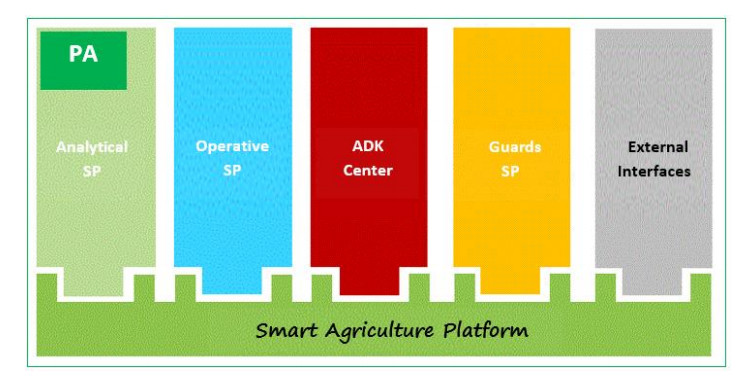
One of the major challenges that smart agriculture is expected to address is the efficient use of water resources. The conservation and the efficient use of clean water is a long-term strategy worldwide. Modeling of smart agriculture systems is an important factor because the processes there are very slow and sometimes it takes a year or more for a full crop cycle. At the same time, a large amount of data is usually needed to make informed decisions. This determines the importance of developing appropriate systems through which to simulate, generate, optimize and analyze various possible scenarios and prepare appropriate plans. In this paper, an infrastructure known as Virtual-Physical Space adapted for agriculture is presented. The space supports integration of the virtual and physical worlds where analysis and decision making are done in the virtual environment and the state of the physical objects (things) of interest is also taken into account at the same time. Special attention is paid to the possibilities for modeling an irrigation system. An ambient-oriented approach has been adopted, using the Calculus of Context-aware Ambients formalism as the basic tool for modeling agriculture processes. Furthermore, the supporting platform is briefly presented. Active components of the platform are implemented as intelligent agents known as assistants. Users (agriculture operators) are serviced by personal assistants. Currently, the presented modeling system is deployed over a two layered system infrastructure in the region of Plovdiv city. Plovdiv is the center of vegetable production in Bulgaria. The process of modeling intelligent irrigation systems and the current results are discussed in this paper.
Citation: Todorka Glushkova, Stanimir Stoyanov, Lyubka Doukovska, Jordan Todorov, Ivan Stoyanov. Modeling of an irrigation system in a virtual physical space[J]. Mathematical Biosciences and Engineering, 2021, 18(5): 6841-6856. doi: 10.3934/mbe.2021340
[1] | Favour Adenugba, Sanjay Misra, Rytis Maskeliūnas, Robertas Damaševičius, Egidijus Kazanavičius . Smart irrigation system for environmental sustainability in Africa: An Internet of Everything (IoE) approach. Mathematical Biosciences and Engineering, 2019, 16(5): 5490-5503. doi: 10.3934/mbe.2019273 |
[2] | Yu Lei, Zhi Su, Xiaotong He, Chao Cheng . Immersive virtual reality application for intelligent manufacturing: Applications and art design. Mathematical Biosciences and Engineering, 2023, 20(3): 4353-4387. doi: 10.3934/mbe.2023202 |
[3] | Roman Gumzej . Intelligent logistics systems in E-commerce and transportation. Mathematical Biosciences and Engineering, 2023, 20(2): 2348-2363. doi: 10.3934/mbe.2023110 |
[4] | Edwin Duque-Marín, Alejandro Rojas-Palma, Marcos Carrasco-Benavides . A soil water indicator for a dynamic model of crop and soil water interaction. Mathematical Biosciences and Engineering, 2023, 20(8): 13881-13899. doi: 10.3934/mbe.2023618 |
[5] | Chenyang Zhan, Min Tian, Yang Liu, Jie Zhou, Xiang Yi . A novel greedy adaptive ant colony algorithm for shortest path of irrigation groups. Mathematical Biosciences and Engineering, 2022, 19(9): 9018-9038. doi: 10.3934/mbe.2022419 |
[6] | Ziyou Zhuang . Optimization of building model based on 5G virtual reality technology in computer vision software. Mathematical Biosciences and Engineering, 2021, 18(6): 7936-7954. doi: 10.3934/mbe.2021393 |
[7] | Yikang Xu, Zhaohua Sun, Wei Gu, Wangping Qian, Qiangru Shen, Jian Gong . Three-dimensional inversion analysis of transient electromagnetic response signals of water-bearing abnormal bodies in tunnels based on numerical characteristic parameters. Mathematical Biosciences and Engineering, 2023, 20(1): 1106-1121. doi: 10.3934/mbe.2023051 |
[8] | Christophe Prud'homme, Lorenzo Sala, Marcela Szopos . Uncertainty propagation and sensitivity analysis: results from the Ocular Mathematical Virtual Simulator. Mathematical Biosciences and Engineering, 2021, 18(3): 2010-2032. doi: 10.3934/mbe.2021105 |
[9] | Radi P. Romansky, Irina S. Noninska . Challenges of the digital age for privacy and personal data protection. Mathematical Biosciences and Engineering, 2020, 17(5): 5288-5303. doi: 10.3934/mbe.2020286 |
[10] | Ruoyi Zhao, Ying Gao, Xingmin Lin, Ye Tian, Xiu Chen, Luting Xia, Yuqiong Jie . Fuzzy theory analysis on imagery modeling of wearable bracelet in the urbanian health management. Mathematical Biosciences and Engineering, 2021, 18(1): 600-615. doi: 10.3934/mbe.2021033 |
