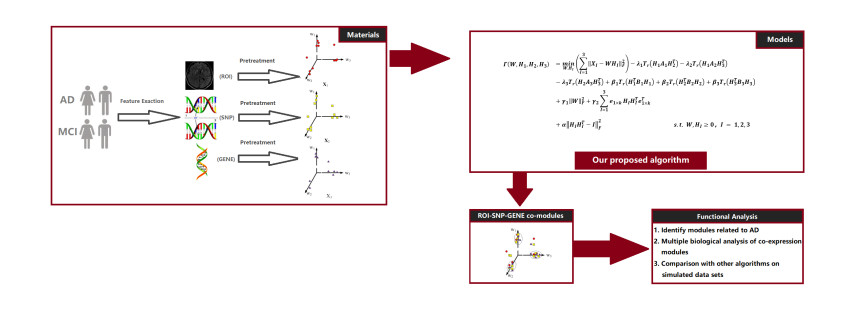
Based on the mining of micro- and macro-relationships of genetic variation and brain imaging data, imaging genetics has been widely applied in the early diagnosis of Alzheimer's disease (AD). However, effective integration of prior knowledge remains a barrier to determining the biological mechanism of AD. This paper proposes a new connectivity-based orthogonal sparse joint non-negative matrix factorization (OSJNMF-C) method based on integrating the structural magnetic resonance image, single nucleotide polymorphism and gene expression data of AD patients; the correlation information, sparseness, orthogonal constraint and brain connectivity information between the brain image data and genetic data are designed as constraints in the proposed algorithm, which efficiently improved the accuracy and convergence through multiple iterative experiments. Compared with the competitive algorithm, OSJNMF-C has significantly smaller related errors and objective function values than the competitive algorithm, showing its good anti-noise performance. From the biological point of view, we have identified some biomarkers and statistically significant relationship pairs of AD/mild cognitive impairment (MCI), such as rs75277622 and BCL7A, which may affect the function and structure of multiple brain regions. These findings will promote the prediction of AD/MCI.
Citation: Wei Kong, Feifan Xu, Shuaiqun Wang, Kai Wei, Gen Wen, Yaling Yu. Application of orthogonal sparse joint non-negative matrix factorization based on connectivity in Alzheimer's disease research[J]. Mathematical Biosciences and Engineering, 2023, 20(6): 9923-9947. doi: 10.3934/mbe.2023435
[1] | Wei Yin, Tao Yang, GuangYu Wan, Xiong Zhou . Identification of image genetic biomarkers of Alzheimer's disease by orthogonal structured sparse canonical correlation analysis based on a diagnostic information fusion. Mathematical Biosciences and Engineering, 2023, 20(9): 16648-16662. doi: 10.3934/mbe.2023741 |
[2] | Zhi Yang, Kang Li, Haitao Gan, Zhongwei Huang, Ming Shi, Ran Zhou . An Alzheimer's Disease classification network based on MRI utilizing diffusion maps for multi-scale feature fusion in graph convolution. Mathematical Biosciences and Engineering, 2024, 21(1): 1554-1572. doi: 10.3934/mbe.2024067 |
[3] | Dominique Duncan, Thomas Strohmer . Classification of Alzheimer's disease using unsupervised diffusion component analysis. Mathematical Biosciences and Engineering, 2016, 13(6): 1119-1130. doi: 10.3934/mbe.2016033 |
[4] | Jiyang Yu, Baicheng Pan, Shanshan Yu, Man-Fai Leung . Robust capped norm dual hyper-graph regularized non-negative matrix tri-factorization. Mathematical Biosciences and Engineering, 2023, 20(7): 12486-12509. doi: 10.3934/mbe.2023556 |
[5] | Atsushi Kawaguchi . Network-based diagnostic probability estimation from resting-state functional magnetic resonance imaging. Mathematical Biosciences and Engineering, 2023, 20(10): 17702-17725. doi: 10.3934/mbe.2023787 |
[6] | Yingying Xu, Songsong Dai, Haifeng Song, Lei Du, Ying Chen . Multi-modal brain MRI images enhancement based on framelet and local weights super-resolution. Mathematical Biosciences and Engineering, 2023, 20(2): 4258-4273. doi: 10.3934/mbe.2023199 |
[7] | Jianhua Song, Lei Yuan . Brain tissue segmentation via non-local fuzzy c-means clustering combined with Markov random field. Mathematical Biosciences and Engineering, 2022, 19(2): 1891-1908. doi: 10.3934/mbe.2022089 |
[8] | Zijian Wang, Yaqin Zhu, Haibo Shi, Yanting Zhang, Cairong Yan . A 3D multiscale view convolutional neural network with attention for mental disease diagnosis on MRI images. Mathematical Biosciences and Engineering, 2021, 18(5): 6978-6994. doi: 10.3934/mbe.2021347 |
[9] | Xiuhan Li, Rui Feng, Funan Xiao, Yue Yin, Da Cao, Xiaoling Wu, Songsheng Zhu, Wei Wang . Sparse reconstruction of magnetic resonance image combined with two-step iteration and adaptive shrinkage factor. Mathematical Biosciences and Engineering, 2022, 19(12): 13214-13226. doi: 10.3934/mbe.2022618 |
[10] | Xinlin Liu, Viktor Krylov, Su Jun, Natalya Volkova, Anatoliy Sachenko, Galina Shcherbakova, Jacek Woloszyn . Segmentation and identification of spectral and statistical textures for computer medical diagnostics in dermatology. Mathematical Biosciences and Engineering, 2022, 19(7): 6923-6939. doi: 10.3934/mbe.2022326 |
Based on the mining of micro- and macro-relationships of genetic variation and brain imaging data, imaging genetics has been widely applied in the early diagnosis of Alzheimer's disease (AD). However, effective integration of prior knowledge remains a barrier to determining the biological mechanism of AD. This paper proposes a new connectivity-based orthogonal sparse joint non-negative matrix factorization (OSJNMF-C) method based on integrating the structural magnetic resonance image, single nucleotide polymorphism and gene expression data of AD patients; the correlation information, sparseness, orthogonal constraint and brain connectivity information between the brain image data and genetic data are designed as constraints in the proposed algorithm, which efficiently improved the accuracy and convergence through multiple iterative experiments. Compared with the competitive algorithm, OSJNMF-C has significantly smaller related errors and objective function values than the competitive algorithm, showing its good anti-noise performance. From the biological point of view, we have identified some biomarkers and statistically significant relationship pairs of AD/mild cognitive impairment (MCI), such as rs75277622 and BCL7A, which may affect the function and structure of multiple brain regions. These findings will promote the prediction of AD/MCI.
Alzheimer's disease (AD) is an irreversible neurodegenerative disease caused by complex nerve cell loss. In recent years, imaging genetics has been an emerging discipline applied to mental diseases, and it is used to analyze the effects of imaging features and genetic features on diseases and to further study the new relationship between genetic factors and human brain diseases.
To explore the relationship between genetic variation and brain function and structure, scholars are committed to using different algorithm models to dig deeper between macro and micro information. canonical correlation analysis (CCA) is a commonly used algorithm for mining data association relationships [1]. CCA can use the correlation between multiple data to reflect the overall correlation between indicators. However, with the CCA algorithm, only one pair of typical correlation variables can be found at a time [2], and simple correlation analysis may not consider the potential correlation between the original data. The result may have a false positive, weakening the biological meaning. We have introduced the non-negative matrix factorization (NMF) algorithm to solve this problem.
As we all know, NMF is a simple low-dimensional dimensionality reduction model that can use data from multiple modalities to extract important features, and to analyze the interactions between different regions of interest (ROIs) and single nucleotide polymorphisms (SNPs) or genes so that the analysis is no longer limited to a pair of correlations. Variables and the potential relationship of the original data can be defined as prior information, further revealing the biological significance generated. In the early days, traditional NMF was often used to process data of different modalities to discover the complex biological mechanisms hidden in multi-modal data. Zhang et al. proposed the joint non-Negative matrix factorization (JNMF) algorithm to fuse multi-dimensional genomic data of cancer [3]. However, the results are subject to certain restrictions due to the lack of prior knowledge of the original data. Zhang et al. proposed the joint sparse network regularization constraint NMF (JSNMNMF) [4], combining the correlation information between data of different modalities. We can discover the potential associations between different data by constructing the adjacency matrix as prior knowledge and fusing it into the NMF algorithm.
