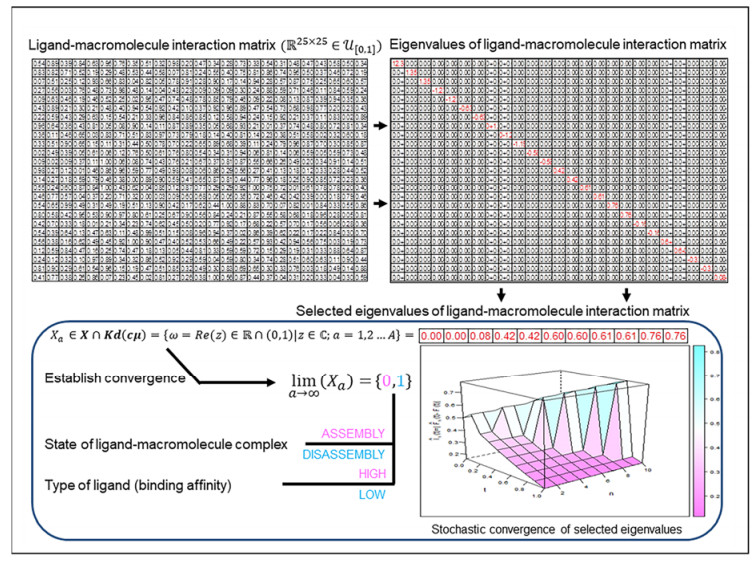
In the traditional person re-identification model, the CNN network is usually used for feature extraction. When converting the feature map into a feature vector, a large number of convolution operations are used to reduce the size of the feature map. In CNN, since the receptive field of the latter layer is obtained by convolution operation on the feature map of the previous layer, the size of this local receptive field is limited, and the computational cost is large. For these problems, combined with the self-attention characteristics of Transformer, an end-to-end person re-identification model (twinsReID) is designed that integrates feature information between levels in this article. For Transformer, the output of each layer is the correlation between its previous layer and other elements. This operation is equivalent to the global receptive field because each element needs to calculate the correlation with other elements, and the calculation is simple, so its cost is small. From these perspectives, Transformer has certain advantages over CNN's convolution operation. This paper uses Twins-SVT Transformer to replace the CNN network, combines the features extracted from the two different stages and divides them into two branches. First, convolve the feature map to obtain a fine-grained feature map, perform global adaptive average pooling on the second branch to obtain the feature vector. Then divide the feature map level into two sections, perform global adaptive average pooling on each. These three feature vectors are obtained and sent to the Triplet Loss respectively. After sending the feature vectors to the fully connected layer, the output is input to the Cross-Entropy Loss and Center-Loss. The model is verified On the Market-1501 dataset in the experiments. The mAP/rank1 index reaches 85.4%/93.7%, and reaches 93.6%/94.9% after reranking. The statistics of the parameters show that the parameters of the model are less than those of the traditional CNN model.
Citation: Keying Jin, Jiahao Zhai, Yunyuan Gao. TwinsReID: Person re-identification based on twins transformer's multi-level features[J]. Mathematical Biosciences and Engineering, 2023, 20(2): 2110-2130. doi: 10.3934/mbe.2023098
[1] | Christian Winkel, Simon Neumann, Christina Surulescu, Peter Scheurich . A minimal mathematical model for the initial molecular interactions of death receptor signalling. Mathematical Biosciences and Engineering, 2012, 9(3): 663-683. doi: 10.3934/mbe.2012.9.663 |
[2] | Hong Yuan, Jing Huang, Jin Li . Protein-ligand binding affinity prediction model based on graph attention network. Mathematical Biosciences and Engineering, 2021, 18(6): 9148-9162. doi: 10.3934/mbe.2021451 |
[3] | Yuewu Liu, Mengfang Zeng, Shengyong Liu, Chun Li . Dynamics analysis of building block synthesis reactions for virus assembly in vitro. Mathematical Biosciences and Engineering, 2023, 20(2): 4082-4102. doi: 10.3934/mbe.2023191 |
[4] | Ronald Lai, Trachette L. Jackson . A Mathematical Model of Receptor-Mediated Apoptosis: Dying to Know Why FasL is a Trimer. Mathematical Biosciences and Engineering, 2004, 1(2): 325-338. doi: 10.3934/mbe.2004.1.325 |
[5] | Max-Olivier Hongler, Roger Filliger, Olivier Gallay . Local versus nonlocal barycentric interactions in 1D agent dynamics. Mathematical Biosciences and Engineering, 2014, 11(2): 303-315. doi: 10.3934/mbe.2014.11.303 |
[6] | Feng Rao, Carlos Castillo-Chavez, Yun Kang . Dynamics of a stochastic delayed Harrison-type predation model: Effects of delay and stochastic components. Mathematical Biosciences and Engineering, 2018, 15(6): 1401-1423. doi: 10.3934/mbe.2018064 |
[7] | Yutong Man, Guangming Liu, Kuo Yang, Xuezhong Zhou . SNFM: A semi-supervised NMF algorithm for detecting biological functional modules. Mathematical Biosciences and Engineering, 2019, 16(4): 1933-1948. doi: 10.3934/mbe.2019094 |
[8] | O. E. Adebayo, S. Urcun, G. Rolin, S. P. A. Bordas, D. Trucu, R. Eftimie . Mathematical investigation of normal and abnormal wound healing dynamics: local and non-local models. Mathematical Biosciences and Engineering, 2023, 20(9): 17446-17498. doi: 10.3934/mbe.2023776 |
[9] | Zhenzhen Zheng, Ching-Shan Chou, Tau-Mu Yi, Qing Nie . Mathematical analysis of steady-state solutions in compartment and continuum models of cell polarization. Mathematical Biosciences and Engineering, 2011, 8(4): 1135-1168. doi: 10.3934/mbe.2011.8.1135 |
[10] | Linlu Song, Shangbo Ning, Jinxuan Hou, Yunjie Zhao . Performance of protein-ligand docking with CDK4/6 inhibitors: a case study. Mathematical Biosciences and Engineering, 2021, 18(1): 456-470. doi: 10.3934/mbe.2021025 |
In the traditional person re-identification model, the CNN network is usually used for feature extraction. When converting the feature map into a feature vector, a large number of convolution operations are used to reduce the size of the feature map. In CNN, since the receptive field of the latter layer is obtained by convolution operation on the feature map of the previous layer, the size of this local receptive field is limited, and the computational cost is large. For these problems, combined with the self-attention characteristics of Transformer, an end-to-end person re-identification model (twinsReID) is designed that integrates feature information between levels in this article. For Transformer, the output of each layer is the correlation between its previous layer and other elements. This operation is equivalent to the global receptive field because each element needs to calculate the correlation with other elements, and the calculation is simple, so its cost is small. From these perspectives, Transformer has certain advantages over CNN's convolution operation. This paper uses Twins-SVT Transformer to replace the CNN network, combines the features extracted from the two different stages and divides them into two branches. First, convolve the feature map to obtain a fine-grained feature map, perform global adaptive average pooling on the second branch to obtain the feature vector. Then divide the feature map level into two sections, perform global adaptive average pooling on each. These three feature vectors are obtained and sent to the Triplet Loss respectively. After sending the feature vectors to the fully connected layer, the output is input to the Cross-Entropy Loss and Center-Loss. The model is verified On the Market-1501 dataset in the experiments. The mAP/rank1 index reaches 85.4%/93.7%, and reaches 93.6%/94.9% after reranking. The statistics of the parameters show that the parameters of the model are less than those of the traditional CNN model.
Ligands, are biochemical modifiers of macromolecular structure and can impact biological function. These can be co-factors (iron, cobalt, copper, zinc), co-enzymes such as Nicotinamide- and Flavin- Adenine Dinucleotides (NAD, FAD) and full-length molecules with short binding sites [1,2]. Ligands, unlike substrates/co-substrates are either reversibly altered or not at all. The biochemical role of ligands, in vivo, is complex and can influence both, enzyme-mediated substrate catalysis and non-enzymatic association and dissociation interactions. Whilst, the interaction with competitive inhibitors, co-factors or co-enzymes involves definitive and direct modifications to the active site residues, the effect of a ligand can be allosteric and indirect [3,4,5]. The latter involves both long-distance conformational changes and non-covalent interactions (hydrogen, Van der Waals, hydrophobic, electrostatic) [6,7,8,9,10,11]. Empirical data suggests that the binding affinity or the strength-of-association of a macromolecule for its ligand is a critical determinant of function [7,8,9,10,11]. For example, 2, 3-Bisphophoglycerate is a potent modifier of Hemoglobin function and does so by shifting the oxygen-dissociation curve to the right. In its absence Hemoglobin retains high affinity for molecular oxygen (left-shift of the oxygen dissociation curve), an undesirable effect on its role as a transporter [10,11]. Similarly, Ascorbic acid maintains iron in its reduced state in the gastrointestinal tract and as part of the active site of non-haem iron (Ⅱ)- and 2-oxoglutarate-dependent dioxygenases [12,13]. Deficiency of Ascorbic acid is implicated in tardy iron absorption in the ileum as well as a range of collagen disorders such as scurvy (Prolyl- and Lysyl-hydroxylases) [14,15]. Conversely, proteins which are modified such as those that may originate from missense or amino acid substitutions and are secondary to genomic variants such as single nucleotide polymorphisms (SNPs) and insertions-deletions (indels), will also result in several clinical outcomes [16,17]. Here, too, enzyme catalysis is directly affected if these are present at the active site or is impacted indirectly (folding, stability, complex formation) when present elsewhere [16,17,18].
