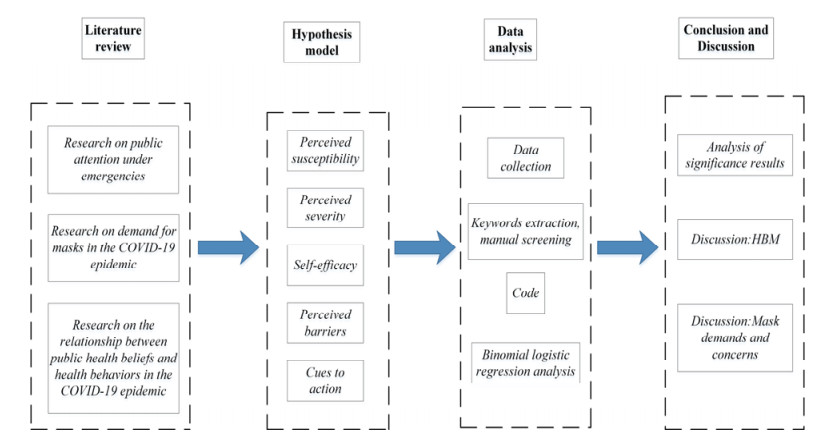
As we all know, vaccination still does not protect people from novel coronavirus infections, and wearing masks remains essential. Research on mask attention is helpful to understand the public's cognition and willingness to wear masks, but there are few studies on mask attention in the existing literature. The health belief model used to study disease prevention behaviors is rarely applied to the research on mask attention, and the research on health belief models basically entails the use of a questionnaire survey. This study was purposed to establish a health belief model affecting mask attention to explore the relationship between perceived susceptibility, perceived severity, self-efficacy, perceived impairment, action cues and mask attention. On the basis of the establishment of the hypothesis model, the Baidu index of epidemic and mask attention, the number of likes and comments on Weibo, and the historical weather temperature data were retrieved by using software. Keyword extraction and manual screening were carried out for Weibo comments, and then the independent variables and dependent variables were coded. Finally, through binomial logistic regression analysis, it was concluded that perceived susceptibility, perceived severity and action cues have significant influences on mask attention, and that the accuracy rate for predicting low attention is 93.4%, and the global accuracy is 84.3%. These conclusions can also help suppliers make production decisions.
Citation: Wei Hong, Xinhang Lu, Linhai Wu, Xujin Pu. Analysis of factors influencing public attention to masks during the COVID-19 epidemic—Data from Sina Weibo[J]. Mathematical Biosciences and Engineering, 2022, 19(7): 6469-6488. doi: 10.3934/mbe.2022304
[1] | Jun Liu, Xiang-Sheng Wang . Dynamic optimal allocation of medical resources: a case study of face masks during the first COVID-19 epidemic wave in the United States. Mathematical Biosciences and Engineering, 2023, 20(7): 12472-12485. doi: 10.3934/mbe.2023555 |
[2] | Fulian Yin, Jiahui Lv, Xiaojian Zhang, Xinyu Xia, Jianhong Wu . COVID-19 information propagation dynamics in the Chinese Sina-microblog. Mathematical Biosciences and Engineering, 2020, 17(3): 2676-2692. doi: 10.3934/mbe.2020146 |
[3] | Ugo Avila-Ponce de León, Angel G. C. Pérez, Eric Avila-Vales . Modeling the SARS-CoV-2 Omicron variant dynamics in the United States with booster dose vaccination and waning immunity. Mathematical Biosciences and Engineering, 2023, 20(6): 10909-10953. doi: 10.3934/mbe.2023484 |
[4] | Shayna Berman, Gavin D'Souza, Jenna Osborn, Matthew Myers . Comparison of homemade mask designs based on calculated infection risk, using actual COVID-19 infection scenarios. Mathematical Biosciences and Engineering, 2023, 20(8): 14811-14826. doi: 10.3934/mbe.2023663 |
[5] | Qinghua Liu, Siyu Yuan, Xinsheng Wang . A SEIARQ model combine with Logistic to predict COVID-19 within small-world networks. Mathematical Biosciences and Engineering, 2023, 20(2): 4006-4017. doi: 10.3934/mbe.2023187 |
[6] | Pannathon Kreabkhontho, Watchara Teparos, Thitiya Theparod . Potential for eliminating COVID-19 in Thailand through third-dose vaccination: A modeling approach. Mathematical Biosciences and Engineering, 2024, 21(8): 6807-6828. doi: 10.3934/mbe.2024298 |
[7] | Jin Guo, Aili Wang, Weike Zhou, Yinjiao Gong, Stacey R. Smith? . Discrete epidemic modelling of COVID-19 transmission in Shaanxi Province with media reporting and imported cases. Mathematical Biosciences and Engineering, 2022, 19(2): 1388-1410. doi: 10.3934/mbe.2022064 |
[8] | Xiaojing Wang, Yu Liang, Jiahui Li, Maoxing Liu . Modeling COVID-19 transmission dynamics incorporating media coverage and vaccination. Mathematical Biosciences and Engineering, 2023, 20(6): 10392-10403. doi: 10.3934/mbe.2023456 |
[9] | Gavin D'Souza, Jenna Osborn, Shayna Berman, Matthew Myers . Comparison of effectiveness of enhanced infection countermeasures in different scenarios, using a dynamic-spread-function model. Mathematical Biosciences and Engineering, 2022, 19(9): 9571-9589. doi: 10.3934/mbe.2022445 |
[10] | Adel Alatawi, Abba B. Gumel . Mathematical assessment of control strategies against the spread of MERS-CoV in humans and camels in Saudi Arabia. Mathematical Biosciences and Engineering, 2024, 21(7): 6425-6470. doi: 10.3934/mbe.2024281 |
As we all know, vaccination still does not protect people from novel coronavirus infections, and wearing masks remains essential. Research on mask attention is helpful to understand the public's cognition and willingness to wear masks, but there are few studies on mask attention in the existing literature. The health belief model used to study disease prevention behaviors is rarely applied to the research on mask attention, and the research on health belief models basically entails the use of a questionnaire survey. This study was purposed to establish a health belief model affecting mask attention to explore the relationship between perceived susceptibility, perceived severity, self-efficacy, perceived impairment, action cues and mask attention. On the basis of the establishment of the hypothesis model, the Baidu index of epidemic and mask attention, the number of likes and comments on Weibo, and the historical weather temperature data were retrieved by using software. Keyword extraction and manual screening were carried out for Weibo comments, and then the independent variables and dependent variables were coded. Finally, through binomial logistic regression analysis, it was concluded that perceived susceptibility, perceived severity and action cues have significant influences on mask attention, and that the accuracy rate for predicting low attention is 93.4%, and the global accuracy is 84.3%. These conclusions can also help suppliers make production decisions.
Ever since the COVID-19 outbreak began in Wuhan in December 2019, the novel coronavirus has shown its contagious characteristics, as it can be transmitted from person to person. Owing to the rapid spread of COVID-19, Wuhan closed down the city for a short period and built new hospitals: HuoShenshan and LeiShenshan. Other local governments also adopted a series of measures to reduce population movement. With the implementation of prevention and control measures in China, new cases have been reduced by more than 90% [1], but the COVID-19 epidemic continues to rapidly sweep across China and the world [2]. As of June 24, 2021, the total confirmed worldwide cases were 178, 837, 204, with 3, 880, 450 deaths [3]. At present, the global spread of COVID-19 remains unstoppable. COVID-19 has seriously affected our daily lives, affecting many fields such as health care, public transportation and education [4]; it has also caused incalculable economic losses.
In the context of COVID-19, diagnostic means are very important. Lella and Alphonse conducted an in-depth analysis of the methods of COVID-19 disease diagnosis based on human respiratory sounds such as coughs, the voice and breathing [5]; they proposed a pre-processing method for COVID-19 speech data sets with an enhanced mechanism. A one-dimensional convolutional network [6] and multi-channel deep convolutional neural network [7] were used to realize the automated diagnosis of COVID-19 disease. At the same time, they also modified the Mel-frequency cepstral coefficients and enhanced the lightweight convolutional neural network for COVID-19 diagnosis by using gamma-tone frequency cepstral coefficients [8]. But, prevention and control measures are equally important. Hand hygiene, social distancing and the use of personal protective equipment were considered to be the most important protective measures to control the spread of the virus [9]. However, long-term self-isolation and social distancing are associated with anxiety and severe stress [10]. Moreover, maintaining self-isolation for a long time means that a large number of industries cannot resume work and production, and that some people will lack income sources, leading to serious social and economic problems. Betsch et al. [11] found that disposable surgical masks have been widely used by the public as effective and inexpensive protective medical equipment. In addition, the novel coronavirus has an incubation period during which people will not show any suspicious symptoms even if they are infected. Masks worn by asymptomatic people can reduce the spread of the virus, and masks worn by uninfected people can also reduce the risk of their own infection. More importantly, in the worldwide outbreak and transmission, the longer the mask is used, the less effective it will be in protecting against the virus. So timely replacement of the mask is necessary. [12].
