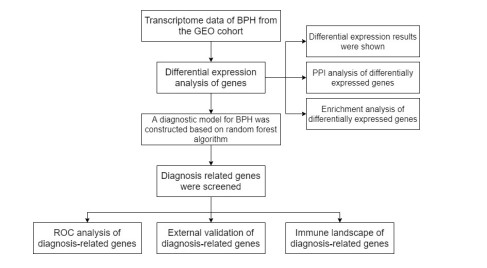
In older adults, benign prostatic hyperplasia (BPH) is the most common cause of lower urinary tract symptoms (LUTS). This study aimed to explore the genes with diagnostic value in patients with BPH, reveal the relationship between the expression of diagnosis-related genes and the immune microenvironment, and provide a reference for molecular diagnosis and immunotherapy of BPH. The combined gene expression data of GSE6099, GSE7307 and GSE119195 in the GEO database were used. The differential expression of autophagy-related genes between BPH patients and healthy controls was obtained by differential analysis. Then the genes related to BPH diagnosis were screened by a machine learning algorithm and verified. Finally, five important genes (IGF1, PSIP1, SLC1A3, SLC2A1 and T1A1) were obtained by random forest (RF) algorithm, and their relationships with the immune microenvironment were discussed. Five genes play an essential role in the occurrence and development of BPH and may become new diagnostic markers of BPH. Among them, immune cells have significant correlation with some genes. The signal transduction of IL-4 mediated by M2 macrophages is closely related to the progress of BPH. There are abundant active mast cells in BPH. The adoption and metastasis of regulatory T cells may be an important method to treat BPH.
Citation: Aiying Ying, Yueguang Zhao, Xiang Hu. Identification of biomarkers related to prostatic hyperplasia based on bioinformatics and machine learning[J]. Mathematical Biosciences and Engineering, 2023, 20(7): 12024-12038. doi: 10.3934/mbe.2023534
[1] | Ahmed Hammad, Mohamed Elshaer, Xiuwen Tang . Identification of potential biomarkers with colorectal cancer based on bioinformatics analysis and machine learning. Mathematical Biosciences and Engineering, 2021, 18(6): 8997-9015. doi: 10.3934/mbe.2021443 |
[2] | Fang Niu, Zongwei Liu, Peidong Liu, Hongrui Pan, Jiaxue Bi, Peng Li, Guangze Luo, Yonghui Chen, Xiaoxing Zhang, Xiangchen Dai . Identification of novel genetic biomarkers and treatment targets for arteriosclerosis-related abdominal aortic aneurysm using bioinformatic tools. Mathematical Biosciences and Engineering, 2021, 18(6): 9761-9774. doi: 10.3934/mbe.2021478 |
[3] | Yuedan Wang, Jinke Huang, Jiaqi Zhang, Fengyun Wang, Xudong Tang . Identifying biomarkers associated with the diagnosis of ulcerative colitis via bioinformatics and machine learning. Mathematical Biosciences and Engineering, 2023, 20(6): 10741-10756. doi: 10.3934/mbe.2023476 |
[4] | Sufang Wu, Hua He, Jingjing Huang, Shiyao Jiang, Xiyun Deng, Jun Huang, Yuanbing Chen, Yiqun Jiang . FMR1 is identified as an immune-related novel prognostic biomarker for renal clear cell carcinoma: A bioinformatics analysis of TAZ/YAP. Mathematical Biosciences and Engineering, 2022, 19(9): 9295-9320. doi: 10.3934/mbe.2022432 |
[5] | Jie Wang, Md. Nazim Uddin, Rehana Akter, Yun Wu . Contribution of endothelial cell-derived transcriptomes to the colon cancer based on bioinformatics analysis. Mathematical Biosciences and Engineering, 2021, 18(6): 7280-7300. doi: 10.3934/mbe.2021360 |
[6] | Kewei Ni, Gaozhong Sun . The identification of key biomarkers in patients with lung adenocarcinoma based on bioinformatics. Mathematical Biosciences and Engineering, 2019, 16(6): 7671-7687. doi: 10.3934/mbe.2019384 |
[7] | Gaozhong Sun, Tongwei Zhao . Lung adenocarcinoma pathology stages related gene identification. Mathematical Biosciences and Engineering, 2020, 17(1): 737-746. doi: 10.3934/mbe.2020038 |
[8] | Li-Sha Pan, Zacharia Ackbarkha, Jing Zeng, Min-Li Huang, Zhen Yang, Hao Liang . Immune marker signature helps to predict survival in uveal melanoma. Mathematical Biosciences and Engineering, 2021, 18(4): 4055-4070. doi: 10.3934/mbe.2021203 |