Since previous studies have used bimodal datasets to mine the biological mechanism, Deng et al. combined the prior knowledge to construct a ceRNA network with three types of RNA data from lung cancer to make its biological explanation more convincing [5]. Since the group-level information among multi-modal imaging genetics data is easily overlooked, which may include important information related to diseases, Wang et al. by integrating the available group-level structural information of FMRI data, SNP genetic data and DNA methylation data, propose a group sparse joint non-negative matrix factorization model [6], which identifies important biomarkers related to the relationship with schizophrenia. In addition, Peng et al. introduced orthogonal constraints based on a matrix W and proposed a group sparse algorithm for JNMF on orthogonal subspaces [2], which makes the extracted features more accurate. In order to control the sparseness of the base matrix W and the coefficient matrix H to approximate the high-dimensional data in the low-dimensional space, Kim and Park proposed an alternating non-negative constrained least-squares method that led to a convergent sparse NMF algorithm; it showed a good result [7]. However, they did not consider the prior connection information of the brain. Later, Deng et al. proposed a multi-constrained JNMF (MCJNMF) algorithm, which used FDG-PET and DNA methylation to identify important modules related to lung metastasis [8]; they found important modules related to lung metastasis. Recently, in order to further explore the strong correlation between image and genetic variation, Deng et al. introduced orthogonal constraints on the coefficient matrix H and proposed a multi-dimensional constraint combined non-negative matrix factorization algorithm model [9]. Progress has been made in research related to sarcoma or lung metastasis. In imaging genetics research, the brain's connectivity information may contain important data mining information. Kim et al. proposed a penalty based on brain connectivity to integrate different data as prior knowledge into sparse CCA (SCCA), namely JCB-SCCA [10], which revealed the most significant features between SNPs and brain regions associated with Parkinson's disease. Recently, Wei et al. added the connectivity information of the brain to JSNMNMF and proposed the joint connection sparse NMF (JCB-SNMF) [11], which significantly improved the performance and bio-interpretability of the algorithm. However, it ignored the importance of obtaining the coefficient matrix H through the NMF algorithm. Improving the coefficient matrix H can make the experimental results more accurate and credible.
In this paper, we propose an orthogonal sparse JNMF (OSJNMF-C) method based on connectivity; it has been applied to the structural magnetic resonance imaging (sMRI), SNP and gene expression data of AD patients to explore the efficient biomarkers for AD's early diagnosis. First of all, in order to find the basic potential associations between different data, we define the Pearson correlation coefficient matrix between different data as prior knowledge, using priori knowledge as a regularization constraint for NMF, so that we can get the features and that the data analysis is more convincing. Then, in order to enable NMF to discover some important basis vectors and screen out important features, we use the method in the sparse NMF algorithm of the alternating non-negative constraint least-squares method to control the sparseness of the basis matrix W and add the l1−norm The number of constraints is used to control the sparsity of the coefficient matrix H. In addition, we added brain connection information about brain regions and genetic attributes; we also used GraphNet regularizers to enhance the accuracy and noise resistance of the model. Finally, the orthogonal constraint has the advantage of high time complexity when solving the optimal value, the orthogonal constraint can minimize the redundancy between different bases to obtain better local features. An orthogonal constraint adds the sparsity of the matrix obtained by decomposing the original data, and it can integrate biological information more efficiently. Therefore, we impose orthogonal constraints on H.
The results show that the co-expression module found by our algorithm contains AD and other diseases. Through a variety of biological analyses (such as GO enrichment analysis, PPI network, etc.) of the genes selected in the co-expression module, the influence or changes of these genes that are found in certain analysis functions have direct or indirect effects on AD. In addition, this study also found risk brain regions and SNP sites related to AD (such as the parahippocampal gyrus, rs449647) and identified risk genes such as APOE. Some unproven genes or brain regions may be the potential for AD and mild cognitive impairment (MCI) biomarkers.
A brief description of the proposed algorithm in this study is given in Figure 1. In the framework, we can see that three different types of datasets, including sMRI, SNP and gene expression data, were integrated to identify their co-expression modules with various biological analyses. In order to reduce the dimensionality of multi-modal data simultaneously, the core of the method used in this paper is the JNMF algorithm which is used to find the co-expression module of different data. The algorithm model we propose is to add a variety of regularization constraints based on JNMF. The addition of the constructed adjacency matrix, l1−norm, brain connectivity information and orthogonal constraints on H makes the features we extract more accurate and sparse, and the brain connectivity information improves the noise resistance of the algorithm. In addition, we briefly introduce the ROI-SNP-GENE co-expression module. The characteristic matrices of ROI, SNP and GENE are projected to a common characteristic space, and heterogeneous variables with more significant coefficients in the same projection direction constitute a standard module.
In order to decompose the matrix more vividly, we use the following description. JNMF decomposes multi-modal imaging genetics data into a new common orthogonal space, where w1, w2 and w3 represent base vectors. Triangles, squares and circles represent different forms of data. In Figure 2(a), the original data X1, X2, X3, ..., XK, are projected into their respective base vectors. In Figure 2(b), the multi-modal data XK is projected into the same set of base vectors. Therefore, our method can use more datasets for matrix decomposition to study the corresponding topics.
Assume that X1, X2 and X3 are three different types of data from the same sample, namely sMRI, SNP, and gene expression data. The JNMF model is
minW,HI(∑3I=1‖XI−WHI‖2F)=∑3I=1[Tr(XIXI)T−2Tr(XIHTIWT)+Tr(WHIHTIWT)]s.t.W,HI≥0,I=1,2,3 | (2.1) |
The data feature matrix XI∈RmnI(I=1,2,3) is decomposed into a common base matrix W∈Rmk and a coefficient matrix HI∈RknI. Among them, m and n are the number of samples and the number of features, respectively.
Existing research shows that the correlation between data can be used as prior information to improve the model's accuracy. By calculating the absolute value of the Pearson correlation coefficient between the two sets of data, the adjacency matrix constructed by the correlation coefficient is defined by the prior information matrices A1,A2 and A3, and the results are as follows:
∑ijaij(hi1)Thj2=Tr(H1A1HT2)∑ijbij(hi1)Thj3=Tr(H1A2HT3)∑ijcij(hi1)Thj3=Tr(H2A3HT3) | (2.2) |
Among them, a,b and c are the elements of the adjacency matrix. 1 indicates that there is a correlation. The correlation may include various regulatory functions or interactions between proteins; 0 indicates irrelevance; hi indicates the i-th row of H, and hj indicates the j-th column of H. A1 is the prior matrix constructed by using MRI and SNP data, A2 is the prior matrix of MRI and genetics data and A3 is the adjacency matrix of SNP and genetics data. Therefore, even if there are various unknown correlations between the two kinds of data, they may be placed in our expected co-expression module, reducing the space for our algorithm to search for features and improving our computational efficiency.
Connectivity-based methods can quantify meaningful neurobiological measurements and are a good source of information for regularization. If a certain node between the brain area and the SNP site is highly connected, the GraphNet regularization program will force the corresponding elements of the canonical vector to be similar [12]. Therefore, we chose to introduce a connectivity penalty method based on GraphNet regularization as follows:
P(H1)=∑p,qLH1(p,q)(H1p−H1q)=Tr(H1TB1H1)P(H2)=∑p,qLH2(p,q)(H2p−H2q)=Tr(H2TB2H2)P(H3)=∑p,qLH3(p,q)(H3p−H3q)=Tr(H3TB3H3) | (2.3) |
Among them, B1,B2 and B3 represent the Laplacian matrices of X1,X2 and X3, respectively.
