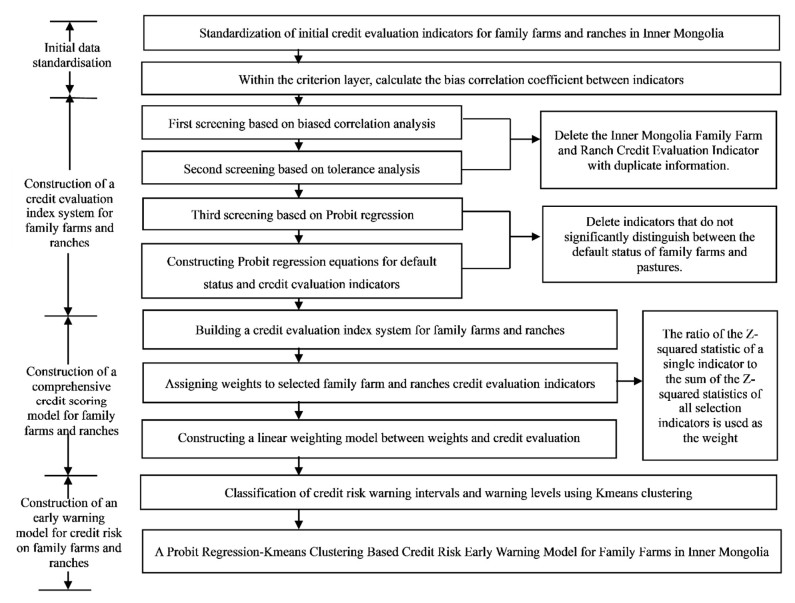
Early warning models credit risk play a crucial role in helping the financial institutions to reasonably predict the credit status of family farms and ranches. An attempt is made in this paper to construct a new credit risk early warning model based on Probit regression and Kmeans clustering algorithm, and testing the model by using data from 246 family farms in 12 leagues and cities in Inner Mongolia. First, the credit risk evaluation indicators of family farms and ranches were screened out through a three-combination model with partial correlation analysis, tolerance analysis and Probit regression. Second, the ratios of the Z-squared statistic of a single indicator to the sum of the Z-squared statistics of all the selected indicators were used to measure the weights of the credit evaluation indicators. Finally, four warning levels containing heavy alert level Ⅰ, medium alert level Ⅱ, light alert level Ⅲ and no alert level Ⅳ were classified by Kmeans clustering with large intra-cluster similarity and small inter-cluster similarity. The empirical evidence shows that the early warning model of credit risk for family farms and ranches is effective.
Citation: Zhanjiang Li, Yixiao Yuan, Tianning Sun, Pengfei Li. Early warning model of credit risk for family farms and ranches in Inner Mongolia based on Probit regression-Kmeans clustering[J]. Mathematical Biosciences and Engineering, 2023, 20(5): 8546-8560. doi: 10.3934/mbe.2023375
[1] | Hui Wang . Model and application of farmers' credit risk early warning system based on T-S fuzzy neural network application. Mathematical Biosciences and Engineering, 2022, 19(8): 7886-7898. doi: 10.3934/mbe.2022368 |
[2] | Qing Zhang, Yixiang Li, Yajun Li, Xiaodong Yang, Qammer Hussain Abbasi . Monitoring of neck activity for early warning of cervical spondylosis. Mathematical Biosciences and Engineering, 2022, 19(1): 792-811. doi: 10.3934/mbe.2022036 |
[3] | Yong Li, Yang Wang . Temporal convolution attention model for sepsis clinical assistant diagnosis prediction. Mathematical Biosciences and Engineering, 2023, 20(7): 13356-13378. doi: 10.3934/mbe.2023595 |
[4] | Yuta Okada, Hiroshi Nishiura . Vaccine-induced reduction of COVID-19 clusters in school settings in Japan during the epidemic wave caused by B.1.1.529 (Omicron) BA.2, 2022. Mathematical Biosciences and Engineering, 2024, 21(9): 7087-7101. doi: 10.3934/mbe.2024312 |
[5] | Qinhua Tang, Xingxing Cen, Changqing Pan . Explainable and efficient deep early warning system for cardiac arrest prediction from electronic health records. Mathematical Biosciences and Engineering, 2022, 19(10): 9825-9841. doi: 10.3934/mbe.2022457 |
[6] | Yi-Wen Chang, Kang-Ping Lu, Shao-Tung Chang . Cluster validity indices for mixture hazards regression models. Mathematical Biosciences and Engineering, 2020, 17(2): 1616-1636. doi: 10.3934/mbe.2020085 |
[7] | Andreas Widder, Christian Kuehn . Heterogeneous population dynamics and scaling laws near epidemic outbreaks. Mathematical Biosciences and Engineering, 2016, 13(5): 1093-1118. doi: 10.3934/mbe.2016032 |
[8] | Chen Zheng, Zhaobang Tan . A novel identified pyroptosis-related prognostic signature of colorectal cancer. Mathematical Biosciences and Engineering, 2021, 18(6): 8783-8796. doi: 10.3934/mbe.2021433 |
[9] | Xu Shen, Xinyu Wang . Prediction of personal default risks based on a sparrow search algorithm with support vector machine model. Mathematical Biosciences and Engineering, 2023, 20(11): 19401-19415. doi: 10.3934/mbe.2023858 |
[10] | Jiyuan Ren, Yunhou Zhang, Zhe Wang, Yang Song . Artificial intelligence-based network traffic analysis and automatic optimization technology. Mathematical Biosciences and Engineering, 2022, 19(2): 1775-1785. doi: 10.3934/mbe.2022083 |
Early warning models credit risk play a crucial role in helping the financial institutions to reasonably predict the credit status of family farms and ranches. An attempt is made in this paper to construct a new credit risk early warning model based on Probit regression and Kmeans clustering algorithm, and testing the model by using data from 246 family farms in 12 leagues and cities in Inner Mongolia. First, the credit risk evaluation indicators of family farms and ranches were screened out through a three-combination model with partial correlation analysis, tolerance analysis and Probit regression. Second, the ratios of the Z-squared statistic of a single indicator to the sum of the Z-squared statistics of all the selected indicators were used to measure the weights of the credit evaluation indicators. Finally, four warning levels containing heavy alert level Ⅰ, medium alert level Ⅱ, light alert level Ⅲ and no alert level Ⅳ were classified by Kmeans clustering with large intra-cluster similarity and small inter-cluster similarity. The empirical evidence shows that the early warning model of credit risk for family farms and ranches is effective.
