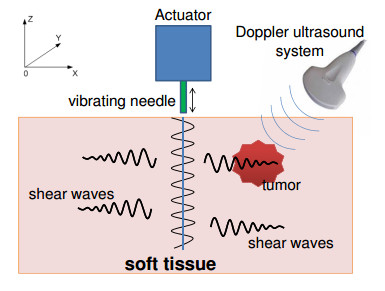
Citation: Thu-Ha Pham-Thi, Quang-Hai luong, Van-Dung Nguyen, Duc-Tan Tran, Huu-Tue Huynh. Two-dimensional complex shear modulus imaging of soft tissues by integration of Algebraic Helmoltz Inversion and LMS filter into dealing with noisy data: a simulation study[J]. Mathematical Biosciences and Engineering, 2020, 17(1): 404-417. doi: 10.3934/mbe.2020022
[1] | Tran Quang-Huy, Phuc Thinh Doan, Nguyen Thi Hoang Yen, Duc-Tan Tran . Shear wave imaging and classification using extended Kalman filter and decision tree algorithm. Mathematical Biosciences and Engineering, 2021, 18(6): 7631-7647. doi: 10.3934/mbe.2021378 |
[2] | Said G. Nassr, Amal S. Hassan, Rehab Alsultan, Ahmed R. El-Saeed . Acceptance sampling plans for the three-parameter inverted Topp–Leone model. Mathematical Biosciences and Engineering, 2022, 19(12): 13628-13659. doi: 10.3934/mbe.2022636 |
[3] | Christopher D. Bertram, Bernard O. Ikhimwin, Charlie Macaskill . Modeling flow in embryonic lymphatic vasculature: what is its role in valve development?. Mathematical Biosciences and Engineering, 2021, 18(2): 1406-1424. doi: 10.3934/mbe.2021073 |
[4] | H. Thomas Banks, Shuhua Hu, Zackary R. Kenz, Carola Kruse, Simon Shaw, John Whiteman, Mark P. Brewin, Stephen E. Greenwald, Malcolm J. Birch . Model validation for a noninvasive arterial stenosis detection problem. Mathematical Biosciences and Engineering, 2014, 11(3): 427-448. doi: 10.3934/mbe.2014.11.427 |
[5] | Ariel Cintrón-Arias, Carlos Castillo-Chávez, Luís M. A. Bettencourt, Alun L. Lloyd, H. T. Banks . The estimation of the effective reproductive number from disease outbreak data. Mathematical Biosciences and Engineering, 2009, 6(2): 261-282. doi: 10.3934/mbe.2009.6.261 |
[6] | H. Thomas Banks, Shuhua Hu, Zackary R. Kenz, Hien T. Tran . A comparison of nonlinear filtering approaches in the context of an HIV model. Mathematical Biosciences and Engineering, 2010, 7(2): 213-236. doi: 10.3934/mbe.2010.7.213 |
[7] | Fengjie Liu, Monan Wang, Yuzheng Ma . Multiscale modeling of skeletal muscle to explore its passive mechanical properties and experiments verification. Mathematical Biosciences and Engineering, 2022, 19(2): 1251-1279. doi: 10.3934/mbe.2022058 |
[8] | Fang Zhu, Wei Liu . A novel medical image fusion method based on multi-scale shearing rolling weighted guided image filter. Mathematical Biosciences and Engineering, 2023, 20(8): 15374-15406. doi: 10.3934/mbe.2023687 |
[9] | Conrad Bertrand Tabi, Alidou Mohamadou, Timoleon Crepin Kofane . Soliton-like excitation in a nonlinear model of DNA dynamics with viscosity. Mathematical Biosciences and Engineering, 2008, 5(1): 205-216. doi: 10.3934/mbe.2008.5.205 |
[10] | Alberto M. Gambaruto, João Janela, Alexandra Moura, Adélia Sequeira . Sensitivity of hemodynamics in a patient specific cerebral aneurysm to vascular geometry and blood rheology. Mathematical Biosciences and Engineering, 2011, 8(2): 409-423. doi: 10.3934/mbe.2011.8.409 |
Elasticity and viscosity are two tissue characteristic parameters; they can be used to detect tumors in the human body [1]. In the last few decades, a number of researchers have adopted a detection technique based on the tissue elasticity [2,3,4,5,6,7], namely the ultrasonic Shear Wave Elasticity Imaging (SWEI). SWEI offers the possibility to image the elasticity of tissues and organs that the traditional ultrasound cannot reach. Meanwhile, for a better understanding of the tissues, it would be beneficial to simultaneously look at these two parameters due to the fact that certain tissues have similar elasticity and different viscosity, for example, in Liver [1]. Many authors have developed different estimation techniques for both the elasticity and viscosity [7,8,9,10,11,12,13,14,15,16].