One of the major challenges that smart agriculture is expected to address is the efficient use of water resources. The conservation and the efficient use of clean water is a long-term strategy worldwide. Modeling of smart agriculture systems is an important factor because the processes there are very slow and sometimes it takes a year or more for a full crop cycle. At the same time, a large amount of data is usually needed to make informed decisions. This determines the importance of developing appropriate systems through which to simulate, generate, optimize and analyze various possible scenarios and prepare appropriate plans. In this paper, an infrastructure known as Virtual-Physical Space adapted for agriculture is presented. The space supports integration of the virtual and physical worlds where analysis and decision making are done in the virtual environment and the state of the physical objects (things) of interest is also taken into account at the same time. Special attention is paid to the possibilities for modeling an irrigation system. An ambient-oriented approach has been adopted, using the Calculus of Context-aware Ambients formalism as the basic tool for modeling agriculture processes. Furthermore, the supporting platform is briefly presented. Active components of the platform are implemented as intelligent agents known as assistants. Users (agriculture operators) are serviced by personal assistants. Currently, the presented modeling system is deployed over a two layered system infrastructure in the region of Plovdiv city. Plovdiv is the center of vegetable production in Bulgaria. The process of modeling intelligent irrigation systems and the current results are discussed in this paper.
The idea for precise and intelligent agriculture arises due to the efforts to obtain biologically clean and at the same time low-cost agricultural products, to minimize the load of the environment with pesticides and early to detect crop diseases. To solve these problems, people rely especially on the application of modern information and communication technologies. Particularly suitable are those that allow analysis and decision making taking into account the surrounding physical world. In the same time, agriculture is becoming an increasingly relevant area for conducting research and practical developments in the field of IoT (Internet-of-Things), CPS (Cyber-Physical Systems), and CPSS (Cyber-Physical-Social Systems). These three relatively new and interconnected technologies provide a variety of unthinkable opportunities to build infrastructures for smart and precise agriculture. IoT implements a concept of unified dynamic environments from proactive objects capable of detecting and interpreting the characteristics of the environment and of exchanging and accessing information aggregated by other objects, or participating as components of complex services; thus building a global infrastructure for the information society [1]. CPS was introduced to describe the rigorous blending and coordination between computing (cyber) and physical resources; there is a close integration between computations, communication, and task execution in the environment, in which the system is located [2]. CPSS first introduced in [3] emphasizes the deep interaction between the physical, cybernetic, and social world by integrating the physical and the social through an intelligent human-machine interaction in cyberspace to achieve the management and control of complex socio-technical systems [4,5].
Inspired by these technological breakthroughs a reference architecture known as Virtual Physical Space (ViPS) was developed at the Faculty of Mathematics and Informatics of Plovdiv University "Paisii Hilendarski", Bulgaria [6]. Recently, we are going to adapt it to build applications for smart agriculture. In this paper, such application for efficient management of water resources is presented; in particular, a model and supporting environment that can be used to analyze various irrigation scenarios; modeling and preliminary testing of possible irrigation plans, as well as the use of the hybrid analytical-algorithmic approach in the evaluation of these plans and scenarios.
The rest of the paper is organized as follows: related works are considered in section 2, which is followed by an overall review of ViPS adapted for smart agriculture in section 3. In section 4, the applicability of the proposed space for modeling irrigation systems is demonstrated. The results of the ambient-oriented modeling of the irrigation scenario are summarized and discussed in section 5. Finally, section 6 concludes the paper.
According to [7], despite the significant amount of fresh water on earth, rapid population growth is putting the world's water resources in crisis. While the amount of fresh water on the planet remains relatively constant over time, the need for clean water to sustain life is growing rapidly. Fresh water makes up a very small part of all the water on the planet; while nearly 70% of the Earth's surface is covered by water only 2.5% of it is fresh. Moreover, only 1% of the freshwater is easily accessible. Unfortunately, people have proved to be inefficient consumers of water. The challenge we face is the efficient use, conservation, management and allocation of water resources.