In the new development era of agriculture, rural areas and farmers, the Ministry of Agriculture and Rural Affairs of China, strategically issued a "Notice on the Implementation of the Action to Enhance the New type of Agricultural Operating Entity" in 2022. The notice proposed to strive to achieve the goal of organically linking the development of small farmers and modern agriculture, mainly family farms and ranches, by the end of the 14th Five-Year Plan [1]. According to the Ministry of Agriculture and Rural Affairs of China, family farm refers to "family members as the main labor force, engaged in large-scale agriculture, intensive, commercial production and operation, and agriculture as the main source of income of new agricultural operating entities". Although, it is distinguished from the big-specialized-households of crop and animal production, the latter's scale is more extensive and has traditional characteristics of agricultural production and management methods, while family farms require, not only moderate scale, but also require specialized and commercialized operations. Since the production and operation of family farms are increasingly standardized, not only for "registered" market business entities, but also for a tightly organized "corporate" organization, from start-up capital, equipment assembly, production and operation to commercial sales, where each link requires financial support, the family farms are in dire need for funds [2]. At present, the financing difficulties are most significant constraints for family farms. According to H. Song et al. [2], especially among the new agricultural operating entities production scale expands and the operational facilities improve, the need for financial support for family farms and ranches becomes increasingly urgent. However, the credit characteristics of family farms and ranches, such as weak foundation, small scale of operation and lack of effective collateral make banks and other financial institutions less willing to lend their funds. Coupled with the imperfect financial system of the family farms and ranches, the existing credit evaluation index system of the enterprises does not apply to them, which limits the financial institutions to assess the risk of lending thus, hindering the financial institutions from lending funds to family farms and ranches. In view of this, the construction of a credit evaluation index system applicable to family farms and ranches is vital to alleviate their financing difficulties.
The perspective of domestic researchers on credit evaluation focused mainly on the enterprises. A relatively complete enterprise credit evaluation research system was evolved right from selecting the credit evaluation indicators to establishing a credit scoring model. Initially, most of the methods used in selecting credit evaluation indexes were questionnaires, descriptive statistical analysis, correlation analysis and expert scoring. Subsequently, B. Shi et al. [3] introduced a Logistic regression model for constructing a bond credit rating index system for the banks and the bond investors, which ensured that the screened indicators could significantly distinguish the default status. Z. Li and L. Guo [4] created a credit index screening model for small enterprises based on a two-stage Bayesian discriminant model; L. Yang et al. [5] used the binary opposite whale optimization algorithm (BOWOA) and the Kolmogorov–Smirnov (KS) statistic to construct a credit index discrimination model for small enterprises; S. Qian [6] used Analytic Hierarchy Processes (AHP) to build an assessment system for enterprise financial credit risk; Y. Sun [7] adopted a correlation analysis, univariate analysis and stepping backward feature selection method to select the indicators. Though, the enterprise credit evaluation index screening methods are becoming more diverse and perfect however, only few scholars have studied the credit evaluation of family farms and ranches. N. Cai and B. Shi [8] used the APRIORI algorithm, term frequency inverse document frequency and sentiment dictionary analysis method to select credit features for farmers. Z. Li and Q. Zhang [9] selected the credit evaluation indexes applicable to family farms based on the depth-weighted Bayesian theory and fuzzy mathematics.
Most of the theoretical studies in the literature stress on the credit evaluation of family farms and ranches. There are few published papers [8,9] on screening credit evaluation indicators for family farms, but they have calculated only credit scores for family farms and ranches, and do not consider the link between the scores and the probability of default in depth. Furthermore, there is no quantitative method to delineate the warning interval. Based on this, we first establish a credit evaluation index system and a scoring model for family farms and ranches. Then, we use the K-means clustering algorithm to classify the early warning intervals. As a result, a complete credit risk early warning model for family farms and ranches in Inner Mongolia (hereinafter referred to as family farms and ranches) is conceptualized to provide theoretical support to the financial institutions for predicting the financial risk of family farms and ranches, so as to develop appropriate financial products.
1) To find a method to construct a credit evaluation index system for family farms and ranches that avoids redundancy of information between the indicators and has high discriminatory power of default.
2) To construct a credit evaluation model that can effectively reflect the actual credit level of family farms and ranches.
3) To method an early warning modelling which can better distinguish the level of the credit risk of family farms and ranches, while ensuring high credit similarity within the same interval and low credit similarity across the gaps.
Approach 1: Partial correlation analysis is used to eliminate the more relevant indicators in the family farms and ranches credit evaluation system for the first time, and tolerance analysis is then used to eliminate the remaining redundant indicators for the second time. Finally, the credit risk evaluation indicators of family farms and ranches that have less influence on whether the operator default is deleted, by constructing a Probit regression model to build a credit risk evaluation index system applicable to Inner Mongolia.
