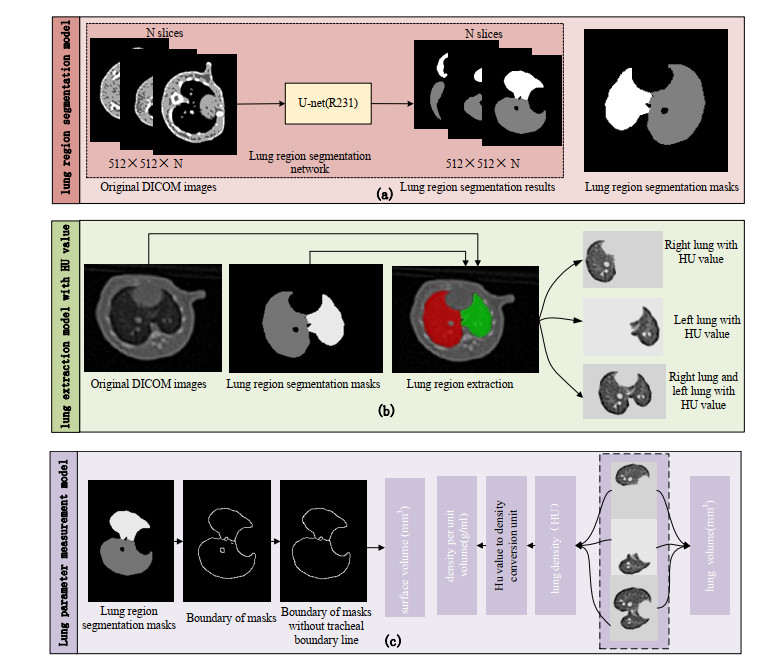
Our paper proposes a method to measure lung parenchyma parameters from pulmonary window computed tomography images based on ResU-Net model including the CT value, the density, the lung volume, and the surface area of the lungs of healthy rats, to help promote the quantitative analysis of lung parenchyma parameters of rats in medical respiratory researches. Through the analysis of the lung parenchyma parameters of the control group and the treatment group, the law of change among the lung parenchyma parameters is given in our paper. After comparing and analyzing the lung parenchyma parameter CT value and the density of the two groups, it is discovered that the lung parenchyma parameter CT value and the density significantly increase in the treatment group which is after continuously inhaling the nebulization of contrast agents. The change of the lung volume with the surface area in both two groups conforms to the law of lung changes during breathing. The relationship between the lung volume and the CT value or the density is analyzed and it is concluded that the lung volume is negatively correlated with the CT value or the density.
Citation: Yingjian Yang, Qiang Li, Yingwei Guo, Yang Liu, Xian Li, Jiaqi Guo, Wei Li, Lei Cheng, Huai Chen, Yan Kang. Lung parenchyma parameters measure of rats from pulmonary window computed tomography images based on ResU-Net model for medical respiratory researches[J]. Mathematical Biosciences and Engineering, 2021, 18(4): 4193-4211. doi: 10.3934/mbe.2021210
[1] | Yingjian Yang, Wei Li, Yan Kang, Yingwei Guo, Kai Yang, Qiang Li, Yang Liu, Chaoran Yang, Rongchang Chen, Huai Chen, Xian Li, Lei Cheng . A novel lung radiomics feature for characterizing resting heart rate and COPD stage evolution based on radiomics feature combination strategy. Mathematical Biosciences and Engineering, 2022, 19(4): 4145-4165. doi: 10.3934/mbe.2022191 |
[2] | Yingjian Yang, Wei Li, Yingwei Guo, Nanrong Zeng, Shicong Wang, Ziran Chen, Yang Liu, Huai Chen, Wenxin Duan, Xian Li, Wei Zhao, Rongchang Chen, Yan Kang . Lung radiomics features for characterizing and classifying COPD stage based on feature combination strategy and multi-layer perceptron classifier. Mathematical Biosciences and Engineering, 2022, 19(8): 7826-7855. doi: 10.3934/mbe.2022366 |
[3] | Tong Shan, Jiayong Yan, Xiaoyao Cui, Lijian Xie . DSCA-Net: A depthwise separable convolutional neural network with attention mechanism for medical image segmentation. Mathematical Biosciences and Engineering, 2023, 20(1): 365-382. doi: 10.3934/mbe.2023017 |
[4] | Michael James Horry, Subrata Chakraborty, Biswajeet Pradhan, Maryam Fallahpoor, Hossein Chegeni, Manoranjan Paul . Factors determining generalization in deep learning models for scoring COVID-CT images. Mathematical Biosciences and Engineering, 2021, 18(6): 9264-9293. doi: 10.3934/mbe.2021456 |
[5] | Pengcheng Zhang, Yi Liu, Zhiguo Gui, Yang Chen, Lina Jia . A region-adaptive non-local denoising algorithm for low-dose computed tomography images. Mathematical Biosciences and Engineering, 2023, 20(2): 2831-2846. doi: 10.3934/mbe.2023133 |
[6] | Vasileios E. Papageorgiou, Georgios Petmezas, Pantelis Dogoulis, Maxime Cordy, Nicos Maglaveras . Uncertainty CNNs: A path to enhanced medical image classification performance. Mathematical Biosciences and Engineering, 2025, 22(3): 528-553. doi: 10.3934/mbe.2025020 |
[7] | Huiying Li, Yizhuang Song . Sparse-view X-ray CT based on a box-constrained nonlinear weighted anisotropic TV regularization. Mathematical Biosciences and Engineering, 2024, 21(4): 5047-5067. doi: 10.3934/mbe.2024223 |
[8] | Zhenwu Xiang, Qi Mao, Jintao Wang, Yi Tian, Yan Zhang, Wenfeng Wang . Dmbg-Net: Dilated multiresidual boundary guidance network for COVID-19 infection segmentation. Mathematical Biosciences and Engineering, 2023, 20(11): 20135-20154. doi: 10.3934/mbe.2023892 |
[9] | Jun Gao, Qian Jiang, Bo Zhou, Daozheng Chen . Convolutional neural networks for computer-aided detection or diagnosis in medical image analysis: An overview. Mathematical Biosciences and Engineering, 2019, 16(6): 6536-6561. doi: 10.3934/mbe.2019326 |
[10] | Binju Saju, Neethu Tressa, Rajesh Kumar Dhanaraj, Sumegh Tharewal, Jincy Chundamannil Mathew, Danilo Pelusi . Effective multi-class lungdisease classification using the hybridfeature engineering mechanism. Mathematical Biosciences and Engineering, 2023, 20(11): 20245-20273. doi: 10.3934/mbe.2023896 |
Our paper proposes a method to measure lung parenchyma parameters from pulmonary window computed tomography images based on ResU-Net model including the CT value, the density, the lung volume, and the surface area of the lungs of healthy rats, to help promote the quantitative analysis of lung parenchyma parameters of rats in medical respiratory researches. Through the analysis of the lung parenchyma parameters of the control group and the treatment group, the law of change among the lung parenchyma parameters is given in our paper. After comparing and analyzing the lung parenchyma parameter CT value and the density of the two groups, it is discovered that the lung parenchyma parameter CT value and the density significantly increase in the treatment group which is after continuously inhaling the nebulization of contrast agents. The change of the lung volume with the surface area in both two groups conforms to the law of lung changes during breathing. The relationship between the lung volume and the CT value or the density is analyzed and it is concluded that the lung volume is negatively correlated with the CT value or the density.