[9] | Jing Cao, Hui Gan, Han Xiao, Hui Chen, Dan Jian, Ning Jiang, Xuan Zhai . Key protein-coding genes related to microglia in immune regulation and inflammatory response induced by epilepsy. Mathematical Biosciences and Engineering, 2021, 18(6): 9563-9578. doi: 10.3934/mbe.2021469 |
[10] | Peng Gu, Dongrong Yang, Jin Zhu, Minhao Zhang, Xiaoliang He . Bioinformatics analysis identified hub genes in prostate cancer tumorigenesis and metastasis. Mathematical Biosciences and Engineering, 2021, 18(4): 3180-3196. doi: 10.3934/mbe.2021158 |
In older adults, benign prostatic hyperplasia (BPH) is the most common cause of lower urinary tract symptoms (LUTS). This study aimed to explore the genes with diagnostic value in patients with BPH, reveal the relationship between the expression of diagnosis-related genes and the immune microenvironment, and provide a reference for molecular diagnosis and immunotherapy of BPH. The combined gene expression data of GSE6099, GSE7307 and GSE119195 in the GEO database were used. The differential expression of autophagy-related genes between BPH patients and healthy controls was obtained by differential analysis. Then the genes related to BPH diagnosis were screened by a machine learning algorithm and verified. Finally, five important genes (IGF1, PSIP1, SLC1A3, SLC2A1 and T1A1) were obtained by random forest (RF) algorithm, and their relationships with the immune microenvironment were discussed. Five genes play an essential role in the occurrence and development of BPH and may become new diagnostic markers of BPH. Among them, immune cells have significant correlation with some genes. The signal transduction of IL-4 mediated by M2 macrophages is closely related to the progress of BPH. There are abundant active mast cells in BPH. The adoption and metastasis of regulatory T cells may be an important method to treat BPH.
BPH is a common disease in the elderly male population. The incidence of BPH increases with age, and the LUTS caused by BPH will seriously affect patients' quality of life [1]. It is reported that almost 90% of men aged 81–90 suffer from BPH [2]. Autophagy delivers cytoplasmic components to lysosomes through autophagosomes, which is not only a necessary process to realize the metabolic needs of cells and the renewal of some organelles but also plays an important role in homeostasis. Existing studies explored the role of autophagy-related genes in tumors [3] and neurological diseases [4]. However, the role of autophagy-related genes in BPH is still unclear.
In older adults, BPH is the most common cause of LUTS. This study aimed to explore the genes with diagnostic value in patients with BPH, reveal the relationship between the expression of diagnosis-related genes and the immune microenvironment, and provide a reference for molecular diagnosis and immunotherapy of BPH. The combined gene expression data of GSE6099, GSE7307 and GSE119195 in the GEO database were used. The differential expression of autophagy-related genes between BPH patients and healthy controls was obtained by differential analysis. Then the genes related to BPH diagnosis were screened by a machine learning algorithm and verified. Finally, five important genes (IGF1, PSIP1, SLC1A3, SLC2A1 and T1A1) were obtained by RF algorithm, and their relationships with the immune microenvironment were discussed. Five genes play an essential role in the occurrence and development of BPH and may become new diagnostic markers of BPH. Among them, immune cells have significant correlation with some genes. The signal transduction of IL-4 mediated by M2 macrophages is closely related to the progress of BPH. There are abundant active mast cells in BPH. The adoption and metastasis of regulatory T cells may be an important method to treat BPH.
