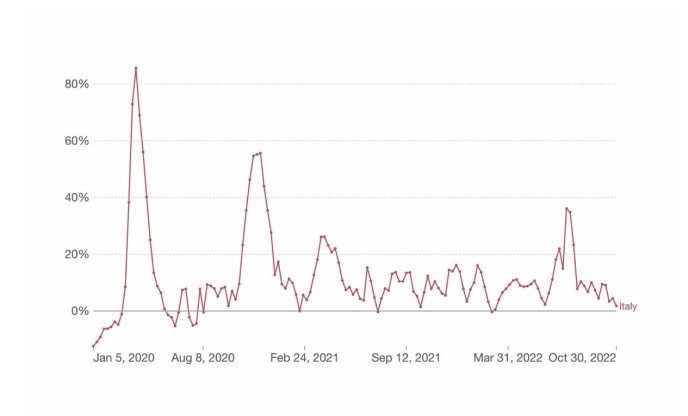
During a sanitary crisis, excess mortality measures the number of all-cause deaths, beyond what we would have expected if that crisis had not occurred. The high number of COVID-19 deaths started a debate in Italy with two opposite positions: those convinced that COVID-19 deaths were not by default excess deaths, because many COVID-19 deaths were not correctly registered, with most being attributable to other causes and to the overall crisis conditions; and those who presented the opposite hypothesis. We analyzed the curve of the all-cause excess mortality, during the period of January 5, 2020–October 31, 2022, compared to the curve of the daily confirmed COVID-19 deaths, investigating the association between excess mortality and the recurrence of COVID-19 waves in Italy. We compared the two curves looking for the corresponding highest peaks, and we found that 5 out of the 6 highest peaks (83.3%) of the excess mortality curve have occurred, on average, just a week before the concomitant COVID-19 waves hit their highest peaks of daily deaths (Mean 6.4 days; SD 2.4 days). This temporal correspondence between the moments when the excess mortality peaked and the highest peaks of the COVID-19 deaths, provides further evidence in favor of a positive correlation between COVID-19 deaths and all-cause excess mortality.
Citation: Marco Roccetti. Excess mortality and COVID-19 deaths in Italy: A peak comparison study[J]. Mathematical Biosciences and Engineering, 2023, 20(4): 7042-7055. doi: 10.3934/mbe.2023304
[1] | Vitaliy Yakovyna, Natalya Shakhovska . Modelling and predicting the spread of COVID-19 cases depending on restriction policy based on mined recommendation rules. Mathematical Biosciences and Engineering, 2021, 18(3): 2789-2812. doi: 10.3934/mbe.2021142 |
[2] | Weike Zhou, Aili Wang, Fan Xia, Yanni Xiao, Sanyi Tang . Effects of media reporting on mitigating spread of COVID-19 in the early phase of the outbreak. Mathematical Biosciences and Engineering, 2020, 17(3): 2693-2707. doi: 10.3934/mbe.2020147 |
[3] | Marco Roccetti . Drawing a parallel between the trend of confirmed COVID-19 deaths in the winters of 2022/2023 and 2023/2024 in Italy, with a prediction. Mathematical Biosciences and Engineering, 2024, 21(3): 3742-3754. doi: 10.3934/mbe.2024165 |
[4] | Youtian Hao, Guohua Yan, Renjun Ma, M. Tariqul Hasan . Linking dynamic patterns of COVID-19 spreads in Italy with regional characteristics: a two level longitudinal modelling approach. Mathematical Biosciences and Engineering, 2021, 18(3): 2579-2598. doi: 10.3934/mbe.2021131 |
[5] | Yuto Omae, Yohei Kakimoto, Makoto Sasaki, Jun Toyotani, Kazuyuki Hara, Yasuhiro Gon, Hirotaka Takahashi . SIRVVD model-based verification of the effect of first and second doses of COVID-19/SARS-CoV-2 vaccination in Japan. Mathematical Biosciences and Engineering, 2022, 19(1): 1026-1040. doi: 10.3934/mbe.2022047 |
[6] | Rafiqul Chowdhury, Gary Sneddon, M. Tariqul Hasan . Analyzing the effect of duration on the daily new cases of COVID-19 infections and deaths using bivariate Poisson regression: a marginal conditional approach. Mathematical Biosciences and Engineering, 2020, 17(5): 6085-6097. doi: 10.3934/mbe.2020323 |
[7] | Salman Safdar, Calistus N. Ngonghala, Abba B. Gumel . Mathematical assessment of the role of waning and boosting immunity against the BA.1 Omicron variant in the United States. Mathematical Biosciences and Engineering, 2023, 20(1): 179-212. doi: 10.3934/mbe.2023009 |
[8] | Ray-Ming Chen . Extracted features of national and continental daily biweekly growth rates of confirmed COVID-19 cases and deaths via Fourier analysis. Mathematical Biosciences and Engineering, 2021, 18(5): 6216-6238. doi: 10.3934/mbe.2021311 |
[9] | Hyeonjeong Ahn, Hyojung Lee . Predicting the transmission trends of COVID-19: an interpretable machine learning approach based on daily, death, and imported cases. Mathematical Biosciences and Engineering, 2024, 21(5): 6150-6166. doi: 10.3934/mbe.2024270 |
[10] | Qian Shen . Research of mortality risk prediction based on hospital admission data for COVID-19 patients. Mathematical Biosciences and Engineering, 2023, 20(3): 5333-5351. doi: 10.3934/mbe.2023247 |
During a sanitary crisis, excess mortality measures the number of all-cause deaths, beyond what we would have expected if that crisis had not occurred. The high number of COVID-19 deaths started a debate in Italy with two opposite positions: those convinced that COVID-19 deaths were not by default excess deaths, because many COVID-19 deaths were not correctly registered, with most being attributable to other causes and to the overall crisis conditions; and those who presented the opposite hypothesis. We analyzed the curve of the all-cause excess mortality, during the period of January 5, 2020–October 31, 2022, compared to the curve of the daily confirmed COVID-19 deaths, investigating the association between excess mortality and the recurrence of COVID-19 waves in Italy. We compared the two curves looking for the corresponding highest peaks, and we found that 5 out of the 6 highest peaks (83.3%) of the excess mortality curve have occurred, on average, just a week before the concomitant COVID-19 waves hit their highest peaks of daily deaths (Mean 6.4 days; SD 2.4 days). This temporal correspondence between the moments when the excess mortality peaked and the highest peaks of the COVID-19 deaths, provides further evidence in favor of a positive correlation between COVID-19 deaths and all-cause excess mortality.
