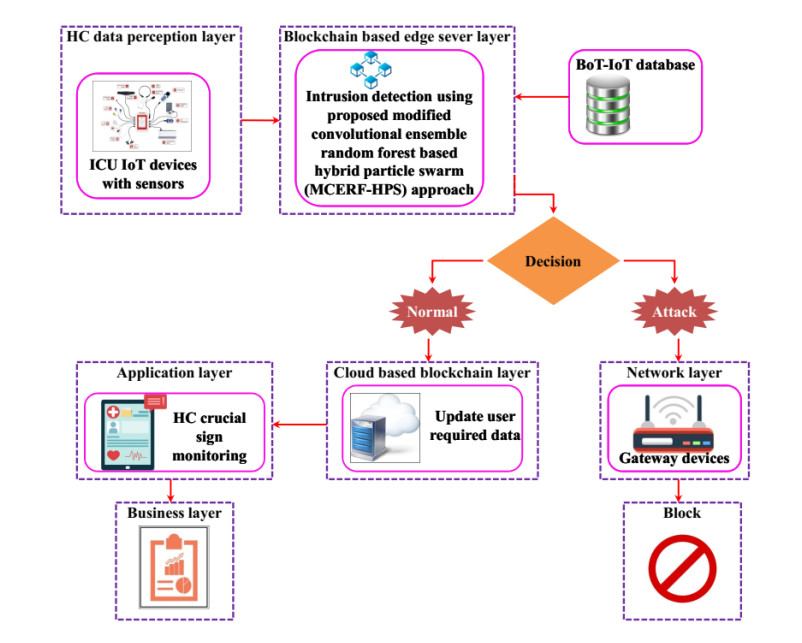
The Internet of Things (IoT) plays a vital role in the rapid progression of healthcare diligence. In recent years, IoT has become one of the most significant sources in the medical domain, since physical devices collect essential patient information to share real-time data with medical practitioners via various sensors. Meanwhile, numerous existing intrusion detection techniques failed to meet the security needs to safeguard the patient data collected. If an attack or intrusion cannot be identified at a particular time, immeasurable damage will be developed, which will fail the system. Utilizing innovative and new technologies, namely Blockchain, edge computing, and machine learning, provides a powerful security solution to preserve the medical data of various patients. This paper proposes a modified convolutional ensemble random forest-based hybrid particle swarm (MCERF-HPS) approach to guarantee healthcare data security with the advancement of blockchain technology. The proposed MCERF-HPS-based intrusion detection system identifies and categorizes attacks and regular traffic in blockchain-based edge systems. In immediate response to the identification, the gateway devices in the network layer block the attack traffic within seconds, with fewer computing and processing abilities. Applying the detection mechanism at the edge layer close to the attack source provides a quick detection response and minimizes the workload of clouds. The proposed MCERF-HPS approach's ability to detect an intrusion is tested using the BoT-IoT database. The analytic result illustrates that the proposed MCERF-HPS approach achieves an improved attack detection accuracy of about 98.7% compared to other methods.
Citation: Sahar Badri. HO-CER: Hybrid-optimization-based convolutional ensemble random forest for data security in healthcare applications using blockchain technology[J]. Electronic Research Archive, 2023, 31(9): 5466-5484. doi: 10.3934/era.2023278
[1] | Mohd. Rehan Ghazi, N. S. Raghava . Securing cloud-enabled smart cities by detecting intrusion using spark-based stacking ensemble of machine learning algorithms. Electronic Research Archive, 2024, 32(2): 1268-1307. doi: 10.3934/era.2024060 |
[2] | Rizhao Cai, Liepiao Zhang, Changsheng Chen, Yongjian Hu, Alex Kot . Learning deep forest for face anti-spoofing: An alternative to the neural network against adversarial attacks. Electronic Research Archive, 2024, 32(10): 5592-5614. doi: 10.3934/era.2024259 |
[3] | Shaochen Lin, Xuyang Liu, Xiujuan Ma, Hongliang Mao, Zijian Zhang, Salabat Khan, Liehuang Zhu . The impact of network delay on Nakamoto consensus mechanism. Electronic Research Archive, 2022, 30(10): 3735-3754. doi: 10.3934/era.2022191 |
[4] | Mohammed Alshehri . Blockchain-assisted cyber security in medical things using artificial intelligence. Electronic Research Archive, 2023, 31(2): 708-728. doi: 10.3934/era.2023035 |
[5] | Abul Bashar . Employing combined spatial and frequency domain image features for machine learning-based malware detection. Electronic Research Archive, 2024, 32(7): 4255-4290. doi: 10.3934/era.2024192 |
[6] | Tej Bahadur Shahi, Cheng-Yuan Xu, Arjun Neupane, Dayle B. Fleischfresser, Daniel J. O'Connor, Graeme C. Wright, William Guo . Peanut yield prediction with UAV multispectral imagery using a cooperative machine learning approach. Electronic Research Archive, 2023, 31(6): 3343-3361. doi: 10.3934/era.2023169 |
[7] | Fukui Li, Hui Xu, Feng Qiu . Modified artificial rabbits optimization combined with bottlenose dolphin optimizer in feature selection of network intrusion detection. Electronic Research Archive, 2024, 32(3): 1770-1800. doi: 10.3934/era.2024081 |
[8] | Feng Qiu, Hui Xu, Fukui Li . Applying modified golden jackal optimization to intrusion detection for Software-Defined Networking. Electronic Research Archive, 2024, 32(1): 418-444. doi: 10.3934/era.2024021 |
[9] | Hui Xu, Longtan Bai, Wei Huang . An optimization-inspired intrusion detection model for software-defined networking. Electronic Research Archive, 2025, 33(1): 231-254. doi: 10.3934/era.2025012 |
[10] | Shuang Yao, Dawei Zhang . A blockchain-based privacy-preserving transaction scheme with public verification and reliable audit. Electronic Research Archive, 2023, 31(2): 729-753. doi: 10.3934/era.2023036 |
The Internet of Things (IoT) plays a vital role in the rapid progression of healthcare diligence. In recent years, IoT has become one of the most significant sources in the medical domain, since physical devices collect essential patient information to share real-time data with medical practitioners via various sensors. Meanwhile, numerous existing intrusion detection techniques failed to meet the security needs to safeguard the patient data collected. If an attack or intrusion cannot be identified at a particular time, immeasurable damage will be developed, which will fail the system. Utilizing innovative and new technologies, namely Blockchain, edge computing, and machine learning, provides a powerful security solution to preserve the medical data of various patients. This paper proposes a modified convolutional ensemble random forest-based hybrid particle swarm (MCERF-HPS) approach to guarantee healthcare data security with the advancement of blockchain technology. The proposed MCERF-HPS-based intrusion detection system identifies and categorizes attacks and regular traffic in blockchain-based edge systems. In immediate response to the identification, the gateway devices in the network layer block the attack traffic within seconds, with fewer computing and processing abilities. Applying the detection mechanism at the edge layer close to the attack source provides a quick detection response and minimizes the workload of clouds. The proposed MCERF-HPS approach's ability to detect an intrusion is tested using the BoT-IoT database. The analytic result illustrates that the proposed MCERF-HPS approach achieves an improved attack detection accuracy of about 98.7% compared to other methods.