Bioinformatics analysis can analyze biomarkers closely related to diseases from the perspective of omics data and provide a reference for targeted treatment, clinical diagnosis and prognosis of diseases. According to the enrichment analysis of BPH and protein-protein interaction (PPI) network, Wang found five key genes, UBE2C, AKT1, MAPK1, CCNB1 and PLK1, may play a vital role in the pathogenesis of BPH. The study also confirmed that the local adhesion pathway, FoxO signaling pathway and autophagy pathway may be the key to BPH progress [5]. Xiang et al. [6] research suggest that there may be differentially expressed genes such as ARG2 and LUM in epithelial cells and stromal cells, which might provide a new understanding of the pathogenesis of BPH. Xu et al. [7] analyzed the transcriptome data of BPH by weighted gene co-expression network analysis (WGCNA) and found that SOCS3, IL6, C3, IGF1, NOTCH1 and VCAN are critical regulators of BPH immunophenotype. However, the role of autophagy-related genes in diagnosing BPH and its relationship with the immune microenvironment is still unclear. Therefore, this paper selects the combined data of GSE6099, GSE7307 and GSE119195 in the GEO database and analyzes the differential expression of 610 autophagy-related genes in these data sets to obtain differentially expressed autophagy-related genes (DEAGS). Then, this paper makes a variety of bioinformatics analyses and uses a machine learning algorithm to screen five essential genes (IGF1, PSIP1, SLC1A3, SLC2A1, T1A1) related to BPH diagnosis. Finally, the correlation between this gene and immune cells was discussed. Sachdeva et al. [8] performed PPI network analysis of differentially expressed genes in BPH and identified 38 hub genes. These genes may serve as drug targets for BPH. Because prostate-specific antigen (PSA) is difficult to distinguish BPH patients from prostate cancer (PCa) patients, Ge et al. [9] identified genes that are significantly differentially expressed in the two diseases by comprehensive analysis of miRNA microarrays in BPH and PCa and validated them using qRT-PCR. Their findings suggest that plasma let-7f-5p combined with PSA can be a potential diagnostic biomarker for PCa.
The data used in this paper are all from the GEO database (https://www.ncbi.nlm.nih.gov/gds). Among them, the GSE119195 data set includes five prostate samples from BPH surgical resection and three from brain-dead young people's donation, and its data platform is GPL6244 (Affymetrix Human Gene 1.0 ST Array). GSE6099 data set includes 21 BPH samples and four control samples, and its data platform is GPL6244 Affymetrix human gene 1.0 ST array. GSE119195 data set includes 5 BPH samples and three control samples, and its data platform is GPL2013 (Chinnaiyan Human 20K Hs6). In addition, 1185 autophagy-related genes were collected from previous literature. After merging the three data sets, 610 autophagy-related genes were obtained. GSE132714 data set includes 18 BPH samples and four control samples, and its data platform is GPL16791 (Illumina HiSeq 2500). We downloaded 222 and 366 autophagy related genes from HADb database (http://www.autophagy.lu/) and HAMdb database (http://hamdb.scbdd.com/home/index/) respectively. From GeneCards (https://www.genecards.org/), we extracted the first 1000 genes related to autophagy. Through the intersection of the above autophagy related genes, 1183 autophagy related genes were finally reserved for subsequent analysis.
The limma algorithm was used to analyze the differential expression of the merged data sets to identify the differentially expressed autophagy genes between BPH patients and healthy controls. The R package "limma" was used for data standardization and difference analysis. The DEGs with p values less than 0.05 were retained.
To explore the pathway information involved in DEGs, this paper introduced three items in gene ontology (GO) enrichment analysis [10]: biological process (BP), cellular component (CC) and molecular function (MF). In addition, the Kyoto Encyclopedia of Genes and Genomes (KEGG) enrichment analysis [11] was introduced to determine the biological function of DEGs. The R package "clusterProfiler" was used to perform GO analysis and KEGG analysis. Pathways with p values less than 0.05 were considered to be significantly enriched.
To explore the interaction of DEGs at the protein level, this paper uses the STRING database (https://cn.string-db.org/) to build a PPI network and sets the highest confidence interactive score to 0.9. Then download the PPI network and visualize it using Cytoscape software (https://cytoscape.org/).
First, we divide the overall dataset into training and test sets. The training set had 19 samples, including 8 BPH samples and 11 control samples. The test set had nine samples, including four BPH samples and five control samples. The RF in the scikit-learn package of python was used to sort the feature weights, and the logistic regression (LR) algorithm was used to build the diagnosis model. We implemented the above two algorithms based on the scikit-learn package. Specifically, we first set the random seed to 12345 for both algorithms. For the RF algorithm, we set the range of n_estimators from 100 to 1000, and the range of n_estimators is gini and entropy. We applied 10-fold cross-validation to the parameter selection and screening training set to obtain hub genes. Next, we classified BPH based on hub genes and used a LR algorithm. The range of the parameter 'C' of the LR algorithm is set from 0.1 to 3.1. We still use ten-fold cross validation on the training set to select the best parameters. The final prediction result is then obtained on the test set.