Rats are commonly used as experimental objects to study various mechanisms in the current medical research, such as the field of medicine [1,2,3,4,5]. To date, lots of biomedical laboratory animals are rats, and they play a vital role in the experiments of newly developed medical preparations [2,6,7,8]. The main reason is that their genetic composition and biological behavior characteristics are extremely similar to humans, and about 80% of them are genetically identical to humans. Moreover, rats modified by genetically modified technology [9,10] can even carry genes that cause illness in humans.
Due to the outbreak of the new coronavirus at the end of 2019 (COVID-19) [11,12,13], many researchers in the medical field are urgently concerned about respiratory diseases research. As a very important branch of respiratory research, chest images [14,15,16,17] obtained through imaging equipment can clearly show abnormal and normal lung lesions. Therefore, clinicians can diagnose most lung diseases through chest images. For most respiratory diseases, such as lung infections including COVID-19 [12,13,17,18], bronchiectasis [19,20], chronic obstructive pulmonary diseases (COPD) [21,22], or idiopathic pulmonary fibrosis [23] etc., the computed tomography (CT) has unique advantages to achieve the purpose of diagnosis. Especially for a patient with COVID-19, although the nucleic acid test [24,25,26] is negative, as long as there is a lesion on the CT image, infection of COVID-19 can be suspected and treated as this disease.
Many researchers currently use rats for respiratory research [27,28,29], but few researchers can effectively measure lung parenchyma parameters of rats. The quantitative analysis of the rat lungs is largely limited. Currently, the gold standard for determining lung function in human is routine pulmonary function testing (PFT) [30,31]. The researches [32,33] have shown that the lung volume, density, pixel index, and other parameters of lung images acquired by CT technology have a good correlation with the pulmonary function data acquired by the PFT. Therefore, the corresponding lung parenchyma parameters from the lung images of rats may be useful for respiratory researches.
At present, most researchers are committed to using artificial intelligence methods to diagnose lung diseases from CT images [14,18,34,35,36]. However, after the diagnosis of the disease, corresponding medical measures need to be taken to treat the disease. For a newly developed drug, it is first necessary to conduct drug experiments on animals to observe the response of diseased animals to the drug. For respiratory diseases, inhalation therapy [37,38,39] is often a very effective solution. Therefore, rats are bred into models with corresponding diseases and use the models to experiment with newly developed inhaled pharmaceutical preparations. The easiest and most feasible way is to image rats through CT and measure the changes in lung parenchyma parameters from the images to understand the actual effects of the drugs.
Our paper proposes a method for measuring rats' lung parenchyma parameters of the right lung (RL), the left lung (LL), and the bilateral lung (BL) including the CT value, the density, the lung volume (LV), and the surface area (SA). After getting the CT value, the density of the BL, LL, and RL, the average CT value (ACT) and the average density (AD) which can reflect the situation of the BL, LL, and RL can be calculated.
The above lung parenchyma parameters all have their important clinical significance [40,41,42]. If the density in local regions is higher than that in other regions of lung tissue, and the ACT in local regions will be correspondingly higher than that in other regions. In clinical diagnosis, an increase or decrease in the ACT of lung tissue will indicate lung tissue disease. An increase in the ACT of lung tissue is generally seen in the lung parenchyma inflammation, masses, nodules, etc., and a decrease in the ACT is more common in emphysema and pneumothorax. If the AD indicating the amount of lung tissue per unit volume is abnormal, there may be some changes such as lung tissue proliferation and remodeling, which may indicate different degrees of disease in the clinic. The LV refers to the volume occupied by the lung tissue in the thoracic cavity. Clinically, the increase in the LV is commonly seen in pathologies such as emphysema and masses, and the decrease in the LV can be seen in pathologies such as lung consolidation and fibrosis. The SA often easily be overlooked is an important parameter for researching the increase or the decrease of lung tissue per unit area due to emphysema.
In this paper, the left lung and right lung of rats are segmented using a convolutional neural network segmentation model, and then the BL, LL, and RL of rats are extracted, and finally lung parenchyma parameters of the BL, LL, and RL can be measured. Based on the available original images, our purpose is not only to show the differences in the same lung parenchymal parameter of the two groups of rats, but to provide a method for measuring the lung parenchymal parameters of rats to promote current medical respiratory researches. To verify the correctness of the method proposed in our paper, the ACT and the AD are calculated and the relationship between the LV and the SA is analyzed. The results show that they are in line with the law of changes in the lung parenchyma. At the same time, it is concluded that the lung volume is negatively correlated with the CT value or the density.
The dataset of rats' pulmonary window images with window level -600 and window width 1200 used in our study is from the department of radiology in the first affiliated hospital of Guangzhou medical university. Original images are 14 sets of datasets in DICOM format obtained by CT scanning for patients. The parameter of the CT made by SIEMENS is set to 80 KV, 50 mAs, Pith: 0.5, and FOV: 100, respectively.