Ultrasound Imaging and other methods such as Mammography, Clinical Examination and MR Imaging can be used to diagnose the breast cancer [17]; among these, shear wave has recently been employed to estimate CSM. In practice, the vibration force used to generate shear wave is in the range 50−250 Hz. In [15], the authors conducted an experiment in which they estimated the elasticity and viscosity of a phantom sample. The true values of the elasticity and viscosity of a phantom sample were known. The results showed that the true and the estimated values of the elasticity and viscosity are quite closed at 150 Hz. In this paper, we propose using the same frequency of 150 Hz in order to compare our results to the results of this study. The relationship between the speed and absorption of shear wave is modeled by Greanleaf in [18]. In order to minimize the reflections, several authors proposed using pulse excitation [19]. However, the influence of noise was more adverse than using harmonic ones [20]. Another advantage of using harmonics is that a much larger amplitudes of shear wave can be obtained. For these reasons, we used harmonic excitation in our work.
In 2004, the shear wave speed dispersion was established by Chen et al. using the relationship between the propagation speed and the vibrating frequency; this speed dispersion was finally used to estimate the CSM [8]. In 2007, Zheng et al. used the same technique proposed by Chen but added a linear Kalman filter to minimize noise influence [20]. Recently, this method was used by several authors [8,10,15,20] where multiple datasets of distinct vibration frequencies must first be obtained. In this paper, only a single-frequency excitation was needed, which tremendously improved the acquisition time. Tran et al. [21] used the maximum likelihood ensemble filter for 1D to detect tumors (if any) in the tissues. However, the propagation model using wave equation in [10,14,21] is too simple to represent the actual propagation in heterogeneous tissues. In 2015, Qiuang et al. [22] proposed a technique using Finite Element Method (FEM) to better produce numerical solutions for the transversal shear wave propagation in any medium. However, FDTD is a more efficient method with lower memory usage than FEM [23]. In [12], Orescanin et al. combined the FDTD method with the AHI algorithm to estimate CSM. In this study, authors used a low-pass second-order Butterworth filter to reduce the noise impact.
In our previous study [24], we integrated Least Mean Square (LMS) with AHI algorithms to estimate 1D CSM. In fact, the 1D CSM estimation did not serve much purpose in the diagnosis. But the findings of this study confirmed that the noise reduction of acquired data before estimating CSM will produce better results. Thus, in this study, we proposed a method for 2D-CSM imaging of soft tissues based on the integration between AHI and Normalize Least Mean Square (NLMS) algorithms. The simulation scenarios were carried out in the MATLAB environment. An actuator was used to vibrate a needle at the frequency of 150 Hz. This needle acted on the surface of tissue, leading to the generation and propagation of shear wave in the tissues. A Doppler device was then used to measure the particle velocity of shear wave at 120×120 spatial locations. At each spatial location, the noise coming from the measured particle velocity was reduced noise using NLMS. After that, CSM was directly estimated by using the AHI algorithm. Finally, the 2D-CSM image was reconstructed. Our proposed method NLMS/AHI offers a very low complexity compared to the previous methods. A scenario with a tumor and noise environment was studied to evaluate the quality of the estimated CSM.
The measurement system consisted of a vibrating needle, an actuator, and a Doppler ultrasound device (see Figure 1). The actuator generated through a needle a mechanical vibration. Finally, we assumed that the Doppler ultrasound device was used to measure the shear wave at each spatial location in the region of interest [10]. In the later numerical simulation, the particle velocity which propagated in tissues was modeled by using FDTD model. This particle velocity, after adding noises, simulated the measured particle velocity (from the Doppler device). Thus the measured particle velocity was the noisy particle velocity.
In this paper, we estimated the elasticity μ and the viscosity η of the tissues, which were based on the Kelvin-Voigt model as follows
G(x,y,ω)=μ(x,y)−iωη(x,y), | (2.1) |
where ω is the angle frequency of the vibration signal, μ is the elasticity and η is the viscosity at the spatial position (x, y).