To filter out the important features that we need, we should control the sparseness of the base matrix W. It has another advantage that it improves the efficiency of our algorithm. Therefore, this study uses the alternating non negative constraint least square method to control the sparsity of W and impose l1−norm constraints on H. In addition, to effectively integrate biological information, we chose to impose orthogonal constraints on H Orthogonal constraints can not only reduce the redundancy between different bases, but they can also ensure that the matrix obtained by the feature matrix decomposition has sparseness, thus finding more biologically significant features. In the end, the objective function we get is as follows:
Γ(W,H1,H2,H3)=minW,HI(∑3I=1‖XI−WHI‖2F)−λ1Tr(H1A1HT2)−λ2Tr(H1A2HT3)−λ3Tr(H2A3HT3)+β1Tr(HT1B1H1)+β2Tr(HT2B2H2)+β3Tr(HT3B3H3)+γ1‖W‖2F+γ2∑3I=1e1×kHIHTIeT1×k+α‖HIHTI−I‖2F | (2.4) |
Based on Eq (2.1), we can get the final objective function as follows:
Γ(W,H1,H2,H3)=∑3I=1[Tr(XIXI)T−2Tr(XIHTIWT)+Tr(WHIHTIWT)]−λ1Tr(H1A1HT2)−λ2Tr(H1A2HT3)−λ3Tr(H2A3HT3)+β1Tr(HT1B1H1)+β2Tr(HT2B2H2)+β3Tr(HT3B3H3)+γ1‖W‖2F+γ2∑3I=1e1×kHIHTIeT1×k+α‖HIHTI−I‖2F | (2.5) |
Among them, the parameters λ1,λ2 and λ3 are the regular constraints of the adjacency matrix, β1,β2 and β3 are the constraint coefficients of the Laplacian matrix, γ1 is used to limit the growth of W, γ2 is used to constrain H and α is the orthogonal constraint hyperparameters.
Effectively solve the objective function according to the update rule used by the MCJNMF algorithm. Let φij and ϕIij be [Wij≥0 and (HI)ij≥0]; the Lagrangian multiplier L can be expressed as follows:
L(W,H1,H2,H3)=Γ(W,H1,H2,H3)+Tr(ΨWT)+3∑I=1Tr(ΦHTI)Ψ=[φij],ΦI=[ϕIij] | (2.6) |
The partial derivatives of L with respect to W and HI can be obtained:
∂L∂W=∑3I=1[−2XIHTI+2WHIHTI]+2γ1W+Ψ∂L∂H1=−2WTX1+2WTWH1+λ1H2AT1+λ2H3AT2+2γ2ek×kH1+2β1H1C1+4αH1HT1H1−4αH1+Φ1∂L∂H2=−2WTX2+2WTWH2+λ1H1A1+λ3H3AT3+2γ2ek×kH2+2β2H2C2+4αH2HT2H2−4αH2+Φ2∂L∂H3=−2WTX3+2WTWH3+λ2H1A2+λ3H2A3+2γ2ek×kH3+2β3H3C3+4αH3HT3H3−4αH3+Φ3 | (2.7) |
Based on the KKT condition, ΨijWij=0 and ΦIij(HI)ij=0. We can get the equations of Wij and (HI)ij as follows:
(W)ij=(W)ij(X1HT1+X2HT2+X3HT3)ij(WH1HT1+WH2HT2+WH3HT3+γ1W)ij(H1)ij=(H1)ij(WTX1+2αH1+λ12H2AT1+λ22H3AT2)ij(WTWH1+2αH1HT1H1+β1H1B1+γ2ek×kH1)ij(H2)ij=(H2)ij(WTX2+2αH2+λ12H1A1+λ32H3AT3)ij(WTWH2+2αH2HT2H2+β2H2B2+γ2ek×kH2)ij(H3)ij=(H3)ij(WTX3+2αH3+λ22H1B2+λ32H2A3)ij(WTWH3+2αH3HT3H3+β3H3B3+γ2ek×kH3)ij | (2.8) |
Algorithm 1: Algorithm for OSJNMF-C. |
Input: Input normalized data X1,X2,X3,βi,λi(i=1,2,3),α,γ1,γ2,τ. Output: Base matrix W and coefficient matrix HI(I=1,2,3). Randomly initialize a set of non-negative W and HI(I=1,2,3),t=1. while: 1 do ![]() |
After solving the above OSJNMF-C algorithm, we decompose the characteristic matrices X1,X2 and X3 of the MRI, SNP and GENE data by applying non-negative matrix decomposition to obtain the base matrix W and the coefficient matrix H. The base matrix W is a common matrix shared by the three data types of X1,X2 and X3, and H1,H2 and H3 represent their respective coefficient matrices. In order to find the weights corresponding to the salient features of each row of W, we use Z-score to calculate the Z-score of each element in each row of H1,H2 and H3. The calculation formula for Z-score is as follows:
Zij=(hij−ui)/σi | (2.9) |
In Eq (2.9), hij, μi and σi respectively represent the element in HI, the average value of feature j in HI and the standard deviation. Then, to determine which elements are eligible to be assigned to the module, a standard value T that can be assigned is set. If its Z-score is greater than the set standard value T, it can be assigned to the module.
Next, the proposed method is used by us to evaluate the importance of the co-expression module; we also use permutation tests to estimate the P-value of the identified modules. We assume that PS=[p1,p2,...,pl1], QS=[q1,q2,...,ql2] and RS=[r1,r2,...,rl3], where pg,qt and re are the column vectors from X1,X2 and X3, respectively. To sum up, the average correlation between the three types of datasets in a module ρ∗ can be expressed as follows:
ρ∗=13(1l1l2∑l1s=1∑l2t=1(ρ(ps,qt))2+1l1l3∑l1s=1∑l2e=1(ρ(ps,re))2+1l2l3∑l2t=1∑l3e=1(ρ(qt,re))2) | (2.10) |
We randomly change the order of the row vectors of the matrices PS and QS in the S-th module, and this process is repeated Δ times. For each permutation, ρ∗θ is the new average correlation coefficient calculated by Eq (2.10) after arranging the rows of the matrices PS and QS. The importance of test statistics can be evaluated by using the following P-value:
P−value=|{θ|ρ∗θ⩾ρ∗,θ=1,2,⋯,Δ}|Δ | (2.11) |
where |⋅| represents the number of times ρ∗θ≥ρ∗; modules with a P-value less than 0.05 are considered significant.
The data we use were downloaded from the ADNI database (http://ADNI.loni.usc.edu/). We used the following three types of data: sMRI, SNP and gene expression data to verify our proposal. These three types of data come from a common 386 samples. We downloaded the imaging and genotyping data of 386 participants, including 113 healthy controls (HCs), 248 MCIs and 25 ADs. The detailed information is shown in Table 1.
Groups | AD | MCI | HC |
Number | 25 | 248 | 113 |
Gender (M/F) | 10/15 | 133/115 | 58/55 |
Age (mean ± std) | 75.99 ± 10.22 | 71.9 ± 7.34 | 75.06 ± 5.68 |
MMSE (mean) | 20.48 | 27.90 | 29.00 |
Note: HC = healthy control group, MCI = mild cognitive impairment, AD = Alzheimer's disease. |
As with our previous preprocessing method, we first, performed head movement correction on the MRI data downloaded from ADNI1, using the CAT in the SPM software package to perform image segmentation to obtain 140 ROI phenotypes. Then, we preprocessed 386 samples through PLINK [13], screened the genotype data and generated 5947 SNP data. Finally, we used the LIMMA software package to screen for genes with significant differential expression [14], and 1477 genes were obtained (P<0.01).
After the preprocessing, three kinds of raw data with 386 samples were obtained. In the experiment, we normalized the obtained data and added the feature matrices of the three sets of data for two types of samples from people suffering from AD and MCI to our algorithm for verification. The HC group was used as the control group for preprocessing SNP and gene expression data. We integrated the AD and MCI sets of data, and we obtained the feature matrices X1,X2 and X3 of the sMRI, SNP and gene expression data for the three modalities of our input data. Their sizes are 273 × 140,273 × 2956 and 273 × 4026.
