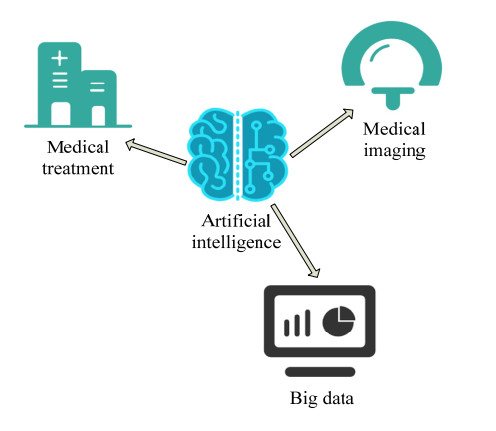
Esophageal cancer has become a malignant tumor disease with high mortality worldwide. Many cases of esophageal cancer are not very serious in the beginning but become severe in the late stage, so the best treatment time is missed. Less than 20% of patients with esophageal cancer are in the late stage of the disease for 5 years. The main treatment method is surgery, which is assisted by radiotherapy and chemotherapy. Radical resection is the most effective treatment method, but a method for imaging examination of esophageal cancer with good clinical effect has yet to be developed. This study compared imaging staging of esophageal cancer with pathological staging after operation based on the big data of intelligent medical treatment. MRI can be used to evaluate the depth of esophageal cancer invasion and replace CT and EUS for accurate diagnosis of esophageal cancer. Intelligent medical big data, medical document preprocessing, MRI imaging principal component analysis and comparison and esophageal cancer pathological staging experiments were used. Kappa consistency tests were conducted to compare the consistency between MRI staging and pathological staging and between two observers. Sensitivity, specificity and accuracy were determined to evaluate the diagnostic effectiveness of 3.0T MRI accurate staging. Results showed that 3.0T MR high-resolution imaging could show the histological stratification of the normal esophageal wall. The sensitivity, specificity and accuracy of high-resolution imaging in staging and diagnosis of isolated esophageal cancer specimens reached 80%. At present, preoperative imaging methods for esophageal cancer have obvious limitations, while CT and EUS have certain limitations. Therefore, non-invasive preoperative imaging examination of esophageal cancer should be further explored.Esophageal cancer has become a malignant tumor disease with high mortality worldwide. Many cases of esophageal cancer are not very serious in the beginning but become severe in the late stage, so the best treatment time is missed. Less than 20% of patients with esophageal cancer are in the late stage of the disease for 5 years. The main treatment method is surgery, which is assisted by radiotherapy and chemotherapy. Radical resection is the most effective treatment method, but a method for imaging examination of esophageal cancer with good clinical effect has yet to be developed. This study compared imaging staging of esophageal cancer with pathological staging after operation based on the big data of intelligent medical treatment. MRI can be used to evaluate the depth of esophageal cancer invasion and replace CT and EUS for accurate diagnosis of esophageal cancer. Intelligent medical big data, medical document preprocessing, MRI imaging principal component analysis and comparison and esophageal cancer pathological staging experiments were used. Kappa consistency tests were conducted to compare the consistency between MRI staging and pathological staging and between two observers. Sensitivity, specificity and accuracy were determined to evaluate the diagnostic effectiveness of 3.0T MRI accurate staging. Results showed that 3.0T MR high-resolution imaging could show the histological stratification of the normal esophageal wall. The sensitivity, specificity and accuracy of high-resolution imaging in staging and diagnosis of isolated esophageal cancer specimens reached 80%. At present, preoperative imaging methods for esophageal cancer have obvious limitations, while CT and EUS have certain limitations. Therefore, non-invasive preoperative imaging examination of esophageal cancer should be further explored.
Citation: Linglei Meng, XinFang Shang, FengXiao Gao, DeMao Li. Comparative study of imaging staging and postoperative pathological staging of esophageal cancer based on smart medical big data[J]. Mathematical Biosciences and Engineering, 2023, 20(6): 10514-10529. doi: 10.3934/mbe.2023464
[1] | Bo An . Construction and application of Chinese breast cancer knowledge graph based on multi-source heterogeneous data. Mathematical Biosciences and Engineering, 2023, 20(4): 6776-6799. doi: 10.3934/mbe.2023292 |
[2] | Na Li, Peng Luo, Chunyang Li, Yanyan Hong, Mingjun Zhang, Zhendong Chen . Analysis of related factors of radiation pneumonia caused by precise radiotherapy of esophageal cancer based on random forest algorithm. Mathematical Biosciences and Engineering, 2021, 18(4): 4477-4490. doi: 10.3934/mbe.2021227 |
[3] | Jun Gao, Qian Jiang, Bo Zhou, Daozheng Chen . Convolutional neural networks for computer-aided detection or diagnosis in medical image analysis: An overview. Mathematical Biosciences and Engineering, 2019, 16(6): 6536-6561. doi: 10.3934/mbe.2019326 |
[4] | Gaozhong Sun, Tongwei Zhao . Lung adenocarcinoma pathology stages related gene identification. Mathematical Biosciences and Engineering, 2020, 17(1): 737-746. doi: 10.3934/mbe.2020038 |
[5] | Linlin Tan, Dingzhuo Cheng, Jianbo Wen, Kefeng Huang, Qin Zhang . Identification of prognostic hypoxia-related genes signature on the tumor microenvironment in esophageal cancer. Mathematical Biosciences and Engineering, 2021, 18(6): 7743-7758. doi: 10.3934/mbe.2021384 |
[6] | Qinwang Niu, Haoyue Li, Yu Liu, Zhibo Qin, Li-bo Zhang, Junxin Chen, Zhihan Lyu . Toward the Internet of Medical Things: Architecture, trends and challenges. Mathematical Biosciences and Engineering, 2024, 21(1): 650-678. doi: 10.3934/mbe.2024028 |