There is a large volume of literature which describes macromolecules in terms of either residues (amino acids, nucleotides) interacting or an all atom-based interaction matrix. The proponent of the 2D approach is the Gaussian network model (GNM), while the Anisotropic network model (ANM) is representative of the 3D approach [19,20,21,22]. The fundamental premise of both these approaches is the elastic network model (ENM) [19]. Here, an atom or residue is modeled as an elastic mass and the interaction between a pair of atoms/residues is dependent on the selection of a pre-determined cut-off distance [22]. The force constant, although, not a parameter by definition, has also been studied and shows good correlation with B-factor data [23]. A major application of these studies is normal mode analysis (NMA), which has been used to glean valuable insights into the structural dynamics of the investigated macromolecule and into B-factor distribution [23,24]. Despite this success, there are significant limitations of this approach, including inadequate descriptors for the type of interactions computed by the Hessian matrix and data points that are dependent on a preselected cut-off distance (5–10 Ang, GNM; 10–15 Ang, ANM) [20,22,25]. Parameter-free versions of the ENM (pfENM), GNM (pfGNM) and ANM (pfANM), to resolve the latter, have been described and compared to establish B-factor distribution (isotropic, anisotropic) [25]. Additionally, many of these studies have focused on inferring biophysical characteristics such as cross-correlational fluctuations and mean square displacements. From a functional standpoint, however, it is not clear whether these data can be utilized to derive/study parameters such as the Michaelis-Menten constant (Km) or the association/dissociation (Ka/Kd). Since, these depend on the presence of an organic or inorganic modifier, i.e., ligand/substrate/co-factor/co-substrate, in addition to the modeled macromolecule, its exclusion is another major lacuna of these studies.
Despite the availability of clinical, empirical, analytical and computational data, a mathematically rigorous explanation for the heterogeneity in biochemical function, for a ligand-macromolecular complex, is missing. The work presented models a ligand and macromolecule as a homo- or hetero-dimer and subsumes a finite and equal number of atoms/residues per monomer. The pairwise interactions of the resulting square matrix will be chosen randomly from a standard uniform distribution. The resulting eigenvalues will be analyzed and modeled in accordance with known literature on biochemical reactions to generate biologically viable and usable dissociation constants. The theoretical results will be complemented by numerical studies where applicable. Additionally, and through various theorems, lemmas and corollaries, a schema to partition ligands into high- and low-affinity variants will also be discussed. The suitability of the transition-state dissociation constants as a model for ligand-macromolecular interactions will be inferentially assessed by analyzing the clinical outcomes of amino acid substitutions of selected enzyme homodimers. The relevance of the model to biochemical function will be discussed by examining the ligand-macromolecular complex for known ligands of non-haem iron (Ⅱ)- and 2-oxoglutarate-dependent dioxygenases (Fe2OG) and the major histocompatibility complex (Ⅰ) (MHC1).
The general outline of the manuscript includes an initial section where the system to be modeled, rationale for this study, and formal definitions are introduced (Section 2). The model is analyzed, formulated and presented as theorems, lemmas and corollaries (Section 3). This section also includes a numerical study to demonstrate and validate the theoretical assertions made. The biological relevance of these findings are discussed with an analysis of clinical outcomes of enzyme sequence variants and case studies of enzyme- and non-enzymatic complex formation (Section 4). A brief conclusion that summarizes the presented study, limitations and future directions is included at the end of the manuscript (Section 5). Details of all proofs are included after the conclusions (Section 6).
Consider the generic interaction between macromolecule (c) and ligand (μ),
cμrf→←rbc+μRXN(1) |
We can represent this interaction/reaction, at a steady state, with the rate equations [26],
Rd(cμ)=rf.[cμ]Lcμ≥0 | (1) |
Ra(cμ)=rb.[c]Lc≥0[μ]Lμ≥0 | (2) |
At steady state,
Rd(cμ)=Ra(cμ) | (3) |
⇒rfrb=[c]Lc≥0.[μ]Lμ≥0[cμ]Lcμ≥0 | (4) |
rfrb=Kd(cμ) | (5) |
Here,
c:=Macromolecule |
μ:=Ligand |
[.]:=Molarconcentrationofreactantinstandardform(M) |
Ra(cμ):=Rateofassociationofcomplex(Ms−1) |
Rd(cμ):=Rateofdissociationofcomplex(Ms−1) |
rf:=Rateconstantsofforwardreaction(s−1) |
rb:=Rateconstantsofreversereaction(M−1s−1) |
L:=Stoichiometryofreactant(s) |
Kd(cμ):=Dissociationconstantforligand−macromolecularcomplex(M) |
It is clear that a ligand-macromolecular complex may exist in one of three distinct states. These include: a) perfect association, b) perfect dissociation and c) an intermediate- or transition-state; and can be represented in terms of the dissociation constant,
Case (1) Perfectassociation Def. (1)
1rb→∞;rf→0 | (6, 7) |
⇒Kd(cμ)≈0 | (8) |
Case (2) Perfectdisassociation Def. (2)
rf>>>rb | (9) |
⇒Kd(cμ)≥1 | (10) |
Case (3) Transient−statedisassociationconstant Def. (3)
rf≶rb | (11) |
⇒Kd(cμ)∈R∩(0,1) | (12) |
Consider an atom/residue-based representation (amino acids/nucleotides) of a generic set of monomeric macromolecules, C={protein,DNA,RNA}, with z=1,2,….,Z components each with i-indexed (i=1,2,….,I) c-atoms/residues,
c≡Cz∈C|c=[c1c2….ci=I]T,I∈N | (13) |
The analogous model of a monomer ligand, L={smallmolecule,peptide,oligonucleotide}, with j-indexed (j=1,2,…,J) μ-atoms/residues is,
μ∈L|μμ=[μ1μ2….μj=J],J∈N | (14) |
It is also assumed that the ligand-macromolecular complex is a homo- or hetero-dimer with an equal number of atoms/residues (I=J) per monomer. The interaction matrix is,
⟨c|μ⟩=[c1c2..ci=I]T×[μ1μ2..μj=J]=Czμ=(ciμj)⊂RI×J | (15) |
The numerical values of this matrix are chosen randomly from the standard uniform distribution,
Czμ=(ciμj)∈U[0,1] | (16) |
The rationale for this choice is that each pairwise interaction is subsumed to be a function of an arbitrary number of non-bonded interactions (long- and short-range) and is therefore, unique. Clearly, this implies the existence of {I,J}-linear independent vectors,
rank(Czμ)={I,J} | (17) |
Since Czμ is diagonalizable there exists a diagonal matrix, KCzμ,
KCzμ=X−1CzμX | (18) |
zi=j=z∈diag(KCzμ)⊂C | (19) |
Czμ, is non-symmetric the computed eigenvalues of the modeled ligand-macromolecular interaction matrix can have positive and negative real parts,
{αij=Re(z)∈Kd(Czμ)⊂R|i=j,z∈C} | (20) |
{αij=Re(z)∈Kd(Czμ)⊂R|i=j,z∈C} | (20.1) |
A further subdivision can be made in accordance with established literature,
{αij=Re(z)∈Kd(Czμ)⊂R|i=j,z∈C}ω∞=Kd(cμ)≥1 | (21) |
Perfectassociation≡Reverseω0=Kd(cμ)=0 | (22) |
This selection generates the set,
ω=αij∈Kd(Czμ)⊂R∩(0,1) | (23) |
ω∈Kd(cμ)Def.(4) |
#Kd(cμ)=A | (23.1) |
We can combine these to get a preliminary definition of the transition-state disassociation constants. These are the strictly positive real part of all complex eigenvalues that characterize a ligand-macromolecular complex with an equal number of atoms/residues per monomer and belong to the open interval (0, 1),
{ω=Re(z)∈Kd(cμ)⊂Kd(Czμ)⊂R∩(0,1)|z∈C} | (Def.(5a)) |
Whilst, the states of perfect association and dissociation are key determinants of whether a reaction will occur or not, the transition-state dissociation constants may offer insights into the origins of threshold values, feedback mechanisms and other regulatory checkpoints. However, in order to ascribe biological relevance to these findings we must establish various bounds which can then be utilized to assess and thence assay the function of a ligand-macromolecular complex.