ROC curve was drawn by R package "pROC", which is used to evaluate the diagnostic model and the prediction accuracy of diagnosis related genes. In addition, wilcox-test was used to analyze the expression difference of diagnosis related genes between the two groups. Based on the genes in the diagnostic model, we used the "rms" R package to construct a nomogram. The calibration curve was used to evaluate the accuracy of the nomogram.
To explore the relationship between the five diagnosis-related genes and the expression of immune cells, the CIBERSORT algorithm was used to calculate the proportion of different immune cell types [12] and the infiltration abundance of 22 kinds of immune cells in a significant sample (p < 0.05). Finally, the Spearman correlation coefficient was used to calculate the correlation between five diagnostic genes and 22 kinds of immune cells.
First, we presented the overall flow diagram of this paper in Figure 1. In this paper, 125 DEGs were obtained by differential analysis of 610 autophagy genes in the merged data set. Figure 2A shows the volcano map obtained by difference analysis, in which green dots represent significantly down-regulated genes and red dots represent up-regulated genes considerably. The expression heat maps of these DEGs in all samples are given in Figure 2B. In addition, PPI networks of these genes were drawn. As can be seen from Figure 2C, there are many interactions among these genes. We performed PPI analysis on 125 differentially expressed autophagy related genes, and retained genes with interaction scores higher than 0.9. A total of 73 autophagy related genes with interaction scores higher than 0.9 were obtained and mapped in Figure 2C.
To explore the signal pathway of DEGs' participation, the BP (Figure 3A), CC (Figure 3B) and MF (Figure 3C) entries in GO enrichment analysis and KEGG enrichment analysis (Figure 3D) were carried out in this study. The discussion section explored these pathways' biological significance in detail.
To determine the DEGs closely related to diagnosis, this paper sorted the features of DEGs based on the RF algorithm and obtained the diagnosis-related genes. We retained the top five most significant genes and visualized their weights (Figure 4A). Then, based on these five genes, the LR algorithm obtained the diagnosis model and the ROC curve of Figure 4B was drawn (AUC = 0.8056). In addition, this paper verified it on the data (training set) after merging three data sets. As can be seen from Figure 5A–E, the AUC of all five genes is more significant than 0.5. Five genes were also verified in the GSE132714 data set (verification set) (Figure 6A–E), and the AUC of all five genes was more significant than 0.5. In addition, we present the results of the difference analysis using the t test in Figure S1 in the supplementary material. According to the difference in expression levels of five genes in the validation set between the BPH group and control group (Figure 6F–J). Among them, there was a significant difference in the expression of IGF1 between the two groups. In order to further explore the clinical value of these five diagnosis-related genes (IGF1, PSIP1, SLC1A3, SLC2A1 and TIA1), we built a nomograph model based on these five diagnosis-related genes (Figure 7A). The calibration curve shows that the nomograph model based on five diagnosis-related genes is more consistent with the ideal model (Figure 7B).
To explore the immune landscape of five genes related to BPH diagnosis, the CIBERSORT algorithm was used to calculate the samples with significant differences in immune abundance, the proportion of 22 kinds of immune cells was visualized (Figure 8A), and the correlation of 22 types of immune cells was analyzed (Figure 8B). In addition, the samples were divided into high-risk and low-risk groups according to the expression levels of five genes. The differences in infiltration abundance between high-risk and low-risk groups in 22 kinds of immune cells were visualized (Figure 8C–G). In addition, the scatter plot of immune cells with significant differences in five genes was drawn (Figure 9A–F). Among them, IGF1 and Macrophages M2 have the strongest correlation. In addition, Pearson test was used to analyze the correlation between diagnostic genes and immune cells. Related scatter plots are shown in Figure S2 in the supplementary material.