Seven healthy SPF SD rats are named the control group before inhaling the nebulization. After continuously inhaling the nebulization, these seven rats were then named the treatment group. Weight of the seven rats is in the range of 90-100 g. During the 12 hours before the inhalation, the seven rats were fasted and could only drink water. A kind of X-ray contrast agent named the iodixanol injection or the Visipaque was used as the nebulized medicine. Basic information of the dataset is that the size of each pulmonary window images is 512 × 512 × N and the voxel spacing is 0.1953 * 0.1953 * 1 (mm). N represents the number of slices of each pulmonary window images, and its range is 37 to 52 in our dataset.
To measure the lung parenchyma parameters of rats, the lung region of rats is segmented to obtain the lung segmentation masks, and then the BL, LL, and RL of rats with Hounsfield unit (HU) values are extracted. Finally, the lung parenchyma parameters of the BL, LL, and RL can measured or calculated, respectively. Figure 1 shows the overall implementation plan of our article to measure the lung parenchyma parameters of rats including a lung region segmentation model, a lung extraction model, and a lung parameter measurement model.
As shown in Figure 1(a), the lung region segmentation model is used to segment the lung region of rats to obtain the lung segmentation masks. Specifically, the original DICOM images are input to the lung region segmentation network named U-net(R231) [43], and the U-net (R231) outputs the lung segmentation masks of the LL and RL. A detailed description of the lung region segmentation model for rats is shown in Section 2.2.1.
After getting the lung segmentation masks in Figure 1(a), the lung parenchyma extraction model in Figure 1(b) is used to extract the lung parenchyma of the BL, LL, and RL with HU values. A detailed description of the lung parenchyma extraction model with HU value is shown in Section 2.2.2.
After obtaining the lung parenchyma through the lung extraction model in Figure 1(b), the lung parameter measurement model is used to measure the CT value and calculate the LV. Then, the HU value to density conversion unit is used to convert the CT value to the density. Before calculating the SA, the tracheal boundary line needs to be removed from the boundary of the lung segmentation masks in Figure 1(c). A detailed description of the parameter measurement model for rats is shown Section 2.2.3.
Since all automatic segmentation methods cannot achieve 100% accuracy, a semi-automatic segmentation method is used in our paper. In the lung region segmentation model, the deep learning network model is used for the coarse segmentation to obtain the mask of the LL and the mask of the RL. To ensure the accuracy of the segmentation, the fine segmentation is manually performed on the results of the coarse segmentation.
1) Coarse segmentation
There is not enough information of the pulmonary window images with 36 to 52 slices, so it is difficult to use traditional methods [44,45,46] to segment the BL, LL, and RL. Until the network model named U-net (R231) [43] is discovered, we try to input the original DICOM images of rats into the U-net (R231) which had trained by the human lung and the segmentation results is beyond our expectations. The U-net (R231) is actually a U network model with residual building block (ResU-Net) and the architecture of ResU-Net model is shown in Figure 2.
Figure 2(a) shows the architecture of U-Net model. The input of the ResU-Net model is the original DICOM images with the size 512 × 512 × N, and the output of the ResU-Net model is the lung segmentation masks of the LL and RL with the size 512 × 512 × N. The U-Net model includes a contracting path (red dotted box) and an expansive path (bule dotted box). The contracting path includes 5 sub-encoder modules named UNetConvBlock (0) to UNetConvBlock (4). Each sub-decoder module followed by an average-pooling operation (red arrow) is as down-sampling modules, and 5 sub-encoder modules are connected in sequence on the left of Figure 2(a). Similarly, the expansive path includes 5 sub-decoder modules named UNetUpBlock (0) to UNetUpBlock (4). Each sub-decoder modules copies the feature map generated by the corresponding layer from sub-encoder modules in the contracting path and connects it with the feature map generated from up-sample operation (green arrow) on the right of Figure 2(b). The output of the ResU-Net model as segmentation result is generated by a 1 × 1 convolution with Softmax.
Figure 2(a), (b) shows the detailed architecture of the contracting path (red dotted box) and the expansive path (bule dotted box) in the U-Net model, respectively. All sub-encoders are residual building blocks with two feedforward branches in the contracting path. The outputs of the two feedforward branches are added together by a shortcut connection as an output of each sub-encoder. The original DICOM images as the input of UNetUpBlock (0) is decoded by a residual building block. A feedforward branch of the UNetUpBlock (0) includes a 3 × 3 convolution, sequentially followed by a rectified linear unit (ReLU), a batch normalization (BN) and a 3 × 3 convolution. The other feedforward branch of the UNetUpBlock (0) includes a 1 × 1 convolution followed by a BN. The UNetConvBlock (1) to UNetConvBlock (4) continue to decode the original DICOM images with the same residual building blocks as shown in Figure 2(b). The residual building blocks of the UNetConvBlock (1) to UNetConvBlock (4) also have two feedforward branches. A feedforward branch of the UNetConvBlock (1) to UNetConvBlock (4) includes a ReLU sequentially followed by a BN, a 3 × 3 convolution, a ReLU, a BN, and a 3 × 3 convolution. The architecture of the other feedforward branch of the UNetConvBlock (1) to UNetConvBlock (4) is the same as that of the UNetUpBlock (0).
Similarly, the UNetUpBlock (0) to UNetUpBlock (4) are the same residual building blocks as shown in Figure 2(c). There are a residual building sub-block (1) and a residual building sub-block (2) in each UNetUpBlock (i), and the residual building sub-block (1) sequentially followed by a 1 × 1 convolution and a BN as a feedforward branch of the residual building sub-block(2). The input of the residual building sub-block (1) is directly as the output the other feedforward branch of the residual building sub-block (1), and the outputs generated from two feedforward branches of the residual building sub-block (2) are added together by a shortcut connection as an output of each UNetUpBlock (i). Specifically, there are two feedforward branches in the residual building sub-block (1). A feedforward branch of the residual building sub-block (1) includes a 3 × 3 convolution, sequentially followed by a ReLU, a BN, a 3 × 3 convolution, a ReLU, and a BN. The other feedforward branch of the residual building sub-block (1) includes a 1 × 1 convolution followed by a BN. A output of the residual building sub-block (1) is generated by an addition of the two feedforward branches in the residual building sub-block (1).
