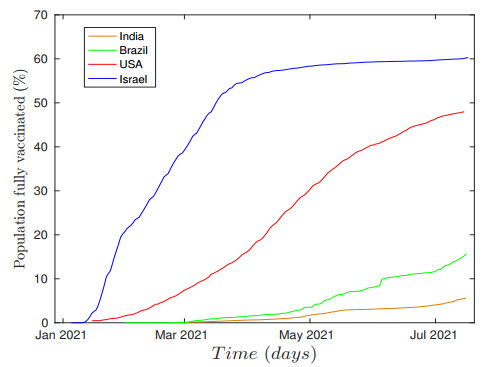
This work deals with the impact of the vaccination in combination with a restriction parameter that represents non-pharmaceutical interventions measures applied to the compartmental SEIR model in order to control the COVID-19 epidemic. This restriction parameter is used as a control parameter, and the univariate autoregressive integrated moving average (ARIMA) is used to forecast the time series of vaccination of all individuals of a specific country. Having in hand the time series of the population fully vaccinated (real data + forecast), the Levenberg–Marquardt algorithm is used to fit an analytic function that models this evolution over time. Here, it is used two time series of real data that refer to a slow vaccination obtained from India and Brazil, and two faster vaccination as observed in Israel and the United States of America. Together with vaccination, two different control approaches are presented in this paper, which enable reduces the infected people successfully: namely, the feedback and nonfeedback control methods. Numerical results predict that vaccination can reduce the peaks of infections and the duration of the pandemic, however, a better result is achieved when the vaccination is combined with any restrictions or prevention policy.
Citation: Vinicius Piccirillo. COVID-19 pandemic control using restrictions and vaccination[J]. Mathematical Biosciences and Engineering, 2022, 19(2): 1355-1372. doi: 10.3934/mbe.2022062
[1] | Pannathon Kreabkhontho, Watchara Teparos, Thitiya Theparod . Potential for eliminating COVID-19 in Thailand through third-dose vaccination: A modeling approach. Mathematical Biosciences and Engineering, 2024, 21(8): 6807-6828. doi: 10.3934/mbe.2024298 |
[2] | Yuto Omae, Yohei Kakimoto, Makoto Sasaki, Jun Toyotani, Kazuyuki Hara, Yasuhiro Gon, Hirotaka Takahashi . SIRVVD model-based verification of the effect of first and second doses of COVID-19/SARS-CoV-2 vaccination in Japan. Mathematical Biosciences and Engineering, 2022, 19(1): 1026-1040. doi: 10.3934/mbe.2022047 |
[3] | Ahmed Alshehri, Saif Ullah . A numerical study of COVID-19 epidemic model with vaccination and diffusion. Mathematical Biosciences and Engineering, 2023, 20(3): 4643-4672. doi: 10.3934/mbe.2023215 |
[4] | Fang Wang, Lianying Cao, Xiaoji Song . Mathematical modeling of mutated COVID-19 transmission with quarantine, isolation and vaccination. Mathematical Biosciences and Engineering, 2022, 19(8): 8035-8056. doi: 10.3934/mbe.2022376 |
[5] | Fen-fen Zhang, Zhen Jin . Effect of travel restrictions, contact tracing and vaccination on control of emerging infectious diseases: transmission of COVID-19 as a case study. Mathematical Biosciences and Engineering, 2022, 19(3): 3177-3201. doi: 10.3934/mbe.2022147 |
[6] | Xinyu Bai, Shaojuan Ma . Stochastic dynamical behavior of COVID-19 model based on secondary vaccination. Mathematical Biosciences and Engineering, 2023, 20(2): 2980-2997. doi: 10.3934/mbe.2023141 |
[7] | Xiaojing Wang, Yu Liang, Jiahui Li, Maoxing Liu . Modeling COVID-19 transmission dynamics incorporating media coverage and vaccination. Mathematical Biosciences and Engineering, 2023, 20(6): 10392-10403. doi: 10.3934/mbe.2023456 |
[8] | Yujie Sheng, Jing-An Cui, Songbai Guo . The modeling and analysis of the COVID-19 pandemic with vaccination and isolation: a case study of Italy. Mathematical Biosciences and Engineering, 2023, 20(3): 5966-5992. doi: 10.3934/mbe.2023258 |
[9] | Biplab Dhar, Praveen Kumar Gupta, Mohammad Sajid . Solution of a dynamical memory effect COVID-19 infection system with leaky vaccination efficacy by non-singular kernel fractional derivatives. Mathematical Biosciences and Engineering, 2022, 19(5): 4341-4367. doi: 10.3934/mbe.2022201 |
[10] | Sarafa A. Iyaniwura, Rabiu Musa, Jude D. Kong . A generalized distributed delay model of COVID-19: An endemic model with immunity waning. Mathematical Biosciences and Engineering, 2023, 20(3): 5379-5412. doi: 10.3934/mbe.2023249 |
This work deals with the impact of the vaccination in combination with a restriction parameter that represents non-pharmaceutical interventions measures applied to the compartmental SEIR model in order to control the COVID-19 epidemic. This restriction parameter is used as a control parameter, and the univariate autoregressive integrated moving average (ARIMA) is used to forecast the time series of vaccination of all individuals of a specific country. Having in hand the time series of the population fully vaccinated (real data + forecast), the Levenberg–Marquardt algorithm is used to fit an analytic function that models this evolution over time. Here, it is used two time series of real data that refer to a slow vaccination obtained from India and Brazil, and two faster vaccination as observed in Israel and the United States of America. Together with vaccination, two different control approaches are presented in this paper, which enable reduces the infected people successfully: namely, the feedback and nonfeedback control methods. Numerical results predict that vaccination can reduce the peaks of infections and the duration of the pandemic, however, a better result is achieved when the vaccination is combined with any restrictions or prevention policy.