Theorem 1 (T1): The linear map between the transition-state dissociation constants and the eigenvalues that characterize the interactions of the homo- or hetero-dimer form of the modeled ligand-macromolecular complex is the injection,
g:ω∈Kd(cμ)↦Kd(Czμ) | (24) |
Theorem 2 (T2): The transition-state dissociation constants that characterize the interactions of the homo- or hetero-dimer form of the modeled ligand-macromolecular complex is a monotonic and non-increasing sequence,
{ωa}a≤a+1|ω∈Kd(cμ) | (25) |
Theorem 3 (T3): The transition-state dissociation constants that characterize the interactions of the homo- or hetero-dimer form of the modeled ligand-macromolecule complex are monotonic, bounded and therefore, convergent,
lima→∞{ωa}a≤a+1={0,1}|ω∈Kd(cμ) | (26) |
Corollary 1 (C1): The transition-state dissociation constants that characterize the interactions of the homo- or hetero-dimer form of the modeled ligand-macromolecule complex is a sequence with defined greatest-lower and least-upper -bounds,
inf{ωa}a≤a+1<{ωa}a≤a+1<sup{ωa}a≤a+1 | (27) |
Corollary 2 (C2; without proof): The cardinality of the set of transition-state dissociation constants that characterize the interactions of the homo- or hetero-dimer form of the modeled ligand-macromolecule complex is finite,
#Kd(cμ)=A<#Kd(Czμ)={I,J} | (28) |
Using T1–T3 and C1, C2 we can refine our definition of the transition-state dissociation constants for the modeled ligand-macromolecular complex,
{ωa}a≤a+1|ω∈Kd(cμ);a=1,2…A | (Def.(5b)) |
where,
ω=Re(z)∈Kd(Czμ)⊂R∩(0,1)|z∈C |
It is clear from the above results that the eigenvalue-based transition-state dissociation constants are continuous and can potentially model the multiplicity of intermediate- or transient-states that a ligand-macromolecular complex may adopt. It should therefore, be possible to partition the transition-state dissociation constants into functionally distinct subsets and will be characteristic for a specific ligand-macromolecular complex.
Theorem 4 (T4): The transition-state dissociation constants that characterize the interactions of the homo- or hetero-dimer form of the modeled ligand-macromolecule complex is a proper subset of the complete set of the real part of all complex eigenvalues that comprise the interaction matrix,
Kd(cμ)⊂Kd(Czμ) | (29) |
Corollary 3 (C3): The distribution of the transition-state dissociation constants that characterize the interactions of the homo- or hetero-dimer form of the modeled ligand-macromolecule complex will result in a schema by which we can annotate the ligand as a high (μhigh)- or low (μlow)-affinity variant,
μ={μhigh,μlow} | (30) |
Biologically relevant macromolecular complexes are characterized by heterogeneity and high-order (Z≥2). This multimer-form of a macromolecule is formed around a primary molecule and its interactions. These may be protein-protein, DNA/RNA-protein or DNA-RNA-protein.
The multimer-form of ligand-macromolecular complex is easily modeled using the mathematical framework defined earlier. Here, the ligand-macromolecular complex is considered as a set of interacting monomers and the binding to a ligand occurs via a single unique monomer,
{Cz∈C|C1=C2⋯=Cz=Z|Z≥2} | (Def.(6a)) |
where,
Cz≡Czμ≡μCz | (Def.(6b)) |
Here,
μ:=Ligand |
Cz:=Uniquemonomerofamacromoleculethatassociateswithaligand |
μCz:=Ligand−macromolecularcomplex |
On the basis of these definitions we can re-index the remaining monomers, i.e., after excluding the unique monomer that binds to the ligand,
{Cy∈˜C=C∖{Cz}|y=1,2…Y;Y=Z−1} | (Def.(7)) |
We now derive an expression for the multimer (higher-order)-form of a ligand-macromolecular complex.
Theorem 5 (T5): The multimer-form of a ligand-macromolecular complex comprising identical monomer units and with an arbitrary unit associating with a ligand is,
∏y=Yy=1Cz.Cy=Cz.CyY|y=1,2…Y;Y=Z−1;Z≥2;z=1,2…Z | (31) |
Rewriting, this result in terms of the definition of the multimer form of a ligand-macromolecular complex,
Cz.CY≡μCz.CY | (Def.(8)) |
Theorem 6 (T6): The linear map between the transition-state dissociation constants that characterize the interactions of the monomer- and multimer-forms of a ligand-macromolecular complex is a bijection,
h−1∘h:ω∈Kd(cμ)↔u∈Kd(μCz) | (32) |
Theorem 7 (T7): The linear map between the transition-state dissociation constants and the eigenvalues that characterize the multimer-form of a ligand-macromolecular complex is a composition and an injection,
g∘h−1∘h:u∈Kd(μCz.CY)↦Kd(Czμ) | (33) |
The aforementioned theoretical results establish the mathematical rigor behind the definition and development of the transition-state dissociation constants as a model for ligand-macromolecular interactions (T1–T7, C1–C3). These assertions are complemented and numerically validated in R-4.1.2. Here, the R-packages, "ConvergenceConcepts" and "pracma" are utilized to investigate and analyze the stochastic convergence of the eigenvalues generated by the interaction matrix of a ligand-macromolecular complex (Supplementary Text 1) [27]. The R-scripts to establish convergence along with data processing are developed in-house (Supplementary Text 2). The stepwise algorithm to compute and numerically validate the transition-state dissociation constants is presented (Figure 1).
Step 1: A ligand and macromolecule with an equal number of atoms/residues (n=25) is chosen. Whilst, the complex can be modeled as a perfect homodimer, imperfect forms such as alternatively spliced isoenzymes are prevalent and commonly observed. Alternatively, the ligand can be modeled as a different macromolecule altogether.
Step 2: Populate the square interaction matrix with values randomly chosen from a uniform distribution, U[0,1]. These will represent one of three potential states for each interacting pair of atoms/residues of the modeled ligand-macromolecular complex (association, complete disassembly, transition-state).
Step 3: Compute the complex eigenvalues of this matrix and extract the real part of each.
Step 4: Form a sequence of the subset comprising those values that are strictly positive and belong to the open interval (0,1).
Step 5: Establish the stochastic convergence in distribution and/or probability of the terms of this sequence to the expected upper (tsup)- and lower (tinf)-bounds, i.e., 0 and 1.
Step 5.1: Construct a sequence of random numbers, X, whose elements are uniquely mapped to the eigenvalue-based transition-state dissociation constants and represent intermediate- or transition-states of the modeled ligand-macromolecular complex,
{Xa∈X∩Kd(cμ)⊂R∩(0,1)|a=1,2…A} | (Def.(9)) |
Here,
A=#(X∩Kd(cμ)) | (34) |
Step 5.2: Establish convergence of this set of random numbers. Here, weak convergence will suffice (distribution, probability),
lima→∞(Xa)→{tinf,tsup}={0,1} | (35) |
The parameters to accomplish this numerically are,
nmax:=NumberofvaluestoanalyseM:=Numberofpathsε:=Thresholdvaluetinf:=Lowerlimitofintervaltoestablishconvergencetsup:=Upperlimitofintervaltoestablishconvergence |
The values of these parameters for the numerically studied example are,
nmax=A=11 | (35.1) |
M=500 | (35.2) |
ε=0.01 | (35.3) |
tinf=0 | (35.4) |
tsup=1 | (35.5) |
The eigenvalue-based model of transition-state dissociation constants of a ligand-macromolecular complex asserts that there are several intermediate- or transition-states of a complex and that each of these has the potential to modify the biochemical process that the complex participates in.
Ligand-macromolecular complexes, in vivo, possess a finite and in most cases, an incomparable number of atoms/residues. The theoretical results establish definition(s), bounds and metrics to assess biochemical function for both, monomer (T1–T4, C1–C3)- and multimer (T5–T7)-forms. The numerical data suggests that the set of transition-state dissociation constants can be finite, converge and retain statistical relevance (Figure 1).