Approach 2: The focus of the credit evaluation model for family farms and ranches is to construct a scientific and reasonable weight matrix. To make the evaluation model discriminate the default status of the operators, the ratio of the Z-squared statistic of a single indicator to the sum of the Z-squared statistic of all the selected indicators is used as the weight. A linear weighting model of the weights and indicators is used as the credit evaluation model to measure the comprehensive credit score of family farms and ranches.
Approach 3: First, the credit evaluation indicators are coalesced into an initial cluster center using the Kmeans cluster analysis. Second, by iterating through the similarities between the indicators and using the final center of mass as the midpoint of the interval and the average of the midpoints of adjacent intervals as the semi-interval length, an early warning model for family farms with small similarity between different intervals and large similarity within the same interval can be obtained. The principle of the early warning model of credit risk for family farms and ranches based on Probit regression-Kmeans clustering is shown in Figure 1.
Three types of indicators namely, positive indicators: "annual profit of family farms", negative indicators: "annual land transfer costs", and interval-type indicators: "operator's age" and "managers' working years", and standardized formulae were used [9]. For the best interval type indicators, the ideal interval of "operator's age" was set to (31, 45), which indicates that operators in this age group have a relatively strong willingness and ability to repay the loan [10]. The ideal range of "managers' working years" was set between (13, 27), which shows that family farmers and ranchers in this range have relatively strong credibility and business ability [10]. For qualitative indicators, such as "manager's marital status" and "manager's physical health", scoring was done by using standards of Y. Cheng [10]. In this way, the inconsistency of units and nature among indicators was eliminated, and the values of indicators were transformed into numbers between (0, 1) to lay the foundation of credit evaluation index screening.
To avoid repetitive indicators, partial correlation analysis was used as the first screening method to eliminate the indicators with overlapping and redundant information. Assume that rkf is the simple correlation coefficient between the kth index and the fth index; xki is the value of the kth index and the ith family farm or ranch; −xk is the average value of the kth credit evaluation index; xfi is the value of the fth index and the ith family farm or ranch; −xf is the average value of the fth credit evaluation index; m is the total number of family farms and ranches; n is the total number of credit evaluation indicators; and R is the correlation coefficient matrix of credit risk indicators, then simple correlation coefficient between the kth index and the fth index is given by Eq (1).
rkf=m∑i=1(xki−ˉxk)(xfi−ˉxf)√m∑i=1(xki−ˉxk)2√m∑i=1(xfi−ˉxf)2 | (1) |
The correlation coefficient matrix R is
R=[r11r12⋯r1nr21r22⋯r2n⋮⋮⋱⋮rn1rn2⋯rnn]. | (2) |
The inverse matrix A of matrix R is represented as
A=R−1=[a11a12⋯a1na21a22⋯a2n⋮⋮⋱⋮an1an2⋯ann]. | (3) |
The partial correlation coefficient between the kth index and the fth index is given by Eq (4):
r′kf=−akf√akkaff. | (4) |
The larger the value of ´rkf, the stronger the correlation between the kth index and the fth index, and vice versa.
To avoid subjectively deleting the effective credit evaluation indicators of family farms and ranches, this paper uses the F-score to screen the indicators that distinguish the weak default ability in the two indicators. Assume that Fk is F-score of the kth credit evaluation index; −x(0)k is the average value of the kth credit evaluation index in the sample of family farms and ranches without a default; −xk is the average value of the kth credit evaluation index; −x(1)k is the average value of the kth credit evaluation index in the defaulting sample; m(0) is the total number of family farms and ranches that have not defaulted; xik is the value of the kth credit evaluation index of the ith family farm or ranch sample; m(1) is the total number of defaulting family farms and ranches and M is the total number of family farms and ranches in Inner Mongolia.
Fk=(ˉx(0)k−ˉxk)2+(ˉx(1)k−ˉxk)21m(0)−1∑yk=0(xik−ˉx(0)k)2+1m(1)−1∑yk=0(xik−ˉx(1)k)2. | (5) |
Equation (5) shows the ability of the kth credit evaluation index to judge the default state of family farms and ranches. The greater the F-score, the stronger is the ability. We eliminated the credit evaluation indicators with small F-scores in both indicators.
After removing the variable by partial correlation analysis, we found that the effect of eliminating variables is insignificant. Considering that multicollinearity brings serious consequences, we used tolerance analysis to distinguish the multicollinearity among the variables, and the tolerance (TOL) is given by:
TOL=1−R2=1−n∑i=1(ˆyi−ˉy)2n∑i=1(yi−ˉy)2=n∑i=1(ˉy−ˆyi)2n∑i=1(ˉy−yi)2, | (6) |
where R2 is the correlation coefficient. When the tolerance is less than the critical value, it indicates a multicollinearity phenomenon between the credit evaluation indicators, affecting the correct estimation of subsequent Probit regression model. Generally, the Variance Inflation Factor (VIF) is considered greater than 5, and then there exists multicollinearity. To ensure the accuracy in this paper, the VIF value is strictly controlled below 1.5. That is, the tolerance is more than 0.7. At this time, there is a weak correlation between the variables hence, the impact on the weight of the indicators can be ignored.