[7] | Kunli Zhang, Shuai Zhang, Yu Song, Linkun Cai, Bin Hu . Double decoupled network for imbalanced obstetric intelligent diagnosis. Mathematical Biosciences and Engineering, 2022, 19(10): 10006-10021. doi: 10.3934/mbe.2022467 |
[8] | Dijian Shen, Youhua Jiang, Kaiyi Tao . Expression and clinical significance of p75NTR in esophageal squamous cell carcinoma. Mathematical Biosciences and Engineering, 2019, 16(6): 8060-8068. doi: 10.3934/mbe.2019405 |
[9] | Macarena Boix, Begoña Cantó . Using wavelet denoising and mathematical morphology in the segmentation technique applied to blood cells images. Mathematical Biosciences and Engineering, 2013, 10(2): 279-294. doi: 10.3934/mbe.2013.10.279 |
[10] | Hassan Ali Khan, Wu Jue, Muhammad Mushtaq, Muhammad Umer Mushtaq . Brain tumor classification in MRI image using convolutional neural network. Mathematical Biosciences and Engineering, 2020, 17(5): 6203-6216. doi: 10.3934/mbe.2020328 |
Esophageal cancer has become a malignant tumor disease with high mortality worldwide. Many cases of esophageal cancer are not very serious in the beginning but become severe in the late stage, so the best treatment time is missed. Less than 20% of patients with esophageal cancer are in the late stage of the disease for 5 years. The main treatment method is surgery, which is assisted by radiotherapy and chemotherapy. Radical resection is the most effective treatment method, but a method for imaging examination of esophageal cancer with good clinical effect has yet to be developed. This study compared imaging staging of esophageal cancer with pathological staging after operation based on the big data of intelligent medical treatment. MRI can be used to evaluate the depth of esophageal cancer invasion and replace CT and EUS for accurate diagnosis of esophageal cancer. Intelligent medical big data, medical document preprocessing, MRI imaging principal component analysis and comparison and esophageal cancer pathological staging experiments were used. Kappa consistency tests were conducted to compare the consistency between MRI staging and pathological staging and between two observers. Sensitivity, specificity and accuracy were determined to evaluate the diagnostic effectiveness of 3.0T MRI accurate staging. Results showed that 3.0T MR high-resolution imaging could show the histological stratification of the normal esophageal wall. The sensitivity, specificity and accuracy of high-resolution imaging in staging and diagnosis of isolated esophageal cancer specimens reached 80%. At present, preoperative imaging methods for esophageal cancer have obvious limitations, while CT and EUS have certain limitations. Therefore, non-invasive preoperative imaging examination of esophageal cancer should be further explored.Esophageal cancer has become a malignant tumor disease with high mortality worldwide. Many cases of esophageal cancer are not very serious in the beginning but become severe in the late stage, so the best treatment time is missed. Less than 20% of patients with esophageal cancer are in the late stage of the disease for 5 years. The main treatment method is surgery, which is assisted by radiotherapy and chemotherapy. Radical resection is the most effective treatment method, but a method for imaging examination of esophageal cancer with good clinical effect has yet to be developed. This study compared imaging staging of esophageal cancer with pathological staging after operation based on the big data of intelligent medical treatment. MRI can be used to evaluate the depth of esophageal cancer invasion and replace CT and EUS for accurate diagnosis of esophageal cancer. Intelligent medical big data, medical document preprocessing, MRI imaging principal component analysis and comparison and esophageal cancer pathological staging experiments were used. Kappa consistency tests were conducted to compare the consistency between MRI staging and pathological staging and between two observers. Sensitivity, specificity and accuracy were determined to evaluate the diagnostic effectiveness of 3.0T MRI accurate staging. Results showed that 3.0T MR high-resolution imaging could show the histological stratification of the normal esophageal wall. The sensitivity, specificity and accuracy of high-resolution imaging in staging and diagnosis of isolated esophageal cancer specimens reached 80%. At present, preoperative imaging methods for esophageal cancer have obvious limitations, while CT and EUS have certain limitations. Therefore, non-invasive preoperative imaging examination of esophageal cancer should be further explored.
The incidence of adenocarcinoma has increased. Many patients are diagnosed at a late stage, but patients with middle- and late-stage esophageal cancer are not indicated for operation. Among all malignant tumors, the prognosis of esophageal cancer is poor and the mortality rate is high. The mortality rate of esophageal cancer increases worldwide. More than 490, 000 new cases of esophageal cancer were reported in 2005. The incidence rate of cancer is predicted to decrease significantly in other areas in 2025, but the incidence rate of esophageal cancer has increased steadily, reaching 140%. According to the National Cancer Institute, about 17, 990 new cases and 15, 210 deaths related to esophageal cancer were reported in 2013. In recent years, esophageal cancer has made great progress in diagnosis and treatment; however, the survival rate in the past 5 years is only 1 to 20%.