The conservation and the efficient use of clean water is a long-term strategy of the European Union [8]. As far back as 1975, a constantly updated directive on water resources was published. In paper [9] recognizes that water is a major resource for human health, agriculture, energy production, transport and nature. Ensuring its sustainable use remains a major challenge globally and within Europe. Currently, only 40% of surface water sources in Europe achieve good ecological status and wetlands are severely degraded as are 80–90% of floodplains. This situation has a critical impact on the conservation status of wetlands and the species that depend on them. Although the pollution of some water sources, nitrogen surpluses and water abstraction are reduced, the freshwater continues to be affected by diffuse pollution, hydro-morphological changes and water abstraction. The diffuse pollution and the water abstraction are expected to continue in response to intensive agricultural practices and energy production.
The current situation requires a new attitude and balancing the needs of society to ensure the availability of fresh water in nature. Climate change is likely to reduce the amount of water available on a regional scale, increasing the need for flood protection or land management and hampering this balance. Improved implementation and increased coherence between EU water-related targets and measures are needed to improve water quality and quantity. In the future, it will become increasingly critical to address and monitor the climate-water-ecosystem-agriculture link and the link to energy needs.
Both in Europe and in the world, great hopes are placed on the intensive use of modern ICT for the efficient management and use of water resources. This issue is becoming the subject of a rapidly growing number of research projects and practical applications. Currently, more and more specialized publications are addressing this issue. With the solution of more and various particular scenarios and situations, significant empirical knowledge is accumulated, which will help to build truly intelligent agriculture in the future.
In [10], a system for overcoming the physical limitations of a traditional irrigation system is presented. It consists of a sprinkler unit that can perceive and evaluate local soil moisture, communicate wirelessly and activate its own sprinkler mechanism, based on a centrally calculated schedule. A model has also been proposed to calculate the change in moisture due to calculation of runoff, absorption and diffusion. The solution is integrated in a framework for optimal planning of a set of valves installed in the irrigated area. The system is applied to intelligently irrigate lawns that are the largest irrigated area in North America with a daily demand for more than 9 billion gallons of fresh water. Paper [11] presents the results of attracting farmers to rural areas of Sri Lanka to study adaptation to water scarcity, including the shift to less water-intensive crops, collective cultivation and divided groundwater use. The impact of climate change on agricultural decision making is also examined based on expected utility and risk prevention. It is assessed how the introduction of individualized access to well irrigation water affects drought mitigation practices in the community. In [12], an intelligent agricultural cyber-physical system for precise agriculture is proposed. The purpose of the study is mainly to track the location of faults in real time in an agricultural field using data received from multidimensional sensors. The computer model uses a hybrid classification approach that combines two classifiers to estimate the location of the sensor devices. The novelty of the proposed method is to predict the places that need more irrigation, nutrients in the soil or direct human intervention using sensor data. In [13], an intelligent irrigation system with a multi-agent architecture using fuzzy logic is presented. The architecture consists of various types of intelligent agents that autonomously monitor the condition of irrigated areas and if necessary make decisions to activate/deactivate the irrigation system. This project provides a real and innovative solution to the problem of inefficient water use in current irrigation systems.
Our research is based on the above, aiming at precise watering by combining information from physical and logical sensor networks; making decisions and creating an appropriate irrigation plan in a multi-agent CPSS system and implementing irrigation using various static and mobile devices and actuators; assessment of the possibilities for optimization of the processes while preserving the traditional agricultural production in the region of Plovdiv.
ViPS supports integration of the virtual and physical worlds and puts users in the spotlight. An essential feature of ViPS is that the above-mentioned technologies—CPS, CPSS and IoT—are closely integrated in the space. In accordance with the ideas of CPS, we create a mixed virtual and physical space. In addition, we take into account the social aspects (mainly affected by the definitions of CPSS) as a major component of ViPS are personal assistants, that support users in their work in the ViPS space. The physical world is seen as a network of intelligent objects "things" operating in accordance with the IoT concept. Unlike the definition of CPS and CPSS, ViPS is space, with the main components being autonomous, reactive and proactive intelligent agents that interact with each other when necessary. As such, they do not fit into the classic multi-layered architecture.
Typically, a ViPS supported application analyses and makes decisions within its virtual environment where the state of the physical world in interest is also taken into account at the same time. The integration means both the physical objects of interest ("things") are virtualized in the virtual part of the space (bottom-up approach) and smart computational nodes are installed in the physical part of the space (top-down approach). ViPS adapted for smart agriculture consists of the following basic components: Analytical Subspace (including Personal Assistants), Operative Subspace, Agriculture Data and Knowledge Center, Guards Subspace, and External Interfaces (see Figure 1).