A Probit regression model was used to analyze the significance of the parameter of the evaluation indicators, after which the evaluation indicators having little ability to assess the default status of the operators of family farms and ranches were excluded based on the significance of the regression coefficients. Probit regression model was constructed using the probability of default of family farm operators as the dependent variable, and the remaining credit evaluation indicators after the previous two screening processes as the independent variables. The credit evaluation indicators that significantly impact the default status of family farms were selected by the significance of the variables. The specific steps followed are as follows:
Step 1: Introduce a potential variable. Assume that y∗i is the actual default status of family farms and ranches; yi is the latent variable, when "y∗i≥0", it is considered that "yi=1", then the sample is judged as credit default. Conversely, when "y∗i<0", it is considered that "yi=0", then the sample is not in default. The potential variable "yi" is introduced because the default status is a discrete variable and cannot be measured directly by a linear regression equation. xki is the value of the kth credit evaluation index and the ith Inner Mongolia family farm or ranch (k = 1, 2, 3…n, i = 1, 2, 3…m); β is column vector formed by the regression coefficient of credit evaluation index; Xi is column vector composed of the total indicator's value of the ith family farm; α is the constant term and μi is the stochastic error term and subject to standard normal distribution.
y∗i=α+∑βkxik+μi=α+Xiβ+μi. | (7) |
Step 2: The default probability of family farms and ranches is as follows:
P(yi=1/yi=1XiXi)=P(y∗i>0/y∗i>0XiXi)=ϕ(y∗i), | (8) |
y∗i=α+Xiβ, and Φ(y∗i) represents the standard normal cumulative distribution function.
Step 3: Estimation of parameters.
lnL=m∑i=1[yiln(ϕ(α+Xiβ))+(1−yi)ln(1−ϕ(α+Xiβ))]. | (9) |
Equation (9) is the log-likelihood function of the model, where both yi and Xi are known, and only α and β are unknown.
The n evaluation indexes and default status yi after removing the multicollinearity are substituted into the Probit regression model of family farms and ranches. Then, the estimated values of parameters α,β1, β2, β3,…,βn are obtained after parameter estimation with the maximum likelihood function. Given an initial value for the parameters α, β, and substituting them into Eq (9) to obtain the log-likelihood function lnL. If lnL has the maximum value at this point, then α and β are the desired one. Otherwise, give new values of α and β, and repeat the above process until the likelihood function lnL of Eq (9) is maximum. The above process is carried out by SPSS software.
Step 4: Solution idea. The estimated parameter values were used to construct Z statistics and put forward the original hypothesis H0(βk=0). H0 indicates that the kth indicator is not significant for the breach of contract of the family farms operators and should be removed. Otherwise, it is significant and should be retained. Assume that Zk is the value of Z statistic of the kth credit evaluation indicator; βk is the parameter estimate value for the kth credit evaluation indicator, SEβk is the standard error of βk,then Zk is represented by:
Zk=βkSEβk. | (10) |
Equation (10) is used to test whether βk is significantly equal to 0 under the assumption of H0.
Assume that Wk is the weight of the kth evaluation index in the credit evaluation index system of family farms and ranches; Zk is the Z statistic value of the kth evaluation index in the credit evaluation index system of family farms and ranches. The formula for calculating the weight of the credit evaluation index of Inner Mongolia family farms and ranches is:
Wk=Z2kn∑k=1Z2k. | (11) |
The calculation of Wk is based on the calculation of n credit evaluation indicators retained after a three-combination model of partial correlation analysis, tolerance analysis and Probit regression. The greater the weight of the credit evaluation indicators, the greater is the discriminant power of the indicators on the default status of the family farms and ranches.
Assume that Si is the credit score of the ith family farm or ranch; n is the number of credit evaluation indicators selected after partial correlation, tolerance analysis and Probit regression; xik is the standardised value of the kth credit evaluation index of the ith family farm or ranch. The Inner Mongolia family farms and ranches' credit scoring model is as follows:
Si=n∑k=1Wk×xik. | (12) |
The higher the value of credit score (S) of family farms and ranches, the less likely there is a chance for the operator to default.
The receiver operating characteristic curve test (ROC) is drawn by using two indicators, namely sensitivity and 1-specificity, as the horizontal and vertical axes, which proves that the credit scoring model of Inner Mongolia family farms and ranches is valid. The area under curve (AUC) is the area between the ROC curve and the horizontal coordinate, and a reasonable ROC curve should be above the 45-degree line, i.e., AUC should be greater than 0.5; the greater the vertical distance between the ROC curve and the 45-degree line, the better the predictive power of the corresponding assessment model, expressed by the AUC value. The larger the AUC value, the better the predictive power of the related credit assessment model [11]. Assume m(0) is the total number of non-defaulting samples among family farms and ranches, and m(1) is the total number of defaulting samples. By correctly determining the number of non-default samples (yj=0) as non-default, and recording it as Tm(0); and determining the number of default samples (yj=1) as default and recording it as Tm(1), the sensitivity and 1-specificity are calculated as follows [12]:
sensitivity=Tm(1)m(1) | (13) |
specificity=Tm(0)m(0). | (14) |
Equation (13) correctly adjudicates the rate at which the samples of family farms and ranches with defaulting operators while, Eq (14) correctly adjudicates the rate at which the samples of family farms and ranches with non-defaulting operators.
To reflect the link between credit score and early warning level, this paper uses the Kmeans cluster analysis to construct the early warning interval. Assume that K is the number of clusters, and the cluster center is C={c1,c2,c3,⋯,cj,⋯,ck}. The clustering distance formula for solving the credit risk early warning level given is by Eq (15).
D(si,cj)=√(si−cj)2, | (15) |
where D(si,cj) is the distance of the credit score of the ith family farm or ranch from the jth clustering cluster.
A randomly selected family farming sample was used as the initial cluster center, and its distance from the weighted family farming sample was calculated. The weighted sample data was divided by the sample with the shortest distance from the k clustering centers, and new clustering centers were coalesced. Finally, the clustering centers were iterated to arrive at the final clustering centers for family farms and ranches' credit risk warning intervals. The cluster centers were ranked from small to large by setting the sorted cluster center as qi(I = 1…k), and selecting (qi−qi−1)/2 as the half interval length of qi and qi−1 to determine the warning intervals for different grades of family farms and ranches. A lower combined credit risk grade indicated a higher risk of default.
