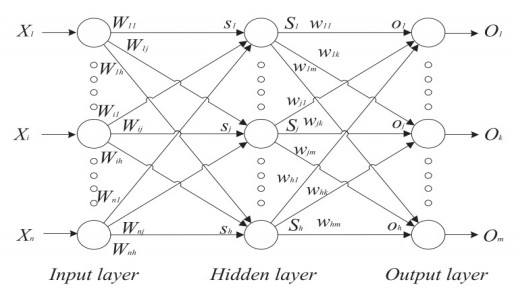
This study mainly used The Cancer Genome Atlas (TCGA) RNA sequencing dataset to screen prognostic snoRNAs of acute myeloid leukemia (AML), and used for the construction of prognostic snoRNAs signature for AML. A total of 130 AML patients with RNA sequencing dataset were used for prognostic snoRNAs screenning. SnoRNAs co-expressed genes and differentially expressed genes (DEGs) were used for functional annotation, as well as gene set enrichment analysis (GSEA). Connectivity Map (CMap) also used for potential targeted drugs screening. Through genome-wide screening, we identified 30 snoRNAs that were significantly associated with the prognosis of AML. Then we used the step function to screen a prognostic signature composed of 14 snoRNAs (SNORD72, SNORD38, U3, SNORA73B, SNORD79, SNORA73, SNORD12B, SNORA74, SNORD116-12, SNORA65, SNORA14, snoU13, SNORA75, SNORA31), which can significantly divide AML patients into high- and low-risk groups. Through GSEA, snoRNAs co-expressed genes and DEGs functional enrichment analysis, we screened a large number of potential functional mechanisms of this prognostic signature in AML, such as phosphatidylinositol 3-kinase-Akt, Wnt, epithelial to mesenchymal transition, T cell receptors, NF-kappa B, mTOR and other classic cancer-related signaling pathways. In the subsequent targeted drug screening using CMap, we also identified six drugs that can be used for AML targeted therapy, they were alimemazine, MG-262, fluoxetine, quipazine, naltrexone and oxybenzone. In conclusion, our current study was constructed an AML prognostic signature based on the 14 prognostic snoRNAs, which may serve as a novel prognostic biomarker for AML.
Citation: Rui Huang, Xiwen Liao, Qiaochuan Li. Integrative genomic analysis of a novel small nucleolar RNAs prognostic signature in patients with acute myelocytic leukemia[J]. Mathematical Biosciences and Engineering, 2022, 19(3): 2424-2452. doi: 10.3934/mbe.2022112
[1] | Rami AL-HAJJ, Mohamad M. Fouad, Mustafa Zeki . Evolutionary optimization framework to train multilayer perceptrons for engineering applications. Mathematical Biosciences and Engineering, 2024, 21(2): 2970-2990. doi: 10.3934/mbe.2024132 |
[2] | Chunmei He, Hongyu Kang, Tong Yao, Xiaorui Li . An effective classifier based on convolutional neural network and regularized extreme learning machine. Mathematical Biosciences and Engineering, 2019, 16(6): 8309-8321. doi: 10.3934/mbe.2019420 |
[3] | Mengya Zhang, Qing Wu, Zezhou Xu . Tuning extreme learning machine by an improved electromagnetism-like mechanism algorithm for classification problem. Mathematical Biosciences and Engineering, 2019, 16(5): 4692-4707. doi: 10.3934/mbe.2019235 |
[4] | Sijie Wang, Shihua Zhou, Weiqi Yan . An enhanced whale optimization algorithm for DNA storage encoding. Mathematical Biosciences and Engineering, 2022, 19(12): 14142-14172. doi: 10.3934/mbe.2022659 |
[5] | Rong Zheng, Heming Jia, Laith Abualigah, Qingxin Liu, Shuang Wang . An improved arithmetic optimization algorithm with forced switching mechanism for global optimization problems. Mathematical Biosciences and Engineering, 2022, 19(1): 473-512. doi: 10.3934/mbe.2022023 |
[6] | Juan Du, Jie Hou, Heyang Wang, Zhi Chen . Application of an improved whale optimization algorithm in time-optimal trajectory planning for manipulators. Mathematical Biosciences and Engineering, 2023, 20(9): 16304-16329. doi: 10.3934/mbe.2023728 |
[7] | Akansha Singh, Krishna Kant Singh, Michal Greguš, Ivan Izonin . CNGOD-An improved convolution neural network with grasshopper optimization for detection of COVID-19. Mathematical Biosciences and Engineering, 2022, 19(12): 12518-12531. doi: 10.3934/mbe.2022584 |
[8] | Chun Li, Ying Chen, Zhijin Zhao . Frequency hopping signal detection based on optimized generalized S transform and ResNet. Mathematical Biosciences and Engineering, 2023, 20(7): 12843-12863. doi: 10.3934/mbe.2023573 |
[9] | Hao Yuan, Qiang Chen, Hongbing Li, Die Zeng, Tianwen Wu, Yuning Wang, Wei Zhang . Improved beluga whale optimization algorithm based cluster routing in wireless sensor networks. Mathematical Biosciences and Engineering, 2024, 21(3): 4587-4625. doi: 10.3934/mbe.2024202 |
[10] | Jia-Gang Qiu, Yi Li, Hao-Qi Liu, Shuang Lin, Lei Pang, Gang Sun, Ying-Zhe Song . Research on motion recognition based on multi-dimensional sensing data and deep learning algorithms. Mathematical Biosciences and Engineering, 2023, 20(8): 14578-14595. doi: 10.3934/mbe.2023652 |
This study mainly used The Cancer Genome Atlas (TCGA) RNA sequencing dataset to screen prognostic snoRNAs of acute myeloid leukemia (AML), and used for the construction of prognostic snoRNAs signature for AML. A total of 130 AML patients with RNA sequencing dataset were used for prognostic snoRNAs screenning. SnoRNAs co-expressed genes and differentially expressed genes (DEGs) were used for functional annotation, as well as gene set enrichment analysis (GSEA). Connectivity Map (CMap) also used for potential targeted drugs screening. Through genome-wide screening, we identified 30 snoRNAs that were significantly associated with the prognosis of AML. Then we used the step function to screen a prognostic signature composed of 14 snoRNAs (SNORD72, SNORD38, U3, SNORA73B, SNORD79, SNORA73, SNORD12B, SNORA74, SNORD116-12, SNORA65, SNORA14, snoU13, SNORA75, SNORA31), which can significantly divide AML patients into high- and low-risk groups. Through GSEA, snoRNAs co-expressed genes and DEGs functional enrichment analysis, we screened a large number of potential functional mechanisms of this prognostic signature in AML, such as phosphatidylinositol 3-kinase-Akt, Wnt, epithelial to mesenchymal transition, T cell receptors, NF-kappa B, mTOR and other classic cancer-related signaling pathways. In the subsequent targeted drug screening using CMap, we also identified six drugs that can be used for AML targeted therapy, they were alimemazine, MG-262, fluoxetine, quipazine, naltrexone and oxybenzone. In conclusion, our current study was constructed an AML prognostic signature based on the 14 prognostic snoRNAs, which may serve as a novel prognostic biomarker for AML.
Since McCulloch and Pitts first proposed the primary theory and learning model of artificial neural networks [1]. ANNs can be considered as an effective model that can get desirable results for processing supervised/unsupervised machine learning tasks [2]. Hence, ANNs have been widely used in various fields as a powerful and practical tool to solve real problems, such as forecast and estimation [3], biology and medicine [4], classification [5], pattern recognition [6] and so on [7]. Among different artificial neural networks, feed-forward neural networks (FNNs) [8], especially two-layer FNNs, is widely applied [9]. In fact, FNNs with two layers is the simplest and most widespread practical application. For any type of neural network, learning is considered an important and critical process and attracts lots of researchers. The learning process is a key field and the purpose of which is to optimize the cost function for feedforward neural networks. The cost function is considered to be the mean square error (MSE) obtained by the optimal connection of the weights and bias values.
Generally, the training methods of feedforward neural networks can be seen in two classes: gradient-based search methods and metaheuristic search methods. The most popular search method in this area is the so-called Back-Propagation (BP) algorithm [10], which is a gradient-based method. When dealing with complex optimal problems, it may quickly converge to the local optimal solution instead of the global optimal solution. As a result, back-propagation cannot obtain a better candidate solution. In addition, the initial solutions of weights, biases, and learning rates determines the performance of the BP algorithm. Even if the learning target is reached, this results in the slow performance of the BP algorithm.
In recent years, a growing number of scholars' regard metaheuristic search methods as the training method of feedforward neural networks to alleviate this main drawback. In the stochastic algorithms, Montana and Davis proposed genetic algorithm (GA) is the first training method to optimize the feedforward neural network [11], they describe a set of experiments performed on data from a sonar image classification problem. The experimental results display that GA is superior to the BP algorithm when solving complex real problems. Li et al. proposed an improving particle swarm optimization algorithm based on neighborhood and historical memory (PSONHM) [12], and employed to investigate the efficiencies of PSONHM in training Multi-Layer Perceptron (MLP). The experimental results indicate that the proposed PSONHM can effectively solve the global optimization problems. Gambhir et al. developed a PSO-ANN based diagnostic model for earlier diagnosis of dengue fever [13]. In the proposed model, PSO technique is applied to optimize the weight and bias parameters of ANN method. The effectiveness of the proposed model is evaluated based on accuracy, sensitivity, specificity, error rate and AUC parameters. The results of the proposed model have been compared with other existing approaches like ANN, DT, NB, and PSO. Mirjalili et al. proposed using a social spider optimization algorithm to train feedforward neural networks [14]. The experimental results show that the algorithm has fast convergence speed and high accuracy in most test data sets. Uzlu et al. study is associated with predicting energy consumption in Turkey [15]. GDP (gross domestic product), population, import and export were used as predictor variables. TLBO (teaching–learning-based optimization) and BP were used to train ANNs. ANN-TLBO predicted the energy consumption more accurately than ANN–BP. Using the ANN–TLBO model, the energy consumption was forecasted until 2020. Aljarah et al. proposed a new training algorithm based on the recently proposed whale optimization algorithm (WOA) [16]. The results are verified by comparisons with back-propagation algorithm and six evolutionary techniques. Kowalski and Łukasik to compare the Krill Herd Algorithm (KHA) optimization algorithm used for learning an artificial neural network (ANN), with other heuristic methods and with more conventional procedures [17]. The proposed ANN training method has been verified for the classification task. It has been concluded that the application of KHA offers promising performance-both in terms of aforementioned metrics, as well as time needed for ANN training. Alboaneen et al. used to glowworm swarm optimisation (GSO) algorithm training the MLP neural network [18]. The GSO based trainer is evaluated on five classification datasets, the results show that our proposed trainer achieves better classification accuracy rate in most datasets compared to the other algorithms. Heidari et al proposed a new hybrid stochastic training algorithm using the recently proposed grasshopper optimization algorithm (GOA) for multilayer perceptrons (MLPs) neural networks [19]. The proposed GOAMLP model is then applied to five important datasets: breast cancer, parkinson, diabetes, coronary heart disease, and orthopedic patients. It is shown and proved that the proposed stochastic training algorithm GOAMLP is substantially beneficial in improving the classification rate of MLPs. Ehsan, et al. proposed an improved cuckoo search algorithm [20]. It is employed for training feedforward neural networks for two benchmark classification problems. Socha and Blum proposed a Ant Colony Optimization (ACO) variant for continuous optimization [21,22]. The results show that the best of our algorithms are comparable to gradient-based algorithms for neural network training [23,24,25,26].
In 2016, Mirjalili and Lewis proposed the whale optimization algorithm [27]. It has proved that the WOA algorithm is more competitive than other famous meta-heuristic algorithms. When dealing with the real problem, it may quickly reach to the local optimal solution instead of the global optimal solution. This phenomenon, called immature convergence, is a common problem in metaheuristics [28]. Another question called the stagnation in local optimal solutions [29]. This question can seriously lead to the degradation of the candidate solution quality. The phenomenon can happen when algorithms fail to get better between exploration and exploitation. Exploration defined as the ability of algorithm to find an unknown area in search space. The exploitation stage must follow the exploration phase. In this stage, the field of the better-explored positions in various regions is included again to get a better candidate solution [30]. Widespread exploration in early iterations and then focused exploitation in the final steps of optimization can help the optimizer to avert local solutions [31].
Studies have shown that some algorithms have better local search capabilities [32], some algorithms have better global search capabilities, and some optimization algorithms profit from a better balance between exploration and exploitation. Hence, hybrid algorithms can decrease the inherent defects of the original algorithm [33]. With this strategy, the hybrid algorithms gain all the superiority of the original algorithm. The primary intention of hybrid optimizers is to decrease the amount of computation, increase the accuracy of the results, enhanced the stability of the algorithm and the convergence trend of the basic algorithm [34]. This paper presents an improved whale optimization algorithm which aimed to enhance the precision of the convergence of the basic WOA algorithm. In order to test the performance of the algorithm, the optimal weight and biases of MLP neural networks are used for classification problems and experimental results indicate that this algorithm has better classification accuracy, convergence speed, and convergence precision than other algorithms in optimizing multi-layer perceptron neural network.
The research content of this paper is as follows: the basic definition of MLP is described in section 2. Section 3 describes the original WOA algorithm. The detailed description of the TSWOA is presented in section 4. Section 5 details how the TSWOA can be used for training MLP. Various experimental results and analyses were conducted in section 6. We conclude in section 7.
Feedforward neural network is a prevalent structure of neural network model which employs its sophisticated parallel layered to understand and approximate computing models [35]. It is made up of many neurons distributed in different levels. The input layer is located in the first layer of feedforward neural network, the output layer is located in the last layer, and the hidden layer is located in the other layer. Feedforward neural networks that have many hidden layers are called multilayer perceptron (MLP), which is displayed in Figure 1. In MLP, neurons are organized in a unidirectional pattern. In the network form, the message is spread in one direction, respectively through the input layer, the hidden layer, and the output layer. As shown in Figure 1, the data is input by the input layer, multiplied by the respective weights, and then taken as the input of the hidden layer. After the calculation of the hidden layer, the data is spread to the output layer by multiplying the respective weights, and the output is calculated by the output layer. The actual output of the neuron is calculated by the activation function. The operation step of MLP is displayed in Eqs (1)–(4).
Firstly, the input value of the hidden layer is obtained by using the Eq (1)
sj=n∑i=1(wi,j∗xi)−θj,j=1,2,…,h | (1) |
where n represents the total number of the input layer, wi,j is the connection weight, bias value of the j-th node is expressed as θj, and xi is i-th input data.
The output value of the hidden layer is obtained by using the Eq (2),
Sj=sigmoid(sj)=1(1+exp(−sj)),j=1,2,…h | (2) |
Finally, the result of the output layer is described as follows:
ok=h∑j=1(wj,k∗Sj)−θ′k,k=1,2,…,m | (3) |
Ok=sigmoid(ok)=1(1+exp(−ok)),k=1,2,…k | (4) |
where wj,k is the connection weight from the j-th node to the k-th node, θ′k expresses the bias value of the k-th node in the output layer, and Ok is the output value of the k-th node. From Eqs (1)–(4), it can be concluded that the connection weights and bias values are the most important parts of the MLP, and they confirm the final value of the output. The ultimate goal of optimizing MLP is to get the optimal connection weights and bias to achieve the output.
There are three main stages in the whale optimization algorithm, which are searching prey, encircling prey and Logarithmic spiral prey. Among them, searching for prey is the exploration stage of the algorithm. Moreover, encircling prey and Logarithmic spiral prey is the exploitation stage of the algorithm. This method of Logarithmic spiral prey is based on creating bubbles by encircling or through a '9' shaped path [27]. It can be modeled as follows:
At this stage, the humpback whale does not know the position of the prey. The humpback whale can only change randomly to update its position. This means that humpback whales randomly select the position of an individual from the current population as prey to update its position. The humpback whales position is shown by the following Eqs (5)–(6):
→D=|→C⋅→Xrand−→X| | (5) |
→X(t+1)=→Xrand−→A⋅→D | (6) |
where →Xrand represents the random vector (a random whale). The coefficient vectors are represented by →A and →C, and the calculation process is expressed as follows:
→A=2→a⋅→r−→a | (7) |
→C=2⋅→r | (8) |
where the vector →a is declining linearly from 2 to 0, and →r is a random vector in the range of 0 and 1.
In the WOA, since it is impossible to know the location of the optimal solution in the search space, the algorithm assumes that the prey is the best candidate solution (the optimal whale location) or close to the optimal solution. After obtaining the best whale position, all whales update their positions towards the current optimal whale position. The calculation process shown as follows:
→D=|→C⋅→X∗(t)−→X(t)| | (9) |
→X(t+1)=→X∗(t)−→A⋅→D | (10) |
where current iteration is shown by t, X∗ represents the position of the optimal solution. if |A|>1, the searching prey is represented by Eqs (5)–(6), otherwise the encircling prey is represented by Eqs (9)–(10), which expresses the shrinking mechanism.
At this stage, the distance between the individual whale and the optimal whale (prey) position is first calculated, and then the whale moves up the water surface in a spiral shape while spitting out many bubbles of varying sizes to prey. The whales are updated as follows:
→X′(t+1)=→D′⋅ebl⋅cos(2πl)+→X∗(t) | (11) |
where →D′=|→X∗(t)−→X(t)| shows the distance from whale to its prey, l is a random number, which is the range of –1 and 1, the shape of the logarithmic spiral is defined as b, which is the constant number.
In the process of predation, whales have two ways of predation. Therefore, we assume that their probability of encircling mechanism is 50%, and the spiral model updates their positions with the same probability, and its model is as follows:
→X(t+1)={→X∗(t)−→A⋅→Difp<0.5→D′⋅ebl⋅cos(2πl)+→X∗(t)ifp>0.5 | (12) |
where p is a random number, which is the range of 0 and 1. The pseudo-code of WOA can be displayed in Algorithm 1 below.
