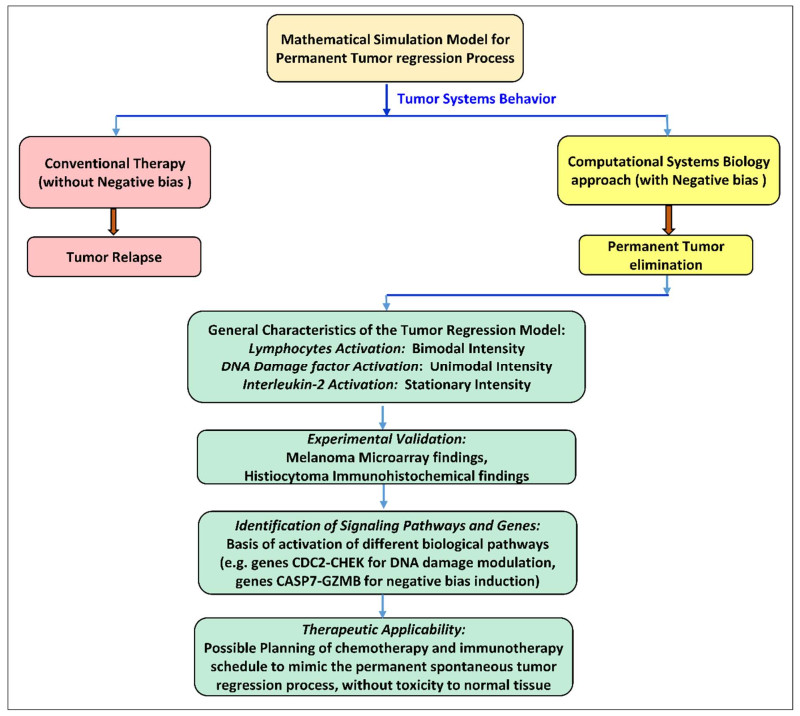
Citation: Mengya Zhang, Qing Wu, Zezhou Xu. Tuning extreme learning machine by an improved electromagnetism-like mechanism algorithm for classification problem[J]. Mathematical Biosciences and Engineering, 2019, 16(5): 4692-4707. doi: 10.3934/mbe.2019235
[1] | Jing Liu, Xuefang Zhang, Ting Ye, Yongjian Dong, Wenfeng Zhang, Fenglin Wu, Huaben Bo, Hongwei Shao, Rongxin Zhang, Han Shen . Prognostic modeling of patients with metastatic melanoma based on tumor immune microenvironment characteristics. Mathematical Biosciences and Engineering, 2022, 19(2): 1448-1470. doi: 10.3934/mbe.2022067 |
[2] | Tongmeng Jiang, Pan Jin, Guoxiu Huang, Shi-Cheng Li . The function of guanylate binding protein 3 (GBP3) in human cancers by pan-cancer bioinformatics. Mathematical Biosciences and Engineering, 2023, 20(5): 9511-9529. doi: 10.3934/mbe.2023418 |
[3] | Linqian Guo, Qingrong Meng, Wenqi Lin, Kaiyuan Weng . Identification of immune subtypes of melanoma based on single-cell and bulk RNA sequencing data. Mathematical Biosciences and Engineering, 2023, 20(2): 2920-2936. doi: 10.3934/mbe.2023138 |
[4] | Janine Egert, Clemens Kreutz . Realistic simulation of time-course measurements in systems biology. Mathematical Biosciences and Engineering, 2023, 20(6): 10570-10589. doi: 10.3934/mbe.2023467 |
[5] | Samantha L Elliott, Emek Kose, Allison L Lewis, Anna E Steinfeld, Elizabeth A Zollinger . Modeling the stem cell hypothesis: Investigating the effects of cancer stem cells and TGF−β on tumor growth. Mathematical Biosciences and Engineering, 2019, 16(6): 7177-7194. doi: 10.3934/mbe.2019360 |
[6] | Moxuan Zhang, Quan Zhang, Jilin Bai, Zhiming Zhao, Jian Zhang . Transcriptome analysis revealed CENPF associated with glioma prognosis. Mathematical Biosciences and Engineering, 2021, 18(3): 2077-2096. doi: 10.3934/mbe.2021107 |
[7] | Hsiu-Chuan Wei . Mathematical modeling of tumor growth: the MCF-7 breast cancer cell line. Mathematical Biosciences and Engineering, 2019, 16(6): 6512-6535. doi: 10.3934/mbe.2019325 |
[8] | Jihong Yang, Hao Xu, Congshu Li, Zhenhao Li, Zhe Hu . An explorative study for leveraging transcriptomic data of embryonic stem cells in mining cancer stemness genes, regulators, and networks. Mathematical Biosciences and Engineering, 2022, 19(12): 13949-13966. doi: 10.3934/mbe.2022650 |
[9] | Sidan Long, Shuangshuang Ji, Kunmin Xiao, Peng Xue, Shijie Zhu . Prognostic and immunological value of LTB4R in pan-cancer. Mathematical Biosciences and Engineering, 2021, 18(6): 9336-9356. doi: 10.3934/mbe.2021459 |
[10] | Jiaping Wang . Prognostic score model-based signature genes for predicting the prognosis of metastatic skin cutaneous melanoma. Mathematical Biosciences and Engineering, 2021, 18(5): 5125-5145. doi: 10.3934/mbe.2021261 |
There are several significant drawbacks to cancer treatment by antitumor agents, as chemotherapy and immunotherapy. The first issue is an apparent "clinical cure" whereby the tumor cells are eliminated to a major extent, so that the tumor is clinically undetectable, even though microscopic amounts of cancer cells remain, which flare up much later after the initial therapy, thus producing tumor recurrence. The second is presence of cancer stem cells which, even though initially forming an miniscule cellular population, goes on multiplying as they have much less sensitivity to therapeutic agents, thus producing resistant tumor relapse [1]. Another issue is the inability of administering therapeutic agents intensively, as the latter produces appreciable normal tissue damage, producing intolerable side-effects that prevent the administration of further therapy. These disadvantages need to be well addressed, even though it is also known that occasionally there is permanent elimination of a tumor by exogenous therapeutic agents. Some examples of such exogenous regression of tumors are multimodal chemoimmunotherapy using drugs (like alkylators as dacarbazine or temozolomide), antitumor lymphocyte therapy, along with interleukin [2,3].
Though the clinician usually encounters a malignant tumor in its progression phase, the reverse process of permanent spontaneous regression of malignant tumors is a well-documented phenomenon, occurring subclinically across human populations at 22–46% rate, as per the Scandinavian and Wisconsin Screening Registries which have tracked a population of 0.33 million and 2.95 million individuals respectively [4,5]. It is evident in autopsy studies that about half the subjects have malignant focus in uterine cervix or prostate, with confirmation of permanent containment, and furthermore, malignant neuroblastoma fully regresses from larger-sized tumors [4,6]. As per PubMed, there are about 14,000 titles of papers dealing with spontaneous cancer regression, covering virtually all types of malignant diseases such as sarcomas, carcinomas, lymphomas, melanomas and so on [7]. Though the regression process eliminates malignant cells, it does not damage the normal tissue, i.e. normal cells are protected overall. Typically, the duration of a tumor's regression generally occurs across a period of months, generally 1 to 2 months. Such endogenously-initiated regression of malignancy also occurs in animals, including worms and molluscs and even took place in dinosaurs which are now extinct [8]. In fact, there are numerous species of animals known to completely regress malignant lesions, which are usually fatal in man, such as melanoma. We have earlier elucidated the energetics and biothermodynamic basis of spontaneous tumor regression [9,10]. Indeed, the investigation of the spontaneous regression process may indicate incisive pointers on inducing the permanent regression process on human malignancies.
Spontaneous cancer regression (both endogenous or exogenous process) depends on various factors like the amount of the tumor load, the invasiveness of the disease, the intensity of the treatment, and the robustness of the patient's immune response. Mathematical modeling is seen to be a potentially vital tool for creating better treatment strategies for cancer patients to address the cancer regression process. Over the years, researchers have used various models to address the biological process of tumor growth and of anticancer treatment [11,12,13,14]. In this study we have considerably modified our previously developed model [15], in which tumor cell kinetics, chemotherapy dynamics, dynamics of immune system (NK cell, circulating lymphocyte and cytotoxic T-cell), chemotherapy dynamics, and immunomodulation/immunotherapy dynamics, are represented by a system of six differential equations. Our novel mathematical model is incisively based on experimentally observed biological processes, namely the lethal effect on the tumor cell, as induced by the immune cells, namely cytotoxic T-cells (CD8+), and natural killer (NK) cells, the delineation of these lethal interactions are delineated next.
It is well established that NK cells target tumor cells and produce cytokines by secreting perforins and granzymes as part of immune responses to malignancies [16,17]. These immune cells release perforins in the immunological synapse, the perforins are pore-forming cytolytic protein that form porous openings in the tumor cell membrane [18]. Once this occurs, the immune cells release granzyme, which is a proteolytic enzyme that travels through the pore into the cytoplasm of the tumor cell, activating the caspase cascade and thereby inducing programmed cell death or apoptosis in the malignant cell [19]. When NK cells and T-cells come into contact with cancer cells, immunological synapses are formed with tumor cells to deliver lysosome-mediated cytolytic agents (as cathepsins and hydrolases) inside the tumor cells [20]. Moreover, granzymes and perforin are two further examples of cytotoxic chemicals that CD8+ T cells can use to kill tumor cells. Additionally, chemokines as interferons (which cytotoxic CD8+ T-cells secrete) can activate the tumor cells to produce more MHC class I antigens, making the malignant cells to become more attractive targets for cytotoxic CD8+ T-cells [21].