BPH is a disease that often occurs in the elderly male population and causes LUTS. The role of autophagy-related genes in the occurrence and development of BPH is still unclear. This paper aimed to mine BPH-related pathways and genes related to diagnosis by using a machine-learning algorithm from the perspective of bioinformatics. Specifically, this paper first obtained three GEO data sets of BPH. DEGs were obtained through the differential expression analysis of the merged data sets. We carried out various enrichment analyses of DEGs, most of which have been proven closely related to the occurrence and development of BPH. In Figure 3, for the BP pathway, Wang et al. [13] clarified that PI3K/AKT/mTOR signal nodes play an essential role in the occurrence and progression of prostate cancer(PCa)through the model of genetically engineered mice. Wang et al. divided the mice into a control group, obesity group (OB) and obesity group plus exercise group (OB+E), and took the aerobic exercise experiment together, and found that the PI3K-Akt signaling pathway was down-regulated to relieve BPH of obese mice [14]. Human papillomavirus infection is sexually transmitted virus, which may be related to the formation of prostate inflammation, leading to BPH and PCa [15]. Literature [16] showed that drugs could cause atrophy and Apoptosis of prostatic secretion cavity cells, reduce prostate volume, improve BPH and relieve LUTS. In the rat model of BPH, Li et al. [17] found that SIRT3 can participate in mitochondrial metabolic recoding through the AMPK signaling pathway, which can significantly alleviate the symptoms of BPH. FoxO signaling and autophagy pathways may be crucial to the progress of BPH. Liu et al. [18] confirmed through experiments that silencing the Beclin-1 gene would hinder the autophagy of BPH cells. BPH patients are related to the regulation of adhesion kinase (FAK) [19]. Through the regulation of FAK, smooth muscle in tissues of BPH patient contracts, which may improve LUTS and play an important role in treating LUTS. Blood oxygen level contrast magnetic resonance imaging can be used as a standard to distinguish PCa from BPH before the operation, instead of a noninvasive assessment of vascular maturity [20]. Penna et al. [21] found that MHC class Ⅱ molecules were expressed in BHP cells. Annexin (ANX) represents a family of calcium and phospholipid-binding proteins and plays an important role in physiological processes such as cell differentiation. Lehnigk et al. [22] found that the immunoreactivity of ANX Ⅰ and Ⅱ was limited to basal cells of glands in samples of BPH patients. In addition, we found that the more is the role of concentration of multiple pathways in BPH has not yet been confirmed, such as the endocytic vesicle, regulation of cell-cell adhesion and epstein-barr virus infection. In future studies, researchers can pay attention to the role of these pathways in BPH and verify them through biological experiments.
To determine the diagnosis-related genes, five genes (IGF1, PSIP1, SLC1A3, SLC2A1 and T1A1) related to the diagnosis were obtained by RF screening. IGF1 has been proven to be the key regulator of the immunogenic phenotype of BPH. In a tissue sample from PCa patients, BPH patients and normal prostate, the mRNA expression level of IGF1 was observed by quantitative real-time RT-PCR. It was found that IGF1 was down-regulated in BPH, which could be used as a potential target for the detection and treatment of BPH [23]. The LEDGF/PSIP1 is one of the autoantibodies of BPH [24]. Notably, in Figure 6A–F, the AUCs of all five genes were more significant than 0.6. However, limited by the quality of BPH data in the GEO database and the limited sample size, only IGF1 had confidence intervals above 0.5. In future studies, we will collect more BPH data to obtain more high-quality samples and further validate the diagnostic genes.
Furthermore, this paper analyzed the correlation between five genes, immune cell infiltration and the immune microenvironment. Among them, it has a significant correlation with some genes. M2 macrophage-mediated IL4 signaling is closely related to the progress of BPH [25]. There are abundant activated mast cells in BPH. Jin et al. [26] showed that the adoptive transfer of regulatory T cells might be an important way to treat BPH.