After 3 years from the beginning of the COVID-19 pandemic, the scientific community is still discussing many of the characteristics of this disease, including its most serious implications, like death. People, in fact, are still dying from COVID-19 all around the world, with an estimated cumulative number of confirmed deaths that officially hit more than 6.8 million, as of the end of January 2023 [1]. The World Health Organization (WHO) has proposed the following definition for deaths due to COVID-19 [2]: "A death due to COVID-19 is defined as a death resulting from a clinically compatible illness, in a probable or confirmed COVID-19 case, unless there is a clear alternative cause of death that cannot be related to COVID disease. There should be no period of complete recovery from COVID-19 between illness and death" and "A death due to COVID-19 may not be attributed to another disease (e.g., cancer) and should be counted independently of preexisting conditions that are suspected of triggering a severe course of COVID-19".
Unfortunately, a dispute has ignited over what a COVID-19 death is with two opposite positions emerging quite clearly: on one side, those who believe that the high number of COVID-19 deaths was an overestimate of the actual mortality from the disease, essentially because those recorded deaths had erroneously COVID-19 as the main underlying cause of death rather than a contributing cause [3,4,5,6]. Others, instead, think that the world has paid, to COVID-19, a death toll which is even larger than that the official figures show, particularly in the early months of the pandemic, and in low- and middle- income countries, claiming that many deaths went unrecorded or not correctly attributed to COVID-19 [7,8].
In epidemiology and public health, the term "all-cause excess mortality" indicates that one compares the all-cause mortality figure of a given period with a value for a similar period, averaged over several previous years. Hence, science is trying to provide a solution to the problem of an accurate estimate of COVID-19 deaths by looking at the all-cause excess deaths during the pandemic, and comparing this number to how many people had COVID-19 on their death records. The intent is to verify whether these excess deaths amount to a quantity that corresponds to the number of those who died of coronavirus or not. Unfortunately, this method has problems counting excess deaths, as it does not take into account changes in the age of populations. With a population that is getting older, counting excess deaths might give a biased perspective, as some of those deaths would have occurred independently of the pandemic, because of the age of some portions of that population. Moreover, during a pandemic, deaths from some causes could have decreased, like those from other infectious diseases due to the positive effect of lockdowns, while others could have increased because of the overall crisis conditions, thus affecting this excess death figure.
A vast amount of recent research has investigated this excess mortality issue often converging towards the hypothesis of a positive correlation between the number of actual COVID-19 deaths and the all-cause excess mortality rate. For example, in [9], Vestergaard et al. studied European-wide weekly mortality estimates, from several European countries in the period from the beginning of 2020 until May 2020, using an age-stratified method. Comparing the excess mortality of this short period with the cumulative excess all-cause mortality for the same period of the previous 4 years (2016–2019), they found that the estimated excess mortality could primarily be attributed to COVID-19 and its implications. In [10], Dorucci et al. compared all-cause excess mortality between the two waves that occurred during the year 2020 in Italy using nationwide data and concluding that males and those aged 80 or over were the most hit groups, with an increase in both during the second wave of the pandemic. Finally, in [11], Wang et al. developed a more complete study, with excess mortality rates from 74 countries and an ensemble of various statistical models to conclude that, although reported COVID-19 deaths between January 1, 2020 and December 31, 2021 totaled 5.94 million worldwide, their estimate amounted to 18, 2 million people died because of the COVID-19 pandemic over that period. This study emphasizes the fact that, although the excess mortality rate is a good predictor for the COVID-19 death rate, it is likely it also includes people died due to other causes due to this planetary crisis.