Experienced radiologists can compare the original DICOM images with the segmentation masks of the corresponding slice to judge whether the segmentation result is correct. If two experienced radiologists out of three believe that a segmentation result is acceptable, then the segmentation result can be considered acceptable. Figure 3 shows the typical segmentation results of a set in the dataset. all slices except the 14th slice of the segmentation results is acceptable judged by experienced radiologists, and only the 14th slice of the segmentation results needs to be manually corrected by experienced radiologists.
2) Fine segmentation
To compensate for the segmentation errors of the lung region segmentation model in certain slices, a tool named ITK-SNAP is used to manually correct those incorrect segmentation results. The software named ITK-SNAP can be available on the website, http://www.itksnap.org/pmwiki/pmwiki.php?n=Downloads.SNAP3.
Figures 4(a1)-6(c1) show two segmentation errors with blue circles and blue rectangular frames from the view of the coronal plane, the sagittal plane, and the axial plane with window setting value 3144 and level setting value 533. Radiologists use the ITK-SNAP to fill in or modify the segmentation errors to get accurate segmentation results for this slice. Specifically, after selecting the Paintbrush Mode the Paintbrush Inspector panel can be seen in the ITK-SNAP Toolbox to fill in or modify the segmentation errors. Due to the structural features of the lung, when modifying or filling in the lung boundary of the lung segmentation masks, we recommend choosing the third type of Brush Styles like the quarter moon and using a small Brush Size in the Paintbrush Inspector panel. In the Paintbrush Inspector panel, the Adaptive Algorithm is the default value with Granularity 20 and Smoothness 15.
After filling or modifying the segmentation errors, the mask of the LL and the mask of the RL is exported as the NIFTI format for each slice shown in Figures 4(a2)-6(c2). If the segmentation errors are modified in Figure 4(a1), the corresponding masks in Figure 4(b1), (c1) can be also automatically modified.
After the fine segmentation, the lung parenchyma extraction model is used to extract the lung parenchyma of the BL, the BL, LL, and RL with HU values. In the lung parenchyma extraction model, the original DICOM images multiply by the corresponding slice of the lung segmentation masks of the BL, LL, and RL to get the lung parenchyma with HU values. For details, please refer to our previous paper [47]. Note that when the original DICOM images multiply by the lung segmentation masks, make sure that each slice of the original DICOM images and the corresponding slice of the lung segmentation masks are on the same slice.
Figure 5 shows the process of typical lung parenchyma extraction of the BL, LL, and RL with HU values. Figure 5(a1)-(a3) show the original DICOM images from three perspectives respectively. Figure 5(b1)-(b3) show the three images of lung segmentation masks from the three perspectives respectively, and Figure 5(c1)-(c3) show the lung parenchyma extraction results of the lung extraction model.
Now that the lung parenchyma with HU values has been extracted, the lung parenchyma parameters of the BL, LL, and RL can be measured, respectively. the HU value corresponding to each pixel in the lung parenchyma can easily got, and then the average HU values to get the ACT are calculated. Before calculating the average HU values, because HU values in the lung parenchyma cannot be greater than or equal to 0 HU, these HU values have been deleted.
When calculating the density, the following formula named the HU value to density conversion is used as mentioned in Figure 1(c) to convert HU values to density values.
The conversion formula:
where mg/mL; HHU__air = -1000 HU.
The parameter HHU__lung is the HU values corresponding to each pixel less than 0 HU. Through the above conversion formula, the density of the BL, LL, and RL can be got, respectively. The unit of density values obtained from the conversion formula is mg/mL, then converted to g/mL.
The LV can be obtained by counting the total number of pixels less than 0 HU and the volume of each pixel. Specifically, the total number of pixels of the BL, LL, and RL is multiplied by the corresponding pixel volume to get the LV of the BL, LL, and RL. The pixel volume is also known as the voxel spacing and can be obtained through the header file of the DICOM file. In our paper, the value of the pixel volume is 0.03814 mm3 (0.1953 mm × 0.1953 mm × 1 mm).
When measuring the SA of the BL, LL, and RL, only the boundary pixels of them are needed by the edge detection method with the first-order Canny operator. The SA of the BL, LL, and RL can be obtained by multiplying the total number of boundary pixels by the corresponding pixel volume. The result of the BL, LL, and RL after using the edge detection method is shown in Figure 6, and there are trachea edges seen in the red circle of Figure 6 (a1)-(c1) which will impact on our calculation result of the SA. To eliminate the impact, a connected domain strategy is adopted. The connected domain strategy can delete trachea edges whose area is smaller than the value P, using 8 neighborhoods. In our paper, the value P can be configured as 50. After using the connected domain strategy, the boundary of the BL, LL, and RL is without the tracheal boundary line shown in Figure 6(a2)-(c2). In our paper, we actually detect the lung region segmentation masks obtained from the lung region segmentation model and get the boundary of masks with the tracheal boundary line. Of course, the boundary of the BL, LL, and RL is multi-slice boundaries. When calculating the SA, the total number of the boundary pixels of all slices together can be counted, and then multiply the total number by the pixel volume with 0.03814 mm3.
In our paper, the lung parenchyma parameter of the BL, the LL and RL from the pulmonary window images of 7 healthy rats before and after inhaling the nebulization is measured, and mainly compare the CT value and the density before and after inhaling the nebulization and analyze the relationship of the lung parenchyma parameter. Healthy rats before inhaling the nebulization are named the control group, and the rats after inhaling the nebulization are named the treatment group. The following analysis of the lung parenchyma parameter is carried out by statistical software named GraphPadPrism (8.0.1).
To verify the method proposed in our paper, the changes of the CT value and the density of the BL, LL, and RL from the two groups are compared by observing whether the changes conform to the law of the inhaling the nebulization.