Algorithm 1. WOA pseudo-code |
1. Initialize the whale population Xi(i=1,2,....n)
2. Initialize a, A, and C, l, and p 3. Calculate the fitness of each search agent 4. X∗ = the best search agent 5. while (t<max_iteration) 6. for each search agent 7. Update a, A, and C, l, and p 8. if1 (p<0.5) 9. if2 (|A|<1) 10. Update the position of the current search agent by Eq (10) 11. else if2 (|A|⩾1) 12. Select a random search agent (Xrand) 13. Update the position of the current search agent by Eq (6) 14. end if2 15. else if1(p⩾0.5) 16. Update the position of the current search by Eq (11) 17. end if1 18. end for 19. Check if any search agent goes beyond the search space and amend it 20. Calculate the fitness of each search agent 21. Update X∗ if there is a better solution 22. t=t+1 23. end while 24. Return X* |
In the literature [36], the effectiveness of WOA in solving some real problems has been verified. Due to the role of leaders, the WOA has better search capability. These random leaders enable the whale algorithm to increase the diversity of the population in the early stages. However, the algorithm has problems such as slow convergence accuracy and difficulty in jumping out of local optimum. In addition, when researchers use WOA to solve complex real-world optimization problems, WOA needs some improvements to obtain better performance. Inspired by the characteristics of the teaching-learning-based optimization algorithm, we tried to improve the search ability of the WOA algorithm by utilizing the advantages of teaching-learning-based optimization algorithm (TLBO) [37].
It is called TWOA that the teaching phase of TLBO is added to the whale optimization algorithm. On the one hand, it enhances the exploitation capability of the algorithm and improves the quality of candidate solutions. On the other hand, from the biological background, whales are considered as highly intelligent animals with thinking, learning, judging, and communication. TWOA enables whales to have the ability of self-learning, which makes the algorithm more consistent with the biological mechanism. Meanwhile, the improved algorithm is more self-organizing and cooperative. We add the simplex method to the WOA to increase the diversity of the population and enrich the exploration capacity of the algorithm. The description of the new method is provided in the next subsections.
TLBO is a metaheuristic algorithm whose idea comes from the traditional learning process to simulate the teaching of teachers and the learning of students in the class to achieve the purpose of optimization [37,47]. A class is a population in the search space, and the number of people in the class is the size of the population. The subject the student learns could interpreted as different design variables. The learning level represents the fitness of the optimization algorithm, and the teacher is the individual with the best fitness value of the population. There are two mechanisms for exchanging information in the classroom: collaboration between teachers and students and between students. These intra-class actions implemented in two different consecutive processes: the teacher phase is to simulate the influence of teachers on students, and the learner phase is to model the cooperative learning between students.
In the teacher phase, the students with the best fitness values are selected as teachers to improve the learning level of other students in the class. The average level of the class continues to improve, moving towards the optimal solution. The difference between the average level of students (population mean) and teachers is as follows:
Ddifference=rand(0,1)(Xteacher−TfXmean) | (13) |
where Xteacher is the individual with the best fitness value of the current population. Xmean is the mean value of the class, which is defined as Xmean=n∑i=1Xi/n. The value of Tf can be either 1 or 2, which is again a heuristic step and decided randomly with equal probability as Tf=round(1+rand(0,1)). In the teacher phase, the i-th learner in the class generates a new individual according to the following:
Xinew=Xiold+Ddifference | (14) |
In the actual teaching process, the teacher's teaching is a heuristic process, and students may not be able to improve themselves effectively according to the teacher's teaching. Continuous learning from other students after class can improve their ability more effectively, which is the learning stage of the TLBO algorithm.
In the learner phase, randomly select two learners Xi and Xj from the class, where i≠j. The i-th learner in the class generates a new individual according to the following:
Xinew={Xiold+round(0,1)(Xi−Xj)f(Xi)<f(Xj)Xiold+round(0,1)(Xj−Xi)f(Xi)>f(Xj) | (15) |
In this section, we mainly introduce the combination of teacher phase and whale optimization algorithm and we explain how to improve the quality of candidate solutions. To increase the exploitation, avoid falling into local optimum, and improve the quality of candidate solutions, we consider adding the teacher phase of TLBO to the algorithm to enable whale agents to have a capacity of self-learning. After each update of the whale agent, the fitness value of each whale agent was calculated. Then select the best whale agent and use formula 14 to guide other whale agents to move towards the current best position to improve the average performance of individuals in the population. The implementation steps of TWOA as follows:
Algorithm 2. TWOA pseudo-code |
1. Initialize the whales population Xi(i=1,2,....n)
2. Initialize a, A, and C, l, and p 3. Calculate the fitness of each search agent 4. X∗ = the best search agent 5. while (t<max_iteration) 6. for each search agent 7. Update a, A, and C, l, and p 8. if1 (p<0.5) 9. if2 (|A|<1) 10. Update the position of the current search agent by Eq (10) 11. else if2 (|A|⩾1) 12. Select a random search agent (Xrand) 13. Update the position of the current search agent by Eq (6) 14. end if2 15. else if1(p⩾0.5) 16. Update the position of the current search by Eq (11) 17. end if1 18. Update the position of each search agent by Eq (14) 19. Check if any search agent goes beyond the search space and amend it 20 end for 21. t=t+1 22 end while 23. Return X* |
Although TWOA enhances the convergence precision of the WOA, it is easy to fall into the local optimum, so the Simplex method (SM) [38] is added to not only increase the diversity of population but also change the search direction of the algorithm and expand the search space. The simplex method obtains higher search accuracy by optimizing and has a good advantage in searching. The simplex method uses the optimization idea of reflection, expansion, and contraction of a convex figure (simplex) in multidimensional space to reach the minimum point [39]. Therefore, it is easy to help the algorithm to jump out of local optima. In this paper, after each update of the whale agent in the TWOA algorithm, the simplex method is run, where the specific addition position is shown in the pseudocode of Algorithm 3. The schematic diagram of the simplex method (Figure 2) is shown below:
The specific process of the simplex method is described as follows:
Step 1: Obtaining the objective function value of all points, and getting the best point as the Xg, and then getting the second-best point as the Xb, supposing that Xs is the one should be substituted. f(Xs), f(Xb) and f(Xg) show the objective function values.
Step 2: Obtaining the objective function value of the middle point Xc between point Xg and point Xb:
XC=Xg+Xb2 | (16) |
Step 3: Obtaining the reflection point Xr by using the following formula. α is the reflection coefficient, which is set as 1:
Xr=Xc+α(Xc−Xs) | (17) |
Step 4: If f(Xr)<f(Xg), getting the expansion point from formula 18:
Xe=Xc+γ(Xr−Xc) | (18) |
where the expansion coefficient is γ, which usually set to 2. If f(Xe)<f(Xg), Xs will be replaced by Xe; otherwise, Xs will substitute Xr.
Step 5: If f(Xr)<f(Xs), the compression point can be acquired by the formula (19):
Xt=Xc+β(Xs−Xc) | (19) |
where β represents the cohesion coefficient, which is set to 0.5. If f(Xt)<f(Xs), replace Xs by Xt; otherwise, Xt substitute Xs.
Step 6: If f(Xg)<f(Xr)<f(Xs), shrink point is Xw, and the shrink coefficient is β.
Xw=Xc−β(Xs−Xc) | (20) |
If f(Xw)<f(Xs), substitute Xs by Xw; otherwise, substitute Xs by Xr.
According to the simplex method above, this method diversifies the population, ensuring the algorithm can search efficiently, and reducing the algorithm fall into local optimum. The implementation steps of TSWOA are as follows.
Algorithm 3. TSWOA pseudo-code |
1. Initialize the whales population Xi(i=1,2,....n)
2. Initialize a, A, and C, l, and p 3. Calculate the fitness of each search agent 4. X∗=the best search agent 5. while (t<max_iteration) 6. for each search agent 7. Update a, A, and C, l, and p 8. if1 (p<0.5) 9. if2 (|A|<1) 10. Update the position of the current search agent by Eq (10) 11. else if2 (|A|⩾1) 12. Select a random search agent (Xrand) 13. Update the position of the current search agent by Eq (6) 14. end if2 15. else if1(p⩾0.5) 16. Update the position of the current search by Eq (11) 17. end if1 18. Update the position of each search agent by Eq (14) 19. Update the position of the worst search agent using the simplex method [Eqs (16)–(20)] 20. end for 21. Check if any search agent goes beyond the search space and amend it 22. Calculate the fitness of each search agent 23. Update X∗ if there is a better solution 24. t=t+1 25. end while 26. Return X* |
The corresponding to the TSWOA flowchart as follow (see Figure 3):
In this part, we focus on ways to solve the problem. It covers the setting of the candidate solution dimension, the setting of the objective function and the setting of assessment to the experiment results.
Before optimizing MLP, we must first obtain the structure of the MLP network. The number of neurons of the input layer and the output layer is decided by classified data sets, while the number of neurons of the hidden layer is decided by the Kolmogorov theorem [40]. The result is determined by Eq (21).
Hidden=2×Input+1 | (21) |
when TSWOA is adopted to obtain the connection weights and bias values, D represents the dimension of the candidate solution. The result is determined by Eq (22):
D=(Input×Hidden)+(Hidden×Output)+Hiddenbias+Outputbias | (22) |
where the number of neurons of the input layer, hidden layer, and output layer is expressed by Input, Hidden and Output. The number of bias of the hidden layer and the output layer is shown by Hiddenbias and Outputbias.
In the study [41], we can get many encoding ways to optimize MPL. The mathematical models of the TSWOA algorithm are all represented by vectors, so we use vectors to represent. X={X1,X2,X3,…,Xn} represents N agents in the population. Each agent in population is denoted by Xi={iw,hw,hb,ob},i=1,2,…,n, the weights of the input layer and the hidden layer are denoted by iw and hw, the biases of the hidden layer and the output layer are shown by hb and ob.
In optimizing MLP, it is crucial to define appropriate objective functions. Generally, after agents are defined by vectors, the objective function of the neural network needs to be used to define the fitness function of the TSWOA algorithm. Hence, the fitness function is considered as the difference between the theoretical output and the actual output in the neural network, which is obtained by using Eq (23):
MSE=∑mi=1(oki−dki)2 | (23) |
where the total number of output is shown by m, the desired output and the actual output of i-th input are exhibited by dki and Oki.
Apparently, training neural networks requires data sets, and there are many training data sets. So, the fitness function is the mean value of MSE, which is obtained by using formula 24:
¯MSE=∑sk=1∑mi=1(oki−dki)2s | (24) |
where the total number of training data sets is shown by s, other parameters are the same as the formula above. Therefore, the objective function of optimizing MLP is defined:
F=Minimize(¯MSE) | (25) |
Because classified data is used to optimize MLP, classification problems often involve learning existing data to forecast undiscovered data. In classification problems, accuracy is considered to assessing the classification capacity of the algorithm, which is defined as follows:
Accuracy=¯NN | (26) |
where ¯N shows the correct number for the classification, the total number of datasets is denoted by N.
In this section to test the validity of the algorithm for optimizing MPL, fifteen data sets from the UCI machine learning knowledge were obtained. There are 15 data sets displaying in Table 1. To better test the performance of TSWOA, the other six state-of-the-art algorithms are adopted: grasshopper optimization algorithm (GOA) [19], glowworm swarm optimization (GSO) [42], social spider optimization algorithm(SSO) [43], flower pollination algorithm(FPA) [19], genetic algorithm (GA) [11], and whale optimization algorithm(WOA) [44].
Datasets | Attribute | Class | Training | Testing | Input | Hidden | Output |
Blood | 4 | 2 | 493 | 255 | 4 | 9 | 2 |
Scale | 4 | 3 | 412 | 213 | 4 | 9 | 3 |
Survival | 3 | 2 | 202 | 104 | 3 | 7 | 2 |
Liver | 6 | 2 | 227 | 118 | 6 | 13 | 2 |
Seeds | 7 | 3 | 139 | 71 | 7 | 15 | 3 |
Wine | 13 | 3 | 117 | 61 | 13 | 27 | 3 |
Iris | 4 | 3 | 99 | 51 | 4 | 9 | 3 |
Statlog | 13 | 2 | 178 | 92 | 13 | 27 | 2 |
Cancer | 9 | 2 | 461 | 238 | 9 | 19 | 2 |
Diabetes | 8 | 2 | 507 | 261 | 8 | 17 | 2 |
Gene | 57 | 2 | 70 | 36 | 57 | 115 | 2 |
Parkinson | 22 | 2 | 129 | 66 | 22 | 45 | 2 |
Splice | 60 | 2 | 660 | 340 | 60 | 121 | 2 |
WDBC | 30 | 2 | 394 | 165 | 30 | 61 | 2 |
Zoo | 16 | 7 | 67 | 34 | 16 | 33 | 7 |
All used codes in this study have been implemented in the same manner using MATLAB R2017 (a) and run on a PC with the Windows 10 64-bit and Intel Core (TM) i5-4590 processor, 3.30 ghz and 4 GB RAM. For all data sets above, data for training and testing respectively accounted for 66% and 34% of the total data [45]. To obtain great accuracy of experimental results, each algorithm runs 20 times. The number of iterations is 500 in independent experiments. The population number is 30 and the main parameter settings for all algorithms are in Table 2.
Algorithms | Parameter values |
GOA [19] | Cmin= 0.00004, Cmax= 1 |
GSO [42] | Luciferin decay value is 0.4, luciferin enhancement is 0.6, the rate of the neighborhood range is 0.08, the number of neighbors is 5, the step size of moving is 0.3, initial luciferin is 5 |
SSO [43] | PF = 0.7 |
FPA [42] | P = 0.8 |
GA [11] | Crossover rate is 1, Mutation rate is 0.01 |
WOA [44] | →α Linearly decreased from 2 to 0 have been used as recommended in [44]. |
TSWOA | →α Linearly decreased from 2 to 0 have been used as recommended in [44] |
The 15 data sets were obtained from the UCI machine learning knowledge, namely, Blood, Scale, Survival, Liver, Seeds, Wine, Iris, Statlog, Cancer, Diabetes, Gene, Parkinson, Splice, WDBC, and Zoo. The experimental results are displayed in Tables 3–17, in which the "Best" is the best value, the "Worst" is worst value, the "Mean" is the mean value and the "Std" is the standard deviation. Accuracy is the best accuracy of 20 independent runs, and rank is the algorithm ranked according to accuracy. Due to the randomness of the swarm intelligence algorithm in the process of operation, the statistical test is important [46]. In order to obtain the difference between the improved algorithm and contrastive algorithms, Wilcoxson's rank-sum test is also added in the experiment to obtain whether there is an obvious difference between the two groups of data. When the value is less than 0.05, the difference between the two groups of data is obvious. The p values are revealed in Table 18.