Proposition (P): The transition-state dissociation constants for the monomer (z=Z=1)- and multimer (Z≥2)-forms of a ligand-macromolecular complex with a finite number of atoms/residues of each (I,J) per monomer,
{u∈Kd(μCz.CY)|Cz∈C,μ∈L;z=1,2…Z;A={I,J}} | (Def.(10)) |
is the finite set,
Kd(μCz.CY)⊂Kd(Czμ) |
Here,
I=#Cz|Cz∈C | (36) |
J=#μ|μ∈L | (37) |
where,
Kd(.):=Setofconstrainedeigenvalue−basedtransition−statedissociationconstants |
I:=Finitenumberofatomsorresiduesofmacromolecule |
J:=Finitenumberofatomsorresiduesofligand |
Cμz.CY:=Multimerformofligand−macromolecularcomplex |
Enzyme-mediated catalysis, or lack thereof, results in metabolic enzyme disorders and may be inherited (inborn errors of metabolism) or acquired [28]. In order to assess the biomedical relevance of modeling ligand-macromolecule interactions as transition-state dissociation constants, the clinical outcomes of amino acid substitutions of selected enzyme homo- or hetero-dimers are examined (Table 1). These outcomes, i.e., benign, likely benign, pathologic, likely pathologic, conflicting, uncertain significance, are defined in accordance with the prevalent nomenclature of the ClinVar database [16]. Here, the data annotated as "uncertain significance" are those sequence variants with a high likelihood (≈90–95%) of being "benign" or "pathogenic" [17]. This means they are likely to classified as "true positive", and if ignored will result in a "false negative". On the other hand, an outcome designated as with a "conflicting interpretation" is likely to be due to unresolved contradictory findings in the presence or absence of confounding factors. If we assume perfect contradiction, i.e., 50%, and couple this with the previous result, we get a ≈70–73% possibility that the variant of interest is a "true positive". This means, that here too, if missed a "false negative" will result. The metric of choice is the Recall (R) percentage,
R=TPTP+FN×100 | (38) |
R:=Recall |
TP:=Knownpositives(benign,likelybenign,pathogenic,likelypathogenic) | (Def.(12)) |
FN:=Likelypositives(conflictingdata,uncertainsignificance) | (Def.(13)) |
Enzyme | EC | CV | SNP | M | Co | US | B | LB | P | LP | FN | TP | R (%) | |
1 | Glucokinase | 2.7.1.2 | 778 | 619 | 370 | 49 | 157 | 4 | 4 | 65 | 113 | 206 | 186 | 47.45 |
2 | Pyruvate kinase | 2.7.1.40 | 1239 | 629 | 209 | 14 | 135 | 7 | 7 | 30 | 20 | 149 | 64 | 30.05 |
3 | Cathepsin A | 3.4.16.x | 1776 | 1270 | 501 | 20 | 382 | 26 | 23 | 26 | 34 | 402 | 109 | 21.33 |
4 | Pyruvate dehydrogenase | 1.2.4.1 | 2317 | 1591 | 584 | 25 | 391 | 35 | 46 | 62 | 43 | 416 | 186 | 30.9 |
5 | Phosphofructokinase 1 | 2.7.1.11 | 165 | 32 | 10 | 2 | 4 | 2 | 1 | 1 | 1 | 6 | 5 | 45.45 |
6 | Phosphofructokinase 2 | 2.7.1.105 | 206 | 76 | 23 | 1 | 17 | 1 | 1 | 2 | 1 | 18 | 5 | 21.74 |
7 | Cystathione beta-synthase | 4.2.1.22 | 930 | 744 | 255 | 21 | 172 | 3 | 4 | 46 | 32 | 193 | 85 | 30.58 |
8 | DNA topoisomerase Ⅱ | 5.6.2.2 | 466 | 319 | 152 | 0 | 122 | 11 | 14 | 3 | 1 | 122 | 29 | 19.21 |
9 | Guanylate cyclase 1 | 4.6.1.2 | 1572 | 1117 | 576 | 29 | 417 | 11 | 10 | 34 | 59 | 446 | 114 | 20.36 |
10 | Phenylalanine hydroxylase | 1.14.16.1 | 1314 | 1102 | 631 | 5 | 182 | 0 | 3 | 161 | 254 | 187 | 418 | 69.09 |
Note: EC: Enzyme commission number; CV: Number of clinical variants; SNP: Single nucleotide polymorphisms; M: Missense mutations; Co: Conflicting data; US: Uncertain significance; B: Benign; LB: Likely; P: Pathogenic; LP: Likely pathogenic; FN: False negative (Co + US); TP: True positive (B + LB + P + LP); R: Recall (TPTP+FN×100). |
It is clear from these data that amino acid substitutions (nature, type), either alone or in combination, comprise distinct transition-states and are significant contributors to the biochemical function of each enzyme dimer (Recall≈19–70%). Since each of these states will result in a distinct Kd, it is easily inferred that the transition-state dissociation constants, for a complex, may be more representative of biochemical function (T1–T4, C1–C3).
The discussion, vide supra, presents and highlights the biomedical relevance of modeling ligand-macromolecular interactions as transition-state dissociation constants. The results for monomer- and multimer-forms of ligand-macromolecular complexes are mathematically rigorous and have been validated, in silico. The results are now examined in context of biochemical function (enzyme, non-enzyme) for selected cases.
Case 1: Oxygen sensitive variants of non-haem iron (II)- and 2-oxoglutarate-dependent dioxygenases
The non-haem iron (Ⅱ)- and 2-oxoglutarate-dependent dioxygenases (EC1.14.x.y), comprise a large superfamily of enzymes, are present in all kingdoms of life and is chemically diverse (variable reaction chemistry, multiple substrates) [12,13,29]. Clinically relevant members include Phytanoyl-CoA dioxygenase (PHYT), Lysine Hydroxylases, and the Proline 4-Hydroxylases (P4H) amongst several others [12,13,14,15,29]. These enzymes have important roles in Phytanic acid metabolism and collagen maturation, with sub-optimal activities contributing to diseases such as Refsum and the Ehlers-Danlos (ED)-syndrome [14,15,30]. Here, too, the outcomes (clinical, non-clinical) associated with substitution mutations for PHYT suggest that modeling ligand-macromolecular interactions as transition-state dissociation constants may be a better index of biochemical function (T1–T4, C1–C3, P) [31].
P4Hs, are classified as being either hypoxia-sensitive (H−P4H≡HP4H;EC1.14.11.29) or collagen transforming (C−P4H≡CP4H;EC1.14.11.2) [28]. These reactions may be written,
HP4H−HIF+O2+2OG+Prolinerf→←rbHydroxyproline+SA+CO2RXN(2)CP4H+O2+2OG+Prolinerf→←rbHydroxyproline+SA+CO2RXN(3) |
rf,rb:=RateconstantsforforwardandbackwardreactionsatsteadystateHP4H:=Hypoxiainduciblefactor−dependentProline4−HydroxylaseHIF≡μhigh:=Hypoxia−induciblefactor(high−affinitymodifier)2OG:=2−oxoglutarateSA:=SuccinicacidCO2:=Carbondioxide |
The amino acid identity between HP4H and CP4H notwithstanding, there are significant differences between the molecular biology that they exhibit. This implies that despite the similarity of co-factor (iron(II)), substrate (L−Proline) and co-substrate (2−oxoglutarate), the binding affinities for molecular dioxygen vary considerably [7,32],
KmHP4H=0.1−0.76mM | (39) |
KmCP4H=0.03−1.5mM | (40) |
The turnover numbers for the cognate substrate, too, differ significantly [7],
KcatHP4H=0.015−0.733s−1 | (41) |
KcatCP4H=0.0188−0.02s−1 | (42) |
Clearly, a plausible explanation for these disparate empirical observations is the binding of the hypoxia-inducible factors (HIF) to P4H. The hypoxia-inducible factors (HIFs), are a family (n=3) of transcription factors which sense hypoxia and trigger the upregulation of hypoxia-dependent genes [32,33,34]. Here, although hypoxia-inducible factor, is a full length protein, the actual binding site is the C-terminal end of HP4H [7,35].
Kd≈0.000016−0.023mM | (43) |
Some of these observations may be inferred from the partitioning of the transition-state dissociation constants into distinct subsets (Table 2):
Case 1 | Case 2 | |
Ligand (μ∈L) | Hypoxia-inducible factor | Peptide |
Macromolecule (c≡Cz∈C) | HP4H, CP4H | M1β |
Primary complex ⟨c|μ⟩ High-affinity variant :=Kd(μhighCz) Low-affinity variant :=Kd(μhighCz) |
⟨HP4H|μ⟩, ⟨CP4H|μ⟩ Kd(HP4H|μhigh)=Kd(μhighHP4H) Kd(CP4H|μlow)=Kd(μlowCP4H) |
⟨M1β|μ⟩ Kd(M1β|μhigh)=Kd(μhighM1β) Kd(M1β|μlow)=Kd(μlowM1β) |
Higher-order complex ⟨c|μ⟩.Cy High-affinity variant :=Kd(μhighCz.Cy) Low-affinity variant :=Kd(μlowCz.Cy) |
--- --- --- |
⟨M1β|μ⟩.PLC Kd(M1β|μhigh.PLC)=Kd(μhighM1β.PLC) Kd(M1β|μlow.PLC)=Kd(μlowM1β.PLC) |
Functional relevance | RHP4H(t)≫RCP4H(t) | M1β|μhigh.PLC→αM1β (Anterograde) M1β|μlow.PLC→rM1β (Retrograde) |
Note: Czμ: All pairwise-atom/residue based square interaction matrix of ligand and macromolecule, ⟨c|μ⟩; KCzμ: Diagonal matrix of the interactions of a ligand and macromolecule; zi=j=z: Set of eigenvalues of KCzμ where z∈diag(KCzμ)⊂C; Kd(cμ): Set of eigenvalue-based transition-state dissociation constants for monomer- and multimer-forms of ligand-macromolecular complexes, {ω∈Kd(cμ)=αi=j=Re(z)∈Kd(Czμ)∩(0,1)}; μhigh,μlow: High- and low-affinity variants of an arbitrary ligand, μ={μhigh,μlow}∈L; μCz.Cy: Higher-order complex of ligand and macromolecule; Kinf: Subset of transition-state dissociation constants of ligand-macromolecular interaction; Ksup:Subset of transition-state dissociation constants of ligand-macromolecular interaction; R(t): Rate of reaction; HP4H: Hypoxia stimulated- and Collagen Proline 4-Hydroxylase; CP4H: M1β: Heterodimer of major histocompatibility complex I (MHC1) with beta-2 microglobulin; PLC: Peptide loading complex. |
In particular, binding of HIF restricts the range of the binding affinity of HP4H for molecular oxygen significantly,
ΔKmHP4HΔKmCP4H×100≈45% | (44) |
Here, the set of conformers of HP4H once bound to HIF ensures that the catalytic activity of HP4H for HIF is significantly reduced in the presence of hypoxia. This will extend the half-life of HIF and facilitate transcription of HIF-responsive genes [36,37]. In contrast, CP4H exhibits no such differential activity. Furthermore, there is a significant variation in the catalytic activity (turnover number) of these enzymes for their cognate substrate (L-Proline),
max(KcatHP4H)−min(KcatHP4H)max(KcatCP4H)−min(KcatCP4H)=ΔKcatHP4HΔKcatCP4H≈600 | (45) |
These data suggest that a ligand when bound to a macromolecule can affect the rate at which the resulting complex assembles or disassembles and thereby influence biochemical function. Hence, partitioning the transition-state dissociation constants of the ligand-macromolecular complex into distinct subsets may offer valuable insights into the in vivo function of enzymes in physiological and pathological states (T4, C2, C3).