Nowadays, healthcare applications are determined by innovative applications such as the Internet of Things (IoT) for maintaining accurate data. Intelligent applications are widely circulating in human life, which re-conceives the quality of living through intelligent healthcare. The process of innovative applications is processed based on layer-wise specifications. The lower layer is the sensing layer used to gain data, and the communication layer is used to transform the data for processing. The intrusion detection method obtained in healthcare applications is widely used to monitor the records of patients. If any illegal modifications have occured in the health record, intrusion detection overcomes the issue and protects the record safely [1]. The health care data are maintained using sensor devices in the earlier stage. Later the redundant data present from a group of data are identified based on the cyber security of intrusion detection methods. It is highly employed in detecting the anomalies obtained in healthcare records. The data transmitted based on Blockchain is secured in intrusion detection [2]. The utilization of IoT in health care improves the efficiency and health of the patient. The IoT in intrusion detection helps to accurately monitor patient health; most healthcare industries focus on this method. Intrusion detection is obtained as a bridge between patients and doctors to communicate about health records, which helps to reduce the spread of fake data [3]. Intrusion detection is categorized in healthcare applications based on detection methods such as anomaly, signature, and specification. The signature-based intrusion detection system (IDS) detects new attacks that help to enhance the accuracy and minimize the false alarm rate [4].
The ensemble learning-based healthcare application frequently monitors the patient's health. The IDS provides security at the physical layer of data [5]. The complex data sources in the healthcare industry are patient records, sensors, embedded devices, and online sources. These features are widely used to predict the disease at an earlier stage. The IDS is a security framework applied to data encryption and restricts malicious attacks in healthcare [6]. The essential data required to analyze the patient's health is Patient Health Record (PHR). Digital healthcare plays a significant role in sending and receiving the patient's health records. The security of health records is considered more critical, so the digital healthcare system is combined with the blockchain method, thereby improving security [7]. The Blockchain contains a peer-to-peer (P2P) and decentralized network, classified into public, private, and consortium blockchains. Healthcare is considered significant in industrial applications to manage blood sugar levels and to check the patient's heart rate.
The cyber attacks occuring on health records create a lag in delivering patient records [8]. The stolen data is modified, which creates malicious health issues. The machine learning method in IDS is used to immediately detect intrusions. The IDS is divided into two types: misuse IDS and anomaly IDS. The misuse of IDS determines system attacks, but it cannot identify new attacks [9]. The malicious attacks are found accurately by merging the data mining techniques with IDS. The attacks are predicted and classified in the machine learning method. The IDS is the cyber security technique obtained in the hybrid optimization of healthcare [10].
Nowadays, healthcare applications are determined by innovative applications such as IoT to maintain accurate data. IoT has become one of the most essential and substantial resources in the medical domain, since physical devices enable real-time patient data to be shared with medical practitioners through various sensors. Meanwhile, many existing techniques fail to resolve security requirements issues and protect accurate data collected from patients. To solve the problem of the existing method, this paper proposes a new intrusion detection mechanism to prevent sensitive medical records from intruders by maintaining secure transactions over the network layers in healthcare applications. A secure blockchain-based edge system using the modified convolutional ensemble random forest-based hybrid particle swarm (MCERF-HPS) approach detects attack traffic to block and protect patients' sensitive medical records from intruders. After detecting the presence of an attack, gateway devices immediately block their paths in a short detection period, with low processing and computing capabilities.
This paper proposes a new intrusion detection mechanism to prevent sensitive medical records from intruders by maintaining secure transactions over the network layers in healthcare applications. The key contributions of this paper are discussed as follows:
● An MCERF-HPS approach is established to detect and to accurately classify attacks by learning their features;
● The proposed intrusion detection module at the blockchain-based edge layer is closer to the attack source to detect attacks within a smaller duration and with fewer computing and processing abilities;
● To develop a hybrid particle swarm optimization algorithm to autotune the modified convolutional neural network parameters and ensemble random forest algorithm to increase the detection efficiency;
● And to prevent and preserve the sensitive medical records of patients from intruders, a secure blockchain-based edge system using the MCERF-HPS approach is developed to detect attack traffic.
The rest of this paper is structured as follows. In Section 2, different authors explain the literary works of IDS based on healthcare applications. Section 3 presents the proposed methodology of the MCERF-HPS algorithm. Section 4 discusses the experimental results, and Section 5 concludes the article.
Kumar et al. [11] illustrated the ImmuneNet framework for recognizing intrusion attacks and healthcare data security. BellNet datasets were employed for the performance evaluation. The metrics such as precision, F1-Score, recall, sensitivity, accuracy, and binary cross entropy loss were used to predict a reasonable performance rate. Moreover, this method achieved a greater accuracy of about 99.63%. ImmuneNet was not biased to the false positive and the negative. Though this framework is practical, it is also time consuming. Rashid et al. [12] elaborated the tree-based stacking ensemble technique (SET) with feature selection for network intrusion detection. The datasets, namely University of New South Wales-network intrusion dataset (UNSW-NB) and network security laboratory-knowledge discovery in databases (NSL-KDD), were applied for a performance evaluation. The combined feature selection technique helped in choosing appropriate features along with the SET. This method was good at identifying the anomaly and regular traffic in the network. However, this technique was more time-consuming in the calculation.
