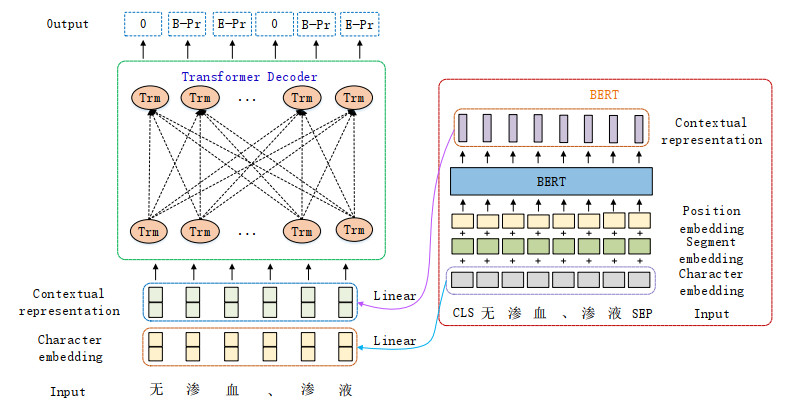
Clinical event detection (CED) is a hot topic and essential task in medical artificial intelligence, which has attracted the attention from academia and industry over the recent years. However, most studies focus on English clinical narratives. Owing to the limitation of annotated Chinese medical corpus, there is a lack of relevant research about Chinese clinical narratives. The existing methods ignore the importance of contextual information in semantic understanding. Therefore, it is urgent to research multilingual clinical event detection. In this paper, we present a novel encoder-decoder structure based on pre-trained language model for Chinese CED task, which integrates contextual representations into Chinese character embeddings to assist model in semantic understanding. Compared with existing methods, our proposed strategy can help model harvest a language inferential skill. Besides, we introduce the punitive weight to adjust the proportion of loss on each category for coping with class imbalance problem. To evaluate the effectiveness of our proposed model, we conduct a range of experiments on test set of our manually annotated corpus. We compare overall performance of our proposed model with baseline models on our manually annotated corpus. Experimental results demonstrate that our proposed model achieves the best precision of 83.73%, recall of 86.56% and F1-score of 85.12%. Moreover, we also evaluate the performance of our proposed model with baseline models on minority category samples. We discover that our proposed model obtains a significant increase on minority category samples.
Citation: Zhichang Zhang, Minyu Zhang, Tong Zhou, Yanlong Qiu. Pre-trained language model augmented adversarial training network for Chinese clinical event detection[J]. Mathematical Biosciences and Engineering, 2020, 17(4): 2825-2841. doi: 10.3934/mbe.2020157
[1] | Ruirui Han, Zhichang Zhang, Hao Wei, Deyue Yin . Chinese medical event detection based on event frequency distribution ratio and document consistency. Mathematical Biosciences and Engineering, 2023, 20(6): 11063-11080. doi: 10.3934/mbe.2023489 |
[2] | Luqi Li, Yunkai Zhai, Jinghong Gao, Linlin Wang, Li Hou, Jie Zhao . Stacking-BERT model for Chinese medical procedure entity normalization. Mathematical Biosciences and Engineering, 2023, 20(1): 1018-1036. doi: 10.3934/mbe.2023047 |
[3] | Chaofan Li, Kai Ma . Entity recognition of Chinese medical text based on multi-head self-attention combined with BILSTM-CRF. Mathematical Biosciences and Engineering, 2022, 19(3): 2206-2218. doi: 10.3934/mbe.2022103 |
[4] | Quan Zhu, Xiaoyin Wang, Xuan Liu, Wanru Du, Xingxing Ding . Multi-task learning for aspect level semantic classification combining complex aspect target semantic enhancement and adaptive local focus. Mathematical Biosciences and Engineering, 2023, 20(10): 18566-18591. doi: 10.3934/mbe.2023824 |
[5] | Xiaoqing Lu, Jijun Tong, Shudong Xia . Entity relationship extraction from Chinese electronic medical records based on feature augmentation and cascade binary tagging framework. Mathematical Biosciences and Engineering, 2024, 21(1): 1342-1355. doi: 10.3934/mbe.2024058 |
[6] | Han Zhu, Xiaohai He, Meiling Wang, Mozhi Zhang, Linbo Qing . Medical visual question answering via corresponding feature fusion combined with semantic attention. Mathematical Biosciences and Engineering, 2022, 19(10): 10192-10212. doi: 10.3934/mbe.2022478 |
[7] | Xin Liu, Chen Zhao, Bin Zheng, Qinwei Guo, Yuanyuan Yu, Dezheng Zhang, Aziguli Wulamu . Spatiotemporal and kinematic characteristics augmentation using Dual-GAN for ankle instability detection. Mathematical Biosciences and Engineering, 2022, 19(10): 10037-10059. doi: 10.3934/mbe.2022469 |
[8] | Xu Zhang, Wei Huang, Jing Gao, Dapeng Wang, Changchuan Bai, Zhikui Chen . Deep sparse transfer learning for remote smart tongue diagnosis. Mathematical Biosciences and Engineering, 2021, 18(2): 1169-1186. doi: 10.3934/mbe.2021063 |
[9] | Suxiang Yu, Shuai Zhang, Bin Wang, Hua Dun, Long Xu, Xin Huang, Ermin Shi, Xinxing Feng . Generative adversarial network based data augmentation to improve cervical cell classification model. Mathematical Biosciences and Engineering, 2021, 18(2): 1740-1752. doi: 10.3934/mbe.2021090 |
[10] | Kaixiong Zhang, Yongbing Zhang, Zhengtao Yu, Yuxin Huang, Kaiwen Tan . A two-stage fine-tuning method for low-resource cross-lingual summarization. Mathematical Biosciences and Engineering, 2024, 21(1): 1125-1143. doi: 10.3934/mbe.2024047 |
Clinical event detection (CED) is a hot topic and essential task in medical artificial intelligence, which has attracted the attention from academia and industry over the recent years. However, most studies focus on English clinical narratives. Owing to the limitation of annotated Chinese medical corpus, there is a lack of relevant research about Chinese clinical narratives. The existing methods ignore the importance of contextual information in semantic understanding. Therefore, it is urgent to research multilingual clinical event detection. In this paper, we present a novel encoder-decoder structure based on pre-trained language model for Chinese CED task, which integrates contextual representations into Chinese character embeddings to assist model in semantic understanding. Compared with existing methods, our proposed strategy can help model harvest a language inferential skill. Besides, we introduce the punitive weight to adjust the proportion of loss on each category for coping with class imbalance problem. To evaluate the effectiveness of our proposed model, we conduct a range of experiments on test set of our manually annotated corpus. We compare overall performance of our proposed model with baseline models on our manually annotated corpus. Experimental results demonstrate that our proposed model achieves the best precision of 83.73%, recall of 86.56% and F1-score of 85.12%. Moreover, we also evaluate the performance of our proposed model with baseline models on minority category samples. We discover that our proposed model obtains a significant increase on minority category samples.