Case 2: Generic model of MHC1-mediated high-affinity peptide export
The peptide loading complex (PLC) is a higher-order (Z>2;Tapasin,ERp57,MHC1) complex that assembles at the endoplasmic reticulum (ER)-membrane and functions to transport cytosolic peptides into the ER-lumen en route to the plasma membrane [38,39]. Whilst, regulation of this process, by Tapasin is well studied, the role of peptides and the possible mechanism(s) of action is unclear [40,41,42,43]. A low-affinity peptide-driven (LAPD)-model of the MHC1-mediated export of high-affinity peptides to the plasma membrane of nucleated cells has been proposed and investigated in silico [44]. A major proponent of this study was simulating the differential disassembly of PLC in response to peptides with varying affinities (high, low) for the MHC1-β2-microglobulin [44]. In fact, data from the simulations suggested that low-affinity peptides may not only actively participate in the transport of high-affinity peptide export, but could also regulate the same [44]. Another interesting observation discussed was the role of low-affinity peptides in priming the MHC1-export apparatus, such that irrespective of the nature of the cellular insult (acute, chronic), export of high-affinity peptides was rapid, continuous and efficient [44].
Utilizing the partition schema for the transition-state dissociation constants from the current analysis (Table 2), we can model and rewrite the differential disassembly of the PLC,
⟨M1β|μhigh⟩.PLCrf→←rbTapasin−ERp57+aM1βRXN(4)⟨M1β|μlow⟩.PLCrf→←rbTapasin−ERp57−μlow+rM1βRXN(5) |
rf,rb:=RateconstantsforforwardandbackwardreactionsatsteadystateM1β:=HeterodimerofMHC1withbeta−2microglobulinμlow:=Low−affinitypeptideμhigh:=High−affinitypeptide⟨M1β|μlow⟩:=SetofpeptideswithlowaffinityforMHC1andincomplexwithMHC1⟨M1β|μhigh⟩:=SetofpeptideswithhighaffinityforMHC1andincomplexwithMHC1eM1β:=Netexportablecomplexofhigh−affinitypeptidewithMHC1aM1β:=Anterograde−derivedexportablecomplexofhigh−affinitypeptidewithMHC1rM1β:=Retrograde−derivedexportablecomplexofhigh−affinitypeptidewithMHC1PLC:=PeptideloadingcomplexERp57:=Endoplasmicreticulumproteindisulfideisomerase |
The appropriate dissociation constants are,
![]() |
(46) |
![]() |
(47) |
Rewriting these equations in terms of the peptide-bound MHC1,
KdRXN4=ζ[M1β|μhigh]LM1β|μhigh≥0 | (48) |
where,
ζ=[Tapasin−ERp57][aM1β][PLC]LPLC≥0 | (48.1) |
KdRXN5=ζ[M1β|μlow]LM1β|μlow≥0 | (49) |
where,
ζ=[Tapasin−ERp57−μlow][rM1β][PLC]LPLC≥0 | (49.1) |
Clearly,
KdRXN4∝1[M1β|μhigh]LM1β|μhigh≥0 | (50) |
KdRXN5∝1[M1β|μlow]LM1β|μlow≥0 | (51) |
These results suggest that,
KdRXN4≃1.0(Perfectdisassociation)and[aM1β]→∞ | (52) |
KdRXN5≃0.0(Perfectassociation)and[rM1β]→0 | (53) |
and is in accordance with existing empirical and simulation data,
[μhigh]<<<[μlow],[aM1β]>>>[rM1β] | (54) |
Here, the partitioning of transition-state dissociation constants into low- and high-affinity peptides for the MHC1 can provide valuable insights into the underlying molecular biology of MHC1-mediated high-affinity peptide transport under physiological and pathological conditions (T5–T7, P) [42,43,44].
The work presented models ligand-macromolecular interactions as eigenvalue-based transition-state disassociation constants. The interaction matrix is an all-atom/residue pairwise comparison between the ligand and macromolecule and comprises numerical values drawn randomly from a standard uniform distribution. The transition-state dissociation constants are the strictly positive real part of all complex eigenvalues of this ligand-macromolecular interaction matrix, belong to the open interval (0, 1) and form a sequence whose terms are finite, monotonic, non-increasing and convergent. The findings are rigorous, numerically robust and can be extended to higher-order complexes. The study, additionally, suggests a schema by which a ligand may be partitioned into high- and low-affinity variants. This study, although theoretical offers a plausible explanation into the underlying biochemistry (enzyme-mediated substrate catalysis, assembly/disassembly and inhibitor kinetics) of ligand-macromolecular complexes. Future investigations may include assigning weights to each interaction, investigating origins of co-operativity in enzyme catalysis and inhibitory kinetics amongst others.
This section provides formal proofs for the included theorems, corollaries and proposition.
Proof (T1):
From Defs. (4) and (5),
For everyω∈Kd(cμ)∃g(ω)∈Kd(Czμ)|g−1∘g(ω)=ω | (55) |
Let,
ωx,ωy∈Kd(cμ)|g(ωx),g(ωy)∈Kd(Czμ);x≠y;{x,y}≤A |
If,
g(ωx)=g(ωy) | (56) |
then,
g−1∘g(ωx)=g−1∘g(ωy) | (57) |
⇒ωx=ωy | (58) |
If,
t=0|t∈Kd(Czμ) | (59) |
From (55),
g−1∘g(t)=0∉Kd(cμ) | (60) |
Similarly, For,
t≥1|t∈Kd(Czμ) | (61) |
From (55),
g−1∘g(ω)≥1∉Kd(cμ) | (62) |
From (58), (60) and (62),
Proof (T2): (By induction)
For a=1,
ωa=max(Kd(cμ))<1 (By Def. (5)), | (63) |
Assume a=A−1,
ωa≥ωA−1 | (64) |
Then ∃a=A,
ωa≥ωA−1≥ωA | (65) |
For a=1,
ωa=min(Kd(cμ))>0 (By Def. (5)), | (66) |
ωa≤ωA−1 | (67) |
⇒0<{ωa}a≤a+1∼Kd(cμ)<1∀ω |
Proof (T3):
From (T2),
{ωa}a≤a+1<1 |
Choose ε∈R+,ε→0,
εa>A<|ωa>A−1|<ε |
For every a>A,
|lima→∞ωa>A−1|<εlima→∞|ωa>A−1|<ε lima→∞ωa>A=1 | (69) |
Similarly,
{ωa}a≤a+1>0 |
Choose ε∈R+,ε→0,
1ε>|ωa>A−0|>ε |
For every a>A,
|lima→∞ωa>A−0|>εlima→∞|ωa>A−0|>εlima→∞ωa>A=0 | (70) |
From (69) and (70),
lima→∞{ωa}a≤a+1={0,1} |
Proof (C1):
Assume,
sup{ωa}a≤a+1=max{ωa}a≤a+1=ωa=1 |
Choose ε∈R+,ε→0
then for any a=1,2..A, we can find,
εA.ωa=1⋘ωa=1 | (71) |
εA.ωa=1≤ωa=A | (72) |
ωa=1≤ωa=A.(1εA) | (73) |
Let δ∈R+,δ→0,
ωa=1−δ<ωa=A.(1εA) | (74) |
Assume,
inf{ωa}a≤a+1=min{ωa}a≤a+1=ωa=A |
Choose ε∈R+,ε→0
then for any a=1,2..A, we can find,
εA.ωa=A<ωa=A | (75) |
ωa=A⋘ωa=A.(1εA) | (76) |
ωa=1≤ωa=1.(1εA) | (77) |
Let δ∈R+,δ→0,
ωa=1<ωa=1.(1εA)+δ | (78) |
From (74) and (78),
Proof (T4):
From (T2 and T3), (C1 and C2)
Case (1)
If
{ωa}a>A=Ksup⊂Kd(Czμ) |
then,
{ωa}a≤A∈Kinf∼Kd(cμ) | (79) |
Case (2)
If
{ωa}a>A=Kinf⊂Kd(Czμ) |
then,
{ωa}a≤A∈Ksup∼Kd(cμ) | (80) |
From (79) and (80),
Kinf∩Ksup={∅} | (81) |
Since,
Kd(Czμ)⊃(Kinf∪Ksup)∪(Kinf∩Ksup) |
From (79)–(81),
Kd(Czμ)⊃Kinf∪Ksup ⇒Kd(cμ)⊂Kd(Czμ) | (82) |
Proof (C3):
If,
∃μ∈L|#Ksup>>>#Kinf∀a |
Then,
μ≡μlow | (83) |
Similarly, if,