It was identified between late 2019 to early 2020, a new coronavirus (SARS-CoV-2), which is the cause of the disease known as COVID-19. This virus has spread rapidly around the world making the World Health Organization declared a pandemic caused by this virus on March 11, 2020. The COVID-19 pandemic has produced not only biomedical and epidemiological repercussions on a global scale but also social, economic, political, and cultural impacts [1]. For almost the entire year of 2020, the main strategy to deal with the COVID-19 outbreak was adopting the use of non-pharmacological measures. One can highlight such measures as social distancing, quarantines, lockdown, masks, hand hygiene, etc [2,3]. Wearing masks and social distancing can help reduce the chance of exposure to the virus or transmitting it to others, but these measures are not enough. For this reason, vaccines are important, because they will work on the immune system so that it is ready to fight the virus if you are exposed. The vaccines are considered the best preventive measures to decrease the morbidity and mortality of infectious diseases [4]. Over the course of 2020, vaccines were developed with the most different technologies and at a speed never seen before. Thus, the United Kingdom started vaccinating its population on 08 December 2020, with an unprecedented vaccine based on Messenger RNA (mRNA), the first vaccine approved for emergency use [5].
Deterministic mathematical models have been used for years as one of the ways to study the behavior of infectious diseases. When making predictions about diseases policymakers can put in practice the vaccination and/or isolation plans, and thus, have a significant reduction in the death rate of an epidemic/pandemic [6]. Among the mathematical models that are used for this purpose, it can highlight the SEIR model (and some variations of it), which is widely used, for example, to model the dynamics of the COVID-19 pandemic [7,8,9,10], to pandemic control [11,12,13], and it is used to simulate the effectiveness of non-phamacological measures [14,15].
Time series analysis is a powerful tool that, among other applications allows to build a mathematical model from experimental data. Different methods have been applied to predict future values from real data. Among them can be highlighted autoregressive methods (AR), auto-regressive moving average method (ARMA), auto-regressive integrated moving average method (ARIMA), autoregressive moving average with exogenous inputs method (ARMAX). These kinds of models have been used for forecasting time series by a number of authors [16,17,18,19], and, in particular, the Box-Jenkins method is used to estimate incidences of COVID-19 [20,21].
In this work, the classical SEIR model is changed by a control (σ) and vaccination terms. A forecasting mechanism based on the ARIMA model to estimate the future of the vaccination process is used. The time series of vaccination (real data + prediction) is fitted using the Levenberg–Marquardt algorithm in order to obtain a mathematical function that describes the vaccination over time. Based on this SEIR model modified, numerical simulations are carried out. Two strategies are used in order to perform the pandemic control. The nonfeedback control with constant and pre-determined values of σ and the feedback control that uses a variable structure control (VSC) and an extended Kalman filter (EKF) are employed in order to reduce the epidemic peaks. In the nonfeedback control case, the pre-established values of σ generate two or three waves in the epidemic curve. On the other hand, since the actions of the feedback control are more restrictive, then only one infection wave is observed. In all these cases, results show that the combination of vaccination and restriction measures is a better alternative for controlling the pandemic spread since it provides a more effective reduction of infected when compared to the case obtained from the non-pharmacological approach only.
Vaccines are considered to be one of the best preventive measures to reduce mortality from infectious diseases. However, the implementation of vaccine policies by health authorities is not an easy task. Mathematical modeling is important in the development of any modern vaccination program and should be used in the evaluation of different vaccination policies [4,22,23]. In this way, mathematical modeling can help us to quantify the protection provided by immunization [4].
Figure 1 shows the real data of the population fully vaccinated until July 2021 obtained from the Our vaccination dataset [24] for India, Brazil, USA, and Israel. Note that the real data of COVID-19 vaccination from India and Brazil are slow, while, the USA and Israel are faster.
Forecasting is made using a known time series called the past to estimate future values of this series. The development of time series forecasting dates back to 1970 when Box & Jenkins (BJ) [25] proposed a methodology quite different from the existing ones. Based on the important result of Wold, (1938), who proved that any time series can be represented by an infinite moving average model, BJ proposes a class of linear models known as ARMA (p,q) (Autoregressive Moving average) models. A generalization of an ARMA model is an autoregressive integrated moving average (ARIMA (p,d,q)) that is applied in cases where the data shows evidence of nonstationarity. In both models p is the number of lag observations included in the model, d is the number of times that the observations are differenced and q is the size of the moving average window, also called the order of moving average. The ARIMA models are particularly useful for forecasting due to the use of lagged variables.
In most cases the series are nonstationary, so it is necessary to use some transformation in order to extract more information from it. This procedure is done so that the stability of the variance occurs, that is, to make the residues of the adjusted model have a constant variance. In general, if a nonstationary time series needs to be differentiated d times to become stationary, we call this integrated time-series order d [26]. If a time series yt is stationary from the start (that is, it does not require any differentiation), we say that it is zero-order integrated. A possible transformation that can be done is the use the successive differences Δyt=yt−yt−1. A nonstationary yt series can be differentiated n times (Δnyt) until it acquires stability. Thus, for each difference applied to the data set, the stationarity verification tests are remade.
The concept of an integrated process of order d, or I(d), comes from the representation of stochastic processes as linear models of the ARIMA (p,d,q) class. In fact, the parameter d of an ARIMA (p,d,q) model represents the number of times that the stochastic process has been differentiated until it becomes stationary [26].