Algorithms | Best | Worst | Mean | Std | Accuracy | Rank |
GOA | 3.28E − 01 | 3.55E − 01 | 3.37E − 01 | 6.32E − 03 | 78.43 | 3 |
GSO | 3.45E − 01 | 3.96E − 01 | 3.66E − 01 | 1.49E − 02 | 76.47 | 6 |
SSO | 3.27E − 01 | 3.65E − 01 | 3.49E − 01 | 9.11E − 03 | 78.82 | 2 |
FPA | 3.30E − 01 | 3.69E − 01 | 3.47E − 01 | 9.69E − 03 | 78.04 | 4 |
GA | 3.30E − 01 | 4.15E − 01 | 3.82E − 01 | 2.60E − 02 | 77.25 | 5 |
WOA | 3.30E − 01 | 3.61E − 01 | 3.47E − 01 | 1.06E − 02 | 76.25 | 7 |
TSWOA | 3.05E − 01 | 3.20E − 01 | 3.11E − 01 | 4.37E − 03 | 80.39 | 1 |
Algorithms | Best | Worst | Mean | Std | Accuracy | Rank |
GOA | 2.78E − 01 | 7.23E − 01 | 4.45E − 01 | 1.11E − 01 | 78.87 | 3 |
GSO | 5.02E − 01 | 1.10E + 00 | 7.95E − 01 | 1.42E − 01 | 61.50 | 6 |
SSO | 2.34E − 01 | 6.13E − 01 | 3.94E − 01 | 7.89E − 02 | 77.94 | 4 |
FPA | 2.01E − 01 | 3.50E − 01 | 2.46E − 01 | 3.73E − 02 | 89.67 | 1 |
GA | 2.82E − 01 | 6.91E − 01 | 5.04E − 01 | 1.09E − 01 | 76.53 | 5 |
WOA | 1.86E − 01 | 4.33E − 01 | 3.06E − 01 | 7.43E − 02 | 86.38 | 2 |
TSWOA | 1.16E − 01 | 5.81E − 01 | 1.75E − 01 | 9.65E − 02 | 89.67 | 1 |
Algorithms | Best | Worst | Mean | Std | Accuracy | Rank |
GOA | 3.81E − 01 | 4.03E − 01 | 3.95E − 01 | 5.57E − 03 | 81.73 | 1 |
GSO | 3.99E − 01 | 4.48E − 01 | 4.23E − 01 | 1.37E − 02 | 81.73 | 1 |
SSO | 3.93E − 01 | 4.33E − 01 | 4.15E − 01 | 1.05E − 02 | 81.73 | 1 |
FPA | 3.82E − 01 | 4.18E − 01 | 4.02E − 01 | 9.55E − 03 | 79.8 | 4 |
GA | 3.99E − 01 | 5.16E − 01 | 4.53E − 01 | 3.16E − 02 | 81.72 | 2 |
WOA | 3.79E − 01 | 4.25E − 01 | 4.06E − 01 | 1.40E − 02 | 81.73 | 1 |
TSWOA | 3.38E − 01 | 3.83E − 01 | 3.64E − 01 | 1.10E − 02 | 80.77 | 3 |
Algorithms | Best | Worst | Mean | Std | Accuracy | Rank |
GOA | 4.46E − 01 | 4.72E − 01 | 4.62E − 01 | 8.53E − 03 | 60.17 | 3 |
GSO | 4.86E − 01 | 5.41E − 01 | 5.14E − 01 | 1.54E − 02 | 55.93 | 6 |
SSO | 4.84E − 01 | 5.25E − 01 | 4.99E − 01 | 9.82E − 03 | 56.80 | 4 |
FPA | 4.49E − 01 | 5.30E − 01 | 4.95E − 01 | 1.82E − 02 | 62.71 | 2 |
GA | 5.06E − 01 | 6.91E − 01 | 5.93E − 01 | 5.33E − 02 | 49.15 | 7 |
WOA | 4.51E − 01 | 4.83E − 01 | 4.73E − 01 | 8.92E − 03 | 56.78 | 5 |
TSWOA | 3.65E − 01 | 4.41E − 01 | 3.94E − 01 | 1.90E − 02 | 72.88 | 1 |
Algorithms | Best | Worst | Mean | Std | Accuracy | Rank |
GOA | 2.69E − 01 | 8.34E − 01 | 4.68E − 01 | 1.89E − 01 | 77.46 | 4 |
GSO | 5.58E − 01 | 1.20E + 00 | 9.45E − 01 | 1.99E − 01 | 43.66 | 6 |
SSO | 1.42E − 01 | 4.56E − 01 | 3.14E − 01 | 7.22E − 02 | 83.10 | 2 |
FPA | 7.19E − 02 | 2.59E − 01 | 1.45E − 01 | 4.49E − 02 | 95.77 | 1 |
GA | 1.82E − 01 | 7.39E − 01 | 4.33E − 01 | 1.45E − 01 | 70.42 | 5 |
WOA | 1.10E − 01 | 4.37E − 01 | 2.34E − 01 | 9.62E − 02 | 81.69 | 3 |
TSWOA | 2.59E − 02 | 6.68E − 01 | 1.62E − 01 | 1.72E − 01 | 95.77 | 1 |
Algorithms | Best | Worst | Mean | Std | Accuracy | Rank |
GOA | 2.79E − 01 | 9.92E − 01 | 6.72E − 01 | 2.18E − 01 | 67.21 | 5 |
GSO | 6.34E − 01 | 1.58E + 00 | 1.18E + 00 | 2.46E − 01 | 44.26 | 7 |
SSO | 1.81E − 01 | 6.75E − 01 | 4.57E − 01 | 1.35E − 01 | 81.97 | 4 |
FPA | 1.11E − 01 | 2.65E − 01 | 1.69E − 01 | 4.59E − 02 | 85.25 | 2 |
GA | 4.27E − 01 | 9.20E − 01 | 6.81E − 01 | 1.38E − 01 | 52.46 | 6 |
WOA | 1.66E − 01 | 3.54E − 01 | 2.68E − 01 | 5.62E − 02 | 83.61 | 3 |
TSWOA | 1.73E − 02 | 5.13E − 01 | 1.62E − 01 | 1.62E − 01 | 96.72 | 1 |
Algorithms | Best | Worst | Mean | Std | Accuracy | Rank |
GOA | 3.04E − 01 | 7.35E − 01 | 4.68E − 01 | 1.17E − 01 | 84.31 | 6 |
GSO | 4.51E − 01 | 8.96E − 01 | 6.77E − 01 | 1.39E − 01 | 70.59 | 7 |
SSO | 2.08E − 01 | 4.64E − 01 | 3.44E − 01 | 6.76E − 02 | 96.08 | 3 |
FPA | 2.02E − 02 | 1.81E − 01 | 9.64E − 02 | 4.04E − 02 | 96.03 | 4 |
GA | 1.39E − 01 | 7.28E − 01 | 4.20E − 01 | 1.76E − 01 | 98.03 | 2 |
WOA | 8.79E − 02 | 3.81E − 01 | 2.47E − 01 | 7.70E − 02 | 94.12 | 5 |
TSWOA | 2.63E − 02 | 6.47E − 01 | 1.51E − 01 | 1.90E − 01 | 98.04 | 1 |
Algorithms | Best | Worst | Mean | Std | Accuracy | Rank |
GOA | 2.54E − 01 | 5.79E − 01 | 4.57E − 01 | 1.19E − 01 | 79.35 | 4 |
GSO | 5.28E − 01 | 7.44E − 01 | 6.35E − 01 | 5.78E − 02 | 46.74 | 7 |
SSO | 3.26E − 01 | 5.25E − 01 | 4.12E − 01 | 4.83E − 02 | 75 | 5 |
FPA | 2.75E − 01 | 3.88E − 01 | 3.13E − 01 | 3.37E − 02 | 80.69 | 3 |
GA | 4.14E − 01 | 6.90E − 01 | 5.67E − 01 | 8.57E − 02 | 70.65 | 6 |
WOA | 2.44E − 01 | 3.51E − 01 | 2.94E − 01 | 2.98E − 02 | 83.69 | 2 |
TSWOA | 1.12E − 01 | 2.00E − 01 | 1.40E − 01 | 2.23E − 02 | 83.70 | 1 |
Algorithms | Best | Worst | Mean | Std | Accuracy | Rank |
GOA | 6.23E − 02 | 2.69E − 01 | 1.72E − 01 | 7.95E − 02 | 97 | 3 |
GSO | 7.96E − 02 | 3.78E − 01 | 2.49E − 01 | 6.78E − 02 | 89 | 4 |
SSO | 7.09E − 02 | 1.72E − 01 | 1.13E − 01 | 2.69E − 02 | 98 | 2 |
FPA | 5.01E − 02 | 8.68E − 02 | 6.79E − 02 | 9.31E − 03 | 99 | 1 |
GA | 7.79E − 02 | 3.61E − 01 | 1.70E − 01 | 7.30E − 02 | 98 | 2 |
WOA | 5.29E − 02 | 1.37E − 01 | 7.53E − 02 | 2.57E − 02 | 99 | 1 |
TSWOA | 2.78E − 02 | 2.50E − 01 | 4.86E − 02 | 4.93E − 02 | 98 | 2 |
Algorithms | Best | Worst | Mean | Std | Accuracy | Rank |
GOA | 3.59E − 01 | 4.04E − 01 | 3.83E − 01 | 1.41E − 02 | 75.87 | 3 |
GSO | 4.24E − 01 | 6.03E − 01 | 5.15E − 01 | 4.54E − 02 | 64.75 | 7 |
SSO | 4.18E − 01 | 5.03E − 01 | 4.52E − 01 | 2.35E − 02 | 65.51 | 6 |
FPA | 3.85E − 01 | 4.75E − 01 | 4.44E − 01 | 2.48E − 02 | 80.08 | 2 |
GA | 4.81E − 01 | 6.40E − 01 | 5.41E − 01 | 4.49E − 02 | 67.05 | 5 |
WOA | 3.54E − 01 | 4.29E − 01 | 3.90E − 01 | 2.20E − 02 | 73.18 | 4 |
TSWOA | 3.04E − 01 | 3.29E − 01 | 3.19E − 01 | 6.10E − 03 | 81.23 | 1 |
Algorithms | Best | Worst | Mean | Std | Accuracy | Rank |
GOA | 8.09E − 01 | 9.28E − 01 | 9.10E − 01 | 2.68E − 02 | 2.66 | 6 |
GSO | 9.31E − 01 | 9.41E − 01 | 9.38E − 01 | 2.67E − 03 | 1.77 | 7 |
SSO | 3.58E − 01 | 4.74E − 01 | 4.08E − 01 | 3.07E − 02 | 5.56 | 5 |
FPA | 2.43E − 01 | 3.86E − 01 | 3.12E − 01 | 3.80E − 02 | 13.89 | 3 |
GA | 4.55E − 01 | 5.85E − 01 | 5.04E − 01 | 3.23E − 02 | 11.11 | 4 |
WOA | 2.74E − 01 | 4.12E − 01 | 3.24E − 01 | 3.58E − 02 | 16.67 | 2 |
TSWOA | 1.46E − 02 | 3.29E − 01 | 9.43E − 02 | 8.36E − 02 | 33.33 | 1 |
Algorithms | Best | Worst | Mean | Std | Accuracy | Rank |
GOA | 1.48E − 01 | 2.66E − 01 | 2.01E − 01 | 3.06E − 02 | 68.18 | 5 |
GSO | 2.63E − 01 | 5.20E − 01 | 4.22E − 01 | 8.04E − 02 | 56.06 | 7 |
SSO | 1.75E − 01 | 2.54E − 01 | 2.16E − 01 | 2.21E − 02 | 69.7 | 4 |
FPA | 9.30E − 02 | 1.86E − 01 | 1.36E − 01 | 1.99E − 02 | 70.21 | 3 |
GA | 2.07E − 01 | 3.81E − 01 | 3.03E − 01 | 5.03E − 02 | 65.15 | 6 |
WOA | 1.32E − 01 | 2.81E − 01 | 1.85E − 01 | 3.76E − 02 | 71.21 | 2 |
TSWOA | 1.48E − 02 | 2.33E − 01 | 7.63E − 02 | 6.08E − 02 | 74.24 | 1 |
Algorithms | Best | Worst | Mean | Std | Accuracy | Rank |
GOA | 9.41E − 01 | 9.46E − 01 | 9.43E − 01 | 1.12E − 03 | 37.24 | 7 |
GSO | 9.42E − 01 | 9.47E − 01 | 9.45E − 01 | 1.48E − 03 | 40.13 | 6 |
SSO | 6.96E − 01 | 8.64E − 01 | 7.92E − 01 | 4.40E − 02 | 41.18 | 4 |
FPA | 4.56E − 01 | 6.67E − 01 | 5.27E − 01 | 4.71E − 02 | 70 | 2 |
GA | 7.91E − 01 | 8.84E − 01 | 8.52E − 01 | 2.53E − 02 | 41.17 | 5 |
WOA | 4.65E − 01 | 5.58E − 01 | 5.10E − 01 | 2.79E − 02 | 51.47 | 3 |
TSWOA | 1.59E − 01 | 2.37E − 01 | 1.97E − 01 | 2.30E − 02 | 80 | 1 |
Algorithms | Best | Worst | Mean | Std | Accuracy | Rank |
GOA | 1.32E − 01 | 2.83E − 01 | 2.15E − 01 | 4.11E − 02 | 90.9 | 5 |
GSO | 4.09E − 01 | 5.38E − 01 | 5.15E − 01 | 2.92E − 02 | 79.39 | 6 |
SSO | 9.51E − 02 | 3.26E − 01 | 2.20E − 01 | 5.60E − 02 | 93.31 | 4 |
FPA | 1.02E − 01 | 1.57E − 01 | 1.24E − 01 | 1.80E − 02 | 93.33 | 3 |
GA | 3.05E − 01 | 6.79E − 01 | 4.93E − 01 | 1.11E − 01 | 78.79 | 7 |
WOA | 9.98E − 02 | 3.17E − 01 | 1.69E − 01 | 5.68E − 02 | 94.55 | 2 |
TSWOA | 3.07E − 02 | 9.93E − 02 | 4.28E − 02 | 1.77E − 02 | 95.15 | 1 |
Algorithms | Best | Worst | Mean | Std | Accuracy | Rank |
GOA | 2.36E + 00 | 4.47E + 00 | 3.60E + 00 | 5.67E − 01 | 23.70 | 7 |
GSO | 3.46E + 00 | 5.54E + 00 | 5.41E + 00 | 4.59E − 01 | 30.73 | 5 |
SSO | 4.83E − 01 | 2.06E + 00 | 1.15E + 00 | 5.21E − 01 | 44.12 | 4 |
FPA | 1.79E − 01 | 4.63E − 01 | 3.32E − 01 | 7.42E − 02 | 63.61 | 3 |
GA | 1.20E + 00 | 1.99E + 00 | 1.53E + 00 | 2.12E − 01 | 26.47 | 6 |
WOA | 1.97E − 01 | 5.52E − 01 | 4.23E − 01 | 9.97E − 02 | 67.65 | 1 |
TSWOA | 1.34E − 01 | 7.61E − 01 | 3.75E − 01 | 1.85E − 01 | 64.71 | 2 |
Functions | TSWOA vs | |||||
GOA | GSO | SSO | FPA | GA | WOA | |
Blood | 6.80E − 08 | 6.80E − 08 | 6.80E − 08 | 6.80E − 08 | 6.80E − 08 | 6.80E − 08 |
Scale | 6.92E − 07 | 7.90E − 08 | 1.05E − 06 | 1.20E − 06 | 5.23E − 07 | 1.20E − 06 |
Survival | 7.90E − 08 | 6.80E − 08 | 6.80E − 08 | 7.90E − 08 | 6.80E − 08 | 7.90E − 08 |
Liver | 6.80E − 08 | 6.80E − 08 | 6.80E − 08 | 6.80E − 08 | 6.80E − 08 | 6.80E − 08 |
Seeds | 4.68E − 05 | 1.23E − 07 | 1.63E − 03 | 6.76E − 02 | 2.60E − 05 | 7.71E − 03 |
Wine | 6.01E − 07 | 6.80E − 08 | 1.10E − 05 | 3.30E − 01 | 1.23E − 07 | 2.39E − 02 |
Iris | 1.41E − 05 | 5.23E − 07 | 8.29E − 05 | 3.23E − 01 | 3.71E − 05 | 1.01E − 03 |
Statlog | 6.80E − 08 | 6.80E − 08 | 6.80E − 08 | 6.73E − 08 | 6.80E − 08 | 6.80E − 08 |
Cancer | 1.05E − 06 | 2.22E − 07 | 2.06E − 06 | 1.59E − 05 | 9.13E − 07 | 9.75E − 06 |
Diabetes | 6.80E − 08 | 6.80E − 08 | 6.80E − 08 | 6.80E − 08 | 6.80E − 08 | 6.80E − 08 |
Gene | 6.80E − 08 | 6.80E − 08 | 6.80E − 08 | 9.37E − 07 | 6.80E − 08 | 6.92E − 07 |
Parkinson | 5.87E − 06 | 6.80E − 08 | 1.80E − 06 | 2.17E − 04 | 1.06E − 07 | 2.30E − 05 |
Splice | 6.80E − 08 | 6.80E − 08 | 6.80E − 08 | 6.79E − 08 | 6.80E − 08 | 6.80E − 08 |
WDBC | 6.80E − 08 | 6.80E − 08 | 7.90E − 08 | 6.73E − 08 | 6.80E − 08 | 6.80E − 08 |
Zoo | 6.78E − 08 | 6.78E − 08 | 7.93E − 07 | 6.55E − 01 | 6.78E − 08 | 2.61E − 01 |
The blood classification problem is the most commonly used data set in the classification problem. The experimental results of the classification problem of Blood dataset trained by intelligence optimization algorithm are shown in Table 3, and the convergence and the anova graphs of algorithms are displayed in Figures 4 and 5, severally. It was found from Table 3 that the mean value and variance of MSE obtained by the TSWOA algorithm are better than other comparison algorithms, indicating that the optimizing result of the TSWOA algorithm is the best. Experimental results show that the accuracy of the algorithm is 80.39, higher than that of other comparison algorithms, ranking first. As can be found from the convergence curve in Figure 4, the convergence speed of the TSWOA algorithm is faster than that of other algorithms and the accuracy value is better than that of other algorithms. According to Figure 5, compared with other comparison algorithms, TSWOA has a better variance value. According to the p-value test shown in Table 18, the results of TSWOA is obviously different from other comparison algorithms.
The experimental results of 20 independent runs of the algorithm are displayed in Table 4, and the convergence and anova graphs of all algorithms are displayed in Figures 6 and 7, severally. As displayed in Table 4, the mean value of MSE obtained by TSWOA algorithm is the smallest in the data set. But, the accuracy of the TSWOA algorithm and the FPA algorithm in optimizing MLP to forecast Scale data set is 89.67, ranking first, and their classification accuracy is far higher than that of other comparison algorithms. As seen in Figure 6, the TSWOA algorithm has faster convergence speed and higher convergence accuracy than other comparison algorithms. Figure 7 exhibits that TSWOA has a small and stable anova. Table 18 also shows the superiority of TSWOA, which is obviously different from all comparison algorithms in Scale dataset.
Table 5 displays the experimental results of the seven algorithms that optimized MLP to forecast the Survival dataset. The convergence and variance graphs of all algorithms are displayed in Figures 8 and 9, severally. As displayed in Table 5, the mean value of MSE obtained by the TSWOA algorithm is the smallest in the comparison algorithms. But, the accuracy of the TSWOA algorithm optimizing MLP in forecasting the Survival dataset is lower than that of GOA, GSO, SSO, and GA, which ranks third. Among them, the classification accuracy of GOA, GSO and SSO algorithms is 81.73, ranking first. The convergence curve in Figure 8 shows that the TSWOA algorithm has a faster convergence speed and a better convergence accuracy value than other algorithms. In Figure 9, compared with other algorithms, TSWOA has a better mean value. Table 18 displays that the TSWOA algorithm has obvious advantages.
The experimental results of the Liver classification problem are shown in Table 6, and Figures 10 and 11 display the convergence and variance graphs of all algorithms, severally. As displayed from Table 6, the mean value of MSE obtained by the TSWOA algorithm is slightly smaller than the mean value of MSE obtained by GOA and WOA. In the Liver dataset, the accuracy of the TSWOA algorithm was higher than that of other comparison algorithms, ranking first. It can be distinctly displayed from Figure 10 that at the beginning of the iteration, the TSWOA algorithm not only converges faster but also has a better mean value of MSE than other comparison algorithms. As shown in Table 18, the p value of the TSWOA algorithm and other algorithms are all less than 0.05, indicating the excellent performance of the modified algorithm in processing the Liver dataset.
