maps.
The authors declare they have not used Artificial Intelligence (AI) tools in the creation of this article.
The authors are grateful for the financial support from Fundação de Amparo à Pesquisa do Estado de São Paulo (2012/18162-4, 2019/23700-4, 2022/15276-0); the physical support from Faculdade de Tecnologia de Pompeia (Fatec Pompeia), Faculdade de Tecnologia de Itaquera (Fatec Itaquera), Instituto de Física da Universidade de São Paulo (IFUSP) and Instituto de Pesquisas Energéticas e Nucleares de São Paulo (IPEN-SP).
The lead author (Sidney Leal da Silva) contributed theory, data, and analysis from his doctoral work [17]. The other co-authors helped with the preparation and first revisions of the article.
The authors declare no conflict of interest.
[1] |
B. R. Lee, S. J. Sung, K. H. Hur, S. E. Kim, S. X. Ma, S. K. Kim, et al., Korean Red Ginseng inhibits methamphetamine addictive behaviors by regulating dopaminergic and NMDAergic system in rodents, J. Ginseng Res., 46 (2022), 147–155. https://doi.org/10.1016/j.jgr.2021.05.007 doi: 10.1016/j.jgr.2021.05.007
![]() |
[2] |
D. M. Stoneberg, R. K. Shukla, M. B. Magness, Global methamphetamine trends: an evolving problem, Int. Crim. Justice Rev., 28 (2018), 136–161. https://doi.org/10.1177/1057567717730104 doi: 10.1177/1057567717730104
![]() |
[3] |
L. Xu, L. Li, Q. Chen, Y. Huang, X. Chen, D. Qiao, The role of non-coding RNAs in methamphetamine-induced neurotoxicity, Cell. Mol. Neurobiol., 2023 (2023), 1–22. https://doi.org/10.1007/s10571-023-01323-x doi: 10.1007/s10571-023-01323-x
![]() |
[4] |
C. J. Kuo, Y. T. Liao, W. J. Chen, S. Y. Tsai, S. K. Lin, C. C. Chen, Causes of death of patients with methamphetamine dependence: a record‐linkage study, Drug Alcohol. Rev., 30 (2011), 621–628. https://doi.org/10.1111/j.1465-3362.2010.00255.x doi: 10.1111/j.1465-3362.2010.00255.x
![]() |
[5] | World Drug Report 2022, UN Office on Drugs and Crime, 2022. Available from: https://www.unodc.org/unodc/data-and-analysis/world-drug-report-2022.html. |
[6] |
P. Jiang, J. Y. Sun, X. B. Zhou, L. Lu, L. Li, X. Q. Huang, et al., Functional connectivity abnormalities underlying mood disturbances in male abstinent methamphetamine abusers, Hum. Brain Mapp., 42 (2021), 3366–3378. https://doi.org/10.1002/hbm.25439 doi: 10.1002/hbm.25439
![]() |
[7] |
P. J. McCarty, A. R. Pines, B. L. Sussman, S. N. Wyckoff, A. Jensen, R. Bunch, et al., Resting state functional magnetic resonance imaging elucidates neurotransmitter deficiency in autism spectrum disorder, J. Pers. Med., 11 (2021), https://doi.org/10.3390/jpm11100969 doi: 10.3390/jpm11100969
![]() |
[8] |
Y. Y. Du, W. H. Yang, J. Zhang, J. Liu, Changes in ALFF and ReHo values in methamphetamine abstinent individuals based on the Harvard-Oxford atlas: A longitudinal resting-state fMRI study, Addict. Biol., 27 (2022), e13080. https://doi.org/10.1111/adb.13080 doi: 10.1111/adb.13080
![]() |
[9] |
M. Q. Gong, Y. X. Shen, W. B. Liang, Z. Zhang, C. X. He, M. W. Lou, et al., Impairments in the default mode and executive networks in methamphetamine usersduring short-term abstinence, Int. J. Gen. Med., 15 (2022), 6073–6084. https://doi.org/10.2147/ijgm.S369571 doi: 10.2147/ijgm.S369571
![]() |
[10] |
O. Sporns, The non-random brain: efficiency, economy, and complex dynamics, Front. Comput. Neurosci., 5 (2011), 5. https://doi.org/10.3389/fncom.2011.00005 doi: 10.3389/fncom.2011.00005