If we focus on Italy, the official data (i.e., WHO) says that this country has paid a death toll of more than 186.000 people, as of January 31, 2023 [12]. Moreover, the Italian Institute of Statistics (ISTAT) has recorded a total number of deaths for all causes in Italy in 2020 equal to 746.146, with an all-cause excess mortality as large as 100.526, which is estimated based on a comparison with the average from the years from 2015 to 2019. This estimate leads to a percentage difference between the reported number of deaths in 2020 and the projected number of deaths for a similar period from previous years equal to 15.6% [13]. Analogous estimates were conducted by ISTAT also for year 2021. The relative figures say that the deaths for all causes were as many as 709.035, and that the all-cause excess mortality was estimated as large as 63.000 deaths, with respect to the average period of reference (2015–2019), thus yielding a percentage of excess deaths in 2021 of 9.8%. As to year 2022, the counting process is still on course, and no announcement on the final estimates on a yearly basis has been given yet. Here, we can only count on partial results extending till the limit of October 31, 2022.
The situation of the public debate in Italy on the issue of the under/over-estimate of the actual number of COVID-19 deaths is similar to that we have already described before, with the two sides bringing mostly the same arguments discussed above, with occasional country-specific remarks.
Following this scientific debate, we decided to choose another perspective in order to investigate the hypothesis of a possible direct association between the actual number of COVID-19 deaths and the all-cause excess mortality rates in Italy (2020–2022), based on the use of techniques from signal processing [14,15]. In particular, we carried out a peak detection analysis of two following curves: i) the percentage difference between the number of all-cause weekly deaths and the projected number of deaths for the same period based on previous years (2015–2019), and ii) the daily confirmed COVID-19 deaths (7-day rolling average). We found that almost all the highest peaks of all the causes of excess mortality curves in Italy occurred, on average, just a week before the concomitant COVID-19 waves hit their highest peaks of daily deaths. In essence, we found that the all-cause excess death curve has always reached its maximum, almost in coincidence, with the peak of the concomitant wave of the COVID-19 deaths, thus providing further evidence, at least in Italy, in favor of a positive correlation between COVID-19 deaths and all-cause excess mortality.
We decided to work with the Italian population-wide data relative to deaths, focusing only on the two following temporal data series: i) the excess mortality for all causes, given by the percentage difference between the number of weekly deaths, in the period January 5, 2020–October 31, 2022 and the estimated number of deaths for the same period based on previous years (2015–2019), and ii) the daily, new confirmed COVID-19 deaths (7-day rolling average) in the period February 26, 2020–October 31, 2022. From this point of view, we have conducted a pure observational study that examines a natural phenomenon from a mere statistical perspective.
The public repository with all data on excess mortality is available at: https://github.com/owid/covid-19-data/tree/master/public/data/excess_mortality.
The excess mortality percentages reported in Figure 1 below are provided by the OurWorldinData.org project, using the following well-known formula:
(1) |
In Eq (1), the reported deaths are sourced from both the Human Mortality Dataset (HMD) and the World Mortality Dataset (WMD) projects [16,17,18,19]. The projections on deaths, instead, come from just WMD. In particular, HMD maintains, on a weekly basis, an updated report on the deaths data, sourced from Eurostat and several national statistical agencies (including the Italian ISTAT). WMD, instead, is a dataset, currently serving 120 countries, whose contents is used to provide an estimate on the projected deaths of all those countries, on a per week basis. Essentially, with deaths data from the period 2015–2019, Karlinsky and Kobak (from WMD) have first fit a regression model for each region of interest (including Italy), and then used the model to project the expected deaths during the various weeks of the period 2020–2022 [18]. Finally, WMD's projected deaths are used by the OurWorldinData.org project as a baseline for estimating the Expected Deaths of the Eq (1) above. At the end of this long process, the OurWorldinData.org project provides temporal series of excess mortality, like those plotted in Figure 1 for Italy.
Figure 2 plots,instead,the number of daily confirmed COVID-19 deaths in Italy in the period February 26,2020–October 31,2022. For each day in the period,the 7-day average rolling is shown in the Figure,which means that the numbers of the daily confirmed deaths of the 7 latest days are taken,added up,and finally divided by 7. Note that the complete COVID-19 dataset on daily deaths is maintained again by the OurWorldinData.org project,with raw data on daily confirmed deaths from 219 countries (including Italy) sourced from the COVID-19 Data Repository by the Center for Systems Science and Engineering (CSSE) at Johns Hopkins University (JHU) [20]. JHU makes them available at the following public repository: https://github.com/owid/covid-19-data/tree/master/public/data/jhu.
The method we adopted for our investigations was a traditional peak detection analysis conducted on both the curves plotted in Figures 1 and 2. This is a common technique used to find the peaks in an incoming signal, and borrowed from fields like electrical signals, digital audio and seismic waves [14,15]. The basic algorithm is a great fit when peaks significantly emerge in comparison to the background data. Its easiest application is when one is looking for just the highest spike. In that case, one simply looks at each point in the data series and records the highest one seen so far. When the end of the data series is reached, the highest recorded value is the highest peak one is looking for.
Obviously, this simple procedure is only able to find just the highest peak in a series. If we are looking for multiple peaks in the same data series, we need to add some simple modifications to the basic procedure. as described before. Hence, based on recent literature on peak detection applied to the analysis of COVID-19 waves [21], we modified the basic procedure as follows:
As to the curve plotted in Figure 2 (i.e., daily confirmed COVID-19 deaths), two additional conditions were added that need to be satisfied for a point in the curve to be considered one of the highest peaks. First, we can say that a point in the curve, corresponding to a given day n of the daily confirmed deaths, can be considered one of the potential highest peaks, if the 7-day rolling average number of the daily confirmed deaths computed in that day is larger than the 7-day rolling average number of daily confirmed deaths recorded in all the 28 days, both before and after, n. Further, to consider that point in the curve as one of the highest peaks, the 7-day rolling average number of registered deaths on that day n has to be larger than a given mobile baseline, computed as the 85% of the cumulative number of deaths recorded on day n-1, averaged over all the days since the beginning of the pandemic until day n-1.