The ACT of the LL and RL from the control group in Figure 6(a1) are -578.3 and -560.3 HU, and that of the LL and RL from the treatment group in Figure 6(a2) are -526.3 and -518.8 HU. Difference between mean (DM) ± standard error of mean (SEM) between the ACT of the LL and the ACT of the RL from the control group is about 17.96 ± 0.50, and that from the treatment group is about 7.52 ± 0.48. Figures 6(a1) and 7(a2) show that the ACT of the LL and RL from their own group are no obvious difference. However, Figure 7(b1-c) clearly show that the ACT of the BL, LL, and RL from the treatment group are significantly greater than that from the control group, respectively. The significant ACT difference between the control group and the treatment group is determined by both t tests (P < 0.0001) and nonparametric tests (P < 0.0001). Specifically, the ACT of the LL from the two groups in Figure 6(b1) are -578.3 and -526.3HU, and the ACT of the RL from the two groups in Figure 6(b2) are -560.3 and -518.8 HU, and the ACT of the BL from the two groups in Figure 6(c) are -567.9 and -521.9 HU. DM ± SEM of the BL, LL, and RL from the two groups is about 51.98 ± 0.52, 41.54 ± 0.45, and 46.04 ± 0.34, respectively. Above all, it is found that the ACT in the treated group have significantly increased.
After analyzing the CT value, we continue to analyze the changes in the density in the BL, LL, and RL under the conditions of the control group and the treatment group. The density values can be calculated by the conversion formula. The relationship between density values and HU value is essentially a linear relationship. The AD of the RL and the LL from the control group is about 0.4404 and 0.4224 g/mL, and that from the treatment group is about 0.4818 and 0.4743 g/mL. DM ± SEM of the RL and the LL from the control group is about 0.0179 ± 0.0005, and that of the RL and the LL from the treatment group is about 0.0075 ± 0.0005. The AD difference of the RL and the LL from their own group is very small. However, the AD of the RL, the LL and the BL in the treatment group is significantly greater than that in the control group. The significant AD difference between the control group and the treatment group is determined by both t tests (P < 0.0001) and nonparametric tests (P < 0.0001). Specifically, the AD of the LL from the two groups are 0.4224 and 0.4743 g/mL, and that of the RL from the two groups are 0.4404 and 0.4818 g/mL, and that of the BL from the two groups in Figure are 0.4327 and 0.4787 g/mL. DM ± SEM of the BL, LL, and RL from the two groups is about 0.0519 ± 0.0005, 0.0414 ± 0.0005, and 0.0460 ± 0.0003, respectively. Above all, it is also found that the AD in the treatment group have significantly increased.
To further verify the accuracy of the method proposed in our paper, the relationship between the LV and the SA is tested.
Figure 8 shows that the relationship between the LV and the SA of the BL, LL, and RL in the control group and the treatment group, respectively. When the SA increases, the LV will increase with the SA, which is consistent with the law of lung changes during breathing. Although the regression relationship changes slowly in the treatment group as shown in Figure 8(b1)-(b3), it can also reflect the positive proportional relationship between the LV and the SA. But the value of both the LV and the SA in the treatment group is significantly less than that in the control group. Specifically, Figure 8 shows that the value of both the LV and the SA in the LL is less than that in the RL.
After verifying the accuracy of the method proposed in our paper, the relationship between the LV and the CT value or the density is analyzed. We make the regression analysis on the CT value and the LV of the BL, LL, and RL in the control group and the treatment group, respectively. The regression equations which show that the CT value and the LV are negatively correlated.
Figure 9 shows that the LV of the BL, LL, and RL decreases as the CT value increases in both the control group and the treatment group. From the overall distribution of CT value in Figure 9, most CT value of the BL, LL, and RL in the treatment group is smaller than that in the control group, and the corresponding LV in the treatment group reduces. As the previous conversion formula, CT value and the density are linear. Similarly, the density and the LV of the BL, LL, and RL are also negatively correlated in both two groups.
The changes in the lung parenchyma parameters often reflect the development of the disease in medical respiratory researches. We propose a method to measure the CT value, the density, the LV, and the SA of the lung parenchyma parameters in our paper. Although the lung region segmentation model is a semi-automatic segmentation model, it has greatly reduced the workload of lung segmentation compared to the manual segmentation, and the semi-automatic segmentation can ensure the accuracy of lung parameters measurement. The dataset of seven healthy SPF SD rats obtained from CT for lung parenchyma segmentation in our paper, and we believe that if the dataset obtained from the micro-computed tomography for small animals has a higher resolution, there will be better results of the lung parenchyma segmentation. Using the lung region segmentation model and lung parenchyma extraction model, the lung parenchyma of the LL and RL is obtained, so quantitative analysis on lung parenchyma parameters of the LL and RL can be performed.
To verify the effectiveness of the method proposed in our paper, the ATC and the AD of the BL, LL, and RL under the conditions of the control group and the treatment group are analyzed, respectively. Theoretically, due to the continuous inhalation of the nebulization, the absorption of X-rays by the lung will increase and the CT value and the density of the treatment group would correspondingly increase. The ATC and the AD have significantly increased in the treatment group, which is consistent with the theoretical changes of the CT value and/or the density. the relationship between the LV and the SA of the BL, LL, and RL in the control group and the treatment group is also analyzed, and it is concluded that they are the positive proportional relationship. Because when the SA increases, the lung including the BL, LL, and RL is in a process of inhalation, and the LV will inevitably increase. Similarly, when the SA decreases, the lung is in a process of exhalation, and the LV will inevitably decrease. The change of the LV with the SA in both two groups conforms to the law of lung changes during breathing which means that the LV and the SA are positively related. We can see from the original DICOM images and Figure 8 that the LV of the LL from rats is also smaller than that of the RL which also conforms to morphological characteristics of the lung.
The relationship between the LV and the CT value or the density of the BL, LL, and RL is also preliminarily analyzed, and then it is concluded that the LV is negatively correlated with the CT value or the density. Due to the inhalation of the nebulization, the breathing movement of the lungs is restricted, so the LV of the BL, LL, and RL is significantly reduced. We think that if the relationship between lung parenchyma parameters can be clearly explained, the amount of dataset in the two groups need to be increased. At the same time, if the breathing process of rats can be controlled, there will be other important discoveries using the method proposed in our paper. Finally, what we have done above is to help promote the quantitative analysis of lung parenchyma parameters of rats in medical respiratory researches.