With the continuous advancement of medical artificial intelligence, it is urgent to build a smart clinical decision support system. As an essential component of clinical decision support system, clinical event detection (CED) has attracted constant attention from academia and industry. The CED task aims to identify and classify all clinically relevant events and situations, including symptoms, exams, treatments and other occurrences in Chinese electronic medical records (EMRs). The Chinese EMRs contain a lot of valuable information. It is important that how to extract these relevant information from a large amount of Chinese EMRs quickly and accurately. The correct extraction result can improve the quality of medical text analysis. Moreover, extracting these information quickly and accurately can help doctors make decisions in the process of treatment. In the last decades, numerous methods have been proposed for Chinese CED task, including Hidden Markov Models (HMMs) [1], Support Vector Machines (SVMs) [2] and Conditional Random Fields (CRFs) [3]. Recently, with the development of deep learning, researchers begin to introduce the neural networks [4,5,6,7] for Chinese CED task.
Although these methods have achieved significant improvements in Chinese CED task, some issues still have not been well addressed. One significant drawback is that there is no publicly annotated corpus for Chinese CED task. The researchers need to annotate a corpus manually. Owing to the limitation of annotation costs, the scale of manually annotated corpora are usually small. The corpora also contain a great deal of noise. However, the improvements of performance and robustness crucially depend on a large amount of annotated training data. The small-scale corpus will limit the performance and robustness of model. To solve this problem, some researchers integrated external features into Chinese character representations to improve the performance of model [8,9]. Nevertheless, the above two approaches rely on external resources. They only work well when external resources are exhaustive. Another weakness is that the above two approaches ignore the importance of contextual information in semantic understanding. Fortunately, the BERT (Bidirectional Encoder Representations from Transformers) [10] trained on massive training data and can generate contextual representations dynamically according to contexts. The contextual information coming from the BERT can help model to understand obscure medical terms correctly. Moreover, the introduction of the BERT can improve the performance and robustness of models trained on a small amount of annotated training data. Thus, how to exploit the contextual information coming from the BERT for Chinese CED task is an important problem.
Another issue is that the distribution of categories is unbalanced in corpus. The class imbalance problem degrades overall performance of model and model's decision is biased to majority category samples, which leads to classifying minority category samples incorrectly. If the performance of model varies too much on each category, we can not evaluate overall performance of model objectively. Therefore, how to improve the performance of model on minority category samples is another important problem.
To address the above mentioned issues, we propose a transfer learning method to integrate contextual representations coming from the BERT into Chinese CED task. Moreover, to solve the class imbalance problem, we introduce an adversarial loss to improve the proportion of loss on minority category samples. Finally, we evaluate our model on our manually annotated corpus. Experimental results show that our proposed model achieves better overall performance than state-of-the-art models. In particular, our proposed model outperforms other models on minority category samples.
Many methods have been proposed for Chinese CED task. All these existing approaches can be roughly divided into four categories: rule-based approaches, knowledge-based approaches, traditional machine learning approaches and deep learning approaches.
Rule-based approaches rely on handcrafted rules to identify clinical events [11]. Because of their simplicity, they were used in early Chinese CED systems widely. They work effectively when rules are exhaustive. Rule-based approaches have a shortage of flexibility.
Knowledge-based approaches do not require annotated training data as they rely on lexicon resources and domain-specific knowledge to identify clinical events [12]. They also have poor flexibility. Moreover, they may achieve high precision and low recall.
Traditional machine learning approaches aim to make predictions by training on example inputs and their corresponding outputs. Typical methods are HMMs [1], SVMs [2] and CRFs [17]. However, they rely on handcrafted features. They work effectively when handcrafted features are excellent.
In recent years, deep learning approaches have been introduced for Chinese CED task [4,5,6,7,13]. Tang et al. [6] exploited an attention-based convolution neural network (CNN) to generate the representation of Chinese characters and fed into a bidirectional long short term memory (BiLSTM) to extract features. Finally, they used a conditional random field (CRF) as a decoder and gave a predicted label to each character in the sentences. The BiLSTM-CRF model achieved state-of-the-art performance in Chinese CED task and obtained a competitive result compared with traditional statistical models. Zhou et al. [7] treated each clinical narrative as a sequence of short sentences and proposed an end-to-end deep neural network framework for Chinese CED task. Moreover, they also proposed a smoothed viterbi decoder as a sequence labeller without additional parameter training, which can be a good alternative to the conditional random field (CRF) when computing resources are limited. Luo et al. [14] integrated attention mechanism into the BiLSTM-CRF model to utilize global information to enforce tag consistency across multiple instances of same token in a document. After that, the BiLSTM-CRF model is usually exploited as a baseline. Recently, transfer learning methods have achieved great success in sequence labeling tasks. For example, Cao et al. [15] introduced an adversarial transfer learning framework to jointly train Chinese named entity recognition (NER) task and Chinese word segmentation (WS) task, aiming to extract task-shared word boundary information from Chinese WS task. Johnson et al. [16] explored a cross-lingual transfer learning for NER task, focusing on bootstrapping Japanese from English. Different from the above transfer learning methods, we utilize a transfer learning method to integrate contextual representations coming from the BERT into Chinese CED task to enhance Chinese character representations.
In recent years, language representation models have been shown to be effective for improving many natural language processing (NLP) tasks [19,20]. So we consider integrating the language representation model BERT into Chinese CED task to improve the performance of task. The BERT trained on massive unlabeled texts and merged both left and right contexts in all layers together to represent contextual information. In this work, we integrate contextual information coming from the BERT into Chinese character representations to enhance semantic understanding.
The class imbalance problem is prevalent in classification tasks and sequence labeling tasks. All these existing approaches solving this issue can be roughly divided into two categories: Data-based approaches and algorithm-based approaches. The data-based approaches address this issue through two strategies: Over-sampling and under-sampling. However, these two strategies may lead to over-fitting and information loss. The algorithm-based approaches solve this problem by accounting for the disadvantages of algorithms. For instance, Lin et al. [18] addressed the class imbalance problem by modifying the standard cross-entropy loss to adjust the loss assigned to well-classified examples. Inspired by Lin et al. [18], we introduce the punitive weight to adjust the proportion of loss on each category. The equal proportion of loss on each category can assist the model to obtain an equal opportunity to optimize performance on each category.