A system of six first-order hyperbolic equations was used to describe wave propagation in 2D space as shown in the following equations (2.2), (2.3), and (2.4) [12].
ρ∂tvz=∂xσzx+∂yσzy, | (2.2) |
∂tσzx=(μ+η∂t)∂xvz, | (2.3) |
∂tσzy=(μ+η∂t)∂yvz, | (2.4) |
where vz is the particle velocity vector on the plane (x, y), σzx and σzy are two elements of the stress tensor σ, ∂t denotes a partial derivative operator ∂/∂t which is applied to the parameters to the right of the symbol, ∂x denotes a partial derivative operator ∂/∂x applied to the parameters to the right of the symbol, ∂y denotes a partial derivative operator ∂/∂y applied to the parameters to the right of the symbol, ρ is the density of the tissues.
We discretized vz, σzx and σzy in Eqs (2.2), (2.3) and (2.4) to obtain the following equations
vz(x,y,t)=vz(iΔx,jΔy,nΔt)=vnz|i,j, | (2.5) |
σzx(x,y,t)=σzx(iΔx,jΔy,nΔt)=σnzx|i,j, | (2.6) |
σzy(x,y,t)=σzy(iΔx,jΔy,nΔt)=σnzy|i,j, | (2.7) |
where Δx and Δy are the distances between continuous spatial locations following the X-axis, and Y-axis, respectively; Δt is the sampling cycle, the index i denotes the spatial step following the X-axis, the index j denotes the spatial step following the Y-axis, and the index n denotes the temporal step, These parameters are illustrated in Figure 2.
By applying the FDTD method in 2D medium, Equations (2.2), (2.3) and (2.4) are transformed into Equations (2.8), (2.9) and (2.10), respectively.
vn+1z|i,j=vnz|i,j+ΔtρΔx(σn+12zx|i+12,j−σn+12zx|i−12,j) +ΔtρΔy(σn+12zy|i,j+12−σn+12zy|i,j−12), | (2.8) |
σn+12zx|i+12,j=σn−12zx|i+12,j+μΔtΔx(vn+1z|i+1,j−vn+1z|i,j) +ηΔx(vn+1z|i+1,j−vn+1z|i,j) −ηΔx(vnz|i+1,j−vnz|i,j), | (2.9) |
σn+12zy|i,j+12=σn−12zy|i,j+12+μΔtΔy(vn+1z|i,j+1−vn+1z|i,j) +ηΔy(vn+1z|i,j+1−vn+1z|i,j) −ηΔy(vnz|i,j+1−vnz|i,j). | (2.10) |
The accuracy of numerical Eqs (2.8), (2.9) and (2.10) is the first order in time and space. The boundary conditions were applied by using an absorption boundary layer. It will reduce the reflections. The layer is defined by a loss profile chosen within a perfectly matched absorptive layer and the attenuation factor τ which is given by [25]
τ(i)=τmax(i−ipmlNpml)m, | (2.11) |
where two constants are set as m=2.15 and τmax=0.11; the layer thickness in grid points Npml=10; and the term i−ipml indicates position within the perfectly matched absorptive layer.
FDTD and AHI can be used to directly estimate CSM at each point in the space (presented in detail in section 2.3). In such an approach, one important point is to minimize the propagation of error, which mean that the influence of measurement noise need to be reduced as much as before the direct estimation. In our work, we propose to use adaptive filtering [26] which was proved to give a mean error of 2.06%.
Figure 3 describes the LMS adaptive filter we used to eliminate noise from the noisy signal. The adaptive filtering algorithm is described in Algorithm 1. The noisy particle velocity generation can be described as follows: the Eqs (2.8), (2.9) and (2.10) were used to create the ideal particle velocity d(n) in each spatial location. This signal was added by a Gaussian white noise v(n) to form a noisy particle velocity vz(n). The level of noise which affected to the particle velocities at the different observed locations was identical. Thus, the signal to noise ratio (SNR) reduced from the locations near to the needle to the ones farthest away from the needle. In our simulation scenario, the average SNR of all the spatial locations was 10.1 dB. The reference noise generation can be described as follows: when the vibration needle stayed still, the measured signal was just the reference noise x(n) as indicated in Figure 3. Note that x(n) was the Gaussian white noise that we measured when the vibration needle stayed still, and v(n) was the Gaussian white noise that we measured when the vibration needle was active. In Figure 3, Var variable was the estimated variance of noise in vz(n). Both x(n) and vz(n) were applied to a filter in [27] (chapter 9, pages 505 to 515).