In order to test the stationarity of the fully vaccinated people time series, the Kwiatkowski, Phillips, Schmidt e Shin (KPSS) test [27] was applied. The KPSS test evaluates the null hypothesis that a univariate time series has a stationary trend. The hypothesis test statistic is given by [28]
LM=1N2N∑t=1S2tˆσ2(p) | (2.1) |
where St=∑tj=1ej(t=1,2,…,N) being ej the residual from the regression process and σ2 the estimate of the error of the variance of this regression, given by equation
ˆσ2(p)=1NN∑t=1e2t+2Np∑j=1wj(p)N∑t=j+1etet−j | (2.2) |
where p is the truncation lag, wj(p) is the optimal weighting function that corresponds to the choice of a Bartlett's special window given by wj(p)=1−jp+1 [28].
To characterize the dynamics during outbreaks of infectious diseases, mathematical models can be used by political and health authorities in the formulation of public health policies. Compartmental models are mathematical models in which the temporal variation of a given quantity is made through well-defined sets, which we call compartments [29]. One of the compartmental models that are being widely used to predict the evolution of the COVID-19 outbreak is the SEIR model, where S represents the Susceptible individuals, E is the Exposed individuals, I represents the Infectious and R is the Removed individuals (see Figure 2).
The SEIR compartmental deterministic model is given by the following set of ordinary differential equations
dSdt=−βNSIdEdt=βNSI−ωEdIdt=ωE−γIdRdt=γI | (3.1) |
where β=R0Tinf, ω=1Tinc and γ=1Tinf and N=S+E+I+R is the total population. The rate at which susceptible are converted into exposed (β) depends both on infection period (Tinf) and the epidemiological parameter R0 known as basic reproductive number of the infection system. In the case of COVID-19, it is estimated R0 between 1.6 and 3.0 [11]. In addition, ω is the rate at which exposed are converted into infected, γ is the rate at which those infected are converted into recovered, and Tinc is the incubation period.
The impact of preventive measures on the infection spread is analyzed by the SEIR model. Preventive measures, or in the context of this paper, control action are restrictive government and individual measures that are proposed to contain a pandemic, such as social distancing, quarantine, restriction of public movement, masks, and other efforts necessary to mitigate the spread of COVID-19. In order to incorporate the control action, the SEIR model is rewritten with an additional control term (1−σ) following the formulation used by [7,8]. In this case, the parameter σ can be associated with the intensity of the restrictions imposed by the control policies adopted in order to reduce the spread of infection [8]. Wearing masks and social distancing can help reduce the chance of exposure to the virus or transmitting it to others, but these measures are not enough. Vaccines will work on your immune system so that it is ready to fight the virus if you are exposed. Assume that the vaccine is effective so that all vaccinated susceptible individuals become immune, that is, susceptible vaccinated transforms into the removed, and by hypothesis, the removed individuals are assumed to be immune to reinfection. Let vk(t) represent the percentage of susceptible individuals being vaccinated per unit of time and θ=1Timm where Timm is the average time for immunization of an individual after vaccination. Figure 3 represents the compartmental SEIR model with the restriction (σ) and vaccination (vk(t)).
Taking all the above considerations, the SEIR model with restriction and vaccination terms is given by:
dSdt=−βNSI(1−σ)−θvk(t)SdEdt=βNSI(1−σ)−ωEdIdt=ωE−γIdRdt=γI+θvk(t)S | (4.1) |
where the vaccination rate vk(t) is defined by the function
vk(t):=Pk100, k=1,2,3,4. | (4.2) |
For instance, if we take k=1 we obtain the function v1(t)=P1100 that refers to the vaccination rate of the people in India. On the other hand, if we take k=2 we obtain the function v2(t)=P2100 that represents the vaccination rate of Brazil and so on. Observe that if vk(t)=0, then no vaccination is done and vk(t)=1 indicates that all susceptible population is vaccinated.
This section presents the results and discussions on the influence of vaccination and government actions to control the COVID-19 pandemic. At first, the vaccination prediction functions are adjusted by the ARIMA model. After that, numerical simulations considering two control methods are used, where σ is the control parameter. The nonfeedback and a feedback method based on the variable structure control (VSC) are applied to the system (4.1) to control the transmission of coronavirus disease (COVID-19). The simulations were performed by using the fourth-order Runge–Kutta method with a fixed step and reference parameter values from the literature are used to simulate the outbreak of COVID-19: R0=2.2 [30], Tinc=6.4 days [31], Tinf=3 days [32]. Moreover, in all simulations, the initial conditions are defined as follows (S0,E0,I0,R0)=(999,0,1,0). As the number of vaccinated people grows, one question is still open: how long does the immunity produced by the vaccine last? This is a question that is not yet possible to predict as the immune response to vaccines is influenced by many factors [33]. However, a study published in the journal Science found that the immunity acquired by individuals who had COVID-19 can last up to 8 months [34]. Therefore, one of the possible scenarios is that perhaps immunization against the new coronavirus will become part of the annual vaccination campaigns. Due to the uncertainty of the duration of the immunization period, it is adopted that Timm=365 days, which implies that θ=1365.
The analysis of vaccination time series is carried out in this section. Initially, the results obtained for the KPSS test are shown in table 1, where the critical values are for right-tail probabilities. If h value is equal to 1 it indicates the rejection of the null hypothesis, while if it is equal to 0 it indicates the acceptance of the null hypothesis;
Time series | test statistics | critical value | h |
India | 0.15 | 0.146 | 1 |
Brazil | 0.302 | 0.216 | 1 |
USA | 0.17 | 0.146 | 1 |
Israel | 0.342 | 0.146 | 1 |
According to table 1, and based on the proposal by [35], the analyzed series are nonstationary, because if the LM statistic is greater than the critical value, then the null hypothesis is rejected, and therefore, the series is nonstationary. All the series presented here are nonstationary, therefore, the ARIMA model will be used to forecast the time series of vaccinated people. First, the parameter d must be identified in order to convert all the nonstationary time series into stationary. The new differentiated series is illustrated in Figure 4.