![]() |
[11] |
W. Liao, G. R. Wu, Q. Xu, G. J. Ji, Z. Q. Zhang, Y. F. Zang, et al., DynamicBC: a MATLAB toolbox for dynamic brain connectome analysis, Brain Connect., 4 (2014), 780–790. https://doi.org/10.1089/brain.2014.0253 doi: 10.1089/brain.2014.0253
![]() |
[12] |
Q. Cui, W. Sheng, Y. Y. Chen, Y. J. Pang, F. M. Lu, Q. Tang, et al., Dynamic changes of amplitude of low-frequency fluctuations in patients with generalized anxiety disorder, Hum. Brain Mapp., 41 (2020), 1667–1676. https://doi.org/10.1002/hbm.24902 doi: 10.1002/hbm.24902
![]() |
[13] |
J. Li, X. J. Duan, Q. Cui, H. F. Chen, W. Liao, More than just statics: temporal dynamics of intrinsic brain activity predicts the suicidal ideation in depressed patients, Psychol. Med., 49 (2019), 852–860. https://doi.org/10.1017/s0033291718001502 doi: 10.1017/s0033291718001502
![]() |
[14] |
Z. Fu, A. Iraji, J. A. Turner, J. Sui, R. Miller, G. D. Pearlson, et al., Dynamic state with covarying brain activity-connectivity: On the pathophysiology of schizophrenia, Neuroimage, 224 (2021), 117385. https://doi.org/10.1016/j.neuroimage.2020.117385 doi: 10.1016/j.neuroimage.2020.117385
![]() |
[15] |
C. G. Yan, X. D. Wang, X. N. Zuo, Y. F. Zang, DPABI: data processing & analysis for (resting-state) brain imaging, Neuroinformatics, 14 (2016), 339–351. https://doi.org/10.1007/s12021-016-9299-4 doi: 10.1007/s12021-016-9299-4
![]() |
[16] |
J. J. Wang, X. Chen, S. K. Sah, C. Zeng, Y. M. Li, N. Li, et al., Amplitude of low-frequency fluctuation (ALFF) and fractional ALFF in migraine patients: a resting-state functional MRI study, Clin. Radiol., 71 (2016), 558–564. https://doi.org/10.1016/j.crad.2016.03.004 doi: 10.1016/j.crad.2016.03.004
![]() |
[17] |
H. Yuan, X. H. Yu, X. Li, S. J. Qin, G. X. Liang, T. Y. Bai, et al., Research on resting spontaneous brain activity and functional connectivity of acupuncture at uterine acupoints, Digital Chin. Med., 5 (2022), 59–67. https://doi.org/10.1016/j.dcmed.2022.03.006 doi: 10.1016/j.dcmed.2022.03.006
![]() |
[18] |
R. Li, W. Liao, Y. Y. Yu, H. Chen, X. N. Guo, Y. L. Tang, et al., Differential patterns of dynamic functional connectivity variability of striato-cortical circuitry in children with benign epilepsy with centrotemporal spikes, Hum. Brain Mapp., 39 (2018), 1207–1217. https://doi.org/10.1002/hbm.23910 doi: 10.1002/hbm.23910
![]() |
[19] |
Q. Li, X. H. Cao, S. Liu, Z. X. Li, Y. F. Wang, L. Cheng, et al., Dynamic alterations of amplitude of low-frequency fluctuations in patients with drug-naive first-episode early onset schizophrenia, Front. Neurosci., 14 (2020), 901. https://doi.org/10.3389/fnins.2020.00901 doi: 10.3389/fnins.2020.00901
![]() |
[20] |
W. Liao, J. Li, G. J. Ji, G. R. Wu, Z. Long, Q. Xu, et al., Endless fluctuations: temporal dynamics of the amplitude of low frequency fluctuations, IEEE Trans. Med. Imaging, 38 (2019), 2523–2532. https://doi.org/10.1109/TMI.2019.2904555 doi: 10.1109/TMI.2019.2904555
![]() |
[21] | A. Ardila, Executive functions brain functional system, in Dysexecutive syndromes: Clinical and experimental perspectives, Springer Press, (2019), 29–41. https://doi.org/10.1007/978-3-030-25077-5_2 |
[22] |
M. Gong, W. Liang, C. He, Y. Shen, Z. Zhang, M. Lou, et al., Neuroimaging mechanisms in short-term heroin-and methamphetamine-abstinent users: Similarities and differences, Neurosci. Lett., 796 (2023), 137057. https://doi.org/10.1016/j.neulet.2023.137057 doi: 10.1016/j.neulet.2023.137057