In this paper, we attempt to quantitatively formulate the general methodology of complete tumor regression (whether endogenous or exogenous) and discern the unitary principles that enable this regression. Thereafter, the validation of the methodology in pre-clinical environment are shown on two malignant systems, melanoma and histiocytoma. To underscore, melanoma cases can undergo fully effective spontaneous regression (PubMed has 585 cases studied in detail [22]), and this melanoma regression occurs appreciably at 10–35% rate [23]. Analysis of 10,098 melanoma regression patients showed that these patients can have incisive clinical correlates [24]. Understanding melanoma regression is critically needed, as it is the type of malignancy whose incidence is accelerating maximally [25]. An important aspect here is that we have showed that a malignant lesion can undergo permanent regression by an optimized synchronization of: 1) antitumor feedback control process, based on optimal dynamic feedback according to tumor load, and 2) normal tissue protection, based on minimization of tissue toxicity, together with 3) ensuring that cancer stem cells are also eliminated. In contrast to this permanent regression approach, we also show that often conventionally dosed antitumor chemotherapy-immunotherapy intervention (i.e., without dynamic feedback control procedure) fails to eliminate the tumor cells completely, with the result that there is tumor recurrence later.
Various differential equation-based quantitative models are available in the literature to replicate the dynamics of the biological process of cancer regression, and some of these models will be delineated now. For instance, Perry [9] has used the laws of mass action and first-order dynamics to characterize the reaction kinetics of tumor cell lysis during exogenous tumor regression brought on by therapeutic agents such as chemotherapy medications that cause DNA damage in the malignant lesion. As a result, the tumor cell population T declines exponentially [9]. Furthermore, when a lesion spontaneously regresses, the tumor cell population reduces exponentially with time [26,27]. However, a residual tumor cell population asymptotically exists under the exponentially-decreasing trajectory and this population of residual cells is frequently a factor in tumor recurrence and incurability. It is well-known that three complementary processes can reduce the tumor cell population:
(ⅰ) Decrease of the proliferation of tumor cells: Here, chemical alkylation or chemomodulation of DNA are two methods for reducing cell proliferation that can lead to DNA damage [28,29],
(ⅱ) Increase of tumor cell lysis: This occurs through the medium of antitumor lymphocytes [18,30,31],
(ⅲ) Further enhancement of tumor cell lysis: The activation of the antitumor lymphocytes can be boosted by cytokines (for example, immunomodulation by interleukin-2) [32].
The mathematical framework of these three processes have been developed by de Pillis et al. [33], Kuznetsov et al. [34], and Kirschner et al. [35] based on experimental data, and the predictions of the modelling have also been empirically validated [36]. These models effectively describe the computational dynamics of antitumor activity by DNA damage and immunological action. However, in all these models, the tumor cell population follows first-order biochemical kinetics and exponential asymptotic decay of tumor population with some residual malignant cells remaining, and complete eradication of all tumor cell fails to occur, and thus future relapse of the cancer lesion happens. In this study we aim to improve the aforesaid models using our procedure. The methodology of this paper can remove the aforesaid asymptotic cancer cell population, and enable eradication of all malignant cells, with permanent elimination of the tumor without any future recurrence.
It may be underscored that our analysis of the extinction of cancer cells is motivated from the well-known process of spontaneous permanent regression of tumors and its system biology evidence, whereby we have developed an approach of how one can replicate this tumor regression process in a clinical situation. We have provided substantiation of the proposed feedback approach by quantitative analysis of signal transduction pathway, gene expression level of G2/M-phase DNA damage pathway, IL-2 expression and T-cell receptor signaling. Finally, the translational aspects and validated corroboration of our approach is furnished, which enables the formulation of a guided controller-based treatment planning system governing the infusion of chemotherapy, interleukin, and antitumor T-cell immunotherapy, for complete extinction of the malignant lesion, cancer stem cell elimination and normal tissue protection. For a comprehensive understanding, Schema 1 summarizes our problem statement and our approach to it.
During exogenous tumor regression induced by therapeutic agents such as chemotherapy drugs that induce DNA damage, the reaction kinetics of tumor cell lysis is described by laws of mass action and first-order dynamics, hence the tumor cell population M decreases exponentially [9]. Likewise, in endogenous or spontaneous regression of tissue lesion or malignancy, the tumor cell population exponentially decreases with time [26,27,37]. For instance, in the former experiment [9], the proliferative cell activity is estimated by metabolic phosphorylation intensity, namely by ATP dynamics. Thus, the trajectory is M=M0exp(−εt), where ε, the rate parameter, is the intensity of the tumor regression effect (Figure 1(a)). Typically, a clinically just-detectable tumor has 107 malignant cells, and for regression in a reasonable time (say 1–2 months), this tumor cell population can decrease essentially to near zero (say 0.01 of cell population, or 10-4 of cell population), which can be enabled by using a suitable value of the rate parameter, induced by the regression process.
It may be noted here that there always persists asymptotically a residual tumor cell population under the trajectory and this can often be the factor of tumor recurrence. However, one can resolve the asymptotic issue and enable the tumor population trajectory to become zero at a definitive time point, using the principle of negative biasing by following a path of guided control [38,39]. Here the tumor cell trajectory exponentially approaches a negative value M*, indicating that the tumor cell population trajectory becomes zero at time tF (Figure 1(b)). This indicates that M=[(M0+M∗)exp(−εt)]–M∗, so that bias M∗=−M0exp(−εtF)/(1−exp(−εtF)).
Regarding exogenous regression (therapy-initiated regression) and endogenous regression (tissue-initiated regression), we have formulated a multimodal equivalence of the two regression processes from a systems biology perspective. Thereby, as Figure 2 elucidates, the three input terms to the tumor-host system (boxes on left side), respectively correspond to the entities of DNA damage or cell-proliferation blockage, tumor-infiltrating lymphocytes, and cytokine as interleukin. For a malignant tumor to undergo elimination, three complimentary processes for tumor cell reduction may need to occur:
(ⅰ) Decreasing the tumor cell proliferation: Proliferation of cells can be reduced by processes that enable DNA impairment, as by chemical alkylation or chemomodulation of DNA,
(ⅱ) Increasing tumor cell lysis, for example, by antitumor lymphocyte formation,
(ⅲ) Activation of these lymphocytes which can be enhanced by cytokines (for instance, immunomodulation by chemokines as interleukin-2).
Implementation of the aforesaid tumor regression processes can happen in two ways:
(a) Exogenous process, where the above three factors, DNA chemomodulation, antitumor lymphocyte formation and cytokine immunomodulation can be respectively induced by externally injecting alkylating chemotherapy drug, tumor infiltrating lymphocyte, and interleukin-2.
(b) Endogenous process, where the three factors are autogenously generated by the host tissue itself, such as, by DNA blockage, cytotoxic lymphocyte infiltration into the tumor, and interleukin-2 upregulation, respectively.
It should be mentioned here that the main route of DNA damage in both exogenous and endogenous tumor regression is by alkylation. For instance, in exogenous regression (e.g., by drugs), the DNA chemotherapy agents such as the most widely used pharmaceuticals as alkylators, function by alkylating the guanine moiety of DNA. Likewise, in endogenous regression (e.g., by spontaneous cancer regression), T-cell upregulation is a major factor, these cells secrete granzyme factor which activates Trex enzyme that induces DNA single-strand breaks at the 3'-portion [18]. It is known that such single-strand breaks at 3'-portion function onwards by alkylating the adjacent guanine moiety of DNA [9]. We have worked out the quantitative equivalence between the dynamics of spontaneous tumor regression and of therapy-induced tumor regression in Section 1 of the Supplement.
We now endeavor to develop a quantitative analysis of endogenous or exogenous tumor regression. Consider the milieu of the tumor's interaction, namely the populations of malignant tumor cells, natural killer cells, and circulating white blood cells (lymphocytes in the blood), which can be denoted respectively by M, K, B (Figure 3). Let C, A and D denote the intensity levels respectively of IL-2 (e.g., concentration), of Antitumor lymphocyte (e.g., T-lymphotyte infiltration population), and of DNA chemomodulation, namely, DNA interference in cells, e.g., concentration level of DNA-damaging moieties in tumor tissue (Figure 3), which can be gauged by the level of TNFα or by the level of DNA damage's checkpoint activation, or other procedures.
We here represent the interaction of the different cellular populations in terms of the flowsheet in Figure 4. The details of the derivations of this section are furnished in [15]. Using primed symbols to denote temporal rates or time derivatives, we can formulate the temporal dynamical model, specifically the rate of change of concentration of IL-2 activation, as given in the equations below.