In this paper, we propose an encoder-decoder structure based on transfer learning for Chinese CED task. The architecture of our proposed model is illustrated in Figure 1. The model mainly consists of two components: Semantic encoder and label decoder. In the following section, we will describe each part of our proposed model in detail.
In this work, we encode input sequences as contextual representations by the BERT. Here, we introduce the structure of the BERT briefly [10].
The BERT is a multi-layer bidirectional transformer encoder. Each transformer encoder consists of two sub-layers. The first layer is a multi-head self-attention mechanism, and the second is a simple position-wise fully connected feed-forward network. The residual connection network and normalization layer follow each sub-layer. The input of the BERT is constructed by summing character embedding, segment embedding and position embedding.
During the semantic encoding stage, we extract the output at the last layer of the BERT as contextual information of Chinese characters. Then, we concatenate each character's contextual representation and initial character embedding as decoder's input. Given an input sequence s={c1,c2,c3,…,cn}, each character's semantic representation Tci can be described as follows:
R=BERT(c1,c2,c3,…,cn) | (3.1) |
Tci=[Rci;Eci] | (3.2) |
where R represents the output at the last layer of the BERT. The Rci,Eci represent contextual information and initialized character embedding of character ci, respectively.
In the decoding stage, we adopt two transformer blocks as our label decoder to predict a label for each character. Each transformer block consists of two sub-layers: A multi-head self-attention mechanism and a simple position-wise fully connected feed-forward network. Here, we introduce the structure of transformer block briefly.
The transformer block exploits the multi-head self-attention mechanism to capture the dependencies between any two characters in the sentence and learn inner structure of sentence. The scaled dot-product attention can be defined as follows:
Attention(Q,K,V)=softmax(QKT√d)V | (3.3) |
where Q, K and V represent the query matrix, key matrix and value matrix, respectively. The d represents the dimension of key matrix. The multi-head self-attention can be expressed as follows:
headj=Attention(QWQj,KWKj,VWVj) | (3.4) |
MultiHead(Q,K,V)=Concat(head1,…,headh)WO | (3.5) |
where WQj, WKj, WVj and WO are trainable projection parameters.
Besides the multi-head self-attention mechanism, another essential component of transformer block is the position-wise fully connected feed-forward network, which is applied to each position separately and identically. The position-wise fully connected feed-forward network can be described as follows:
FFN(x)=max(0,xW1+b1)W2+b2 | (3.6) |
This formula consists of two linear transformations with a ReLU activation function in between.
We introduce the punitive weight into a standard cross-entropy loss to adjust the contribution of loss on each category. This strategy changes the proportion of loss on each category. The proportion of loss on majority category samples will confront the proportion of loss on minority category samples. More specifically, we utilize a penalty factor to balance the difference of data scale between different categories. We exploit the probability of labels to adjust the contribution of loss on each category. We call this novel loss "adversarial loss". The adversarial loss can be defined as follows:
L=−1NN∑i=1K∑k=1(1−yi,kPi,k)αyi,klogPi,k | (3.7) |
where N represents the amount of characters, K represents the amount of Chinese clinical event's categories, yi,k represents the true value of the ith character on the kth category, Pi,k represents the probability which the ith character is predicted as the kth category, 1−yi,kPi,k represents the penalty term and α represents the penalty factor.
To evaluate the effectiveness of our proposed model, we conduct a range of experiments on our manually annotated corpus. Specially, we name our manually annotated corpus Chinese CED corpus. The Chinese CED corpus consists of 2000 Chinese EMRs, coming from a third-level grade-A hospital in Gansu province. The Chinese CED corpus is divided as follows during the stage of experiment. Firstly, we divide 2000 annotated clinical narratives into initial train set and test set at a ratio of 3:1. Then, we select 400 clinical narratives from initial train set and test set as the development set randomly. Finally, we choose the rest of initial train set and test set to construct the final train set and test set, respectively. Table 1 shows the distribution of Chinese CED corpus.
Train | Development | Test | Total |
1300 | 400 | 300 | 2000 |
In the stage of dataset annotation, we do as follows. More specifically, we work out an annotation specification and develop an annotation tool for Chinese CED corpus. The annotation specification refers to 2012 i2b2 (Informatics for Integrating Biology & the Bedside) clinical temporal relations challenge annotation guidelines. Moreover, we make the following improvements and supplements. We annotate the discharge summaries and progress notes with seven types of clinical events (Problem, Exam, Treatment, Clinical department, Evidence, Occurrence and Aspectual). The "Problem" event type includes patient's complaints, symptoms, diseases and diagnoses. The "Exam" event type is used for clinical tests (laboratory and physical) and test results. The "Treatment" event type includes medications, surgeries and other procedures. The "Clinical department" event type is used to mark the clinical unit. The "Evidence" event type is used to state the source of information. The "Occurrence" event type is used for all the other kinds of clinically relevant events which happened to the patient. The "Aspectual" event type includes the state of current clinical event. Just marking the type of each clinical event is not enough. In order for the annotation to be useful in text analysis, we need to describe each clinical event in more detail. Besides clinical event category, we also annotate another two attributes of clinical events: Polarity and degree. The polarity attribute marks whether a clinical event is positive or negative. Most of the clinical events have "POS" polarity value, that is, the clinical event is not negated. It is to be noted that a clinical event can be POS even if it did not actually occur (If the clinical event is hypothetical or proposed.). If a clinical event is negated by words such as "not", "deny", and so on, its polarity is "NEG". Moreover, we also utilize degree attribute to mark the degree of clinical events. There are three type of clinical event degree attributes: "MOST", "LITTLE" and "NA". Table 2 lists the examples of Chinese clinical events. The bold words represent the clinical events in the sentence.
Sentence | Category | Polarity | Degree |
右侧肢体麻木加重 (Numbness in the right limb has increased) |
Problem | POS | MOST |
密切监测血常规 (Monitor blood routine closely) |
Exam | POS | MOST |
胰岛素强化治疗 Intensive insulin therapy) |
Treatment | POS | MOST |
患儿收住儿科 (The kid was admitted to pediatrics) |
Clinical department | POS | NA |
双肺叩诊呈过清音 (The sound of percussion in both lungs is too clear) |
Evidence | POS | NA |
患者收住入院 (The patient was admitted to hospital) |
Occurrence | POS | NA |
患者停用丹红注射液 (The patient stopped using Danhong injection) |
Aspectual | POS | NA |
Twenty copies of same clinical narratives were annotated in pairs before the formal annotation. The annotators record the uncertain textfields in the process of annotation. Two people in the group exchange annotation results and proofread their partner's annotation results after the own data annotation. They also record inconsistent textfields. All crews discuss the uncertain and inconsistent textfields and modify the annotation specification and annotation results together after a round of annotation. Then, we conduct five round of preliminary annotations. The clinical narratives in the five round of preliminary annotations are different. When we conduct the fifth round preliminary annotation, the error rate of all annotators were both less than 5%. So, we think that the annotation specification and annotators have met the requirements of formal annotation. Moreover, the annotators may encounter difficult medical terms during the process of annotation. So our annotation group members consider that twelve laboratory members and two medical students with more than five years of learning experience are involved in annotation specification development and annotation discussion. Besides difficult medical terms, there are other challenges in the process of corpus construction. For example, the corpus may contain a great deal of noise. Noise comes from two stages. Doctors may make writing errors in the EMRs. Moreover, the annotators may make annotation mistakes. In order to reduce the noise, we take the following two steps. Firstly, two medical students in our annotation group modify the writing errors in the EMRs before the formal annotation. Secondly, all annotation members discuss the annotation results and make a double check after the annotation.