To check whether the stationarity has been achieved, the KPSS test was remade for the differentiated series and the results are presented in Table 2. Note that to achieve the stationarity from India and Israel time series is necessary to derivative the respective series one time (d=1), and the time series from Brazil and the USA, it is necessary to derivative two times (d=2).
Time series | d | test statistics | critical value | h |
India | 1 | 0.08 | 0.146 | 0 |
Brazil | 2 | 0.05 | 0.146 | 0 |
USA | 2 | 0.07 | 0.146 | 0 |
Israel | 1 | 0.117 | 0.146 | 0 |
Now, it is necessary to find the values of p and q in order to select an appropriate ARIMA model to forecast the evolution of the fully vaccinated people times series. In order to determine the best ARIMA model to predict the time series the Bayesian Information Criterion (BIC) is used. The best model is achieved when the BIC measure will be as small as possible (the BIC measure can be negative [26]). As the fit of the model improves, the BIC will approach −∞ [26]. It is considered that parameter d is constant like observed in table 2, and the BIC is used to determine the parameters p and q. Table 3 reports the best ARIMA model with the respective minimum values of BIC for Indian, Brazil, USA, and Israel time series of the fully vaccinated people, considering the interval given by p∈[1,5] and q∈[1,5].
Time series | ARIMA(p, d, q) | BIC |
India | 1, 1, 1 | -596.5372 |
Brazil | 1, 2, 1 | -174.7926 |
USA | 4, 2, 2 | -416.2727 |
Israel | 4, 1, 3 | -119.4096 |
Figure 5 shows the predictions of the populations fully vaccinated using the ARIMA model as values described in Table 3. It is important to note that considering these forecasts the population of India will be fully vaccinated in 1314 days after starting the vaccination, the Brazilian people will be vaccinated in 414 days, the Israeli people in 398 days, and the US people in 488 days.
The mathematical model of the population fully vaccinated is obtained from a curve fit of real data together with the forecast performed, where each series was fit to a Fourier series (see Eq. 5.1).
Pk=a0+n∑j=1ajcos(jωt)+bjsin(jωt), k=1,2,3,4 | (5.1) |
where P1,P2,P3,P4 corresponds to India, Brazil, USA and Israel functions, respectively. The Brazil time series can be described by a 2th-order Fourier series, the Indian time series is fit to a 3th-order Fourier series and the USA and Israel time series can be fitting to the 4th-order Fourier series.
The coefficients of the Fourier series were obtained based on the Levenberg-Marquardt method for curve-fitting problems. Table 4 shows the coefficient value of each series.
![]() |
India | Brazil | USA | Israel |
a0 | 52.5211 | 143.0738 | 59.2779 | 56.2703 |
a1 | -40.8105 | -154.1863 | -31.8553 | -40.2695 |
a2 | -11.6672 | 11.2329 | -18.7046 | -17.3305 |
a3 | -0.1266 | 0 | -5.8297 | -2.6721 |
a4 | 0 | 0 | -1.2258 | 0.5241 |
b1 | -22.4162 | -29.2240 | -23.4229 | -3.7607 |
b2 | 6.9607 | 11.7953 | 1.5793 | 19.2970 |
b3 | 2.9042 | 0 | 2.0832 | 11.3469 |
b4 | 0 | 0 | 0.2832 | 2.2685 |
ω | 0.0029 | 0.0038 | 0.0076 | 0.0094 |
The proposed nonfeedback control of the system (4.1) consists of adopting constant values for σ for a certain amount of time and then altering it up or down to reduce the curve of infected individuals.
Case 1. In this case, the restrictions added to the system (4.1) result in two waves of the pandemic. The restrictions imposed are given by
σ={0.0,ift≤300.4,if30<t≤1300.6,if130<t≤2500.4,if250<t≤3650.0,if365<t | (5.2) |
Figure 6 shows the time response of the Infected individuals of the system (4.1) where the nonfeedback action (see Eq. 5.2) is applied. The gray curve in Figure 6 shown the system response without restriction (σ=0,∀t) and vaccination (vk(t)=0,∀t). As expected, the only infectious peak is very high which could lead to an overload of the health care system, implying the need for a large expansion of the hospital infrastructure, a fact that proved to be unfeasible in this pandemic. On the other hand, the black curve shows a scenario in which restrictions provided by Eq. 5.2 are put into practice but still without vaccination (v(t)=0,∀t). Note that in this case the infected peak is drastically reduced, however, as these restraint measures are smoothed and hardened over time a second peak (second wave) is observed. The advantage of adopting these restrictions is that the infectious peak decreases and probably, fewer deaths will be observed, in return, the pandemic duration increases.
Figure 7 depicts the time series of the infected people subjects to restrictions (see Eq. 5.2) and vaccinations rate (vk(t)). In these cases, the vaccination started 335 days after the beginning of the outbreak of COVID-19. Initially, the impact of a faster vaccination (see v3(t) and v4(t) cases) on susceptible individuals leads to a decrease in the peak of infected as compared to the case where only restrictions were imposed (see Figure 7a). Figures 7b and 7c are magnifications of the infected curve (Figure 7a). Figure 7b shows that even when a low speed of vaccination occurs, the magnitude response of infected people decreases, when using the v1(t) vaccination rate approximately 7% reduction is observed, while for v2(t) this reduction is 10 %. It can be said that even with the slow pace of vaccination it is recommended that the population are vaccinated since the number of infected is reduced, and, hence the chances that people will develop severe forms of the disease, decrease as well. On the other hand, Figure 7c shows that due to a high speed of vaccination the amplitude of peaks decreases considerably. It is observed the peak level decreases approximately 36% and 75% to the v3(t) and v4(t) vaccinations rate, respectively. Figure 7d shows the evolution of vaccination for the v3(t) and v4(t). It should be noted here that the attenuation of the second peak occurs because at this moment approximately 50% and 60% of the susceptible have already been vaccinated in the v3(t) and v4(t), respectively.