The treatment of esophageal cancer focuses on surgery, radiotherapy, chemotherapy and targeted treatment. With the rapid development of science and technology and medical technology, the treatment of esophageal cancer has improved considerably and the survival rate of patients increases year by year. Many patients with esophageal cancer are found after infection because of its atypical symptoms at first. The discovery time is very late, and the optimal treatment period is missed. During this period, radiotherapy and chemotherapy have become an important means of alleviating the disease. With the development of antitumor drugs, chemotherapy has made great progress for treatment of esophageal cancer. However, the tolerance and complications of chemotherapy have been the focus of attention and debate. Targeted drugs are basic drugs for the treatment of esophageal cancer. The targeted treatment of esophageal cancer is mostly in phase II/III clinical research. The efficacy is not yet clear. Targeted drugs for esophageal cancer need to be tested quickly prior to its commercialization to prevent the spread and expansion of the disease. Therefore, further research is needed for targeted treatment of esophageal cancer.
The main pathological types of esophageal cancer are squamous cell carcinoma and esophageal adenocarcinoma, and the other pathological types are rare. Squamous cell carcinoma is the most common type of esophageal cancer worldwide, the overall incidence rate increases with age. The most common type of esophageal cancer is the middle and lower segment of the esophagus. The incidence rate of Black people is three times that of White people. Tang reported that intensive data analysis is a major challenge for smart cities because sensors are everywhere. The natural characteristics of geographical distribution require a new computing paradigm to provide position sensing and delay sensitive monitoring and intelligent control. Fog computing extends the computation to the edge of the network, which meets this demand. Intelligence should be integrated in fog computing architecture, such as data representation and feature extraction, identification of abnormal and dangerous events, and provision of optimal response and control, to ensure the security of future communities [1]. Riva G stated that cloud computing big data analysis is one of the emerging fields of processing and analysis. It is used to mine fog computing for big data analysis in geographic space and medical and health applications and for detecting the real characteristics of diabetic patients. A deep learning framework based on machine learning is proposed to analyze the pathological characteristics of smart watch worn by diabetic patients and the geographical parameters of the geospatial database of the Ganghe River Basin. Results show that fog computing has great application prospect in medical and geospatial big data analysis [2]. Lenoir J developed a computer technology for high-performance processing, analysis and interpretation of medical and diagnostic images. He proposed a new method to analyze the interest of the distribution region of geometric and texture parameters based on different types of images. A new method of unstructured information feature space generation based on big data mining is proposed. This technology can be used to extract areas of interest from fundus images, which contain four types of objects: exudates, complete regions, thick vessels and thin vessels. With the application of big data technology, training of the samples is improved and classification errors are reduced because of the large amount of data, thereby improving the accuracy of diagnosis to 95%. The technique has been applied to the key problems of diabetic retinopathy. The experimental results on real fundus images show that the method is effective [3]. Elbamby reported that big data technology is gradually penetrating into various application fields, such as smart transportation, smart cities and smart medical care; in particular, smart medical care is the core part of smart cities and is changing the medical structure. Specifically, it can be used to improve treatment plans for various diseases. A sustainable treatment planning strategy in intelligent medical treatment should be determined because of the unique treatment cost, pollution effect and side effects on patients. From the perspective of sustainable development, a three-dimensional evaluation model of medical raw data is proposed and used to develop a sustainable treatment plan, which has feasible and practical application [4]. However, these methods lack numerical analysis and specific data for experiments.
This study aims to compare imaging stage and post-operation pathological stage of esophageal cancer based on intelligent medical big data, pretreatment of medical documents, principal component analysis and contrast-enhanced MRI. A new method is proposed to analyze and compare the MRI and pathological staging of esophageal cancer due to differences in the layers of the esophageal wall in the images. High-resolution MRI can clearly show the tissue layer of the esophageal wall. Therefore, high-resolution MRI can stage esophageal cancer more accurately than other imaging methods.
Medical health has been the main concern of people. Only when people are healthy that they can have more energy to do other things and enjoy life [5,6]. The combination of science and technology and medical technology is the main direction in the future. Any industry should embrace the Internet for further development. Science and technology also makes medical technology more convenient [7]. With the significant improvement of people's living standards, the continuous improvement of economic and social modernization and the people's growing desire for a healthy and better life, people's demand for medical and health services is growing rapidly, showing diversification and multilevel characteristics [8,9]. Every aspect of life would produce a large number of data fragments every day. In the Internet era, the most important thing is to analyze and extract valuable data hidden in big data, transform scattered data into useful information, promote production and improve the quality of life. Intelligent analysis of different sources of health data and application technology in daily life, medical detection data and ECG signals are the most common health data; however, the two types of data are difficult to analyze and identify [10]. Medical test sheet, ECG signal search and analysis model is applied to the system prototype. The intelligent medical health is shown in Figure 1.
1) Graying
In text recognition in the late stage of imaging, features should be extracted. After common hog and image graying, the color information is lost, the image dimension is reduced, and the amount of calculation is relatively reduced [11]. For the image represented by HSV, the image can be converted into RGB image according to the relationship of HSV components:
(1) |
(2) |
(3) |
2) Binarization
Binarization divides the image pixel into 0 or 1 according to the threshold value. Threshold segmentation is essential. The threshold selection method adopted in this paper is the Otsu algorithm [12]. Based on the idea of clustering, the algorithm divides the image into two parts: background and foreground according to the gray value distribution of the image and determines the threshold value in the local area to maximize the variance between the two parts. The larger the variance between the two parts is, the smaller the probability of being wrongly divided by the threshold value will be.