The MSE results obtained by all algorithms listed in Table 7 display that there is little difference between the mean values obtained by the TSWOA algorithm and the FPA algorithm, and the performance of the two algorithms is much better than other algorithms. Meanwhile, the accuracy of both the TSWOA algorithm and the FPA algorithm is 95.77, which is far higher than the accuracy of other comparison algorithms. Figure 12 exhibits that the convergence rate of the TSWOA algorithm is much faster than that of GOA, GSO, SSO, GA, and WOA, but slightly worse than that of FPA. Before 250 iterations, the convergence speed and convergence accuracy of the TSWOA algorithm was much higher than that of other comparison algorithms, but in the later iteration period, the convergence accuracy of the FPA algorithm was slightly higher than that of TSWOA algorithm. Wilcoxson's rank-sum test in Table 18 shows that the value of the TSWOA algorithm and the FPA algorithm are greater than 0.05, but the comparison results of other comparison algorithms are less than 0.05.
The results in Table 8 display that the TSWOA algorithm obtains better results than the WOA algorithm and the other five algorithms. Table 8 shows that, as far as MSE is concerned, the mean value of TSWOA algorithm is the smallest and the classification accuracy of the algorithm is 96.72, which is much higher than that of other comparison algorithms, indicating that TSWOA algorithm can achieve better classification accuracy than other comparison algorithms. Figure 14 exhibits the convergence curve of the average MSE of all the seven algorithms, which distinctly indicates that GOA, GA, and WOA quickly fall into local optima and their convergence speed has stopped when the number of iterations is 300. Meanwhile, about 500 iterations of FPA and TSWOA reached the corresponding minimum value, but the MSE of TSWOA was smaller than that of FPA. According to the test results in Table 18, the results of the TSWOA algorithm are significantly different from that of GOA, GSO, SSO, GA and WOA algorithms.
The experimental results of the Iris classification problem are exhibited in Table 9, and Figures 16 and 17 display the convergence and variance graphs of all algorithms, severally. The mean value of MSE obtained by the algorithm shown in Table 9 exhibits that the value obtained by FPA is superior to that of other comparison algorithms, while the value obtained by TSWOA ranks the second. However, the accuracy obtained by TSWOA and GA is much higher than other algorithms. Figure 16 shows that before the 240 iterations, the convergence speed and convergence accuracy of the TSWOA algorithm is much higher than that of other comparison algorithms. But, after the 240 iterations, the convergence accuracy of FPA is greater than that of TSWOA. Meanwhile, GOA, GA, SSO and WOA algorithms quickly fall into local optimization. As displayed in Figure 16, TSWOA has a better variance value than other algorithms. According to the p-value test in Table 18, the results of TSWOA are obviously differences from those of GOA, GSO, SSO, GA, and WOA.
The results in Table 10 exhibit that, in terms of MSE, the mean value and variance of the TSWOA algorithm are the smallest compared with other comparison algorithms. At the same time, the accuracy in Table 10 indicates that the TSWOA algorithm can achieve a better classification effect than other comparison algorithms. Figure 18 displays the convergence curves of MSE obtained by all the seven algorithms, which clearly shows that GSO, GA, SSO, and WOA quickly fall into local optima and their convergence speed has stopped when the number of iterations is 200. However, the TSWOA algorithm has been in a declining state in the process of 500 iterations. Figure 19 exhibits that the TSWOA algorithm has a relatively stable variance. Table 18 also displays the superiority of TSWOA, which is obviously different from all algorithms.
Figure 20 exhibits the convergence of the seven algorithms. As displayed from Figure 20, compared with other comparison algorithms, TSWOA obtained the minimum mean value. FPA is approximately the same mean value obtained by WOA, while the convergence accuracy and speed of the TSWOA algorithm are better than other algorithms. At the same time, it can also be observed that during the optimization process, GA and GOA obtained the same minimum mean, while GSO performed the worst, stagnating in the early stage. Table 11 shows the comparison of the mean values of different algorithms. It can be clearly found from Table 11 that after 500 iterations, the mean value obtained by TSWOA is the smallest. WOA and FPA obtained the same classification effect and ranked first, while TSWOA, GA, and SSO obtained the same classification effect and ranked second. The results above show that TSWOA provides very competitive results in the classification effect and the p-value in Table 18 further displays that the TSWOA algorithm is significantly superior to all comparison algorithms in the classification effect of the Cancer dataset.
The experimental results of the diabetes classification problem are displayed in Table 12, and Figures 22 and 23 exhibit the convergence and variance of all algorithms, severally. As exhibited from Table 12, as far as MSE is a concern, TSWOA provides the best mean value and the standard deviation of MSE, the result is better than other comparison algorithms, and the accuracy of classification of Diabetes data set is better than that of other comparison algorithms. Figure 22 shows that TSWOA not only has a fast convergence rate but also has a better mean value than other comparison algorithms. In addition, In Figure 23, TSWOA has the smallest variance value. Table 18 also displays the superiority of TSWOA, which is significantly different from all comparison algorithms.
The results in Table 13 display that the mean valve of MSE obtained by the TSWOA algorithm is better than that of the WOA algorithm and the other five algorithms. The accuracy of the modified algorithm is 33.33, which is much higher than that of other algorithms and ranks first, indicating that the training of the Gene dataset with the TSWOA algorithm can achieve better classification accuracy than other comparison algorithms. Figure 24 exhibits the convergence graphs of all the seven algorithms, which distinctly exhibit that the three algorithms, GSO, GOA, and GA, quickly fall into local optima and their convergence speed has stopped in the early stage of the iteration. However, during the optimization process, TSWOA did not stop still and continued to search for the appropriate minimum. Figure 25 also shows that TSWOA has superior performance. Table 18 displays that TSWOA is obviously different from the other six algorithms. In this case, all values are less than 0.05.
The experimental results of Parkinson's classification problem are shown in Table 14, and the convergence and variance of all algorithms are exhibited in Figures 26 and 27. It displayed from Table 14 that the mean value of MSE obtained by the proposed algorithm is the minimum, indicating that the training effect of the improved algorithm is the best. In the test data, the accuracy of the modified algorithm is 74.24, higher than that of other algorithms, ranking first. In Figure 26, the convergence speed of our algorithm is faster than that of other algorithms. It displayed in Figure 27 that TSWOA has a better variance value than other algorithms. Two abnormalities appeared in the experiment, whose average value was less than that of other algorithms. The p-value test results in Table 18 further indicate that the results of TSWOA are obviously superior to all comparison algorithms.
Table 15 displays the experimental results of the splice classification problem, the convergence and variance diagrams of all algorithms are exhibited in Figures 28 and 29, severally. It displayed in Table 15 that the mean value of MSE obtained by the modified algorithm is the minimum. The mean values obtained by GOA and GSO are almost the same. In the Splice dataset, the accuracy of the proposed algorithm is 80, which is higher than the accuracy of other algorithms, so it ranks first. In Figure 28, our algorithm has a great advantage over other algorithms in terms of convergence speed and convergence accuracy. Meanwhile, GSO, GOA, and GA soon fell into local optima. In Figure 29, compared with other algorithms, TSWOA has a better variance value. Experimental results found no singularities, and the mean value of the TSWOA algorithm was lower than that of the other algorithms. In Table 18, p-value test results display that the TSWOA algorithm is superior to all comparison algorithms.
The results in Table 16 show that, as far as MSE is concerned, the mean value and variance of the TSWOA algorithm are the smallest, indicating that the TSWOA algorithm can achieve better classification effect than other comparison algorithms. In the test data, the accuracy of the modified algorithm is 95.15, which is higher than the accuracy of other algorithms, so it ranks first. Figure 30 exhibits that GSO, GA, GOA, and WOA fall into local optimality early in the optimization process, resulting in premature convergence of the algorithm. However, in the optimization process, TSWOA did not stop and continued to search for the corresponding optimal value. All the results exhibit that TSWOA is superior to other algorithms, with the fastest convergence speed and the smallest value. As can be displayed in Figure 31, compared with other algorithms, TSWOA has a better variance value. It can be concluded in Table 18 that TSWOA is obviously different from other comparison algorithms.
The MSE results obtained by the algorithm di splayed in Table 17 exhibit that there is little difference between the mean values obtained by FPA and TSWOA, and the performance of these two algorithms is much better than that of other algorithms. However, the classification accuracy of the modified algorithm is slightly lower than that of WOA in test data. In the Zoo dataset, the accuracy of the modified algorithm is 64.71, which ranks second. The convergence of the seven algorithms is displayed in Figure 32. As can be shown from Figure 32, in the later iteration, TSWOA, WOA and FPA obtained convergence accuracy with little difference. However, GSO and GA tend to fall into local optima in the early stage. As can be seen from Figure 33, compared with other algorithms, TSWOA has a good mean value in training data. The results above exhibit that TSWOA provides very competitive results. It can be concluded from Table 18 that, except for FPA, the values obtained by TSWOA are significantly different from the other five algorithms.
As can be seen from Tables 3–17, except Survival, Cancer, and Zoo, the accuracy of the modified algorithm is the greatest, indicating that the classification failure rate of TSWOA is small in optimizing MLP. In terms of Survival, Cancer, and Zoo, the accuracy of TSWOA is also in the top three. Moreover, the rest are ranked first. From Figures 4–33, it can be seen that the improved algorithm has faster convergence speed, higher accuracy, and stronger stability for optimizing MLP. The reasons are as follows:
For any optimization algorithm, it is important to obtain the balance between its exploration and exploitation. Exploration makes the algorithm to have feasible solutions and exploitation provides search intensity for any optimization problem. As displayed in section 3. It is vital to have the arguments α in the position update of WOA. By changing the value of the arguments α, a better balance can be realized between the global search and local search. However, in the WOA, α is declining linearly from 2 to 0 with consistently of the iterations. At the beginning of the iteration, the arguments α can be a max value that is beneficial to global search, but the algorithm has a poor search efficiency. At the end of the iteration, a small value is acquired, which is beneficial to the convergence of the algorithm, but the algorithm is prone to a local optimum. Based on the problems above, the teaching phase of TLBO is add to the basic whale optimization algorithm and selects the best agent to guide other agents to move towards the current optimal position. On the one hand, we heighten the exploitation capacity of the algorithm and improve the quality of candidate solutions. On the other hand, it evades the stagnation state of the algorithm and decreases the risk of the algorithm running into local optimum. In order to strengthen the exploration capacity of the algorithm, the simplex method is added to the algorithm, which not only increases the variety of the population but also enlarges the search scope of the solution space, guiding the algorithm to find the most promising area of the solution space. Therefore, this modified algorithm can be applied as a new MLP optimizing method.