In addition, we also record the detailed statistics of the Chinese CED corpus. Table 3 shows the detailed statistics of Chinese CED corpus. We can find that the data scale belonging to "Clinical department" is very small. We view "Clinical department" as a minority category. We adopt the Precision, Recall and F1-score as an evaluation metrics of overall performance in our experiments. Besides, we also use the Recall and F1-score as an evaluation metrics to evaluate the performance of "Clinical department".
Category | Train | Development | Test | Total |
Problem | 47669 | 4006 | 14811 | 66486 |
Exam | 18939 | 1949 | 5983 | 26871 |
Treatment | 9622 | 989 | 2943 | 13554 |
Clinical department | 114 | 9 | 44 | 167 |
Evidence | 6624 | 666 | 2136 | 9426 |
Occurrence | 3104 | 356 | 980 | 4440 |
Aspectual | 2386 | 291 | 812 | 3489 |
Other | 568897 | 50763 | 173099 | 792759 |
Total | 657355 | 59029 | 200808 | 917192 |
For hyper-parameter configurations, we adjust them according to our proposed model's performance on development set of Chinese CED corpus. It's worth noting that we fix all parameters coming from the BERT and only update all parameters coming from label decoder during the model's training stage. In this section, we will introduce the information about semantic encoder and label decoder in detail.
In this work, we apply the BERT-Base-Chinese Pre-trained Model for obtaining each character's contextual information. The BERT-Base-Chinese Pre-trained Model trained on massive cased Chinese simplified and traditional texts. Table 4 shows the detailed statistics of pre-trained language model.
Parameter description | Value |
The amount of transformer encoder blocks | 12 |
The dimension of intermediate layer | 768 |
The amount of multi-head attention mechanism's heads | 12 |
The amount of parameters | 110M |
In addition, we exploit two transformer blocks as our proposed model's decoder. Each transformer block consists of two sub-layers: A multi-head self-attention mechanism and a simple position-wise fully connected feed-forward network. Table 5 shows the major settings of decoder.
Parameter description | Value |
The amount of transformer decoder blocks | 2 |
Chinese character embedding size | 308 |
Contextual representation size | 204 |
The amount of multi-head attention mechanism's heads | 8 |
The dimension of intermediate layer | 512 |
Initial learning rate | 0.001 |
Batch size | 50 |
Penalty factor | 2 |
Dropout rate | 0.1 |
In the experimental section, we use multiple baseline models to compare with our proposed method. Here, we introduce baseline models briefly.
● CRF: In this work, we use the CRF++ to implement the CRF model.
● CNN-Softmax: The model utilizes the CNN to extract features and feeds into the multilayer perceptron (MLP) to decode.
● CNN-CRF: The model adopts the CNN to extract features and feeds into the CRF to decode.
● BiGRU-Softmax: The model exploits the bidirectional gated recurrent unit (BiGRU) to extract features and feeds into the MLP to decode.
● BiLSTM-CRF: The model adopts the BiLSTM to extract features and feeds into the CRF to decode.
● Attention-based CNN-BiLSTM-CRF: Tang et al. [6] exploited an attention-based CNN to generate the representations of Chinese characters and fed into the BiLSTM to extract features. Finally, they used the CRF as the model's decoder.
● BERT-Softmax: The model utilizes the BERT to obtain semantic representations and feeds into the MLP to decode.
● BERT-BiLSTM-Softmax: The model uses the BERT to obtain semantic representations and feeds into the BiLSTM to decode.
● BERT-Transformer-Softmax: The model adopts the BERT to obtain the semantic representations and feeds into the transformer to decode.
We compare overall performance of our proposed model with baseline models on test set of Chinese CED corpus. Table 6 shows the detailed experimental results of baseline models and our proposed model. The first column of Table 6 lists baseline models and our proposed model. Our proposed model achieves the highest precision of 83.73%, recall of 86.56% and F1-score of 85.12%. Compared with the BiLSTM-CRF model, our proposed model improves the F1-score from 82.97 to 85.12% and obtains an increase of 2.15%. Compared with the BERT-Softmax model, our proposed model improves the F1-score from 83.34 to 85.12% and obtains an increase of 1.78%. All in all, our proposed model outperforms other state-of-the-art methods significantly and consistently.
Model | Precision(%) | Recall(%) | F1-score(%) |
CRF | 79.96 | 85.37 | 82.58 |
CNN-Softmax | 75.20 | 83.40 | 79.09 |
CNN-CRF | 72.93 | 74.38 | 73.65 |
BiGRU-Softmax | 80.27 | 85.62 | 82.86 |
BiLSTM-CRF | 81.32 | 84.68 | 82.97 |
CNN-BiLSTM-CRF | 80.98 | 74.84 | 77.79 |
BERT-Softmax | 81.43 | 85.34 | 83.34 |
BERT-BiLSTM-Softmax | 82.62 | 84.77 | 83.68 |
BERT-Transformer-Softmax | 83.54 | 85.23 | 84.38 |
Ours | 83.73 | 86.56 | 85.12 |
Here, we summarize several reasons for the success of our proposed model. Firstly, we adopt the BERT to generate contextual representations and integrate contextual representations into Chinese character embeddings, which enhances the representations of Chinese characters. Secondly, we use the transformer block as our model's decoder, which pays different attention to each position of semantic representations. Thirdly, we utilize an "adversarial loss" to balance the difference of data scale on each category.
Besides, we also record the performance of our proposed model on each category. Table 7 shows the detailed experimental results. As shown in Table 7, our proposed model achieves impressive results on majority category samples. For example, the F1-score of "Problem" and "Exam" are 88.77% and 85.85%, respectively.