Abbas et al. [13] developed a concept of an ensemble learning-based intrusion detection system for the detection of all types of attacks. Logistic regression, decision tree, and naive bayes were deployed with a voting classifier. The CICIDS2017 dataset was employed for a good performance evaluation. Moreover, the accuracy was taken in both multi-class and Binary-class for the performance evaluation. Thus, the result showed that the accuracy of this method is improved concerning multi and binary-class classificationd. However, high-level security is not possible with this method. Ashraf et al. [14] illustrated an intrusion detection system for healthcare applications in the IoT by using the federated learning-based artificial neural network (FL-ANN) technique. Automatically monitoring health conditions, saving medical data on a cloud environment, and sending that medical report directly to doctors were challenging tasks. The FL was introduced to prevent the problem of centralized manners such as locally trained data and protects from poisoning attacks. The established FL-ANN technique was tested and validated using several data sets such as BoT-IoT and the KDD cup 99 data set. As a result, the introduced technique was achieved to minimize the insufficient storage space, to effectively secure the patients' data, and to minimize the computing time. On the other hand, the behavior of the network was not defined.
Chandol et al. [15] established using the Border collie cat optimization-based deep neural network (BCCO-DNN) algorithm for detecting intrusion in healthcare applications. Protecting medical data from attacks was a major problem. Detecting the attacks on the IoT was to improve security. In this thesis, the BCCO-DNN algorithm was developed to prevent healthcare data. Several evaluation parameters were attained to improve the detecting accuracy rate of 0.9375.
Meanwhile, it was more suitable with a small amount of data. If there was an excessive amount of data, it gave a poor detecting accuracy rate. Fouda et al. [16] elaborated on intrusion detection in the healthcare IoT utilizing the Deep subclass dispersion one-class support vector machine (Deep SDOSVM) method. Securing healthcare data from various attacks was the primary goal of this article. The dataset was collected from the TON-IoT repository. The Deep SDOSVM algorithm effectively reduced the data dispersion and enhanced the intrusion detection's classification performance and discriminative power. However, a lack of probability was the major limitation of using these methods.
Saif et al. [17] discussed the hybrid intelligent intrusion detection system (HIIDS) based on metaheuristic algorithms and machine learning for healthcare applications. This paper aimed at intrusion detection on cloud servers for detecting security attacks. The popular NSL-k DD dataset was used for evaluating the HIIDS. Various techniques were utilized for feature selection, and supervised learning algorithms such as a decision tree (DT) and a known nearest neighbor (KNN) were used to classify selected features. The result found that the HIIDS obtained maximum accuracy with limited features.
Meanwhile, hybrid intelligent intrusion detection systems require more devices to respond to attacks. Saif et al. [18] illustrated the machine learning-based intrusion detection system (MLIDS) for healthcare applications. The intrusion detection model used the specialized dataset WSN-DS, which included attacks such as flooding, scheduling, grayhole, and regular data packets. The classification algorithms such as J48, KNN, random forest, naive bayes, and SVM were used for generating and selecting the better model based on detection accuracy. The result found that the intrusion detection model based on the random forest algorithm achieved a better accuracy of 99.9% for regular data packets. On the other hand, MLIDS required more processing time, and the complexity was high.
Taloba et al. [19] developed a blockchain-based hybrid technology to secure healthcare applications in the IoT. The main objective was to manage the vast data, provide more security, and prevent the healthcare application from attacks. The developed approach provides a security framework by creating a hash for each piece of information. Any changes or modifications in information and violations of medicines can be proven for the entire blockchain framework. On the other hand, this method requires a higher cost.
Kanagala et al. [20] established a cyber-physical system (CPS) based on a modified DL approach to improving data security. The DL method helps to classify and process data generated from the IoT and transforms that data into knowledge data. Data is protected based on policy access control against attacks like DDoS and DoS. As a result, the established approach enables the effective classification of data and the maintenance of reliable data with a higher accuracy rate. Meanwhile, this method was possible due to data loss.
Liu et al. [21] illustrated a blockchain-based federated learning method to provide security in healthcare applications based on CPS. The task content committee maintains the distributed ledger, which is comprised of representatives from hospitals performing FL tasks. They used a safe FL task model to develop standard modules. They evaluate the proposed computational platform based on real-time healthcare data, and the results show its effectiveness in providing incentives to FL participants and achieving FL model integration truthfulness. However, the set of familiar entities in participating devices in FL was minimal.
Although there are many ways to secure the healthcare data application over the IoT, there are still many gaps in the literature. Some of the research gaps are described as follows:
● Data privacy: Protecting healthcare applications from unauthorized access is highly significant, but the existing techniques are affected by the loss of confidential information. The proposed method enhances security and prevents data loss, and also solves the problem of interoperability and control of healthcare applications.
● Data security: Maximizing healthcare digital data requires innovative methods to process and store the data. Improving the security of transmission and the storage of healthcare applications is challenging. Several existing studies have been conducted to improve healthcare application security using Blockchain. However, confidential information can be easily hacked. The proposed method enhances security by adding transport layer security.
Cyber-physical systems (CPS) are more susceptible to attacks due to large-scale employment, reliance on confidential information, and their heterogeneous nature. One of the crucial and challenging problems raised in the healthcare system is a security risk, in which it faces difficulties in transmitting and processing data to provide secure access to authorized users. Aimed at improving the security of healthcare applications where the sensitive medical records of patients are stored, this paper comes up with a novel MCERF-HPS-based intrusion detection mechanism applied in blockchain-based edge systems to prevent malicious traffic/attack interference on sensitive information. The proposed secure blockchain-based healthcare (HC) system is composed of different layers: the data perception layer, edge layer, network layer, cloud layer, application layer, and business layer. The ICU IoT devices, namely patient health and room environment monitoring devices, collect patient medical records in the data perception layer. To prevent and preserve the sensitive medical records of patients from intruders, a secure blockchain-based edge system using the MCERF-HPS approach is developed for detecting attack traffic. After identifying the presence of an attack, the gateway devices immediately block their pathways in a short detection duration with diminshed processing and computing capacities. This procedure enables a robust security solution to safeguard the privacy of medical information.