We propose an effective method to measure the lung parenchyma parameters of rats from pulmonary window computed tomography images based on ResU-Net model. The above results show that the CT value, the density, the LV, and the SA of the lung parenchyma parameters conform to the law changes of rats' lung. After comparing and analyzing the lung parenchyma parameter CT value and the density of the two groups, it is discovered that the lung parenchyma parameter CT value and the density significantly increase in the treatment group. The relationship between the lung volume and the CT value or the density is also analyzed and get the conclusion that the LV is negatively correlated with the CT value and/or the density.
Thanks to the Department of Radiology, the First Affiliated Hospital of Guangzhou Medical University for providing the dataset of rats' pulmonary window images and the funding support from the Natural Science Foundation of Guangdong Province, China (2019A1515011382) and the National Natural Science Foundation of China (62071311).
The authors declare no conflict of interest in this paper.
[1] |
J. Morita, H. Yamashita, K. Sugihara, M. Wakamatsu, M. Sasaki, Spontaneous ocular abnormalities in sprague-dawley rats, Comp. Med., 70 (2020), 140-144. doi: 10.30802/AALAS-CM-19-000076
![]() |
[2] | J. H. Hwang, H. W. Jung, S. Y. Kang, A. N. Kang, J. N. Ma, X. L. Meng, et al., Therapeutic effects of acupuncture with MOK, a polyherbal medicine, on PTU-induced hypothyroidism in rats, Exp. Ther. Med., 16 (2018), 310-320. |
[3] | J. F. Zhang, J. Liu, G. H. Gong, B. Zhang, C.X. Wei, Mongolian medicine RuXian-I treatment of estrogen-induced mammary gland hyperplasia in rats related to TCTP regulating apoptosis, Evidence-Based Complementary Altern. Med., 11 (2019), 1-10. |
[4] |
Z. Wei, C. Xu, S. Liu, F. Song, Z. Liu, X. Qu, Metabonomics study of the effects of traditional Chinese medicine formula Ermiaowan on hyperuricemic rats, J. Sep. Sci., 41 (2018), 560-570. doi: 10.1002/jssc.201700985
![]() |
[5] | T. W. Shen, T. J. Liu, D. Uta, C. C. Lee, Acoustic perturbation of breathing: A newly discovered response to soft sounds in rats using an approach of image analysis, J. Med. Biol. Eng, 39 (2019), 43-53. |
[6] | A. Morita, M. Ouchi, M. Terada, H. Kon, S. Kishimoto, K. Satoh, et al., Reproducible insulin secretion from isolated rat pancreas preparations using an organ bath, Exp. Anim., 67 (2017), 15-22. |
[7] | J. d. M. Silveira, D. S. Rocha, G. de Morais, R. de Siqueira, J. Leal-Cardoso, J. Evangelista, Inhibitory effect of linalool in preparations of isolated smooth muscle of rat trachea with epithelium stimulated by electromechanical coupling, Ciência Anim., 27 (2017), 20-30. |
[8] | C. Rohleder, F. Pahlisch, R. Graf, H. Endepols, F. M. Leweke, Different pharmaceutical preparations of 33 Δ9-tetrahydrocannabinol differentially affect its behavioral effects in rats, Addict. Biol., 25 (2020), 1-10. |
[9] | R. Vega, C. Padilla, M. Trujillo, N. Quirk, E. Ferreira, Contribution of implanted, genetically modified muscle progenitor cells expressing BMP-2 to new bone formation in a rat osseous defect, Mol. Ther., 26 (2018), 208-218. |
[10] |
X. Ren, D. A. Butterfield, Fidelity of the PINK1 knockout rat to oxidative stress and other characteristics of Parkinson disease, Free Radical Biol. Med., 163 (2021), 88-101. doi: 10.1016/j.freeradbiomed.2020.12.004
![]() |
[11] |
I. D. Apostolopoulos, S. I. Aznaouridis, M. A. Tzani, Extracting possibly representative COVID-19 biomarkers from X-ray images with deep learning approach and image data related to pulmonary diseases, J. Med. Biol. Eng, 40 (2020), 462-469. doi: 10.1007/s40846-020-00529-4
![]() |
[12] | H. Panwar, P. K. Gupta, M. K. Siddiqui, R. Morales-Menendez, V. Singh, Application of deep learning for fast detection of covid-19 in x-rays using nCOVnet, Chaos, Solitons Fractals, 138 (2020), 1-8. |
[13] | A. Pan, L. Liu, C. Wang, H. Guo, X. Hao, Q. Wang, et al., Association of public health interventions with the epidemiology of the COVID-19 outbreak inWuhan, China, JAMA, 323 (2020), 1915-1923. |
[14] | L. Wang, Z. Q. Lin, A. Wong, Covid-net: A tailored deep convolutional neural network design for detection of covid-19 cases from chest x-ray images, Sci. Rep., 10 (2020), 1-12. |
[15] |
Y. Li, L. Xia, Coronavirus disease 2019 (COVID-19): role of chest CT in diagnosis and management, Am. J. Roentgenol., 214 (2020), 1-7. doi: 10.2214/AJR.19.22415
![]() |
[16] | K. Doi, M. L. Giger, R. M. Nishikawa, K. R. Hoffmann, H. MacMahon, R. A. Schmidt, et al., Digital radiography: A useful clinical tool for computer-aided diagnosis by quantitative analysis of radiographic images, Acta Radiol., 34 (1993), 426-439. |
[17] | K. Li, J. Wu, F. Wu, D. Guo, L. Chen, Z. Fang, et al., The clinical and chest CT features associated with severe and critical COVID-19 pneumonia, Invest. Radiol., 55 (2020), 327-331. |
[18] |