(ⅰ) Interleukin:
![]() |
(1) |
where vC(t) represents the interleukin-2 formation in the system, and the interleukin degradation or elimination being according to the rate principle, i.e., proportional to its concentration, with µC being the decay rate. Similarly, we have the formulation of the rate of change of level of DNA interference:
(ⅱ) DNA damage:
![]() |
(2) |
where γ is the corresponding decay rate of the elimination or degradation of the DNA blockage factor. Likewise, we can formulate the dynamics of circulating white blood cells B:
(ⅲ) Circulating leucocyte:
![]() |
(3) |
where the leucocytes are generated at rate α from marrow, and they age with a death rate β, while the last expression (1 – e-D) is the saturation term denoting the fractional kill of these cells by the DNA blockage factor. After that, we formulate the natural killer cell dynamics:
(ⅳ) Natural killer cells:
![]() |
(4) |
Here, the last expression [KMn/h+MN] is a modified Michaelis-Menten type term, providing a saturation effect in cell-cell interactions, and g, h and n are three logistic constants (n ≈ 2 here). Furthermore, we consider another saturation effect, the intensity Q of the interaction between tumor cells (M) and antitumor lymphocytes (A). Using another three logistic constants d, s, and l, we can elucidate Q as:
(v) Tumor cell–Cytotoxic T cell interaction:
Q=d(A╱M)ls+(A╱M)l↓Saturationfunction(Tcell−Tumorcellinteraction:Michaelis−Menten(MM)type) | (5) |
The cytotoxic T-cell dynamics can now be delineated as:
(ⅵ) Cytotoxic T-lymplocyte (CTL) dynamics: CD8+ T-cells:
![]() |
(6) |
where mA represents T-cell death (senescence), and the last term vA(t) represents Tumor infiltrating lymphocyte formation rate. Here the terms are explained by the captions beneath; the right-side's second term [(QM)2/{k+(QM)2}] and second-last term [(pCAC)/(gC + C)], are saturation effects in cell-cell interactions. Lastly, we formulate the tumor cell dynamics as:
(ⅶ) Tumor cell dynamics:
![]() |
(7) |
It may be mentioned that the right-side of Eqs (1)–(7) has several cellular parameters (such as a, b…, α, β…), and their symbols, numerical values, significance and references, are given in Table S1 of the Supplement.
Note that DNA damage, whether endogenous or exogenous, induces lysis of the host's cellular populations: antitumor lymphocytes, natural-killer cells, and circulating leucocytes, the lysis rate parameters being respectively kM, kA, kK, kB in Eqs (3), (4), (6) and (7). However, tumor cells are more sensitive to the DNA damage lysis than other cells (kM > kA, kK, kB [33]). An important aspect here is that normal host cells should not be damaged appreciably and should be protected. This is taken care of by setting up limits that should not be crossed by the host system during the regression process since the host cell populations need to be maintained within a minimum and maximum value (upper and lower bounds). Too high a value of an entity can be toxic to the system, and the population of host cells that protect against infection (as circulating lymphocytes and natural killer cells) should be above a minimum value, whereby the normal tissue will be protected. The importance of the lysis parameters and limit thresholds for both endogenous and exogenous regression are described in Supplementary (Section 2.2). These values are displayed therein, in Table S2.
A fundamental prerequisite is that the regression process should take place in such a way that all the tumor cells are eradicated, but there should be least damage and cellular toxicity to the host. A measure of normal tissue damage due to the aforesaid antitumor entities (chemomodulation or immunomodulation) can be described by the standard toxicity cost functional J, wherein toxicity to the cell, a second-order mass-action effect [40], depends on second-power of the intensity-level of
J=1╱2(r1U21+r2U22+….) | (8) |
where U1 , U2, …. are the levels or effects of different antitumor entities, while r1 , r2 , …. are the weighting factors of each of the various entities. For instance, in the case of endogenous tumor regression, the effect level of immunomodulation U1 can be taken to be the intensity of tumor-cell lysis by cytotoxic T-lymphocyte, i.e., term Q in Eq (7), while the effect level of chemomodulation U2 can be gauged by the intensity of tumor-cells lysed by the DNA damage factor, i.e., the last term kM(1 – e–D) in Eq (7). We use this least damage principle to probe the conditions of tumor regression that would be optimal for the system, producing the minimal damage to normal tissue.
As the tumor undergoes permanent regression as per the formulation in Eq (7), we now endeavor to find out the temporally-varying activation level of the five associated factors: interleukin C, DNA damage D, circulating leucocytes B, natural-killer cells K, and antitumor lymphocytes A. In other words, we need to solve the Eqs (1)–(7) using the boundary conditions that these biological antitumor parameters (C, D, B, K and A) do not cross the physiological limits or bounds, see first paragraph of this section above and also the Supplement (Section 2 and Table S2).
Through solution of the Eqs (1)–(7), we find the values of the five antitumor factors and their alteration with time, which are necessary for inducing the tumor to follow the temporal trajectory of Figure 1(b), so that there is elimination of full tumor cell population at time tF, of that figure. Solving the equations, we have following pattern of activation intensity of the factors that together produce the complete tumor regression. The description of the equations are first mentioned below, and then information is given on their derivation. These equations are:
(ⅰ) Temporally-varying pattern of the activation level of DNA damage for tumor extinction:
D(t)‡=–ln[1–{bMgM1/rM1G}] | (9) |
where D(t)‡ indicates the required DNA blockage factor concentration in blood which enables the tumor to undergo complete regression by the extinction time tF, [the duration tF, is shown in Figure 1(b). Further, in the above equation, bM denotes the combined tumor cell-killing effect of DNA blockage factor and cytotoxic T-cell lymphocytes. If bM is negative, the tumor will have regression; if bM is zero, the tumor becomes stable in steady state; and if bM is positive, then the tumor is in progression phase. The parameters gM1 and gM2 are respectively the biological toxicity effect on normal tissue due to the DNA blockage factor and cytotoxic T-cell. The parameters rM1 and rM1 denote the toxicity weighting index respectively of DNA blockage factor and cytotoxic T-cell; these toxicity weighing indices are the weighing factors mentioned in Eq (8). The term G is the combined normalized toxicity weighting factor for both DNA blockage agent and cytotoxic T cells together, namely
G=[(g2M1/rM1)+(g2M2/rM2)] | (10) |
(ⅱ) Temporal pattern of cytotoxic lymphocyte activation for tumor extinction:
A(t)‡=–[sMl(bMgM2/rM2G)/{d–(bMgM2/rM2G)}]1/l | (11) |
where A(t)‡ signifies the desired cytotoxic T-cell population in the blood which enables the tumor to undergo complete regression by time tF. In Eq (1), the terms s, l and d are the tumor cell lysis parameters due to action of cytotoxic T cell on the tumor (explained in Table S1 of the Supplement). The parameters bM, gM2, rM2, and G have already been explained in the above paragraph.
(ⅲ) Temporal pattern of interleukin-2 activation for tumor extinction:
C(t)‡=gCbA/(pCArA1H–bA) | (12) |
where C(t)‡ represents the Interleukin-2 concentration in the blood which enables the tumor to undergo complete regression by time tF. In the above equation, gC denotes the steepness index of cytotoxic T cell recruitment by interleukin IL-2, and bA is the total cytotoxic T cell activation effect induced by DNA blockage factor and autocatalytically by cytotoxic T cell. The parameter pC is the maximum value of the rate of cytotoxic T cell recruitment by IL-2, and A represents the cytotoxic T-cell population. The terms rA1 and rA2 are the toxicity weighing factors denoting the toxicity effect on cytotoxic T cell due to both IL-2 and autocatalytically by cytotoxic T-cell respectively. The term H is related to the above-mentioned toxicity weighing factors rA1 and rA2, namely
H=[(1/rA1)+(1/rA2)] | (13) |
The details of the aforesaid derivations have already been explained in our previous work [15]. However, in that work, we had neither formulated nor provided negative bias, and so there were some amount of residual malignant cells persisting. In contrast, in this present report, we have furnished our novel methodology of negative bias, and we thereby show that all malignant cells become extinct in the desired duration. Furthermore, our previous publication [15] was a mathematical derivation paper, there was absence of any biological, tissue-based, clinical or molecular biology analysis. In the current report, we have satisfactorily given all these biological and clinical findings for enhancement and validation of our new approach of tumor eradication under the novel approach of negative biasing.
The parameters bM, bA, gM1, gM2, G and H depend on the value of the tumor characteristics (M, kM , kA) and on the toxicity-minimizing indices (rM1, rM2, rA1, rA2,) [15]; the other parameters in the equations (s, l, d, gC, pC) depend on the tumor growth and tumor lysis characteristics, having the values as in [33]. The temporal pattern of the circulating leucocyte population B and natural-killer cell population K, can be found by respectively solving Eqs (3) and (4), where we use the values of the relevant parameters which are also available [33]. The tumor cell population can be obtained as follows. We substitute these values of C(t), D(t), and A(t) into Eqs (1), (2) and (6) and thus arrive at the values of Circulating leucocyte, NK-cells, and Cytotoxic T-cells. Then we put the latter three values in Eq (7) and thus obtain the value of the tumor cell population M(t).
The quantitative formulation of our simulation model of endogenous or exogenous tumor regression is explained in Figure 5. We have used a Matlab platform to compute the profiles of the three entities (DNA damage, cytotoxic T-cell, and Interleukin-2), using tuning parameters values (rT2 and rL2) described in [15]. [Note that DNA damage events of endogenous regression are estimated in terms of equivalent alkylation activity of an alkylator agent (units of dacarbazine equivalents), as per Supplementary (section 2.1)]. For the initialization of the model, we have to provide initial intensity value of all the different cellular populations, i.e., their values at time point t0 (at the beginning of tumor regression). After that, we need to provide all the biological parameters used in Eqs (1)–(7) [33]. Thence, we need to calculate the negative bias value (M*) and set the tuning parameters (rT2 and rL2). The tuning parameter rT2 is used to calculate the control parameter vA and vD, and the tuning parameter rL2 is used to calculate vC [15].