The motivation behind the choice of 28 days with a lower value of daily confirmed deaths comes from the definition of a COVID-19 wave provided in [22]. In that paper, it is shown that the three quarters of the upward periods before a peak of many studied COVID-19 waves typically last less than a month. This is also similar, for the downward periods. Having a baseline, instead, is useful whenever one is looking for multiple peaks in the same curve or wave. When the curve is examined in search for a new peak, if the current value drops below this baseline, that value cannot be considered as a peak. Vice versa, the current value is added as a new peak. In our case, the mobile baseline has been set at the 85% of the cumulative number of deaths averaged on all previous n-1 days [21].
Similar conditions need to be satisfied to consider a point in the curve of Figure 1 (i.e., the percentage excess mortality) as one of the highest peaks of that curve. Precisely, to consider day n as one where one of the highest peaks was hit, we need to have 28 days, both before and after day n, all with a value of the percentage excess mortality rate lower than the value recorded at day n. Moreover, the value of the percentage of excess mortality recorded on that day n should be larger than a given baseline. In the specific case of the percentage of excess mortality rates in Italy, the baseline value was chosen equal to 15.6%. The motivation for choosing this specific value comes from the already cited analysis conducted by ISTAT that considered the first year of the pandemic as the worst one from its beginning, with an average percentage of the all-cause excess mortality rate as large as 15.6% [13]. Different countries could decide for different values of the baseline, based on occasional country-specific considerations.
Note that all the data used for the peak detection analysis of the curves plotted in Figures 1 and 2 are available at the already mentioned public repositories, that is: https://github.com/owid/covid-19-data/tree/master/public/data/excess_mortality (percentage excess mortality of Figure 1) and: https://github.com/owid/covid-19-data/tree/master/public/data/jhu (daily confirmed COVID-19 deaths of Figure 2). The results of the peak detection calculations presented in the following section (Section 3) are reproducible by using the data and the methods described above. Given the simplicity of the described procedures, these calculations can be conducted with a simple calculator and a spreadsheet to temporarily record the data.
However, we also provide a simplified pseudo code of the corresponding multiple peaks detection algorithm in the following Table 1. The input of the algorithm is either the data of the curve of the all-cause excess mortality, or the data of the curve of the daily COVID-19 deaths, while the output are the corresponding peaks (lines 1–2 in Table 1). With the loop of lines 5–13 (Table 1), the entire input curve is searched for peaks. The term d represents either the value of the percentage mortality (in the case of the all-cause excess mortality curve) or the value of the daily number of deaths (in the case of the curve of the COVID-19 deaths). In particular, d(i) represents that specific value registered on a given day i. If d(i) is larger than the corresponding d values registered both on all the subsequent 28 days (line 6) and on all the previous 28 days (line 7), and d(i) is larger than the baseline (line 8), then a peak is detected on that day i (line 9), with its relative value (line 10).
Algorithm |
1: Input: All causes excess mortality curve or Daily COVID-19 deaths curve 2: Output: Peaks of the curve 3: begin 4: n = last data of the curve 5: for i = 1 to n increment 1 do 6: if {((d(i) > d (i+1)) & (d (i) > d (i+2)) & … & (d (i) > d (i+28))) & 7: ((d (i) > d(i-1)) & (d (i) > d (i-2)) & … & (d (i) > d (i-28))) & 8: (d (i) > baseline)} 9: then {peak(i) = i; 10: peak_value(i)= d(i)} 11: else {null} 12: end if 13: end for 14: end |
This study used publicly available, aggregated data that contains no private information. Therefore, ethical approval is not required.
Table 2 reports the results we obtained with our multiple peaks detection procedure when applied to: i) the curve of the percentage excess of mortality for all causes in Italy, in the period January 5, 2020–October 31, 2022, and ii) the curve of the daily confirmed COVID-19 deaths in Italy, in the period January 5, 2020–October 31, 2022.
Peak 1 | Peak 2 | Peak 3 | Peak 4 | Peak 5 | Peak 6 | ||
Excess Mortality | last day of the week with the highest peak | March 29, 2020 | November 29, 2020 | April 4, 2021 | December 19, 2021 | January 30, 2022 | July 24, 2022 |
corresponding value of % excess mortality | 85.6% | 55.7% | 26.2% | 16.2% | 16% | 35.5% | |
COVID-19 Deaths | date of the peak | April 1, 2020 | December 6, 2020 | April 13, 2021 | Not Applicable | February 4, 2022 | August 1, 2022 |
corresponding 7-day rolling average of deaths | 807 | 739 | 477 | Not Appl. | 376 | 175 | |
baseline in number of deaths | 302 | 179 | 236 | Not Appl. | 177 | 167 |
In particular, the first row of Table 2 presents all the peaks detected for the curve of the percentage excess of all causes of mortality, with the dates when they occurred and the corresponding percentage values. Those peaks were considered, as they had occurred on the last day of the corresponding week, and the excess mortality rates are given on a weekly basis. The six detected peaks were registered as occurring on the following dates: March 29, 2020, November 29, 2020, April 4, 2021, December 19, 2021, January 30, 2022 and July 24, 2022. They all have 28 days with lower values of the percentage excess of mortality, both before and after the days of the peaks. Moreover, they are all above the baseline of 15.6% [3]. In the second row of Table 2, all the five peaks of the curve of the daily confirmed COVID-19 deaths are reported as detected by our multiple peaks detection procedure, with their dates and their relative number of deaths (7-day rolling averages), plus the values of the corresponding mobile baseline computed per those dates. The considered period is January 5, 2020–October 31, 2022. The five detected peaks happened on the following dates: April 1, 2020; December 6, 2020; April 13, 2021; February 4, 2022; August 1, 2022. They all have 28 days, with lower values of the 7-day rolling average of the daily confirmed COVID-19 deaths, both before and after the days of the peaks. They are all above their baselines, set at different values depending on the peak dates.