The data perception layer includes an intensive care unit IoT with sensors. ICU devices can be divided into two devices: patient healthcare sensing devices and room environmental sensing devices [14]. The data perception layer is comprised of diverse sensors to determine the unique object, thereby dealing with collected data.
The edge layer contains the IoT gateway. Every gateway contains some health monitoring services. In health care monitoring, there is no universal internal protocol. Thus, gateways help to accept many network protocols. Additionally, the gateway is responsible for detecting a multi-attack. A lightweight intrusion detection system (IDS) is employed to identify the various attacks and upgrade data. The time to detect an intrusion is shorter when the attack sources are closer. Moreover, diminished computing and processing power are required because the federated learning mechanism deals with lesser data sets. After finishing the learning process, every local sample weight is transmitted to a blockchain-distributed ledger and saved in chain blocks.
At last, the chain is secured using a cryptographic hash function, which binds the blocks together. As a result, the edge layer working consensus algorithms cannot be changed. This mechanism helps to solve the problem of poisoning attacks. Each edge server receives the upgraded weight values and converts the collected data, utilizing its secret key to create the equivalent signature. The edge server then integrates the cryptography and gives it a signature to the implemented blockchain layer. If each edge server data is received, the intelligent contract validates the messages by public keys of the edge server, and blockchain hash blocks data are saved under the bright contract conditions.
This section utilizes a modified convolutional neural network (CNN) and processes various hidden layers and three channels [22]. The modified CNN is utilized for learning the feature representation and for processing more background noise. The feature map size of every layer is minimized gradually by extracting features. The CCCP layer is used to enhance the information within various channels. The convolutional layers feature map is computed is expressed as follows:
giu(j,k)=N∑nO∑oYi(j−n,k−o)lmu(n,o). | (1) |
From the above equation, the total number of convolutional layers is represented by Ui, the input of the layer is indicated by Yi, and the pixel index is represented by (j,k). The i convolutional layer contains sub-characteristic maps Gi, and is expressed as follows:
Gi=(gi1,gi2,.....,giU). | (2) |
The CCCP layer compresses the feature map, and is calculated and expressed as follows:
GiCCCP(u′)=Ii,u′CCCPGi=U∑uIi,u′CCCPgiu, | (3) |
GiCCCP=(Ii,1CCCP,Ii,2CCCP,.....,Ii,dCCCP)Gi. | (4) |
From the above equation, the CCCP layer's convolutional kernel index is represented by u′, the total number of the convolutional kernel layer is indicated by d, the feature size is indicated by GiCCCP, and it has lower channels to complete the dimension reduction and information compression. The fusion features are acquired by connecting the feature maps with the top convolutional layers. The fusion features contain a feature that is the extraction of convolutional layers and has a higher generalization performance. It serves as the input for softmax and a fully connected layer for classifying the networks.
The development of a random forest is made with various decision trees, which are not broken while processing [23]. The presence of multiple decision trees obtained the result more accurately and does not contain redundant data. The features of a random forest are selected automatically. A bootstrap sampling is modified in the minor class with a replacement algorithm, and in minority class samples, the K-nearest neighbor (KNN) [24] is determined. The classifier of the random forest is designed in every sampling group. Every classifier validates the training dataset which is terminated from the class. After execution, the dataset is uniformly tested by the random forest, and finally, the estimated outcomes are stored by the spark driver. These are the issues obtained in the random forest algorithm. The problems mentioned above are solved by designing the global partition algorithm. Store the datasets with similar keys into the same partition, and the partitions depend on the identical node. The formation of the sampling datasets is generated several times in an ensemble random forest, and the user feature information gain upgrades the iteration process. Therefore, the Apache Spark platform executes the complete algorithm parallel to estimate the calculation. The ensemble learning algorithms are generated in Spark MLlib, such as boosting trees and random forests. Various algorithms optimize the directed acyclic graph (DAG) computing model and merge ensemble and sampling algorithms to evaluate the imbalance feature dataset based on the classification model. So the random forest algorithm implemented in Spark MLlib is altered to obtain the bootstrap sampling model while performing classification [25].
In the search process, the inertia weight δ is the crucial parameter contributing greatly to the exploration and exploitation phase [26]. Hence, the choice of appropriate parameter δ is notable, and the inertia weight is a complicated nonlinear system from the physical point of view. For the adjustment δ, a nonlinear chaotic map is applied in the PSO algorithm [27]. Moreover, sensitivity, ergodicity, and randomness are the special features of the chaotic map. The numerical expression δ is given in the following equation, where B = 4:
yu=B.yu−1.(1−yu−1)yu∈(0,1), | (5) |
δ(u)=(δMin−δMax).(UMax−u)UMax+δMin.yu. | (6) |
Strategies of dimensional and elite learning
The particle swarm optimization (PSO) adopts the global and persona learning strategies in guiding the position update and velocity of the particle. The PSO best experience, namely the global best experience, helps speed up the solution progress. However, this strategy gets caught in a local optimal when resolving multimodal functions. Therefore, a dimensional and elite learning strategy is introduced to solve this issue. Every particle j learns from the four different qbestej particles and randomly chooses from the population.
Dqbest(u)=ArgMin{g(qbesteb(u)),....e(qbestec(u))} | (7) |
Gqbestej(u)={Dqbset(u),g(Dqbest(u))<g(qbestec(u))qbestej(u),otherwise | (8) |
The above equation g(.) represents the fitness function. The population diversity is caused by overemphasis hbeste, and the dimensional learning method solves the potential problem. The mathematical expression of the global particle Nqbeste is formulated as follows:
Nqbestu(u)=(1O∑oj=1qbest1j(u),1O∑Oj=1qbest2j(u),....1O∑Oj=1(u)). | (9) |
The velocity update equation is changed into the following:
Wej(u+1)=δ(u)Wej(u)+d1∗s1∗(Gqbestej(u)−Yej(u))+d2∗s2∗(Nqbeste(u)−Yej(u)) | (10) |
The strategy of adaptive position update
In the search process, the exploitation and exploration are not balanced effectively by the PSO algorithm. The position is the one that allows the particle to shift the previous best position and decrease the neighborhood searching ability. A spiral-shaped mechanism is suggested as a local search. To this inspiration, the adaptive position update strategy is applied to produce particle positions based on exploitation and exploration. Thus, the particle position is defined as follows:
α=exp(g(Yej(u)))exp(1O∑Oj=1g(Yej(u))) | (11) |
Yej(s+1)={E1.exp(c.1).cos(2πm)+hbest(u),α<sandYej(u)+Wej(u+1),otherwise | (12) |
where
E1=|hbeste(u)+Wej|. | (13) |
The above equation represents the distance between the particle j and the current best location, the random number m, and the constant s. The article is in a poor position, and a premature convergence is ignored by enhancing the exploration capability.