In this paper, an improved teaching learning-based whale optimization algorithm was presented to optimize MLP. The modified TSWOA algorithm utilizes the teacher phase of TLBO to heighten the exploration and increase the overall quality of candidate solutions. The simplex method is added to TWOA to enlarge the diversification of search agents, which ensures that the algorithm can availably explore the search location, and make the algorithm quickly converge to the global optimal. This method was adopted to optimize connection weights and biases of multi-layer perceptron (MLP) neural network for the classification problems, compared with several recognized meta-heuristic algorithms. The experimental results display that the modified TSWOA is quite effective in optimizing MLP. Future research may apply TSWOA to optimize convolutional neural networks for image processing. Another opportunity is to further heighten the performance of TSWOA to get a better balance between exploration and exploitation and apply it to solve the real problems.
This work is supported by National Science Foundation of China under Grants No. 61563008, and Project of Guangxi Natural Science Foundation under Grant No. 2018GXNSFAA138146.
The authors declare no conflict of interest.
[1] |
A. Khwaja, M. Bjorkholm, R. E. Gale, R. L. Levine, C. T. Jordan, G. Ehninger, et al., Acute myeloid leukaemia, Nat. Rev. Dis. Primers, 2 (2016), 16010. https://doi.org/10.1038/nrdp.2016.10 doi: 10.1038/nrdp.2016.10
![]() |
[2] |
E. Estey, H. Dohner, Acute myeloid leukaemia, Lancet, 368 (2006), 1894-1907. https://doi.org/10.1016/S0140-6736(06)69780-8 doi: 10.1016/S0140-6736(06)69780-8
![]() |
[3] |
L. Bullinger, K. Dohner, E. Bair, S. Frohling, R. F. Schlenk, R. Tibshirani, et al., Use of gene-expression profiling to identify prognostic subclasses in adult acute myeloid leukemia, N. Eng. J. Med., 350 (2004), 1605-1616. https://doi.org/10.1056/NEJMoa031046 doi: 10.1056/NEJMoa031046
![]() |
[4] |
E. Papaemmanuil, M. Gerstung, L. Bullinger, V. I. Gaidzik, P. Paschka, N. D. Roberts, et al., Genomic classification and prognosis in acute myeloid leukemia, N. Eng. J. Med., 374 (2016), 2209-2221. https://doi.org/10.1056/NEJMoa1516192 doi: 10.1056/NEJMoa1516192
![]() |
[5] |
C. C. Coombs, M. S. Tallman, R. L. Levine, Molecular therapy for acute myeloid leukaemia, Nat. Rev. Clin. Oncol., 13 (2016), 305-318. https://doi.org/10.1038/nrclinonc.2015.210 doi: 10.1038/nrclinonc.2015.210
![]() |
[6] |
J. W. Tyner, C. E. Tognon, D. Bottomly, B. Wilmot, S. E. Kurtz, S. L. Savage, et al., Functional genomic landscape of acute myeloid leukaemia, Nature, 562 (2018), 526-531. https://doi.org/10.1038/s41586-018-0623-z doi: 10.1038/s41586-018-0623-z
![]() |
[7] |
S. Abelson, G. Collord, S. W. K. Ng, O. Weissbrod, N. M. Cohen, E. Niemeyer, et al., Prediction of acute myeloid leukaemia risk in healthy individuals, Nature, 559 (2018), 400-404. https://doi.org/10.1038/s41586-018-0317-6 doi: 10.1038/s41586-018-0317-6
![]() |
[8] |
S. C. Meyer, R. L. Levine, Translational implications of somatic genomics in acute myeloid leukaemia, Lancet Oncol., 15 (2014), e382-394. https://doi.org/10.1016/S1470-2045(14)70008-7 doi: 10.1016/S1470-2045(14)70008-7
![]() |
[9] |
T. Bratkovic, J. Bozic, B. Rogelj, Functional diversity of small nucleolar RNAs, Nucleic Acids Res., 48 (2020), 1627-1651. https://doi.org/10.1093/nar/gkz1140 doi: 10.1093/nar/gkz1140
![]() |
[10] |
J. Ni, A. L. Tien, M. J. Fournier, Small nucleolar RNAs direct site-specific synthesis of pseudouridine in ribosomal RNA, Cell, 89 (1997), 565-573. https://doi.org/10.1016/s0092-8674(00)80238-x doi: 10.1016/s0092-8674(00)80238-x
![]() |
[11] |
V. Chikne, K. S. Rajan, M. Shalev-Benami, K. Decker, S. Cohen-Chalamish, H. Madmoni, et al., Small nucleolar RNAs controlling rRNA processing in Trypanosoma brucei, Nucleic Acids Res., 47 (2019), 2609-2629. https://doi.org/10.1093/nar/gky1287 doi: 10.1093/nar/gky1287
![]() |
[12] |
L. Xing, X. Zhang, X. Zhang, D. Tong, Expression scoring of a small-nucleolar-RNA signature identified by machine learning serves as a prognostic predictor for head and neck cancer, J. Cell Phys., 235 (2020), 8071-8084. https://doi.org/10.1002/jcp.29462 doi: 10.1002/jcp.29462
![]() |
[13] |
Y. Zhao, Y. Yan, R. Ma, X. Lv, L. Zhang, J. Wang, et al., Expression signature of six-snoRNA serves as novel non-invasive biomarker for diagnosis and prognosis prediction of renal clear cell carcinoma, J. Cell Mol. Med., 24 (2020), 2215-2228. https://doi.org/10.1111/jcmm.14886 doi: 10.1111/jcmm.14886
![]() |
[14] | L. Huang, X. Z. Liang, Y. Deng, Y. B. Liang, X. Zhu, X. Y. Liang, et al., Prognostic value of small nucleolar RNAs (snoRNAs) for colon adenocarcinoma based on RNA sequencing data, Pathol. Res. Pract., 216 (2020), 152937. https: //doi.org/10.1016/j.prp.2020.152937 |
[15] |
J. Gong, Y. Li, C. J. Liu, Y. Xiang, C. Li, Y. Ye, et al., A pan-cancer analysis of the expression and clinical relevance of small nucleolar RNAs in human cancer, Cell Rep., 21 (2017), 1968-1981. https://doi.org/10.1016/j.celrep.2017.10.070 doi: 10.1016/j.celrep.2017.10.070
![]() |
[16] | The Cancer Genome Atlas Research Network, Genomic and epigenomic landscapes of adult de novo acute myeloid leukemia, N. Eng. J. Med., 368 (2013), 2059-2074. https://doi.org/10.1056/NEJMoa1301689 |
[17] |
M. D. Robinson, D. J. McCarthy, G. K. Smyth, edgeR: a Bioconductor package for differential expression analysis of digital gene expression data, Bioinformatics, 26 (2010), 139-140. https://doi.org/10.1093/bioinformatics/btp616 doi: 10.1093/bioinformatics/btp616
![]() |
[18] |
R. Huang, X. Liao, Q. Li, Identification and validation of potential prognostic gene biomarkers for predicting survival in patients with acute myeloid leukemia, Oncol. Targets Ther., 10 (2017), 5243-5254. https://doi.org/10.2147/OTT.S147717 doi: 10.2147/OTT.S147717
![]() |
[19] |
X. Liao, X. Wang, K. Huang, C. Yang, T. Yu, C. Han, et al., Genome-scale analysis to identify prognostic microRNA biomarkers in patients with early stage pancreatic ductal adenocarcinoma after pancreaticoduodenectomy, Cancer Manage. Res., 10 (2018), 2537-2551. https://doi.org/10.2147/CMAR.S168351 doi: 10.2147/CMAR.S168351
![]() |
[20] |
X. Liao, X. Wang, K. Huang, C. Han, J. Deng, T. Yu, et al., Integrated analysis of competing endogenous RNA network revealing potential prognostic biomarkers of hepatocellular carcinoma, J. Cancer, 10 (2019), 3267-3283. https://doi.org/10.7150/jca.29986 doi: 10.7150/jca.29986
![]() |
[21] |
W. H. Da, B. T. Sherman, R. A. Lempicki, Systematic and integrative analysis of large gene lists using DAVID bioinformatics resources, Nat. Protoc., 4 (2009), 44-57. https://doi.org/10.1038/nprot.2008.211 doi: 10.1038/nprot.2008.211
![]() |
[22] |
V. K. Mootha, C. M. Lindgren, K. F. Eriksson, A. Subramanian, S. Sihag, J. Lehar, et al., PGC-1alpha-responsive genes involved in oxidative phosphorylation are coordinately downregulated in human diabetes, Nat. Genet., 34 (2003), 267-273. https://doi.org/10.1038/ng1180 doi: 10.1038/ng1180
![]() |
[23] |
A. Subramanian, P. Tamayo, V. K. Mootha, S. Mukherjee, B. L. Ebert, M. A. Gillette, et al., Gene set enrichment analysis: a knowledge-based approach for interpreting genome-wide expression profiles, Proc. Nat. Acad. Sci. U. S. A., 102 (2005), 15545-15550. https://doi.org/10.1073/pnas.0506580102 doi: 10.1073/pnas.0506580102
![]() |
[24] |
A. Liberzon, C. Birger, H. Thorvaldsdottir, M. Ghandi, J. P. Mesirov, P. Tamayo, The molecular signatures database (MSigDB) hallmark gene set collection, Cell Syst., 1 (2015), 417-425. https://doi.org/10.1016/j.cels.2015.12.004 doi: 10.1016/j.cels.2015.12.004
![]() |
[25] |
A. Liberzon, A. Subramanian, R. Pinchback, H. Thorvaldsdottir, P. Tamayo, J. P. Mesirov, Molecular signatures database (MSigDB) 3.0, Bioinformatics, 27 (2011), 1739-1740. https://doi.org/10.1093/bioinformatics/btr260 doi: 10.1093/bioinformatics/btr260
![]() |
[26] |
J. Lamb, The connectivity map: a new tool for biomedical research, Nat. Rev. Cancer, 7 (2007), 54-60. https://doi.org/10.1038/nrc2044 doi: 10.1038/nrc2044
![]() |
[27] |
J. Lamb, E. D. Crawford, D. Peck, J. W. Modell, I. C. Blat, M. J. Wrobel, et al., The connectivity map: using gene-expression signatures to connect small molecules, genes, and disease, Science, 313 (2006), 1929-1935. https://doi.org/10.1126/science.1132939 doi: 10.1126/science.1132939
![]() |
[28] | E. W. Sayers, J. Beck, J. R. Brister, E. E. Bolton, K. Canese, D. C. Comeau, et al., Database resources of the national center for biotechnology information, Nucleic Acids Res., 48 (2020), D9-D16. https://doi.org/10.1093/nar/gkz899 |
[29] | S. Kim, J. Chen, T. Cheng, A. Gindulyte, J. He, S. He, et al., PubChem 2019 update: improved access to chemical data, Nucleic Acids Res., 47 (2019), D1102-D1109. https://doi.org/10.1093/nar/gky1033 |
[30] | M. Kuhn, D. Szklarczyk, A. Franceschini, M. Campillos, C. V. Mering, L. J. Jensen, et al., STITCH 2: an interaction network database for small molecules and proteins, Nucleic Acids Res., 38 (2010), D552-556. https://doi.org/10.1093/nar/gkp937 |
[31] | M. Kuhn, C. V. Mering, M. Campillos, L. J. Jensen, P. Bork, STITCH: interaction networks of chemicals and proteins, Nucleic Acids Res., 36 (2008), D684-688. https://doi.org/10.1093/nar/gkm795 |
[32] | D. Szklarczyk, A. Santos, C. V. Mering, L. J. Jensen, P. Bork, M. Kuhn, STITCH 5: augmenting protein-chemical interaction networks with tissue and affinity data, Nucleic Acids Res., 44 (2016), D380-384. https://doi.org/10.1093/nar/gkv1277 |
[33] | K. Yoshihara, M. Shahmoradgoli, E. Martinez, R. Vegesna, H. Kim, W. Torres-Garcia, et al., Inferring tumour purity and stromal and immune cell admixture from expression data, Nat. Commun., 4 (2013), 2612. https://doi.org/10.1038/ncomms3612 |
[34] |
B. Chen, M. S. Khodadoust, C. L. Liu, A. M. Newman, A. A. Alizadeh, Profiling tumor infiltrating immune cells with CIBERSORT, Methods Mol. Biol., 1711 (2018), 243-259. https://doi.org/10.1007/978-1-4939-7493-1_12 doi: 10.1007/978-1-4939-7493-1_12
![]() |
[35] |
Y. Benjamini, D. Drai, G. Elmer, N. Kafkafi, I. Golani, Controlling the false discovery rate in behavior genetics research, Behav. Brain Res., 125 (2001), 279-284. https://doi.org/10.1016/s0166-4328(01)00297-2 doi: 10.1016/s0166-4328(01)00297-2
![]() |
[36] |
P. Shannon, A. Markiel, O. Ozier, N. S. Baliga, J. T. Wang, D. Ramage, et al., Cytoscape: a software environment for integrated models of biomolecular interaction networks, Genome Res., 13 (2003), 2498-2504. https://doi.org/10.1101/gr.1239303 doi: 10.1101/gr.1239303
![]() |
[37] | D. Otasek, J. H. Morris, J. Boucas, A. R. Pico, B. Demchak, Cytoscape automation: empowering workflow-based network analysis, Genome Biol., 20 (2019), 185. https://doi.org/10.1186/s13059-019-1758-4 |
[38] | D. Ronchetti, L. Mosca, G. Cutrona, G. Tuana, M. Gentile, S. Fabris, et al., Small nucleolar RNAs as new biomarkers in chronic lymphocytic leukemia, BMC Med. Genomics, 6 (2013), 27. https://doi.org/10.1186/1755-8794-6-27 |
[39] |
E. Bignotti, S. Calza, R. A. Tassi, L. Zanotti, E. Bandiera, E. Sartori, et al., Identification of stably expressed reference small non-coding RNAs for microRNA quantification in high-grade serous ovarian carcinoma tissues, J. Cell Mol. Med., 20 (2016), 2341-2348. https://doi.org/10.1111/jcmm.12927 doi: 10.1111/jcmm.12927
![]() |
[40] |
L. H. Mao, S. Y. Chen, X. Q. Li, F. Xu, J. Lei, Q. L. Wang, et al., LncRNA-LALR1 upregulates small nucleolar RNA SNORD72 to promote growth and invasion of hepatocellular carcinoma, Aging (Albany NY), 12 (2020), 4527-4546. https://doi.org/10.18632/aging.102907 doi: 10.18632/aging.102907
![]() |
[41] |
F. G. Lafaille, O. Harschnitz, Y. S. Lee, P. Zhang, M. L. Hasek, G. Kerner, et al., Human SNORA31 variations impair cortical neuron-intrinsic immunity to HSV-1 and underlie herpes simplex encephalitis, Nat. Med., 25 (2019), 1873-1884. https://doi.org/10.1038/s41591-019-0672-3 doi: 10.1038/s41591-019-0672-3
![]() |
[42] |
H. Davanian, A. Balasiddaiah, R. Heymann, M. Sundstrom, P. Redenstrom, M. Silfverberg, et al., Ameloblastoma RNA profiling uncovers a distinct non-coding RNA signature, Oncotarget, 8 (2017), 4530-4542. https://doi.org/10.18632/oncotarget.13889 doi: 10.18632/oncotarget.13889
![]() |
[43] | I. Nepstad, K. J. Hatfield, I. S. Gronningsaeter, H. Reikvam, The PI3K-Akt-mTOR signaling pathway in human acute myeloid leukemia (AML) cells, Int. J. Mol. Sci., 21 (2020), 2907. https://doi.org/10.3390/ijms21082907 |
[44] |
L. Herschbein, J. L. Liesveld, Dueling for dual inhibition: Means to enhance effectiveness of PI3K/Akt/mTOR inhibitors in AML, Blood Rev., 32 (2018), 235-248. https://doi.org/10.1016/j.blre.2017.11.006 doi: 10.1016/j.blre.2017.11.006
![]() |
[45] |
J. Bertacchini, N. Heidari, L. Mediani, S. Capitani, M. Shahjahani, A. Ahmadzadeh, et al., Targeting PI3K/AKT/mTOR network for treatment of leukemia, Cell Mol. Life Sci., 72 (2015), 2337-2347. https://doi.org/10.1007/s00018-015-1867-5 doi: 10.1007/s00018-015-1867-5