Here we have divided the profile of the three entities (DNA damage, T-cell, and IL-2) in 4 loops (M - Loop, A- Loop, D - Loop and C - Loop) as elucidated in [15]. M-Loop is again divided into 3 loops M1- Loop used to calculate value of UA, UD, A*, and D*, M2- loop is for the calculation of UA and A*, and M3-loop is for UD and D* (note that here UA and UD indicate the anti-tumor behavior of cytotoxic T-cell and DNA damage respectively). Similarly, A-loop is again divided in 3 loops; A1- Loop is used to calculate value of UC, vA, and C*, A2- loop is for the calculation of UC and C*, and A3-loop is for vA calculation alone (here UC denotes the anti-tumor behavior of IL-2). At last, the D-loop and C - loop is used to calculate vD and vC dose rates according to Figure 3. In other words, initially, the curve of tumor decreases and extinction is formulated by allotting a desired suitable extinction time tF (this gives the negative bias M*, Figure 1(b)). Then the modelling is performed, whereby the aforesaid methodology and algorithm above will simulate the tumor regression process, so that the tumor cell population follows the extinction process of Figure 1(b). The moment the simulation gives the tumor cell population as a (small) negative value, the procedure will convert the negative value to zero (the tumor cells have become extinct before attaining a negative value). From this time point onwards, the simulation procedure will maintain the tumor cell population at 0 henceforth, and so there will be no tumor relapse. Thus, the simulation process will give (for each time step) the levels of DNA damage, cytotoxic T-cell, and Interleukin-2, whereby these three entities jointly gradually eradicate the tumor cell population by time tF.
The mathematical model is verified on immunohistochemical experiments and microarray assay of a preclinical model of permanent spontaneous tumor regression in melanoma. Here, numerous tumors appear in pigs, they grow rapidly for the first 1½ months, and then spontaneously regress completely by 3–4 months in half of the animals, but in the other half, the tumors spread and kill the animals [24]. In the study, six pigs who went into the regression mode, were investigated, tumors had biopsy under anesthesia at three weekly intervals across three months, at these five different time points as follows, t0 = day-of-birth (d) + 8 days after birth (i.e., d + 8), thence t1 = d + 28 days; t2 = d + 49; t3 = d + 70, and t4 = d + 91 (Schema-2). At each time point 5–6 tumor-masses were biopsied, and subjected to microarray investigation using Ingenuity algorithm.
(ⅰ) Pre-clinical investigation
Regarding the aforesaid melanoma regression analysis, we accessed the gene expression profiles of E-MEXP-1152 from the ArrayExpress facility (https://www.ebi.ac.uk/arrayexpress/) [41]. The microarray assay was based on Transcription profiling of tumor from the melanoblastoma-bearing Libechov Minipig (MeLiM) model and the Porcine Genome Arrays platform. This involved 5 different tumors of 5 pig siblings, each across 5 time points, from day 8 to day 91 after birth (Submission date: September 10, 2008). Also, the time-dependent gene expression profiling of the spontaneously regressing melanoma tumor biopsies was performed. Further, from the ArrayExpress platform, we analyzed all the raw information CEL-Files (E-MEXP-1152.raw.1.zip) belonging to this experiment (https://www.ebi.ac.uk/arrayexpress/experiments/E-MEXP-1152/).
(ⅱ) Normalization and statistical analysis
We then performed normalization, statistical analysis and the analysis of variance (ANOVA) of the microarray information, using R platform and Bioconductor statistical facility (http://www.bioconductor.org/) [42]. We did the preprocessing step utilizing the GeneChip Robust Multiarray Average (GC-RAM) method to get the gene expression matrix from Affymetrix information. For identification of differentially expressed gene in log2 scale, we used the Transformation for t-test and one-way ANOVA. Thence, differentially expressed Probe sets were selected on the bases of p value and fold change (FC) value [(FC) > 2 and p value < 0.05]. Moreover, the significant probe sets after ANOVA were used for biological functional analysis and comparison.
(ⅲ) Time dependent biological function analysis using pathway study
We then identified the temporal profile of the microarray data for the different Biological Classes category, utilizing the Ingenuity Pathway Analysis procedure (IPA). We also elucidated the expressional changes at each time-point, the functional interpretations, and the biological interaction of the genes, which were also demarcated using IPA. This furnished the temporal pattern of gene-expression levels corresponding to the effector components of Figure 4, such as (i) DNA damage (G2/M checkpoint regulation), (ii) Antitumor lymphocyte activation (T-lymphocyte Receptor Signaling), Natural Killer cell activation (NK signaling), etc. We also clustered the genes corresponding to different canonical pathways utilizing IPA methodology, out of these genes we identified the most significant genes with high FC-value.
We first obtained the inferences from the computational modelling analysis developed above. Then we furnished experimental findings from the preclinical studies, to validate the theoretical quantitative formulation.
We have used the MATLAB platform to numerically solve the Eqs (1)–(7), and find the values of these parameters and their alteration with time, while the tumor follows the temporal trajectory of Figure 1(b); the complete flow-chart is shown in Figure 5 and the simulation has already been described in the "Materials and methods" Section, item 2.5.
Here we deal with an indicative case of melanoma. Melanoma is a neural crest cell tumor and shares many similarities with common neural system tumor as glioma. We can take the initial realistic situation as starting malignant cell population (T0) of 2 × 107 cells, natural killer cell population of 105, cytotoxic T-cell population of 5 × 104, and circulating lymphocytes of 109. These realistic values are adapted from an earlier analysis of tumor dynamics [33]. The number of tumor cells corresponds to a realistic tumor which has just been radiologically detected, say a metastatic melanoma mass in the liver (radiological detection threshold ≈ 1 cc. tumor, having 107 malignant cells [43]). All the other constants used in the model are given in [33], with the tumor cell growth rate, a = 0.301 per day, and the deceleration rate of logistic tumor growth b = 1.01 × 10-8. Here, we have considered a more aggressive melanoma tumor, so the tumor cell growth rates a can be taken to be 50% higher of the aforesaid a value (i.e., new value of a is now 0.43).
To be able to compare endogenous and exogenous regression in a common platform, we need to estimate the DNA blockage in both endogenous and exogenous tumor regression using a common unit, we have formulated this common unit as an equivalent unit of alkylation blockage activity (i.e., in terms of alkylation activity of equivalent amount of chemotherapy dacarbazine which will produce the same number of DNA blockage events either in exogenous or endogenous regression of tumor) (Section 2.1 of the Supplement).
(ⅰ). Tumor regression under conventional therapy (without Negative Bias): Tumor Relapse
We now formulate the case of a conventional protocol of chemotherapy and immunotherapy of melanoma, utilizing (ⅰ) Chemotherapy: Six pulses of chemotherapy, one every 10 days (dacarbazine 5 mg/kg/day pulse), (ⅱ) Interleukin-2: Six pulses of 500,000 i.u/kg/day, from day-8 to day-11. (ⅲ) Cytotoxic T-cells: Total 109 cells during an infusion at day-7 through day-8. We have applied these values to the initial Eqs (1)–(7) which are solved to obtain tumor cell population M as time elapses. Figure 6 shows that the tumor cell population initially decreases to about 100,000 malignant cells, but later manifests as cancer relapse and increases to high values (≈109 malignant cells) that corresponds to a large tumor of diameter ≈6 cm., such a tumor will have penetrated the blood vessels, producing wide dissemination and lethality.
(ⅱ). Tumor regression by negative bias formulation: Permanent tumor elimination
Here we have used the different sets of Eqs (9), (11) and (12), which give the levels of the three entities (DNA damage, cytotoxic T cells, interleukin-2) that are required to enforce the cell population of the melanoma tumor to follow the exponentially declining trajectory to zero cell at a specific desired duration (46 days). We now simulated the equations at a time-step of a 0.01 second, and obtain the values of the levels of DNA damage, Interleukin-2, and Cytotoxic T-cells, which would enable the tumor population to follow the targeted diminishing curve trajectory of Figure 1(b), aimed at tumor extinction in 46 days. These levels of the above three entities are then used to obtain the tumor cell population (using the other set of Eqs (1)–(7)). Therein, the populations of the circulating leucocytes and natural killer cells are also calculated. Note that the actual tumor cell population obtained (Figure 7(a)) follows in principle the desired exponentially decreasing trajectory that we planned, whereby all tumor cells undergo eradication. Note that none of the system parameters (DNA damage level, circulating lymphocytes, NK cell, cytotoxic T-cells) crosses the respective upper and lower physiological bounds or thresholds of Table S2 (Supplement), thereby ensuring normal tissue protection. Here, we found the negative bias to be M* = 1.8986 × 105 cells. Since the total number of initial tumor cells M0 = 2 × 107 cells, we observed that the relative value of M* is small, at about 1% of the initial tumor load.