Algorithm 1: NLMS algorithm for signal improvement |
Step 1: Set up the parameters: step size β, filter order L, and noise variance.
Step 2: Start the simulation by assigning w(n)=0. Step 3: For n=0,1,2,…. 3.1. Calculate the output y(n) y(n)=w(n)∗x(n) 3.2. Calculate the error e(n) e(n) = vz(n) - y(n) 3.3. Update the filter's coefficient w(n+1) = w(n) + β e(n)x(n) 3.4. Extract the improved signal ˆvz(n) ˆvz(n) = e(n) 3.5. Change the value of β based on the amplitude of vz(n) |
In practice, the shear wave was quickly attenuated with the distance from the position of the vibration needle. Due to this fact, the Signal-to-Noise Ratio (SNR) varied with the space points where we would like to estimate the CSM. The optimal step size, giving a trade-off between the speed of convergence and residual error, depended on the SNR. Therefore, the commonly used modification of the LMS algorithm was a normalized version of the step size. This leads to the normalized LMS (NLMS) algorithm, in which the step size was scaled (divided) by an estimated power of the input data (i.e the variable Var as shown in Figure 3). This observation required that we use a different step size β. The complexity of this process suggested that we should use a simple structure for adaptive filtering with fast convergence [28].
The NLMS algorithm which was used in this study is shown in Algorithm 1 below. We adjusted the step size at each spatial location using the formula β=β1(ε+Var), where β1 and ε are constant, and Var is the variance of vz(n) which was estimated at each spatial location as shown in Figure 3.
The filtered particle velocity was brought to the AHI algorithm [29] to directly estimate the CSM. We assumed that the viscoelastic properties of the medium were isotropic and there was negligible compression applied to the medium by the source. Thus, the particle velocity vector vz can be described by the Navier wave equation in a homogeneous solid [12]. By combining (2.2), (2.3) and (2.4):
ρ∂2vz∂t2=G′(x,y,t)∇2vz, | (2.12) |
where G′(x,y,t) is the CSM in the time domain and ∇2vz is the Laplace operator of vz which is defined as ∇2vz=∂2vz/∂x2.
AHI algorithm was then used to solve (2.12), which then converted to the Helmholtz equation
(G(x,y,ω)ρ∇2+ω2)Vz(x,y,ω)|ω=ω0=0, | (2.13) |
where G(x,y,ω) is the CSM in the frequency domain and is defined in (2.1), Vz(x,y,ω) is the temporal Fourier transform of the particle velocity vz(x,y,t), Vz(x,y,ω)=Ft{vz(x,y,t)}, and ω0 is the angular frequency ω0=2πf0. Solving (2.13), we could directly estimate CSM
G(x,y,ω)=−ρω20Vz(x,y,ω0)∇2Vz(x,y,ω0), | (2.14) |
Following (2.1), μ(x,y) and η(x,y) were real and imaginary parts of G(x,y,ω). Thus, elasticity and viscosity of the tissues at each spatial point (x,y) were calculated as
μ(x,y)=ℜ{−ρω20Vz(x,y,ω0)∇2Vz(x,y,ω0)},η(x,y)=ℑ{−ρω0Vz(x,y,ω0)∇2Vz(x,y,ω0)}, | (2.15) |
where Vz(x,y,ω0) was computed by using Fourier transform at the specific angular frequency ω0; ∇2Vz(x,y,ω0) was computed by using the function Discrete Laplacian (The MathWorks) del2(Vz(x,y,ω0)) which provided a discrete approximation of Laplace's differential operator applied to Vz(x,y,ω0).
Our proposed NLMS/AHI algorithm is summarized in Algorithm 2.
Algorithm 2: NLMS/AHI algorithm for 2D-CSM imaging |
Step 1: Initiate the parameters for simulation.