![]() |
[23] | J. G. Scott, M. R. Schoenberg, Frontal lobe/executive functioning, in The little black book of neuropsychology: A syndrome-based approach, Springer Press, (2010) 219–248. https://doi.org/10.1007/978-0-387-76978-3_10 |
[24] |
W. Sato, M. Toichi, S. Uono, T. Kochiyama, Impaired social brain network for processing dynamic facial expressions in autism spectrum disorders, BMC Neurosci., 13 (2012), 1–17. https://doi.org/10.1186/1471-2202-13-99 doi: 10.1186/1471-2202-13-99
![]() |
[25] |
W. Xie, J. I. Chapeton, S. Bhasin, C. Zawora, J. H. Wittig Jr, S. K. Inati, et al., The medial temporal lobe supports the quality of visual short-term memory representation, Nat. Hum. Behav., 7 (2023), 627–641. https://doi.org/10.1038/s41562-023-01529-5 doi: 10.1038/s41562-023-01529-5
![]() |
[26] |
A. Avena-Koenigsberger, B. Misic, O. Sporns, Communication dynamics in complex brain networks, Nat. Rev. Neurosci., 19 (2018), 17–33. https://doi.org/10.1038/nrn.2017.149 doi: 10.1038/nrn.2017.149
![]() |
[27] |
Y. Li, L. Liu, E. Wang, H. Zhang, S. Dou, L. Tong, et al., Abnormal neural network of primary insomnia: evidence from spatial working memory task fMRI, Eur. Neurol., 75 (2016), 48–57. https://doi.org/10.1159/000443372 doi: 10.1159/000443372
![]() |
[28] |
T. P. Zanto, A. Gazzaley, Fronto-parietal network: flexible hub of cognitive control, Trends Cognit. Sci., 17 (2013), 602–603. https://doi.org/10.1016/j.tics.2013.10.001 doi: 10.1016/j.tics.2013.10.001
![]() |
[29] |
D. Vuletic, P. Dupont, F. Robertson, J. Warwick, J. R. Zeevaart, D. J. Stein, Methamphetamine dependence with and without psychotic symptoms: A multi-modal brain imaging study, Neuroimage Clin., 20 (2018), 1157–1162. https://doi.org/10.1016/j.nicl.2018.10.023 doi: 10.1016/j.nicl.2018.10.023
![]() |
[30] |
U. Wolf, M. J. Rapoport, T. A. Schweizer, Evaluating the affective component of the cerebellar cognitive affective syndrome, J. Neuropsychiatry Clin. Neurosci., 21 (2009), 245–253. https://doi.org/10.1176/jnp.2009.21.3.245 doi: 10.1176/jnp.2009.21.3.245
![]() |
[31] |
E. A. Moulton, I. Elman, L. R. Becerra, R. Z. Goldstein, D. Borsook, The cerebellum and addiction: insights gained from neuroimaging research, Addict. Biol., 19 (2014), 317–331. https://doi.org/10.1111/adb.12101 doi: 10.1111/adb.12101
![]() |
[32] |
X. T. Li, H. Su, N. Zhong, T. Z. Chen, J. Du, K. Xiao, et al., Aberrant resting-state cerebellar-cerebral functional connectivity in methamphetamine-dependent individuals after six months abstinence, Front. Psychiatry, 11 (2020), 191. https://doi.org/10.3389/fpsyt.2020.00191 doi: 10.3389/fpsyt.2020.00191
![]() |
[33] |
M. Malina, S. Keedy, J. Weafer, K. Van Hedger, H. de Wit, Effects of methamphetamine on within-and between-network connectivity in healthy adults, Cereb. Cortex, 2 (2021), tgab063. https://doi.org/10.1093/texcom/tgab063 doi: 10.1093/texcom/tgab063
![]() |
[34] | A. Sathyanesan, J. Zhou, J. Scafidi, D. H. Heck, R. V. Sillitoe, V. Gallo, Emerging connections between cerebellar development, behaviour and complex brain disorders, Nat. Rev. Neurosci., 20 (2019), 298–313. https://doi.org/10.1038/s41583-019-0152-2 |