To illustrate the overall credit status for family farms and ranches in Inner Mongolia, the team visited and distributed questionnaires in 12 leagues and cities in the Autonomous Region starting in October 2021. Two hundred forty-six valid questionnaires on family farms and ranches' credit information in Inner Mongolia were returned by April 2022. The distribution of the sample is shown in Table 1.
No. | Region | No. of farms & ranches | No. | Region | No. of farms & ranches |
1 | Hulun Buir | 25 | 7 | Baotou | 23 |
2 | Hinggan League | 20 | 8 | Hohhot | 15 |
3 | Tongliao | 22 | 9 | Bayan Nur | 25 |
4 | Chifeng | 22 | 10 | Ordos | 26 |
5 | Xilingol League | 19 | 11 | Alxa League | 15 |
6 | Ulanqab | 15 | — | — | — |
Four elements viz. basic information, ability to repay, past credibility and environmental conditions were used to construct criterion layers to reflect the quality of the operator, repayment ability, financial situation, guarantee situation and the support and development environment of the family farms and ranches operators, based on the 5C credit evaluation theory. Based on the sample of family farm and ranch loans provided by the Inner Mongolia Agricultural and Commercial Bank and high-frequency credit evaluation indicators from relevant literature studies, a set of credit evaluation indicators for Inner Mongolia family farms and ranches containing 54 indicators was initially formed, as shown in Table 2.
No. | Criterion layer | Indicator name | Screening results |
1 | Basic Information | Birth date of the operator | Probit delete |
… | … | … | |
13 | Labor force population / total household size | TOL delete | |
14 | Ability to repay | Registered capital or initial invested capital | Probit delete |
… | … | … | |
48 | Insurance coverage ratio | Probit delete | |
49 | Past Credibility | Does the operator receive frequent reminders | TOL delete |
50 | Whether payments are made on time | TOL delete | |
51 | Environment Conditions | Whether to drive neighboring farmers and herdsmen/poor households | Probit delete |
… | … | … | |
54 | Possible natural disasters | Reserved |
Out of the selected loans sample of 246 family farms and ranches, 221 were non-default samples, and 25 were default samples. The credit evaluation indicators were standardized according to the method elaborated in Section 3.1.
By substituting the data into Eqs (1)–(4), the partial correlation coefficients among the credit evaluation indicators of family farms and ranches were calculated, and the results are shown in Table 3. The critical value of the partial correlation coefficient was set at 0.8 to represent high partial correlation. Two indicators with partial correlation coefficients greater than 0.8 were selected, namely "the number of cooperatives joined" and "form of production and management decision" from Table 3. The F-scores of these two indicators were calculated by substituting them into Eq (5), where the F-score of "the number of cooperatives joined" was 0.047 and the F-score of "form of production and management decision" was 0.033. The "form of production and management decision" was removed in this paper.
No. | Indicator name | Indicator name | ||||||
(1) Birth year of the operator | (35) The number of cooperatives that joined | (44) Form of production and management decision | (54) Possible natural disasters | |||||
1 | Year of birth of the operator | 1.000 | … | -0.007 | … | -0.003 | … | -0.120 |
… | … | … | … | … | … | … | ||
35 | The number of cooperatives that joined | -0.007 | … | 1.000 | … | 0.874* | … | 0.005 |
… | … | … | … | … | … | … | ||
54 | Possible natural disasters | -0.120 | … | 0.005 | … | 0.005 | … | 1.000 |
By substituting the remaining variables into Eq (6), the tolerance of the corresponding indexes was calculated with the help of SPSS. In the first round of results, the tolerance of purchasing insurance was 0.456, less than 0.7, and hence eliminated as shown in Table 4. A total of 11 credit evaluation indicators, such as "Labor force population" and "purchase of insurance" were eliminated, and the deleted indicators are marked in column 4 of Table 2. The results of the first tolerance calculation are shown in Table 4.
NO. | Indicator name | TOL | VIF |
1 | Birth year of the operator | 0.802 | 1.247 |
… | … | … | … |
46 | Purchasing insurance | 0.456 | 2.191 |
… | … | … | … |
53 | Possible natural disasters | 0.777 | 1.287 |
The Probit regression model was constructed using Eqs (7) and (8). The Z statistic was built according to Eq (10), which followed normal distribution. Since the sample size of this paper was small, the confidence level α was set to 0.1. Through SPSS 25.0, stepwise regression was performed on the remaining indicators screened in the first two steps, in which the significance values of 33 indicators, such as the year of birth of the operator, the insurance coverage ratio, and whether to drive neighboring farmers and poor households, were 0.898, 0.809, 0.959, etc., which were greater than 0.1, and, hence, deleted and the results are displayed in the fourth column of Table 2. The first Probit regression is shown in Table 5. After the three-combination model with partial correlation analysis, tolerance analysis and Probit regression for the credit risk evaluation indicators, the final credit evaluation index system for Inner Mongolia family farms and ranches with nine indicators is shown in Table 6.