Competitive substitution mechanism
The PSO performance is enhanced by introducing the competitive substitution mechanism known as CSO-PSO. The substitution in each iteration takes place using the worst particle.
OYej(u)=ArgMax{g(Ye2(u),g(Ye2(u)),.....,g(YeO(u))} | (14) |
The substitution mechanism is defined in the following mathematical expression:
OYej(u)={hbeste(u)+s3.(qbestef(u)−qbestek(u)),f≠g≠j∈[1,2,......O], | (15) |
XYej(u)={OYej(u),g(OYej(u))<g(XYej(u))XYej(u),otherwiase, | (16) |
where OYej(u) represents the new position and s3 is a random number. A condition is for triggering the disturbance strategy; thus, the time wasted on poor conditions is minimized. The mathematical expression of the disturbance strategy is given below:
Obest(u)=s4.hbeste(u)+(1−s4).(hbaete(u)−qbest(u)),j∈[1,2,.....,O] | (17) |
hbeste(u)={Obest(u),g(Obset(u)<g(hbeste(u)))hbeste(u),otherwise | (18) |
where the random parameter is denoted as s4.
Figure 2 describes the working operation of the proposed MCERF-HPS approach developed to accurately identify attacks and regular data traffic to preserve sensitive medical information from invaders.
● The BoT-IoT database consisting of multiple network traffic data is used to evaluate the proposed MCERF-HPS approach.
● The proposed intrusion detection system uses a modified convolutional neural network and ensemble random forest components to learn the traffic features and classify attack traffics from regular traffic separately.
● The convolutional layer at the top of the modified CNN learns each traffic feature while their smaller dimension than the hidden layer induces significant information loss. Therefore, the CCCP layer is embedded with it.
● The deep feature representations obtained from the convolutional and CCCP layers are spliced to form an output vector.
● The fully connected and softmax layers of the modified CNN are replaced with ensemble random forest (ERF) classifiers to gain high classification results. The output vectors generated on the modified CNN are distributed to 'n' decision trees of the random forests to make the final decision.
● Based on the majority voting process, the classification output of each decision tree is concatenated to identify normal and attack traffic. Generally, the detection performance of machine learning and deep learning approaches heavily depends on their parameters. Its random initialization may not constantly provide optimal detection results on every iteration.
● Therefore, it requires an optimal parameter tuning process. In this paper, the modified CNN and ERF parameters are auto-tuned using a hybrid particle swarm (HPS) algorithm.
● By adopting four algorithm enhancement procedures, such as chaotic mapping, adaptive positing updating, elite and dimensional learning, and competitive substituting schemes, the standard PSO algorithm can better solve optimization problems.
● Using these advantages, the HPS algorithm searches and selects the most optimal parameter values for the classifier parameters. Immediately after determining attack traffic in the network by the proposed MCERF-HPS approach, the attacks are blocked in gateway devices within a short time interval, with fewer processing and computing capacities.
● Thus, the proposed MCERF-HPS approach guarantees security to medical records stored in the IoT-based healthcare applications and advances blockchain technology by accurately determining and blocking attack traffic.
The network layer is responsible for protecting the data transmission from the lower layer to the higher layer. The main objective of this network layer is to provide routing management; it is also called a connective layer. In addition, the network layer collects the data via the existing communication network from the perception layer.
The cloud is responsible for accumulating weights from the edge server to the blockchain ledger, accepting the average weight and upgrading the global weight of the proposed algorithm. At regular intervals, the cloud transmits updated weights to each gateway to update the weights of the local models to effectively secure the network. The cloud layer can facilitate the technologies employed in healthcare, including mobile applications, health records, IoT devices, patient portals, extensive data analysis, etc. The cloud layer provides hassle-free flexibility and scalability, enhancing the decision-making processes.
The application layer is responsible for sensing the essential sign of health. The application layer is presented to the user as either a patient or an administrator. Regarding patients, the application layer presents a portal where the pertinent data can be viewed. In the case of the administrator, the application layer can present various other options.
The business layer enables the entire healthcare utility managers to analyze flow charts, business models, and management reports based on data obtained from the lowest layer.
A MCERF-HPS algorithm is presented in this paper for intrusion detection in the healthcare system. The BOT-IoT dataset is utilized to validate the performance, which has 80% of training data and 20% of testing data. This paper is comprised of different parameters: accuracy, precision, recall, specificity, F1-measure, training accuracy, testing accuracy, testing loss, training loss, time cost, execution time, and time complexity for evaluating the performance. The experimental details of this paper are clearly explained in the following upcoming sections.
The proposed MCERF-HPS algorithm's experimental evaluation is conducted using solidity (version 6.0) and Hyper ledger Fabric version 1.1.2. The configuration of the intrusion detection systems depends on the software and hardware requirement of the Blockchain, and the performance is evaluated through the IoT datasets. A predictive analytics model's processing system can be used to predict patient appointment scheduling requests. A test system consists of computer components such as CPU, IDE, operating system, primary programming language, and main memory. Each dataset is divided into 80 and 20% data for training and testing, respectively.
The BoT is the IoT traffic-based dataset that validates the MCERF-HPS method. The development of this dataset is obtained to design the testbed environment in the Cyber Range Lab of UNSW. The Canberra testbed is evaluated in botnet attack traffic, generating 73 million records that determine 46 features in each set. The dataset contains three methods of attacks, namely DoS, information theft, and information gathering. The training and testing datasets extract a lower set (5%) from an entire dataset. The lower dataset is involved in four functions that attained 3 million records, with a size of 1.07 GB.