[35] | E. A. Evans, C. E. Grella, D. M. Upchurch, Gender differences in the effects of childhood adversity on alcohol, drug, and polysubstance-related disorders, Social Psychiatry Psychiatr. Epidemiol., 52 (2017), 901–912. https://doi.org/10.1007/s00127-017-1355-3 |
[36] |
K. Ratheesh, L. Seah, V. Murukeshan, Spectral phase-based automatic calibration scheme for swept source-based optical coherence tomography systems, Phys. Med. Biol., 61 (2016), 7652. https://doi.org/10.1088/0031-9155/61/21/7652 doi: 10.1088/0031-9155/61/21/7652
![]() |
[37] |
R. K. Meleppat, K. E. Ronning, S. J. Karlen, M. E. Burns, E. N. Pugh, R. J. Zawadzki, In vivo multimodal retinal imaging of disease-related pigmentary changes in retinal pigment epithelium, Sci. Rep., 11 (2021), 1–14. https://doi.org/10.1038/s41598-021-95320-z doi: 10.1038/s41598-021-95320-z
![]() |
[38] |
R. K. Meleppat, C. R. Fortenbach, Y. Jian, E. S. Martinez, K. Wagner, B. S. Modjtahedi, et al., In vivo imaging of retinal and choroidal morphology and vascular plexuses of vertebrates using swept-source optical coherence tomography, Transl. Vision Sci. Technol., 11 (2022), 11. https://doi.org/10.1167/tvst.11.8.11 doi: 10.1167/tvst.11.8.11
![]() |
[39] |
R. K. Meleppat, C. Shearwood, S. L. Keey, M. V. Matham, Quantitative optical coherence microscopy for the in situ investigation of the biofilm, J. Biomed. Opt., 21 (2016), 127002–127002. http://dx.doi.org/10.1117/1.JBO.21.12.127002 doi: 10.1117/1.JBO.21.12.127002
![]() |
[40] | W. Abada, A. Bouramoul, Using machinelearning techniques to predict people at-risk for drug addiction: A Bayesian-Based Model, in 2022 4th International Conference on Pattern Analysis and Intelligent Systems (PAIS), IEEE, (2022), 1–7. https://doi.org/10.1109/PAIS56586.2022.9946914 |
[41] |
H. Chen, C. Li, G. Wang, X. Li, M. M. Rahaman, H. Sun, et al., GasHis-Transformer: A multi-scale visual transformer approach for gastric histopathological image detection, Pattern Recognit., 130 (2022), 108827. https://doi.org/10.1016/j.patcog.2022.108827 doi: 10.1016/j.patcog.2022.108827
![]() |
1. | Francisco J. G. Silva, Rita C. M. Sales-Contini, Advances in laser materials and processing technologies: An overview, 2025, 12, 2372-0484, 23, 10.3934/matersci.2025002 |
−F sample (modulus of elasticity) | +F sample (modulus of elasticity) | |
Holography (MPa) | 1.589 ± 0.032 | 1.320 ± 0.024 |
Mechanical elasticity (10 MPa) | 3.35 ± 0.16 | 2.86 ± 0.11 |
⟨a⟩=⟨E/E⟩(10−2) | 4.75 ± 0.26 | 4.62 ± 0.20 |
−F sample [Poisson's coefficient (10−1)] | +F sample [Poisson's coefficient (10−1)] | |
Holography | 3.723 ± 0.022 | 3.735 ± 0.025 |
Mechanical elasticity | 3.822 ± 0.095 | 3.90 ± 0.11 |
−F sample [stress-optical coefficient (10−12 m2/N)] |
+F sample [stress-optical coefficient (10−12 m2/N)] |
|
Holography | 3.921 ± 0.08 | 4.442 ± 0.09 |
Mechanical elasticity | 3.95 ± 0.21 | 4.50 ± 0.22 |
−F sample (modulus of elasticity) | +F sample (modulus of elasticity) | |
Holography (MPa) | 1.589 ± 0.032 | 1.320 ± 0.024 |
Mechanical elasticity (10 MPa) | 3.35 ± 0.16 | 2.86 ± 0.11 |
⟨a⟩=⟨E/E⟩(10−2) | 4.75 ± 0.26 | 4.62 ± 0.20 |
−F sample [Poisson's coefficient (10−1)] | +F sample [Poisson's coefficient (10−1)] | |
Holography | 3.723 ± 0.022 | 3.735 ± 0.025 |
Mechanical elasticity | 3.822 ± 0.095 | 3.90 ± 0.11 |
−F sample [stress-optical coefficient (10−12 m2/N)] |
+F sample [stress-optical coefficient (10−12 m2/N)] |
|
Holography | 3.921 ± 0.08 | 4.442 ± 0.09 |
Mechanical elasticity | 3.95 ± 0.21 | 4.50 ± 0.22 |