The Agriculture Data and Knowledge Center (ADK Center) stores background knowledge about agriculture and data obtained from the sensor networks located in the physical world. In this center, the objects are presented as instances of two structural templates. The first one, known as static (or features) template, contains the inherent attributes (characteristics) of the objects of interest. The second one called dynamic template (or meta-template), stores information about spatial, temporal and event aspects related to the corresponding object. Basically, the information is stored in ontologies and relational databases mutually integrated. The ADK Center provides also tools for receiving, storing, and processing large volume of structured, semi-structured, and unstructured agriculture data.
The Analytical Subspace (SP) is the operative center assisting agricultural operators in managing, controlling and coordinating all stages of needed activities. For this purpose, virtualization is mainly performed here, supported by various modeling environments located in this subspace. Currently, the temporal aspects of the things are modeled through the TNet (Temporal Net) component implemented on the basis of the Interval Temporal Logics [14]. The events in the analytical subspace are specified in accordance with the Event Model and are interpreted by the corresponding Event Engine [15].
In the current state of development, a major role in virtualization is assigned to the AmbiNet component that supports the spatial aspects of the things of interest. AmbiNet is an ambient-oriented modelling environment implementing the CCA (Calculus of Context-aware Ambients) [16]. It is a formalism for the presentation of ambients along with their boundaries, contexts and channels of communication. In AmbiNet, the "things" of interest (for instance, an irrigation system) are modelled as separate or hierarchical ambients.
The data for the operation of the modeling environment are provided by the ADK Center. The necessary information is accessed through the operational assistants located in the Operative Subspace. In this way, the decision making is completed in the virtual world of the space. If necessary, the Analytical Subspace is able to interact directly with the guards to deliver data from the sensor networks.
In the Analytical Subspace, the Personal Assistants are located as well; each agriculture operator may have its own assistant to interact with the space. Thus, the Personal Assistant operates as specialized interface between the smart agriculture space and the operators. Furthermore, an operator can prepare and run plans for various agricultural activities depending on the specific conditions. The work of personal assistants is supported by operational assistants. Usually, these are server components performing various auxiliary functions. The personal and the operative assistant are implemented as rational BDI agents [17].
The Guards Subspace operates as an interface between the virtual and physical worlds of the space. The guards implemented also as rational BDI agents serve to transform and transmit data between the two worlds. The physical world is an IoT Nodes Network that is able to collect up-to-date information on the "situation on the ground", i.e. sensory data from the observed open and closed agriculture areas (for example, greenhouses). In view of the fact that the climatic conditions and the terrain can be very different (especially in open areas) a wider variety of types of devices that make up the sensor network is required. The support network will be constructed from interconnected static devices such as sensors, actuators, and controllers. Currently, the guard network is complemented by mobile devices such as unmanned aerial vehicles (drones). An IoT node can be configured as intelligent one; i.e. it can house assistants and data repositories and is able to make local decisions. The communication between the separate IoT Nodes is mainly implemented on public Internet and private LoRaWAN network [18].
The task of the Smart Agriculture Platform is to provide basic software components with the help of which to implement the necessary functional modules of the space. The kernel of the platform is the JaCaMo framework [19]. The active components of the space (mainly the personal assistants and the operative assistants) are implemented as intelligent agents with BDI architecture. The assistants' environment is specified as artifacts [20]. The next section presents a part of the platform for solving a specific task of intelligent agriculture.
Currently, the presented space is applied to refine the use of water resources needed for watering crops. In order to adapt the proposed space to solve a specific problem, it is first necessary to create an abstract task model. Before presenting such a model, let's look at an agricultural area for which irrigation infrastructure will be built (see Figure 2).
The agricultural area is supplied with several water sources (S1, S2, …, Sk), stationary hydrants (h1, h2, …, hm) and an underground network of pipes and taps. This area can be divided into separate plots (p1, p2, …, pm) so that each hydrant (inside the plot) is able to supply the required amount of water for watering this plot. Inside the individual plots, separate irrigation systems can be located, which can be changed (drip irrigation, sprinkling, spraying, etc.).
Firstly, two physical things - underground and surface – are modelled as follows:
● SU: = {irrUi | i = 1, …, n } – a set of underground elements (pipes and taps) that ensure the transport of water to each plot in the cultivated area. We will call this space the Underground Irrigation Subspace (SU), and its elements - irrigation units.