If we compare the dates of the peaks of the percentage excess mortality curve with those of the daily confirmed COVID-19 deaths, we find that 5 out of the 6 highest peaks (83.3%) of the percentage excess mortality curve have occurred, on average, a week before the concomitant COVID-19 waves hit their highest peaks of daily confirmed deaths. In particular, Peak 1 of the daily confirmed COVID-19 deaths occurred 3 days after the corresponding Peak 1 of the percentage excess mortality. Peak 2 of the daily confirmed COVID-19 deaths occurred 7 days after the corresponding Peak 2 of the percentage excess mortality. Peak 3 of the daily confirmed COVID-19 deaths occurred 9 days after the corresponding Peak 3 of the percentage excess mortality. Peak 5 of the daily confirmed COVID-19 deaths occurred 5 days after the corresponding Peak 5 of the percentage excess mortality. Peak 6 of the daily confirmed COVID-19 deaths occurred 8 days after the corresponding Peak 6 of the percentage excess mortality, thus yielding a mean value of 6.4 days, with a standard deviation of 2.4 days. In Tables 3 and 4, we summarized the results above. The first rows of Table 3 shows the number of the days, between corresponding pairs of peaks of the two curves, while the first row of Table 4, reports the relative statistics: mean value and standard deviation, measured in days.
Peak 1 | Peak 2 | Peak 3 | Peak 4 | Peak 5 | Peak 6 | ||
Days between corresponding pairs of peaks of the two curves | 3 | 7 | 9 | Null | 5 | 8 |
Mean (days) | Std. Dev. (days) | |
Statistics relative to the number of days between corresponding pairs of peaks of the two curves | 6.4 | 2.4 |
To provide a graphical representation of the results reported in Table 2, we created Figures 3 and 4 plots, with red boxes, respectively: i) the highest peaks (six) of the curve of the percentage excess of mortality for all causes, and ii) the highest peaks (five) of the curve of the daily confirmed COVID-19 deaths in Italy.
One of the first issues to mention regarding the results we achieved is that, of the six highest peaks of the percentage excess mortality curve, only one (December 19, 2021) breaks the pattern, as there is no correspondence with any peak in the curve of the daily COVID-19 confirmed deaths. This single peak may tell us something more about the dynamic of the contagion in that specific period in Italy. In fact, that was the period when the Omicron variant began to take over in Italy, becoming seroprevalent very fast. If we look at the shape of the curve of the percentage excess mortality in that temporal interval, we notice a very anomalous situation, with two peaks very close each other (December 19, 2021 and January 30, 2022), both of the same magnitude, and with a kind of steep canyon in the middle of the two with the excess mortality almost falling to 0. This situation has all the characteristics of an outlier, caused by the advent of Omicron variant that changed the course of the contagion in Italy.
Another relevant issue is about the fact that, while the all-cause excess mortality has been considered a more appropriate measure of the total impact of the COVID-19 pandemic on deaths than the confirmed COVID-19 death count alone [16], the interpretation of the result of the difference between the excess mortality and the amount of confirmed COVID-19 death remains open to discussion. Hence, rather than developing complex statistical models that, in the end, have a hard time distinguishing between the proportion of excess mortality that was directly caused by COVID-19 and the number of deaths which were only an indirect consequence of the pandemic, we resorted to a simpler and more neutral method to investigate the hypothesis of an association between excess mortality and COVID-19 deaths. Essentially, we identified and counted all the highest peaks in the percentage excess mortality curve in Italy, and compared them to the highest peaks of the concomitant COVID-19 waves that have led to the maximum number of confirmed deaths.
Our peak detection analysis has showed that the highest peaks of the COVID-19 deaths curve happen, on average, only a week after that the percentage excess mortality curve has hit its maximal values. Ideally, one could notice that the pairs of peaks of the two curves should appear simultaneously. However, even if we cannot observe a perfect synchrony, the distance in days between the two peaks in each pair is, on average, very short (circa 6 days), and also the standard deviation is very low (circa 2 days). A plausible motivation for this short difference can be attributed mostly to delays in reporting.
Certainly, multiple confounding factors may have played a role in the final attribution of many of the COVID-19 deaths, but our opinion is that the almost perfect coincidence between when the percentage excess mortality curve peaked and the highest peaks of the COVID-19 deaths curve provide evidence in favor of the hypothesis of a positive correlation between COVID-19 deaths and all-cause excess mortality [23].