(ⅲ). Behaviour of cancer stem cells, natural killer cells and circulating lymphocytes
From Figure 7(e), (f), we see that the population of circulating lymphocytes and natural killer cells in the body attain the values respectively of 6 × 1010 cells and 3 × 105 cells, which are very much below their corresponding upper bounds, and are only about 10 and 0.001% of the respective upper bounds in Table S2 (Supplement). Furthermore, it is known that cancer stem cells become much resistant to anti-tumor drugs as they have drug efflux channels which ejects out the drugs from the tumor cells. For instance, the sensitivity of the cancer stem cells to a drug can become 8–23% of the sensitivity of general cancer cells to that drug [44], this drug sensitivity of tumor cell is the parameter D in Eq (2). Hence, to formulate cancer stem cells in the model, we decrease the drug sensitivity D to 1% of the value that we have used in the earlier paragraph, this very low value of D has been put as a precautionary measure. We performed the simulation of the earlier paragraph again, and we observed that there is also complete tumor eradication (Figure 8), though a somewhat longer therapy duration is needed (59 days). Thus, our negative bias procedure can successfully eliminate the cancer stem cell base in the tumor, though the time taken is a little longer.
(ⅳ). Adaptability and robustness of tumor elimination process
In real-life conditions, different patients can have different initial conditions and constitutions. Hence, different patient-specific initial situations are now considered, for example, we examined the different initial condition of immunological system (like populations of the different effector cells) and carried out 500 arbitrarily simulations for complete tumor elimination for melanoma tumor with different biological parameters or characteristics (Figure 9).
We found that tumor extinction occurred in 100% cases if the coefficient of variation in effector cell population was 0%, while extinction happened in 98% of the cases if the coefficient of variation was increased to 10%. It has been known that the physiological parameters are generally kept constant homeostatically by organisms, with a 10% variation around the mean level [45]. Thus, within the considered range of physiological variation, it transpires that our proposed approach may be able to induce tumor elimination in the majority of the cases (98% of cases).
(ⅴ). General characteristics of tumor regression process
We have simulated the permanent tumor regression process using different values of the tumor parameters, and we have always observed the similar type of patterns as in Figure 7. This general pattern is exhibited in Figure 10, whereby we note that for inducing permanent tumor regression, the three antitumor entities should have three distinct temporal profiles (tri-phasic activation):
1) Bimodal intensity for lymphocyte activation, showing two temporal peaks (Figure 10 (a)),
2) Unimodal intensity for activation of DNA damage (such as strand blockade or alkylation), displaying one peak temporally (Figure 10(b)).
3) Stationary intensity for cytokine activation (interleukin-2) exhibiting uniform level (Figure 10(c)).
(ⅵ). Basis of the tri-phasic activation
The three aforesaid activational phases can be accounted for from a dynamic perspective of Figure 11. Initially, in the tumor cell surroundings, there occurs tumor cell antigen binding to T-lymphocyte receptor, stimulating the secretion of IL-2 (event-1). Thereby, T-cell is activated to cytotoxic T-cell (event-2). This T-lymphocyte then counters a tumor cell, secretes granzyme protease, which binds to tumor cell DNA, cleaving the nucleic acid binding protein, blockading the DNA and damaging tumor cell DNA replication (event-3). Note that in event-1, since there are no second-order deceleration terms nor Michelis-Menten terms in Eq (1), the interleukin level can rise rapidly or steeply, nevertheless the increase of interleukin halts or saturates as soon as the interleukin toxicity level or upper bound is reached.
The aforesaid three sequences of events are clearly reflected in the three temporal profiles above. The first event corresponds to Interleukin-2, the earliest agent to increase (Figure 10(c)). Then, the second event correlates with cytotoxic T-cell increase, but sometime later (Figure 10(a)). Thereafter, the third event relates to the increase of tumor cell's DNA damage intensity, which happens still later Figure 10(b). Here, tumor cells are increasingly lyzed, and their debris thus produced deactivates the cytotoxic T-cells whose activated population hence decreases, this corresponds to the negative term "-qAM" in Eq (6). As tumor cell population continues to decrease, its debris production falls, so that the negative term becomes smaller, whereby cytotoxic T lymphocyte population increases again (second temporal peak) (Figure 10(a)).
We may recollect that the step-like shape and stationarity in the interleukin-2 concentration is due to its level being initiated rapidly at the requisite intensity (Figure 10(c)). Such a brisk interleukin response has also been independently observed in the immunological system, where requisite stimulation can enable interleukin-2 receptor activation to reach substantial intensity within 2-4 days [46]. The result is that a stationary level of interleukin activation is rapidly attained, and tumor regression occurs. Indeed, it is well known from clinical experience [32], that interleukin-2 administered at significantly augmented dose, induces long-lasting immunomodulation to act against those residual malignant cells that bypass usual therapeutic intervention.
We now furnish experimental findings of complete permanent tumor regression that provide substantiation and corroboration to our mathematical theoretical model of complete elimination of tumor by the five-pattern temporal profile (Figure 10), namely the activation of antitumor lymphocyte (bi-modality), DNA damage or impairment (unimodality), interleukin-2 (stationarity), circulating lymphocyte (saturation) and NK cell (saturation).
(i) Melanoma microarray data preprocessing:
The microarray raw data of file of the spontaneous regression of malignant melanoma tumor was downloaded from the ArrayExpress system (E-MEXP-1152) and analyzed using the Bioconductor package on the R platform. Furthermore, one-way ANOVA was performed for five different time points (taking time t0 as the reference) to find the differentially expressed genes based on p value and FC value (p value < 0.05 and FC > 2). The ANOVA analysis results showing the differentially expressed genes (DEGs) is displayed in Figure 12.
(ii) Identification of signaling pathways:
Permanent endogenous melanoma regression microarray data of pigs were investigated by IPA. We assessed the antitumor T-cell activation intensity by the level of the IPA's T-cell pathway, named "PD-1/PD-L1 cancer immunotherapy pathway". Similarly, we gauged the DNA impairment level by the IPA pathway "G2/M DNA Damage Checkpoint Regulation". Likewise, we estimated the Natural-Killer cell level by IPA pathways "NK cell signaling". Furthermore, we assay the Circulating lymphocyte level by the IPA pathway ("Leucocyte extravasation signaling"); indeed, one knows that during immune response, the extravasation of circulating leucocyte through the vascular wall into tumorous tissue, correlates with activation of circulating lymphocytes [47].
Thus, we found the specific temporal behaviour of the activation levels of cytotoxic T-lymphocytes (Figure 13(a)), DNA impairment (Figure 13(b)), circulating leucocytes (Figure 13(c)), and natural killer cells (Figure 13(d)). It is evident that the first and second curves (Figure 13(a, b) follow respectively the bimodality and unimodality pattern of Figure 10(a, b). Also, the third and fourth curves (Figure 13(c, d)) both follow the saturation pattern of Figure 10(d). Thus the four experimental curves of Figure 13 closely correspond to the mathematically predicted patterns of Figure 10.
To show that our theoretically calculated model of tumor regression (Figure 7) adequately describes the experimentally observed tumor regression behaviour (Figure 13), we used the goodness-of-fit criterion (Kolmogorov-Smirnov test). Accordingly, we found that the experimental graphs of panels (a)–(d) of Figure 13 are satisfactorily accounted respectively by the corresponding theoretical graphs of panels (b, c, e, f) of Figure 7, and the corresponding goodness-of-fit Kolmogorov-Smirnov's statistical test was satisfied (α = 5%).
Now, we arrive at the molecular biological basis of aforesaid biological entities involved in permanent endogenous tumor regression. Using genetic analysis, we also found the genes associated with these different entities, which we elucidate below. Table 1 (left three columns) summarizes the main aspects of our findings.
Characteristic function predicted by mathematical model | Biological entities involved | Biological basis of the characteristic function | Exemplars of genes involved in the antitumor characteristic | Illustrative findings |
Monophasic Activation (DNA blockage) | DNA. Chemomodulation: Cell multiplication blockage. | Build-up and then decay of DNA chemomodulative or blockage activity. | Cell kinase gene: CDC2, CHEK1 Cell cycle gene: CCNB1, CCNB3 | Figure 7(c), Figure 13(b) |
Biphasic activation (Cytotoxic T-cell) | Lymphocyte enhancement: Lysis of tumor cells. | Second rise (biphasicity) in T- cell population due to decline of chemomodulation, i.e., decrease in blockage of T-cell growth. | T-cell receptor activation: TRGV5 gene, CD28 gene. G-protein coupled receptor activation: CALCR gene, CBLB gene. | Figure 7(b), Figure 13(a) |
Uniform Stationary activation (Interleukin-2) | Cytokine enhancement: Escalating the leucocyte-tumor cell interaction. | Toxicity limit of cytokine (homeostasis). | Interleukin-2 receptor gene: IL2RG, AKT3 gene. Transmembrane protein: CD74, GRB2 gene. | Figure 7(d), Figure 14(a) |
Saturating Activation (Natural killer cell) | Natural killer cell function (normal tissue protection). | Equilibrium state after Tumor regression. | NK cell activator gene: NKG2D gene, STAT4 gene Actuates NK cell function activators: KLRK1 gene, MAP3K12 gene. | Figure 7(f), Figure 13(d) |
Saturating Activation (Circulating lymphocyte) | Circulating lymphocyte function (host defense). | Equilibrium state after Tumor regression. | Chemokine ligand 1 activity: TCA gene - Actuates lymphocytes & monocytes. Rantes ligand activity: CCL5 and PRKCB genes - Actuates lymphocyte & monocytes. | Figure 7(e), Figure 13(c) |
Negative biasing | Apoptosis pathway | Elimination of residual tumor cells. | Extrinsic apoptosis pathway activator genes: CASP7 gene, GZMB gene. Intrinsic apoptosis pathway activator gene: BCL2L1 gene | Figure 1(b), Section 3.3.1 (ⅳ) |
(ⅲ) Identification of genes
We found the signaling pathways and related genes for spontaneous regression using IPA. We selected the gene based on FC-value from the cluster of gene obtained from IPA, for different biological classes. Accordingly, we have furnished below the two lead exemplar genes for the antitumor functions as follows (also see Table 1, right two columns):
(a) DNA Damage-related genes: CDC2, CHEK;
(b) Interleukin-2 signaling-related genes: IL2RG, AKT3;
(c) Natural-killer cell signaling-related genes: NKG2D, KLRK;
(d) Cytotoxic T-cell activation-related genes: TRGV5, CD28;
(e) Circulating lymphocyte activation-related genes: TCA, CCL5.