● SS: = {pi|i = 1, 2, …, m } – a network of arable plots that correspond to one main hydrant hi, i = 1, 2, …, m and one measuring station that receives and processes information on the level of soil and air humidity in the plot. We assume that in each plot the water is received and distributed by one hydrant, and in the plot there is some type of irrigation network. We also assume that there is no overlap of the irrigated and monitored plots. We will call this subspace the Surface Subspace (SS), and its elements are arable plots. i.e., SS=m⋃i=1pi and m⋂i=1pi=∅
Then the whole physical space is SPACE = SU ∪ Ss. We look for irrigation plan in SU, taking into account the state of SS.
Informal irrigation plan is a set of operators (activities) for watering a certain agricultural area. An irrigation plan consists of two parts:
● Irrigation scheme—is a network of plots (their representations in cyberspace), which are designated for irrigation.
● Irrigation cycle—a series of operations performing watering, which can be performed sequentially or in parallel.
In this step we will model the creation of an irrigation plan, which involves elements from both subspaces:
● irrPlans = {irrPi | irrPi ⊆ 2SU} is the set of all irrigation plans in SU, ie. each irrigation plan is a subset of the elements of SU. With 2SU2SU we denote the Power Set, which contains all possible subsets of SU.
● IrrPi = (irrUi0, irrUi1, …, irrUig) is a specific irrigation plan such as irrUi ∈ irrPlans, as irrUi0 is the starting point (eg pumping station or other water source), and irrUig is the target hydrant hi. The ordered couple (irrUik, irrUik+1) for every k = 0, …, g-1 presents two connected adjacent irrigation units in the irrigation plan. In other words, an irrigation plan is a sequence of interconnected adjacent irrigation units.
● Connected Irrigation Units will be presented using the function Connected (irrUi, irrUj), if in SU there is an irrigation plan between irrUi to irrUj.
Let's first introduce some definitions related to Surface Subspace SS:
● Adjacent Plots in Ss are areas with a common border. In the model we will denote this fact by the function Adjacent (pi, pj).
● "Connection" of plots in SS - we say that a sequence of plots p1, p2, p3…pk is "connected" if for every two zones pi and pi+1 in the sequence is fulfilled: Adjacent(pi, pi+1) = True.
In the developed model, each plot in the arable area is connected to one measuring station. We will introduce a function (drought function-df) to determine the degree of drought for each plot.
● df: SS ⟶ R, this function compares to each plot a certain real number, which reflects the degree of drought. Ie df (p) = r, where the variable "p" represents the individual plots and "r" is a real number.
● DT is a real constant that represents the drought limit in the model.
Then we can give the following definitions:
● "Dried" plot p ∈ SS, is a plot for which the condition df (p) > DT is fulfilled.
● "Irrigated Plot" p ∈ SS is a plot for which the condition df (p) ≤ DT is fulfilled.
● "Totally irrigated" is the subspace SS, when for each plot pi ∈ SS, i = 1… m is satisfied: df (pi) ≤ DT.
● "Totally Dried" is the subspace SS, when for each plot pi ∈ SS, i = 1… m is satisfied: df (pi) > DT.
● "Plot limit humidity" is a plot p ∈ SS for which df (p) = DT is satisfied.
The current state of soil moisture must be taken into account when generating the irrigation plan. For this purpose, it is necessary to define different correspondences between the two subspaces SU and SS (in both directions), such as:
● irrU2p: SU ⟶ SS as a certain plot is compared on one irrigation unit.
● irrP2c: 2SU ⟶ 2SS. In this relation of one irrigation plan in SU we compare one "Connection" in SS.
● "Dry" irrigation plan irrP ∈ irrPlans is a plan, all irrigation units of which correspond to "Dried" plots.
In Figure 3, a part of the platform, which is used for the irrigation example considered in the paper, is presented.
The Personal Assistant (PA) and AmbiNet share a common environment modeled as artifacts. In this case, the artifacts are plots and the irrigation systems built in them. The artifacts were implemented using a typical object-oriented approach; i.e. some classes such as parcel and irrigator are specified as pattern for generating the necessary instances (objects) depending on the specific irrigation area. The AmbiNet complements artifacts with additional spatial metadata (such as location, boundaries, etc.). The work of PA and of the AmbiNet is supported by operational assistants.
A small scenario including a personal assistant, a supporting operative assistant, an artifacts environment, and a section of the assistants' plan library illustrates the work of the platform (see Figure 4.). The actions of the personal assistant agent are determined by two plans. One of these is for the initialization: the agent creates an instance of its own UserGUI artefact, so that its user can interact with it, and the agent needs to focus on it (in order to perceive any changes made by the user). The second plan reacts to changes in the user activity by sending the preferred moisture to the device agent. The changes in the activity are perceived by changes in the observable property activity from the UserGUI artefact on which the agent is focusing. In this plan we used "?pref_moist(M)". This is a test goal and different from achievement goals, these are used to retrieve information from the agents' belief base within the course of action determined by an executing plan. The "Irrigator" artifact is defined in main application file. Also there, the device agent is initialized to focus on it. Whenever the belief base (of device agent by the received message from personal assistant) is updated with new preferred moisture, the device agent takes actions to achieve the new preferred moisture.