Step 2: Vibrate the needle at a frequency of f0=150Hz. Step 3: Propagate the shear wave into the tissues. Step 4: Use the Doppler ultrasound device to measure vz at 120×120 spatial locations. Step 5: Extract the variance of noise and forward to the NLMS filter. Step 6: Employ NLMS filter (as shown in Algorithm 1) in order to improve the SNR. Step 7: Compute the temporal Fourier transform of the filtered signal. Step 8: Directly estimate CSM in each spatial location using (2.15). Step 9: Reconstruct the 2D-CSM image. |
The researchers set up a simulation scenario to test the proposed method. Details are as follows: The 2D medium had a size of 120×120mm, which contained a tumor at the coordinate (40mm,40mm), the radius of the tumor was 20mm. The elasticity of the medium was μ1=6000Pa and the viscosity of the medium was η=1.2Pa.s, The elasticity and viscosity of the tumor were μ1=9000Pa and η=1.8Pa.s. The parameters of the medium and tumor are summarized in Table 1.
Coordinate (mm, mm) |
Radius (mm) |
μ1 (Pa) | η (Pa.s) | |
Medium | 6000 | 1.2 | ||
Tumor | (40, 40) | 20 | 9000 | 1.8 |
The mass density of the medium was ρ=1000Kg/m3. On the 2D plane of the medium (tissue), the particle velocity of shear wave was measured at locations which were equally spaced of 1mm following both the X–axis and Y–axis. Thus, the number of measured locations was 120×120=14400. Figure 4 illustrates the ideal–elasticity and ideal–viscosity images.
The ideal particle velocity at a location was modeled following the FDTD method (as shown in Figure 5 at the spatial location (56, 56)). Combining with the Gaussian noise, it presented the measured particle velocity using the Doppler system.
Figure 6 indicates two signals of the particle velocity of the shear wave at the coordinate (56, 56). where the dotted–blue signal shows the measured particle velocity (affected by noise), while the continuous–red signal shows the filtered particle velocity.
Figures 7(a) and 7(b) show estimated–elasticity and estimated–viscosity images of the tissues, respectively. In both images, tumor is seen clearly in in terms of location and shape.
Observing 1D–data is easy to compare between the estimated and ideal values. Figure 8 illustrates results of elasticity estimation on Line 10 and Line 40. In detail, Figure 8(b) indicates the result of elasticity estimation on Line 10 which does not across tumor, Figure 8(c) shows the result of elasticity estimation on Line 40 which crosses tumor.
Figure 9 illustrates the results of viscosity estimation on Line 10 and Line 40. In detail, Figure 9(b) indicates the result of viscosity estimation on Line 10, Figure 9(c) shows the result of viscosity estimation on Line 40.
Observe Figures 8(b), 8(c), 9(b) and 9(c), it is clearly seen that the results of estimation were less accurate at locations which were far from excited source (the right of figures). This can explain as follows as shear wave was attenuated when it propagated in the tissues and it was a damped oscillation. While CSM was estimated base on measurement of velocity of shear wave. At locations which were far from excited source, velocity value was very small, thus CSM estimation was less accurate.
To evaluate the quality of 2D CSM estimation, the normalized error was used. This norm which was applied to elasticity and viscosity images was calculated using the following equation (3.1).
ϵμ=1M×N∑Mi=1∑Nj=1|μi,j−ˆμi,j|μi,j,ϵη=1M×N∑Mi=1∑Nj=1|ηi,j−ˆηi,j|ηi,j, | (3.1) |
where ϵμ and ϵη are the normalized errors of 2D elasticity and viscosity estimation, respectively, M×N is the size of the images, μi,j and ˆμi,j are the ideal and estimated elasticity at the pixel (i,j), respectively, ηi,j and ˆηi,j are the ideal and estimated viscosity at the pixel (i,j), respectively.
The calculated results show that the normalized error ϵμ for 2D elasticity estimation was 1.63%, while the normalized error ϵη for 2D viscosity estimation was 2.49%.
It is noteworthy that the estimated images contain many interesting features. In Figures 7(a) and 7(b), near the vibration needle, the reconstructed image is of very high quality, i.e. the reconstructed image looks like the original one (used in the simulation scenario). The area where the estimation gives good result is the circle of radius of 120 mm centered around the needle position. At spatial locations outside of this area, the estimation is not accurate because of the high attenuation of the particle velocity. Combining different estimates of CSM with the varying positions of the vibration needle is a good subject for future research.