![]() |
[46] |
Y. Su, X. Li, J. Ma, J. Zhao, S. Liu, G. Wang, et al., Targeting PI3K, mTOR, ERK and Bcl-2 signaling network shows superior antileukemic activity against AML ex vivo, Biochem. Pharmacol., 148 (2018), 13-26. https://doi.org/10.1016/j.bcp.2017.11.022 doi: 10.1016/j.bcp.2017.11.022
![]() |
[47] |
Y. Tabe, A. Tafuri, K. Sekihara, H. Yang, M. Konopleva, Inhibition of mTOR kinase as a therapeutic target for acute myeloid leukemia, Expert Opin. Ther. Targets, 21 (2017), 705-714. https://doi.org/10.1080/14728222.2017.1333600 doi: 10.1080/14728222.2017.1333600
![]() |
[48] |
N. Guo, M. Azadniv, M. Coppage, M. Nemer, J. Mendler, M. Becker, et al., Effects of neddylation and mTOR inhibition in acute myelogenous leukemia, Transl. Oncol., 12 (2019), 602-613. https://doi.org/10.1016/j.tranon.2019.01.001 doi: 10.1016/j.tranon.2019.01.001
![]() |
[49] |
J. Wu, G. Hu, Y. Dong, R. Ma, Z. Yu, S. Jiang, et al., Matrine induces Akt/mTOR signalling inhibition-mediated autophagy and apoptosis in acute myeloid leukaemia cells, J. Cell Mol. Med., 21 (2017), 1171-1181. https://doi.org/10.1111/jcmm.13049 doi: 10.1111/jcmm.13049
![]() |
[50] | Y. Feng, L. Wu, mTOR up-regulation of PFKFB3 is essential for acute myeloid leukemia cell survival, Biochem. Biophys. Res. Commun., 483 (2017), 897-903. |
[51] |
J. Bertacchini, C. Frasson, F. Chiarini, D. D'Avella, B. Accordi, L. Anselmi, et al., Dual inhibition of PI3K/mTOR signaling in chemoresistant AML primary cells, Adv. Biol. Regul., 68 (2018), 2-9. https://doi.org/10.1016/j.jbior.2018.03.001 doi: 10.1016/j.jbior.2018.03.001
![]() |
[52] |
V. Stavropoulou, S. Kaspar, L. Brault, M. A. Sanders, S. Juge, S. Morettini, et al., MLL-AF9 expression in hematopoietic stem cells drives a highly invasive AML expressing EMT-related genes linked to poor outcome, Cancer Cell, 30 (2016), 43-58. https://doi.org/10.1016/j.ccell.2016.05.011 doi: 10.1016/j.ccell.2016.05.011
![]() |
[53] |
T. J. Zhang, J. D. Zhou, J. C. Ma, Z. Q. Deng, Z. Qian, D. M. Yao, et al., CDH1 (E-cadherin) expression independently affects clinical outcome in acute myeloid leukemia with normal cytogenetics, Clin. Chem. Lab. Med., 55 (2017), 123-131. https://doi.org/10.1515/cclm-2016-0205 doi: 10.1515/cclm-2016-0205
![]() |
[54] | S. Wu, Y. Du, J. Beckford, H. Alachkar, Upregulation of the EMT marker vimentin is associated with poor clinical outcome in acute myeloid leukemia, J. Transl. Med., 16 (2018), 170. https://doi.org/10.1186/s12967-018-1539-y |
[55] |
L. Zhong, J. Chen, X. Huang, Y. Li, T. Jiang, Monitoring immunoglobulin heavy chain and T-cell receptor gene rearrangement in cfDNA as minimal residual disease detection for patients with acute myeloid leukemia, Oncol. Lett., 16 (2018), 2279-2288. https://doi.org/10.3892/ol.2018.8966 doi: 10.3892/ol.2018.8966
![]() |
[56] |
A. G. Chapuis, D. N. Egan, M. Bar, T. M. Schmitt, M. S. McAfee, K. G. Paulson, et al., T cell receptor gene therapy targeting WT1 prevents acute myeloid leukemia relapse post-transplant, Nat. Med., 25 (2019), 1064-1072. https://doi.org/10.1038/s41591-019-0472-9 doi: 10.1038/s41591-019-0472-9
![]() |
[57] |
H. J. Stauss, S. Thomas, M. Cesco-Gaspere, D. P. Hart, S. A. Xue, A. Holler, et al., WT1-specific T cell receptor gene therapy: improving TCR function in transduced T cells, Blood Cells Mol. Dis., 40 (2008), 113-116. https://doi.org/10.1016/j.bcmd.2007.06.018 doi: 10.1016/j.bcmd.2007.06.018
![]() |
[58] |
Y. Wang, A. V. Krivtsov, A. U. Sinha, T. E. North, W. Goessling, Z. Feng, et al., The Wnt/beta-catenin pathway is required for the development of leukemia stem cells in AML, Science, 327 (2010), 1650-1653. https://doi.org/10.1126/science.1186624 doi: 10.1126/science.1186624
![]() |
[59] | A. M. Gruszka, D. Valli, M. Alcalay, Wnt signalling in acute myeloid leukaemia, Cells, 8 (2019), 1403. https://doi.org/10.3390/cells8111403 |
[60] | F. J. Staal, F. Famili, L. G. Perez, K. Pike-Overzet, Aberrant Wnt signaling in leukemia, Cancers (Basel), 8 (2016), 78. https://doi.org/10.3390/cancers8090078 |
[61] |
A. Valencia, J. Roman-Gomez, J. Cervera, E. Such, E. Barragan, P. Bolufer, et al., Wnt signaling pathway is epigenetically regulated by methylation of Wnt antagonists in acute myeloid leukemia, Leukemia, 23 (2009), 1658-1666. https://doi.org/10.1038/leu.2009.86 doi: 10.1038/leu.2009.86
![]() |
[62] |
E. A. Griffiths, S. D. Gore, C. Hooker, M. A. McDevitt, J. E. Karp, B. D. Smith, et al., Acute myeloid leukemia is characterized by Wnt pathway inhibitor promoter hypermethylation, Leuk. Lymphoma, 51 (2010), 1711-1719. https://doi.org/10.3109/10428194.2010.496505 doi: 10.3109/10428194.2010.496505
![]() |
[63] |
C. Gasparini, C. Celeghini, L. Monasta, G. Zauli, NF-kappaB pathways in hematological malignancies, Cell Mol. Life Sci., 71 (2014), 2083-2102. https://doi.org/10.1007/s00018-013-1545-4 doi: 10.1007/s00018-013-1545-4
![]() |
[64] |
M. Breccia, G. Alimena, NF-kappaB as a potential therapeutic target in myelodysplastic syndromes and acute myeloid leukemia, Expert Opin. Ther. Targets, 14 (2010), 1157-1176. https://doi.org/10.1517/14728222.2010.522570 doi: 10.1517/14728222.2010.522570
![]() |
[65] |
M. C. Bosman, J. J. Schuringa, E. Vellenga, Constitutive NF-kappaB activation in AML: Causes and treatment strategies, Crit. Rev. Oncol. Hematol., 98 (2016), 35-44. https://doi.org/10.1016/j.critrevonc.2015.10.001 doi: 10.1016/j.critrevonc.2015.10.001
![]() |
[66] |
J. Zhou, Y. Q. Ching, W. J. Chng, Aberrant nuclear factor-kappa B activity in acute myeloid leukemia: from molecular pathogenesis to therapeutic target, Oncotarget, 6 (2015), 5490-5500. https://doi.org/10.18632/oncotarget.3545 doi: 10.18632/oncotarget.3545
![]() |
[67] |
C. H. Choi, H. Xu, H. Bark, T. B. Lee, J. Yun, S. I. Kang, et al., Balance of NF-kappaB and p38 MAPK is a determinant of radiosensitivity of the AML-2 and its doxorubicin-resistant cell lines, Leuk. Res., 31 (2007), 1267-1276. https://doi.org/10.1016/j.leukres.2006.11.006 doi: 10.1016/j.leukres.2006.11.006
![]() |
[68] |
A. Volk, J. Li, J. Xin, D. You, J. Zhang, X. Liu, et al., Co-inhibition of NF-kappaB and JNK is synergistic in TNF-expressing human AML, J. Exp. Med., 211 (2014), 1093-1108. https://doi.org/10.1084/jem.20130990 doi: 10.1084/jem.20130990
![]() |
[69] |
M. C. Bosman, H. Schepers, J. Jaques, A. Z. Brouwers-Vos, W. J. Quax, J. J. Schuringa, et al., The TAK1-NF-kappaB axis as therapeutic target for AML, Blood, 124 (2014), 3130-3140. https://doi.org/10.1182/blood-2014-04-569780 doi: 10.1182/blood-2014-04-569780
![]() |
[70] | M. Ma, X. Wang, N. Liu, F. Shan, Y. Feng, Low-dose naltrexone inhibits colorectal cancer progression and promotes apoptosis by increasing M1-type macrophages and activating the Bax/Bcl-2/caspase-3/PARP pathway, Int. Immunopharmacol., 83 (2020), 106388. https://doi.org/10.1016/j.intimp.2020.106388 |
[71] | N. Liu, M. Ma, N. Qu, R. Wang, H. Chen, F. Hu, et al., Low-dose naltrexone inhibits the epithelial-mesenchymal transition of cervical cancer cells in vitro and effects indirectly on tumor-associated macrophages in vivo, Int. Immunopharmacol., 86 (2020), 106718. https://doi.org/10.1016/j.intimp.2020.106718 |
[72] |
A. C. Menezes, M. Carvalheiro, J. M. P. F. de Oliveira, A. Ascenso, H. Oliveira, Cytotoxic effect of the serotonergic drug 1-(1-Naphthyl)piperazine against melanoma cells, Toxicol. Int. Vitro, 47 (2018), 72-78. https://doi.org/10.1016/j.tiv.2017.11.011 doi: 10.1016/j.tiv.2017.11.011
![]() |
[73] | G. G. Wei, L. Gao, Z. Y. Tang, P. Lin, L. B. Liang, J. J. Zeng, et al., Drug repositioning in head and neck squamous cell carcinoma: An integrated pathway analysis based on connectivity map and differential gene expression, Pathol. Res. Pract., 215 (2019), 152378. https://doi.org/10.1016/j.prp.2019.03.007 |
[74] |
J. Takezawa, Y. Ishimi, K. Yamada, Proteasome inhibitors remarkably prevent translesion replication in cancer cells but not normal cells, Cancer Sci., 99 (2008), 863-871. https://doi.org/10.1111/j.1349-7006.2008.00764.x doi: 10.1111/j.1349-7006.2008.00764.x
![]() |
[75] |
P. G. Richardson, C. Mitsiades, T. Hideshima, K. C. Anderson, Bortezomib: proteasome inhibition as an effective anticancer therapy, Annu. Rev. Med., 57 (2006), 33-47. https://doi.org/10.1146/annurev.med.57.042905.122625 doi: 10.1146/annurev.med.57.042905.122625
![]() |
[76] |
I. Zavrski, C. Naujokat, K. Niemoller, C. Jakob, U. Heider, C. Langelotz, et al., Proteasome inhibitors induce growth inhibition and apoptosis in myeloma cell lines and in human bone marrow myeloma cells irrespective of chromosome 13 deletion, J. Cancer Res. Clin. Oncol., 129 (2003), 383-391. https://doi.org/10.1007/s00432-003-0454-6 doi: 10.1007/s00432-003-0454-6
![]() |
[77] | W. X. Wang, B. H. Kong, P. Li, K. Song, X. Qu, B. X. Cui, et al., Effect of extracellular signal regulated kinase signal pathway on apoptosis induced by MG262 in ovarian cancer cells, Zhonghua Fu Chan Ke Za Zhi, 43 (2008), 690-694 |
[78] |
J. Y. Wu, S. S. Lin, F. T. Hsu, J. G. Chung, Fluoxetine inhibits DNA repair and NF-kB-modulated metastatic potential in non-small cell lung cancer, Anticancer Res., 38 (2018), 5201-5210. https://doi.org/10.21873/anticanres.12843 doi: 10.21873/anticanres.12843
![]() |
[79] | L. C. Hsu, H. F. Tu, F. T. Hsu, P. F. Yueh, I. T. Chiang, Beneficial effect of fluoxetine on anti-tumor progression on hepatocellular carcinoma and non-small cell lung cancer bearing animal model, Biomed. Pharmacother., 126 (2020), 110054. https://doi.org/10.1016/j.biopha.2020.110054 |
[80] | A. R. Mun, S. J. Lee, G. B. Kim, H. S. Kang, J. S. Kim, S. J. Kim, Fluoxetine-induced apoptosis in hepatocellular carcinoma cells, Anticancer Res., 33 (2013), 3691-3697 |
[81] | D. Sun, L. Zhu, Y. Zhao, Y. Jiang, L. Chen, Y. Yu, et al., Fluoxetine induces autophagic cell death via eEF2K-AMPK-mTOR-ULK complex axis in triple negative breast cancer, Cell Prolif., 51 (2018), e12402. https://doi.org/10.1111/cpr.12402 |
[82] |
A. M. Kabel, A. A. Elkhoely, Ameliorative potential of fluoxetine/raloxifene combination on experimentally induced breast cancer, Tissue Cell, 48 (2016), 89-95. https://doi.org/10.1016/j.tice.2016.02.002 doi: 10.1016/j.tice.2016.02.002
![]() |
[83] |
M. Bowie, P. Pilie, J. Wulfkuhle, S. Lem, A. Hoffman, S. Desai, et al., Fluoxetine induces cytotoxic endoplasmic reticulum stress and autophagy in triple negative breast cancer, World J. Clin. Oncol., 6 (2015), 299-311. https://doi.org/10.5306/wjco.v6.i6.299 doi: 10.5306/wjco.v6.i6.299
![]() |
[84] |
T. M. Khing, W. W. Po, U. D. Sohn, Fluoxetine enhances anti-tumor activity of paclitaxel in gastric adenocarcinoma cells by triggering apoptosis and necroptosis, Anticancer Res., 39 (2019), 6155-6163. https://doi.org/10.21873/anticanres.13823 doi: 10.21873/anticanres.13823
![]() |
[85] |
P. P. Khin, W. W. Po, W. Thein, U. D. Sohn, Apoptotic effect of fluoxetine through the endoplasmic reticulum stress pathway in the human gastric cancer cell line AGS, Naunyn Schmiedebergs Arch. Pharmacol., 393 (2020), 537-549. https://doi.org/10.1007/s00210-019-01739-7 doi: 10.1007/s00210-019-01739-7
![]() |
[86] | M. Marcinkute, S. Afshinjavid, A. A. Fatokun, F. A. Javid, Fluoxetine selectively induces p53-independent apoptosis in human colorectal cancer cells, Eur. J. Pharmacol., 857 (2019), 172441. https://doi.org/10.1016/j.ejphar.2019.172441 |
[87] |
V. Kannen, S. B. Garcia, W. A. Silva, M. Gasser, R. Monch, E. J. Alho, et al., Oncostatic effects of fluoxetine in experimental colon cancer models, Cell Signal, 27 (2015), 1781-1788. https://doi.org/10.1016/j.cellsig.2015.05.008 doi: 10.1016/j.cellsig.2015.05.008
![]() |
[88] | V. Kannen, H. Hintzsche, D. L. Zanette, W. A. Silva, S. B. Garcia, A. M. Waaga-Gasser, et al., Antiproliferative effects of fluoxetine on colon cancer cells and in a colonic carcinogen mouse model, PLoS One, 7 (2012), e50043. https://doi.org/10.1371/journal.pone.0050043 |
[89] |
H. Stopper, S. B. Garcia, A. M. Waaga-Gasser, V. Kannen, Antidepressant fluoxetine and its potential against colon tumors, World J. Gastrointest. Oncol., 6 (2014), 11-21. https://doi.org/10.4251/wjgo.v6.i1.11 doi: 10.4251/wjgo.v6.i1.11
![]() |
[90] | S. J. Koh, J. M. Kim, I. K. Kim, N. Kim, H. C. Jung, I. S. Song, et al., Fluoxetine inhibits NF-kappaB signaling in intestinal epithelial cells and ameliorates experimental colitis and colitis-associated colon cancer in mice, Am. J. Physiol. Gastrointest. Liver Phys., 301 (2011), G9-19. https://doi.org/10.1152/ajpgi.00267.2010 |
[91] |
K. H. Liu, S. T. Yang, Y. K. Lin, J. W. Lin, Y. H. Lee, J. Y. Wang, et al., Fluoxetine, an antidepressant, suppresses glioblastoma by evoking AMPAR-mediated calcium-dependent apoptosis, Oncotarget, 6 (2015), 5088-5101. https://doi.org/10.18632/oncotarget.3243 doi: 10.18632/oncotarget.3243
![]() |
[92] |