An agent can have multiple plans that are relevant for the same goal to be achieved, as alternatives to be used depending on the situation. If the perceived current moisture is lower, greater or equal to the preferred one, then the necessary actions are taken: watering, drainage or no action accordingly.
From an architectural point of view, AmbiNet is part of the Analytical subspace of ViPS and its main task is to implement preliminary modeling of various situations and scenarios in the domain, taking into account the possible change of conditions in the physical and virtual worlds. On the other hand, AmbiNet is a network of ambients that present different physical and virtual components and nodes in a unified way. In this sense, AmbiNet implements its main functionalities based on a hierarchical structure of "ambients" [21].
AmbiNet's main task is to model possible scenarios; to prepare, analyze and record the appropriate irrigation plans in the storage of the ADK center. To achieve this goal, the mathematical notation and formal semantics of the Calculus of Context-Aware Ambients (CCA) [22] are used, which allows not only to be formally specified as a program (in program language ccaPL), but also programmatically interpreted. Through a specially developed visual editor, various possible scenarios can be modeled, which can be tested and verified using ccaPL Interpreter in console and animated mode (see Figure 5). As a result, a collection of pre-tested scenarios and interactions between the individual components of the environment is created.
The creation of CCA-models and the testing of possible irrigation plans make it possible to determine in advance their applicability, to correct discrepancies and to identify critical ambients that are overloaded in the process of interactions.
An important component of AmbiNet has been developed and integrated as an artifact into the AmbiNet Analyzer environment. It can work in both simulation and dynamic mode.
In simulation mode, various irrigation plans can be tested and analyzed using AmbiNet Analyzer, determining their level of usability according to the water saving goal. The measuring stations in the individual plots periodically send information about the values of the function df(p). In addition, information on the condition and activity of water sources, the presence of impassable sections (such as rocks, walls and other obstacles) is submitted to the Analytical Subspace. In AmbiNet this information is processed and the data is periodically sent to the farmer's PA. Figure 6 shows part of the user interface of the personal assistant of the farmer's mobile device. The personal assistant as well as the other agents in JaCaMo are developed in Java, and the user interface is available for all types of mobile operating systems.
In real-time operation, in the event that sensors from some of the plots provide information in excess of the critical drought value (df(pi) > DT), PA initiates communication with the user, who must take action, associated with watering these plots. In this situation, there are two main scenarios, as follows:
● If there is an appropriate plan in the plan library to ensure that the desired goal is achieved, the PA offers the plan to the user and, after obtaining the consent, informs the Operative SP, which activates its implementation.
● If there is no plan in the plan library to achieve the goal in the specific situation, the values of df(pi) > DT are passed to AmbiNet Analyzer, which works in dynamic mode. Through its component AmbiNet Irrigation Plan Generator different variants are considered and possible irrigation plans are generated.
Figure 7 shows the AmbiNet Irrigation Plan Generator for a specific situation - thirty-five plots; three water sources (S / A01, S / A12 and S / A22 in blue); five impassable obstacles (A03, A06, A07, A08, A13 and A21 in black); five dried plots (A10, A15, A17, A24 and A31 in gray) and fourteen irrigated areas in green.
When choosing a water source, analytical information is printed about the possibility of generating an irrigation plan. When generating an irrigation plan (from the selected water source) and information about the number of steps for its implementation is displayed.
In case such a plan does not exist, information about it is displayed and a procedure is started to look for other possible options for watering—by sprinkling, the use of surface canals, mobile equipment, sprinklers, etc.
To generate possible irrigation plans, we use an algorithm modified with an appropriate heuristic function from the classic AI "greedy search", which is guaranteed to find an irrigation plan, if one exists. The algorithm is not optimal, which requires the development of a special AmbiNet Plan Optimizer.
We are currently solving this problem through the capabilities of the existing AmbiNet Analyzer by analyzing the NumSteps parameter for each possible irrigation plan and sending this information to the farmer via his PA (see Figure 8).
The solution of the problems in the considered aspect is realized by using an analytical and algorithmic approach. The analytical approach makes it possible to conclude whether there is a possible irrigation plan. If such a plan does not exist, the PA, Operative SP and Analytical Subspace receive information about it immediately and do not start the search algorithm. This increases the speed of decision making and saves machine time and communication resources. Analytical information is currently displayed in the following cases:
● Тhe some water source is inaccessible.
● Horizontal obstacle line between selected water source and some dried plot.