It is noteworthy that the vaccination has been started 335 days after the pandemic beginning, and the value of σ in Eq. 5.2 corresponding to this date is 0.4, i.e. (t,σ)=(335,0.4), which indicates a reasonable level of restrictions, but, 30 days after the vaccination started occurs a complete easing of restrictions (σ=0−see Eq. 5.2). However, the amplitude of the second peak can be reduced considerably if an integrated strategy using restriction and vaccination is considered. Eqs. (5.2), (5.3), and (5.4) are defined taking into account this strategy (restrictions + vaccination) where until t=365 the values of σ are the same as Eq. (5.2), but to t>365 the value of σ=0.1, σ=0.2 or σ=0.3 are assumed in Eqs. (5.3), Eq. (5.4) and Eq. (5.5), respectively.
σ={ ⋮0.1,if365<t | (5.3) |
σ={ ⋮0.2,if365<t | (5.4) |
σ={ ⋮0.3,if365<t | (5.5) |
Figure 8 shows the effects of this strategy on pandemic control adopting the v2(t) vaccination rate. The impact of vaccination and restrictions is clearly seen in the decrease of the amplitude of infected people of the second wave. Thus, for the considered σ parameters, the amplitude reduction are: 38% for σ=0.1, 67% for σ=0.2 and 85% for σ=0.3 in comparison with the σ=0.0 case. Thus, it is possible to say that the combination of vaccination and restrictions (governmental actions) provide a better strategy to suppress the epidemic spreading because the restrictions flatten the peak of the infected curve, and the vaccination makes the number of infected people can be minimized.
Case 2. Now the restrictions added to system 4.1 result in three waves of infections. The restriction is given by
σ={0.0,ift≤300.6,if30<t≤1300.4,if130<t≤2500.6,if250<t≤3350.4,if335<t≤4650.0,if465<t | (5.6) |
Figure 9 presents the time series of infected people without any restriction (gray color) and with the restriction defined by Eq. (5.6) (black color). Note that the restrictions dramatically alter the response of infected individuals, it significantly reduced the amplitude of the first peak, but three waves of infections are observed instead of one wave. Similar to the previous case, restrictions increase the pandemic duration.
Figure 10 presents the infected population considering σ given by Eq. (5.6) and different vaccinations rate (vk(t)). As previously, the vaccination is implemented 335 days after the spreading outbreak. Once again, it can be observed that different vaccination rates can result in dramatically different numbers of infected individuals (see Figure 10a). Figure 10a shows the time series of infected indicating when the vaccination starts and when the restriction is withdrawn. Figures 10b and 10c are magnifications of the infected curve (Figure 10a). It can be seen from Figure 10b that for both v1(t) and v2(t) rates of vaccination, the infected decreases 8% and 15%, respectively. Figure 10c presents a high-speed vaccination rate, and thus, the amplitude of the third peak decreases considerably, approximately 45% when v3(t) vaccination rate is used and 74% for v4(t). Figure 10d shows the evolution of vaccination for v2(t) and v3(t). One should note that after 130 days of vaccination all restrictions disappear (σ=0). In this instant of time, the fully vaccinated people arrive at about 67% when v3(t) is considered. On the other hand, when the v2(t) vaccination rate is applied only 10% of susceptible people are completely immunized. The decrease in restriction actions, even in the v3(t) case, demonstrated that the infected increase and hence vaccination alone is not enough to combat the pandemic. Therefore, the vaccination and restriction policies should combine action in order to avoid a larger number of infected people and consequently deaths.
To control the COVID-19 epidemic spreading will be using a variable structure controller (VSC). The switching surface Λ=λ−ˉσ is defined based on the number of total infected individuals Itσ=E+I where the controller design is separated into two steps. The VSC feedforward controller computes the ˉσ and the VSC feedback controller adjusts the control input (σ) to suppress the epidemic spreading. The controller uses an extended Kalman filter (EKF) to estimate the λ -parameter. A detailed description of the control and EKF design can be found in [8]. A schematic of the VSC controller is shown in Figure 11.
The function σ in Eq. (4.1) is defined in [8] as follows:
σ={aˉσ+bλ, ifΛ>0ˉσ ifΛ<0 | (5.7) |
Figure 12 shows the variable structure controller performance where the control actions being taken every 7 days for a=0.1 and b=0.9. The control of the spread of COVID-19 without vaccination but with restrictions is shown with the gray curve. Note that the number of infected is low but the restrictions persist for a long period of time (approx. 740 days until σ=ˉσ - the lowest value of restrictions) with the peak of infected being achieved 317 days after beginning the first infection. Note that, due to vaccination, the reduction is observed in infected followed by a decrease in the duration of the COVID-19 pandemic. Once again, it can be observed that different vaccination actions result in different numbers of infected. Figure 12b shows the influence of vaccination on the action of the restriction parameter. It is interesting to note that with a higher speed of vaccination rate the restrictions are reduced more quickly. The results also show that a higher vaccination coverage renders benefits like easing the restrictions.