J. Ma, Y. R. Yang, W. Chen, M. H. Chen, H. Wang, X. D. Wang, et al., Fluoxetine synergizes with temozolomide to induce the CHOP-dependent endoplasmic reticulum stress-related apoptosis pathway in glioma cells, Oncol. Rep., 36 (2016), 676-684. https://doi.org/10.3892/or.2016.4860 doi: 10.3892/or.2016.4860
![]() |
![]() |
![]() |
1. | Xiaojia Ye, Wei Liu, Hong Li, Mingjing Wang, Chen Chi, Guoxi Liang, Huiling Chen, Hailong Huang, Ramon Costa-Castelló, Modified Whale Optimization Algorithm for Solar Cell and PV Module Parameter Identification, 2021, 2021, 1099-0526, 1, 10.1155/2021/8878686 | |
2. | Naser Arya Azar, Nazila Kardan, Sami Ghordoyee Milan, Developing the artificial neural network–evolutionary algorithms hybrid models (ANN–EA) to predict the daily evaporation from dam reservoirs, 2021, 0177-0667, 10.1007/s00366-021-01523-3 | |
3. | Reenadevi Rajendran, Sathiyabhama Balasubramaniam, Vinayakumar Ravi, Sankar Sennan, Hybrid optimization algorithm based feature selection for mammogram images and detecting the breast mass using multilayer perceptron classifier, 2022, 38, 0824-7935, 1559, 10.1111/coin.12522 | |
4. | Muhammad Zaiyad Muda, Adrian R. Solis, George Panoutsos, An evolving feature weighting framework for radial basis function neural network models, 2022, 0266-4720, 10.1111/exsy.13201 | |
5. | Ying Xu, Xiaobo Li, Qian Li, A discrete teaching–learning based optimization algorithm with local search for rescue task allocation and scheduling, 2023, 134, 15684946, 109980, 10.1016/j.asoc.2022.109980 | |
6. | Xiaodi Hu, David Sturdivant, Mohammad Farukh Hashmi, Dynamical Alert of Thought and Politics Teaching Based on the Long- and Short-Term Memory Neural Network, 2022, 2022, 1530-8677, 1, 10.1155/2022/7465860 | |
7. | Hue Yee Chong, Hwa Jen Yap, Shing Chiang Tan, Keem Siah Yap, Shen Yuong Wong, Advances of metaheuristic algorithms in training neural networks for industrial applications, 2021, 25, 1432-7643, 11209, 10.1007/s00500-021-05886-z | |
8. | Purnawarman Musa, Eri Prasetyo Wibowo, Matrissya Hermita, Raihan Firas Muzhaffar, 2022, Classify Malaria Dataset Human Blood Images Using Convolutional Neural Networks, 978-1-6654-5395-0, 1, 10.1109/ICORIS56080.2022.10031575 | |
9. | Yu Li, Peihua Liu, Peican Zhu, Artificial Intelligence-Based Real-Time Signal Sample and Analysis of Multiperson Dragon Boat Race in Complex Networks, 2022, 2022, 1099-0526, 1, 10.1155/2022/4915973 | |
10. | Cao Haiou, Cui Yu, Song Liangliang, Du Yunlong, Yi Xin, Huang Xiang, 2022, Research on Intelligent Verification Technology of Bus Protection SCD File in Intelligent Substation Reconstruction and Expansion Project Based on Convolutional Neural Network, 978-1-6654-5066-9, 1924, 10.1109/ICPSAsia55496.2022.9949813 | |
11. | Jie Wu, Huihua Chen, A Product Styling Design Evaluation Method Based on Multilayer Perceptron Genetic Algorithm Neural Network Algorithm, 2021, 2021, 1687-5273, 1, 10.1155/2021/2861292 | |
12. | Fuli Bao, Chong Wang, Pan Zheng, A Method for Evaluating the Quality of Mathematics Education Based on Artificial Neural Network, 2022, 2022, 1748-6718, 1, 10.1155/2022/6976654 | |
13. | Ying Xu, XIAOBO LI, QIAN LI, A Discrete Teaching–Learning Based Optimization Algorithm with Local Search for Rescue Task Allocation and Scheduling, 2022, 1556-5068, 10.2139/ssrn.4061447 | |
14. | Zhi Wang, Yayun Li, Lei Wu, Qiang Guo, A Nonlinear Adaptive Weight-Based Mutated Whale Optimization Algorithm and Its Application for Solving Engineering Problems, 2024, 12, 2169-3536, 40225, 10.1109/ACCESS.2024.3350336 | |
15. | Rohit Salgotra, Nitin Mittal, Vikas Mittal, A New Parallel Cuckoo Flower Search Algorithm for Training Multi-Layer Perceptron, 2023, 11, 2227-7390, 3080, 10.3390/math11143080 | |
16. | Yingwen Wu, Zetong Li, Dafa Zhao, Tian Luan, Xutao Yu, Zaichen Zhang, 2024, Data Driven Non-Markovian Quantum Process, 979-8-3503-7352-3, 54, 10.1109/ICTC61510.2024.10602090 | |
17. | Paras Jain, Garima Sharma, Himanshu Jindal, Monika Bharti, Vishan Kumar Gupta, Mukesh Kumar Singh, 2024, Identification and Prediction of Sugarcane Diseases using Convolution Neural Network, 979-8-3503-7651-7, 1, 10.1109/ICICEC62498.2024.10808872 | |
18. | Vishan Kumar Gupta, Garima Sharma, Mukesh Kumar Singh, 2024, A Deep Learning Based Approach for Sugarcane Disease Detection, 979-8-3315-1859-2, 1, 10.1109/DELCON64804.2024.10866920 |
Datasets | Attribute | Class | Training | Testing | Input | Hidden | Output |
Blood | 4 | 2 | 493 | 255 | 4 | 9 | 2 |
Scale | 4 | 3 | 412 | 213 | 4 | 9 | 3 |
Survival | 3 | 2 | 202 | 104 | 3 | 7 | 2 |
Liver | 6 | 2 | 227 | 118 | 6 | 13 | 2 |
Seeds | 7 | 3 | 139 | 71 | 7 | 15 | 3 |
Wine | 13 | 3 | 117 | 61 | 13 | 27 | 3 |
Iris | 4 | 3 | 99 | 51 | 4 | 9 | 3 |
Statlog | 13 | 2 | 178 | 92 | 13 | 27 | 2 |
Cancer | 9 | 2 | 461 | 238 | 9 | 19 | 2 |
Diabetes | 8 | 2 | 507 | 261 | 8 | 17 | 2 |
Gene | 57 | 2 | 70 | 36 | 57 | 115 | 2 |
Parkinson | 22 | 2 | 129 | 66 | 22 | 45 | 2 |
Splice | 60 | 2 | 660 | 340 | 60 | 121 | 2 |
WDBC | 30 | 2 | 394 | 165 | 30 | 61 | 2 |
Zoo | 16 | 7 | 67 | 34 | 16 | 33 | 7 |
Algorithms | Parameter values |
GOA [19] | Cmin= 0.00004, Cmax= 1 |
GSO [42] | Luciferin decay value is 0.4, luciferin enhancement is 0.6, the rate of the neighborhood range is 0.08, the number of neighbors is 5, the step size of moving is 0.3, initial luciferin is 5 |
SSO [43] | PF = 0.7 |
FPA [42] | P = 0.8 |
GA [11] | Crossover rate is 1, Mutation rate is 0.01 |
WOA [44] | →α Linearly decreased from 2 to 0 have been used as recommended in [44]. |
TSWOA | →α Linearly decreased from 2 to 0 have been used as recommended in [44] |
Algorithms | Best | Worst | Mean | Std | Accuracy | Rank |
GOA | 3.28E − 01 | 3.55E − 01 | 3.37E − 01 | 6.32E − 03 | 78.43 | 3 |
GSO | 3.45E − 01 | 3.96E − 01 | 3.66E − 01 | 1.49E − 02 | 76.47 | 6 |
SSO | 3.27E − 01 | 3.65E − 01 | 3.49E − 01 | 9.11E − 03 | 78.82 | 2 |
FPA | 3.30E − 01 | 3.69E − 01 | 3.47E − 01 | 9.69E − 03 | 78.04 | 4 |
GA | 3.30E − 01 | 4.15E − 01 | 3.82E − 01 | 2.60E − 02 | 77.25 | 5 |
WOA | 3.30E − 01 | 3.61E − 01 | 3.47E − 01 | 1.06E − 02 | 76.25 | 7 |
TSWOA | 3.05E − 01 | 3.20E − 01 | 3.11E − 01 | 4.37E − 03 | 80.39 | 1 |
Algorithms | Best | Worst | Mean | Std | Accuracy | Rank |
GOA | 2.78E − 01 | 7.23E − 01 | 4.45E − 01 | 1.11E − 01 | 78.87 | 3 |
GSO | 5.02E − 01 | 1.10E + 00 | 7.95E − 01 | 1.42E − 01 | 61.50 | 6 |
SSO | 2.34E − 01 | 6.13E − 01 | 3.94E − 01 | 7.89E − 02 | 77.94 | 4 |
FPA | 2.01E − 01 | 3.50E − 01 | 2.46E − 01 | 3.73E − 02 | 89.67 | 1 |
GA | 2.82E − 01 | 6.91E − 01 | 5.04E − 01 | 1.09E − 01 | 76.53 | 5 |
WOA | 1.86E − 01 | 4.33E − 01 | 3.06E − 01 | 7.43E − 02 | 86.38 | 2 |
TSWOA | 1.16E − 01 | 5.81E − 01 | 1.75E − 01 | 9.65E − 02 | 89.67 | 1 |
Algorithms | Best | Worst | Mean | Std | Accuracy | Rank |
GOA | 3.81E − 01 | 4.03E − 01 | 3.95E − 01 | 5.57E − 03 | 81.73 | 1 |
GSO | 3.99E − 01 | 4.48E − 01 | 4.23E − 01 | 1.37E − 02 | 81.73 | 1 |
SSO | 3.93E − 01 | 4.33E − 01 | 4.15E − 01 | 1.05E − 02 | 81.73 | 1 |
FPA | 3.82E − 01 | 4.18E − 01 | 4.02E − 01 | 9.55E − 03 | 79.8 | 4 |
GA | 3.99E − 01 | 5.16E − 01 | 4.53E − 01 | 3.16E − 02 | 81.72 | 2 |
WOA | 3.79E − 01 | 4.25E − 01 | 4.06E − 01 | 1.40E − 02 | 81.73 | 1 |
TSWOA | 3.38E − 01 | 3.83E − 01 | 3.64E − 01 | 1.10E − 02 | 80.77 | 3 |
Algorithms | Best | Worst | Mean | Std | Accuracy | Rank |
GOA | 4.46E − 01 | 4.72E − 01 | 4.62E − 01 | 8.53E − 03 | 60.17 | 3 |
GSO | 4.86E − 01 | 5.41E − 01 | 5.14E − 01 | 1.54E − 02 | 55.93 | 6 |
SSO | 4.84E − 01 | 5.25E − 01 | 4.99E − 01 | 9.82E − 03 | 56.80 | 4 |
FPA | 4.49E − 01 | 5.30E − 01 | 4.95E − 01 | 1.82E − 02 | 62.71 | 2 |
GA | 5.06E − 01 | 6.91E − 01 | 5.93E − 01 | 5.33E − 02 | 49.15 | 7 |
WOA | 4.51E − 01 | 4.83E − 01 | 4.73E − 01 | 8.92E − 03 | 56.78 | 5 |
TSWOA | 3.65E − 01 | 4.41E − 01 | 3.94E − 01 | 1.90E − 02 | 72.88 | 1 |
Algorithms | Best | Worst | Mean | Std | Accuracy | Rank |
GOA | 2.69E − 01 | 8.34E − 01 | 4.68E − 01 | 1.89E − 01 | 77.46 | 4 |
GSO | 5.58E − 01 | 1.20E + 00 | 9.45E − 01 | 1.99E − 01 | 43.66 | 6 |
SSO | 1.42E − 01 | 4.56E − 01 | 3.14E − 01 | 7.22E − 02 | 83.10 | 2 |
FPA | 7.19E − 02 | 2.59E − 01 | 1.45E − 01 | 4.49E − 02 | 95.77 | 1 |
GA | 1.82E − 01 | 7.39E − 01 | 4.33E − 01 | 1.45E − 01 | 70.42 | 5 |
WOA | 1.10E − 01 | 4.37E − 01 | 2.34E − 01 | 9.62E − 02 | 81.69 | 3 |
TSWOA | 2.59E − 02 | 6.68E − 01 | 1.62E − 01 | 1.72E − 01 | 95.77 | 1 |
Algorithms | Best | Worst | Mean | Std | Accuracy | Rank |
GOA | 2.79E − 01 | 9.92E − 01 | 6.72E − 01 | 2.18E − 01 | 67.21 | 5 |
GSO | 6.34E − 01 | 1.58E + 00 | 1.18E + 00 | 2.46E − 01 | 44.26 | 7 |
SSO | 1.81E − 01 | 6.75E − 01 | 4.57E − 01 | 1.35E − 01 | 81.97 | 4 |
FPA | 1.11E − 01 | 2.65E − 01 | 1.69E − 01 | 4.59E − 02 | 85.25 | 2 |
GA | 4.27E − 01 | 9.20E − 01 | 6.81E − 01 | 1.38E − 01 | 52.46 | 6 |
WOA | 1.66E − 01 | 3.54E − 01 | 2.68E − 01 | 5.62E − 02 | 83.61 | 3 |
TSWOA | 1.73E − 02 | 5.13E − 01 | 1.62E − 01 | 1.62E − 01 | 96.72 | 1 |
Algorithms | Best | Worst | Mean | Std | Accuracy | Rank |
GOA | 3.04E − 01 | 7.35E − 01 | 4.68E − 01 | 1.17E − 01 | 84.31 | 6 |
GSO | 4.51E − 01 | 8.96E − 01 | 6.77E − 01 | 1.39E − 01 | 70.59 | 7 |
SSO | 2.08E − 01 | 4.64E − 01 | 3.44E − 01 | 6.76E − 02 | 96.08 | 3 |
FPA | 2.02E − 02 | 1.81E − 01 | 9.64E − 02 | 4.04E − 02 | 96.03 | 4 |
GA | 1.39E − 01 | 7.28E − 01 | 4.20E − 01 | 1.76E − 01 | 98.03 | 2 |
WOA | 8.79E − 02 | 3.81E − 01 | 2.47E − 01 | 7.70E − 02 | 94.12 | 5 |
TSWOA | 2.63E − 02 | 6.47E − 01 | 1.51E − 01 | 1.90E − 01 | 98.04 | 1 |
Algorithms | Best | Worst | Mean | Std | Accuracy | Rank |
GOA | 2.54E − 01 | 5.79E − 01 | 4.57E − 01 | 1.19E − 01 | 79.35 | 4 |
GSO | 5.28E − 01 | 7.44E − 01 | 6.35E − 01 | 5.78E − 02 | 46.74 | 7 |
SSO | 3.26E − 01 | 5.25E − 01 | 4.12E − 01 | 4.83E − 02 | 75 | 5 |
FPA | 2.75E − 01 | 3.88E − 01 | 3.13E − 01 | 3.37E − 02 | 80.69 | 3 |
GA | 4.14E − 01 | 6.90E − 01 | 5.67E − 01 | 8.57E − 02 | 70.65 | 6 |
WOA | 2.44E − 01 | 3.51E − 01 | 2.94E − 01 | 2.98E − 02 | 83.69 | 2 |
TSWOA | 1.12E − 01 | 2.00E − 01 | 1.40E − 01 | 2.23E − 02 | 83.70 | 1 |
Algorithms | Best | Worst | Mean | Std | Accuracy | Rank |
GOA | 6.23E − 02 | 2.69E − 01 | 1.72E − 01 | 7.95E − 02 | 97 | 3 |
GSO | 7.96E − 02 | 3.78E − 01 | 2.49E − 01 | 6.78E − 02 | 89 | 4 |
SSO | 7.09E − 02 | 1.72E − 01 | 1.13E − 01 | 2.69E − 02 | 98 | 2 |
FPA | 5.01E − 02 | 8.68E − 02 | 6.79E − 02 | 9.31E − 03 | 99 | 1 |
GA | 7.79E − 02 | 3.61E − 01 | 1.70E − 01 | 7.30E − 02 | 98 | 2 |
WOA | 5.29E − 02 | 1.37E − 01 | 7.53E − 02 | 2.57E − 02 | 99 | 1 |
TSWOA | 2.78E − 02 | 2.50E − 01 | 4.86E − 02 | 4.93E − 02 | 98 | 2 |
Algorithms | Best | Worst | Mean | Std | Accuracy | Rank |
GOA | 3.59E − 01 | 4.04E − 01 | 3.83E − 01 | 1.41E − 02 | 75.87 | 3 |
GSO | 4.24E − 01 | 6.03E − 01 | 5.15E − 01 | 4.54E − 02 | 64.75 | 7 |
SSO | 4.18E − 01 | 5.03E − 01 | 4.52E − 01 | 2.35E − 02 | 65.51 | 6 |
FPA | 3.85E − 01 | 4.75E − 01 | 4.44E − 01 | 2.48E − 02 | 80.08 | 2 |
GA | 4.81E − 01 | 6.40E − 01 | 5.41E − 01 | 4.49E − 02 | 67.05 | 5 |
WOA | 3.54E − 01 | 4.29E − 01 | 3.90E − 01 | 2.20E − 02 | 73.18 | 4 |
TSWOA | 3.04E − 01 | 3.29E − 01 | 3.19E − 01 | 6.10E − 03 | 81.23 | 1 |
Algorithms | Best | Worst | Mean | Std | Accuracy | Rank |
GOA | 8.09E − 01 | 9.28E − 01 | 9.10E − 01 | 2.68E − 02 | 2.66 | 6 |
GSO | 9.31E − 01 | 9.41E − 01 | 9.38E − 01 | 2.67E − 03 | 1.77 | 7 |
SSO | 3.58E − 01 | 4.74E − 01 | 4.08E − 01 | 3.07E − 02 | 5.56 | 5 |
FPA | 2.43E − 01 | 3.86E − 01 | 3.12E − 01 | 3.80E − 02 | 13.89 | 3 |
GA | 4.55E − 01 | 5.85E − 01 | 5.04E − 01 | 3.23E − 02 | 11.11 | 4 |
WOA | 2.74E − 01 | 4.12E − 01 | 3.24E − 01 | 3.58E − 02 | 16.67 | 2 |
TSWOA | 1.46E − 02 | 3.29E − 01 | 9.43E − 02 | 8.36E − 02 | 33.33 | 1 |
Algorithms | Best | Worst | Mean | Std | Accuracy | Rank |
GOA | 1.48E − 01 | 2.66E − 01 | 2.01E − 01 | 3.06E − 02 | 68.18 | 5 |
GSO | 2.63E − 01 | 5.20E − 01 | 4.22E − 01 | 8.04E − 02 | 56.06 | 7 |
SSO | 1.75E − 01 | 2.54E − 01 | 2.16E − 01 | 2.21E − 02 | 69.7 | 4 |
FPA | 9.30E − 02 | 1.86E − 01 | 1.36E − 01 | 1.99E − 02 | 70.21 | 3 |
GA | 2.07E − 01 | 3.81E − 01 | 3.03E − 01 | 5.03E − 02 | 65.15 | 6 |
WOA | 1.32E − 01 | 2.81E − 01 | 1.85E − 01 | 3.76E − 02 | 71.21 | 2 |
TSWOA | 1.48E − 02 | 2.33E − 01 | 7.63E − 02 | 6.08E − 02 | 74.24 | 1 |
Algorithms | Best | Worst | Mean | Std | Accuracy | Rank |
GOA | 9.41E − 01 | 9.46E − 01 | 9.43E − 01 | 1.12E − 03 | 37.24 | 7 |
GSO | 9.42E − 01 | 9.47E − 01 | 9.45E − 01 | 1.48E − 03 | 40.13 | 6 |
SSO | 6.96E − 01 | 8.64E − 01 | 7.92E − 01 | 4.40E − 02 | 41.18 | 4 |
FPA | 4.56E − 01 | 6.67E − 01 | 5.27E − 01 | 4.71E − 02 | 70 | 2 |
GA | 7.91E − 01 | 8.84E − 01 | 8.52E − 01 | 2.53E − 02 | 41.17 | 5 |
WOA | 4.65E − 01 | 5.58E − 01 | 5.10E − 01 | 2.79E − 02 | 51.47 | 3 |
TSWOA | 1.59E − 01 | 2.37E − 01 | 1.97E − 01 | 2.30E − 02 | 80 | 1 |
Algorithms | Best | Worst | Mean | Std | Accuracy | Rank |
GOA | 1.32E − 01 | 2.83E − 01 | 2.15E − 01 | 4.11E − 02 | 90.9 | 5 |
GSO | 4.09E − 01 | 5.38E − 01 | 5.15E − 01 | 2.92E − 02 | 79.39 | 6 |
SSO | 9.51E − 02 | 3.26E − 01 | 2.20E − 01 | 5.60E − 02 | 93.31 | 4 |
FPA | 1.02E − 01 | 1.57E − 01 | 1.24E − 01 | 1.80E − 02 | 93.33 | 3 |