However, Eke and Eke [13] pointed out that the novel coronavirus was rapidly spreading to many countries, resulting in a serious shortage of personal protective equipment. Jiang et al. [14] also indicated that, since the outbreak of COVID-19, the extreme shortage of masks has exposed a major deficiency in the current production and operation model of health products. These findings suggest that the production and supply of masks did not increase in tandem with the rapid outbreak and development of COVID-19. At the time, even if people wanted to wear masks, not everyone had access to them because manufacturers were not producing enough masks, and stores were running low on stock. The scarcity of masks caused people to buy in panic [15]. When people have the opportunity to buy protective products such as masks, they are likely to buy too much, leading to the inability of other people to buy masks. In the late stages of the epidemic, the mask shortage resulted in the establishment of a large number of mask enterprises, but the increase in mask production was accompanied by steady improvement in the epidemic situation, which gradually reduced the demand for masks. These enterprises invested a lot of money to produce superfluous masks, but the lack of demand for them may yield adverse effects, such as mask accumulation and warehousing cost increases. These situations are not beneficial for the development of a mask supply chain. Therefore, understanding the trend of demand for mask is important for the mask industry.
The Holt-Winters model [16], autoregressive integrated moving average models [17], recurrent neural network models, long short-term memory models [18] and support vector machine models [19] are commonly used in research on demand prediction. However, for emergencies, the demand forecasting model may have poor forecasting results [20]. In addition, in the case of the COVID-19 epidemic, the people's demand for masks is more uncertain. Previous studies on trends and seasonal factors may not be sufficient to predict the demand for masks.
In this era of highly developed Internet, Weibo is a famous social media platform in China, where users can instantly share and communicate information. Baidu is a famous search engine in China, and an important platform for Internet users to search for information. When major public health emergencies occur in China, searching information about epidemics and masks by using Weibo and Baidu is the main way for people to stay informed on the situation. Moreover, the influence of epidemic information on the attention to masks is generated through the information in the network, and the public's attention to masks is easier to grasp on platforms such as Weibo and Baidu. On the basis of these reasons, this study mainly entailed the use of Weibo and Baidu data to study the influencing factors affecting public attention to masks.
In some previous studies, the attention to a product has been shown to have a positive effect on product demand [21,22]. People usually pay a certain amount of attention to a product before creating a demand for it. By paying attention to this type of product information, they choose the product that suits them according to their own preferences. In the case of the COVID-19 epidemic, people are also paying attention to protective equipment such as masks, and when people pay more attention to these products, it might relate to more demand for such products. Therefore, understanding people's attention to masks is very important for the mask industry. To some extent, the change in public attention to masks reflects the trend of people's demand for masks.
The model architecture of this paper is shown in Figure 1. First, a literature review was conducted for studies on public attention in emergencies, the demand for masks in the COVID-19 epidemic and the relationship between public health beliefs and health behaviors in the COVID-19 epidemic. Then, a hypothetical model of the relationship between perceived susceptibility, perceived severity, self-efficacy, perceived barriers, cues to action and mask attention was established. Next, we through the temperature, Weibo and Baidu data collection, Weibo comments keyword extraction and manual screening, for independent variables and dependent variables for coding processing, and regression analysis. Finally, the results of regression analysis are summarized and discussed.
With the development of information networks and the rapid rise of Weibo and Baidu, it is easy for influential emergencies to attract extensive public attention on the Internet [23]. The smog that broke out in China in 2013 had a huge impact on people's lives and garnered wide attention in a short time. Wei et al. [24] analyzed the relevant data collected on Sina Weibo and explored the determinants that influenced the public attention to the smog events.
In the case of the COVID-19 epidemic, there is a large amount of news about the epidemic on Weibo. Baidu is mostly used by Chinese people to search for COVID-19 information. The data from public searches not only reflect people's attention during the COVID-19 epidemic, but they also include the phased characteristics and evolutionary trend of the epidemic. Gong et al. [25] used a Baidu index to monitor public attention to information, conducted time-lag cross-correlation analysis with focus on time trends and spatial distribution and verified the effectiveness of the monitoring results. On this basis, Feng et al. [26] transformed time series data into a visibility network by utilizing the principle that a visibility algorithm can dig out more hidden information from time series data, which may help us fully understand the attention to the COVID-19 epidemic. In addition to studying the attention on the Baidu platform, some scholars [27,28] analyzed the comments on Weibo, in combination with performing data mining and text analysis, to realize the correlation between attention and COVID-19. Zhao et al. [29] found that social media can be used to measure public attention to public health emergencies, and that understanding the hot issues caused by COVID-19 can help is to develop more targeted prevention and control measures.
As we all know, the simplest and most specific way to suppress the transmission of the novel coronavirus and protect themselves, as well as the others, is to wear a mask in crowded places [30]. However, after China implemented the policy that all people had to wear masks, people's demand for masks increased sharply, and the national reserve of masks was not enough, resulting in a shortage of masks [31]. Some studies [32,33,34] found that, during the COVID-19 pandemic, the demand for N95 masks far exceeded their production and supply, although N95 masks provided the best protection and could be reused. Wang et al. [35] found that medical surgical masks and medical protective masks met the national technical standards and could effectively block pathogens, while there was no unified technical standard for other types of masks, which only had a certain mechanical blocking effect. In view of the shortage of masks, it is necessary to forecast the demand for masks and increase the production of N95 masks and surgical masks, so as to balance the supply and demand of masks as much as possible. Goel and Haruna [36] applied economic theory to analyze the impact of three policies, namely those requiring front-line staff to wear masks, those not requiring the entire public to wear masks and those forcing the entire public to wear masks, on the demand for medical surgical masks during the COVID-19 pandemic. In addition, aiming to address the shortage of masks, Shanghai has established an online distribution and sales system for masks throughout the city, providing real-time updates of mask booking and sales information for all designated pharmacies and neighborhood committees in Shanghai [37].
COVID-19 has had many psychological effects on people, and most of them cause varying degrees of anxiety [38,39]. Some people are anxious because they think that the novel coronavirus is very dangerous, and that they may be infected or even at risk of death, while others are anxious because of the enormous economic pressure caused by COVID-19. People with high anxiety may have more preventive health behaviors [40]. Health beliefs and behaviors are crucial to mental health in the COVID-19 epidemic, and the study of health belief models can help people understand the occurrence of mental health problems during the COVID-19 epidemic [41]. Since health belief models can effectively understand people's subjective perception of the COVID-19 epidemic, and they have been proven to be a good model to explain the impact of health belief on health prevention behavior [42], many scholars have studied public health behavior by using health belief models.
Bechard et al. [43] studied the relationship between age group and perceived COVID-19 susceptibility, severity, impact and health behavior efficacy and adoption; they verified the relationship between health beliefs and behaviors by using a structural equation model. There are also methods that were developed based on questionnaire analysis [44,45] to analyze the effects of the participants' perceived susceptibility, perceived severity, self-efficacy, perceived barriers and perceived benefits on health behaviors. Tan et al. [46] classified user comments on the COVID-19 epidemic posts posted on Facebook and analyzed the relationship between comments and the COVID-19 epidemic situation by using a health belief model.
For consumers, there is a certain relationship between masks purchase behavior and attention to masks. Existing literature [47,48] shows that major public health emergencies attract extensive public attention. The COVID-19 epidemic is very serious and has drawn widespread attention. The physical and mental health of the public has also been greatly affected, with most people feeling anxious and fearful. During this time, people took protective measures, such as wearing masks, social distancing and improving hygiene; additionally, the demand for masks increased sharply.