In this paper, the non-pharmacological and vaccination terms are incorporated into the SEIR model in order to control the COVID-19 outbreak. The Autoregressive Integrated Moving Average (ARIMA) model was proposed for the vaccination time series forecasting in four different countries around the World. In particular, this procedure is performed on the time series from India, Brazil, the USA, and Israel. All series are nonstationary that need to be transformed into stationary ones. After that, the prediction made by the ARIMA model together with real data is fit to a basis of harmonic functions where the Levenberg–Marquardt algorithm estimated the coefficients of this function. This analytical function is used to model the vaccination rate in the simulations on the SEIR model. This paper presents two strategies for controlling the COVID-19 pandemic considering the vaccination and restriction, where the restriction parameter is considered as the control parameter. The nonfeedback control is capable to reduces the first peak but other waves are observed. On the other hand, feedback control is proposed, and, as it is more restricted than the nonfeedback one, only one peak is observed. Numerical simulations indicate clearly that vaccination is indispensable to reducing the number of infected people. In addition, the vaccination combined with governmental actions (like non-pharmacological ones) are capable to minimize the infected peaks.
The authors declare there is no conflict of interest.
[1] |
C. A. Varotsos, V. F. Krapivin, Y. Xue, Diagnostic model for the society safety under COVID-19 pandemic conditions, Saf. Sci., 136 (2021), 105164. doi: 10.1016/j.ssci.2021.105164. doi: 10.1016/j.ssci.2021.105164
![]() |
[2] |
X. Lao, L. Luo, Z. Lei, T. Fang, Y. Chen, Y. Liu, et al., The epidemiological characteristics and effectiveness of countermeasures to contain coronavirus disease 2019 in Ningbo City, Zhejiang Province, China, Sci. Rep., 11 (2021), 1–12. doi: 10.1038/s41598-021-88473-4. doi: 10.1038/s41598-021-88473-4
![]() |
[3] |
S. Jamshidi, M. Baniasad, D. Niyogi, Global to USA county scale analysis of weather, urban density, mobility, homestay, and mask use on COVID-19, Int. J. Environ. Res. Public Health, 17 (2020), 7847. doi: 10.3390/ijerph17217847. doi: 10.3390/ijerph17217847
![]() |
[4] |
A. Huppert, G. Katriel, Mathematical modelling and prediction in infectious disease epidemiology, Clin. Microbiol. Infect., 19 (2013), 999–1005. doi:10.1111/1469-0691.12308. doi: 10.1111/1469-0691.12308
![]() |
[5] | Coronavirus (COVID-19) in the UK - COVID-19 dataset. https://coronavirus.data.gov.uk/details/about-data, 2021. Accessed on: 2021-11-21. |
[6] |
M. Baniasad, M. G. Mofrad, B. Bahmanabadi, S. Jamshidi, COVID-19 in Asia: Transmission factors, re-opening policies, and vaccination simulation, Environ. Res., 202 (2021), 111657. doi: 10.1016/j.envres.2021.111657. doi: 10.1016/j.envres.2021.111657
![]() |
[7] |
S. L. de Souza, A. M. Batista, I. L. Caldas, K. C. Iarosz, J. D. Szezech Jr, Dynamics of epidemics: Impact of easing restrictions and control of infection spread, Chaos Solitons Fractals, 142 (2020), 110431. doi: 10.1016/j.chaos.2020.110431. doi: 10.1016/j.chaos.2020.110431
![]() |
[8] |
P. M. Pacheco, M. A. Savi, P. V. Savi, COVID-19 dynamics considering the influence of hospital infrastructure: an investigation into Brazilian scenarios, Nonlinear Dyn., 106 (2021), 1325–1346. doi: 10.1007/s11071-021-06323-4. doi: 10.1007/s11071-021-06323-4
![]() |
[9] |
S. He, Y. Peng, K. Sun, SEIR modeling of the COVID-19 and its dynamics, Nonlinear Dyn., 127 (2020), 1667–1680. doi: 10.1007/s11071-020-05743-y. doi: 10.1007/s11071-020-05743-y
![]() |
[10] |
C. A. Varotsos, V. F. Krapivin, A new model for the spread of COVID-19 and the improvement of safety, Saf. Sci., 132 (2020), 104962. doi: 10.1016/j.ssci.2020.104962. doi: 10.1016/j.ssci.2020.104962
![]() |
[11] |
V. Piccirillo, Nonlinear control of infection spread based on a deterministic SEIR model, Chaos Solitons Fractals, 149 (2021), 111051. doi: 10.1016/j.chaos.2021.111051. doi: 10.1016/j.chaos.2021.111051
![]() |
[12] |
S. Zhai, G. Luo, T. Huang, X. Wang, J. Tao, P. Zhou, Vaccination control of an epidemic model with time delay and its application to COVID-19, Nonlinear Dyn., 106 (2021), 1279–1292. doi: 10.1007/s11071-021-06533-w. doi: 10.1007/s11071-021-06533-w
![]() |
[13] |