(iv) Negative bias related genes: CASP7, GZMB.
Now, for identifying the genes that function as effecting the negative bias in tumor cell reduction trajectory, we have selected the genes which are responsible for the apoptosis process of the tumor cells, namely the Perforin/Granzyme apoptosis pathway activator genes: CASP7, GZMB. In other words, the pathway is a serine protease pathway, which is a primary signaling route used by cytotoxic T cells and natural killer cells to eliminate virus-infected or mutated malignant cells [48]. Cytotoxic T cells have perforin, which is a pore forming complex, while Granzyme B (GZMB) is an apoptotic signaling molecule that is able to initiate apoptotic signal by the process of exocytosis. After getting into the mutated malignant cell, this GZMB molecules interact with BID protein of the cells, which is actually the BH3 interacting domain death family protein that initiates the apoptosis process by activating caspase 7. As shown in Table 2, the expression values of these two genes (CASP7 and GZMB) increased from time point t1 through t4, while the tumor regression process advanced with time and this regression process was maximum at t4 time point (where the tumor has completely regressed and become extinct). Indeed, the aforesaid proteins actuated by these two genes were able to attack and lyze all the residual tumor cells in the last time segment (t3–t4), thereby preventing tumor relapse.
Gene Name | Log2FC value (at time t1) | Log2FC value (at time t2) | Log2FC value (at time t3) | Log2FC value (at time t4) | p. value | F | Average Expression |
GZMB (Granzyme-B) | −0.07314 | 0.525236 | 1.124219 | 2.326586 | 0.00011 | 10.0495 | 6.086244 |
CASP7 (Caspase-7) | −0.02369 | 0.12626 | 0.570303 | 1.488125 | 0.03849 | 4.8372 | 7.215214 |
Here complete endogenous regression of malignant histiocyte tumor in rodent system was analyzed. The experimental investigation is available, along with biochemical parameters [49]. In Figure 13 we delineated the temporal behaviour of the relevant parameters, which we have calculated from that initial study. The experimental study was done for 32 days, and two types of tumor regression regimes were observed: (ⅰ) Early regressor animals, where tumor size increased up to the 10th day, then tumor regression occurred, (ⅱ) Late regressor animals, who display tumor size increased till about the 20th day, and tumor regression started thereafter [49]. Hence, to observe the tumor decline process for a substantial duration, we analyzed the findings of the first group, where regression deviation was observed longer, namely across 22 days (in the second group, the regression duration was much shorter, only for 12 days). We note that the experimental graphs showing temporal profile of interleukin-2 and DNA impairment (Figures 14(a, b)) are satisfactorily described by the corresponding theoretical graphs predicted (Figure 7(d, c)), the Kolmogorov-Smirnov statistical test of goodness-of-fit being also satisfied (α = 5%).
We have developed a quantitative mechanistic formulation and analysis of the permanent spontaneous regression of the malignant tumor, with experimental validation and its clinically-relevant implications. This extinction of tumor cells is possible due to a negative biasing process. We have delineated the temporal profile of the causative factors that enable the complete extinction of the tumor, namely:
(ⅰ) the three separate activation characteristics (unimodal, bimodal and uniform stationary activation function) respectively of DNA damage or mitosis blockage, cytotoxic lymphocyte and cytokine IL-2
(ⅱ) the two separate saturation activation profiles of both the natural killer cells and circulating lymphocytes.
This multiphasic temporal orchestration of the antitumor entities minimizes toxicity to normal tissue, without damaging the usual host tissue. Furthermore, we have also obtained experimental corroboration of our theoretical mathematical formulation of the process of permanent regression of tumor, whether spontaneous regression of tumor or therapy-induced regression of the tumor. We now elaborate the clinically-pertinent implications of the research.
An important aspect of the methodology developed is survival and safeguarding of normal tissue, while at the same time the tumor lesion is being eliminated. Taking care of these two contradictory objectives, our procedure performs the following two contrasting functions:
(ⅰ) Minimization of toxicity to the normal tissue due to the antitumor entities: This is done by developing a toxicity cost parameter which is kept minimal using standard Lagrangian method.
(ⅱ) Keeping the concentration of antitumor entities within tolerated ranges: This is done by using physiological limits to the level of DNA damage, cytotoxic T-cells, IL-2, circulating lymphocytes and NK cells.
A major handicap in oncology is that often treatment (chemotherapy and radiotherapy) is started vigorously [50,51], but soon the intervention has to be stopped or downscaled due to the toxicity to normal tissue. Thus, in the latter stage, the tumor proliferates and spreads out through vascular metastasis. In the clinical scenario, an important aspect is that the therapeutic agents may cause considerable damage to normal tissue, such as tissue inflammation and infection, since the normal immunological balance and homeostasis has been impaired due to the drug-induced lysis of the circulating lymphocytes and natural killer cells whereby these cell populations fall below normal protective range. This hazardous condition does not occur in our formulation, since the antitumor entities (or therapeutic agents) and their toxicity functions are always kept within biological bounds, such that the immunological cells (circulating leucocytes and NK cell levels) are always maintained within normal limits, thus protecting against infection and inflammation.
As is well-known, a critical factor responsible for the failure of tumor containment is the presence of a small side-population of cancer stem cells, which are distinct from the usual cancer cells. Conventional antitumor intervention may act well on the usual cancer cells (the majority part of tumor tissue), the tumor may shrink and become clinically undetectable. However, the small population of cancer stem cells are far more resistant to antitumor drugs since they have drug ejecting efflux-channels, so that these cells survive the drug effect and proliferate continuously, and the tumor soon recurs and disseminates [52]. For instance, the sensitivity of these cancer stem cells to antitumor drugs are only 8–23% of the sensitivity of usual cancer cells to the drug [44].
On the other hand, our negative biasing procedure can successfully eliminate cancer stem cell based tumors, though the time taken is longer than eliminating usual cancer cells. This indicates that more DNA damage induction (i.e., DNA-damaging drug agent) would be needed for lysing the cancer stem cells which are more chemotherapy resistant. However, it may be noted, that there are no normal tissue toxicity issues in our cancer stem cell elimination process, as the physiological bounds are always being maintained. Furthermore, our procedure is robust enough (Figure 9), that is all the malignant stem cells are eliminated even if there is appreciable patient-specific variation in the tumor cell population.
A critical element enabling complete tumor regression is the bimodal activation of antitumor lymphocytes. The requirement of such bimodal activation, one peak each in the initial and in the later phases of the regression process, is corroborated by the experimental studies presented here. In conventional multimodal therapy (Figure 6) where often temporary but not permanent regression occurs, here the T-cell immunotherapy is administered usually at a week's time (day 7–8), and the second peak in T-cell population is absent. We can construe that the second T-cell peak is essential to completely eradicate the residual malignant cells in the late stage of the regression process. Just before the occurrence of the second peak, the tumor cell population is below 5% of the initial tumor population (Figure 7(a)), i.e., the vast majority of the tumor cells has already been eliminated by that time, nevertheless a second T-cell peak is needed to eliminate the residual tumor cells to prevent relapse. As we have shown, the second peak can be therapeutically induced by suitable dosing of the tumor infiltrating lymphocyte injection from the first day itself (Figure 13(a)), instead of having the week-long delay that customary therapy prescribes. The lack of the second T-cell dosage peak might be a factor why in the well-known clinical trial of the T-cell therapy [53], one observes that the majority of patients (54%) showed no response, while the others showed only a partial response with tumor somewhat shrinking yet remaining extant, a portent of future relapse.
Our cytotoxic T-cells (CTL) immuno-modulation used is of tactical utility, as these cells can exhibit [54] a range of unique behaviors, that chemotherapeutic drugs cannot, such as:
(a) the T-cells can migrate to the primary and secondary growths of the tumor, even in hidden tissue depths,
(b) CTLs can continue to automatically multiply in response to immunogenic proteins of malignant cells, until all those tumor cells become extinct,
(c) T-cells enable immune memory to be stored, allowing further elimination of the tumor, if there is recurrence.
Though our proposal of doublet pulse therapy has not been earlier used in oncology, a doublet pulse approach to cytotoxic therapy has been satisfactorily used in other cell proliferation disorders, as Wegener's granulomatosis [55]. Hence, our proposed double pulse lymphocyte activation procedure may hold appreciable potentiality in the clinical oncology scenario.