(4) |
In shooting the paper test sheet, light intensity and shooting angle would affect image quality [13]. It can reduce some interference factors in the actual scene, optimize the visual effect of the test sheet image, and improve the accuracy of the test sheet. The general formula of binarization and other pretreatment methods is as follows:
(5) |
3) Convolution operation
Convolution layer, also known as filter layer, is the core of the convolution neural network [14]. In image recognition, the convolution is two dimensional. The so-called convolution in mathematics usually refers to the integral operation between two functions. Assuming that h and K are measurable functions in M domain, and the cycle of H and K is expressed as h * k. The convolution represents the integral of the product of one function and another function after inverse transformation. The calculation formula is as follows:
(6) |
where G is the convolution kernel for an image, and the input matrix is equivalent to the pixels in the image. In image recognition, the convolution of an image is called an independent two-dimensional filter [15,16]. Image convolution aims to transmit the two-dimensional image to all position filters and use a pixel and its pixel domain to each position of the inner product for attribute mapping. Each characteristic curve is connected to a region of adjacent neurons in the previous layer. The calculation in the two-dimensional discrete plane is as follows because the convolution activity of the convolution core is a part of this region:
(7) |
where H is the two-dimensional matrix, f is the convolution kernel and X and y are the convolution dimensions, respectively. In the actual operation, it needs to specify the step size of convolution kernel movement. Because the input is a two-dimensional matrix, people need to specify the horizontal step size and vertical step size. For more intuitive expression, a convolution kernel would produce a feature map after convolution on the input, and the input of all convolution kernels is shared; the complete feature mapping is obtained using different kernels [17]. Suppose that it represents the element value of the i-th row and j-th column of the k-th characteristic graph in the L-th layer, the calculation method is as follows:
(8) |
where H and x represent the weight and deviation vectors of the convolution kernel, respectively. The input region centered on (I, J) is shared by multiple function diagrams in the region. It has many advantages, such as reducing the complexity of the model. Grouping can reduce the amount of computation by reducing the quota. The pooling layer is usually placed in the middle of two convolution layers, and the average pooling layer is the element of the next layer after calculating the average value of each region:
(9) |
At present, large-scale intelligent devices can be seen everywhere in hospitals, which can greatly help people to check and treat diseases and realize medical intelligence. However, the main medical data are not used for clinical diagnosis and treatment decision-making, public health monitoring, health management, etc. due to the limitations of various physical and technical management methods; the intelligent medical system depends on the environment [18]. Environment dependence means that the intelligent medical system must operate in a specific medical environment, which mainly includes patients. With the introduction of intelligent medical systems, the original medical processes are used in parallel; some problems may arise in the integration process. Research on the process of intelligent medical systems should test the reliability of the system in the medical environment (Figure 2).
According to the complex medical environment, the general specification of medical system integration is usually formulated, which constitutes the technical structure of medical information sharing. The participants include clinical staff, such as doctors and nurses, and medical equipment manufacturing enterprises. In the IHE framework, people have a unified understanding of the process of formulating medical standards for various medical participants, which can better guide the work of clinical workers. Better medical procedures can be designed through continuous discussion of the framework, and general medical procedures can greatly promote the development of intelligent medical information platform [19].
Principal component analysis forms independent principal components after transforming original index variables. The analysis forms independent principal components after transforming the original index variables. The higher the degree of correlation between indicators is, the better the principal component analysis effect will be. This method is helpful for the comparative study of imaging staging and postoperative pathological staging of esophageal cancer in big data.
Principal component analysis is a statistical technique based on dimension reduction. The principle is to transform a large number of original variables into a group of new and unrelated variable sets after processing. The recombined variables can reflect the information of the original variables. The data standardization is as follows:
(10) |
(11) |
(12) |
A small number of support vectors determine the final result and are not sensitive to outliers. This cannot only help us grasp key samples and "weed out" a large number of redundant samples but also proves that the method is simple in the algorithm and has good "robustness." Support vector machine is a kind of data mining technology that uses optimization methods to solve machine learning-related problems [20]. In recent years, this method has made great progress and has become an important method to solve the problem of learning and dimension disaster. Assuming that the average of the variables of each characteristic dimension can take N different values (for the case of continuous values, N partitions of the space on the corresponding dimension), the result of the combination of D dimension variables is the D power of N. The number of combinations increases exponentially, which is called "combination explosion." X is the input space. H (x) on H is as follows to find the real valued function:
(13) |
The inclusion criteria were as follows: patients diagnosed with esophageal cancer, underwent radical external lens resection and did not receive other antitumor treatment; chest CT and routine hematology performed before the operation; lack of distant metastasis and serious cardiovascular and cerebrovascular diseases; and detailed pathology results. The exclusion criteria were as follows: palliative operation or thoracotomy for esophageal cancer; radiotherapy, chemotherapy and other treatments before operation; and other histories of malignant tumors.
This study is a potential research development. The following cases of esophageal cancer were obtained through interviews with qualified experimental observers and included in the standard: 1) gastroscopy biopsy for diagnosis of esophageal cancer and conduct of in vitro MRI scan 6528, 2) no preoperative radiotherapy or chemotherapy performed; and 3) MRI images that met the diagnostic requirements. In this study, 100 patients with esophageal cancer were included (65 males and 35 females, with average age of 63 years). The patients included 26 cases of high esophageal cancer, 40 cases of middle-stage esophageal cancer and 34 cases of low-stage esophageal cancer. A total of 68 cases had esophageal squamous cell carcinoma. Detailed information is shown in Table 1.