The propagation of noise in a scheme such as AHI and FDTD is a serious problem. Our research showed that by filtering noise with NLMS, the situation was nicely ameliorated and the estimation estimation error was reduced to 1.63% for elasticity and 2.49% for viscosity. In the existing research works, for instance [12], the noise impact was reduced by applying a low-pass filter. With the same simulation condition, in our study, the normalized error for 2D elasticity estimation was 4.15%, and for 2D viscosity estimation was 6.24%. The reason is that, the low pass filter used in [12] could only remove the high frequency noise.
While there are significant findings reported in our study, the study itself suffers from certain limitations. Firstly, due to the variation of SNR in the measurement at each point in the space, if the step size of the adaptive filtering is kept fixed, the efficiency of the filter would be reduced. In our work, this parameter was changed depending on the position of the needle. Secondly, certain sets of data obtained from the measurement need to be eliminated. In fact, to estimate CSM using AHI, it requires that the Fourier transform Vz(x,y,ω0) be at the frequency ω0. The transient in the measurement process could badly affect the estimation of Vz(x,y,ω0); consequently, the transient has to be eliminated before we estimate Vz(x,y,ω0) (see step 7, Algorithm 2).
In addition, in the shear wave propagation model, we assumed that the reflections and the refractions from the surrounding tissues are trivial, thus we ignored them. Finally, this research is only concerned with numerical simulation. The estimation using experimental data is our next target.
This paper presented a method of constructing 2D CSM images using the estimation method AHI in a noisy environment. In our method, we just measured the particle velocity at only one frequency (150 Hz) at different positions in the focused area. Noise in the measurement of particle velocity was eliminated by using a NLMS adaptive filter in order to improve the image reconstruction. The quality of image reconstruction was improved by (1) Changing the adaptive filter step size at each point of the observation space and (2) Eliminating the transient data before computing the Fourier transform of the particle velocity at the frequency 150 Hz; this helped to improve the estimation of CSM using Eq (2.15). These estimates computed at each point in the space as illustrated in Figure 2. were finally used to reconstruct the CSM images. Our proposed method delivered a good performance by reducing the estimation error to 1.63% for elasticity and to 2.49% for viscosity. The next steps in our future research will be applying our proposed method to real data and extending it to 3D CSM imaging.
The authors would like to sincerely thank Professor Michael F. Insana from the University of Illinois at Urbana–Champaign for introducing us to shear wave imaging. This work was supported by the Asia Research Center (ARC), Vietnam National University, code CA.17.6A.
The authors declare there is no conflicts of interest.
[1] | J. Bercoff, A. Criton, C. Bacrie, et al., ShearWave Elastography A new real time imaging mode for assessing quantitatively soft tissue viscoelasticity, in Ultrason. Symposium, 2008, IEEE, 2008, 321-324. |
[2] | A. P. Sarvazyan, O. V. Rudenko, S. D. Swanson, et al., Shear wave elasticity imaging: a new ultrasonic technology of medical diagnostics, Ultrasound Med. Biol., 24 (1998), 1419-1435. |
[3] | J.-L. Gennisson, T. Deffieux, M. Fink, et al., Ultrasound elastography: principles and techniques, Diagn. Interv. Imag., 94 (2013), 487-495. |
[4] | G. Ferraioli, P. Parekh, A. B. Levitov, et al., Shear wave elastography for evaluation of liver fibrosis, J. Ultrasound Med., 33 (2014), 197-203. |
[5] | Y. Kobayashi, M. Tsukune, T. Miyashita, et al., Simple empirical model for identifying rheological properties of soft biological tissues, Phys. Rev., 95. |
[6] | S. Woo, S. Y. Kim, M. S. Lee, et al., Shear wave elastography assessment in the prostate: an intraobserver reproducibility study, Clin. Imag., 39 (2015), 484-487. |