C. Bao, X. Liu, Z. B. Han, Y. Li, J. Liu, Coronavirus disease 2019 (COVID-19) CT findings: A systematic review and meta-analysis, J. Am. Coll. Radiol., 17 (2020), 701-709. doi: 10.1016/j.jacr.2020.03.006
![]() |
[19] | J. C. Cooke, D. C. Currie, A. D. Morgan, I. H. Kerr, D. Delany, B. Strickland, et al., Role of computed tomography in diagnosis of bronchiectasis, Thorax, 42 (1987), 272-277. |
[20] | J. J. Meerburg, G. D. M. Veerman, S. Aliberti, H. Tiddens, Diagnosis and quantification of bronchiectasis using computed tomography or magnetic resonance imaging: A systematic review, Respir. Med., 170 (2020), 1-8. |
[21] |
O. M. Mets, P. Jong, B. V. Ginneken, H. A. Gietema, J. Lammers, Quantitative computed tomography in COPD: possibilities and limitations, Lung, 190 (2012), 133-145. doi: 10.1007/s00408-011-9353-9
![]() |
[22] | N. L. Ford, I. Lee, A. Tam, D. D. Sin, Micro-computed tomography imaging of a rodent model of chronic obstructive pulmonary disease (copd), in Medical Imaging 2020: Biomedical Applications in Molecular, Structural, and Functional Imaging. International Society for Optics and Photonics, 11317 (2020). |
[23] |
H. Ohkubo, H. Nakagawa, A. Niimi, Computer-based quantitative computed tomography image analysis in idiopathic pulmonary fibrosis: A mini review, Respir. Invest., 56 (2018), 5-13. doi: 10.1016/j.resinv.2017.10.003
![]() |
[24] | J. Zhifeng, A. Feng, T. Li, Consistency analysis of COVID-19 nucleic acid tests and the changes of lung CT, J. Clin. Virol., 127(2020), 1-3. |
[25] | Z. Yongchen, H. Shen, X. Wang, X. Shi, Y. Li, J. Yan, et al., Different longitudinal patterns of nucleic acid and serology testing results based on disease severity of COVID-19 patients, Emerging Microbes Infect., 9 (2020), 1-14. |
[26] | J. Wu, J. Liu, S. Li, Z. Peng, J. Luo, Detection and analysis of nucleic acid in various biological samples of COVID-19 patients, Travel Med. Infect. Dis., 37 (2020), 1-2. |
[27] | A. M. Kunig, V. Balasubramaniam, N. E. Markham, D. Morgan, G. Montgomery, T. R. Grover, et al., Recombinant human VEGF treatment enhances alveolarization after hyperoxic lung injury in neonatal rats, Am. J. Physiol.-Lung Cell. Mol. Physiol., 289 (2005), 529-535. |
[28] | R. He, L. Han, P. Liu, H. Hu, J. Yang, H. Cai, et al., Lung function decline after 24 weeks of moxa smoke exposure in rats, Evidence-Based Complementary Altern. Med., 2019 (2019), 1-7. |
[29] |
M. Mao, J. Li, A. Bi, H. Jia, S. Xia, Thymoquinone ameliorates the PM2.5-induced lung injury in rats, Exp. Lung Res., 46 (2020), 297-307. doi: 10.1080/01902148.2020.1801895
![]() |
[30] |
U. Frey, J. Stocks, A. Coates, P. Sly, J. Bates, Specifications for equipment used for infant pulmonary function testing, Eur. Respir. J., 16 (2000), 1016-1022. doi: 10.1183/09031936.00.16510160
![]() |
[31] |
A. Virani, S. Baltaji, M. Young, T. Dumont, T. Cheema, Chronic obstructive pulmonary disease: diagnosis and gold classification, Crit. Care Nurs. Q., 44 (2021), 9-18. doi: 10.1097/CNQ.0000000000000335
![]() |
[32] |
A. K. Boutou, Z. Zoumot, A. Nair, C. Davey, D. M. Hansell, The impact of homogeneous versus heterogeneous emphysema on dynamic hyperinflation in patients with severe COPD assessed for lung volume reduction, COPD: J. Chronic Obstruct. Pulm. Dis., 12 (2015), 598-605. doi: 10.3109/15412555.2015.1020149
![]() |
[33] |
Y. Li, Y. L. Dai, N. Yu, Y. M. Guo, Sex-related differences in bronchial parameters and pulmonary function test results in patients with chronic obstructive pulmonary disease based on three-dimensional quantitative computed tomography, J. Int. Med. Res., 46 (2018), 135-142. doi: 10.1177/0300060517721309
![]() |
[34] |
N. Das, M. Topalovic, W. Janssens, Artificial intelligence in diagnosis of obstructive lung disease: current status and future potential, Current Opin. Pulm. Med., 24 (2018), 117-123. doi: 10.1097/MCP.0000000000000459
![]() |
[35] | H. Liang, B. Y. Tsui, H. Ni, C. C. Valentim, S. L.Baxter, G. Liu, et al., Evaluation and accurate diagnoses of pediatric diseases using artificial intelligence, Nat. Med., 25 (2019), 433-438. |
[36] | L. Li, L. Qin, Z. Xu, Y. Yin, X. Wang, B. Kong, et al., Artificial intelligence distinguishes COVID-19 from community acquired pneumonia on chest CT, Radiology, 296 (2020), 65-72. |
[37] | D. J. Maselli, H. Keyt, M. I. Restrepo, Inhaled antibiotic therapy in chronic respiratory diseases, Int. J. Mol. Sci., 18 (2017), 1-23. |
[38] | A. Guillon, T. Sécher, L. A. Dailey, L. Vecellio, M. De Monte, N. Heuzé-Vourc'h, Insights on animal models to investigate inhalation therapy: relevance for biotherapeutics, Int. J. Pharmaceutics, 536 (2018), 116-126. |
[39] |
J. E. Phillips, Inhaled phosphodiesterase 4 (PDE4) inhibitors for inflammatory respiratory diseases, Front. Pharmacol., 11 (2020), 1-7. doi: 10.3389/fphar.2020.00001
![]() |
[40] | N. M. Cheng, C. T. Yu, K. C. Ho, Y. C. Wu, Y. C. Liu, C. W. Wang, et al., Respiration-averaged CT for attenuation correction in non-small-cell lung cancer, Eur. J. Nucl. Med. Mol. Imaging, 36 (2009), 607-615. |