Parameter | Number of patients |
Age | |
≥ 65 | 85 (85%) |
< 65 | 15 (15%) |
Gender | |
Male | 65 (65%) |
Female | 35 (35%) |
Number of metastases | |
Simple | 44 (44%) |
Multiple | 56 (56%) |
Serum CEA | |
≤ 5 ng/mL | 38 (38%) |
> 5 ng/mL | 62 (62%) |
T stage | |
2 | 20 (20%) |
3 | 64 (64%) |
4 | 16 (16%) |
N stage | |
0 | 14 (14%) |
1 | 26 (26%) |
2 | 60 (60%) |
Using 3.0-T MRI scanner, 4-channel 3.5 inch small animal coil, high-resolution cross-sectional tw1i and TW2 images were scanned with FSE sequence. TW1 scanning parameters included the following: tr = 588 msec; TE = 68 msec; slice thickness = 3 mm, no interval scanning; FOV = 60 × 36 mm, 2; Matrix = 256 × 192; NEX = 2. TW2 scanning parameters: tr = 700 msec; TE = 85 msec; layer thickness = 3 mm; FOV = 60 mm × 36 mm 2; Matrix = 512 × 352; NEX = 14, TR = 8008 msec; TE = 95 msec; and scanning time = 20 minutes when the slice thickness was 5 mm. MRI scanning was performed within 30 minutes after the specimen was removed. After scanning, the specimen was immediately fixed and sent for pathological examination (Table 2).
Equipment | TR | TE | Thickness | Time |
TW1 | 588 | 68 | 3 | 0 |
TW2 | 700 | 85 | 3 | 0 |
TW2 | 800 | 95 | 5 | 20 |
Two experienced diagnostic physicians randomly performed MRI through independent and blind evaluation. In the pre-experiment, two physicians had mastered the anatomy and histology of the esophagus. MRI analysis included the depth of invasion of the normal esophageal wall. When the depth of invasion was inconsistent, they reached an agreement through discussion. MRI staging was based on AJCC 7th esophageal cancer staging standard. MRI and corresponding pathological images were matched by a third imaging diagnostic physician. The morphology of the esophageal lumen, tumor and surrounding tissues was compared (Figure 3).
The detection rate of MRI for early esophageal tumor is not satisfactory. The accuracy rate of conventional T1-T2 weighted MRI for T staging of the tumor is only about 60%. High-resolution T2 weighted MRI can show the fine image of the esophageal wall and surrounding tissue, which can assist in the delineation of the radiotherapy target. Recent MRI studies of esophageal cancer showed that 33% of T1 tumors, 58% of T2 tumors, 96% of T3 tumors and 100% of T4 tumors could be detected by DWI sequence and high-resolution T2 weighted MRI. MRI may be a noninvasive method for determining the staging of esophageal cancer. However, up to now, although the application of DWI in the study of esophageal gross tumors has made some progress, no relevant research has reported on whether tumor invasion of adjacent unresectable tissue is feasible for surgical treatment. DWI images only reflect the process of random movement of water molecules in a certain period of time due to lack of specificity. At present, DWI images cannot be used for clinical diagnosis because of their great limitations.
The MRI findings of esophageal carcinoma at different stages are as follows: mucosa (T1a): the mass was confined to the mucosa, with mucosal epithelial thickening, ISO cycle interruption in the lower layer of muscular mucosa and no slightly higher mass signal in the submucosa; Subduction type (T1b): infiltrative mass in submucosa with slightly high signal intensity, irregular mass shadow, limited compression of inner ring muscle, but complete low signal ring; T2: the mass infiltrated into the muscle with low signal and slightly high signal, but the mass signal was only limited to the muscle; prevention (T3/T4): tumor infiltrates with slightly higher signal intensity, and the continuity of high signal intensity was interrupted (Table 3).
MR staging | Mucosa | Submucosa | Muscularis propria | Adventitia |
Mucosa | 9 | 3 | 0 | 0 |
submucosa | 4 | 18 | 2 | 1 |
muscularis propria | 1 | 3 | 15 | 2 |
adventitia | 1 | 1 | 1 | 23 |
In the advanced stage of esophageal cancer, the wall of the esophagus would be thickened, and the lumen would be relatively narrow. The change in the esophageal wall thickness can be clearly seen on CT images, and the expansion of the surrounding lumen is evident. The thickness of the esophageal wall can be accurately evaluated only by clearly showing the esophageal lumen. However, the lumen of patients with esophageal cancer tends to be narrowed, which makes the lesion less air in the esophagus, resulting in an unclear display. Patients often need to have an empty stomach before examination to avoid the interference of diet on the esophagus. Thin-layer scanning of the diseased esophagus can be used to enlarge and reconstruct the original data of the diseased area by four times.
The thickness of the esophageal wall is 3 mm, which is normal; thickness greater than 5 mm is abnormal. In the early stage of esophageal cancer, the lesion is not significant, only slight local pathological changes occur and the thickness of the esophagus almost does not change; thus, CT examination of early esophageal cancer has little significance.
Eighty-six cases (90.5%, 86/95) of esophageal cancer were consistent with pathological staging by MRI (the remaining 14 cases are inconsistent with the MRI pathological stage, and they would not be considered in this paper). The results showed a high coincidence rate (kappa value = 0.870, P < 0.01), and the two doctors had good consistency in MRI staging (kappa value = 0.869, P < 0.01).