No. | Criterion layer | Indicator name | Coefficient | Z | Sig |
1 | Basic information | Gender of the operator | 0.090 | 0.129 | 0.898 |
… | … | … | … | … | |
11 | Number of students enrolled in the operator's household | 0.279 | 0.615 | 0.539 | |
12 | Ability to repay | Area of production operations | 0.163 | 0.363 | 0.716 |
… | … | … | … | … | |
39 | Insurance coverage ratio | -0.129 | -0.241 | 0.809 | |
40 | Environment Factors | Types of concessions enjoyed | -0.415 | -0.779 | 0.436 |
… | … | … | … | … | |
43 | Whether to drive surrounding farming and poor households | 0.014 | 0.051 | 0.959 |
No. | Criterion layer | Indicator name | Index weight |
1 | Basic Information | Whether the children of the person in charge have any intention to engage in farming and animal husbandry | 0.122 |
2 | Ability to repay | Asset value | 0.110 |
3 | Number of years in circulation | 0.222 | |
4 | Distribution channels for agricultural and livestock products | 0.118 | |
5 | Annual profit | 0.050 | |
6 | Business license registration | 0.117 | |
7 | Whether the discharge of pollutants meets environmental requirements | 0.077 | |
8 | Availability of professional financial management staff | 0.096 | |
9 | Environmental conditions | Possible natural disasters | 0.090 |
In this paper, we used the value of Z statistic Zk, calculated by Eq (10), to assign weights to the nine evaluation indicators in the credit evaluation system of Inner Mongolia family farms and ranches, by bringing the corresponding Z statistic values of the indicators into Eq (11). The detailed results are shown in column 4 of Table 6.
By substituting the calculated credit evaluation index weights into Eq (12), the credit evaluation model of Inner Mongolia family farms and ranches can be obtained as follows:
Si=0.122x1+0.110x2+0.222x3+0.118x4+0.050x5+0.117x6+0.077x7+0.096x8+0.090x9. |
The credit score of each family farm or ranch was then obtained by substituting the standardized sample data into the equation above.
The accuracy of the credit risk early warning model for Inner Mongolia family farms and ranches is tested using the ROC curve. Table 7 shows the classification results at a critical value of 0.5. We substitute data in the first row of Table 7 into Eq (13), and substitute data in the second row of Table 7 into Eq (14), resulting in the first point of the ROC curve (0, 0.936). Multiple sets of sensitivity and 1-specificity were calculated using different critical values, leading to multiple ROC curve points. The ROC curve is shown in Figure 2. The results of the ROC curve test showed that the curve of the credit risk early warning model for Inner Mongolia family farms was above the diagonal. The AUC value corresponding to the ROC curve was 0.646 > 0.6, which indicated that the credit risk early warning model for Inner Mongolia family farms showed better classification of default status.
Substantial breach | Anticipatory breach | ||
Defaulting | Non-defaulting | Summation | |
Defaulting | 0 | 25 | 25 |
Non-defaulting | 14 | 207 | 221 |
Summation | 14 | 232 | 246 |
The clustering distance was calculated from Eq (15) to find the minimum distance between the family farms and ranches sample and the clustering center. The final clustering center of the credit risk warning interval of family farms and ranches can be derived after iteration, and (qi−qi−1)/2 was used as the half-interval length of qi and qi−1 to construct the early warning score interval of credit risk for family farms and ranches to classify the risk level. The results of credit risk classification for Inner Mongolia family farms and ranches are shown in Table 8.
Clustering group | Clustering Centers | Rating range | Risk level |
3 | 0.185 | [0.000, 0.245) | Level Ⅰ (Severe warning) |
4 | 0.305 | [0.245, 0.356) | Level Ⅱ (Moderate warning) |
1 | 0.407 | [0.356, 0.464) | Level Ⅲ (Mild warning) |
2 | 0.521 | [0.464, 1.000] | Level Ⅳ (No warning) |
This paper selected a new evaluation index system for family farms and ranches in Inner Mongolia through a three-combination model with partial correlation analysis, tolerance analysis and Probit regression. This has led to the construction of an evaluation system to study the credit risk of family farms in Inner Mongolia, based on nine indicators, including asset value and the length of time in circulation, which have high default discriminatory power and avoid redundancy of information between indicators. A credit evaluation model for family farms in Inner Mongolia was constructed from the ratio of the Z-squared statistic of a single indicator to the sum of the Z-squared statistics of all selected indicators as weights. The AUC obtained from the ROC curve test was 0.646, which indicated that the credit evaluation model constructed by this method truly and effectively reflects the credit level of family farms and ranches operators. The four warning levels of credit risk for family farms and ranches, and the corresponding early warning intervals were classified by Kmeans clustering with large intra-cluster similarity and slight inter-cluster similarity were level Ⅰ with heavy warning [0.000, 0.245), level Ⅱ with medium warning [0.245, 0.356), level Ⅲ with mild warning [0.356, 0.464) and level Ⅳ with no warning [0.464, 1.000]. Thus, the study of credit risk assessment of family farms and ranches in Inner Mongolia is not only cutting-edge, but also crucial in enhancing the development of family farms and ranches, and modernizing the farming industry in Inner Mongolia as a new research perspective.
Due to the limitation of research capacity, this paper still has shortcomings. The unbalanced samples can be equalized in the future to improve the early warning model fit and make the results more accurate.
The research was supported by the National Natural Science Foundation of China (72161033) and Inner Mongolia Institute for Rural Development of China.
We declare that there are no conflicts of interest.