The hyperparameter configuration of this paper is clearly explained in Table 1. The parameter tuning process is used to determine the optimal parameter values, and these optimal parameters provide the effectiveness of the proposed MCERF-HPS algorithm.
Methods | Hyperparameters | Hyperparameter ranges |
MCNN | Weight decay | 0.0001 |
Number of epochs | 20 | |
Learning rate | 0.003 | |
Batch size | 64 | |
Momentum | 0.93 | |
Dropout ratio | 0.2 | |
HPSO | Number of iterations | 1000 |
Population size | 50 | |
Dimension | 30 | |
Inertia weight | 0.8 | |
d1 | 1.85 | |
d2 | 2 | |
ERF | Number of folds | 3 |
Maximum depth | -1 | |
Batch size | 100 | |
Probability of minimum variance | 0.001 |
The performance of this paper is computed in terms of accuracy (τaccuracy), precision (τprecision), recall (τrecall), specificity (τspecificity), F1-measure (τF1−measure), training accuracy, testing accuracy, testing loss, training loss, time cost, execution time, and time complexity.
τaccuracy=τTP+τTNτTP+τTN+τFP+τFN | (19) |
τrecall=τTPτTP+τFN | (20) |
τprecision=τTPτTP+τFP | (21) |
τspecificity=τTNτTN+τFP | (22) |
τF1−measure=2∗τrecall∗τprecisionτrecall+τprecision | (23) |
The performance rate of the proposed MCERF-HPS algorithm is tabulated in Table 2 to quickly understand the efficiency of this paper because it has objective information.
Performance metrics | Performance ranges |
Accuracy | 98.7% |
Precision | 98.9% |
Recall | 97.5% |
Specificity | 96% |
F1-measure | 98.1% |
Training accuracy | 0.987 |
Testing accuracy | 0.956 |
Training loss | 0.01 |
Testing loss | 0.03 |
Time cost | 3400 seconds |
Execution time | 23 ms |
Time complexity | 16 seconds |
Figure 3(a, b) portrays the training and testing process of the proposed MCERF-HPS algorithm by using accuracy and loss analysis. Figure 3(a) represents the analysis of training and testing accuracy for intrusion detection in the healthcare system. In the accuracy analysis, the training process attained a higher performance rate of 0.987, and the testing process obtained a lower performance rate of 0.956. The training loss and testing loss analysis are performed in Figure 3(b), and the lowest loss rate of 0.01 is predicted from the training process compared to the testing process, which denotes better intrusion detection in the healthcare system.
The proposed MCERF-HPS algorithm is compared with other state-of-the-art methods such as the FL-ANN model, the BCCO-DNN) model, the Deep SDOSVM model and Naive Bayes (NB) for determining superiority. The comparative analysis estimates the time cost, execution time, and time complexity. The performance of each method is tabulated in Table 3, and the proposed MCERF-HPS algorithm has the lowest performance among all those methods. The lowest values are denoted as the best performance in the intrusion detection of the healthcare system. The comparative analysis of accuracy, precision, recall, specificity, and F1-measure is delineated in Figure 4(a−e). The comparative analysis has different methods: the proposed MCERF-HPS algorithm, FL-ANN, BCCO-DNN, Deep SDOSVM, and NB. From this comparative analysis, the proposed MCERF-HPS algorithm provides a better outcome. Figure 4(a) shows the accuracy analysis, and the proposed MCERF-HPS algorithm achieved a maximum accuracy rate of 98.7% compared to other existing methods. Figure 4(b) denotes the precision analysis, and the precision rate of 93.5, 88, 86, 91, and 98.9% are obtained from FL-ANN, BCCO-DNN, Deep SDOSVM, NB, and the proposed MCERF-HPS algorithm, respectively.
Methods | Metrics | ||
Time cost (seconds) | Execution time (ms) | Time complexity (seconds) | |
FL-ANN | 4230 | 42 | 31 |
BCCO-DNN | 3800 | 31 | 25 |
Deep SDOSVM | 4160 | 35 | 27 |
NB | 3600 | 25 | 19 |
Proposed MCERF-HPS algorithm | 3400 | 23 | 16 |
Figure 4(c) denotes the recall evaluation based on the comparative analysis. The proposed MCERF-HPS algorithm provides a better recall rate of 97.5% among all those methods. The specificity analysis is performed according to the comparative analysis depicted in Figure 4(d), and the highest performance rate of 96% is obtained from the proposed MCERF-HPS algorithm. Figure 4(e) shows the F1-measure of approaches such as Deep SDOSVM, FL-ANN, BCCO-DNN, NB, and the proposed MCERF-HPS algorithm. This comparative analysis achieved the F1-measure of 80% from FL-ANN, 84% from BCCO-DNN, 87.5% from Deep SDOSVM, 92.6% from NB, and 98.1% from the proposed MCERF-HPS algorithm.
In this paper, the MCERF-HPS algorithm is proposed for intrusion detection in the healthcare system, which uses the BOT-IoT dataset for performance evaluation. Here, 80% and 20% of data are used for training and testing, respectively. The parameter tuning process is used to determine the optimal parameter values, and these optimal parameters provide the effectiveness of the proposed MCERF-HPS algorithm. This paper is comprised of different parameters, namely accuracy, precision, recall, specificity, F1-measure, training accuracy, testing accuracy, testing loss, training loss, time cost, execution time, and time complexity, to evaluate the performance. The proposed MCERF-HPS algorithm achieved the maximum accuracy rate of 98.7%, precision rate of 98.9%, recall rate of 97.5%, specificity rate of 96%, F1-measure of 98.1%, training accuracy of 0.987, testing accuracy of 0.956, and minimum training loss of 0.01, testing loss of 0.03, the time cost of 3400 seconds, the execution time of 23 ms and time complexity of 16 seconds, respectively. From this comparative analysis, the proposed MCERF-HPS algorithm performed better than other state-of-the-art methods. The performance of the MCERF-HPS algorithm will be improved by minimizing feature space, selecting optimal parameters, and implementing more sophisticated attacks in the future.
The author declares that he has not used any Artificial Intelligence (AI) tools in creating this article.
The author declares there is no conflict of interest.