In a few words, this paper found the relevant pathways of abnormal expression genes of BPH through various information analyses and identified several diagnosis-related genes through a machine learning algorithm. We further analyzed the top five genes' relationship with immune infiltration and the immune microenvironment. This study helps discover the molecular mechanism of BPH. However, the scope of the method applied in this paper is limited by public databases, and the number of samples and data quality cannot be guaranteed. In future studies, we will collect more samples and try multiple machine learning models while ensuring data quality. Furthermore, we note that advances in interaction prediction studies in various fields of computational biology will provide valuable insights into BPH-related genetic markers and ncrnas [27]. Most of the existing development methods are based on deep learning models. Wang et al. [28] developed a method based on a graph convolutional neural network and conditional random field to predict the interaction between human miRNA and lncRNA and obtained high prediction accuracy. In a similar study, Sun et al. [29] combined a graph convolutional network and a graph attention network to predict diseases and their potential association with related metabolites. Zhang et al. [30] developed a computational method for predicting interactions between large-scale mirnas and lncrnas by computing similarity networks of lncrnas and mirnas and integrating them with Gaussian interaction spectrum kernel similarity. Then, network distance analysis is performed on the integral similarity network, and the final score is obtained after confidence calculation and score conversion. This method has achieved good results on large-scale datasets. In future studies, we will try combining the advantages of the above computational methods to develop RNA intermolecular interaction relationship prediction methods with higher accuracy.
We thank the authors for their contributions and the reviewers for their critical review of the manuscript.
The authors declare there is no conflict of interest.
[1] |
H. Xiao, Y. Jiang, W. He, D. Xu, P. Chen, D. Liu, et al., Identification and functional activity of matrix-remodeling associated 5 (MXRA5) in benign hyperplastic prostate, Aging, 12 (2020), 8605–8621. https://doi.org/10.18632/aging.103175 doi: 10.18632/aging.103175
![]() |
[2] |
C. Wang, X. Du, R. Yang, J. Liu, D. Xu, J. Shi, et al., The prevention and treatment effects of tanshinone ⅡA on oestrogen/androgen-induced benign prostatic hyperplasia in rats, J. Steroid Biochem. Mol. Biol., 145 (2015), 28–37. https://doi.org/10.1016/j.jsbmb.2014.09.026 doi: 10.1016/j.jsbmb.2014.09.026
![]() |
[3] |
X. Li, Z. Dai, X. Wu, N. Zhang, H. Zhang, Z. Wang, et al., The comprehensive analysis identified an autophagy signature for the prognosis and the immunotherapy efficiency prediction in lung adenocarcinoma, Front. Immunol., 13 (2022), 749241. https://doi.org/10.3389/fimmu.2022.749241 doi: 10.3389/fimmu.2022.749241
![]() |
[4] |
S. He, Z. Deng, Z. Li, W. Gao, D. Zeng, Y. Shi, et al., Signatures of 4 autophagy-related genes as diagnostic markers of MDD and their correlation with immune infiltration, J. Affective Disord., 295 (2021), 11–20. https://doi.org/10.1016/j.jad.2021.08.005 doi: 10.1016/j.jad.2021.08.005
![]() |
[5] |
Z. Ke, H. Cai, Y. Wu, Y. Lin, X. Li, J. Huang, et al., Identification of key genes and pathways in benign prostatic hyperplasia, J. Cell. Physiol., 234 (2019), 19942–19950. https://doi.org/10.1002/jcp.28592 doi: 10.1002/jcp.28592
![]() |
[6] |