[1] | Ministry of Agriculture and Rural Affairs of the People's Republic of China, Circular of the Ministry of Agriculture and Rural Affairs on the implementation of the action of upgrading new type of agricultural operating entities, 2022. Available from: http://www.moa.gov.cn/nybgb/2022/202204/202206/t20220607_6401742.htm. |
[2] | H. Song, B. Shi, B. Wu, The new agricultural business entities: basic characteristics, financing needs and policy implication, Rural Econ., 10 (2020), 73–80. Available from: https://www.cnki.com.cn/Article/CJFDTOTAL-NCJJ202010010.htm. |
[3] |
B. Shi, J. Wang, J. Qi, Y. Cheng, A novel imbalanced data classification approach based on Logistic regression and Fisher discriminant, Math. Probl. Eng., 2015 (2015), 945359. https://doi.org/10.1155/2015/945359 doi: 10.1155/2015/945359
![]() |
[4] |
Z. Li, L. Guo, Construction of credit evaluation index system for two-stage Bayesian discrimination: an empirical analysis of small Chinese enterprises, Math. Probl. Eng., 2021 (2021), 8837419. https://doi.org/10.1155/2021/8837419 doi: 10.1155/2021/8837419
![]() |
[5] |
Y. Lu, L. Yang, B. Shi, J. Li, M. Z. Abedin, A novel framework of credit risk feature selection for SMEs in Industry 4.0, Ann. Oper. Res., 2022 (2022), 1–28. https://doi.org/10.1007/s10479-022-04849-3 doi: 10.1007/s10479-022-04849-3
![]() |
[6] | S. Qian, Construction of financial credit risk evaluation system model based on analytic hierarchy process, in CSIA 2022: Cyber Security Intelligence and Analytics, (2022), 488–496. https://doi.org/10.1007/978-3-030-96908-0_61 |
[7] |
Y. Sun, N. Chai, Y. Dong, B. Shi, Assessing and predicting small industrial enterprises' credit ratings: a fuzzy decision-making approach, Int. J. Forecasting, 38 (2022), 1158–1172. https://doi.org/10.1016/j.ijforecast.2022.01.006 doi: 10.1016/j.ijforecast.2022.01.006
![]() |
[8] |
N. Cai, B. Shi, Evaluating farmers' credit risk: a decision combination approach based on credit feature, Int. J. Financ. Eng., 9 (2022), 2250015. https://doi.org/10.1142/S2424786322500153 doi: 10.1142/S2424786322500153
![]() |
[9] |
Z. Li, Q. Zhang, Credit index screening model of family farms and family ranches based on fuzzy Bayesian theory of depth weighting, Complexity, 2022 (2022), 5381208. https://doi.org/10.1155/2022/5381208 doi: 10.1155/2022/5381208
![]() |
[10] | Y. Q. Cheng, Research on Evaluation and Decision of Small Amount Loans for Farmers Based on Support Vector Machines, MD. thesis, Dalian University of Technology, 2011. |
[11] |
J. Cheng, X. Zhu, Research on performance validation of credit risk models, J. Shanxi Finance Econ. Univ., (2007), 86–92. https://doi.org/10.3969/j.issn.1007-9556.2007.02.016 doi: 10.3969/j.issn.1007-9556.2007.02.016
![]() |
[12] | D. Liu, Z. Li, X. Zheng, Selection model of credit index combination based on WOE-Probit stepwise regression and its application, Math. Pract. Theory, 48 (2018), 76–87. |
1. | Lanlan Wan, 2023, Financial Risk Early Warning Based on Big Data Analysis, 979-8-3503-1646-9, 1, 10.1109/AIKIIE60097.2023.10390274 | |
2. | Jaap Beltman, Marcos R. Machado, Joerg R. Osterrieder, Predicting retail customers' distress in the finance industry: An early warning system approach, 2025, 82, 09696989, 104101, 10.1016/j.jretconser.2024.104101 |
No. | Region | No. of farms & ranches | No. | Region | No. of farms & ranches |
1 | Hulun Buir | 25 | 7 | Baotou | 23 |
2 | Hinggan League | 20 | 8 | Hohhot | 15 |
3 | Tongliao | 22 | 9 | Bayan Nur | 25 |
4 | Chifeng | 22 | 10 | Ordos | 26 |
5 | Xilingol League | 19 | 11 | Alxa League | 15 |
6 | Ulanqab | 15 | — | — | — |
No. | Criterion layer | Indicator name | Screening results |
1 | Basic Information | Birth date of the operator | Probit delete |
… | … | … | |
13 | Labor force population / total household size | TOL delete | |
14 | Ability to repay | Registered capital or initial invested capital | Probit delete |
… | … | … | |
48 | Insurance coverage ratio | Probit delete | |
49 | Past Credibility | Does the operator receive frequent reminders | TOL delete |
50 | Whether payments are made on time | TOL delete | |
51 | Environment Conditions | Whether to drive neighboring farmers and herdsmen/poor households | Probit delete |
… | … | … | |
54 | Possible natural disasters | Reserved |
No. | Indicator name | Indicator name | ||||||
(1) Birth year of the operator | (35) The number of cooperatives that joined | (44) Form of production and management decision | (54) Possible natural disasters | |||||
1 | Year of birth of the operator | 1.000 | … | -0.007 | … | -0.003 | … | -0.120 |
… | … | … | … | … | … | … | ||
35 | The number of cooperatives that joined | -0.007 | … | 1.000 | … | 0.874* | … | 0.005 |
… | … | … | … | … | … | … | ||
54 | Possible natural disasters | -0.120 | … | 0.005 | … | 0.005 | … | 1.000 |
NO. | Indicator name | TOL | VIF |
1 | Birth year of the operator | 0.802 | 1.247 |
… | … | … | … |
46 | Purchasing insurance | 0.456 | 2.191 |
… | … | … | … |
53 | Possible natural disasters | 0.777 | 1.287 |
No. | Criterion layer | Indicator name | Coefficient | Z | Sig |