[1] | A. Omran, M. Abouyoussef, M. Ismail, S. Bhatia, Sharded blockchain-based online diagnostic system for suspected patients during pandemics, in 2022 IEEE Wireless Communications and Networking Conference (WCNC), (2022), 2715−2720. https://doi.org/10.1109/WCNC51071.2022.9771790 |
[2] |
G. N. Nguyen, N. H. Le Viet, M. Elhoseny, K. Shankar, B. B. Gupta, A. A. Abd El-Latif, Secure blockchain enabled cyber–physical systems in healthcare using deep belief networks with the ResNet model, J. Parallel Distrib. Comput., 153 (2021), 150−160. https://doi.org/10.1016/j.jpdc.2021.03.011 doi: 10.1016/j.jpdc.2021.03.011
![]() |
[3] |
A. Rehman, S. Abbas, M. A. Khan, T. M. Ghazal, K. M. Adnan, A. Mosavi, A secure healthcare 5.0 system based on blockchain technology entangled with federated learning technique, Comput. Biol. Med., 150 (2022), 106019. https://doi.org/10.1016/j.compbiomed.2022.106019 doi: 10.1016/j.compbiomed.2022.106019
![]() |
[4] |
A. Verma, V. Ranga, Machine learning-based intrusion detection systems for IoT applications, Wireless Pers. Commun., 111 (2020), 2287−2310. https://doi.org/10.1007/s11277-019-06986-8 doi: 10.1007/s11277-019-06986-8
![]() |
[5] |
A. Kore, S. Patil, IC-MADS: IoT-enabled cross-layer man-in-middle attack detection system for smart healthcare applications, Wireless Pers. Commun., 113 (2020), 727−746. https://doi.org/10.1007/s11277-020-07250-0 doi: 10.1007/s11277-020-07250-0
![]() |
[6] |
P. T. Nguyen, V. D. B. Huynh, K. D. Vo, P. T. Phan, M. Elhoseny, D. N. Le, Deep learning-based optimal multimodal fusion framework for intrusion detection systems for healthcare data, CMC-Comput. Mater. Continua, 66 (2021), 2555−2571. https://doi.org/10.32604/cmc.2021.012941 doi: 10.32604/cmc.2021.012941
![]() |
[7] |
M. Abouyoussef, S. Bhatia, P. Chaudhary, S. Sharma, M. Ismail, Blockchain-enabled online diagnostic platform of suspected Patients of COVID-19 like pandemics, IEEE Internet Things Mag., 4 (2021), 94−99. https://doi.org/10.1109/IOTM.1001.2100046 doi: 10.1109/IOTM.1001.2100046
![]() |
[8] | S. Kumar, B. Bhushan, S. Bhatia, Blockchain-based big data solutions for internet of things (IoT) and smart cities, in New Trends and Applications in Internet of Things (IoT) and Big Data Analytics, Cham: Springer International Publishing, (2022), 225−253. https://doi.org/10.1007/978-3-030-99329-0_15 |
[9] |
S. P. Ramu, P. K. R. Maddikunta, M. Parimala, S. Koppu, T. R. Gadekallu, C. L. Chowdhary, et al., An effective feature engineering for DNN using hybrid PCA-GWO for intrusion detection in IoMT architecture, Comput. Commun., 160 (2020), 139−149. https://doi.org/10.1016/j.comcom.2020.05.048 doi: 10.1016/j.comcom.2020.05.048
![]() |
[10] |
A. Singh, G. Sharma, R. Krishnamurthi, A. Kumar, S. Bhatia, A. Mashat, Cybersecurity for battlefield of things – a comprehensive review, J. Circuits, Syst. Comput., 31 (2020), 2230010. https://doi.org/10.1142/S0218126622300100 doi: 10.1142/S0218126622300100
![]() |
[11] |
M. A. Kumar, D. Samiayya, P. M. Vincent, K. Srinivasan, C. Y. Chang, H. Ganesh, A hybrid framework for intrusion detection in healthcare systems using deep learning, Front. Public Health, 9 (2022), 2295. https://doi.org/10.3389/fpubh.2021.824898 doi: 10.3389/fpubh.2021.824898
![]() |
[12] |
M. Rashid, J. Kamruzzaman, T. Imam, S. Wibowo, S. Gordon, A tree-based stacking ensemble technique with feature selection for network intrusion detection, Appl. Intell., 52 (2022), 1−14. https://doi.org/10.1007/s10489-021-02968-1 doi: 10.1007/s10489-021-02968-1
![]() |
[13] |
A. Abbas, M. A. Khan, S. Latif, M. Ajaz, A. A. Shah, J. Ahmad, A new ensemble-based intrusion detection system for the Internet of things, Arabian J. Sci. Eng., 47 (2022), 1805−1819. https://doi.org/10.1007/s13369-021-06086-5 doi: 10.1007/s13369-021-06086-5
![]() |
[14] |
E. Ashraf, N. F. Areed, H. Salem, E. H. Abdelhay, A. Farouk, Fidchain: Federated intrusion detection system for blockchain-enabled IoT healthcare applications, Healthcare, 10 (2022), 1110. https://doi.org/10.3390/healthcare10061110 doi: 10.3390/healthcare10061110
![]() |
[15] |
M. K. Chandol, M. K. Rao, Border collie cat optimization for intrusion detection system in healthcare IoT network using deep recurrent neural network, Comput. J., 65 (2022), 3181−3198. https://doi.org/10.1093/comjnl/bxab136 doi: 10.1093/comjnl/bxab136
![]() |
[16] |
M. Fouda, R. Ksantini, W. Elmedany, A novel intrusion detection system for internet of healthcare things based on deep subclasses dispersion information, IEEE Internet Things J., 10 (2023), 8395−8407. https://doi.org/10.1109/JIOT.2022.3230694 doi: 10.1109/JIOT.2022.3230694
![]() |
[17] |
S. Saif, P. Das, S. Biswas, M. Khari, V. Shanmuganathan, HIIDS: Hybrid intelligent intrusion detection system empowered with machine learning and metaheuristic algorithms for application in IoT-based healthcare, Microprocess. Microsyst., 2022 (2022), 104622. https://doi.org/10.1016/j.micpro.2022.104622 doi: 10.1016/j.micpro.2022.104622