Most of the previous studies on masks in the COVID-19 pandemic focused on the protective capabilities of other types of masks when medical masks were in short supply. Therefore, using network data from Weibo, Baidu and other platforms, we developed a health belief model to study the factors influencing public attention to masks during the COVID-19 epidemic in order to understand the public attention to masks, and to help suppliers better predict the public's demand for masks and thus assist decision-making.
Previous literature studies on health belief models mainly explored the effects on health behaviors from the perspectives of perceived susceptibility, perceived severity, self-efficacy, perceived benefits, perceived obstacles and behavioral cues. However, considering the fact that wearing a mask can reduce the probability of novel coronavirus infection, we did not include the dimension of perceived benefits. We constructed a hypothetical model of five factors influencing mask attention, including perceived susceptibility, perceived severity, self-efficacy, perceived barriers and cues to action, as shown in Figure 2.
In this study, perceived susceptibility is defined as the public's subjective perception of their own likelihood of contracting the novel coronavirus. The perception of susceptibility may be related to the trend of the epidemic and the public's own mental and physical health. If people are more pessimistic about the development of the epidemic or have health problems, they feel they are at greater risk of infection. As the largest social network platform in China, Weibo records a large amount of COVID-19 epidemic information that can describe the epidemic situation, including public comments, thumbs up reactions and forwards on related posts [49]. People with high perceived susceptibility will pay more attention to the information about the epidemic, and they are more likely to resonate with the information posted by the main microblog, and comment on it, give it a thumbs up and forward it. When Jihyuk [50] studied particulate matter in the atmosphere, he found that perceived susceptibility was positively correlated with health behavioral intention from the perspective of health. Based on this, we developed the following hypothesis:
H1: During the COVID-19 epidemic, perceived susceptibility had a positive impact on attention to masks.
In this study, perceived severity is defined as the public's subjective perception of the severity of the consequences of their infection with the novel coronavirus. and the impact of the current COVID-19 epidemic. These perceptions of severity include the physical and psychological trauma of people infected with the novel coronavirus, as well as the pressure of unemployment and the economy in the context of economic recession during the pandemic [51]. In addition, after the resumption of work and production, there is also a requirement for people to wear masks at work. However, the shortage of masks has also caused problems for people who cannot buy masks. In these cases, the public is panicked by the possibility of death from the disease, and by the possibility of suffering from the disease. In this case, people will post comments on Weibo to express their emotions. Wang et al. [52] showed that perceived severity was significantly positively correlated with safety behaviors. Therefore, we believe that there is a significant relationship between the concern for masks and perceived severity during the epidemic. Based on this, we developed the following hypothesis:
H2: During the COVID-19 epidemic, perceived severity has a positive impact on the attention to masks.
Self-efficacy mainly refers to the subjective judgment of the public on their ability to carry out certain behaviors, and the possible results of such behaviors. In this study, self-efficacy is defined as the public's subjective perception of the necessity of epidemic prevention measures and the ability to take epidemic prevention measures to prevent infection and transmission on any occasion. These perceptions of self-efficacy include whether vaccination is necessary and effective in preventing infection in places where there are not many people or an epidemic. Marle et al. [53] found that self-efficacy was positively correlated with positive emotions and negatively correlated with negative emotions. On Weibo, many users actively urged others to take preventive measures to prevent infection and contagion. Duong et al. [54] showed that public attitude was positively correlated with behavioral intention. Based on this, the following hypothesis was developed:
H3: During the COVID-19 epidemic, self-efficacy has a positive impact on the attention to masks.
Perceived barriers mainly refers to people finding it difficult to carry out certain behaviors. In this paper, "perceived barriers" mainly refers to the perception that wearing a mask may cause some side effects. Previous studies [55] have shown that people's subjective feelings when wearing masks are mainly reflective of their thermal comfort and ease of breathing. When the protective effect is not considered, consumers will have different mask-wearing experiences at different temperatures. Wearing a mask when the temperature is too high may cause a hot microclimate inside the mask [56], resulting in thermal discomfort and dyspnea. When people feel heat discomfort, they may reduce their willingness to wear masks and gradually lose focus on them. Based on this, the following hypothesis was developed:
H4: During the COVID-19 epidemic, perceived barriers have a negative impact on mask attention.
In this paper, the cues to action mainly refer to the promotions to influence whether people take preventive measures; they include media publicity [57], reminders from medical staff and the experiences of others. In the case of the COVID-19 epidemic, these factors can also promote measures to prevent the novel coronavirus. News related to the epidemic released by the media will attract many people's attention, and they will usually search Baidu and other search engines for the information they are interested in, so as to update their understanding of the epidemic information. Kendal [58] considered the incentives behind engaging in preventive behaviors during disease outbreaks, and the role that news and mass media might play in influencing these behaviors. Ye et al. [59] also showed, through the use of a questionnaire survey, that cues to action in the epidemic were related to preventive behaviors. Based on this, the following hypothesis was developed:
H5: During the COVID-19 epidemic, cues to action have a positive impact on the attention to masks.
The COVID-19 epidemic is a major public health emergency that has brought challenges to China in many aspects. Since the outbreak of the COVID-19 epidemic, Sina Weibo has played a central role as a source of information and group gathering hub for public discussion. As the official Weibo account of the National Health Commission, Health China has also published a considerable amount of information on COVID-19 that is representative, authoritative and topical. Official updates are announced on the microblog daily, including the new death toll and the number of new diagnoses. Using the data crawling software Octopus, data from January 21, 2020 to January 31, 2021 were obtained, including the Weibo content, Weibo thumbs up number, the number of comments and forwards and Weibo comments. There were 376 main posts and 48, 454 valid comments because no relevant information was posted on one day. Among the data, the number of thumbs up represents the data related to perceived susceptibility, the number of comments containing negative public emotions represents the data related to perceived severity, and the number of comments containing epidemic prevention measures represents the data related to self-efficacy.
The basic research data applied in some studies [60,61] that have used network search data for prediction in China has come from the Baidu search platform. Therefore, we used the Baidu index on the epidemic as the source for the data of cues to action and the Baidu index on masks as the source for the data of attention to masks.
From January 21, 2020 to January 31, 2021, the four provinces with the highest attention to masks in the Baidu Index were Jiangsu, Guangdong, Zhejiang and Shandong. Therefore, the temperatures from January 21, 2020 to January 31, 2021 in Taizhou, Guangzhou, Jinhua and Zibo, which are the central cities of the four provinces, were obtained from the historical weather network (Lishi.tianqi.com) as the data representing perceived barriers.
For the hypothetical model constructed in this study, the independent variables are perceived susceptibility, perceived severity, perceived barriers, self-efficacy and cues to action. The dependent variable is attention to masks. The specific processing methods are as follows.
1) Because the number of thumbs up reactions to microblog posts is generally much larger than the number of comments and microblogs, the number of thumbs up reactions to microblog posts was used to represent perceptual susceptibility. The number of thumbs up reactions had an exponential distribution with a large order of magnitude, so we used logarithmic processing [62,63]. The minimum value was 1.079, and the maximum value was 5.841. After quantitative processing, when the number of thumbs up reactions ∈ (1, 2), the perceived susceptibility was very low, and its index was 1. When the number of thumbs up reactions ∈ (2, 3), the perceived susceptibility was low, and the index was 2. When the number of thumbs up reactions ∈ (3, 4), the perceived susceptibility was moderate and the index was 3. When the number of thumbs up reactions ∈ (4, 5), the perceived susceptibility was high and the index was 4. When the number of thumbs up reactions ∈ (5, 6), the perceived susceptibility was very high, and the index was 5.
2) The perceived severity was represented by the emotion of the comment. Regarding dealing with the comments posted on the National Health Commission's official Weibo page for daily information on COVID-19, we chose the method of keyword extraction to extract comments containing emotional keywords, as shown in Table 1. Since there were not many such comments among all of the comments, if the comments of the day contained such keywords, the public would have a high perception of severity, so the index was set to be 1. If the comments of the day do not contain such keywords, the public perception of severity is low, so the index was set to be 0.