Parameter selection is very important for our research. In order to select more qualified parameters, we conducted experiments on real datasets in this section. First, the selection of iteration times in the experiment is very important. We think that the convergence is that the error gradually decreases and tends to be stable with the increase in convergence times, which is independent of the number of times, so it was set to 350 times a set of non-negative W and HI (I=1,2,3) was initialized randomly by us, and we randomly selected a set of parameters for 350 iterations; the objective function obtained is shown in Figure 3A. It can be seen in Figure 3A that our algorithm has convergence. As the number of iterations increases, the value of the objective function gradually decreases until it becomes stable. Since K≪m, the upper limit of K was set to 80 by us and iterated 350 times with different K values. The results in Figure 3B show that as the value of K increases, the error gradually decreases; thus, increasing the K value can indeed result in better relative error convergence. However, there has always been no clear standard for choosing the K value. Experiments have shown that if the K value we choose is too small, it will lead to relatively large correlation errors and make the features extracted by our algorithm inaccurate. We should choose a suitable K value. If the K value is too large, the matrix will be over-decomposed, the final result will deviate from the purpose of our initial matrix low-rank decomposition and the effect of dimensionality reduction is greatly reduced, which will affect the extraction of hidden structures in the data. Therefore, we set K≈0.3∗min(m,n1,n2,n3), that is, K=40.
The choice of hyperparameters has always been a problem that machine learning needs to overcome. For our research, the optimal parameters βi,λi(i=1,2,3),α,γ1 and γ2 in our proposed algorithm need to be selected by us. We set K=40 and adjusted βi,λi,α,γ1 and γ2 from the set [0.0001, 0.0005, 0.001, 0.005, 0.01]; each set of parameters was iterated 350 times. Figure 3C shows the relative errors obtained by substituting 3125 sets of hyperparameters into our algorithm. Through screening, we found that when the parameter is the 423rd group of 3125 groups, the correlation error reaches the minimum value of 0.3855; the set of regularization parameters with the smallest reconstruction error was selected by us and the subsequent analysis and research were carried out. That is, λi=0.001,βi=0.0005,α=0.01,γ1=0.005 and γ2=0.0001. Finally, to prevent the randomness of the initial value from causing the objective function to fall into a local minimum, we selected 100 sets of different initialization values W and HI (I=1,2,3) to iterate our algorithm 350 times. As shown in Figure 3D, among these 100 groups of initialization values, the minimum value was 0.3871 for the 39th group. Therefore, the initial values of W and HI (I=1,2,3) in the 39th group were selected by us for subsequent analysis and research.
After selecting all of the required hyperparameters, we applied the W and HI values obtained to the ADNI database for real data experiments; we ran our code on an AMD Ryzen5 4600H CPU with the Windows 10 platform 350 times; the calculation time was 111.2013 seconds. We got K (K=40) co-expression modules, but we found that one module did not include any SNPs, so we kept the final 39 modules. Among them, the average numbers of features of ROI, SNP and gene data were 27,228 and 424 respectively.
As shown in Figure 4, we have verified the reliability of the OSJNMF-C algorithm by calculating the Pearson correlation coefficient between the original matrix and the reconstructed matrix of the three kinds of data. It can be seen in the histogram in Figure 4A that the reconstruction of the three types of data and the original matrix are almost the same; especially the correlations between the reconstructions of ROIs and genetics data and the original matrix were as high as 0.9953 and 0.9472. In addition, Figure 4(B–D) shows three data-related distributions. It can be seen in the figure that the difference between the reconstructed matrix and the original matrix after our algorithm is decomposed is very small, which further proves the robustness of our algorithm.
The modules with P<0.05 were obtained by using Eq (2.11), as shown in Table 2. Then we drew the Venn diagrams for these three types of data and compared the escape rates of these three data features in different modules (escape rate: the ratio of the number of modules that do not overlap with the other four modules to the total number of modules). Figure 5 shows that the first module has the lowest comprehensive escape rate among the three data types, so the first module was selected for in-depth analysis.
Module | ROI | SNP | GENE | P-value |
1 | 34 | 88 | 388 | 2.73E-108 |
3 | 22 | 175 | 344 | 0.0021 |
19 | 26 | 313 | 400 | 0.0018 |
25 | 33 | 227 | 497 | 2.30E-42 |
38 | 29 | 365 | 574 | 1.98E-11 |
The results of OSJNMF-C showed that many brain regions and genes with significant biological processes of neurodegenerative diseases were found. Table 3 shows the relevant brain regions identified from the SNPs in Module 1, including the inferior temporal gyrus, lingual gyrus, superior frontal gyrus, middle temporal gyrus, supra marginal gyrus, parahippocampus gyrus, superior temporal gyrus, temporal pole, posterior central gyrus, anterior central gyrus, angular gyrus, occipital poles and other brain regions, many of which are closely related to AD, such as the parahippocampal gyrus, superior temporal gyrus, inferior temporal gyrus and superior frontal gyrus [15]. Studies have shown that the severity of neurodegenerative diseases is related to changes in the parahippocampal gyrus connectivity, which helps to understand the cognitive decline and impaired brain activity in AD/MCI [16]. The inferior temporal gyrus plays an important role in speech fluency, which is a cognitive function affected in the early stage of AD. The inferior temporal gyrus is affected in the prodromal stage of the disease and may be the basis of some early clinical dysfunctions associated with AD [17]. APOE gene methylation may not be affected by genotype, and it is related to AD pathology or the level of APOE protein in the superior frontal gyrus [18]. Using BrainNet Viewer (http://www.nitrc.org/projects/bnv/), the brain network visualization of the four abnormal brain regions listed above was drawn. As shown in Figure 6, the four brain areas are closely related to AD or neurodegenerative diseases in general [19].
Left/Right Exterior Cerebellum | Left/Right Superior Temporal Gyrus |
Left/Right Temporal Pole | Left/Right Cerebrum and Motor |
Left/Right Inferior Temporal Gyrus | Left/Right Middle Temporal Gyrus |
Left Calcarine and Cerebrum | Left Gyrus Rectus |
Left Inferior Frontal Angular Gyrus | Left Lingual Gyrus |
Left Medial Frontal Cerebrum | Left Middle Occipital Gyrus |
Left Occipital Pole | Left Parahippocampus Gyrus |
Left Posterior Orbital Gyrus | Left Superior Occipital Gyrus |
Right Angular Gyrus | Right Central Operculum |
Right Middle Cingulate Gyrus | Right Middle Frontal Gyrus |
Right Postcentral Gyrus | Right Precentral Gyrus |
Right Superior Frontal Gyrus | Right Superior Medial Frontal Gyrus |
Right Superior Parietal Lobule | Right Supramarginal Gyrus |
Right Thalamus Proper |
For the SNPs in Module 1, some risk genes were identified from SNPs: TF, BFSP2, CELF1, LOC105369536, SORL1, CD33, LINC02287 CHGA, SLC24A4, ITPK1, RIN3, CNN2, ABCA7, APOC1, CASS4, APOE, ERCC1 and POLR1G. By consulting the published literature, it was found that most of these genes are direct or indirect pathogenic factors of AD. The expression of multiple genes of CELF1 is related to each other, and the gene expression of CELF1 is closely related to the physiological state of AD [20]. The dominant AD genes of familial autosomes are ABCA7 and SORL1. At present, risk genes such as ABCA7, CASS4, CD33, CELF1, SLC24A4, SORL1 and ZCWPW1 have been discovered by genome-wide association studies. In the next-generation sequencing studies, it has been proved that ABCA7, SORL1 and APOE have a higher odds ratio (>2) in AD risk [21]. It has been demonstrated in studies of hereditary prostate dementia that symptomatic carriers of the GRN mutation have significantly reduced CHGA [22]. The gene expression of CASS4 is consistent with that in the hippocampus of AD mice, proving that the blood transcriptome may be a biomarker of AD [23]. SNPs in CASS4 and AD-related genes may contribute to the susceptibility of cognitive aging [24]. The risk factors for AD are believed to be caused by genes such as APOC1, CD33 and CNN2 [25,26]. The methylation of several CPG sites in ABCA7 is closely related to AD [27]. The changes in the microstructure of the brain explain the relationship between ITPK1 and cognition in later life. The higher the abundance of ITPK1, the slower the cognitive decline [28]. RIN3 is related to in vivo dysfunction, which can cause endocrine dysfunction in AD patients [29].