∃μ∈L|#Kinf>>>#Ksup∀a |
Then,
μ≡μhigh | (84) |
From (83) and (84),
Proof (T5) (By induction)
Assume z=1;Z≥2, y=1;Z=2,
C1.∏y=Y=Z−1y=1Cy=C1.∏y=1y=1Cy | (85) |
=C1.CY | (85.1) |
=C1.CY | (85.2) |
Assume truth for y=Y≫1,
C1.∏y=Y=Z−1y=1Cy=Cz.(C1….CY) | (86) |
=C1.CY | (86.1) |
For y=Y+1,
C1.∏y=Y+1y=1Cy=C1.∏y=Y+1y=1Cy | (87) |
=C1.(∏y=Yy=1Cy).(∏y=1y=1Cy) | (87.1) |
=C1.CYY.C11 | (87.2) |
=C1.CY+1 | (87.3) |
From (85)–(87),
Proof (T6):
From Defs. (6–8),
μCz.CY≡μCz≡⟨c|μ⟩ | (88) |
⇒Kd(μCz.CY)∼Kd(cμ) | (89) |
⇒Kd(μCz.CY)∩Kd(cμ) | (90) |
From Def. (5),
ωa∈[1,A]∈(Kd(μCz.CY)∩Kd(cμ)) | (91) |
(ωa∈[1,A]∈Kd(μCz.CY))∩(ωa∈[1,A]∈Kd(cμ)) | (92) |
From (T2–T4), (C1–C3),
∀a(ωa=1,2…A⊆Kd(μCz.CY))∩(ωa=1,2…A⊆Kd(cμ)) | (93) |
⇒({ωa}a=1,2…A∼Kd(μCz.CY))∩({ωa}a=1,2…A∼Kd(cμ)) | (94) |
Conversely, let,
u∈Kd(μCz.CY);ω∈Kd(cμ) |
From (92) and (94),
∀ω∈Kd(cμ)∃u∈Kd(μCz.CY)|u=h(ω) | (95) |
∀u∈Kd(μCz.CY)∃ω∈Kd(cμ)|ω=h−1(u)=h−1(h(ω)) | (96) |
From (95) and (96),
Proof (T7):
From (T6),
h−1∘h:ω∈Kd(cμ) |
From (T1), Def. (4)
g:ω∈Kd(cμ)↦Kd(cμ)∪R=Kd(Czμ) |
Rewriting,
g:ω↦Kd(Czμ) | (97) |
g(h−1(u))↦Kd(Czμ) | (98) |
g∘h−1(h(ωu))↦Kd(Czμ) | (99) |
g∘h−1∘h(ω)↦Kd(Czμ) | (100) |
Proof (P):
From (T1–T7) and Defs. (4–8, 10),
For z=Z=1,
μCz.CY=μCz | (101) |
g∘h−1(u∈Kd(μCz))↦Kd(Czμ)⊂R | (102) |
g∘h−1∘h(ω)↦Kd(Czμ) | (102.1) |
⇒Kd(μCz)⊂Kd(Czμ) | (103) |
For Z≥2,
g∘h−1(u∈Kd(μCz.CY))↦Kd(Czμ)⊂R | (104) |
g∘h−1∘h(ω)↦Kd(Czμ) | (104.1) |
⇒Kd(μCz.CY)⊂Kd(Czμ) | (105) |
From (103) and (105),
This work is funded by an early career intramural grant (Code No. A-766) awarded to SK by the All India Institute of Medical Sciences (AIIMS, New Delhi, INDIA).
The author declare there is no conflict of interest.
[1] |
W. Luo, Y. Li, R. Urtasun, R. Zemel, Understanding the effective receptive field in deep convolutional neural networks, Neural Information Processing Systems (NIPS 2017), 29 (2017). https://doi.org/10.48550/arXiv.1701.04128 doi: 10.48550/arXiv.1701.04128
![]() |
[2] | A. Dosovitskiy, L. Beyer, A. Kolesnikov, D. Weissenborn, X. H. Zhai, T. Unterthiner, et al., An image is worth 16x16 words: Transformers for image recognition at scale, (2020), preprint. https://doi.org/10.48550/arXiv.2010.11929 |
[3] | J. Devlin, M. W. Chang, K. Lee, K. Toutanova, Bert: Pre-training of deep bidirectional transformers for language understanding, (2018), preprint. https://doi.org/10.48550/arXiv.1810.04805 |
[4] | A. Radford, K. Narasimhan, T. Salimans, I. Sutskever, Improving language understanding by generative pre-training, (2018), work in progress. |
[5] |
X. X. Chu, Z. Tian, Y. Q. Wang, B. Zhang, H. B. Ren, X. L. Wei, et al., Twins: Revisiting the design of spatial attention in vision transformers, Neural Information Processing Systems (NIPS 2021), 34 (2021). https://doi.org/10.48550/arXiv.2104.13840 doi: 10.48550/arXiv.2104.13840
![]() |
[6] |
S. Cheng, I. C. Prentice, Y. Huang, Y. Jin, Y. K. Guo, R. Arcucci, Data-driven surrogate model with latent data assimilation: Application to wildfire forecasting, J. Comput. Phys., 464 (2022), 111302. https://doi.org/10.1016/j.jcp.2022.111302 doi: 10.1016/j.jcp.2022.111302
![]() |
[7] |
J. A. Weyn, D. R. Durran, R. Caruana, Improving data-driven global weather prediction using deep convolutional neural networks on a cubed sphere, J. Adv. Model. Earth Syst., 12 (2020). https://doi.org/10.1029/2020MS002109 doi: 10.1029/2020MS002109
![]() |
[8] | M. Chen, A. Radford, J. Wu, H. W. Jun, P. Dhariwal, D. Luan, et al., Generative pretraining from pixels, Proceed. Mach. Learn. Res., 199 (2020), 1691–1703. |
[9] |
H. Touvron, M. Cord, M. Douze, F. Massa, A. Sablayrolles, H. Jégou, Training data-efficient image transformers & distillation through attention, Proceed. Mach. Learn. Res., 139 (2021), 10347–10357. https://doi.org/10.48550/arXiv.2012.12877 doi: 10.48550/arXiv.2012.12877
![]() |
[10] |
K. Han, A. Xiao, E. Wu, J. Guo, C. Xu, Y. Wang, Transformer in transformer, Neural Information Processing Systems, 34 (2021), 15908–15919. https://doi.org/10.48550/arXiv.2103.00112 doi: 10.48550/arXiv.2103.00112
![]() |
[11] | N. Carion, F. Massa, G. Synnaeve, N. Usunier, A. Kirillov, S. Zagoruyko, End-to-end object detection with transformers, in European conference on computer vision (ECCV 2020), (2020), 213–229. https://doi.org/10.1007/978-3-030-58452-8_13 |
[12] | R. Strudel, R. Garcia, I. Laptev, C. Schmid, Segmenter: Transformer for semantic segmentation, in 2021 IEEE/CVF International Conference on Computer Vision (ICCV), (2021), 7242–7252. https://doi.org/10.48550/arXiv.2105.05633 |
[13] | S. He, H. Luo, P. Wang, F. Wang, H. Li, W. Jiang, TransReID: Transformer-based Object Re-Identification, in 2021 IEEE/CVF International Conference on Computer Vision (ICCV), (2021), 14993–15002. https://doi.org/10.1109/ICCV48922.2021.01474 |
[14] | Z. Liu, Y. Lin, Y. Cao, et al., Swin transformer: Hierarchical vision transformer using shifted windows, in 2021 IEEE/CVF International Conference on Computer Vision (ICCV), (2021), 9992–10002. https://doi.org/10.1109/ICCV48922.2021.00986 |
[15] | K. He, X. Zhang, S. Ren, J. Sun, Deep residual learning for image recognition, in 2016 IEEE Conference on Computer Vision and Pattern Recognition (CVPR), (2016), 770–778. https://doi.org/10.1109/CVPR.2016.90 |
[16] | W. Wang, E. Xie, X. Li, D. P. Fan, K. T. Song, D. Liang, et al., Pyramid vision transformer: A versatile backbone for dense prediction without convolutions, in 2021 IEEE/CVF International Conference on Computer Vision (ICCV), (2021), 548–558. https://doi.org/10.1109/ICCV48922.2021.00061 |
[17] | X. Chu, Z. Tian, B. Zhang, X. Wang, X. Wei, H. Xia, et al., Conditional positional encodings for vision transformers, (2021), preprint. https://doi.org/10.48550/arXiv.2102.10882 |
[18] |
A. Vaswani, N. Shazeer, N. Parmar, J. Uszkoreit, L. Jones, A. N. Gomez, et al., Attention is all you need, Neural Information Processing Systems (NIPS 2017), 30 (2017). https://doi.org/10.48550/arXiv.1706.03762 doi: 10.48550/arXiv.1706.03762
![]() |
[19] | L. Sifre, S. Mallat, Rigid-Motion Scattering for Image Classification, (2014), preprint. https://doi.org/10.48550/arXiv.1403.1687 |