K. Prem, L. Yang, W. R. Timothy, J. K. Adam, M. E. Rosalind, D. Nicholas, et al., The effect of control strategies to reduce social mixing on outcomes of the COVID-19 epidemic in Wuhan, China: A modelling study, Lancet Public Health, 55 (2020), 261–270. doi: 10.1016/S2468-2667(20)30073-6. doi: 10.1016/S2468-2667(20)30073-6
![]() |
[14] |
C. N. Ngonghala, E. Iboi, S. Eikenberry, M. Scotch, C. R. MacIntyre, M. H. Bonds, et al., Mathematical assessment of the impact of non-pharmaceutical interventions on curtailing the 2019 novel Coronavirus, Math. Biosci., 325 (2020), 1083. doi: 10.1016/j.mbs.2020.108364. doi: 10.1016/j.mbs.2020.108364
![]() |
[15] |
C. A. Varotsos, V. F. Krapivin, Y. Xue, V. Soldatov, T. Voronova, COVID-19 Pandemic Decision Support System for an Appropriate Population Defense Strategy and Vaccination Effectiveness, Saf. Sci., 142 (2021), 105370. doi: doi.org/10.1016/j.ssci.2021.105370. doi: 10.1016/j.ssci.2021.105370
![]() |
[16] |
R. G. Kavasseri, K. Seetharaman, Day-ahead wind speed forecasting using f-ARIMA models, Renew. Energy, 34 (2009), 1388–1393. doi: 10.1016/j.renene.2008.09.006. doi: 10.1016/j.renene.2008.09.006
![]() |
[17] |
H. B. Hwarng, Insights into neural-network forecasting of time series corresponding to ARMA (p, q) structures, Omega, 29 (2001), 273–289. doi: 10.1016/S0305-0483(01)00022-6. doi: 10.1016/S0305-0483(01)00022-6
![]() |
[18] |
E. Cadenas, W. Rivera, Wind speed forecasting in three different regions of Mexico, using a hybrid ARIMA–ANN model, Renew. Energy, 35 (2010), 2732–2738. doi: 10.1016/j.renene.2010.04.022. doi: 10.1016/j.renene.2010.04.022
![]() |
[19] |
Y. Lai, D. A. Dzombak, Use of the autoregressive integrated moving average (ARIMA) model to forecast near-term regional temperature and precipitation, Weather and Forecasting, 35 (2020), 959–976. doi: 10.1175/WAF-D-19-0158.1. doi: 10.1175/WAF-D-19-0158.1
![]() |
[20] |
R. Katoch, A. Sidhu, An Application of ARIMA Model to Forecast the Dynamics of COVID-19 Epidemic in India, Glob. Bus. Rev. (2021), 0972150920988653. doi: 10.1177/0972150920988653. doi: 10.1177/0972150920988653
![]() |
[21] |
R. C. Das, Forecasting incidences of COVID-19 using Box-Jenkins method for the period July 12-September 11, 2020: A study on highly affected countries, Chaos Solitons Fractals, 140 (2020), 110248. doi: 10.1016/j.chaos.2020.110248. doi: 10.1016/j.chaos.2020.110248
![]() |
[22] |
K. Canfell, H. Chesson, S. L. Kulasingam, J. Berkhof, M. Diaz, J. J. Kim, Modeling preventative strategies against human papillomavirus-related disease in developed countries, Vaccine, 30 (2012), F157–-F167. doi: 10.1016/j.vaccine.2012.06.091. doi: 10.1016/j.vaccine.2012.06.091
![]() |
[23] |
E. Shim, Z. Feng, M. Martcheva, C. Castillo-Chavez, An age-structured epidemic model of rotavirus with vaccination, J. Math. Biol., 53 (2006), 719–-746. doi: 10.1007/s00285-006-0023-0. doi: 10.1007/s00285-006-0023-0
![]() |
[24] |
E. Mathieu, H. Ritchie, E. Ortiz-Ospina, M. Roser, C. Giattino, A global database of COVID-19 vaccinations, Nat. Hum. Behav., 5 (2021), 947–-953. doi: 10.1038/s41562-021-01122-8. doi: 10.1038/s41562-021-01122-8
![]() |
[25] | G. E. P. Box, G. M. Jenkins, Time Series Analysis. Forecasting and Control, Halden-Day, San Francisco, 1970. |
[26] | W. Enders, Applied econometric time series, John Wiley & Sons (2008). |
[27] |
D. Kwiatkowski, P. C. B. Phillips, P. Schmidt, Y. Shin, Testing the null hypothesis of stationarity against the alternative of a unit root, J. Econom., 54 (1992), 159-–178. doi: 10.1016/0304-4076(92)90104-Y. doi: 10.1016/0304-4076(92)90104-Y
![]() |
[28] | W. Wang, Stochastic, Nonlinearity and Forecasting of Streamflow. Amsterdan: Deft University Press (2006). |
[29] |
I. Sandberg, On the mathematical foundations of compartmental analysis in biology, medicine, and ecology, IEEE Trans. Circuits Syst., 25 (1978), 273–279. doi: 10.1109/TCS.1978.1084473. doi: 10.1109/TCS.1978.1084473
![]() |
[30] |
A. J. Kucharski, T. W. Russell, C. Diamond, Y. Liu, J. Edmunds, S. Funk, et al., Early dynamics of transmission and control of COVID-19: a mathematical modelling study, Lancet Infect. Dis., 20 (2020), 553–558. doi: 10.1016/S1473-3099(20)30144-4. doi: 10.1016/S1473-3099(20)30144-4
![]() |
[31] |
J. A. Backer, D. Klinkenberg, J. Wallinga, Incubation period of 2019 novel coronavirus (2019-nCoV) infections among travellers from Wuhan, China, 20–28 January 2020, Euro Surveill, 25 (2020), 2000062. doi: 10.2807/1560-7917.ES.2020.25.5.2000062. doi: 10.2807/1560-7917.ES.2020.25.5.2000062