Studies have confirmed that the APOE allele is the strongest genetic risk factor for sporadic AD. It is also a genetic risk gene for cardiovascular disease, stroke and other neurodegenerative diseases [30,31]. We also found some SNP sites with significant pathological effects. These interesting findings also support the application of genetics in neurological diseases. [32] The results of the survey showed that rs449647 is an SNP site that is resistant to AD. The rs1133174-G allele (T/G/G) is associated with the atrophy of the entire brain in men and women, and the SNP locus rs1133174 is independently associated with hippocampal atrophy in men and women [33]. rs1131497 is the SNP locus of the SORL1 gene, which is a powerful evidence for testing the genetic correlation between MRI and brain aging. [34].
We performed KEGG enrichment analysis of the 388 gene expression data selected from Module 1 and screened out the pathways with significant effects to make a bubble chart, as shown in Figure 7. The KEGG enrichment results show that the genes we selected are involved in signal pathways related to AD pathology. For example, infections induced by the Epstein-Barr virus and cytomegalovirus may play a role in the development of MCI and typical immunological changes in the progression of AD [35]. The prevalence of autoimmune thyroid disease in familial AD families is very high, and there is evidence that the genetic factors leading to the development of autoimmune thyroid disease are similar to familial AD genes [36]. In addition, chemokine ligands play a key role in regulating microglia activation, and dysregulated microglia activation can lead to AD pathology [37].
A protein-protein interaction network for the 388 genes selected in Module 1 was constructed, and two modules A and B with significant correlation (higher weight) were selected for show in Figure 8. The brightness of the color of genes indicates how much the gene is associated with other genes in this network. There are 10 genes with significant interaction in the A network. IFIH1 has been identified as a mutation that may affect function in the neurodevelopmental disorder gene [38]. The ubiquitin-like protein ISG15 is stimulated by the ubiquitin-like protein interferon, and its constitutively elevated expression is a potential cause of mitochondrial autophagy defects in neurodegenerative diseases [39]. Studies have found that OAS1 is a new AD risk gene, and that Oas1a may directly respond to amyloid at the transcriptional level [40]. In the B network, the protein network encoded by CD8A and other genes connects immunity to neurodegenerative processes and highlights the potential role of HLA-A2 in maintaining synaptic AD pathogenesis [41]. The experimental results in [42] showed that deletion of CCR7 in 5xF AD transgenic mice could cause harmful neurovascular and microglial activation while increasing Aβ deposition in the brain.
For the ROI, SNPs and genes selected from the salient Module 1, we used MATLAB software to make a paired correlation heat map of ROI-SNP and ROI-GENE, as shown in Figure 9. We can see in Figure 9 that there is a strong correlation between ROI-SNP/gene pairs. The first 10 pairs of ROI-SNP and ROI-GENE with P<0.01 were found from Module 1, as shown in Table 4. Although there is no related literature report on SNPs in the ROI-SNP pair, since most ROIs have been proven to be dangerous brain areas for AD/MCI, the obtained SNP-ROI pair still has a specific reference value. The SNP site rs75277622 is associated with multiple brain regions at the same time. Among them, the precuneus has been confirmed to be related to Parkinson's disease [43], the right postcentral gyrus is the damaged hub node in AD/MCI [44] and the cognitive decline of patients. The functional connection density of the left superior occipital gyrus at rest changed significantly [45]. Additionally, rs75277622 may directly or indirectly affect the structure and function of multiple brain regions. This research has yet to be confirmed. In addition, the area where the functional connectivity of AD patients changes in the left lingual gyrus [46]. It is no coincidence that the brain regions found in the first 10 pairs of ROI-GENE and the brain regions we found directly from Module 1 contain the lingual gyrus and inferior temporal gyrus. In addition, the loss of KANK1 on chromosome 9p24.3 has a potential impact on neurodevelopment [47]. ZFX can regulate the expression of SET, and this gene has multiple functions in different diseases such as cancer and AD [48]. BCL7A strongly correlates with multiple brain regions, but the conclusion that many brain regions are affected by it remains to be confirmed.
ROI-SNP | P-Value | ROI-GENE | P-Value |
rPCu-rs75277622 | 2.39E-16 | rExtCbe-BCL7A | 3.23E-07 |
lExtCbe-rs75277622 | 2.64E-16 | lExtCbe-BCL7A | 8.91E-07 |
rPoCGy-rs75277622 | 1.31E-14 | lMedFroCbr-BCL7A | 2.73E-06 |
rExtCbe-rs75277622 | 1.01E-12 | lLinGy-PPAT | 2.86E-06 |
lMidOccGy-rs75277622 | 1.07E-12 | lLinGy-KANK1 | 3.05E-06 |
lSupOccGy-rs75277622 | 1.44E-12 | lRecGy-PPAT | 3.32E-06 |
rMidTemGy-rs2868996 | 2.21E-12 | lInfTemGy-HBG1 | 5.20E-06 |
rCenOpe-rs55663874 | 2.03E-11 | lMidTemGy-ZNF285 | 5.79E-06 |
rSupMedFroGy-rs28529220 | 7.65E-11 | lSupOccGy-BCL7A | 6.03E-06 |
lLinGy-rs11160070 | 2.26E-10 | lInfTemGy-ZFX | 7.47E-06 |
To verify the performance of our model, we conducted comparative experiments using our proposed algorithm and the degraded algorithm on the synthetic data. Due to the large sample size of our real data (m=273), we generated a set of simulated data with a small sample size (m=100). Here, we use m,n1,n2 and n3 to denote the number of samples, sMRI, SNP and the feature number of genes, respectively, where m=100,n1=650,n2=350,n3=600 and K=10. The following formula generates our simulation data:
α[n]={βi|βi=α+lηi(i=1,2,⋯,n)} | (4.1) |
α is a matrix of uniformly distributed random numbers U(0,1); ηi represents Gaussian noise, and l represents the noise level.
We compared our proposed algorithm with JNMF and JSNMNMF at different noise levels. It can be seen in Figure 10 that, after adding different levels of noise, the reconstruction error and objective function value of OSJNMF-C were significantly smaller than those for JNMF and JSNMNMF, which fully reflects the superiority of the anti-noise performance of the OSJNMF-C algorithm.
In addition, based on the simulated data experiments, we verified our algorithm performance through ablation research. As with the above simulated data experimental settings, we performed experimental reconstruction error and objective function comparison at different noise levels, as shown in Figure 11. The ablation algorithm is the algorithm without orthogonal constraints. From the results, our algorithm is superior to the ablation algorithm, and orthogonal constraints play a positive role in the algorithm. For the results obtained by the two methods, we can judge which is better by the ROI and SNP sites extracted by the two methods. The experiment shows that the ROIs not extracted by the ablation algorithm include the left/right inferior temporary gyrus and left parahoppocampus gyrus, and the SNP sites not extracted include SORL1, CD33 and POLR1G. Therefore, under orthogonal constraints, the algorithm extracts information more accurately and completely, while the ablation method lacks some important biomarkers.
In this study, we developed a flexible and efficient improved JNMF algorithm to identify and predict abnormal brain regions and risk genes related to AD, to further interpret the close relationship between imaging omics and genomics. To verify our algorithm's performance and practical significance of our algorithm, real and simulated datasets of three modes of data, i.e., sMRI, SNP and gene expression data were applied to explore the early diagnostic biomarkers of AD. The real experimental data results show that our proposed method (OSJNMF-C) can effectively mine AD- or MCI-related modules. By scoring and comparing the selected modules, we have found some brain regions closely related to the pathogenesis of AD (such as parahippocampal gyrus), as well as the risk genes (such as APOE) and SNP sites directly or indirectly affected by AD (such as rs449647). It was also found that rs75277622 may directly or indirectly affect the structure and function of multiple brain areas, and that BCL7A has a strong correlation with multiple brain areas. All of the extracted factors can provide new research ideas for the discovery of more reliable biomarkers of the early diagnosis of AD. Experimental results on the synthetic data show that our algorithm's anti-noise performance is better than that of JNMF and JSNMNMF, and that the correlation error is much smaller than for these two algorithms. In addition to the performance improvement, we proved the convergence of our algorithm. OSJNMF-C may be well applied to the analysis mode of other diseases. In the future, to more accurately identify the hidden association patterns between imaging data, genetic data and diseases, four or more types of omics data can be considered to identify and predict AD.