Category | Precision(%) | Recall(%) | F1-score(%) |
Problem | 87.42 | 90.16 | 88.77 |
Exam | 82.81 | 89.12 | 85.85 |
Treatment | 81.85 | 88.06 | 84.84 |
Clinical department | 74.72 | 62.44 | 68.03 |
Evidence | 76.13 | 86.40 | 80.94 |
Occurrence | 89.13 | 92.44 | 90.75 |
Aspectual | 75.96 | 86.87 | 81.05 |
In our manually annotated corpus, "Clinical department" is viewed as a minority category. The main reason is that the data scale of "Clinical department" is much less than the data scale of other clinical event's categories. As is shown in Table 3, the data scale of "Clinical department" varies from tens to hundreds of times compared with other clinical event's categories. In this work, we introduce an "adversarial loss" to adjust the proportion of loss on each category to improve the performance of model on each category. This strategy compels model to learn more knowledge about "Clinical department". To some extent, it reduces the gap of data scale on each category. Our proposed model achieves the recall of 62.44% and F1-score of 68.03% on "Clinical department".
To prove the effectiveness of "adversarial loss", we conduct a contrasting experiment on Chinese CED corpus. The only difference between contrasting experiment and our proposed model is loss function. Our proposed model exploits the "adversarial loss" as loss function. The contrasting experiment uses a standard cross-entropy loss as loss function. Table 8 shows the experimental results of contrasting experiment.
Category | Precision(%) | Recall(%) | F1-score(%) |
Problem | 84.17 | 88.94 | 86.49 |
Exam | 80.42 | 90.12 | 84.99 |
Treatment | 81.92 | 88.34 | 85.01 |
Clinical department | 80.52 | 57.65 | 67.19 |
Evidence | 75.58 | 84.19 | 79.65 |
Occurrence | 90.21 | 90.39 | 90.30 |
Aspectual | 76.34 | 85.71 | 80.75 |
As shown in Table 8, the F1-score of "Problem" and "Exam" are 86.49 and 84.99%, respectively. We discover that the introduction of "adversarial loss" hardly degrades the performance of model on majority category samples. If model's performance degrades in a particular clinical event, the degradation is minimal. For example, the F1-score of "Treatment" is 85.01% on contrasting experiment. The F1-score of "Treatment" is 84.84% on our proposed model. Specially, our proposed model outperforms contrasting experiment on "Clinical department". The F1-score of "Clinical department" is 67.19% on contrasting experiment. The F1-score of "Clinical department" is 68.03% on our proposed model. Our proposed model obtains an increase of 0.84%.
Besides, we also record the F1-score of existing models and our proposed model on "Clinical department". Figure 2 shows the detailed experimental results.
As shown in Figure 2, our proposed model achieves the highest F1-score of 68.03% on "Clinical department". Compared with the BiLSTM-CRF model, our proposed model improves the F1-score from 63.66 to 68.03% and obtains an increase of 4.37%. Compared with the BERT-Softmax model, our proposed model improves the F1-score from 65.67 to 68.03% and obtains an increase of 2.36%. The above results demonstrate that our proposed strategy can alleviate the class imbalance problem effectively. The main reason is that the introduction of "adversarial loss" can adjust the proportion of loss on each category. More specifically, models usually learn more knowledge from large amount of samples and learn less knowledge from small amount of samples. It will lead to giving correct labels to majority category samples. When model encounters a minority category sample, it will give an incorrect label to sample. In this work, we utilize an "adversarial loss" to adjust the proportion of loss on each category. It will reduce incorrect decisions caused by unbalanced data scale.
To validate the effectiveness of contextual information, we conduct the following experiments. The contrasting experiment only uses initial character embeddings from the BERT as the representations of Chinese characters. Table 9 shows the detailed experimental results.
Model | Precision(%) | Recall(%) | F1-score(%) |
Ours(-contextual representation) | 82.34 | 84.07 | 83.20 |
Ours | 83.73 | 86.56 | 85.12 |
Our proposed model achieves the F1-score of 85.12%. The contrasting experiment gets the F1-score of 83.20%. Our proposed model outperforms contrasting experiment by 1.92%, which indicates contextual information from the BERT is effective for Chinese CED task. The main reason is that our proposed model can utilize contextual information to understand the meaning of sentences under a specific semantic context. This strategy will assist model in harvesting a skill of semantic understanding. This approach is consistent with the process of human thought.
To evaluate the effectiveness of transformer decoder, we conduct the following experiments. The contrasting experiment utilizes the BiLSTM as its decoder and "adversarial loss" to compute loss. Table 10 shows the experimental results.
Model | Precision(%) | Recall(%) | F1-score(%) |
BERT-BiLSTM-Softmax(adversarial loss) | 82.75 | 86.35 | 84.51 |
Ours | 83.73 | 86.56 | 85.12 |
Our proposed model achieves the best precision of 83.73%, recall of 86.56% and F1-score of 85.12%. Compared with the BERT-BiLSTM-Softmax (adversarial loss) model, our proposed model improves the F1-score from 84.51 to 85.12% and obtains an increase of 0.61%. It verifies that transformer decoder owns a better decoding capacity than the BiLSTM. The main reason of our proposed model's success is multi-head self-attention mechanism can assist transformer block in paying different attention to each position of sentence. It will help model to capture pivotal information and use these information to assist model in making decisions.
To confirm the effectiveness of "adversarial loss" on "Clinical department", we conduct the following experiments. We choose the BiLSTM-CRF model and the BERT-Softmax model as basic models. Then, we adopt the standard cross-entropy loss and "adversarial loss" to compute loss, respectively. In addition, we also exploit the standard cross-entropy loss to replace "adversarial loss" to conduct extra experiment. Table 11 shows the detailed experimental results.
Model | Precision(%) | Recall(%) | F1-score(%) |
BiLSTM-CRF | 71.80 | 57.18 | 63.66 |
BiLSTM-CRF (adversarial loss) | 73.14 | 59.28 | 65.48 |
BERT-Softmax | 81.16 | 55.15 | 65.67 |
BERT-Softmax(adversarial loss) | 73.28 | 61.74 | 67.02 |
Ours (standard cross-entropy loss) | 80.52 | 57.65 | 67.19 |
Ours (fixed rate of loss allocation) | 70.25 | 57.34 | 63.14 |
Ours | 74.72 | 62.44 | 68.03 |
The BiLSTM-CRF model using "adversarial loss" outperforms the standard BiLSTM-CRF model and obtains an increase of 1.82% on the F1-score. The BERT-Softmax model using "adversarial loss" outperforms the standard BERT-Softmax model and obtains an increase of 1.35% on the F1-score. Our proposed model outperforms corresponding contrasting experiment and obtains an increase of 0.84% on the F1-score. It proves that "adversarial loss" can alleviate class imbalance problem effectively. Moreover, we also explore the allocation strategy of loss on each category. The contrasting experiment sets a fixed rate of loss allocation on each category. We find that our proposed model outperforms contrasting experiment and obtains an increase of 4.89% on the F1-score. The main reason is that "adversarial loss" can allocate the proportion of loss for each category flexibly.