Comment content | Keywords |
I'm afraid! | afraid |
Watching the numbers go up, I'm a little scared! | scared |
It's scary! | scary |
I am in a panic and praying for all of China! | panic |
3) Self-efficacy was represented by comments on epidemic prevention measures. Regarding dealing with the comments posted on the National Health Commission's official Weibo page for daily information on COVID-19, we also used keyword searches to extract the keywords related to epidemic prevention measures, as shown in Table 2. However, because only some of the comments containing quarantine measures could be found through keyword searching, the comments containing quarantine measures were also found by performing manual filtering after each keyword search. Among them, if the daily comments contained keywords related to epidemic prevention measures, the public's self-efficacy was high and the index was set to be 1; if the comments did not contain related keywords, the public's self-efficacy was low and the index was set to be 0.
Comment content | Keywords |
All Chinese should be forced to not go out unless they are sick! The only place to go is a hospital! All public places, except hospitals, will be closed. | not go out |
Please make sure you wear masks when you go out | wear masks |
The growth rate is too fast, try not to go out, wash your hands and wear masks, be responsible for yourself and society and do not run around and increase the rate of transmission | try not to go out, wash your hands and wear masks |
4) The concept of perceived barriers was represented by the comfort level of the public wearing masks. A previous study [64] has shown that, when the temperature was greater than 24 ℃, people wearing masks felt significantly more uncomfortable. Therefore, when the highest temperature in a place on any day was less than or equal to 24 ℃, the comfort level for that day was 1. When the lowest temperature of the day was greater than 24 ℃, the comfort level for the day was -1. When the minimum temperature of the day was less than or equal to 24 ℃ and the maximum temperature was greater than 24 ℃, the comfort level was 0. We collected the temperature data for four prefecture-level cities by using the historical weather network, obtained the daily comfort level for four cities and weighted them. Then, the total comfort was set as [-4, 4]. Therefore, for comfort level ∈ [-4, -2], the perceived barriers equaled 3. For comfort level ∈ [-1, 1], the perceived barriers equaled 2. For comfort level ∈ [2,4], the perceived barriers equaled 1.
5) The Baidu index for the epidemic was used as the cues to action. According to the statistics, the minimum value of the epidemic Baidu index was 24, 641, and the maximum value was 1, 204, 910. We found a large gap between the minimum value and maximum value of this data; the order of magnitude was also large. Therefore, the Baidu index was used for logarithmic processing, and three decimal places were retained. At this point, the minimum value was 4.392, and the maximum value was 6.081. Given that the action cues needed to be classified and quantified, we divided the values into five categories on average. Under the condition that the daily Baidu index data ∈ [4.392, 4.729], very few cues to action were found, making the index 1. Under the condition that the data ∈ [4.730, 5.067], few cues to action were found, making the index 2. Under the condition that the data ∈ [5.068, 5.405], the number of cues to action was moderate, making the index 3. Under the condition that the data ∈ [5.406, 5.743], many cues to action were found, making the index 4. Under the condition that the data ∈ [5.744, 6.081], very many cues to action were found, making the index 5.
6) The mask attention-related Baidu index for mask searches was selected. The minimum value of the Baidu index for masks was 1739, and the maximum value was 164, 319. Similarly, we first applied logarithmic processing to the data. After processing, we found that all other values were within the scale of [3,5], except for a very few values over 5. Therefore, under the condition that the level of attention to masks is divided into two categories, namely, high and low, according to the actual situation, 4.0 is appropriate as the dividing line for the level of attention when applying logarithms. So, when the value ∈ (3, 4), the attention to masks was low, and the index was 0. When the value ∈ (4, 5), the attention to masks was high, and the index was 1.
Given that Weibo and Baidu are the most commonly used social networking and search software for Chinese people, the Weibo comments and Baidu index obtained by crawling have practical significance. On the basis of the analysis of the attention to masks during the epidemic, and of the quantitative data of the influencing factors, we adopted a regression method to conduct a quantitative study on the influencing factors. Compared with linear regression, logistic regression is more suitable for the dependent variable of a classification model; in this study, due to the value of the dependent variable is 0 or 1 (mask attention with low or high), multiple logistic regression is not applicable for this situation, so we used binomial logistic regression to establish the following model:
Logitattention=ln(p1−p)=α0+α1Perceivedsusceptibility+α2Perceivedseverity+α3selfefficacy+α4Perceivedbarriers+α5Cuesaction | (1) |
The explained variable is attention. Perceived susceptibility, perceived severity, self-efficacy, perceived barriers and cues to action are the explanatory variables. The value of attention was {0, 1}, the value of perceived susceptibility was {1, 2, 3, 4, 5}, the value of perceived severity was {0, 1}, the value of self-efficacy was {0, 1}, the value of perceived barriers was {1, 2, 3} and the value of cues to action was {1, 2, 3, 4, 5}.
In this study, statistical analysis was used to better express the dependent and independent variables. Five different explanatory variables were selected; they are shown in Table 3. In a collinearity statistical test on the explanatory variables, only a Variance Inflation Factor (VIF) value above 5 and tolerance value less than 0.1 indicate the existence of a multi-collinearity problem in the data set [65]. Therefore, multi-collinearity problems related to the dependent and independent variables were not found in this study.
Variables | Collinearity Tolerance | Statistical VIF |
perceived susceptibility | 0.610 | 1.640 |
perceived severity | 0.567 | 1.764 |
self-efficacy | 0.465 | 2.149 |
perceived barriers | 0.213 | 4.699 |
cues to action | 0.211 | 4.743 |
Regarding the comprehensive test for the model coefficients in Table 4, the likelihood ratio test result showing whether all parameters in the logistic regression model were 0 was output on the model line. P < 0.05 means that the Odds Ratio (OR) value for at least one variable in the fitted model was statistically significant (i.e., the model is generally significant).
Chi-square | df.* | Sig.* | |
Step 1 Step | 204.420 | 5 | 0.000 |
Block | 204.420 | 5 | 0.000 |
Model | 204.420 | 5 | 0.000 |
*Note: Sig. represents significance and df. represents degrees of freedom. |
The Hosmer and Lemeshow test was used to evaluate the goodness of fit of the model. When the P-value was not less than the test level (P > 0.05), the information in the current data was considered to have been fully extracted. The results are shown in Table 5, indicating a high goodness of fit of the model.
Chi-square | df | Sig | -2 Log likelihood | Cox & Snell R Square | Nagelkerke R Square |
15.414 | 8 | 0.052 | 264.975 | 0.419 | 0.588 |
Table 6 shows that the significance values for the three independent variables (i.e., perceived susceptibility, perceived severity and cues to action) were all less than 0.1, indicating that they significantly affect the public's attention to masks with the confidence level of 0.1. The smaller the significance value, the stronger the significance. However, if self-efficacy and perceived barriers failed to pass the significance test, the null hypothesis was rejected, indicating that self-efficacy and perceived barriers had no significant effect on the attention to masks. Therefore, perceived susceptibility (0.000), perceived severity (0.078) and cues to action (0.001) positively affect the public's attention to masks.
Explanatory variables | B | S.E | Wald | df. | Sig. | Exp(B) | 95% C.I. for EXP(B) | |
Lower | Upper | |||||||
perceived susceptibility | 1.608 | 0.254 | 40.010 | 1 | 0.000 | 4.992 | 3.033 | 8.215 |
perceived severity | 1.131 | 0.643 | 3.096 | 1 | 0.078 | 3.099 | 0. 879 | 10.927 |
self-efficacy | 0.089 | 0.431 | 0.043 | 1 | 0.836 | 1.094 | 0.470 | 2.545 |
perceived barriers | 0.080 | 0.490 | 0.027 | 1 | 0.870 | 1.084 | 0.415 | 2.830 |
cues to action | 1.541 | 0.472 | 10.661 | 1 | 0.001 | 4.671 | 1.852 | 11.783 |
Constant | -6.588 | 1.211 | 29.593 | 1 | 0.000 | 0.001 |
The predictive performance of the obtained data was tested. The obfuscation matrix was applied and the results are shown in Table 7. The table shows that the global prediction accuracy of the binary logistic regression model reached 84.3%. The accuracy rate for those with low attention reached 93.4%.
Predicted deforested area | 0 | 1 | Corrected percentage |
Observed deforested area | |||
0 | 240 | 17 | 93.4 |
1 | 42 | 77 | 64.7 |
Overall corrected percentage | - | - | 84.3 |
In this work, we used the health belief model to understand the factors influencing the public's attention to masks. First, we established the relationship between perceived susceptibility, perceived severity, self-efficacy, perceived barriers, cues to action and mask attention by using a health belief model. Binary logistic regression analysis revealed that perceived susceptibility, perceived severity and cues to action had significant effects on mask attention, and that the accuracy of predicting low attention was 93.4%, and the global accuracy was 84.3%.