This work was partly supported by the National Natural Science Foundation of China (No. 61803257) and Natural Science Foundation of Shanghai (No. 18ZR1417200).
The authors declare that there is no conflict of interest.
[1] | E. Parkhomenko, D. Tritchler, J. Beyene, Sparse canonical correlation analysis with application to genomic data integration, Stat. Appl. Genet. Mol. Biol., 2009 (2009). https://doi.org/10.2202/1544-6115.1406 |
[2] |
P. Peng, Y. Zhang, Y. Ju, K. Wang, G. Li, V. Calhoun, et al., Group sparse joint non-negative matrix factorization on orthogonal subspace for multi-modal imaging genetics data analysis, IEEE/ACM Trans. Comput. Biol. Bioinf., 19 (2022), 479–490. https://doi.org/10.1109/TCBB.2020.2999397 doi: 10.1109/TCBB.2020.2999397
![]() |
[3] |
S. Zhang, C. Liu, W. Li, H. Shen, P. Laird, X. Zhou, Discovery of multi-dimensional modules by integrative analysis of cancer genomic data, Nucleic Acids Res., 40 (2012), 9379–9391. https://doi.org/10.1093/nar/gks725 doi: 10.1093/nar/gks725
![]() |
[4] |
S. Zhang, Q. Li, J. Liu, X. Zhou, A novel computational framework for simultaneous integration of multiple types of genomic data to identify microRNA-gene regulatory modules, Bioinformatics., 27 (2011), 401–409. https://doi.org/10.1093/bioinformatics/btr206 doi: 10.1093/bioinformatics/btr206
![]() |
[5] |
J. Deng, W. Kong, S. Wang, X. Mou, W. Zeng, Prior knowledge driven joint NMF algorithm for ceRNA co-module identification, Int. J. Biol. Sci., 14 (2018), 1822–1833. https://doi.org/10.7150/ijbs.27555 doi: 10.7150/ijbs.27555
![]() |
[6] |
M. Wang, T. Huang, J. Fang, V. Calhoun, Y. Wang, Integration of imaging (epi) genomics data for the study of schizophrenia using group sparse joint nonnegative matrix factorization, IEEE/ACM Trans. Comput. Biol. Bioinf., 17 (2020), 1671–1681. https://doi.org/10.1109/TCBB.2019.2899568 doi: 10.1109/TCBB.2019.2899568
![]() |
[7] |
H. Kim, H. Park, Sparse non-negative matrix factorizations via alternating non-negativity-constrained least squares for microarray data analysis, Bioinformatics, 23 (2007), 1495–1502. https://doi.org/10.1093/bioinformatics/btm134 doi: 10.1093/bioinformatics/btm134
![]() |
[8] |
J. Deng, W. Zeng, W. Kong, Y. Shi, X. Mou, J. Guo, Multi-constrained joint non-negative matrix factorization with application to imaging genomic study of lung metastasis in soft tissue sarcomas, IEEE Trans. Biomed. Eng., 67 (2020), 2110–2118. https://doi.org/10.1109/TBME.2019.2954989 doi: 10.1109/TBME.2019.2954989
![]() |
[9] |
J. Deng, W. Zeng, S. Luo, W. Kong, Y. Shi, Y. Li, et al., Integrating multiple genomic imaging data for the study of lung metastasis in sarcomas using multi-dimensional constrained joint non-negative matrix factorization, Inf. Sci., 576 (2021), 24–36. https://doi.org/10.1016/j.ins.2021.06.058 doi: 10.1016/j.ins.2021.06.058
![]() |
[10] | M. Kim, J. Won, J. Youn, H. Park, Joint-connectivity-based sparse canonical correlation analysis of imaging genetics for detecting biomarkers of Parkinson's disease, IEEE Trans. Med. Imaging, 39 (2020), 23–34. |
[11] |
K. Wei, W. Kong, S. Wang, Integration of imaging genomics data for the study of Alzheimer's disease using joint-connectivity-based sparse nonnegative matrix factorization, J. Mol. Neurosci., 72 (2022), 255–272. https://doi.org/10.1007/s12031-021-01888-6 doi: 10.1007/s12031-021-01888-6
![]() |
[12] |
K. Wei, W. Kong, S. Wang, An improved multi-task sparse canonical correlation analysis of imaging genetics for detecting biomarkers of Alzheimer's disease, IEEE Access, 9 (2021), 30528– 30538. https://doi.org/10.1109/ACCESS.2021.3059520 doi: 10.1109/ACCESS.2021.3059520
![]() |
[13] |
S. Purcell, B. Neale, K. Brown, L. Thomas, M. Ferreira, D. Bender, et al., PLINK: A tool set for whole-genome association and population-based linkage analyses, Am. J. Hum. Genet., 81 (2007), 559–575. https://doi.org/10.1086/519795 doi: 10.1086/519795
![]() |
[14] | M. Ritchie, B. Phipson, D. Wu, Y. Hu, C. Law, W. Shi, et al., Limma powers differential expression analyses for RNA-sequencing and microarray studies, Nucleic Acids Res., 43 (2015). https://doi.org/10.1093/nar/gkv007 |
[15] |
J. Liu, X. Zhang, C. Yu, Y. Duan, J. Zhuo, Y. Cui, et al., Impaired parahippocampus connectivity in mild cognitive impairment and Alzheimer's disease, J. Alzheimers Dis., 49 (2016), 1051–1064. https://doi.org/10.3233/JAD-150727 doi: 10.3233/JAD-150727
![]() |
[16] |
J. Sun, J. Maller, L. Guo, P. Fitzgerald, Superior temporal gyrus volume change in schizophrenia: a review on region of interest volumetric studies, Brain Res. Rev., 61 (2009), 14–32. https://doi.org/10.1016/j.brainresrev.2009.03.004 doi: 10.1016/j.brainresrev.2009.03.004
![]() |
[17] |
S. Scheff, D. Price, F. Schmitt, M. Scheff, E. Mufson, Synaptic loss in the inferior temporal gyrus in mild cognitive impairment and Alzheimer's disease, J. Alzheimers Dis., 24 (2011), 547–557. https://doi.org/10.3233/JAD-2011-101782 doi: 10.3233/JAD-2011-101782
![]() |
[18] |
N. Bezuch, S. Bradburn, A. Robinson, N. Pendleton, A. Payton, C. Murgatroyd, Superior frontal gyrus TOMM40-APOELocus DNA methylation in Alzheimer's disease, J. Alzheimers Dis. Rep., 5 (2021), 275–282. https://doi.org/10.3233/ADR-201000 doi: 10.3233/ADR-201000
![]() |
[19] | M. Xia, J. Wang, Y. He, BrainNet viewer: A network visualization tool for human brain connectomics, Plos One, 8 (2013). https://doi.org/10.1371/journal.pone.0068910 |
[20] | C. M. Karch, L. A. Ezerskiy, S. Bertelsen, A. M. Goate, Alzheimer's disease risk polymorphisms regulate gene expression in the ZCWPW1 and the CELF1 loci, Plos One, 11 (2016). https://doi.org/10.1371/journal.pone.0148717 |
[21] |
J. H. Kim, Genetics of Alzheimer's Disease, Dementia Neurocogn. Disord., 17 (2018), 131–136. https://doi.org/10.12779/dnd.2018.17.4.131 doi: 10.12779/dnd.2018.17.4.131