● Vertical obstacle line between selected water source and some dried plot – see Figure 9 (a).
● All areas adjacent to the source are inaccessible—see Figure 9 (b).
● All plots adjacent to the source are irrigated. Choose another water source – see Figure 9 (c).
● Horizontal irrigated line between source and dried plot. Choose another water source to save water.
● Vertical irrigated line between source and dried plot. Choose another water source to save water – see Figure 9 (d).
For the functioning of the space it is especially important to determine the situations that can be processed analytically and those that require an algorithmic solution. To achieve this goal in the Analytical Subspace we implemented a special tool in AmbiNet Analyzer (see Figure 10).
Its task is to make statistical processing of the various situations and to enable informed decisions to be made - for the parceling of the areas, for the number and location of the measuring stations, for the determination of the possible impassable zones, etc.
The simulated situations and the processed data make it possible to determine different dependencies between the observed parameters. Figure 11 shows the relationship between the analytical and algorithmic solutions in % according to the number of plots (35) at three water sources and the presence of three impassable obstacles.
The analysis of the results of the testing of the created prototype of the system determined the need to add some additional functionality. We believe that the development of an algorithm for generating an irrigation plan with the combined use of different water sources with different flow rates and locations will ensure optimization of the created irrigation plans and will allow to more fully realize the goal of water saving. Further analysis of common situations through the use of heuristics and expertise in the domain in question is needed. Thus prepared in advance possible irrigation plans will increase the productivity of the system in real time. We believe that the use of various approaches such as Case-Based Reasoning [23] to extract irrigation plans from ADK Center, which have a high percentage of similarity with the specific values of the observed parameters, will also support the system optimization process.
The analysis of different situations for different values of the observed parameters - number and location of plots, number and location of water sources and location of obstacles gives grounds for optimizing the parceling process and creating an appropriate model available at the measuring stations. The aim is to increase the situations that can be solved analytically, which will improve the speed of information processing at all infrastructure levels (edge, fog, cloud) and will increase the overall usability of the space.
AmbiNet Irrigation Plan Generator and AmbiNet Analyser are programmed as separate artifacts in the multi-agent JaCaMo platform. Upon receiving the specific information about the state of the physical environment, their task is to analyze and generate irrigation plans and to provide the information in simulation and real mode to the operational and personal assistants. In addition, these tools can be used directly by system administrators.
The paper deals with the infrastructure of a system for intelligent agriculture. The model of an irrigation installation is presented in more detail. Currently, the space is deployed over a two layered system infrastructure in the region of Plovdiv city. Plovdiv is the center of vegetable production in Bulgaria. A specific irrigation area has been selected, modeled as shown in the paper. The selected area is parceled, a drip irrigation system is built and irrigation plans are generated in accordance with the described model and individual regimes for watering the individual plots are determined. The local level consists of two open area sensor networks and one tomato greenhouse sensor network. The second regional level is a private cloud. In the future, it is planned to build a national center for intelligent agriculture, which will be located on a national supercomputer. A link between the regional and national levels is also envisaged.
Pre-modeling, simulation, verification and optimization of appropriate plans and scenarios are especially important in the context of agriculture, as processes develop slowly over time and sometimes a cycle takes more than a year.
The proposed infrastructure can also be used for other agricultural activities, such as fertilizers and plant treatment, provided that fertilizers and medicines are dissolved in the water. In addition, we plan to implement other functions in the infrastructure such as reporting and forecasting of water resources, control and management of water quality. Another group of functions can help maintain, repair and improve the technical infrastructure.
This work was supported by the Bulgarian Ministry of Education and Science under the National Research Programme "Smart crop production" approved by Decision of the Ministry Council №866/26.11.2020 and partly supported by the project BG PLANTNET, Grand KP-06X36/2.
We acknowledge the provided access to the e-infrastructure of the Centre for Advanced Computing and Data Processing, with the financial support by the Grant BG05M2OP001-1.001-0003, financed by the Science and Education for Smart Growth Operational Program (2014–2020) and co-financed by the European Union through the European structural and Investment funds.
All authors declare no conflict of interest in this paper.