As shown in Table 4, high-resolution imaging can accurately determine the eight-layer structure of the normal esophageal wall in vitro, which corresponds to the anatomical properties of tissue. The multilayer tissues show different signal characteristics in MRI, and the depth of invasion of esophageal cancer can be correctly judged according to the changes in the MRI signal. The mucous epithelium of the esophageal wall is composed of flat squamous epithelial cells, covering the lamina propria. The cell density is high, the content of extracellular fluid is low, the signal intensity of T2 wi is low and the submucosa is composed of dissolved connective tissue, abundant capillary and lymphatic network. T2WI shows mixed signal, and the MRI features of each layer of the esophageal wall reflect the structure of the inner tissue. Based on the difference in the tissue structure of each layer of the esophageal wall, high-resolution MRI imaging can clearly show the stratification of the wall tissue to ensure more accurate staging than other imaging methods.
Diagnostic index | Mucosa | Submucosa | Muscularis propria | Adventitia |
Sensitivity | 75.4 | 78.3 | 98 | 100 |
Specificity | 95.4 | 89.3 | 93.9 | 96.4 |
Accuracy | 92.6 | 86.8 | 67.7 | 93.8 |
False positive | 3.5 | 5.8 | 6.4 | 0 |
False negative | 22.8 | 20.6 | 4.6 | 2.4 |
Univariate analysis showed significant differences in NIC distribution, length and thickness in T2–T4 esophageal cancer (P < 0.05) but no significant differences in lesion location distribution, pathological type, differentiation degree and age. According to logistic regression analysis, NIC and thickness were risk factors in t quarter, while lesion length was not a risk factor in t point. The univariate analysis of potential risk factors for T stage of esophageal cancer is shown in Table 5.
Factors | T2 | T3 | T4 | P | |
Upper thoracic segment | 3 | 2 | 1 | 0.882 | |
Lesion location | Middle chest | 5 | 9 | 8 | 0 |
Lower thoracic segment | 4 | 5 | 3 | 0 | |
Medullary type | 15 | 9 | 6 | 0.457 | |
Pathological type | Ulcerative type | 2 | 3 | 4 | 0 |
Mushroom umbrella type | 2 | 2 | 3 | 0 |
All data were analyzed by SPSS17.0 statistical software. Logistic regression analysis was used to analyze factors affecting the T-phase of esophageal cancer. Spearman rank correlation grading was used to analyze the correlation between T-phase and NIC length. Based on the analysis of the receiver characteristic operation curve, the diagnostic effect of each phase was evaluated (Figure 4).
A significant positive correlation was found between T stage and NIC, lesion thickness and lesion length. NIC was positively correlated with lesion thickness but not with lesion length (Figure 5).
In the era of big data, machine learning is a high-performance computer system that combines AI with big data technology. The theoretical research of machine learning is very comprehensive and has made great progress in all aspects. The current research center is a typical example for the development of machine learning. It will classify the data as a category and automatically classify them. Bayesian mail recognition is based on classification. In the design of the algorithm, we set a threshold to determine the attributes of the mail. The classification result is applied to a subset of data that is completely independent of the data used in training. If this value is obtained by this algorithm, then it is a normal email. If it exceeds this value, then it will be regarded as garbage. Regression algorithm, SVM, dimensionality reduction algorithm and so on are used in this machine learning algorithm.
As the work of medical institutions becomes increasingly difficult, many diseases have no clinical solutions and are only judged by MRI, CT and other means, leading to a large number of medical images. At present, research on this image can only be carried out by manual means. Due to the qualification, ability and experience of employees, research on this image can only be carried out and the efficiency and accuracy are uncertain. It may lead to wrong evaluation. Intelligent medical image processing technology can effectively avoid the influence of human factors, provide technical support for improving the accuracy of image content interpretation, and provide strong support for image-based medical diagnosis methods.
This study aims to compare imaging staging and postoperative pathological staging of esophageal cancer by using intelligent medical big data, preprocessing of medical documents, principal component analysis and comparative experiment of MRI staging and pathological staging of esophageal cancer. In vitro 3.0 T MRI T2 mapping imaging can distinguish the normal esophageal wall and tumor tissue according to their T2 value. The normal esophageal wall layering shown on T2 mapping image corresponds to the histological and anatomical layering shown on pathological anatomy. The difference in T2 values between the normal esophageal wall and tumor tissue was statistically significant. The difference in T2 values between adjacent normal esophageal wall was statistically significant. Moreover, 3.0 T MRI T2 mapping imaging of esophageal cancer specimens in vitro can accurately determine the depth of invasion in different stages of esophageal cancer, with high sensitivity, specificity and accuracy in the diagnosis of esophageal cancer. Intelligent medical electronic medical records contain patients' symptoms, disease information and diagnostic consultation conclusions. Extraction technology can help analyze and explore the relationship between symptoms and diagnostic conclusions, thereby improving the clinical diagnosis and treatment efficiency of the medical team. The technology can be used as a foundation for assisted clinical diagnosis and treatment in the medical field. Therefore, intelligent diagnosis assistance can be realized by developing the natural language of intelligent medical text information.
This work was supported by Xingtai City key R & D program self-funded project(NO: 2020ZC162).
The authors declare that there is no conflict of interest.