1 | Basic information | Gender of the operator | 0.090 | 0.129 | 0.898 |
… | … | … | … | … | |
11 | Number of students enrolled in the operator's household | 0.279 | 0.615 | 0.539 | |
12 | Ability to repay | Area of production operations | 0.163 | 0.363 | 0.716 |
… | … | … | … | … | |
39 | Insurance coverage ratio | -0.129 | -0.241 | 0.809 | |
40 | Environment Factors | Types of concessions enjoyed | -0.415 | -0.779 | 0.436 |
… | … | … | … | … | |
43 | Whether to drive surrounding farming and poor households | 0.014 | 0.051 | 0.959 |
No. | Criterion layer | Indicator name | Index weight |
1 | Basic Information | Whether the children of the person in charge have any intention to engage in farming and animal husbandry | 0.122 |
2 | Ability to repay | Asset value | 0.110 |
3 | Number of years in circulation | 0.222 | |
4 | Distribution channels for agricultural and livestock products | 0.118 | |
5 | Annual profit | 0.050 | |
6 | Business license registration | 0.117 | |
7 | Whether the discharge of pollutants meets environmental requirements | 0.077 | |
8 | Availability of professional financial management staff | 0.096 | |
9 | Environmental conditions | Possible natural disasters | 0.090 |
Substantial breach | Anticipatory breach | ||
Defaulting | Non-defaulting | Summation | |
Defaulting | 0 | 25 | 25 |
Non-defaulting | 14 | 207 | 221 |
Summation | 14 | 232 | 246 |
Clustering group | Clustering Centers | Rating range | Risk level |
3 | 0.185 | [0.000, 0.245) | Level Ⅰ (Severe warning) |
4 | 0.305 | [0.245, 0.356) | Level Ⅱ (Moderate warning) |
1 | 0.407 | [0.356, 0.464) | Level Ⅲ (Mild warning) |
2 | 0.521 | [0.464, 1.000] | Level Ⅳ (No warning) |
No. | Region | No. of farms & ranches | No. | Region | No. of farms & ranches |
1 | Hulun Buir | 25 | 7 | Baotou | 23 |
2 | Hinggan League | 20 | 8 | Hohhot | 15 |
3 | Tongliao | 22 | 9 | Bayan Nur | 25 |
4 | Chifeng | 22 | 10 | Ordos | 26 |
5 | Xilingol League | 19 | 11 | Alxa League | 15 |
6 | Ulanqab | 15 | — | — | — |
No. | Criterion layer | Indicator name | Screening results |
1 | Basic Information | Birth date of the operator | Probit delete |
… | … | … | |
13 | Labor force population / total household size | TOL delete | |
14 | Ability to repay | Registered capital or initial invested capital | Probit delete |
… | … | … | |
48 | Insurance coverage ratio | Probit delete | |
49 | Past Credibility | Does the operator receive frequent reminders | TOL delete |
50 | Whether payments are made on time | TOL delete | |
51 | Environment Conditions | Whether to drive neighboring farmers and herdsmen/poor households | Probit delete |
… | … | … | |
54 | Possible natural disasters | Reserved |
No. | Indicator name | Indicator name | ||||||
(1) Birth year of the operator | (35) The number of cooperatives that joined | (44) Form of production and management decision | (54) Possible natural disasters | |||||
1 | Year of birth of the operator | 1.000 | … | -0.007 | … | -0.003 | … | -0.120 |
… | … | … | … | … | … | … | ||
35 | The number of cooperatives that joined | -0.007 | … | 1.000 | … | 0.874* | … | 0.005 |
… | … | … | … | … | … | … | ||
54 | Possible natural disasters | -0.120 | … | 0.005 | … | 0.005 | … | 1.000 |
NO. | Indicator name | TOL | VIF |
1 | Birth year of the operator | 0.802 | 1.247 |
… | … | … | … |
46 | Purchasing insurance | 0.456 | 2.191 |
… | … | … | … |
53 | Possible natural disasters | 0.777 | 1.287 |
No. | Criterion layer | Indicator name | Coefficient | Z | Sig |
1 | Basic information | Gender of the operator | 0.090 | 0.129 | 0.898 |
… | … | … | … | … | |
11 | Number of students enrolled in the operator's household | 0.279 | 0.615 | 0.539 | |
12 | Ability to repay | Area of production operations | 0.163 | 0.363 | 0.716 |
… | … | … | … | … | |
39 | Insurance coverage ratio | -0.129 | -0.241 | 0.809 | |
40 | Environment Factors | Types of concessions enjoyed | -0.415 | -0.779 | 0.436 |
… | … | … | … | … | |
43 | Whether to drive surrounding farming and poor households | 0.014 | 0.051 | 0.959 |
No. | Criterion layer | Indicator name | Index weight |
1 | Basic Information | Whether the children of the person in charge have any intention to engage in farming and animal husbandry | 0.122 |
2 | Ability to repay | Asset value | 0.110 |
3 | Number of years in circulation | 0.222 | |
4 | Distribution channels for agricultural and livestock products | 0.118 | |
5 | Annual profit | 0.050 | |
6 | Business license registration | 0.117 | |
7 | Whether the discharge of pollutants meets environmental requirements | 0.077 | |
8 | Availability of professional financial management staff | 0.096 | |
9 | Environmental conditions | Possible natural disasters | 0.090 |
Substantial breach | Anticipatory breach | ||
Defaulting | Non-defaulting | Summation | |
Defaulting | 0 | 25 | 25 |
Non-defaulting | 14 | 207 | 221 |
Summation | 14 | 232 | 246 |
Clustering group | Clustering Centers | Rating range | Risk level |
3 | 0.185 | [0.000, 0.245) | Level Ⅰ (Severe warning) |
4 | 0.305 | [0.245, 0.356) | Level Ⅱ (Moderate warning) |
1 | 0.407 | [0.356, 0.464) | Level Ⅲ (Mild warning) |
2 | 0.521 | [0.464, 1.000] | Level Ⅳ (No warning) |