![]() |
[22] |
E. van der Ende, L. Meeter, C. Stingl, J. van Rooij, M. P. Stoop, D. Nijholt, et al., Novel CSF biomarkers in genetic frontotemporal dementia identified by proteomics, Ann. Clin. Transl. Neurol., 6 (2019), 698–707. https://doi.org/10.1002/acn3.745 doi: 10.1002/acn3.745
![]() |
[23] |
S. Ochi, J. Iga, Y. Funahashi, Y. Yoshino, K. Yamazaki, H. Kumon, et al., Identifying blood transcriptome biomarkers of Alzheimer's disease using transgenic mice, Mol. Neurobiol., 57 (2020), 4941–4951. https://doi.org/10.1007/s12035-020-02058-2 doi: 10.1007/s12035-020-02058-2
![]() |
[24] | C. Lin, E. Lin, H. Lane, Genetic biomarkers on age-related cognitive decline, Front. Psychiatry, 8 (2017). https://doi.org/10.3389/fpsyt.2017.00247 |
[25] | R. Armstrong, Risk factors for Alzheimer's disease, Folia Neuropathol., 57 (2019), 87–105. |
[26] |
M. J. Chen, S. Ramesha, L. D. Weinstock, T. W. Gao, L. Y. Ping, H. L. Xiao, et al., Extracellular signal-regulated kinase regulates microglial immune responses in Alzheimer's disease, J. Neurosci. Res., 99 (2021), 1704–1721. https://doi.org/10.1002/jnr.24829 doi: 10.1002/jnr.24829
![]() |
[27] |
A. de Roeck, C. van Broeckhoven, K. Sleegers, The role of ABCA7 in Alzheimer's disease: evidence from genomics, transcriptomics and methylomics, Acta Neuropathol., 138 (2019), 201–220. https://doi.org/10.1007/s00401-019-01994-1 doi: 10.1007/s00401-019-01994-1
![]() |
[28] |
N. Kim, L. Yu, R. Dawe, V. A. Petyuk, C. Gaiteri, P. L. Jager, et al., Microstructural changes in the brain mediate the association of AK4, IGFBP5, HSPB2, and ITPK1 with cognitive decline, Neurobiol. Aging, 84 (2019), 17–25. https://doi.org/10.1016/j.neurobiolaging.2019.07.013 doi: 10.1016/j.neurobiolaging.2019.07.013
![]() |
[29] | R. Shen, X. Zhao, L. He, Y. Ding, W. Xu, S. Lin, et al., Upregulation of RIN3 induces endosomal dysfunction in Alzheimer's disease, Transl. Neurodegener., 9 (2020). https://doi.org/10.1186/s40035-020-00206-1 |
[30] |
A. S. Pozo, S. Das, B. T. Hyman, APOE and Alzheimer's disease: advances in genetics, pathophysiology, and therapeutic approaches, Lancet Neurol., 20 (2021), 68–80. https://doi.org/10.1016/S1474-4422(20)30412-9 doi: 10.1016/S1474-4422(20)30412-9
![]() |
[31] |
M. E. Belloy, V. Napolioni, M. D. Greicius, A quarter century of APOE and Alzheimer's disease: Progress to date and the path forward, Neuron, 101 (2019), 820–838. https://doi.org/10.1016/j.neuron.2019.01.056 doi: 10.1016/j.neuron.2019.01.056
![]() |
[32] |
J. Bratosiewicz-Wasik, P. P. Liberski, B. Peplonska, M. Styczynska, J. Smolen-Dzirba, M. Cycon, et al., Regulatory region single nucleotide polymorphisms of the apolipoprotein E gene as risk factors for Alzheimer's disease, Neurosci. Lett., 684 (2018), 86–90. https://doi.org/10.1016/j.neulet.2018.07.010 doi: 10.1016/j.neulet.2018.07.010
![]() |
[33] | A. A. Assareh, O. Piguet, T. C. Lye, K. A. Mather, G. A. Broe, P. R. Schofield, et al., Association of SORL1 gene variants with hippocampal and cerebral atrophy and Alzheimerµs disease, Curr. Alzheimer Res., 11 (2014), 558–563. |
[34] | S. Seshadri, A. L. DeStefano, R. Au, J. M. Massaro, A. S. Beiser, M. Kelly-Hayes, et al., Genetic correlates of brain aging on MRI and cognitive test measures: a genome-wide association and linkage analysis in the Framingham study, BMC Med. Genet., 8 (2007). https://doi.org/10.1186/1471-2350-8-S1-S15 |
[35] |
S. A. Krynskiy, I. K. Malashenkova, D. P. Ogurtsov, N. A. Khailov, E. I. Chekulaeva, O. Y. Shipulina, et al., Herpesvirus infections and immunological disturbances in patients with different stages of Alzheimer's disease, Probl. Virol., 66 (2021), 129–139. https://doi.org/10.36233/0507-4088-32 doi: 10.36233/0507-4088-32
![]() |
[36] |
D. L. Ewins, M. N. Rossor, J. Butler, P. K. Roques, M. J. Mullan, A. M. McGregor, Association between autoimmune thyroid disease and familial Alzheimer's disease, Clin. Endocrinol., 35 (1991), 93–96. https://doi.org/10.1111/j.1365-2265.1991.tb03502.x doi: 10.1111/j.1365-2265.1991.tb03502.x
![]() |
[37] | P. Suresh, S. Phasuk, I. Y. Liu, Modulation of microglia activation and Alzheimer's disease: CX3 chemokine ligand 1/CX3CR and P2X7R signaling, Tzu Chi Med. J., 33 (2021), 1–6. |
[38] |
B. Popp, A. B. Ekici, C. T. Thiel, J. Hoyer, A. Wiesener, C. Kraus, et al., Exome Pool-Seq in neurodevelopmental disorders, Eur. J. Hum. Genet., 25 (2017), 1364–1376. https://doi.org/10.1038/s41431-017-0022-1 doi: 10.1038/s41431-017-0022-1
![]() |
[39] |
S. Desai, M. Juncker, C. Kim, Regulation of mitophagy by the ubiquitin pathway in neurodegenerative diseases, Exp. Biol. Med., 243 (2018), 554–562. https://doi.org/10.1177/1535370217752351 doi: 10.1177/1535370217752351
![]() |
[40] | D. A. Salih, S. Bayram, S. Guelfi, R. H. Reynolds, M. Shoai, M. Ryten, et al., Genetic variability in response to amyloid beta deposition influences Alzheimer's disease risk, Brain Commun., 1 (2019). https://doi.org/10.1093/braincomms/fcz022 |
[41] | R. A. Cifuentes, J. Murillo-Rojas, Alzheimer's disease and HLA-A2: linking neurodegenerative to immune processes through an in silico approach, Biomed Res. Int., 2014 (2014). https://doi.org/10.1155/2014/791238 |
[42] | S. da Mesquita, J. Herz, M. Wall, T. Dykstra, K. A. Lima, G. T. Norris, et al., Aging-associated deficit in CCR7 is linked to worsened glymphatic function, cognition, neuroinflammation, and β-amyloid pathology, Sci. Adv., 7 (2021). https://doi.org/10.1126/sciadv.abe4601 |
[43] |
M. G. Kitzbichler, A. R. Aruldass, G. J. Barker, T. C. Wood, N. G. Dowell, S. A. Hurley, et al., Peripheral inflammation is associated with micro-structural and functional connectivity changes in depression-related brain networks, Mol. Psychiatry, 26 (2021), 7346–7354. https://doi.org/10.1038/s41380-021-01272-1 doi: 10.1038/s41380-021-01272-1