[1] | О. Vermesan, P. Friess, Internet of Things: Converging Technologies for Smart Environments and Integrated Ecosystems, River Publishers, 2013. |
[2] | R. Ragunathan, I. Lee, L. Sha, J. Stankovic, Cyber-Physical Systems: The Next Computing Revolution, in Proceedings of the Design Automation Conference (2010), Anaheim, California, USA, 731-736. |
[3] | F. Y. Wang, The emergence of intelligent enterprises: From CPS to CPSS, in IEEE Intelligent Systems, 25 (2010), 85-88. |
[4] |
M. Dong, R. Ranjan, A. Y. Zomaya, M. Lin, Guest editorial on advances in tools and techniques for enabling cyber-physical-social systems - Part Ⅰ, IEEE Trans. Comput. Soc. Syst., 2 (2015), 38-40. doi: 10.1109/TCSS.2016.2527158
![]() |
[5] |
M. Dong, R. Ranjan, A. Y. Zomaya, M. Lin, Guest editorial on advances in tools and techniques for enabling cyber-physical-social systems - Part Ⅱ, IEEE Trans. Comput. Soc. Syst., 2 (2015), 124-126. doi: 10.1109/TCSS.2016.2527159
![]() |
[6] | S. Stoyanov, T. Glushkova, E. Doychev, A. Stoyanova-Doycheva, V. Ivanova, Cyber-Physical- Social Systems and Applications. Part Ⅰ: Reference Architecture, LAP LAMBERT Academic Publishing, 2019. |
[7] | Freshwater crisis. Available from: https://www.nationalgeographic.com/environment/freshwater/freshwater-crisis. (visited at 06.08.2020). |
[8] | European Commission, EU Water Framework Directive. Available from: https://ec.europa.eu/environment/water/water-framework/info/intro_en.htm (visited at 10.04.2021). |
[9] | The European environment - state and outlook 2020. Knowledge for transition to a sustainable Europe, European Environment Agency, 2019. |
[10] | D. A. Winkler, R. Wang, F. Blanchette, M. Carreira-Perpinan, A. E. Cerpa, MAGIC: Model-Based Actuation for Ground Irrigation Control, in Proceedings of the 15th ACM/IEEE International Conference on Information Processing in Sensor Networks, (2016), 91-102. |
[11] | E. K. Burchfield, J. M. Gilligan, Dynamic of Individual and Collective Agricultural Adaptation to Water Scarcity, in Proceedings of the 2016 Winter Simulation Conference, (2016), 1678-1689. |
[12] | A. Pandey, P. Tiwary, S. Kumar, S. K. Das, A Hybrid Classifier Approach to Multivariate Sensor Data for Climate Smart Agriculture Cyber-Physical Systems, in Proceedings of the 20th International Conference on Distributed Computing and Networking, (2019), 337-341. |
[13] | R. Salazar, J. C. Rangel, C. Pinzón, A. Rodríguez, Irrigation System through Intelligent Agents Implemented with Arduino Technology, Adv. Distrib. Comput. Artif. Intell. J., 2 (2013), 29-36. |
[14] | B. Moszkowski, Compositional reasoning using Interval Temporal Logic and Tempura, in International Symposium on Compositionality, (1997), 439-464. |
[15] | Z. Guglev, S. Stoyanov, Hybrid approach for manipulation of events in the Virtual Referent Space, in Proceedings of the International Scientific Conference Blue Economy and Blue Development, (2018), 197-203. |
[16] |
F. Siewe, H. Zedan, A. Cau, The Calculus of Context-aware Ambients, J. Comput. Syst. Sci., 77 (2011), 597-620. doi: 10.1016/j.jcss.2010.02.003
![]() |
[17] | M. Wooldridge, An Introduction to MultiAgent Systems, John Wiley & Son, 2009. |
[18] | LoRa Alliance. Available from: https://lora-alliance.org/about-lorawan. |
[19] | O. Boissier, R. H. Bordini, J. F. Hübner, A. Ricci, Multi-Agent Oriented Programming: Programming Multi-Agent Systems Using JaCaMo, The MIT Press, 2020. |
[20] | A. Ricci, M. Piunti, M. Viroli, Environment programming in multi-agent systems: an artifact-based perspective, Auton. Agents Multi-Agent Syst., 23 (2010), 158-192. |
[21] |
D. J. Cook, J. C. Augusto, V. R. Jakkula, Ambient intelligence: Technologies, applications, and opportunities, Pervasive Mobile Comput., 5 (2009), 277-298. doi: 10.1016/j.pmcj.2009.04.001
![]() |
[22] | F. Siewe, H. Zedan, A. Cau, The calculus of context-aware Ambients, J. Comput. Syst. Sci., 77 (2010), 597-620. |
[23] | S. Stojanov, I. Popchev, D. Chaushkova, M. Trendafilova, A case based reasoning approach for development of intelligent services, in Proceedings of the 5th international conference on Computer systems and technologies (2004), 1-7. |
1. | Carey Louise B. Arroyo, Newton John O. Suganob, Ronnie S. Concepcion, Robert Kerwin C. Billones, 2023, Interplay of Cyber Physical Systems for Space Technologies with Emphasis on Space Crop Farming, 979-8-3503-8117-7, 1, 10.1109/HNICEM60674.2023.10589061 |