GA | 3.05E − 01 | 6.79E − 01 | 4.93E − 01 | 1.11E − 01 | 78.79 | 7 |
WOA | 9.98E − 02 | 3.17E − 01 | 1.69E − 01 | 5.68E − 02 | 94.55 | 2 |
TSWOA | 3.07E − 02 | 9.93E − 02 | 4.28E − 02 | 1.77E − 02 | 95.15 | 1 |
Algorithms | Best | Worst | Mean | Std | Accuracy | Rank |
GOA | 2.36E + 00 | 4.47E + 00 | 3.60E + 00 | 5.67E − 01 | 23.70 | 7 |
GSO | 3.46E + 00 | 5.54E + 00 | 5.41E + 00 | 4.59E − 01 | 30.73 | 5 |
SSO | 4.83E − 01 | 2.06E + 00 | 1.15E + 00 | 5.21E − 01 | 44.12 | 4 |
FPA | 1.79E − 01 | 4.63E − 01 | 3.32E − 01 | 7.42E − 02 | 63.61 | 3 |
GA | 1.20E + 00 | 1.99E + 00 | 1.53E + 00 | 2.12E − 01 | 26.47 | 6 |
WOA | 1.97E − 01 | 5.52E − 01 | 4.23E − 01 | 9.97E − 02 | 67.65 | 1 |
TSWOA | 1.34E − 01 | 7.61E − 01 | 3.75E − 01 | 1.85E − 01 | 64.71 | 2 |
Functions | TSWOA vs | |||||
GOA | GSO | SSO | FPA | GA | WOA | |
Blood | 6.80E − 08 | 6.80E − 08 | 6.80E − 08 | 6.80E − 08 | 6.80E − 08 | 6.80E − 08 |
Scale | 6.92E − 07 | 7.90E − 08 | 1.05E − 06 | 1.20E − 06 | 5.23E − 07 | 1.20E − 06 |
Survival | 7.90E − 08 | 6.80E − 08 | 6.80E − 08 | 7.90E − 08 | 6.80E − 08 | 7.90E − 08 |
Liver | 6.80E − 08 | 6.80E − 08 | 6.80E − 08 | 6.80E − 08 | 6.80E − 08 | 6.80E − 08 |
Seeds | 4.68E − 05 | 1.23E − 07 | 1.63E − 03 | 6.76E − 02 | 2.60E − 05 | 7.71E − 03 |
Wine | 6.01E − 07 | 6.80E − 08 | 1.10E − 05 | 3.30E − 01 | 1.23E − 07 | 2.39E − 02 |
Iris | 1.41E − 05 | 5.23E − 07 | 8.29E − 05 | 3.23E − 01 | 3.71E − 05 | 1.01E − 03 |
Statlog | 6.80E − 08 | 6.80E − 08 | 6.80E − 08 | 6.73E − 08 | 6.80E − 08 | 6.80E − 08 |
Cancer | 1.05E − 06 | 2.22E − 07 | 2.06E − 06 | 1.59E − 05 | 9.13E − 07 | 9.75E − 06 |
Diabetes | 6.80E − 08 | 6.80E − 08 | 6.80E − 08 | 6.80E − 08 | 6.80E − 08 | 6.80E − 08 |
Gene | 6.80E − 08 | 6.80E − 08 | 6.80E − 08 | 9.37E − 07 | 6.80E − 08 | 6.92E − 07 |
Parkinson | 5.87E − 06 | 6.80E − 08 | 1.80E − 06 | 2.17E − 04 | 1.06E − 07 | 2.30E − 05 |
Splice | 6.80E − 08 | 6.80E − 08 | 6.80E − 08 | 6.79E − 08 | 6.80E − 08 | 6.80E − 08 |
WDBC | 6.80E − 08 | 6.80E − 08 | 7.90E − 08 | 6.73E − 08 | 6.80E − 08 | 6.80E − 08 |
Zoo | 6.78E − 08 | 6.78E − 08 | 7.93E − 07 | 6.55E − 01 | 6.78E − 08 | 2.61E − 01 |
Datasets | Attribute | Class | Training | Testing | Input | Hidden | Output |
Blood | 4 | 2 | 493 | 255 | 4 | 9 | 2 |
Scale | 4 | 3 | 412 | 213 | 4 | 9 | 3 |
Survival | 3 | 2 | 202 | 104 | 3 | 7 | 2 |
Liver | 6 | 2 | 227 | 118 | 6 | 13 | 2 |
Seeds | 7 | 3 | 139 | 71 | 7 | 15 | 3 |
Wine | 13 | 3 | 117 | 61 | 13 | 27 | 3 |
Iris | 4 | 3 | 99 | 51 | 4 | 9 | 3 |
Statlog | 13 | 2 | 178 | 92 | 13 | 27 | 2 |
Cancer | 9 | 2 | 461 | 238 | 9 | 19 | 2 |
Diabetes | 8 | 2 | 507 | 261 | 8 | 17 | 2 |
Gene | 57 | 2 | 70 | 36 | 57 | 115 | 2 |
Parkinson | 22 | 2 | 129 | 66 | 22 | 45 | 2 |
Splice | 60 | 2 | 660 | 340 | 60 | 121 | 2 |
WDBC | 30 | 2 | 394 | 165 | 30 | 61 | 2 |
Zoo | 16 | 7 | 67 | 34 | 16 | 33 | 7 |
Algorithms | Parameter values |
GOA [19] | Cmin= 0.00004, Cmax= 1 |
GSO [42] | Luciferin decay value is 0.4, luciferin enhancement is 0.6, the rate of the neighborhood range is 0.08, the number of neighbors is 5, the step size of moving is 0.3, initial luciferin is 5 |
SSO [43] | PF = 0.7 |
FPA [42] | P = 0.8 |
GA [11] | Crossover rate is 1, Mutation rate is 0.01 |
WOA [44] | →α Linearly decreased from 2 to 0 have been used as recommended in [44]. |
TSWOA | →α Linearly decreased from 2 to 0 have been used as recommended in [44] |
Algorithms | Best | Worst | Mean | Std | Accuracy | Rank |
GOA | 3.28E − 01 | 3.55E − 01 | 3.37E − 01 | 6.32E − 03 | 78.43 | 3 |
GSO | 3.45E − 01 | 3.96E − 01 | 3.66E − 01 | 1.49E − 02 | 76.47 | 6 |
SSO | 3.27E − 01 | 3.65E − 01 | 3.49E − 01 | 9.11E − 03 | 78.82 | 2 |
FPA | 3.30E − 01 | 3.69E − 01 | 3.47E − 01 | 9.69E − 03 | 78.04 | 4 |
GA | 3.30E − 01 | 4.15E − 01 | 3.82E − 01 | 2.60E − 02 | 77.25 | 5 |
WOA | 3.30E − 01 | 3.61E − 01 | 3.47E − 01 | 1.06E − 02 | 76.25 | 7 |
TSWOA | 3.05E − 01 | 3.20E − 01 | 3.11E − 01 | 4.37E − 03 | 80.39 | 1 |
Algorithms | Best | Worst | Mean | Std | Accuracy | Rank |
GOA | 2.78E − 01 | 7.23E − 01 | 4.45E − 01 | 1.11E − 01 | 78.87 | 3 |
GSO | 5.02E − 01 | 1.10E + 00 | 7.95E − 01 | 1.42E − 01 | 61.50 | 6 |
SSO | 2.34E − 01 | 6.13E − 01 | 3.94E − 01 | 7.89E − 02 | 77.94 | 4 |
FPA | 2.01E − 01 | 3.50E − 01 | 2.46E − 01 | 3.73E − 02 | 89.67 | 1 |
GA | 2.82E − 01 | 6.91E − 01 | 5.04E − 01 | 1.09E − 01 | 76.53 | 5 |
WOA | 1.86E − 01 | 4.33E − 01 | 3.06E − 01 | 7.43E − 02 | 86.38 | 2 |
TSWOA | 1.16E − 01 | 5.81E − 01 | 1.75E − 01 | 9.65E − 02 | 89.67 | 1 |
Algorithms | Best | Worst | Mean | Std | Accuracy | Rank |
GOA | 3.81E − 01 | 4.03E − 01 | 3.95E − 01 | 5.57E − 03 | 81.73 | 1 |
GSO | 3.99E − 01 | 4.48E − 01 | 4.23E − 01 | 1.37E − 02 | 81.73 | 1 |
SSO | 3.93E − 01 | 4.33E − 01 | 4.15E − 01 | 1.05E − 02 | 81.73 | 1 |
FPA | 3.82E − 01 | 4.18E − 01 | 4.02E − 01 | 9.55E − 03 | 79.8 | 4 |
GA | 3.99E − 01 | 5.16E − 01 | 4.53E − 01 | 3.16E − 02 | 81.72 | 2 |
WOA | 3.79E − 01 | 4.25E − 01 | 4.06E − 01 | 1.40E − 02 | 81.73 | 1 |
TSWOA | 3.38E − 01 | 3.83E − 01 | 3.64E − 01 | 1.10E − 02 | 80.77 | 3 |
Algorithms | Best | Worst | Mean | Std | Accuracy | Rank |
GOA | 4.46E − 01 | 4.72E − 01 | 4.62E − 01 | 8.53E − 03 | 60.17 | 3 |
GSO | 4.86E − 01 | 5.41E − 01 | 5.14E − 01 | 1.54E − 02 | 55.93 | 6 |
SSO | 4.84E − 01 | 5.25E − 01 | 4.99E − 01 | 9.82E − 03 | 56.80 | 4 |
FPA | 4.49E − 01 | 5.30E − 01 | 4.95E − 01 | 1.82E − 02 | 62.71 | 2 |
GA | 5.06E − 01 | 6.91E − 01 | 5.93E − 01 | 5.33E − 02 | 49.15 | 7 |
WOA | 4.51E − 01 | 4.83E − 01 | 4.73E − 01 | 8.92E − 03 | 56.78 | 5 |
TSWOA | 3.65E − 01 | 4.41E − 01 | 3.94E − 01 | 1.90E − 02 | 72.88 | 1 |
Algorithms | Best | Worst | Mean | Std | Accuracy | Rank |
GOA | 2.69E − 01 | 8.34E − 01 | 4.68E − 01 | 1.89E − 01 | 77.46 | 4 |
GSO | 5.58E − 01 | 1.20E + 00 | 9.45E − 01 | 1.99E − 01 | 43.66 | 6 |
SSO | 1.42E − 01 | 4.56E − 01 | 3.14E − 01 | 7.22E − 02 | 83.10 | 2 |
FPA | 7.19E − 02 | 2.59E − 01 | 1.45E − 01 | 4.49E − 02 | 95.77 | 1 |
GA | 1.82E − 01 | 7.39E − 01 | 4.33E − 01 | 1.45E − 01 | 70.42 | 5 |
WOA | 1.10E − 01 | 4.37E − 01 | 2.34E − 01 | 9.62E − 02 | 81.69 | 3 |
TSWOA | 2.59E − 02 | 6.68E − 01 | 1.62E − 01 | 1.72E − 01 | 95.77 | 1 |
Algorithms | Best | Worst | Mean | Std | Accuracy | Rank |
GOA | 2.79E − 01 | 9.92E − 01 | 6.72E − 01 | 2.18E − 01 | 67.21 | 5 |
GSO | 6.34E − 01 | 1.58E + 00 | 1.18E + 00 | 2.46E − 01 | 44.26 | 7 |
SSO | 1.81E − 01 | 6.75E − 01 | 4.57E − 01 | 1.35E − 01 | 81.97 | 4 |
FPA | 1.11E − 01 | 2.65E − 01 | 1.69E − 01 | 4.59E − 02 | 85.25 | 2 |
GA | 4.27E − 01 | 9.20E − 01 | 6.81E − 01 | 1.38E − 01 | 52.46 | 6 |
WOA | 1.66E − 01 | 3.54E − 01 | 2.68E − 01 | 5.62E − 02 | 83.61 | 3 |
TSWOA | 1.73E − 02 | 5.13E − 01 | 1.62E − 01 | 1.62E − 01 | 96.72 | 1 |
Algorithms | Best | Worst | Mean | Std | Accuracy | Rank |
GOA | 3.04E − 01 | 7.35E − 01 | 4.68E − 01 | 1.17E − 01 | 84.31 | 6 |
GSO | 4.51E − 01 | 8.96E − 01 | 6.77E − 01 | 1.39E − 01 | 70.59 | 7 |
SSO | 2.08E − 01 | 4.64E − 01 | 3.44E − 01 | 6.76E − 02 | 96.08 | 3 |
FPA | 2.02E − 02 | 1.81E − 01 | 9.64E − 02 | 4.04E − 02 | 96.03 | 4 |
GA | 1.39E − 01 | 7.28E − 01 | 4.20E − 01 | 1.76E − 01 | 98.03 | 2 |
WOA | 8.79E − 02 | 3.81E − 01 | 2.47E − 01 | 7.70E − 02 | 94.12 | 5 |
TSWOA | 2.63E − 02 | 6.47E − 01 | 1.51E − 01 | 1.90E − 01 | 98.04 | 1 |
Algorithms | Best | Worst | Mean | Std | Accuracy | Rank |
GOA | 2.54E − 01 | 5.79E − 01 | 4.57E − 01 | 1.19E − 01 | 79.35 | 4 |
GSO | 5.28E − 01 | 7.44E − 01 | 6.35E − 01 | 5.78E − 02 | 46.74 | 7 |
SSO | 3.26E − 01 | 5.25E − 01 | 4.12E − 01 | 4.83E − 02 | 75 | 5 |
FPA | 2.75E − 01 | 3.88E − 01 | 3.13E − 01 | 3.37E − 02 | 80.69 | 3 |
GA | 4.14E − 01 | 6.90E − 01 | 5.67E − 01 | 8.57E − 02 | 70.65 | 6 |
WOA | 2.44E − 01 | 3.51E − 01 | 2.94E − 01 | 2.98E − 02 | 83.69 | 2 |
TSWOA | 1.12E − 01 | 2.00E − 01 | 1.40E − 01 | 2.23E − 02 | 83.70 | 1 |
Algorithms | Best | Worst | Mean | Std | Accuracy | Rank |
GOA | 6.23E − 02 | 2.69E − 01 | 1.72E − 01 | 7.95E − 02 | 97 | 3 |
GSO | 7.96E − 02 | 3.78E − 01 | 2.49E − 01 | 6.78E − 02 | 89 | 4 |
SSO | 7.09E − 02 | 1.72E − 01 | 1.13E − 01 | 2.69E − 02 | 98 | 2 |
FPA | 5.01E − 02 | 8.68E − 02 | 6.79E − 02 | 9.31E − 03 | 99 | 1 |
GA | 7.79E − 02 | 3.61E − 01 | 1.70E − 01 | 7.30E − 02 | 98 | 2 |
WOA | 5.29E − 02 | 1.37E − 01 | 7.53E − 02 | 2.57E − 02 | 99 | 1 |
TSWOA | 2.78E − 02 | 2.50E − 01 | 4.86E − 02 | 4.93E − 02 | 98 | 2 |
Algorithms | Best | Worst | Mean | Std | Accuracy | Rank |
GOA | 3.59E − 01 | 4.04E − 01 | 3.83E − 01 | 1.41E − 02 | 75.87 | 3 |
GSO | 4.24E − 01 | 6.03E − 01 | 5.15E − 01 | 4.54E − 02 | 64.75 | 7 |
SSO | 4.18E − 01 | 5.03E − 01 | 4.52E − 01 | 2.35E − 02 | 65.51 | 6 |
FPA | 3.85E − 01 | 4.75E − 01 | 4.44E − 01 | 2.48E − 02 | 80.08 | 2 |
GA | 4.81E − 01 | 6.40E − 01 | 5.41E − 01 | 4.49E − 02 | 67.05 | 5 |
WOA | 3.54E − 01 | 4.29E − 01 | 3.90E − 01 | 2.20E − 02 | 73.18 | 4 |
TSWOA | 3.04E − 01 | 3.29E − 01 | 3.19E − 01 | 6.10E − 03 | 81.23 | 1 |
Algorithms | Best | Worst | Mean | Std | Accuracy | Rank |
GOA | 8.09E − 01 | 9.28E − 01 | 9.10E − 01 | 2.68E − 02 | 2.66 | 6 |
GSO | 9.31E − 01 | 9.41E − 01 | 9.38E − 01 | 2.67E − 03 | 1.77 | 7 |
SSO | 3.58E − 01 | 4.74E − 01 | 4.08E − 01 | 3.07E − 02 | 5.56 | 5 |
FPA | 2.43E − 01 | 3.86E − 01 | 3.12E − 01 | 3.80E − 02 | 13.89 | 3 |
GA | 4.55E − 01 | 5.85E − 01 | 5.04E − 01 | 3.23E − 02 | 11.11 | 4 |
WOA | 2.74E − 01 | 4.12E − 01 | 3.24E − 01 | 3.58E − 02 | 16.67 | 2 |
TSWOA | 1.46E − 02 | 3.29E − 01 | 9.43E − 02 | 8.36E − 02 | 33.33 | 1 |
Algorithms | Best | Worst | Mean | Std | Accuracy | Rank |
GOA | 1.48E − 01 | 2.66E − 01 | 2.01E − 01 | 3.06E − 02 | 68.18 | 5 |
GSO | 2.63E − 01 | 5.20E − 01 | 4.22E − 01 | 8.04E − 02 | 56.06 | 7 |
SSO | 1.75E − 01 | 2.54E − 01 | 2.16E − 01 | 2.21E − 02 | 69.7 | 4 |
FPA | 9.30E − 02 | 1.86E − 01 | 1.36E − 01 | 1.99E − 02 | 70.21 | 3 |
GA | 2.07E − 01 | 3.81E − 01 | 3.03E − 01 | 5.03E − 02 | 65.15 | 6 |
WOA | 1.32E − 01 | 2.81E − 01 | 1.85E − 01 | 3.76E − 02 | 71.21 | 2 |
TSWOA | 1.48E − 02 | 2.33E − 01 | 7.63E − 02 | 6.08E − 02 | 74.24 | 1 |
Algorithms | Best | Worst | Mean | Std | Accuracy | Rank |
GOA | 9.41E − 01 | 9.46E − 01 | 9.43E − 01 | 1.12E − 03 | 37.24 | 7 |
GSO | 9.42E − 01 | 9.47E − 01 | 9.45E − 01 | 1.48E − 03 | 40.13 | 6 |
SSO | 6.96E − 01 | 8.64E − 01 | 7.92E − 01 | 4.40E − 02 | 41.18 | 4 |
FPA | 4.56E − 01 | 6.67E − 01 | 5.27E − 01 | 4.71E − 02 | 70 | 2 |
GA | 7.91E − 01 | 8.84E − 01 | 8.52E − 01 | 2.53E − 02 | 41.17 | 5 |
WOA | 4.65E − 01 | 5.58E − 01 | 5.10E − 01 | 2.79E − 02 | 51.47 | 3 |
TSWOA | 1.59E − 01 | 2.37E − 01 | 1.97E − 01 | 2.30E − 02 | 80 | 1 |
Algorithms | Best | Worst | Mean | Std | Accuracy | Rank |
GOA | 1.32E − 01 | 2.83E − 01 | 2.15E − 01 | 4.11E − 02 | 90.9 | 5 |
GSO | 4.09E − 01 | 5.38E − 01 | 5.15E − 01 | 2.92E − 02 | 79.39 | 6 |
SSO | 9.51E − 02 | 3.26E − 01 | 2.20E − 01 | 5.60E − 02 | 93.31 | 4 |
FPA | 1.02E − 01 | 1.57E − 01 | 1.24E − 01 | 1.80E − 02 | 93.33 | 3 |
GA | 3.05E − 01 | 6.79E − 01 | 4.93E − 01 | 1.11E − 01 | 78.79 | 7 |
WOA | 9.98E − 02 | 3.17E − 01 | 1.69E − 01 | 5.68E − 02 | 94.55 | 2 |
TSWOA | 3.07E − 02 | 9.93E − 02 | 4.28E − 02 | 1.77E − 02 | 95.15 | 1 |
Algorithms | Best | Worst | Mean | Std | Accuracy | Rank |
GOA | 2.36E + 00 | 4.47E + 00 | 3.60E + 00 | 5.67E − 01 | 23.70 | 7 |
GSO | 3.46E + 00 | 5.54E + 00 | 5.41E + 00 | 4.59E − 01 | 30.73 | 5 |
SSO | 4.83E − 01 | 2.06E + 00 | 1.15E + 00 | 5.21E − 01 | 44.12 | 4 |
FPA | 1.79E − 01 | 4.63E − 01 | 3.32E − 01 | 7.42E − 02 | 63.61 | 3 |
GA | 1.20E + 00 | 1.99E + 00 | 1.53E + 00 | 2.12E − 01 | 26.47 | 6 |
WOA | 1.97E − 01 | 5.52E − 01 | 4.23E − 01 | 9.97E − 02 | 67.65 | 1 |
TSWOA | 1.34E − 01 | 7.61E − 01 | 3.75E − 01 | 1.85E − 01 | 64.71 | 2 |
Functions | TSWOA vs | |||||
GOA | GSO | SSO | FPA | GA | WOA | |
Blood | 6.80E − 08 | 6.80E − 08 | 6.80E − 08 | 6.80E − 08 | 6.80E − 08 | 6.80E − 08 |
Scale | 6.92E − 07 | 7.90E − 08 | 1.05E − 06 | 1.20E − 06 | 5.23E − 07 | 1.20E − 06 |
Survival | 7.90E − 08 | 6.80E − 08 | 6.80E − 08 | 7.90E − 08 | 6.80E − 08 | 7.90E − 08 |
Liver | 6.80E − 08 | 6.80E − 08 | 6.80E − 08 | 6.80E − 08 | 6.80E − 08 | 6.80E − 08 |
Seeds | 4.68E − 05 | 1.23E − 07 | 1.63E − 03 | 6.76E − 02 | 2.60E − 05 | 7.71E − 03 |
Wine | 6.01E − 07 | 6.80E − 08 | 1.10E − 05 | 3.30E − 01 | 1.23E − 07 | 2.39E − 02 |
Iris | 1.41E − 05 | 5.23E − 07 | 8.29E − 05 | 3.23E − 01 | 3.71E − 05 | 1.01E − 03 |
Statlog | 6.80E − 08 | 6.80E − 08 | 6.80E − 08 | 6.73E − 08 | 6.80E − 08 | 6.80E − 08 |
Cancer | 1.05E − 06 | 2.22E − 07 | 2.06E − 06 | 1.59E − 05 | 9.13E − 07 | 9.75E − 06 |
Diabetes | 6.80E − 08 | 6.80E − 08 | 6.80E − 08 | 6.80E − 08 | 6.80E − 08 | 6.80E − 08 |
Gene | 6.80E − 08 | 6.80E − 08 | 6.80E − 08 | 9.37E − 07 | 6.80E − 08 | 6.92E − 07 |
Parkinson | 5.87E − 06 | 6.80E − 08 | 1.80E − 06 | 2.17E − 04 | 1.06E − 07 | 2.30E − 05 |
Splice | 6.80E − 08 | 6.80E − 08 | 6.80E − 08 | 6.79E − 08 | 6.80E − 08 | 6.80E − 08 |
WDBC | 6.80E − 08 | 6.80E − 08 | 7.90E − 08 | 6.73E − 08 | 6.80E − 08 | 6.80E − 08 |
Zoo | 6.78E − 08 | 6.78E − 08 | 7.93E − 07 | 6.55E − 01 | 6.78E − 08 | 2.61E − 01 |