Another interesting source of discussion could be examining our results in the light of the variations happened in the mortality rates during the pandemic period due to several factors. It is quite sure, for example, that COVID-19 vaccinations have had an impact on the mortality during the various periods we have considered. The study developed in [24] is of particular interest to this aim. This analysis, in fact, shows the differences in mortality rates for COVID-19 amongst Italian physicians in 2020 and 2021, revealing that the mortality was 12 times higher in the period March–May 2020, compared to the same period in 2021 after the beginning of the vaccinations, at least in that specific group of workers. It is of similar relevance the investigation conducted in [25], where the occupational risk is discussed for healthcare workers who died a lot, especially at the beginning of the pandemic. This confirms that, at the beginning of the pandemic, the excess mortality was probably underestimated in various situations (e.g., elderly population and specific groups of workers) due to a combination of factors, including the lack of appropriate diagnostic tools, the absence of vaccines and various others.
Our approach has also several limitations. First, we recognize that, with our multiple peaks detection analysis, we have avoided quantifying the number of deaths precisely attributable to COVID-19. Nonetheless, we have followed this approach deliberately, in order to avoid to being trapped in the never-ending loop circa the correct estimate of the number of COVID-19 deaths in Italy. We have tried to look at this phenomenon from the perspective that a simple mathematical technique with neutrality, and regardless of the underlying discussion on what a COVID-19 death really is [26].
Another limitation of our study is concerned with the limited amount of time during which we have analyzed the percentage all-cause excess mortality curve. From the viewpoint of signal processing techniques, in fact, a period that returns only six peaks could be considered to offer just a limited perspective on the underlying phenomenon. However, one should consider that the period of time we have studied corresponds almost entirely to that of the pandemic. Our analysis has quit around the end of October 2022, for the simple reason that those are the latest data on the excess mortality weekly rates provided by the Italian statistical and medical authorities. Moreover, our opinion is that, if we were able to analyze the percentage excess mortality rate also in the residual period of November 2022–January 2023, this would add almost nothing to our findings. Also, considering that during that period in Italy, the count of COVID-19 deaths has continued to stabilize in an almost predictable, endemic state (with circa 70/80 deaths per day, on average), probably due to the high immunity degree achieved by the Italian population, with vaccinations and past infections. Finally, another limitation of this study resides in the use of Italian data only. The extension to different geographies could obviously result in more robust results, and it would allow us to discuss our findings and their implications in a broader and more challenging context.
With the scientific community that appears divided into two factions, which alternatively claim that the COVID-19 deaths are either underestimated or overestimated, we have investigated the correlation between excess mortality and confirmed COVID-19 deaths, in Italy. We compared the temporal series, looking for the corresponding highest peaks, and we found that 5 out of the 6 highest peaks of the excess mortality curve have occurred, on average, just a week before the concomitant COVID-19 waves hit their highest peaks of daily deaths. This provides evidence in favor of a correlation between COVID-19 deaths and all-cause excess mortality. With this analysis, we think we have provided an improved understanding of this issue, regardless of the presence of all the factors that have confounded the general scenario.
This research received no external funding.
The authors declare that there are no conflicts of interest.
[1] | World Health Organization: Coronavirus (COVID-19) Dashboard, 2023 Available from: https://covid19.who.int/. |
[2] | World Health Organization: International Guidelines for Certification and Classification (Coding) of COVID-19 as Cause of Death, 2023 Available from: https://www.who.int/publications/m/item/international-guidelines-for-certification-and-classification-(coding)-of-covid-19-as-cause-of-death. |
[3] | M. Lewiński, P Abreu, Arguing About "COVID": Metalinguistic arguments on what counts as a "COVID-19 Death", in The Pandemic of Argumentation, Springer, Cham, Switzerland, (2022), 17-41. https://doi.org/10.1007/978-3-030-91017-4_2 |