The class imbalance problem is prevalent in Chinese CED tasks. The categories containing scarce instances may be significant. We shouldn't ignore the performance of model on minority category samples. In this work, we adopt an "adversarial loss" to solve the class imbalance problem. Here, we take a sentence from test set of Chinese CED corpus as an example for illustrating the effectiveness of our proposed strategy. Table 12 shows the detailed results. In the example, baselines give an "O" label to "神经外科" (neurosurgery department). Our proposed model gives a "Cd" label (Clinical department) to "神经外科" (neurosurgery department). The label predicted by our proposed model is correct. The baselines make incorrect predictions. The reason is that baselines adopt the standard cross-entropy loss to compute loss, which compels model to be biased toward majority category samples and can not obtain crucial information from samples belonging to minority category. Different from baseline models, our proposed model introduces the punitive weight into loss to balance the difference of each category's data scale. It will assist model in learning more crucial information from data belonging to minority category. Moreover, contextual information coming from the BERT can help model understand the meaning of sentence under a specific context.
Sentence | 患 | 者 | 就 | 诊 | 于 | 神 | 经 | 外 | 科 |
Golden | O | O | O | O | O | B-Cd | M-Cd | M-Cd | E-Cd |
Baselines | O | O | O | O | O | O | O | O | O |
Ours | O | O | O | O | O | B-Cd | M-Cd | M-Cd | E-Cd |
In this paper, we propose a novel encoder-decoder structure based on transfer learning for Chinese CED task, which integrates contextual representations into Chinese character embeddings to assist model in semantic understanding. Besides, we introduce an "adversarial loss" to solve the class imbalance problem. Experimental results on test set of Chinese CED corpus demonstrate that our proposed model outperforms state-of-the-art methods significantly and consistently. In particular, our model achieves superior performance than other models on minority category samples. In the future, we will explore more allocation strategies of loss and compare experiment result of each strategy.
We would like to thank the anonymous reviewers for their valuable comments. The Publication of the article is supported by the National Natural Science Foundation of China (No. 61762081, No. 61662067, No. 61662068) and the Key Research and Development Project of Gansu Province (No. 17YF1GA016).
The authors declare that they have no competing interests.
[1] | A. Vlachos, Evaluating and combining and biomedical named entity recognition systems, Proceedings of the Workshop on BioNLP: Biological, translational, and clinical language processing, Association for Computational Linguistics, 2007,199-206. Available from: https://dl.acm.org/doi/10.5555/1572392.1572430. |
[2] | Z. F. Ju, J. Wang, F. Zhu, Named entity recognition from biomedical text using SVM, International Conference on Bioinformatics and Biomedical Engineering, Institute of Electrical and Electronics Engineers, 2011, 1-4. Available from: https://ieeexplore.ieee.xilesou.top/abstract/document/5779984. |
[3] | A. McCallum, W. Li, Early results for named entity recognition with conditional random fields, feature induction and web-enhanced lexicons, Proceedings of the Seventh Conference on Natural Language Learning at HLT-NAACL, Association for Computational Linguistics, 2003,188-191. Available from: https://dlacm.xilesou.top/doi/10.3115/1119176.1119206. |
[4] | L. S. Li, L. K. Jin, Z. C Jiang, D. X. Song, D. G. Huang, Biomedical named entity recognition based on extended recurrent neural networks, International Conference on Bioinformatics and Biomedicine (BIBM), Institute of Electrical and Electronic Engineers Computer Society, 2015,649-652. Available from: https://ieeexplore.ieee.xilesou.top/abstract/document/7359761/authors#authors. |
[5] | L. S. Li, Y. X. Jiang, Biomedical named entity recognition based on the two channels and sentencelevel reading control conditioned LSTM-CRF, International Conference on Bioinformatics and Biomedicine (BIBM), Institute of Electrical and Electronic Engineers Computer Society, 2017,380-385. Available from: https://ieeexplore.ieee.xilesou.top/abstract/document/8217679. |
[6] |
B. Z. Tang, X. L. Wang, J. Yan, Q. C. Chen, Entity recognition in Chinese clinical text using attention-based CNN-LSTM-CRF, BMC Med. Inf. Decis. Making, 19 (2019), 74. doi: 10.1186/s12911-019-0787-y
![]() |
[7] |
X. S. Zhou, H. Q. Xiong, S. H. Zeng, X. L. Fu, J. Wu, An approach for medical event detection in Chinese clinical notes of electronic health records, BMC Med. Inf. Decis. Making, 19 (2019), 54. doi: 10.1186/s12911-019-0756-5
![]() |
[8] | E. Ouyang, Y. X. Li, L. Jin, Z. F. Li, X. Y. Zhang, Exploring n-gram character presentation in bidirectional RNN-CRF for Chinese clinical named entity recognition, CEUR Workshop Proceedings, Institute of Electrical and Electronic Engineers Computer Society, 2017, 37-42. Available from: http://ceur-ws.org/Vol-1976/paper07.pdf. |
[9] | Y. F. Wang, S. Ananiadou, J. I. Tsujii, Improve Chinese clinical named entity recognition performance by using the graphical and phonetic feature, International Conference on Bioinformatics and Biomedicine (BIBM), Institute of Electrical and Electronic Engineers Computer Society, 2018, 1582-1586. Available from: https://ieeexplore.ieee.xilesou.top/abstract/document/8621201. |