P. Xiang, D. Liu, D. Guan, Z. Du, Y. Hao, W. Yan, et al., Identification of key genes in benign prostatic hyperplasia using bioinformatics analysis, World J. Urol., 39 (2021), 3509–3516. https://doi.org/10.1007/s00345-021-03625-5 doi: 10.1007/s00345-021-03625-5
![]() |
[7] |
X. Xu, Y. Wang, Z. Sihong, J. Lu, X. Zheng, J. Wang, et al., Immune infiltration pattern associated with diagnosis and development in benign prostatic hyperplasia, Urol. J., 18 (2021), 564–572. https://doi.org/10.22037/uj.v18i.6678 doi: 10.22037/uj.v18i.6678
![]() |
[8] |
R. Sachdeva, N. Kaur, P. Kapoor, P. Singla, N. Thakur, S. Singhmar, Computational analysis of protein-protein interaction network of differentially expressed genes in benign prostatic hyperplasia, Mol. Biol. Res. Commun., 11 (2022), 85–96. https://doi.org/10.22099/mbrc.2022.43721.1746 doi: 10.22099/mbrc.2022.43721.1746
![]() |
[9] |
Y. Ge, Q. Wang, W. Shao, Y. Zhao, Q. Shi, Q. Yuan, et al., Circulating let-7f-5p improve risk prediction of prostate cancer in patients with benign prostatic hyperplasia, J. Cancer, 11 (2020), 4542–4549. https://doi.org/10.7150/jca.45077 doi: 10.7150/jca.45077
![]() |
[10] |
M. A. Harris, J. Clark, A. Ireland, J. Lomax, M. Ashburner, R. Foulger, et al., The Gene Ontology (GO) database and informatics resource, Nucleic Acids Res., 32 (2004), 258–261. https://doi.org/10.1093/nar/gkh036 doi: 10.1093/nar/gkh036
![]() |
[11] |
H. Ogata, S. Goto, K. Sato, W. Fujibuchi, H. Bono, M. Kanehisa, KEGG: Kyoto encyclopedia of genes and genomes, Nucleic Acids Res., 27 (1999), 29–34. https://doi.org/10.1093/nar/27.1.29 doi: 10.1093/nar/27.1.29
![]() |
[12] |
A. M. Newman, C. B. Steen, C. L. Liu, A. J. Gentles, A. A. Chaudhuri, F. Scherer, et al., Determining cell type abundance and expression from bulk tissues with digital cytometry, Nat. Biotechnol., 37 (2019), 773–782. https://doi.org/10.1038/s41587-019-0114-2 doi: 10.1038/s41587-019-0114-2
![]() |
[13] |
S. Wang, C. Zhang, Z. Xu, M. H. Chen, H. Yu, L. Wang, et al., Differential impact of PI3K/AKT/mTOR signaling on tumor initiation and progression in animal models of prostate cancer, Prostate, 83 (2023), 97–108. https://doi.org/10.1002/pros.24441 doi: 10.1002/pros.24441
![]() |
[14] |
S. Wang, K. Li, Z. Liu, S. Gui, N. Liu, X. Liu, Aerobic exercise ameliorates benign prostatic hyperplasia in obese mice through downregulating the AR/androgen/PI3K/AKT signaling pathway, Exp. Gerontol., 143 (2021), 111152. https://doi.org/10.1016/j.exger.2020.111152 doi: 10.1016/j.exger.2020.111152
![]() |
[15] |
G. J. Leiros, S. R. Galliano, M. E. Sember, T. Kahn, E. Schwarz, K. Eiguchi, Detection of human papillomavirus DNA and p53 codon 72 polymorphism in prostate carcinomas of patients from Argentina, BMC Urol., 5 (2005). https://doi.org/10.1186/1471-2490-5-15 doi: 10.1186/1471-2490-5-15
![]() |
[16] |
D. B. Joseph, G. H. Henry, A. Malewska, J. C. Reese, R. J. Mauck, J. C. Gahan, et al., 5-Alpha reductase inhibitors induce a prostate luminal to club cell transition in human benign prostatic hyperplasia, J. Pathol., 256 (2022), 427–441. https://doi.org/10.1002/path.5857 doi: 10.1002/path.5857
![]() |
[17] |
Y. Li, Q. Wang, J. Li, B. Shi, Y. Liu, P. Wang, SIRT3 affects mitochondrial metabolic reprogramming via the AMPK-PGC-1α axis in the development of benign prostatic hyperplasia, Prostate, 81 (2021), 1135–1148. https://doi.org/10.1002/pros.24208 doi: 10.1002/pros.24208
![]() |
[18] |
R. Liu, S. Zhang, R. Wan, J. Deng, W. Fang, Effect of Beclin-1 gene silencing on autophagy and apoptosis of the prostatic hyperplasia epithelial cells, Clinics, 77 (2022), 100076. https://doi.org/10.1016/j.clinsp.2022.100076 doi: 10.1016/j.clinsp.2022.100076
![]() |
[19] |