The results of this study show that perceived susceptibility has a positive effect on mask attention, consistent with our hypothesis. The higher the perceived susceptibility, the higher the likelihood that the public would think they will be infected with the COVID-19 virus. Given that wearing a mask can greatly reduce the risk of infection with the novel coronavirus, a population with a high perceived susceptibility is more inclined to wear a mask outdoors to prevent infection. When people's perceived susceptibility is high during an epidemic, there will be more demand for masks and a more urgent need for information about masks, thereby generating more attention to masks.
The results of this study show that perceived severity has a positive impact on mask attention, which is consistent with the hypothesis above. The higher the perceived severity of coronavirus, the more convinced is the public that novel coronavirus infection has a serious impact on their physical and mental health. For their own health, they are more eager to learn the online information on purchasing channels for masks and the correct information on wearing masks, which will increase the public's attention to masks.
The results of this study show that self-efficacy has no significant impact on mask attention, which is completely inconsistent with the hypothesis above. Self-efficacy mainly refers to the subjective judgment of the public on their ability to carry out certain behaviors, and the possible results of such behaviors. Although wearing masks by the public can reduce the risk of novel coronavirus infection, there is no risk of infection if they maintain social distancing and work from home. So, if conditions permit, some people with high self-efficacy are more likely to maintain social distancing or stay at home than wear a mask. They do not pay more attention to the mask information.
The results of this study show that perceived barriers have no significant effect on mask attention, which is completely inconsistent with our hypothesis. Although wearing a mask in a high-temperature environment can cause breathing difficulties and thermal discomfort, for those who have to go out, such as office workers, wearing masks is a necessary measure of protection. In addition, the high temperature outside does not mean that the office environment is also in a high-temperature state. The office environment of many enterprises is at an appropriate temperature that will not make employees feel very hot and stuffy with masks. However, people can endure commuting even if they are outdoors and the temperature is high for a short period. In addition, people who experience thermal discomfort from wearing masks in high temperatures may pay more attention to the situation of masks and search for ways to avoid feeling thermal discomfort from wearing masks.
The results of this study show that cues to action have a positive effect on mask attention, which is consistent with the hypothesis above. By searching for "epidemic situation" using Baidu, the public can obtain a great deal of information about the epidemic, including the real-time situation of the epidemic, the channel of infection, and the protection measures to take. When more people are following the epidemic situation and more searches are conducted on Baidu and other search platforms, more people will understand the necessity of epidemic prevention measures and wearing masks. In this case, they will pay more attention to messaging on masks.
As shown in Table 8, the results of our health belief model are different from those for non-communicable disease prevention behaviors [66]. In the case of the health belief model of non-communicable disease prevention behaviors, perceived benefits, perceived barriers, self-efficacy, cues to action, perceived benefits, perceived severity and perceived susceptibility all have direct or indirect effects. But, the most significant effects are perceived barriers and self-efficacy. Interestingly, perceived barriers and self-efficacy did not have a significant effect in this study. Among the non-communicable disease prevention behaviors, the most common unhealthy behaviors were sedentary living and working, mood disorders and staying up late. The novel coronavirus is quite different, as it is highly infectious, and an infection can have serious consequences, even death. So, in the case of the novel coronavirus, some people with high self-efficacy might choose to stay at home or practice social distancing to avoid the risk of infection. In terms of perceived barriers, wearing a mask can reduce the risk of infection and help to avoid serious consequences, even if people feel uncomfortable.
Health belief model in this study | Health belief model of non-communicable disease prevention behaviors | |||
Significant | Non-significant | Significant | Non-significant | |
perceived susceptibility, perceived severity, cues to action | self-efficacy, perceived barriers | perceived benefits, perceived barriers, self-efficacy, cues to action, perceived benefits, perceived severity, and perceived susceptibility |
COVID-19 has been going on for some time, and, during this time, countries have developed vaccines that have made a significant contribution to preventing novel coronavirus. The current situation shows that the vaccine, although effective, does not prevent novel coronavirus infection, so the long-term use of masks is still necessary. But, many people around the world have reduced their attention to and awareness of mask wearing, and novel coronavirus transmission continues. To eliminate this situation, we need to work together to improve public attention to masks and raise awareness of wearing masks.
The results of this study suggest that the public's perceived susceptibility, self-efficacy and cues to action remain important factors influencing consumers' attention to masks during the COVID-19 epidemic, while perceived severity and perceived barriers are not very important factors. Therefore, we put forward the following specific suggestions:
First, suppliers need to understand the public's perceived susceptibility when predicting the public's demand for masks. Changes in the public's perceived susceptibility affect people's attitudes toward wearing masks, which will affect the demand for masks. Especially, when the perceived susceptibility of the public is high, there may be a sharp increase in demand for masks.
Secondly, it is important to pay attention to perceived severity. People's perception of the more severe consequences of a novel coronavirus infection means that they are more likely to take measures to reduce their risk of infection. In this case, the demand for masks will also be higher, requiring suppliers to increase production capacity.
Finally, as Baidu is the most commonly used search platform in China, the more people search for epidemic information, the more likely they are to form an awareness of wearing masks, which also helps to increase the demand for masks. When epidemic information diminishes, suppliers need to be more vigilant to prevent inventory buildup, storage costs increases and oversupply.
First, in this study, only the health belief model was used to analyze the factors that might affect the attention to masks and the possible effects on the overall demand in the mask industry; we did not focus on predictions for masks with specific defensive properties. Therefore, the purchasing intention and behavior of consumers for different protective masks can be deeply analyzed based on relevant theories.
Second, this study only involved crawling the posts and online comments on the Weibo platform. The sample selection has certain limitations, so whether the conclusions of this study are suitable for online comments on other platforms also needs to be further studied. Subsequent research cannot only be confined to a single platform, but should involve the use of multiple domestic social platforms during the same period, as well as include conduct comparative analysis.
Finally, the use of keyword searches and manual processing in the context of big data may have affected the accuracy and precision of the results. Therefore, future research can use deep learning and other methods to study text data to improve the accuracy and efficiency of data analysis.
This work was supported by the Major Program of National Funds for Philosophy and Social Science of China (Grant No. 20 & ZD117).
The authors have no conflict or competing financial interest.