[1] |
B. Tang, Z. Chen, G. Hefferman, S. Pei, T. Wei, H. He, et al., Incorporating intelligence in fog computing for big data analysis in smart cities, IEEE Trans. Ind. Inf., 13 (2017), 2140–2150. https://doi.org/10.1109/TⅡ.2017.2679740 doi: 10.1109/TⅡ.2017.2679740
![]() |
[2] |
R. K. Barik, R. Priyadarshini, H. Dubey, V. Kumar, K. Mankodiya, FogLearn: leveraging fog-based machine learning for smart system big data analytics, Int. J. Fog Comput., 1 (2018), 15–34. https://doi.org/10.4018/IJFC.2018010102 doi: 10.4018/IJFC.2018010102
![]() |
[3] |
M. Chen, J. Yang, J. Zhou, Y. Hao, J. Zhang, C. H. Youn, 5G-smart diabetes: Toward personalized diabetes diagnosis with healthcare big data clouds, IEEE Commun. Mag., 56 (2018), 16–23. https://doi.org/10.1109/MCOM.2018.1700788 doi: 10.1109/MCOM.2018.1700788
![]() |
[4] |
N. Y. Ilyasova, A. S. Shirokanev, A. V. Kupriyanov, R. A. Paringev, D. V. Kirsh, A. V. Soifer, Methods of intellectual analysis in medical diagnostic tasks using smart feature selection, Pattern Recognit. Image Anal., 28 (2018), 637–645. https://doi.org/10.1134/S1054661818040144 doi: 10.1134/S1054661818040144
![]() |
[5] | F. Hao, D. S. Park, S. Y. Woo, S. D. Min, S. Park, Treatment planning in smart medical: A sustainable strategy, J. Inf. Process. Syst., 12 (2016), 711–723. |
[6] |
W. El-Shafai, F. Khallaf, E. S. M. El-Rabaie, F. E. A. El-Samie, Proposed neural SAE-based medical image cryptography framework using deep extracted features for smart IoT healthcare applications, Neural Comput. Appl., 34 (2022), 10629–10653. https://doi.org/10.1007/s00521-022-06994-z doi: 10.1007/s00521-022-06994-z
![]() |
[7] |
Y. Z. Yu, Y. Liu, C. Zhu, Application of propofol in oral and maxillofacial surgery anesthesia based on smart medical blockchain technology, J. Healthcare Eng., 1 (2021), 1–11. https://doi.org/10.1155/2021/5529798 doi: 10.1155/2021/5529798
![]() |
[8] |
M. Rath, Real time analysis based on intelligent applications of big data and iot in smart health care systems, Int. J. Big Data Anal. Healthcare, 3 (2018), 45–61. https://doi.org/10.4018/IJBDAH.2018070104 doi: 10.4018/IJBDAH.2018070104
![]() |
[9] |
Y. Zhang, M. Qiu, C. W. Tsai, M. M. Hassan, A. Alamri, Health-CPS: Healthcare cyber-physical system assisted by cloud and big data, IEEE Syst. J., 11 (2017), 88–95. https://doi.org/10.1109/JSYST.2015.2460747 doi: 10.1109/JSYST.2015.2460747
![]() |
[10] |
M. S. Kang, Y. G. Jung, Big data analysis using Python in agriculture forestry and fisheries, Int. J. Adv. Smart Converg., 5 (2016), 47–50. https://doi.org/10.7236/IJASC.2016.5.1.47 doi: 10.7236/IJASC.2016.5.1.47
![]() |
[11] | C. Cao, S. Kan, X. Dong, Preoperative staging and postoperative pathological staging of esophageal carcinoma by endoscopic ultrasonography, Modern Digest. Interventional Diagn. Treat., 022 (2017), 1–4 |
[12] |
T. L. Tio, F. C. A. D. H. Jager, P. Coene, Esophageal carcinoma: Clinical TNM staging with endosonography and computed tomography, J. Canad. De Gastroenterol., 4 (2016), 603–607. https://doi.org/10.1155/1990/835307 doi: 10.1155/1990/835307
![]() |
[13] | G. Li, L. Qian, Application of diffusion weighted magnetic resonance imaging in radiation for esophageal cancer, Chin. J. Radiat. Oncol., 26 (2017), 239–242 |
[14] |
M. Li, H. Xie, F. Zhen, H. Wang, Z. Peng, L. Xu, Clinicopathologic factors associated with pathologic upstaging in patients clinically diagnosed stage T2N0M0 squamous cell esophageal carcinoma, J. Cancer Res. Ther., 16 (2020), 1106–1111. https://doi.org/10.4103/jcrt.JCRT_1171_19 doi: 10.4103/jcrt.JCRT_1171_19
![]() |
[15] |
X. Wang, M. Liu, Y. Wang, J. Fan, E. Meijering, A 3D tubular flux model for centerline extraction in neuron volumetric images, IEEE Trans. Med. Imaging, 41 (2022), 1069–1079. https://doi.org/10.1109/TMI.2021.3130987 doi: 10.1109/TMI.2021.3130987
![]() |
[16] |