[4] |
M. C., Amoretti, E. Lalumera, COVID-19 as the underlying cause of death: disentangling facts and values, Hist. Philos. Life Sci., 43 (2021), 1-4. https://doi.org/10.1007/s40656-020-00355-6 doi: 10.1007/s40656-020-00355-6
![]() |
[5] |
B. I. B. Lindahl, COVID-19 and the selection problem in national cause-of-death statistics, Hist. Philos. Life Sci., 43 (2021), 43-72. https://doi.org/10.1007/s40656-021-00420-8 doi: 10.1007/s40656-021-00420-8
![]() |
[6] | N. Schwalbe, We could be vastly overestimating the death rate for COVID-19. Here's why. World Economic Forum, 2023. Available from: https://www.weforum.org/agenda/2020/04/we-could-be-vastly-overestimating-the-death-rate-for-covid-19-heres-why/ |
[7] |
D. Adam, The pandemic's true death toll: millions more than official counts, Nature, 601 (2022), 312-315. https://doi.org/10.1038/d41586-022-00104-8 doi: 10.1038/d41586-022-00104-8
![]() |
[8] |
P. Jha, Y. Deshmukh, C. Tumbe, W. Suraweera, A. Bhowmick, S. Sharma, et al., COVID mortality in India: National survey data and health facility deaths, Science, 375 (2022), 667-671. https://doi.org/10.1126/science.abm5154 doi: 10.1126/science.abm5154
![]() |
[9] | L. S. Versteergard, J. Nielsen, L. Richter, D. Schmid, N. Bustos, T. Braeye, et al., Excess all-cause mortality during the COVID-19 pandemic in Europe - preliminary pooled estimates from the EuroMOMO network, March to April 2020, Eurosurveillance, 25 (2020), 1-6. https://doi.org/10.2807/1560-7917.ES.2020.25.26.2001214 |
[10] | M. Dorucci, G. Minelli, S Boros, V. Manno, S. Prati, M. Battaglini, et al., Excess mortality in Italy during the COVID-19 pandemic: Assessing the differences between the first and the second wave, Year 2020, Front. Public Health, 9 (2020), 1-9. https://doi.org/10.3389/fpubh.2021.669209 |
[11] | COVID-19 Excess Mortality Collaborators, Estimating excess mortality due to the COVID-19 pandemic: a systematic analysis of COVID-19-related mortality, 2020-21, Lancet, 399 (2022), 1513-1536. https://doi.org/10.1016/S0140-6736(21)02796-3 |
[12] | World Health Organization: Coronavirus (COVID-19) Dashboard, 2023. Available from: https://covid19.who.int/region/euro/country/it. |
[13] | Italian Institute of Statistics: Epidemia COVID-19 in Italia - Anni 2020 - 2021, 2023. Available from: https://www.istat.it/it/files//2022/03/Epidemia-Covid-19_Infografica-accessibile.pdf. |
[14] | K. Harmer, G. Howells, W. Sheng, M. Fairhurst, F. Deravi, A Peak-Trough detection algorithm based on momentum, in Proceedings of IEEE 2008 Congress on Image and Signal Processing, (2008), 454-458. https://doi.org/10.1109/CISP.2008.704 |
[15] | G. K. Palshikar, Simple algorithms for peak detection in time series, in Proceedings of First Conference on Advanced Data Analysis, Business Analytics and Intelligence, 2009. |
[16] | E. Mathieu, H. Ritchie, L. Rodés Guirao, C. Appel, D. Gavrilov, C. Giattino, et al., Coronavirus Pandemic (COVID-19), 2023. Available from: https://ourworldindata.org/covid-deaths. |
[17] |
L. Németh, D. A. Jdanov, V. M. Shkolnikov, An open-sourced, web-based application to analyze weekly excess mortality based on the short-term mortality fluctuations data series, PLoS ONE, 16 (2021). https://doi.org/10.1371/journal.pone.0246663 doi: 10.1371/journal.pone.0246663
![]() |
[18] |
A. Karlinsky, D. Kobak, Tracking excess mortality across countries during the COVID-19 pandemic with the World Mortality Dataset, eLife, 2021, https://doi.org/10.7554/eLife.69336 doi: 10.7554/eLife.69336
![]() |
[19] | J. Aron, J. Muellbauer, A pandemic primer on excess mortality statistics and their compatibility across countries, 2023. Available from: https://ourworldindata.org/covid-excess-mortality. |
[20] |
M. Miller, Novel coronavirus COVID-19 (2019-nCoV) data repository, Bull. Assoc. Can. Map Libr. Arch., 164 (2020). https://doi.org/10.15353/acmla.n164.1730 doi: 10.15353/acmla.n164.1730
![]() |
[21] |
R. Cappi, L. Casini, D. Tosi, M. Roccetti, Questioning the seasonality of SARS-COV-2: a Fourier spectral analysis, BMJ Open, 12 (2022), e061602. https://doi.org/10.1136/bmjopen-2022-061602 doi: 10.1136/bmjopen-2022-061602
![]() |
[22] |
S. X. Zhang, F. Arroyo Marioli, R. Gao, S. Wang, A second wave? What do people mean by COVID waves? - a working definition of epidemic waves, Risk Manag. Healthc Policy, 14 (2021), 3775-82. https://doi.org/10.2147/RMHP.S326051 doi: 10.2147/RMHP.S326051
![]() |
[23] |
L. Casini, M. Roccetti, Reopening Italy's schools in September 2020: A Bayesian estimation of the change in the growth rate of new SARS-CoV-2 cases, BMJ Open, 2021. https://doi.org/10.1136/bmjopen-2021-051458 doi: 10.1136/bmjopen-2021-051458
![]() |
[24] |