[20] | J. Hu, L. Shen, G. Sun, Squeeze-and-excitation networks, in 2018 IEEE/CVF Conference on Computer Vision and Pattern Recognition, (2018), 7032–7141. https://doi.org/10.1109/CVPR.2018.00745 |
[21] | D. Misra, Mish: A self regularized non-monotonic activation function, (2019), preprint. https://doi.org/10.48550/arXiv.1908.08681 |
[22] | H. Luo, Y. Gu, X. Liao, S. Lai, W. Jiang, Bag of tricks and a strong baseline for deep person re-identification, in 2019 IEEE/CVF Conference on Computer Vision and Pattern Recognition Workshops (CVPRW), (2019), 1487–1495. https://doi.org/10.1109/CVPRW.2019.00190 |
[23] | C. Szegedy, V. Vanhoucke, S. Ioffe, J. Shlens, Z. Wojna, Rethinking the inception architecture for computer vision, in 2016 IEEE Conference on Computer Vision and Pattern Recognition (CVPR), (2016), 2818–2826. https://doi.org/10.1109/CVPR.2016.308 |
[24] | F. Schroff, D. Kalenichenko, J. Philbin, Facenet: A unified embedding for face recognition and clustering, in 2015 IEEE Conference on Computer Vision and Pattern Recognition (CVPR), (2015), 815–823. https://doi.org/10.1109/CVPR.2015.7298682 |
[25] | Y. Wen, K. Zhang, Z. Li, Y. Qiao, A Discriminative Feature Learning Approach for Deep Face Recognition, in European conference on computer vision (ECCV 2016), (2016). https://doi.org/10.1007/978-3-319-46478-7_31 |
[26] |
O. Russakovsky, J. Deng, H. Su, J. Krause, S. Satheesh, S. Ma, et al., ImageNet large scale visual recognition challenge, Int. J. Comput. Vision, 115 (2015), 211–252. https://doi.org/10.1007/s11263-015-0816-y doi: 10.1007/s11263-015-0816-y
![]() |
[27] | Z. Zhong, L. Zheng, G. Kang, S. Li, Y. Yang, Random erasing data augmentation, in Proceedings of the AAAI conference on artificial intelligence, 34 (2020). https://doi.org/10.48550/arXiv.1708.04896 |
[28] | Z. Zhong, L. Zheng, D. Cao, S. Li, Re-ranking person re-identification with k-reciprocal encoding, in 2017 IEEE Conference on Computer Vision and Pattern Recognition (CVPR), (2017), 3652–3661. https://doi.org/10.1109/CVPR.2017.389 |
[29] | K. Zhou, Y. Yang, A. Cavallaro, T. Xiang, Omni-scale feature learning for person re-identification, in 2019 IEEE/CVF Conference on Computer Vision and Pattern Recognition Workshops (CVPRW), (2019), 3701–3711. https://doi.org/10.1109/ICCV.2019.00380 |
[30] |
P. Wang, Z. Zhao, F. Su, X. Zu, N.V. Boulgouris, HOReID: Deep high-order mapping enhances pose alignment for person re-identification, IEEE Transact. Image Process., 30 (2021), 2908–2922. https://doi.org/10.1109/TIP.2021.3055952 doi: 10.1109/TIP.2021.3055952
![]() |
[31] | R. Quan, X. Dong, Y. Wu, L. Zhu, Y. Yang, Auto-ReID: Searching for a part-aware ConvNet for person re-identification, in 2019 IEEE/CVF International Conference on Computer Vision (ICCV), (2019), 3749–3758. https://doi.org/10.1109/ICCV.2019.00385 |
[32] |
H. Luo, W. Jiang, X. Zhang, X. Fan, J. Qian, C. Zhang, Alignedreid++: Dynamically matching local information for person re-identification, Pattern Recogn. J. Pattern Recogn. Soc., 94 (2019), 53–61. https://doi.org/10.1016/j.patcog.2019.05.028 doi: 10.1016/j.patcog.2019.05.028
![]() |
[33] | C.-P. Tay, S. Roy, K.-H. Yap, AANet: Attribute Attention Network for Person Re-Identifications, in 2019 IEEE/CVF Conference on Computer Vision and Pattern Recognition (CVPR), (2019), 7127–7136. https://doi.org/10.1109/CVPR.2019.00730 |
[34] | M. Zheng, S. Karanam, Z. Wu, R.J. Radke, Re-Identification With Consistent Attentive Siamese Networks, in 2019 IEEE/CVF Conference on Computer Vision and Pattern Recognition (CVPR), (2019), 5728–5737. https://doi.org/10.1109/CVPR.2019.00588 |
[35] | B. Chen, W. Deng, J. Hu, Mixed High-Order Attention Network for Person Re-Identification, in 2019 IEEE/CVF International Conference on Computer Vision (ICCV), (2019), 371–381. https://doi.org/10.48550/arXiv.1908.05819 |
[36] | M. M. Kalayeh, E. Basaran, M. Gökmen, M. E. Kamasak, M. Shah, Human semantic parsing for person re-identification, in 2018 IEEE/CVF Conference on Computer Vision and Pattern Recognition, (2018), 1062–1071. https://doi.org/10.1109/CVPR.2018.00117 |
[37] | M. S. Sarfraz, A. Schumann, A. Eberle, R. Stiefelhagen, A pose-sensitive embedding for person re-identification with expanded cross neighborhood re-ranking, in 2018 IEEE/CVF Conference on Computer Vision and Pattern Recognition, (2018), 420–429. https://doi.org/10.1109/CVPR.2018.00051 |
[38] | J. Wang, X. Zhu, S. Gong, W. Li, Transferable joint attribute-identity deep learning for unsupervised person re-identification, in 2018 IEEE/CVF Conference on Computer Vision and Pattern Recognition, (2018), 2275–2284. https://doi.org/10.1109/CVPR.2018.00242 |
[39] | J. Liu, Z.-J. Zha, D. Chen, R. Hong, M. Wang, Adaptive transfer network for cross-domain person re-identification, in 2019 IEEE/CVF Conference on Computer Vision and Pattern Recognition (CVPR), (2019), 7195–7204. https://doi.org/10.1109/CVPR.2019.00737 |
[40] | I. Goodfellow, J. Pouget-Abadie, M. Mirza, Conditional generative adversarial nets, in Neural Information Processing Systems, 27 (2014). https://doi.org/10.48550/arXiv.1411.1784 |
[41] | H. Park, B. Ha, Relation network for person re-identification, in Proceedings of the AAAI Conference on Artificial Intelligence, (2020). https://doi.org/10.48550/arXiv.1911.09318 |
[42] |
H. Tan, H. Xiao, X. Zhang, B. Dai, S. M. Lai, Y. Liu, et al., MSBA: Multiple scales, branches and attention network with bag of tricks for person re-identification, IEEE Access, 8 (2020), 63632–63642. https://doi.org/10.1109/ACCESS.2020.2984915 doi: 10.1109/ACCESS.2020.2984915
![]() |
[43] | G. Wang, Y. Yuan, X. Chen, J. Li, X. Zhou, Learning discriminative features with multiple granularities for person re-identification, in Proceedings of the 26th ACM international conference on Multimedia, (2018). https://doi.org/10.1145/3240508.3240552 |
1. | Siddhartha Kundu, ReDirection: an R-package to compute the probable dissociation constant for every reaction of a user-defined biochemical network, 2023, 10, 2296-889X, 10.3389/fmolb.2023.1206502 | |
2. | Siddhartha Kundu, A mathematically rigorous algorithm to define, compute and assess relevance of the probable dissociation constants in characterizing a biochemical network, 2024, 14, 2045-2322, 10.1038/s41598-024-53231-9 |