[1] |
A. Remuzzi, G. Remuzzi, COVID-19 and Italy: What next? Lancet, 395 (2020), 1225-1228. https://doi.org/10.1016/S0140-6736(20)30627-9 doi: 10.1016/S0140-6736(20)30627-9
![]() |
[2] | World Health Organization, WHO Director General's Statement on IHR Emergency Committee on Novel Coronavirus (2019-nCoV), 2021. Available from: https://www.sunupauto.com/114755.html. |
[3] | World Health Organization, Coronavirus Disease (COVID-19) Dashboard, 2021. Available from: https://covid19.who.int. |
[4] |
A. Barnawi, P. Chhikara, R. Tekchandani, N. Kumar, B. Alzahrani, Artificial intelligence-enabled Internet of Things-based system for COVID-19 screening using aerial thermal imaging, Future Gener. Comput. Syst., 124 (2021), 119-132. https://doi.org/10.1016/j.future.2021.05.019 doi: 10.1016/j.future.2021.05.019
![]() |
[5] |
K. K. Lella, P. J. A. Alphonse, A literature review on COVID-19 disease diagnosis from respiratory sound data, AIMS Bioeng., 8 (2021), 140-153. https://doi.org/10.3934/bioeng.2021013 doi: 10.3934/bioeng.2021013
![]() |
[6] |
K. K. Lella, P. J. A. Alphonse, Automatic COVID-19 disease diagnosis using 1D convolutional neural network and augmentation with human respiratory sound based on parameters: Cough, breath, and voice, AIMS Public Health, 8 (2021), 240-264. https://doi.org/10.3934/publichealth.2021019 doi: 10.3934/publichealth.2021019
![]() |
[7] |
K. K. Lella, P. J. A. Alphonse, Automatic diagnosis of COVID-19 disease using deep convolutional neural network with multi-feature channel from respiratory sound data: Cough, voice, and breath, Alexandria Eng. J., 61 (2021), 1110-0168, https://doi.org/10.1016/j.aej.2021.06.024 doi: 10.1016/j.aej.2021.06.024
![]() |
[8] |
K. K. Lella, P. J. A. Alphonse, COVID-19 disease diagnosis with light-weight CNN using modified MFCC and enhanced GFCC from human respiratory sounds, Eur. Phys. J. Spec. Top., (2022), 1-18. https://doi.org/10.1140/epjs/s11734-022-00432-w doi: 10.1140/epjs/s11734-022-00432-w
![]() |
[9] |
A. K. Thakur, R. Sathyamurthy, R. Velraj, I. Lynch, R. Saidur, A. K. Pandey, et al., Secondary transmission of SARS-Cov-2 through wastewater: Concerns and tactics for treatment to effectively control the pandemic, J. Environ. Manage., 290 (2021), 112668. https://doi.org/10.1016/j.jenvman.2021.112668 doi: 10.1016/j.jenvman.2021.112668
![]() |
[10] |
J. Paananen, J. Rannikko, M. Harju, J. Pirhonen, The impact of Covid-19-related distancing on the well-being of nursing home residents and their family members: A qualitative study, Int. J. Nurs. Stud. Adv., 3 (2021), 100031. https://doi.org/10.1016/j.ijnsa.2021.100031 doi: 10.1016/j.ijnsa.2021.100031
![]() |
[11] |
C. Betsch, L. Korn, L. Felgendreff, S. Eitze, H. Thaiss, School opening during the SARS-CoV-2 pandemic: Public acceptance of wearing fabric masks in class, Public Health Pract., 2 (2021), 100115. https://doi.org/10.1016/j.puhip.2021.100115 doi: 10.1016/j.puhip.2021.100115
![]() |
[12] |
M. Shen, Z. Zeng, B. Song, H. Yi, T. Hu, Y. Zhang, et al., Neglected microplastics pollution in global COVID-19: Disposable surgical masks, Sci. Total Environ., 790 (2021), 148130. https://doi.org/10.1016/j.scitotenv.2021.148130 doi: 10.1016/j.scitotenv.2021.148130
![]() |
[13] |
U. A. Eke, A. C. Eke, Personal protective equipment in the siege of respiratory viral pandemics: strides made and next steps, Expert Rev. Respir. Med., 15 (2020), 441-452. https://doi.org/10.1080/17476348.2021.1865812 doi: 10.1080/17476348.2021.1865812
![]() |
[14] | Y. Jiang, Y. Li, Y. Zhao, Mask dilemma and innovation for production and operation mode of public health products, Sci. Res. Manage., 41 (2020), 37-46. |
[15] |
G. Chua, K. Yuen, X. Wang, Y. Wong, The determinants of panic buying during COVID-19, Int. J. Environ. Res. Public Health , 18 (2021), 3247. https://doi.org/10.3390/ijerph18063247 doi: 10.3390/ijerph18063247
![]() |
[16] |
G. Tirkes, C. Guray, N. Celebi, Demand forecasting: A comparison between the Holt-Winters, trend analysis and decomposition models, Teh. Vjesn., 24 (2017), 503-509. https://doi.org/10.17559/TV-20160615204011 doi: 10.17559/TV-20160615204011
![]() |
[17] |
H. Yang, H. Jing, Forecasting of fresh agricultural products demand based on the ARIMA model, Guangdong Agric. Sci., 5 (2013), 855-858. https://doi.org/10.16768/j.issn.1004-874x.2013.11.001 doi: 10.16768/j.issn.1004-874x.2013.11.001
![]() |
[18] |
K. K. Chandriah, R. V. Naraganahalli, RNN/LSTM with modified Adam optimizer in deep learning approach for automobile spare parts demand forecasting, Multimedia Tools Appl., 80 (2021), 26145-26159. https://doi.org/10.1007/s11042-021-10913-0 doi: 10.1007/s11042-021-10913-0
![]() |
[19] | L. Si, Y. Wang, G. Xu, Logistics demand forecasting based on improved grey model, Comput. Simul., 29 (2012), 192-194,213. |
[20] |
X. Xu, Y. Qi, Z. Hua, Forecasting demand of commodities after natural disasters, Expert Syst. Appl., 37 (2010), 4313-4317. https://doi.org/10.1016/j.eswa.2009.11.069 doi: 10.1016/j.eswa.2009.11.069
![]() |
[21] |
E. Shin, J. E. Lee, What makes consumers purchase apparel products through social shopping services that social media fashion influencers have worn, J. Bus. Res., 132 (2021), 416-428. https://doi.org/10.1016/j.jbusres.2021.04.022 doi: 10.1016/j.jbusres.2021.04.022
![]() |
[22] |
A. S. Al-Adwan, G. Sammour, What makes consumers purchase mobile apps: Evidence from Jordan, J. Theor. Appl. Electron. Comm., 16 (2020), 562-583. https://doi.org/10.3390/jtaer16030034 doi: 10.3390/jtaer16030034
![]() |
[23] | C. Li, Y. Wang, H. Qu, Study on spatial and temporal characteristics of textile intangible cultural heritage and its communication strategy: An empirical analysis based on Baidu index, J. Silk, 58 (2021), 52-58. |
[24] |
J. Wei, W. Zhan, X. Guo, D. Marinova, Public attention to the great smog event: A case study of the 2013 smog event in Harbin, China, Nat. Hazards, 89 (2017), 923-938. https://doi.org/10.1007/s11069-017-3000-6 doi: 10.1007/s11069-017-3000-6
![]() |
[25] |
X. Gong, Y. Han, M. Hou, R. Guo, Online public attention during the early days of the COVID-19 pandemic: Infoveillance study based on Baidu index, JMIR Public Health Surveill., 6 (2020), 225-237. https://doi.org/10.2196/23098 doi: 10.2196/23098
![]() |
[26] |
Q. Feng, H. Wei, J. Hu, F. Li, P. Lv, P. Wu, Analysis of the attention to COVID-19 epidemic based on visibility graph network, Mod. Phys. Lett. B, 35 (2021), 2150316. https://doi.org/10.1142/S0217984921503164 doi: 10.1142/S0217984921503164
![]() |
[27] | C. Zhang, X. Ma, Y. Zhou, R. Guo, Analysis of public opinion evolution in COVID-19 pandemic from a perspective of sentiment variation, J. Geo-Inf. Sci., 23 (2021), 341-350. |
[28] |
K. Hou, T. Hou, L. Cai, Public attention about COVID-19 on social media: An investigation based on data mining and text analysis, Pers. Individ. Differ., 175 (2021), 110701. https://doi.org/10.1016/j.paid.2021.110701 doi: 10.1016/j.paid.2021.110701
![]() |
[29] | Y. Zhao, S. Cheng, X. Yu, H. L. Xu, Chinese public's attention to the COVID-19 epidemic on social media: Observational descriptive study, J. Med. Internet Res., 22 (2020), e18825. http://preprints.jmir.org/preprint/18825 |
[30] |
T. L. Phan, C. T. S. Ching, A reusable mask for coronavirus disease 2019 (COVID-19), Arch. Med. Res., 51 (2020), 455-457. https://doi.org/10.1016/j.arcmed.2020.04.001 doi: 10.1016/j.arcmed.2020.04.001
![]() |