Utilizing a systems analysis methodology, our investigation endeavors to elucidate a general unitary basis of tumor regression, which can be applied to both processes: (a) endogenous or spontaneous regression (b) exogenous or therapy-induced regression. Our investigation has formulated that the basic dynamics of both regression processes are comparable and equivalent, and consists of three aspects: (ⅰ) DNA interference in malignant cell, (ⅱ) cytokine-based activation of tumor tissue environment (IL-2), and (ⅲ) actuation of antitumor white blood cells (lymphocytes). The paradoxical phenomenon of spontaneous tumor regression has intrigued physicians since the time of St. Peregrines, the patron saint of cancer, these inquirers pondered how the process could be therapeutically replicated on patients, though now the process is known to be much ubiquitous, as the Wisconsin [5] and Scandinavian [4] cancer population registries show.
To analyze the spontaneous cancer regression phenomenon more precisely, we have quantified the regression behaviour in terms of numerically-based DNA interference sites across the tissue (section 2.1 of Supplement). This same DNA interference formulation was shown to apply to drug-induced tumor regression, delineating the unitariness of the two processes. To paraphrase, our investigation of the spontaneous tumor regression process can help design newer modalities of therapeutic regression or treatment. Actually, our approach using three antitumor agents does notably satisfy the requirements needed for mimicking the occurrence of spontaneous tumor regression, as analyzed from the immunoediting perspective. From this immunoediting analysis, the requirements for spontaneous regression are (ⅰ) lymphocyte activation (ⅱ) cytokine/interleukin-based activation of antitumor cells (ⅱ) tumor DNA interference using microbial metabolites [56].
Our model applied for both endogenous and exogenous tumor regression, the latter implying tumor regression under therapy. Hence our formulation has incisive implications for clinical treatment. A seminal aspect of our formulation is the incorporation of feedback approach (Figure 4), whereby the antitumor entities (DNA damaging entity, interleukin-2, and lymphocytes) are primally and accurately varied with time, such that the tumor cell population undergoes extinction in the specific time duration (around 40–60 days), by following an optimum natural exponentially decreasing trajectory (with negative bias, Figure 1(b)). In other words, this declining curve furnishes the guidance for a trajectory which needs to be followed by the tumor cell population to reach a population of zero in the definitive time period. During the simulation process, after suitable time interval (time-step), one updates the tumor cell population which has declined a bit in the earlier time interval due to the action of the antitumor entities (Section 2.5 and Figure 5). This updated tumor cell population is used (at the next time-step) to determine the new values of antitumor entities, which are implemented iteratively in the simulation loop, and the tumor cell population declines a further bit, so that across successive time intervals, the tumor cell population follows the exponentially decreasing curve to extinction.
As time progresses, and if at any point, there is any incongruence of the actual tumor cell population from the mathematical tumor cell population of the exponential curve, the feedback control system (Figure 5) acts by altering the values of the antitumor entities, so that the error is corrected, and the trajectory of the tumor cell population is also corrected, thereby following the exponential curve. The feedback control approach is in marked contrast to the customary approach in clinical oncology, where the antitumor entities are given in fixed-dose planned out beforehand, and the dosages do not adapt to the variable patient response, and neither to the varying tumor load day-to-day. There were earlier attempts to use feedback control system for antitumor therapy [57] but, as far as we know, they have not been on the lines proposed here, namely trajectory guidance control that enforces the tumor system to persistently reach the target (zero malignant cell population), with inbuilt ability for error correction and adaptation.
We can now clarify the modus operandi for clinical applications. We may formulate the generalization of the tissue-induced process of endogenous tumor elimination, so as to develop the therapy-induced process of exogenous tumor regression. For permanently eliminating the tumor, Eqs (9), (11) and (12) respectively provides the required time-varying profile of the intensities of the three entities in the tissue: (ⅰ) DNA damage, D(t)‡, (ⅱ) cytotoxic lymphocyte population A(t)‡, and (ⅲ) cytokine interleukin-2 concentration, C(t)‡. In endogenous tumor regression, these levels are generated intrinsically by the host tissue, such as by cellular metabolism and gene activation/deactivation as seen in our analysis of melanoma regression (Figure 12). Likewise, for clinical applicability on patients, we need to induce the exogenous tumor elimination by externally administering therapeutic agents that would produce the requisite temporally-altering levels of the aforesaid entities (ⅰ)–(ⅲ), namely the three agents would be a chemotherapeutic agent (e.g., dacarbazine, or cyclophosphamide etc.), cytotoxic T-cells, and interleukin-2 preparation (Figure 2). Each of these agents can be given by time-varying continuous intravenous infusion by an injected fluctuating dose-rate function, vD(t)‡, vA(t)‡ and vC(t)‡ (Section 2.5), which can be readily calculated from the required levels of the three entities in the tissue D(t)‡, A(t)‡ and C(t)‡ (Figure 5 and Section 2.5). Thus, if those temporal dose-rate patterns of the three therapeutic agents are injected, then the levels of those agents in the tissue would be D(t)‡, A(t)‡ and C(t)‡, whereby the tumor cell population would follow the trajectory in Figure 15, becoming extinct at time tp days, with no tumor recurrence nor toxicity to the patient.
In retrospect, our approach, validated by experimental findings, shows that a robust quantitative systems biology formulation can be developed to obtain incisive mechanistic insights into the process of complete permanent tumor regression, which often occurs naturally in the form of endogenous spontaneous regression as noted in cancer registries of general populations. Alternatively, the tumor regression process can be replicated therapeutically by antitumor agents, as chemotherapy and immunotherapy. The salient feature of our formulation is that the regression is enabled by very specific but universal characteristics of the antitumor entities, namely single peak level of DNA damaging factor, double pulse level of white blood cell activation (T-lymphocyte), and uniform activation level of the immunomodulator cytokine (IL-2). Indeed, the second pulse feature of lymphocyte activation is an unexpected finding and accounts for the complete extinction of all the residual tumor cells which is in the order of 1% of the initial tumor load. The absence of this second pulse of lymphocytes in customary multimodal therapies may be a factor that prevents these therapies to induce lasting tumor eradication, and one often observes tumor relapse in these cases. Our proposed formulation does not have high-intensity levels of any of the therapeutic agents for a prolonged time, their levels can become much less at intervening times, and there is no appreciable drug-induced toxicity as the immune system (circulating lymphocytes and natural killer cells) are kept always protected. Thus, it can be suggested that combinational multi-pulsed multimodal therapy, using systems biology based analysis, can offer a principled approach to permanent tumor elimination, with germane implications for the clinical oncology scenario.
Bindu Kumari is thankful for the student opportunity furnished by Indian Institute of Technology–Banaras Hindu University, Varanasi. We are thankful for the facilitation extended by iHub NTIHAC Foundation, sponsored by Department of Science and Technology, Ministry of Science and Technology, Govt. of India. For providing research facilitation, Prasun K. Roy is grateful to Shiv Nadar University, his mailing address being Dept. of Life Sciences, R-206, Shiv Nadar University, Dadri, 201316, India.
The authors declare that they have no conflict of interest.