[41] | H. Y. Santema, J. Stolk, M. Los, B. C. Stoel, R. Tsonaka, I. T. Merth, Prediction of lung function and lung density of young adults who had bronchopulmonary dysplasia, ERJ Open Res., 6 (2020), 1-9. |
[42] |
D. M. Vasilescu, A. B. Phillion, D. Kinose, S. E. Verleden, J. C. Hogg, Comprehensive Stereological assessment of the human lung using multi-resolution computed tomography, J. Appl. Physiol., 128 (2020), 1604-1616. doi: 10.1152/japplphysiol.00803.2019
![]() |
[43] | J. Hofmanninger, F. Prayer, J. Pan, S. Rohrich, H. Prosch, G. Langs, Automatic lung segmentation in routine imaging is a data diversity problem, not a methodology problem, Eur. Radiol. Exp., 4 (2020), 1-13. |
[44] |
Q. Li, L. Chen, X. Li, X. Lv, S. Xia, Y. Kang, PRF-RW: a progressive random forest-based random walk approach for interactive semi-automated pulmonary lobes segmentation, Int. J. Mach. Learn. Cybern., 11 (2020), 2221-2235. doi: 10.1007/s13042-020-01111-9
![]() |
[45] | Q. Li; L. Chen, X. Li, S. Xia, Y. Kang, An improved random forests approach for interactive lobar segmentation on emphysema detection, Granular Comput., 5 (2019), 503-512. |
[46] |
F. Gibou, D. Levy, C Cárdenas, P. Liu, A. Boyer, Partial differential equations-based segmentation for radiotherapy treatment planning, Math. Biosci. Eng., 2 (2005), 209-226. doi: 10.3934/mbe.2005.2.209
![]() |
[47] | Y. Yang, Y. Guo, J. Guo, Y. Gao, Y. Kang, A method of abstracting single pulmonary lobe from computed tomography pulmonary images for locating COPD, in Proceedings of the Fourth International Conference on Biological Information and Biomedical Engineering (2020), 1-6. |
1. | Yingjian Yang, Wei Li, Yingwei Guo, Nanrong Zeng, Shicong Wang, Ziran Chen, Yang Liu, Huai Chen, Wenxin Duan, Xian Li, Wei Zhao, Rongchang Chen, Yan Kang, Lung radiomics features for characterizing and classifying COPD stage based on feature combination strategy and multi-layer perceptron classifier, 2022, 19, 1551-0018, 7826, 10.3934/mbe.2022366 | |
2. | Yingjian Yang, Wei Li, Yan Kang, Yingwei Guo, Kai Yang, Qiang Li, Yang Liu, Chaoran Yang, Rongchang Chen, Huai Chen, Xian Li, Lei Cheng, A novel lung radiomics feature for characterizing resting heart rate and COPD stage evolution based on radiomics feature combination strategy, 2022, 19, 1551-0018, 4145, 10.3934/mbe.2022191 | |
3. | Yingjian Yang, Ziran Chen, Wei Li, Nanrong Zeng, Yingwei Guo, Shicong Wang, Wenxin Duan, Yang Liu, Huai Chen, Xian Li, Rongchang Chen, Yan Kang, Multi-modal data combination strategy based on chest HRCT images and PFT parameters for intelligent dyspnea identification in COPD, 2022, 9, 2296-858X, 10.3389/fmed.2022.980950 | |
4. | Yingjian Yang, Shicong Wang, Nanrong Zeng, Wenxin Duan, Ziran Chen, Yang Liu, Wei Li, Yingwei Guo, Huai Chen, Xian Li, Rongchang Chen, Yan Kang, Lung Radiomics Features Selection for COPD Stage Classification Based on Auto-Metric Graph Neural Network, 2022, 12, 2075-4418, 2274, 10.3390/diagnostics12102274 | |
5. | Yingjian Yang, Wei Li, Yingwei Guo, Yang Liu, Qiang Li, Kai Yang, Shicong Wang, Nanrong Zeng, Wenxin Duan, Ziran Chen, Huai Chen, Xian Li, Wei Zhao, Rongchang Chen, Yan Kang, Early COPD Risk Decision for Adults Aged From 40 to 79 Years Based on Lung Radiomics Features, 2022, 9, 2296-858X, 10.3389/fmed.2022.845286 | |
6. | Peng Sun, Zengnan Mo, Fangrong Hu, Fang Liu, Taiping Mo, Yewei Zhang, Zhencheng Chen, Kidney Tumor Segmentation Based on FR2PAttU-Net Model, 2022, 12, 2234-943X, 10.3389/fonc.2022.853281 | |
7. | Yingjian Yang, Nanrong Zeng, Ziran Chen, Wei Li, Yingwei Guo, Shicong Wang, Wenxin Duan, Yang Liu, Rongchang Chen, Yan Kang, Weihua Yang, Multi‐Layer Perceptron Classifier with the Proposed Combined Feature Vector of 3D CNN Features and Lung Radiomics Features for COPD Stage Classification, 2023, 2023, 2040-2295, 10.1155/2023/3715603 | |
8. | Yingjian Yang, Jie Zheng, Peng Guo, Tianqi Wu, Qi Gao, Xueqiang Zeng, Ziran Chen, Nanrong Zeng, Zhanglei Ouyang, Yingwei Guo, Huai Chen, Hemi-diaphragm detection of chest X-ray images based on convolutional neural network and graphics, 2024, 32, 08953996, 1273, 10.3233/XST-240108 | |
9. | Yingjian Yang, Jie Zheng, Peng Guo, Tianqi Wu, Qi Gao, Yingwei Guo, Ziran Chen, Chengcheng Liu, Zhanglei Ouyang, Huai Chen, Yan Kang, Automatic cardiothoracic ratio calculation based on lung fields abstracted from chest X-ray images without heart segmentation, 2024, 15, 1664-042X, 10.3389/fphys.2024.1416912 | |
10. | Biswajit Jena, Suchismita Das, Bemnet Girma, Minakhi Rout, 2024, Comparison of Deep Neural Networks for Lung Image Segmentation on Chest X-Ray, 979-8-3503-5437-9, 1, 10.1109/ODICON62106.2024.10797610 | |
11. | Yingjian Yang, Jie Zheng, Peng Guo, Qi Gao, Yingwei Guo, Ziran Chen, Chengcheng Liu, Tianqi Wu, Zhanglei Ouyang, Huai Chen, Yan Kang, Three-stage registration pipeline for dynamic lung field of chest X-ray images based on convolutional neural networks, 2025, 8, 2624-8212, 10.3389/frai.2025.1466643 |