[31] |
H. Wu, J. Huang, C. Zhang, Z. L. He, W. K. Ming, Facemask shortage and the novel coronavirus disease (COVID-19) outbreak: Reflections on public health measures, E Clin. Med., 21 (2020), 100329. https://doi.org/10.1016/j.eclinm.2020.100329 doi: 10.1016/j.eclinm.2020.100329
![]() |
[32] |
C. E. Rodriguez-Martinez, M. P. Sossa-Briceo, J. A. Cortes, Decontamination and reuse of N95 filtering facemask respirators: A systematic review of the literature, Am. J. Infect. Control, 48 (2020), 1520-1532. https://doi.org/10.1016/j.ajic.2020.07.004 doi: 10.1016/j.ajic.2020.07.004
![]() |
[33] |
Y. Tao, F. You, Can decontamination and reuse of N95 respirators during COVID-19 pandemic provide energy, environmental, and economic benefits? Appl. Energy, 304 (2021), 117848. https://doi.org/10.1016/j.apenergy.2021.117848 doi: 10.1016/j.apenergy.2021.117848
![]() |
[34] |
E. Deniz, S. Sibar, O. Kilic, S. Ayhan, Comparison of effectiveness of four facial masks used during the COVID-19 pandemic using indocyanine green and fluorescent angiography device: A plastic surgeon perspective, Turk. J. Plast. Surg., 29 (2021), 166-171. https://doi.org/10.4103/tjps.tjps_105_20 doi: 10.4103/tjps.tjps_105_20
![]() |
[35] | L. Wang, X. Zhao, J. Zhang, W. Ma, H. Zhao, Rational selection and application of medical masks, Chin. J. Nosocomiol., 21 (2011), 3908-3909. |
[36] |
R. K. Goel, S. Haruna, Unmasking the demand for masks: Analytics of mandating coronavirus masks, Metroeconomica, 72 (2021), 580-591. https://doi.org/10.1111/meca.12334 doi: 10.1111/meca.12334
![]() |
[37] |
T. Zhang, Q. Wang, W. Shi, T. Sheng, J. X. Liu, J. J. Zhao, et al., Achieving universal wearing of face masks during the COVID-19 pandemic: A practical solution from Shanghai, China, Risk Manage. Healthcare Policy, 13 (2020), 3067-3077. https://doi.org/10.2147/RMHP.S280672 doi: 10.2147/RMHP.S280672
![]() |
[38] | F. J. Siyal, Z. A. Shaikh, S. Z. Ahmed, M. A. Shahid, F. Agha, M. Khoso, et al., Anxiety among COVID-19 physicians during the pandemic in the health care center of the rural region, Arch. Pharm. Pract., 11 (2020), 91-93. |
[39] |
E. M. Kleiman, A. L. Yeager, J. L. Grove, J. K. Kellerman, J. S. Kim, Real-time mental health impact of the COVID-19 pandemic on college students: Ecological momentary assessment study, JMIR Mental Health, 7 (2020), e24815. https://doi.org/10.2196/24815 doi: 10.2196/24815
![]() |
[40] |
Y. P. Hsieh, C. Yen, C. Wu, P. Wang, Nonattendance at scheduled appointments in outpatient clinics due to COVID-19 and related factors in Taiwan: A health belief model approach, Int. J. Environ. Res. Public Health, 18 (2021), 4445. https://doi.org/10.3390/ijerph18094445 doi: 10.3390/ijerph18094445
![]() |
[41] |
C. Zhou, X. Yue, X. Zhang, F. Shangguan, X. Zhang, Self-efficacy and mental health problems during COVID-19 pandemic: A multiple mediation model based on health belief model, Pers. Individ. Differ., 179 (2021), 110893. https://doi.org/10.1016/j.paid.2021.110893 doi: 10.1016/j.paid.2021.110893
![]() |
[42] |
M. Aval, A. Ansari-Moghadam, G. Masoudy, Educational intervention based on health belief model on the adoption of preventive behaviors of Crimean-Congo hemorrhagic fever in ranchers, Health Scope, 8 (2019), e14112. https://doi.org/10.5812/jhealthscope.14112 doi: 10.5812/jhealthscope.14112
![]() |
[43] |
L. E. Bechard, M. Bergelt, B. Neudorf, T. C. DeSouza, L. E. Middleton, Using the health belief model to understand age differences in perceptions and responses to the COVID-19 pandemic, Front. Psychol., 12 (2021), 609893. https://doi.org/10.3389/fpsyg.2021.609893 doi: 10.3389/fpsyg.2021.609893
![]() |
[44] |
A. B. Coe, M. H. Elliott, S. B. S. Gatewood, J. R. Goode, L. R. Moczygemba, Perceptions and predictors of intention to receive the COVID-19 vaccine, Res. Soc. Adm. Pharm., 18 (2021), 2593-2599. https://doi.org/10.1016/j.sapharm.2021.04.023 doi: 10.1016/j.sapharm.2021.04.023
![]() |
[45] |
A. R. Mercadante, A. V. Law, Will they, or won't they? Examining patients' vaccine intention for flu and COVID-19 using the health belief model, Res. Soc. Adm. Pharm., 17 (2020), 1596-1605. https://doi.org/10.1016/j.sapharm.2020.12.012 doi: 10.1016/j.sapharm.2020.12.012
![]() |
[46] |
S. G. Tan, A. S. Raamkumar, H. L. Wee, Users' beliefs toward physical distancing in Facebook pages of public health authorities during COVID-19 pandemic in early 2020, Health Educ. Behav., 48 (2021), 404-411. https://doi.org/10.1177/10901981211014428 doi: 10.1177/10901981211014428
![]() |
[47] | N. Li, Z. Tang, Cognition and demand for health education of rural residents for public health emergencies and common infectious diseases, J. Catastrophology, 35 (2020), 33-37. |
[48] | J. Cao, X. Yang, S. Wang, Key scientific problems in public emergency management, J. Public Manage., 4 (2007), 84-93. |
[49] |
J. Dono, K. Ettridge, M. Wakefield, S. Pettigrew, J. Coveney, D. Roder, et al., Intentions to reduce sugar-sweetened beverage consumption: The importance of perceived susceptibility to health risks, Public Health Nutr., 24 (2021), 5663-5672. https://doi.org/10.1017/S1368980021000239 doi: 10.1017/S1368980021000239
![]() |
[50] |
J. Joo, Exploration of structural relations on health behavior related to particulate matter: Focused on multi-dimensional health locus of control, perceived susceptibility and severity, and health behavioral intention, J. Korea Convergence Soc., 8 (2017), 413-421. https://doi.org/10.15207/JKCS.2017.8.11.413 doi: 10.15207/JKCS.2017.8.11.413
![]() |
[51] |
A. Almehmadi, COVID-19 pandemic data predict the stock market, Comput. Syst. Sci. Eng., 36 (2021), 451-460. https://doi.org/10.32604/csse.2021.015309 doi: 10.32604/csse.2021.015309
![]() |
[52] |
M. Wang, L. Huang, X. Liang, B. Li, Consumer knowledge, risk perception and food-handling behaviors—A national survey in China, Food Control, 122 (2021), 107789. https://doi.org/10.1016/j.foodcont.2020.107789 doi: 10.1016/j.foodcont.2020.107789
![]() |
[53] |
J. L. Marle, F. Parmentier, F. Vinchon, M. Torme, X. Borteyrou, T. Lubart, Evolution and impact of self-efficacy during French COVID-19 confinement: A longitudinal study, J. Gen. Physiol., 148 (2021), 360-381. https://doi.org/10.1080/00221309.2021.1904815 doi: 10.1080/00221309.2021.1904815
![]() |
[54] |
H. T. Duong, H. T. Nguyen, S. J. Mcfarlane, L. T. V. Nguyen, Risk perception and COVID-19 preventive behaviors: Application of the integrative model of behavioral prediction, Soc. Sci. J., (2021), 1-14. https://doi.org/10.1080/03623319.2021.1874176 doi: 10.1080/03623319.2021.1874176
![]() |
[55] |
M. Morishima, K. Kishida, M. Fukagawa, Investigating facemask problems associated with wearing comfort and fit, Int. J. Clothing Sci. Technol., (2021). https://doi.org/10.1108/IJCST-05-2020-0067 doi: 10.1108/IJCST-05-2020-0067
![]() |
[56] |
Y. Lin, C. Chen, Thermoregulation and thermal sensation in response to wearing tight-fitting respirators and exercising in hot-and-humid indoor environment, Build. Environ., 160 (2019), 106158. https://doi.org/10.1016/j.buildenv.2019.05.036 doi: 10.1016/j.buildenv.2019.05.036
![]() |
[57] |
A. A. Sadore, D. W. Handiso, T. E. Wontamo, D. E. Mekango, S. Moges, Influence of social media use on practice of Covid-19 preventive measures among Ethiopian residents: An online cross-sectional study, Disaster Med. Public, (2021), 1-6. https://doi.org/10.1017/dmp.2021.184 doi: 10.1017/dmp.2021.184