[1] | W. Cao, X. Wang, Z. Ming, et al., A review on neural networks with random weights, Neurocomputing, (2017), S0925231217314613. |
[2] | G. Camps-Valls, D. Tuia, L. Bruzzone, et al., Advances in hyperspectral image classification: earth monitoring with statistical learning methods, IEEE Signal Proc. Mag., 31 (2013), 45–54. |
[3] | L. Wang, Y. Zeng and T. Chen, Back propagation neural network with adaptive differential evolution algorithm for time series forecasting, Expert Syst. Appl., 42 (2015), 855–863. |
[4] | E. Maggiori, Y. Tarabalka, G. Charpiat, et al., Convolutional neural networks for large-scale remote sensing image classification, IEEE T. Geosci. Remote, 55 (2016), 645–657. |
[5] | G. B. Huang, Q. Y. Zhu and C. K. Siew, Extreme learning machine: theory and applications, Neurocomputing, 70 (2006), 489–501. |
[6] | J. Zhang, Y. F. Lu, B. Q. Zhang, et al., Device-free localization using empirical wavelet transform-based extreme learning machine, Proceedings of the 30th Chinese Control and Decision Conference, (2018), 2585–2590. |
[7] | Y. J. Li, S. Zhang, Y. X. Yin, et al., A soft sensing scheme of gas utilization prediction for blast furnace via improved extreme learning machine, Neural Process. Lett. (2018), 10.1007/s11063-018-9888-3. |
[8] | J. Zhang, Y. F. Xu, J. Q. Xue, et al., Real-time prediction of solar radiation based on online sequential extreme learning machine, Proceedings of the 13th IEEE Conference on Industrial Electronics and Applications, (2018), 53–57. |
[9] | R. Z. Song, W. D. Xiao, Q. L. Wei, et al., Neural-network-based approach to finite-time optimal control for a class of unknown nonlinear systems, Soft Comput., 18 (2014), 1645–1653. |
[10] | J. Zhang, W. D. Xiao, Y. J. Li, et al., Multilayer probability extreme learning machine for device-free localization. Neurocomputing, (2019), 10.1016/j.neucom.2018.11.106. |
[11] | Y. Park, and H. S. Yang, Convolutional neural network based on an extreme learning machine for image classification, Neurocomputing, 339 (2019), 66–76. |
[12] | G. B. Huang, H. Zhou, X. Ding, et al., Extreme learning machine for regression and multiclass classification, IEEE T. Syst. Man Cy. B., 42 (2012), 513–529. |
[13] | F. Han, H. F. Yao and Q. H. Ling, An improved evolutionary extreme learning machine based on particle swarm optimization, Neurocomputing, 116 (2013), 87–93. |
[14] | A. Rashno, B. Nazari, S. Sadri, et al., Effective pixel classification of mars images based on ant colony optimization feature selection and extreme learning machine, Neurocomputing, 226 (2017), 66–79. |
[15] | G. Li, P. Niu, Y. Ma, et al., Tuning extreme learning machine by an improved artificial bee colony to model and optimize the boiler efficiency, Knowl-Based Syst., 67 (2014), 278–289. |
[16] | İ. B. Ş, and S. Fang, An electromagnetism-like mechanism for global optimization, J. Global Optim., 25 (2003), 263–282. |
[17] | C. J. Zhang, X. Y. Li, L. Gao, et al., An improved electromagnetism-like mechanism algorithm for constrained optimization, Expert Syst. Appl., 40 (2013), 5621–5634. |
[18] | C. T. Tseng, C. H. Lee, Y. S. P. Chiu, et al., A discrete electromagnetism-like mechanism for parallel machine scheduling under a grade of service provision, Int. J. Prod. Res., 55 (2017), 3149–3163. |
[19] | X. Y. Li, L. Gao, Q. K. Pan, et al., An effective hybrid genetic algorithm and variable neighborhood search for integrated process planning and scheduling in a packaging machine workshop, IEEE T. Syst. Man Cy. Syst., (2018), 10.1109/TSMC.2018.2881686. |
[20] | X. Y. Li, C. Lu, L. Gao, et al., An Effective Multi-Objective Algorithm for Energy Efficient Scheduling in a Real-Life Welding Shop, IEEE T. Ind. Inform., 14 (2018), 5400–5409. |
[21] | X. Y. Li, S. Q. Xiao, C. Y. Wang, et al., Mathematical Modeling and a Discrete Artificial Bee Colony Algorithm for the Welding Shop Scheduling Problem, Memetic Comp., (2019), 10.1007/s12293-019-00283-4. |
[22] | Q. Wu, L. Gao, X. Y. Li, et al., Applying an electromagnetism-like mechanism algorithm on parameter optimisation of a multi-pass milling process, Int. J. Prod. Res., 51 (2013), 1777–1788. |
[23] | K. J. Wang, A. M. Adrian, K. H. Chen, et al., An improved electromagnetism-like mechanism algorithm and its application to the prediction of diabetes mellitus, J. Biomed. Inform., 54 (2015), 220–229. |
[24] | S. Mirjalili, Dragonfly algorithm: a new meta-heuristic optimization technique for solving single-objective, discrete, and multi-objective problems, Neural Comput. Appl., 27 (2016), 1053–1073. |
[25] | G. Huang, G. B. Huang, S. Song, et al., Trends in extreme learning machines: a review, Neural Networks, 61 (2015), 32–48. |
[26] | P. L. Bartlett, The sample complexity of pattern classification with neural networks: the size of the weights is more important than the size of the network, IEEE T. Inform. Theory, 44 (2002), 525–536. |
[27] | Q. Y. Zhu, A. K. Qin, P. N. Suganthan, et al., Evolutionary extreme learning machine, Pattern Recogn., 38 (2005), 1759–1763. |
[28] | D. Dua, and E. K. Taniskidou, UCI Machine Learning Repository Irvine, CA: University of California, School of Information and Computer Science, 2017. Available from: http://archive.ics.uci.edu/ml. |
[29] | Y. Wang, A. Wang, Q. Ai, et al., A novel artificial bee colony optimization strategy-based extreme learning machine algorithm, Prog. Artif. Intell., 6 (2016), 1–12. |
1. | Ying Li, Fang Huang, Ruoying Deng, Da Jiang, Delivery of IL2RG mRNA via nanoparticles to enhance CD8+ T cell promotes anti-tumor effects against late-stage triple-negative breast cancer, 2024, 15, 1868-6958, 10.1186/s12645-024-00290-2 |
Characteristic function predicted by mathematical model | Biological entities involved | Biological basis of the characteristic function | Exemplars of genes involved in the antitumor characteristic | Illustrative findings |
Monophasic Activation (DNA blockage) | DNA. Chemomodulation: Cell multiplication blockage. | Build-up and then decay of DNA chemomodulative or blockage activity. | Cell kinase gene: CDC2, CHEK1 Cell cycle gene: CCNB1, CCNB3 | Figure 7(c), Figure 13(b) |
Biphasic activation (Cytotoxic T-cell) | Lymphocyte enhancement: Lysis of tumor cells. | Second rise (biphasicity) in T- cell population due to decline of chemomodulation, i.e., decrease in blockage of T-cell growth. | T-cell receptor activation: TRGV5 gene, CD28 gene. G-protein coupled receptor activation: CALCR gene, CBLB gene. | Figure 7(b), Figure 13(a) |
Uniform Stationary activation (Interleukin-2) | Cytokine enhancement: Escalating the leucocyte-tumor cell interaction. | Toxicity limit of cytokine (homeostasis). | Interleukin-2 receptor gene: IL2RG, AKT3 gene. Transmembrane protein: CD74, GRB2 gene. | Figure 7(d), Figure 14(a) |
Saturating Activation (Natural killer cell) | Natural killer cell function (normal tissue protection). | Equilibrium state after Tumor regression. | NK cell activator gene: NKG2D gene, STAT4 gene Actuates NK cell function activators: KLRK1 gene, MAP3K12 gene. | Figure 7(f), Figure 13(d) |
Saturating Activation (Circulating lymphocyte) | Circulating lymphocyte function (host defense). | Equilibrium state after Tumor regression. | Chemokine ligand 1 activity: TCA gene - Actuates lymphocytes & monocytes. Rantes ligand activity: CCL5 and PRKCB genes - Actuates lymphocyte & monocytes. | Figure 7(e), Figure 13(c) |
Negative biasing | Apoptosis pathway | Elimination of residual tumor cells. | Extrinsic apoptosis pathway activator genes: CASP7 gene, GZMB gene. Intrinsic apoptosis pathway activator gene: BCL2L1 gene | Figure 1(b), Section 3.3.1 (ⅳ) |
Gene Name | Log2FC value (at time t1) | Log2FC value (at time t2) | Log2FC value (at time t3) | Log2FC value (at time t4) | p. value | F | Average Expression |
GZMB (Granzyme-B) | −0.07314 | 0.525236 | 1.124219 | 2.326586 | 0.00011 | 10.0495 | 6.086244 |
CASP7 (Caspase-7) | −0.02369 | 0.12626 | 0.570303 | 1.488125 | 0.03849 | 4.8372 | 7.215214 |
Characteristic function predicted by mathematical model | Biological entities involved | Biological basis of the characteristic function | Exemplars of genes involved in the antitumor characteristic | Illustrative findings |
Monophasic Activation (DNA blockage) | DNA. Chemomodulation: Cell multiplication blockage. | Build-up and then decay of DNA chemomodulative or blockage activity. | Cell kinase gene: CDC2, CHEK1 Cell cycle gene: CCNB1, CCNB3 | Figure 7(c), Figure 13(b) |
Biphasic activation (Cytotoxic T-cell) | Lymphocyte enhancement: Lysis of tumor cells. | Second rise (biphasicity) in T- cell population due to decline of chemomodulation, i.e., decrease in blockage of T-cell growth. | T-cell receptor activation: TRGV5 gene, CD28 gene. G-protein coupled receptor activation: CALCR gene, CBLB gene. | Figure 7(b), Figure 13(a) |
Uniform Stationary activation (Interleukin-2) | Cytokine enhancement: Escalating the leucocyte-tumor cell interaction. | Toxicity limit of cytokine (homeostasis). | Interleukin-2 receptor gene: IL2RG, AKT3 gene. Transmembrane protein: CD74, GRB2 gene. | Figure 7(d), Figure 14(a) |
Saturating Activation (Natural killer cell) | Natural killer cell function (normal tissue protection). | Equilibrium state after Tumor regression. | NK cell activator gene: NKG2D gene, STAT4 gene Actuates NK cell function activators: KLRK1 gene, MAP3K12 gene. | Figure 7(f), Figure 13(d) |
Saturating Activation (Circulating lymphocyte) | Circulating lymphocyte function (host defense). | Equilibrium state after Tumor regression. | Chemokine ligand 1 activity: TCA gene - Actuates lymphocytes & monocytes. Rantes ligand activity: CCL5 and PRKCB genes - Actuates lymphocyte & monocytes. | Figure 7(e), Figure 13(c) |
Negative biasing | Apoptosis pathway | Elimination of residual tumor cells. | Extrinsic apoptosis pathway activator genes: CASP7 gene, GZMB gene. Intrinsic apoptosis pathway activator gene: BCL2L1 gene | Figure 1(b), Section 3.3.1 (ⅳ) |
Gene Name | Log2FC value (at time t1) | Log2FC value (at time t2) | Log2FC value (at time t3) | Log2FC value (at time t4) | p. value | F | Average Expression |
GZMB (Granzyme-B) | −0.07314 | 0.525236 | 1.124219 | 2.326586 | 0.00011 | 10.0495 | 6.086244 |
CASP7 (Caspase-7) | −0.02369 | 0.12626 | 0.570303 | 1.488125 | 0.03849 | 4.8372 | 7.215214 |