[10] | J. Devlin, M. W. Chang, K. Lee, K. Toutanova, Bert: Pre-training of deep bidirectional transformers for language understanding, arXiv, 2018, arXiv: 1810.04805. |
[11] | X. Wang, Y. Zhang, Q. Li, Cathy H. Wu, J. W. Han, PENNER: Pattern-enhanced nested named entity recognition in biomedical literature, International Conference on Bioinformatics and Biomedicine (BIBM), Institute of Electrical and Electronic Engineers Computer Society, 2018. Available from: https://ieeexplore.ieee.xilesou.top/abstract/document/8621485. |
[12] |
M. Gerner, G. Nenadic, C. M. Bergman, LINNAEUS: A species name identification system for biomedical literature, BMC Bioinf., 11 (2010), 85. doi: 10.1186/1471-2105-11-85
![]() |
[13] | Z. H. Zhao, Z. H. Yang, L. Luo, Y. Zhang, L. Wang, H. F. Lin, et al., MLCNN: A novel deep learning based disease named entity recognition architecture, International Conference on Bioinformatics and Biomedicine (BIBM), Institute of Electrical and Electronic Engineers Computer Society, 2016,794-794. Available from: https://ieeexplore.ieee.xilesou.top/abstract/document/7822625. |
[14] |
L. Luo, Z. H. Yang, P. Yang, Y. Zhang, L. Wang, H. F. Lin, et al., An attention-based BiLSTMCRF approach to document-level chemical named entity recognition, Bioinformatics, 34 (2018), 1381-1388. doi: 10.1093/bioinformatics/btx761
![]() |
[15] | P. F. Cao, Y. B. Chen, K. Liu, J. Zhao, S. P. Liu, Adversarial transfer learning for chinese named entity recognition with self-attention mechanism, Conference on Empirical Methods in Natural Language Processing, Association for Computational Linguistics, (2018), 182-192. Available from: https://www.aclweb.org/anthology/D18-1017/. |
[16] | A. Johnson, P. Karanasou, J. Gaspers, D. Klakow, Cross-lingual transfer learning for Japanese named entity recognition, Conference of the North American Chapter of the Association for Computational Linguistics: Human Language Technologies, Volume 2 (Industry Papers), Association for Computational Linguistics, 2019,182-189. Available from: https://www.aclweb.org/anthology/N19-2023/. |
[17] | R. Leaman, C. H. Wei, C. Zou, Z. Y. Lu, Mining chemical patents with an ensemble of open systems, Database, 2016 (2016), baw065. |
[18] | T. Y. Lin, P. Goyal, R. Girshick, K. He, P. Dollár, Focal loss for dense object detection, The IEEE International Conference on Computer Vision (ICCV), 2017, 2980-2988. Available from: http://openaccess.thecvf.com/content_iccv_2017/html/Lin_Focal_Loss_for_ICCV_2017_paper.html. |
[19] | H. Jeremy, R. Sebastian, Universal language model fine-tuning for text classification, arXiv, 2018, arXiv: 1801.06146. |
[20] | A. Williams, N. Nangia, S. R. Bowman, A broad-coverage challenge corpus for sentence understanding through inference, arXiv, 2017, arXiv: 1704.05426. |
1. | Yunjin Yum, Jeong Moon Lee, Moon Joung Jang, Yoojoong Kim, Jong-Ho Kim, Seongtae Kim, Unsub Shin, Sanghoun Song, Hyung Joon Joo, A Word Pair Dataset for Semantic Similarity and Relatedness in Korean Medical Vocabulary: Reference Development and Validation, 2021, 9, 2291-9694, e29667, 10.2196/29667 | |
2. | Ting-Huai Ma, Xin Yu, Huan Rong, A comprehensive transfer news headline generation method based on semantic prototype transduction, 2022, 20, 1551-0018, 1195, 10.3934/mbe.2023055 | |
3. | Wenjun Xiang, Zhichang Zhang, Ziqin Zhang, Deyue Yin, 2024, Chapter 11, 978-981-99-9863-0, 164, 10.1007/978-981-99-9864-7_11 | |
4. | Ruirui Han, Zhichang Zhang, Hao Wei, Deyue Yin, Chinese medical event detection based on event frequency distribution ratio and document consistency, 2023, 20, 1551-0018, 11063, 10.3934/mbe.2023489 |
Train | Development | Test | Total |
1300 | 400 | 300 | 2000 |
Sentence | Category | Polarity | Degree |
右侧肢体麻木加重 (Numbness in the right limb has increased) |
Problem | POS | MOST |
密切监测血常规 (Monitor blood routine closely) |
Exam | POS | MOST |
胰岛素强化治疗 Intensive insulin therapy) |
Treatment | POS | MOST |
患儿收住儿科 (The kid was admitted to pediatrics) |
Clinical department | POS | NA |
双肺叩诊呈过清音 (The sound of percussion in both lungs is too clear) |
Evidence | POS | NA |
患者收住入院 (The patient was admitted to hospital) |
Occurrence | POS | NA |
患者停用丹红注射液 (The patient stopped using Danhong injection) |
Aspectual | POS | NA |
Category | Train | Development | Test | Total |
Problem | 47669 | 4006 | 14811 | 66486 |
Exam | 18939 | 1949 | 5983 | 26871 |
Treatment | 9622 | 989 | 2943 | 13554 |
Clinical department | 114 | 9 | 44 | 167 |
Evidence | 6624 | 666 | 2136 | 9426 |
Occurrence | 3104 | 356 | 980 | 4440 |
Aspectual | 2386 | 291 | 812 | 3489 |
Other | 568897 | 50763 | 173099 | 792759 |
Total | 657355 | 59029 | 200808 | 917192 |
Parameter description | Value |
The amount of transformer encoder blocks | 12 |
The dimension of intermediate layer | 768 |
The amount of multi-head attention mechanism's heads | 12 |
The amount of parameters | 110M |
Parameter description | Value |
The amount of transformer decoder blocks | 2 |
Chinese character embedding size | 308 |
Contextual representation size | 204 |
The amount of multi-head attention mechanism's heads | 8 |
The dimension of intermediate layer | 512 |
Initial learning rate | 0.001 |
Batch size | 50 |
Penalty factor | 2 |
Dropout rate | 0.1 |
Model | Precision(%) | Recall(%) | F1-score(%) |
CRF | 79.96 | 85.37 | 82.58 |