Enzyme | EC | CV | SNP | M | Co | US | B | LB | P | LP | FN | TP | R (%) | |
1 | Glucokinase | 2.7.1.2 | 778 | 619 | 370 | 49 | 157 | 4 | 4 | 65 | 113 | 206 | 186 | 47.45 |
2 | Pyruvate kinase | 2.7.1.40 | 1239 | 629 | 209 | 14 | 135 | 7 | 7 | 30 | 20 | 149 | 64 | 30.05 |
3 | Cathepsin A | 3.4.16.x | 1776 | 1270 | 501 | 20 | 382 | 26 | 23 | 26 | 34 | 402 | 109 | 21.33 |
4 | Pyruvate dehydrogenase | 1.2.4.1 | 2317 | 1591 | 584 | 25 | 391 | 35 | 46 | 62 | 43 | 416 | 186 | 30.9 |
5 | Phosphofructokinase 1 | 2.7.1.11 | 165 | 32 | 10 | 2 | 4 | 2 | 1 | 1 | 1 | 6 | 5 | 45.45 |
6 | Phosphofructokinase 2 | 2.7.1.105 | 206 | 76 | 23 | 1 | 17 | 1 | 1 | 2 | 1 | 18 | 5 | 21.74 |
7 | Cystathione beta-synthase | 4.2.1.22 | 930 | 744 | 255 | 21 | 172 | 3 | 4 | 46 | 32 | 193 | 85 | 30.58 |
8 | DNA topoisomerase Ⅱ | 5.6.2.2 | 466 | 319 | 152 | 0 | 122 | 11 | 14 | 3 | 1 | 122 | 29 | 19.21 |
9 | Guanylate cyclase 1 | 4.6.1.2 | 1572 | 1117 | 576 | 29 | 417 | 11 | 10 | 34 | 59 | 446 | 114 | 20.36 |
10 | Phenylalanine hydroxylase | 1.14.16.1 | 1314 | 1102 | 631 | 5 | 182 | 0 | 3 | 161 | 254 | 187 | 418 | 69.09 |
Note: EC: Enzyme commission number; CV: Number of clinical variants; SNP: Single nucleotide polymorphisms; M: Missense mutations; Co: Conflicting data; US: Uncertain significance; B: Benign; LB: Likely; P: Pathogenic; LP: Likely pathogenic; FN: False negative (Co + US); TP: True positive (B + LB + P + LP); R: Recall (TPTP+FN×100). |
Case 1 | Case 2 | |
Ligand (μ∈L) | Hypoxia-inducible factor | Peptide |
Macromolecule (c≡Cz∈C) | HP4H, CP4H | M1β |
Primary complex ⟨c|μ⟩ High-affinity variant :=Kd(μhighCz) Low-affinity variant :=Kd(μhighCz) |
⟨HP4H|μ⟩, ⟨CP4H|μ⟩ Kd(HP4H|μhigh)=Kd(μhighHP4H) Kd(CP4H|μlow)=Kd(μlowCP4H) |
⟨M1β|μ⟩ Kd(M1β|μhigh)=Kd(μhighM1β) Kd(M1β|μlow)=Kd(μlowM1β) |
Higher-order complex ⟨c|μ⟩.Cy High-affinity variant :=Kd(μhighCz.Cy) Low-affinity variant :=Kd(μlowCz.Cy) |
--- --- --- |
⟨M1β|μ⟩.PLC Kd(M1β|μhigh.PLC)=Kd(μhighM1β.PLC) Kd(M1β|μlow.PLC)=Kd(μlowM1β.PLC) |
Functional relevance | RHP4H(t)≫RCP4H(t) | M1β|μhigh.PLC→αM1β (Anterograde) M1β|μlow.PLC→rM1β (Retrograde) |
Note: Czμ: All pairwise-atom/residue based square interaction matrix of ligand and macromolecule, ⟨c|μ⟩; KCzμ: Diagonal matrix of the interactions of a ligand and macromolecule; zi=j=z: Set of eigenvalues of KCzμ where z∈diag(KCzμ)⊂C; Kd(cμ): Set of eigenvalue-based transition-state dissociation constants for monomer- and multimer-forms of ligand-macromolecular complexes, {ω∈Kd(cμ)=αi=j=Re(z)∈Kd(Czμ)∩(0,1)}; μhigh,μlow: High- and low-affinity variants of an arbitrary ligand, μ={μhigh,μlow}∈L; μCz.Cy: Higher-order complex of ligand and macromolecule; Kinf: Subset of transition-state dissociation constants of ligand-macromolecular interaction; Ksup:Subset of transition-state dissociation constants of ligand-macromolecular interaction; R(t): Rate of reaction; HP4H: Hypoxia stimulated- and Collagen Proline 4-Hydroxylase; CP4H: M1β: Heterodimer of major histocompatibility complex I (MHC1) with beta-2 microglobulin; PLC: Peptide loading complex. |
Enzyme | EC | CV | SNP | M | Co | US | B | LB | P | LP | FN | TP | R (%) | |
1 | Glucokinase | 2.7.1.2 | 778 | 619 | 370 | 49 | 157 | 4 | 4 | 65 | 113 | 206 | 186 | 47.45 |
2 | Pyruvate kinase | 2.7.1.40 | 1239 | 629 | 209 | 14 | 135 | 7 | 7 | 30 | 20 | 149 | 64 | 30.05 |
3 | Cathepsin A | 3.4.16.x | 1776 | 1270 | 501 | 20 | 382 | 26 | 23 | 26 | 34 | 402 | 109 | 21.33 |
4 | Pyruvate dehydrogenase | 1.2.4.1 | 2317 | 1591 | 584 | 25 | 391 | 35 | 46 | 62 | 43 | 416 | 186 | 30.9 |
5 | Phosphofructokinase 1 | 2.7.1.11 | 165 | 32 | 10 | 2 | 4 | 2 | 1 | 1 | 1 | 6 | 5 | 45.45 |
6 | Phosphofructokinase 2 | 2.7.1.105 | 206 | 76 | 23 | 1 | 17 | 1 | 1 | 2 | 1 | 18 | 5 | 21.74 |
7 | Cystathione beta-synthase | 4.2.1.22 | 930 | 744 | 255 | 21 | 172 | 3 | 4 | 46 | 32 | 193 | 85 | 30.58 |
8 | DNA topoisomerase Ⅱ | 5.6.2.2 | 466 | 319 | 152 | 0 | 122 | 11 | 14 | 3 | 1 | 122 | 29 | 19.21 |
9 | Guanylate cyclase 1 | 4.6.1.2 | 1572 | 1117 | 576 | 29 | 417 | 11 | 10 | 34 | 59 | 446 | 114 | 20.36 |
10 | Phenylalanine hydroxylase | 1.14.16.1 | 1314 | 1102 | 631 | 5 | 182 | 0 | 3 | 161 | 254 | 187 | 418 | 69.09 |
Note: EC: Enzyme commission number; CV: Number of clinical variants; SNP: Single nucleotide polymorphisms; M: Missense mutations; Co: Conflicting data; US: Uncertain significance; B: Benign; LB: Likely; P: Pathogenic; LP: Likely pathogenic; FN: False negative (Co + US); TP: True positive (B + LB + P + LP); R: Recall (TPTP+FN×100). |
Case 1 | Case 2 | |
Ligand (μ∈L) | Hypoxia-inducible factor | Peptide |
Macromolecule (c≡Cz∈C) | HP4H, CP4H | M1β |
Primary complex ⟨c|μ⟩ High-affinity variant :=Kd(μhighCz) Low-affinity variant :=Kd(μhighCz) |
⟨HP4H|μ⟩, ⟨CP4H|μ⟩ Kd(HP4H|μhigh)=Kd(μhighHP4H) Kd(CP4H|μlow)=Kd(μlowCP4H) |
⟨M1β|μ⟩ Kd(M1β|μhigh)=Kd(μhighM1β) Kd(M1β|μlow)=Kd(μlowM1β) |
Higher-order complex ⟨c|μ⟩.Cy High-affinity variant :=Kd(μhighCz.Cy) Low-affinity variant :=Kd(μlowCz.Cy) |
--- --- --- |
⟨M1β|μ⟩.PLC Kd(M1β|μhigh.PLC)=Kd(μhighM1β.PLC) Kd(M1β|μlow.PLC)=Kd(μlowM1β.PLC) |
Functional relevance | RHP4H(t)≫RCP4H(t) | M1β|μhigh.PLC→αM1β (Anterograde) M1β|μlow.PLC→rM1β (Retrograde) |
Note: Czμ: All pairwise-atom/residue based square interaction matrix of ligand and macromolecule, ⟨c|μ⟩; KCzμ: Diagonal matrix of the interactions of a ligand and macromolecule; zi=j=z: Set of eigenvalues of KCzμ where z∈diag(KCzμ)⊂C; Kd(cμ): Set of eigenvalue-based transition-state dissociation constants for monomer- and multimer-forms of ligand-macromolecular complexes, {ω∈Kd(cμ)=αi=j=Re(z)∈Kd(Czμ)∩(0,1)}; μhigh,μlow: High- and low-affinity variants of an arbitrary ligand, μ={μhigh,μlow}∈L; μCz.Cy: Higher-order complex of ligand and macromolecule; Kinf: Subset of transition-state dissociation constants of ligand-macromolecular interaction; Ksup:Subset of transition-state dissociation constants of ligand-macromolecular interaction; R(t): Rate of reaction; HP4H: Hypoxia stimulated- and Collagen Proline 4-Hydroxylase; CP4H: M1β: Heterodimer of major histocompatibility complex I (MHC1) with beta-2 microglobulin; PLC: Peptide loading complex. |