L. Modenese, T. Loney, F. Gobba, COVID-19-related mortality amongst physicians in Italy: Trend pre- and post-SARS-CoV-2 vaccination campaign, Healthcare, 10 (2022). https://doi.org/10.3390/healthcare10071187 doi: 10.3390/healthcare10071187
![]() |
[25] |
L. Modenese, F. Gobba, Increased risk of COVID-19-related deaths among general practitioners in Italy, Healthcare, 8 (2020). https://doi.org/10.3390/healthcare8020155 doi: 10.3390/healthcare8020155
![]() |
[26] |
K. Jabłońska, S. Aballéa, M. Toumic, Factors influencing the COVID-19 daily deaths' peak across European countries, Public Health, 194 (2021), 135-142. https://doi.org/10.1016/j.puhe.2021.02.037 doi: 10.1016/j.puhe.2021.02.037
![]() |
1. | Marco Roccetti, Predictive health intelligence: Potential, limitations and sense making, 2023, 20, 1551-0018, 10459, 10.3934/mbe.2023460 | |
2. | Mauro Gaspari, The impact of test positivity on surveillance with asymptomatic carriers, 2022, 11, 2161-962X, 10.1515/em-2022-0125 | |
3. | Mauro Gaspari, A Low-Cost Early Warning Method for Infectious Diseases with Asymptomatic Carriers, 2024, 12, 2227-9032, 469, 10.3390/healthcare12040469 | |
4. | Dharwada Sesha Sriram, Aseem Ranjan, Vedant Ghuge, Naveen Rathore, Raghav Agarwal, Tausif Diwan, Jitendra V. Tembhurne, Personalized federated learning for the detection of COVID-19, 2023, 83, 1573-7721, 29067, 10.1007/s11042-023-16810-y | |
5. | Marco Roccetti, Drawing a parallel between the trend of confirmed COVID-19 deaths in the winters of 2022/2023 and 2023/2024 in Italy, with a prediction, 2024, 21, 1551-0018, 3742, 10.3934/mbe.2024165 | |
6. | F. Scott, G. McCartney, D. Walsh, S. Wild, D. Rae, J. Ramsay, G. Donaghy, M. Douglas, Explanations for higher-than-expected all-cause mortality from April 2021: A scoping review, 2025, 238, 00333506, 73, 10.1016/j.puhe.2024.10.010 | |
7. | Nádia Cristina Pinheiro Rodrigues, Mônica Kramer de Noronha Andrade, Evaluation of post-COVID mortality risk in cases classified as severe acute respiratory syndrome in Brazil: a longitudinal study for medium and long term, 2024, 11, 2296-858X, 10.3389/fmed.2024.1495428 |
Algorithm |
1: Input: All causes excess mortality curve or Daily COVID-19 deaths curve 2: Output: Peaks of the curve 3: begin 4: n = last data of the curve 5: for i = 1 to n increment 1 do 6: if {((d(i) > d (i+1)) & (d (i) > d (i+2)) & … & (d (i) > d (i+28))) & 7: ((d (i) > d(i-1)) & (d (i) > d (i-2)) & … & (d (i) > d (i-28))) & 8: (d (i) > baseline)} 9: then {peak(i) = i; 10: peak_value(i)= d(i)} 11: else {null} 12: end if 13: end for 14: end |
Peak 1 | Peak 2 | Peak 3 | Peak 4 | Peak 5 | Peak 6 | ||
Excess Mortality | last day of the week with the highest peak | March 29, 2020 | November 29, 2020 | April 4, 2021 | December 19, 2021 | January 30, 2022 | July 24, 2022 |
corresponding value of % excess mortality | 85.6% | 55.7% | 26.2% | 16.2% | 16% | 35.5% | |
COVID-19 Deaths | date of the peak | April 1, 2020 | December 6, 2020 | April 13, 2021 | Not Applicable | February 4, 2022 | August 1, 2022 |
corresponding 7-day rolling average of deaths | 807 | 739 | 477 | Not Appl. | 376 | 175 | |
baseline in number of deaths | 302 | 179 | 236 | Not Appl. | 177 | 167 |
Peak 1 | Peak 2 | Peak 3 | Peak 4 | Peak 5 | Peak 6 | ||
Days between corresponding pairs of peaks of the two curves | 3 | 7 | 9 | Null | 5 | 8 |
Mean (days) | Std. Dev. (days) | |
Statistics relative to the number of days between corresponding pairs of peaks of the two curves | 6.4 | 2.4 |
Algorithm |
1: Input: All causes excess mortality curve or Daily COVID-19 deaths curve 2: Output: Peaks of the curve 3: begin 4: n = last data of the curve 5: for i = 1 to n increment 1 do 6: if {((d(i) > d (i+1)) & (d (i) > d (i+2)) & … & (d (i) > d (i+28))) & 7: ((d (i) > d(i-1)) & (d (i) > d (i-2)) & … & (d (i) > d (i-28))) & 8: (d (i) > baseline)} 9: then {peak(i) = i; 10: peak_value(i)= d(i)} 11: else {null} 12: end if 13: end for 14: end |
Peak 1 | Peak 2 | Peak 3 | Peak 4 | Peak 5 | Peak 6 | ||
Excess Mortality | last day of the week with the highest peak | March 29, 2020 | November 29, 2020 | April 4, 2021 | December 19, 2021 | January 30, 2022 | July 24, 2022 |
corresponding value of % excess mortality | 85.6% | 55.7% | 26.2% | 16.2% | 16% | 35.5% | |
COVID-19 Deaths | date of the peak | April 1, 2020 | December 6, 2020 | April 13, 2021 | Not Applicable | February 4, 2022 | August 1, 2022 |
corresponding 7-day rolling average of deaths | 807 | 739 | 477 | Not Appl. | 376 | 175 | |
baseline in number of deaths | 302 | 179 | 236 | Not Appl. | 177 | 167 |
Peak 1 | Peak 2 | Peak 3 | Peak 4 | Peak 5 | Peak 6 | ||
Days between corresponding pairs of peaks of the two curves | 3 | 7 | 9 | Null | 5 | 8 |
Mean (days) | Std. Dev. (days) | |
Statistics relative to the number of days between corresponding pairs of peaks of the two curves | 6.4 | 2.4 |