S. Ren, D. K. Jain, K. Guo, T. Xu, T. Chi, Towards efficient medical lesion image super-resolution based on deep residual networks, Signal Process. Image Commun., 75 (2019), 1–10. https://doi.org/10.1016/j.image.2019.03.008 doi: 10.1016/j.image.2019.03.008
![]() |
[17] |
M. I. Razzak, M. Imran, G. Xu, Big data analytics for preventive medicine, Neural Comput. Appl., 32 (2020), 4417–4451. https://doi.org/10.1007/s00521-019-04095-y doi: 10.1007/s00521-019-04095-y
![]() |
[18] |
J. H. Hong, H. H. Kim, E. J. Han, J. H. Byun, H. S. Jang, E. K. Choi, et al., Total lesion glycolysis using 18F-FDG PET/CT as a prognostic factor for locally advanced esophageal cancer, J. Korean Med. Sci., 31 (2016), 39–46. https://doi.org/10.3346/jkms.2016.31.1.39 doi: 10.3346/jkms.2016.31.1.39
![]() |
[19] |
T. DaVee, J. A. Ajani, J. H. Lee, Is endoscopic ultrasound examination necessary in the management of esophageal cancer?, World J. Gastroenterol., (2017), 751–762. https://doi.org/10.3346/jkms.2016.31.1.39 doi: 10.3346/jkms.2016.31.1.39
![]() |
[20] |
L. Wu, C. Wang, X. Tan, Z. Cheng, K. Zhao, L.Yan, et al., Radiomics approach for preoperative identification of stages Ⅲ and ⅢIV of esophageal cancer, Chin. J. Cancer Res., 30 (2018), 96–405. https://doi.org/10.21147/j.issn.1000-9604.2018.04.02 doi: 10.21147/j.issn.1000-9604.2018.04.02
![]() |
Parameter | Number of patients |
Age | |
≥ 65 | 85 (85%) |
< 65 | 15 (15%) |
Gender | |
Male | 65 (65%) |
Female | 35 (35%) |
Number of metastases | |
Simple | 44 (44%) |
Multiple | 56 (56%) |
Serum CEA | |
≤ 5 ng/mL | 38 (38%) |
> 5 ng/mL | 62 (62%) |
T stage | |
2 | 20 (20%) |
3 | 64 (64%) |
4 | 16 (16%) |
N stage | |
0 | 14 (14%) |
1 | 26 (26%) |
2 | 60 (60%) |
Equipment | TR | TE | Thickness | Time |
TW1 | 588 | 68 | 3 | 0 |
TW2 | 700 | 85 | 3 | 0 |
TW2 | 800 | 95 | 5 | 20 |
MR staging | Mucosa | Submucosa | Muscularis propria | Adventitia |
Mucosa | 9 | 3 | 0 | 0 |
submucosa | 4 | 18 | 2 | 1 |
muscularis propria | 1 | 3 | 15 | 2 |
adventitia | 1 | 1 | 1 | 23 |
Diagnostic index | Mucosa | Submucosa | Muscularis propria | Adventitia |
Sensitivity | 75.4 | 78.3 | 98 | 100 |
Specificity | 95.4 | 89.3 | 93.9 | 96.4 |
Accuracy | 92.6 | 86.8 | 67.7 | 93.8 |
False positive | 3.5 | 5.8 | 6.4 | 0 |
False negative | 22.8 | 20.6 | 4.6 | 2.4 |
Factors | T2 | T3 | T4 | P | |
Upper thoracic segment | 3 | 2 | 1 | 0.882 | |
Lesion location | Middle chest | 5 | 9 | 8 | 0 |
Lower thoracic segment | 4 | 5 | 3 | 0 | |
Medullary type | 15 | 9 | 6 | 0.457 | |
Pathological type | Ulcerative type | 2 | 3 | 4 | 0 |
Mushroom umbrella type | 2 | 2 | 3 | 0 |
Parameter | Number of patients |
Age | |
≥ 65 | 85 (85%) |
< 65 | 15 (15%) |
Gender | |
Male | 65 (65%) |
Female | 35 (35%) |
Number of metastases | |
Simple | 44 (44%) |
Multiple | 56 (56%) |
Serum CEA | |
≤ 5 ng/mL | 38 (38%) |
> 5 ng/mL | 62 (62%) |
T stage | |
2 | 20 (20%) |
3 | 64 (64%) |
4 | 16 (16%) |
N stage | |
0 | 14 (14%) |
1 | 26 (26%) |
2 | 60 (60%) |
Equipment | TR | TE | Thickness | Time |
TW1 | 588 | 68 | 3 | 0 |
TW2 | 700 | 85 | 3 | 0 |
TW2 | 800 | 95 | 5 | 20 |
MR staging | Mucosa | Submucosa | Muscularis propria | Adventitia |
Mucosa | 9 | 3 | 0 | 0 |
submucosa | 4 | 18 | 2 | 1 |
muscularis propria | 1 | 3 | 15 | 2 |
adventitia | 1 | 1 | 1 | 23 |
Diagnostic index | Mucosa | Submucosa | Muscularis propria | Adventitia |
Sensitivity | 75.4 | 78.3 | 98 | 100 |
Specificity | 95.4 | 89.3 | 93.9 | 96.4 |
Accuracy | 92.6 | 86.8 | 67.7 | 93.8 |
False positive | 3.5 | 5.8 | 6.4 | 0 |
False negative | 22.8 | 20.6 | 4.6 | 2.4 |
Factors | T2 | T3 | T4 | P | |
Upper thoracic segment | 3 | 2 | 1 | 0.882 | |
Lesion location | Middle chest | 5 | 9 | 8 | 0 |
Lower thoracic segment | 4 | 5 | 3 | 0 | |
Medullary type | 15 | 9 | 6 | 0.457 | |
Pathological type | Ulcerative type | 2 | 3 | 4 | 0 |
Mushroom umbrella type | 2 | 2 | 3 | 0 |