![]() |
[18] |
S. Saif, K. Karmakar, S. Biswas, S. Neogy, MLIDS: Machine learning enabled intrusion detection system for health monitoring framework using BA-WSN, Int. J. Wireless Inf. Networks, 29 (2022), 491−502. https://doi.org/10.1007/s10776-022-00574-7 doi: 10.1007/s10776-022-00574-7
![]() |
[19] |
A. I. Taloba, A. Elhadad, A. Rayan, R. M. Abd El-Aziz, M. Salem, A. A. Alzahrani, et al., A blockchain-based hybrid platform for multimedia data processing in IoT-healthcare, Alexandria Eng. J., 65 (2023), 263−274. https://doi.org/10.1016/j.aej.2022.09.031 doi: 10.1016/j.aej.2022.09.031
![]() |
[20] |
P. Kanagala, Effective cyber security system to secure optical data based on deep learning approach for healthcare application, Optik, 272 (2023), 170315. https://doi.org/10.1016/j.ijleo.2022.170315 doi: 10.1016/j.ijleo.2022.170315
![]() |
[21] |
Y. Liu, W. Yu, Z. Ai, G. Xu, L. Zhao, Z. Tian, A blockchain-empowered federated learning in healthcare-based cyber physical systems, IEEE Trans. Network Sci. Eng., 2022 (2022). https://doi.org/10.1109/TNSE.2022.3168025 doi: 10.1109/TNSE.2022.3168025
![]() |
[22] | Z. Hu, X. Zhu, Gesture detection from RGB hand image using modified convolutional neural network, in 2019 2nd International Conference on Information Systems and Computer Aided Education (ICISCAE), IEEE, (2019), 143−146. 10.1109/ICISCAE48440.2019.221606 |
[23] |
W. Lin, Z. Wu, L. Lin, A. Wen, J. Li, An ensemble random forest algorithm for insurance big data analysis, IEEE Access, 5 (2017), 16568−16575. https://doi.org/10.1109/ACCESS.2017.2738069 doi: 10.1109/ACCESS.2017.2738069
![]() |
[24] |
W. Xing, Y. Bei, Medical health big data classification based on the KNN classification algorithm, IEEE Access, 8 (2019), 28808−28819. https://doi.org/10.1109/ACCESS.2019.2955754 doi: 10.1109/ACCESS.2019.2955754
![]() |
[25] |
X. Wang, B. Yu, A. Ma, C. Chen, B. Liu, Q. Ma, Protein–protein interaction site prediction by ensemble random forests with synthetic minority oversampling technique, Bioinformatics, 35 (2019), 2395−2402. https://doi.org/10.1093/bioinformatics/bty995 doi: 10.1093/bioinformatics/bty995
![]() |
[26] |
R. Wang, K. Hao, L. Chen, T. Wang, C. Jiang, A novel hybrid particle swarm optimization using adaptive strategy, Inf. Sci., 579 (2021), 231−250. https://doi.org/10.1016/j.ins.2021.07.093 doi: 10.1016/j.ins.2021.07.093
![]() |
[27] |
S. Lalwani, H. Sharma, S. C. Satapathy, K. Deep, J. C. Bansal, A survey on parallel particle swarm optimization algorithms, Arabian J. Sci. Eng., 44 (2019), 2899−2923. https://doi.org/10.1007/s13369-018-03713-6 doi: 10.1007/s13369-018-03713-6
![]() |
Methods | Hyperparameters | Hyperparameter ranges |
MCNN | Weight decay | 0.0001 |
Number of epochs | 20 | |
Learning rate | 0.003 | |
Batch size | 64 | |
Momentum | 0.93 | |
Dropout ratio | 0.2 | |
HPSO | Number of iterations | 1000 |
Population size | 50 | |
Dimension | 30 | |
Inertia weight | 0.8 | |
d1 | 1.85 | |
d2 | 2 | |
ERF | Number of folds | 3 |
Maximum depth | -1 | |
Batch size | 100 | |
Probability of minimum variance | 0.001 |
Performance metrics | Performance ranges |
Accuracy | 98.7% |
Precision | 98.9% |
Recall | 97.5% |
Specificity | 96% |
F1-measure | 98.1% |
Training accuracy | 0.987 |
Testing accuracy | 0.956 |
Training loss | 0.01 |
Testing loss | 0.03 |
Time cost | 3400 seconds |
Execution time | 23 ms |
Time complexity | 16 seconds |
Methods | Metrics | ||
Time cost (seconds) | Execution time (ms) | Time complexity (seconds) | |
FL-ANN | 4230 | 42 | 31 |
BCCO-DNN | 3800 | 31 | 25 |
Deep SDOSVM | 4160 | 35 | 27 |
NB | 3600 | 25 | 19 |
Proposed MCERF-HPS algorithm | 3400 | 23 | 16 |
Methods | Hyperparameters | Hyperparameter ranges |
MCNN | Weight decay | 0.0001 |
Number of epochs | 20 | |
Learning rate | 0.003 | |
Batch size | 64 | |
Momentum | 0.93 | |
Dropout ratio | 0.2 | |
HPSO | Number of iterations | 1000 |
Population size | 50 | |
Dimension | 30 | |
Inertia weight | 0.8 | |
d1 | 1.85 | |
d2 | 2 | |
ERF | Number of folds | 3 |
Maximum depth | -1 | |
Batch size | 100 | |
Probability of minimum variance | 0.001 |
Performance metrics | Performance ranges |
Accuracy | 98.7% |
Precision | 98.9% |
Recall | 97.5% |
Specificity | 96% |
F1-measure | 98.1% |
Training accuracy | 0.987 |
Testing accuracy | 0.956 |
Training loss | 0.01 |
Testing loss | 0.03 |
Time cost | 3400 seconds |
Execution time | 23 ms |
Time complexity | 16 seconds |
Methods | Metrics | ||
Time cost (seconds) | Execution time (ms) | Time complexity (seconds) | |
FL-ANN | 4230 | 42 | 31 |
BCCO-DNN | 3800 | 31 | 25 |
Deep SDOSVM | 4160 | 35 | 27 |
NB | 3600 | 25 | 19 |
Proposed MCERF-HPS algorithm | 3400 | 23 | 16 |