![]() |
[58] | E. Kendal, Public health crises in popular media: How viral outbreak films affect the public's health literacy, Med. Humanit., 47 (2019), 11-19. https://10.1136/medhum-2018-011446 |
[59] |
Y. Ye, R. Wang, D. Feng, R. J. Wu, Z. F. Li, C. X. Long, et al., The recommended and excessive preventive behaviors during the COVID-19 pandemic: A community-based online survey in China, Int. J. Environ. Res. Public Health, 17 (2020), 6953. https://doi.org/10.3390/ijerph17196953 doi: 10.3390/ijerph17196953
![]() |
[60] |
Z. Liu, T. Huynh, P. Dai, The impact of COVID-19 on the stock market crash risk in China, Res. Int. Bus. Finance, 57 (2021), 101419. https://doi.org/10.1016/j.ribaf.2021.101419 doi: 10.1016/j.ribaf.2021.101419
![]() |
[61] |
X. Zhu, C. Xia, Visual network analysis of the Baidu-index data on greenhouse gas, Int. J. Mod. Phys. B, 35 (2021), 2150115. https://doi.org/10.1142/S0217979221501150 doi: 10.1142/S0217979221501150
![]() |
[62] |
Y. Yuan, X. Wang, Exploring the effectiveness of location-based social media in modeling user activity space: A case study of Weibo, Trans. GIS., 22 (2018), 930-957. https://doi.org/10.1111/tgis.12450 doi: 10.1111/tgis.12450
![]() |
[63] |
T. Kawada, Indices of insulin sensitivity and resistance: Adequate logarithmic transformation is needed to keep mathematical equivalence, Early Hum. Dev., 89 (2013), 515. https://doi.org/10.1016/j.earlhumdev.2013.03.010 doi: 10.1016/j.earlhumdev.2013.03.010
![]() |
[64] |
R. Zhang, J. Liu, L. Zhang, J. D. Lin, Q. Q. Wu, The distorted power of medical surgical masks for changing the human thermal psychology of indoor personnel in summer, Indoor Air, 31 (2021), 1645-1656. https://doi.org/10.1111/ina.12830 doi: 10.1111/ina.12830
![]() |
[65] |
R. Johnston, K. Jones, D. Manley, Confounding and collinearity in regression analysis: A cautionary tale and an alternative procedure, illustrated by studies of British voting behaviour, Qual. Quant., 52 (2018), 1957-1976. https://doi.org/10.1007/s11135-017-0584-6 doi: 10.1007/s11135-017-0584-6
![]() |
[66] |
T. J. Wang, H. J. Wang, Y. J. Zeng, X. Q. Cai, L. D. Xie, Health beliefs associated with preventive behaviors against non-communicable diseases, Patient Educ. Couns., 105 (2022), 173-181. https://doi.org/10.1016/j.pec.2021.05.024 doi: 10.1016/j.pec.2021.05.024
![]() |
1. | Xiao Ren, Jie Hua, Xin Chi, Yao Tan, Visual analysis of social events and stock market volatility in China and the USA during the pandemic, 2022, 20, 1551-0018, 1229, 10.3934/mbe.2023056 |
Comment content | Keywords |
I'm afraid! | afraid |
Watching the numbers go up, I'm a little scared! | scared |
It's scary! | scary |
I am in a panic and praying for all of China! | panic |
Comment content | Keywords |
All Chinese should be forced to not go out unless they are sick! The only place to go is a hospital! All public places, except hospitals, will be closed. | not go out |
Please make sure you wear masks when you go out | wear masks |
The growth rate is too fast, try not to go out, wash your hands and wear masks, be responsible for yourself and society and do not run around and increase the rate of transmission | try not to go out, wash your hands and wear masks |
Variables | Collinearity Tolerance | Statistical VIF |
perceived susceptibility | 0.610 | 1.640 |
perceived severity | 0.567 | 1.764 |
self-efficacy | 0.465 | 2.149 |
perceived barriers | 0.213 | 4.699 |
cues to action | 0.211 | 4.743 |
Chi-square | df.* | Sig.* | |
Step 1 Step | 204.420 | 5 | 0.000 |
Block | 204.420 | 5 | 0.000 |
Model | 204.420 | 5 | 0.000 |
*Note: Sig. represents significance and df. represents degrees of freedom. |
Chi-square | df | Sig | -2 Log likelihood | Cox & Snell R Square | Nagelkerke R Square |
15.414 | 8 | 0.052 | 264.975 | 0.419 | 0.588 |
Explanatory variables | B | S.E | Wald | df. | Sig. | Exp(B) | 95% C.I. for EXP(B) | |
Lower | Upper | |||||||
perceived susceptibility | 1.608 | 0.254 | 40.010 | 1 | 0.000 | 4.992 | 3.033 | 8.215 |
perceived severity | 1.131 | 0.643 | 3.096 | 1 | 0.078 | 3.099 | 0. 879 | 10.927 |
self-efficacy | 0.089 | 0.431 | 0.043 | 1 | 0.836 | 1.094 | 0.470 | 2.545 |
perceived barriers | 0.080 | 0.490 | 0.027 | 1 | 0.870 | 1.084 | 0.415 | 2.830 |
cues to action | 1.541 | 0.472 | 10.661 | 1 | 0.001 | 4.671 | 1.852 | 11.783 |
Constant | -6.588 | 1.211 | 29.593 | 1 | 0.000 | 0.001 |
Predicted deforested area | 0 | 1 | Corrected percentage |
Observed deforested area | |||
0 | 240 | 17 | 93.4 |
1 | 42 | 77 | 64.7 |
Overall corrected percentage | - | - | 84.3 |
Health belief model in this study | Health belief model of non-communicable disease prevention behaviors | |||
Significant | Non-significant | Significant | Non-significant | |
perceived susceptibility, perceived severity, cues to action | self-efficacy, perceived barriers | perceived benefits, perceived barriers, self-efficacy, cues to action, perceived benefits, perceived severity, and perceived susceptibility |
Comment content | Keywords |
I'm afraid! | afraid |
Watching the numbers go up, I'm a little scared! | scared |
It's scary! | scary |
I am in a panic and praying for all of China! | panic |
Comment content | Keywords |
All Chinese should be forced to not go out unless they are sick! The only place to go is a hospital! All public places, except hospitals, will be closed. | not go out |
Please make sure you wear masks when you go out | wear masks |
The growth rate is too fast, try not to go out, wash your hands and wear masks, be responsible for yourself and society and do not run around and increase the rate of transmission | try not to go out, wash your hands and wear masks |
Variables | Collinearity Tolerance | Statistical VIF |
perceived susceptibility | 0.610 | 1.640 |
perceived severity | 0.567 | 1.764 |
self-efficacy | 0.465 | 2.149 |
perceived barriers | 0.213 | 4.699 |
cues to action | 0.211 | 4.743 |
Chi-square | df.* | Sig.* | |
Step 1 Step | 204.420 | 5 | 0.000 |
Block | 204.420 | 5 | 0.000 |
Model | 204.420 | 5 | 0.000 |
*Note: Sig. represents significance and df. represents degrees of freedom. |
Chi-square | df | Sig | -2 Log likelihood | Cox & Snell R Square | Nagelkerke R Square |
15.414 | 8 | 0.052 | 264.975 | 0.419 | 0.588 |
Explanatory variables | B | S.E | Wald | df. | Sig. | Exp(B) | 95% C.I. for EXP(B) | |
Lower | Upper | |||||||
perceived susceptibility | 1.608 | 0.254 | 40.010 | 1 | 0.000 | 4.992 | 3.033 | 8.215 |
perceived severity | 1.131 | 0.643 | 3.096 | 1 | 0.078 | 3.099 | 0. 879 | 10.927 |
self-efficacy | 0.089 | 0.431 | 0.043 | 1 | 0.836 | 1.094 | 0.470 | 2.545 |
perceived barriers | 0.080 | 0.490 | 0.027 | 1 | 0.870 | 1.084 | 0.415 | 2.830 |
cues to action | 1.541 | 0.472 | 10.661 | 1 | 0.001 | 4.671 | 1.852 | 11.783 |
Constant | -6.588 | 1.211 | 29.593 | 1 | 0.000 | 0.001 |
Predicted deforested area | 0 | 1 | Corrected percentage |
Observed deforested area | |||
0 | 240 | 17 | 93.4 |
1 | 42 | 77 | 64.7 |
Overall corrected percentage | - | - | 84.3 |
Health belief model in this study | Health belief model of non-communicable disease prevention behaviors | |||
Significant | Non-significant | Significant | Non-significant | |
perceived susceptibility, perceived severity, cues to action | self-efficacy, perceived barriers | perceived benefits, perceived barriers, self-efficacy, cues to action, perceived benefits, perceived severity, and perceived susceptibility |