CNN-Softmax | 75.20 | 83.40 | 79.09 |
CNN-CRF | 72.93 | 74.38 | 73.65 |
BiGRU-Softmax | 80.27 | 85.62 | 82.86 |
BiLSTM-CRF | 81.32 | 84.68 | 82.97 |
CNN-BiLSTM-CRF | 80.98 | 74.84 | 77.79 |
BERT-Softmax | 81.43 | 85.34 | 83.34 |
BERT-BiLSTM-Softmax | 82.62 | 84.77 | 83.68 |
BERT-Transformer-Softmax | 83.54 | 85.23 | 84.38 |
Ours | 83.73 | 86.56 | 85.12 |
Category | Precision(%) | Recall(%) | F1-score(%) |
Problem | 87.42 | 90.16 | 88.77 |
Exam | 82.81 | 89.12 | 85.85 |
Treatment | 81.85 | 88.06 | 84.84 |
Clinical department | 74.72 | 62.44 | 68.03 |
Evidence | 76.13 | 86.40 | 80.94 |
Occurrence | 89.13 | 92.44 | 90.75 |
Aspectual | 75.96 | 86.87 | 81.05 |
Category | Precision(%) | Recall(%) | F1-score(%) |
Problem | 84.17 | 88.94 | 86.49 |
Exam | 80.42 | 90.12 | 84.99 |
Treatment | 81.92 | 88.34 | 85.01 |
Clinical department | 80.52 | 57.65 | 67.19 |
Evidence | 75.58 | 84.19 | 79.65 |
Occurrence | 90.21 | 90.39 | 90.30 |
Aspectual | 76.34 | 85.71 | 80.75 |
Model | Precision(%) | Recall(%) | F1-score(%) |
Ours(-contextual representation) | 82.34 | 84.07 | 83.20 |
Ours | 83.73 | 86.56 | 85.12 |
Model | Precision(%) | Recall(%) | F1-score(%) |
BERT-BiLSTM-Softmax(adversarial loss) | 82.75 | 86.35 | 84.51 |
Ours | 83.73 | 86.56 | 85.12 |
Model | Precision(%) | Recall(%) | F1-score(%) |
BiLSTM-CRF | 71.80 | 57.18 | 63.66 |
BiLSTM-CRF (adversarial loss) | 73.14 | 59.28 | 65.48 |
BERT-Softmax | 81.16 | 55.15 | 65.67 |
BERT-Softmax(adversarial loss) | 73.28 | 61.74 | 67.02 |
Ours (standard cross-entropy loss) | 80.52 | 57.65 | 67.19 |
Ours (fixed rate of loss allocation) | 70.25 | 57.34 | 63.14 |
Ours | 74.72 | 62.44 | 68.03 |
Sentence | 患 | 者 | 就 | 诊 | 于 | 神 | 经 | 外 | 科 |
Golden | O | O | O | O | O | B-Cd | M-Cd | M-Cd | E-Cd |
Baselines | O | O | O | O | O | O | O | O | O |
Ours | O | O | O | O | O | B-Cd | M-Cd | M-Cd | E-Cd |
Train | Development | Test | Total |
1300 | 400 | 300 | 2000 |
Sentence | Category | Polarity | Degree |
右侧肢体麻木加重 (Numbness in the right limb has increased) |
Problem | POS | MOST |
密切监测血常规 (Monitor blood routine closely) |
Exam | POS | MOST |
胰岛素强化治疗 Intensive insulin therapy) |
Treatment | POS | MOST |
患儿收住儿科 (The kid was admitted to pediatrics) |
Clinical department | POS | NA |
双肺叩诊呈过清音 (The sound of percussion in both lungs is too clear) |
Evidence | POS | NA |
患者收住入院 (The patient was admitted to hospital) |
Occurrence | POS | NA |
患者停用丹红注射液 (The patient stopped using Danhong injection) |
Aspectual | POS | NA |
Category | Train | Development | Test | Total |
Problem | 47669 | 4006 | 14811 | 66486 |
Exam | 18939 | 1949 | 5983 | 26871 |
Treatment | 9622 | 989 | 2943 | 13554 |
Clinical department | 114 | 9 | 44 | 167 |
Evidence | 6624 | 666 | 2136 | 9426 |
Occurrence | 3104 | 356 | 980 | 4440 |
Aspectual | 2386 | 291 | 812 | 3489 |
Other | 568897 | 50763 | 173099 | 792759 |
Total | 657355 | 59029 | 200808 | 917192 |
Parameter description | Value |
The amount of transformer encoder blocks | 12 |
The dimension of intermediate layer | 768 |
The amount of multi-head attention mechanism's heads | 12 |
The amount of parameters | 110M |
Parameter description | Value |
The amount of transformer decoder blocks | 2 |
Chinese character embedding size | 308 |
Contextual representation size | 204 |
The amount of multi-head attention mechanism's heads | 8 |
The dimension of intermediate layer | 512 |
Initial learning rate | 0.001 |
Batch size | 50 |
Penalty factor | 2 |
Dropout rate | 0.1 |
Model | Precision(%) | Recall(%) | F1-score(%) |
CRF | 79.96 | 85.37 | 82.58 |
CNN-Softmax | 75.20 | 83.40 | 79.09 |
CNN-CRF | 72.93 | 74.38 | 73.65 |
BiGRU-Softmax | 80.27 | 85.62 | 82.86 |
BiLSTM-CRF | 81.32 | 84.68 | 82.97 |
CNN-BiLSTM-CRF | 80.98 | 74.84 | 77.79 |
BERT-Softmax | 81.43 | 85.34 | 83.34 |
BERT-BiLSTM-Softmax | 82.62 | 84.77 | 83.68 |
BERT-Transformer-Softmax | 83.54 | 85.23 | 84.38 |
Ours | 83.73 | 86.56 | 85.12 |
Category | Precision(%) | Recall(%) | F1-score(%) |
Problem | 87.42 | 90.16 | 88.77 |
Exam | 82.81 | 89.12 | 85.85 |
Treatment | 81.85 | 88.06 | 84.84 |
Clinical department | 74.72 | 62.44 | 68.03 |
Evidence | 76.13 | 86.40 | 80.94 |
Occurrence | 89.13 | 92.44 | 90.75 |
Aspectual | 75.96 | 86.87 | 81.05 |
Category | Precision(%) | Recall(%) | F1-score(%) |
Problem | 84.17 | 88.94 | 86.49 |
Exam | 80.42 | 90.12 | 84.99 |
Treatment | 81.92 | 88.34 | 85.01 |
Clinical department | 80.52 | 57.65 | 67.19 |
Evidence | 75.58 | 84.19 | 79.65 |
Occurrence | 90.21 | 90.39 | 90.30 |
Aspectual | 76.34 | 85.71 | 80.75 |
Model | Precision(%) | Recall(%) | F1-score(%) |
Ours(-contextual representation) | 82.34 | 84.07 | 83.20 |
Ours | 83.73 | 86.56 | 85.12 |
Model | Precision(%) | Recall(%) | F1-score(%) |
BERT-BiLSTM-Softmax(adversarial loss) | 82.75 | 86.35 | 84.51 |
Ours | 83.73 | 86.56 | 85.12 |
Model | Precision(%) | Recall(%) | F1-score(%) |
BiLSTM-CRF | 71.80 | 57.18 | 63.66 |
BiLSTM-CRF (adversarial loss) | 73.14 | 59.28 | 65.48 |
BERT-Softmax | 81.16 | 55.15 | 65.67 |
BERT-Softmax(adversarial loss) | 73.28 | 61.74 | 67.02 |
Ours (standard cross-entropy loss) | 80.52 | 57.65 | 67.19 |
Ours (fixed rate of loss allocation) | 70.25 | 57.34 | 63.14 |
Ours | 74.72 | 62.44 | 68.03 |
Sentence | 患 | 者 | 就 | 诊 | 于 | 神 | 经 | 外 | 科 |
Golden | O | O | O | O | O | B-Cd | M-Cd | M-Cd | E-Cd |
Baselines | O | O | O | O | O | O | O | O | O |
Ours | O | O | O | O | O | B-Cd | M-Cd | M-Cd | E-Cd |