T. Kunit, C. Gratzke, A. Schreiber, F. Strittmatter, R. Waidelich, B. Rutz, et al., Inhibition of smooth muscle force generation by focal adhesion kinase inhibitors in the hyperplastic human prostate, Am. J. Physiol. Renal. Physiol., 307 (2014), 823–832. https://doi.org/10.1152/ajprenal.00011.2014 doi: 10.1152/ajprenal.00011.2014
![]() |
[20] |
N. Di, N. Mao, W. Cheng, H. Pang, Y. Ren, N. Wang, et al., Blood oxygenation level-dependent magnetic resonance imaging during carbogen breathing: differentiation between prostate cancer and benign prostate hyperplasia and correlation with vessel maturity, Onco Targets Ther., 9 (2016), 4143–4150. https://doi.org/10.2147/OTT.S105480 doi: 10.2147/OTT.S105480
![]() |
[21] |
G. Penna, B. Fibbi, S. Amuchastegui, C. Cossetti, F. Aquilano, G. Laverny, et al., Human benign prostatic hyperplasia stromal cells as inducers and targets of chronic immuno-mediated inflammation, J. Immunol., 182 (2009), 4056–4064. https://doi.org/10.4049/jimmunol.0801875 doi: 10.4049/jimmunol.0801875
![]() |
[22] |
U. Lehnigk, U. Zimmermann, C. Woenckhaus, J. Giebel, Localization of annexins Ⅰ, Ⅱ, Ⅳ and Ⅶ in whole prostate sections from radical prostatectomy patients, Histol. Histopathol., 20 (2005), 673–680. https://doi.org/10.14670/HH-20.673 doi: 10.14670/HH-20.673
![]() |
[23] | N. Soulitzis, I. Karyotis, D. Delakas, D. A. Spandidos, Expression analysis of peptide growth factors VEGF, FGF2, TGFB1, EGF and IGF1 in prostate cancer and benign prostatic hyperplasia, Int. J. Oncol., 29 (2006), 305–314. |
[24] |
D. J. O'Rourke, D. A. DiJohnson, R. J. Caiazzo Jr, J. C. Nelson, D. Ure, M. P. O'Leary, et al. Autoantibody signatures as biomarkers to distinguish prostate cancer from benign prostatic hyperplasia in patients with increased serum prostate specific antigen, Clin. Chim. Acta, 413 (2012): 561–567. https://doi.org/10.1016/j.cca.2011.11.027 doi: 10.1016/j.cca.2011.11.027
![]() |
[25] |
J. Sheng, Y. Yang, Y. Cui, S. He, L. Wang, L. Liu, et al., M2 macrophage-mediated interleukin-4 signalling induces myofibroblast phenotype during the progression of benign prostatic hyperplasia, Cell Death Dis., 9 (2018), 755. https://doi.org/10.1038/s41419-018-0744-1 doi: 10.1038/s41419-018-0744-1
![]() |
[26] |
X. Jin, T. Lin, G. Yang, H. Cai, B. Tang, X. Liao, et al. Use of Tregs as a cell-based therapy via CD39 for benign prostate hyperplasia with inflammation, J. Cell. Mol. Med., 24 (2020), 5082–5096. https://doi.org/10.1111/jcmm.15137 doi: 10.1111/jcmm.15137
![]() |
[27] |
C. Wang, C. Han, Q. Zhao, X. Chen, Circular RNAs and complex diseases: from experimental results to computational models, Brief Bioinform, 22 (2021), 286. https://doi.org/10.1093/bib/bbab286 doi: 10.1093/bib/bbab286
![]() |
[28] |
W. Wang, L. Zhang, J. Sun, Q. Zhao, J. Shuai, Predicting the potential human lncRNA-miRNA interactions based on graph convolution network with conditional random field, Brief. Bioinform., 23 (2022), 463. https://doi.org/10.1093/bib/bbac463 doi: 10.1093/bib/bbac463
![]() |
[29] |
F. Sun, J. Sun, Q. Zhao, A deep learning method for predicting metabolite-disease associations via graph neural network, Brief. Bioinform., 23 (2022), 266. https://doi.org/10.1093/bib/bbac266 doi: 10.1093/bib/bbac266
![]() |
[30] |
L. Zhang, P. Yang, H. Feng, Q. Zhao, H. Liu, Using network distance analysis to predict lncRNA-miRNA interactions, Interdiscip Sci, 3 (2021), 535–545. https://doi.org/10.1007/s12539-021-00458-z doi: 10.1007/s12539-021-00458-z
![]() |
1. | Honglei Wang, Qinghe Gao, Shengjing Liu, Boda Guo, Network Pharmacology Prediction and Molecular Docking-based Strategy to Explore the Potential Mechanism of Guiyi Tonglong Prescription in the Treatment of Benign Prostatic Hyperplasia, 2024, 11, 2773-0395, 10.1097/IMNA-D-24-00047 |