![]() |
[32] | R. Woelfel, V. M. Corman, W. Guggemos, M. Seilmaier, C. Wendtner, Clinical presentation and virological assessment of hospitalized cases of coronavirus disease 2019 in a travel-associated transmission cluster, MedRxiv, (2020). doi: 10.1101/2020.03.05.20030502. |
[33] |
P. Zimmermann, N. Curtis, Factors that influence the immune response to vaccination, Clin. Microbiol. Rev., 32 (2019), e00084-18. doi:10.1128/CMR.00084-18. doi: 10.1128/CMR.00084-18
![]() |
[34] | J. M. Dan, J. Mateus, Y. Kato, K. M. Hastie, E. D. Yu, C. E. Faliti, et al., Immunological memory to SARS-CoV-2 assessed for up to 8 months after infection, Science, 371 (2021). doi: 10.1126/science.abf4063. |
[35] | S. Nusair, Testing the validity of purchasing power parity for asian countries during the current float, J. Econ. Dev., 28 (2003), 129–147. |
1. | Mustafa Kamal, Mintodê Nicodème Atchadé, Yves Morel Sokadjo, Sabir Ali Siddiqui, Fathy H. Riad, M. M. Abd El-Raouf, Ramy Aldallal, Eslam Hussam, Huda M. Alshanbari, Hassan Alsuhabi, Ahmed M. Gemeay, Influence of COVID-19 vaccination on the dynamics of new infected cases in the world, 2022, 20, 1551-0018, 3324, 10.3934/mbe.2023156 | |
2. | Dimitrios Koumatzidis, Ioannis Seimenis, Constantinos Loukas, Theodoros Constantinidis, Adam Adamopoulos, Impact of Quarantine and Vaccination Policies on Viral Load, 2022, 13, 2076-3417, 396, 10.3390/app13010396 | |
3. | Xinyu Bai, Shaojuan Ma, Stochastic dynamical behavior of COVID-19 model based on secondary vaccination, 2022, 20, 1551-0018, 2980, 10.3934/mbe.2023141 | |
4. | Khanh N.C. Duong, Danielle T. Nguyen, Warittakorn Kategeaw, Xi Liang, Win Khaing, Lindsay D. Visnovsky, Sajesh K. Veettil, Mary M. McFarland, Richard E. Nelson, Barbara E. Jones, Andrew T. Pavia, Emma Coates, Karim Khader, Jay Love, George G. Vega Yon, Yue Zhang, Tina Willson, Egenia Dorsan, Damon J.A. Toth, Makoto M. Jones, Matthew H. Samore, Nathorn Chaiyakunapruk, Incorporating social determinants of health into transmission modeling of COVID-19 vaccine in the US: a scoping review, 2024, 35, 2667193X, 100806, 10.1016/j.lana.2024.100806 | |
5. | Naied A. Nayied, Firdous A. Shah, Mukhtar A. Khanday, Kottakkaran Sooppy Nisar, Numerical investigation of fractional order SEIR models with newborn immunization using Vieta–Fibonacci wavelets, 2024, 12, 26668181, 100995, 10.1016/j.padiff.2024.100995 |
Time series | test statistics | critical value | h |
India | 0.15 | 0.146 | 1 |
Brazil | 0.302 | 0.216 | 1 |
USA | 0.17 | 0.146 | 1 |
Israel | 0.342 | 0.146 | 1 |
Time series | d | test statistics | critical value | h |
India | 1 | 0.08 | 0.146 | 0 |
Brazil | 2 | 0.05 | 0.146 | 0 |
USA | 2 | 0.07 | 0.146 | 0 |
Israel | 1 | 0.117 | 0.146 | 0 |
Time series | ARIMA(p, d, q) | BIC |
India | 1, 1, 1 | -596.5372 |
Brazil | 1, 2, 1 | -174.7926 |
USA | 4, 2, 2 | -416.2727 |
Israel | 4, 1, 3 | -119.4096 |
![]() |
India | Brazil | USA | Israel |
a0 | 52.5211 | 143.0738 | 59.2779 | 56.2703 |
a1 | -40.8105 | -154.1863 | -31.8553 | -40.2695 |
a2 | -11.6672 | 11.2329 | -18.7046 | -17.3305 |
a3 | -0.1266 | 0 | -5.8297 | -2.6721 |
a4 | 0 | 0 | -1.2258 | 0.5241 |
b1 | -22.4162 | -29.2240 | -23.4229 | -3.7607 |
b2 | 6.9607 | 11.7953 | 1.5793 | 19.2970 |
b3 | 2.9042 | 0 | 2.0832 | 11.3469 |
b4 | 0 | 0 | 0.2832 | 2.2685 |
ω | 0.0029 | 0.0038 | 0.0076 | 0.0094 |
Time series | test statistics | critical value | h |
India | 0.15 | 0.146 | 1 |
Brazil | 0.302 | 0.216 | 1 |
USA | 0.17 | 0.146 | 1 |
Israel | 0.342 | 0.146 | 1 |
Time series | d | test statistics | critical value | h |
India | 1 | 0.08 | 0.146 | 0 |
Brazil | 2 | 0.05 | 0.146 | 0 |
USA | 2 | 0.07 | 0.146 | 0 |
Israel | 1 | 0.117 | 0.146 | 0 |
Time series | ARIMA(p, d, q) | BIC |
India | 1, 1, 1 | -596.5372 |
Brazil | 1, 2, 1 | -174.7926 |
USA | 4, 2, 2 | -416.2727 |
Israel | 4, 1, 3 | -119.4096 |
![]() |
India | Brazil | USA | Israel |
a0 | 52.5211 | 143.0738 | 59.2779 | 56.2703 |
a1 | -40.8105 | -154.1863 | -31.8553 | -40.2695 |
a2 | -11.6672 | 11.2329 | -18.7046 | -17.3305 |
a3 | -0.1266 | 0 | -5.8297 | -2.6721 |
a4 | 0 | 0 | -1.2258 | 0.5241 |
b1 | -22.4162 | -29.2240 | -23.4229 | -3.7607 |
b2 | 6.9607 | 11.7953 | 1.5793 | 19.2970 |
b3 | 2.9042 | 0 | 2.0832 | 11.3469 |
b4 | 0 | 0 | 0.2832 | 2.2685 |
ω | 0.0029 | 0.0038 | 0.0076 | 0.0094 |