![]() |
[44] |
X. Wang, X. Cui, C. Ding, D. Li, C. Cheng, B. Wang, et al., Deficit of cross‐frequency integration in mild cognitive impairment and Alzheimer's disease: A multilayer network approach, J. Magn. Reson. Imaging, 53 (2021), 1387–1398. https://doi.org/10.1002/jmri.27453 doi: 10.1002/jmri.27453
![]() |
[45] | Y. Mao, Z. Liao, X. Liu, T. Li, J. Hu, D. Le, et al., Disrupted balance of long and short-range functional connectivity density in Alzheimer's disease (AD) and mild cognitive impairment (MCI) patients: a resting-state fMRI study, Ann. Transl. Med., 9 (2021). https://doi.org/10.21037/atm-20-7019 |
[46] |
Y. Chang, J. Hsu, S. Huang, S. Hsu, C. Lee, C. Chang, Functional connectome and neuropsychiatric symptom clusters of Alzheimer's disease, J. Affect Disord., 273 (2020), 48–54. https://doi.org/10.1016/j.jad.2020.04.054 doi: 10.1016/j.jad.2020.04.054
![]() |
[47] |
R. J. Vanzo, H. Twede, K. S. Ho, A. Prasad, M. M. Martin, S. T. South, et al., Clinical significance of copy number variants involving KANK1 in patients with neurodevelopmental disorders, Eur. J. Med. Genet., 62 (2019), 15–20. https://doi.org/10.1016/j.ejmg.2018.04.012 doi: 10.1016/j.ejmg.2018.04.012
![]() |
[48] | S. Soleimani, N. Nasim, F. Esfandi, M. Karimipoor, V. K. Oskooei, M. N. Gol, et al., SE translocation gene but not zinc finger or X-linked factor is down-regulated in gastric cancer, Gastroenterol. Hepatol. Bed Bench, 13 (2020), 8–13. |
1. | Mahsa Samareh-Jahani, Farid Saberi-Movahed, Mahdi Eftekhari, Gholamreza Aghamollaei, Prayag Tiwari, Low-Redundant Unsupervised Feature Selection based on Data Structure Learning and Feature Orthogonalization, 2024, 240, 09574174, 122556, 10.1016/j.eswa.2023.122556 | |
2. | Atsushi Kawaguchi, Yuko Ishimaru, Ryosuke Osako, Ensemble clustering multiblock sparse multivariable analysis for multimodal brain imaging, 2025, 2520-8756, 10.1007/s42081-025-00300-6 |
Groups | AD | MCI | HC |
Number | 25 | 248 | 113 |
Gender (M/F) | 10/15 | 133/115 | 58/55 |
Age (mean ± std) | 75.99 ± 10.22 | 71.9 ± 7.34 | 75.06 ± 5.68 |
MMSE (mean) | 20.48 | 27.90 | 29.00 |
Note: HC = healthy control group, MCI = mild cognitive impairment, AD = Alzheimer's disease. |
Module | ROI | SNP | GENE | P-value |
1 | 34 | 88 | 388 | 2.73E-108 |
3 | 22 | 175 | 344 | 0.0021 |
19 | 26 | 313 | 400 | 0.0018 |
25 | 33 | 227 | 497 | 2.30E-42 |
38 | 29 | 365 | 574 | 1.98E-11 |
Left/Right Exterior Cerebellum | Left/Right Superior Temporal Gyrus |
Left/Right Temporal Pole | Left/Right Cerebrum and Motor |
Left/Right Inferior Temporal Gyrus | Left/Right Middle Temporal Gyrus |
Left Calcarine and Cerebrum | Left Gyrus Rectus |
Left Inferior Frontal Angular Gyrus | Left Lingual Gyrus |
Left Medial Frontal Cerebrum | Left Middle Occipital Gyrus |
Left Occipital Pole | Left Parahippocampus Gyrus |
Left Posterior Orbital Gyrus | Left Superior Occipital Gyrus |
Right Angular Gyrus | Right Central Operculum |
Right Middle Cingulate Gyrus | Right Middle Frontal Gyrus |
Right Postcentral Gyrus | Right Precentral Gyrus |
Right Superior Frontal Gyrus | Right Superior Medial Frontal Gyrus |
Right Superior Parietal Lobule | Right Supramarginal Gyrus |
Right Thalamus Proper |
ROI-SNP | P-Value | ROI-GENE | P-Value |
rPCu-rs75277622 | 2.39E-16 | rExtCbe-BCL7A | 3.23E-07 |
lExtCbe-rs75277622 | 2.64E-16 | lExtCbe-BCL7A | 8.91E-07 |
rPoCGy-rs75277622 | 1.31E-14 | lMedFroCbr-BCL7A | 2.73E-06 |
rExtCbe-rs75277622 | 1.01E-12 | lLinGy-PPAT | 2.86E-06 |
lMidOccGy-rs75277622 | 1.07E-12 | lLinGy-KANK1 | 3.05E-06 |
lSupOccGy-rs75277622 | 1.44E-12 | lRecGy-PPAT | 3.32E-06 |
rMidTemGy-rs2868996 | 2.21E-12 | lInfTemGy-HBG1 | 5.20E-06 |
rCenOpe-rs55663874 | 2.03E-11 | lMidTemGy-ZNF285 | 5.79E-06 |
rSupMedFroGy-rs28529220 | 7.65E-11 | lSupOccGy-BCL7A | 6.03E-06 |
lLinGy-rs11160070 | 2.26E-10 | lInfTemGy-ZFX | 7.47E-06 |
Groups | AD | MCI | HC |
Number | 25 | 248 | 113 |
Gender (M/F) | 10/15 | 133/115 | 58/55 |
Age (mean ± std) | 75.99 ± 10.22 | 71.9 ± 7.34 | 75.06 ± 5.68 |
MMSE (mean) | 20.48 | 27.90 | 29.00 |
Note: HC = healthy control group, MCI = mild cognitive impairment, AD = Alzheimer's disease. |
Module | ROI | SNP | GENE | P-value |
1 | 34 | 88 | 388 | 2.73E-108 |
3 | 22 | 175 | 344 | 0.0021 |
19 | 26 | 313 | 400 | 0.0018 |
25 | 33 | 227 | 497 | 2.30E-42 |
38 | 29 | 365 | 574 | 1.98E-11 |
Left/Right Exterior Cerebellum | Left/Right Superior Temporal Gyrus |
Left/Right Temporal Pole | Left/Right Cerebrum and Motor |
Left/Right Inferior Temporal Gyrus | Left/Right Middle Temporal Gyrus |
Left Calcarine and Cerebrum | Left Gyrus Rectus |
Left Inferior Frontal Angular Gyrus | Left Lingual Gyrus |
Left Medial Frontal Cerebrum | Left Middle Occipital Gyrus |
Left Occipital Pole | Left Parahippocampus Gyrus |
Left Posterior Orbital Gyrus | Left Superior Occipital Gyrus |
Right Angular Gyrus | Right Central Operculum |
Right Middle Cingulate Gyrus | Right Middle Frontal Gyrus |
Right Postcentral Gyrus | Right Precentral Gyrus |
Right Superior Frontal Gyrus | Right Superior Medial Frontal Gyrus |
Right Superior Parietal Lobule | Right Supramarginal Gyrus |
Right Thalamus Proper |
ROI-SNP | P-Value | ROI-GENE | P-Value |
rPCu-rs75277622 | 2.39E-16 | rExtCbe-BCL7A | 3.23E-07 |
lExtCbe-rs75277622 | 2.64E-16 | lExtCbe-BCL7A | 8.91E-07 |
rPoCGy-rs75277622 | 1.31E-14 | lMedFroCbr-BCL7A | 2.73E-06 |
rExtCbe-rs75277622 | 1.01E-12 | lLinGy-PPAT | 2.86E-06 |
lMidOccGy-rs75277622 | 1.07E-12 | lLinGy-KANK1 | 3.05E-06 |
lSupOccGy-rs75277622 | 1.44E-12 | lRecGy-PPAT | 3.32E-06 |
rMidTemGy-rs2868996 | 2.21E-12 | lInfTemGy-HBG1 | 5.20E-06 |
rCenOpe-rs55663874 | 2.03E-11 | lMidTemGy-ZNF285 | 5.79E-06 |
rSupMedFroGy-rs28529220 | 7.65E-11 | lSupOccGy-BCL7A | 6.03E-06 |
lLinGy-rs11160070 | 2.26E-10 | lInfTemGy-ZFX | 7.47E-06 |