[7] | W. Zhang and S. Holm, Estimation of shear modulus in media with power law characteristics, Ultrasonics, 64 (2016), 170-176. |
[8] | S. Chen, M. Fatemi and J. F. Greenleaf, Quantifying elasticity and viscosity from measurement of shear wave speed dispersion, J. Acoust. Soc. Am., 115 (2004), 2781-2785. |
[9] | D. Liu and E. S. Ebbini, Viscoelastic property measurement in thin tissue constructs using ultrasound, IEEE T. Ultrason. Ferr., 55 (2008), 368-383. |
[10] | M. Orescanin and M. F. Insana, Model-based complex shear modulus reconstruction: A Bayesian approach, in Ultrason. Symposium., IEEE, 2010, 61-64. |
[11] | M. Orescanin and M. F. Insana, Shear modulus estimation with vibrating needle stimulation, IEEE T. Ultrason. Ferr., 57 (2010), 1358-1367. |
[12] | M. Orescanin, Y. Wang and M. F. Insana, 3D fdtd simulation of shear waves for evaluation of complex modulus imaging, IEEE T. Ultrason. Ferr., 58 (2011), 389-398. |
[13] | C. T. Barry, B. Mills, Z. Hah, et al., Shear wave dispersion measures liver steatosis, Ultrasound Med. Biol., 38 (2012), 175-182. |
[14] | N. T. Hao, T. Thuy-Nga, V. Dinh-Long, et al., 2D Shear Wave Imaging Using Maximum Likelihood Ensemble Filter, in International Conference on Green and Human Information Technology (ICGHIT), 2013, 88-94. |
[15] | Y. Wang and M. F. Insana, Viscoelastic properties of rodent mammary tumors using ultrasonic shear-wave imaging, Ultrason. Imag., 35 (2013), 126-145. |
[16] | Q. Wang, Y. Shi, F. Yang, et al., Quantitative photoacoustic elasticity and viscosity imaging for cirrhosis detection, Appl. Phys. Lett., 112 (2018), 211902. |
[17] | W. A. Berg, L. Gutierrez, M. S. NessAiver, et al., Diagnostic accuracy of mammography, clinical examination, us, and mr imaging in preoperative assessment of breast cancer, Radiology, 233 (2004), 830-849. |
[18] | J. F. Greenleaf, M. Fatemi and M. Insana, Selected methods for imaging elastic properties of biological tissues, Ann. Rev. Biomed. Eng., 5 (2003), 57-78. |
[19] | L. Sandrin, B. Fourquet, J.-M. Hasquenoph, et al., Transient elastography: a new noninvasive method for assessment of hepatic fibrosis, Ultrasound Med. Biol., 29 (2003), 1705-1713. |
[20] | Y. Zheng, S. Chen, W. Tan, et al., Detection of tissue harmonic motion induced by ultrasonic radiation force using pulse-echo ultrasound and kalman filter, IEEE T. Ultrason. Ferr., 54 (2007), 290-300. |
[21] | T. Tran-Duc, Y. Wang, N. Linh-Trung, et al., Complex Shear Modulus Estimation Using Maximum Likelihood Ensemble Filters, in 4th International Conference on Biomedical Engineering in Vietnam, Springer Berlin Heidelberg, 2013, 313-316. |
[22] | B. Qiang, J. Brigham, S. Aristizabal, et al., Modeling transversely isotropic, viscoelastic, incompressible tissue-like materials with application in ultrasound shear wave elastography, Phys. Med. Biol., 3 (2015), 1289-1306. |
[23] | F. L. Teixeira, Time-domain finite-difference and finite-element methods for maxwell equations in complex media, IEEE T. Antenn. Propag., 56 (2008), 2150-2166. |
[24] | H. Luong Quang, C. Nguyen Manh, L. Ton That, et al., Complex shear modulus estimation using integration of lms/ahi algorithm, International Journal of Advanced Computer Science and Applications (IJACSA), 9 (2018), 584-589. |
[25] | C. T. SCHRODER and W. R. SCOTT, A finite-difference model to study the elastic-wave interactions with buried land mines, IEEE T. Geosci. Remote, 38.4 (2000), 1505-1512. |
[26] | S. Haykin and B. Widrow, Least-mean-square adaptive filters, vol. 31, John Wiley & Sons, 2003. |
[27] | M. H. Hayes, Statistical digital signal processing and modeling, John Wiley & Sons, 2009. |
[28] | D. Bismor, Lms algorithm step size adjustment for fast convergence, Arch. Acoust., 37 (2012), 31-40. |
[29] | S. Papazoglou, U. Hamhaber, J. Braun, et al., Algebraic helmholtz inversion in planar magnetic resonance elastography, Phys. Med. Biol., 53 (2008), 3147. |
1. | Tran Quang-Huy, Van Tu Duong, Luong Quang Hai, Tran Duc-Tan, 2020, Image Reconstruction Utilizing Algebraic Helmoltz Inversion and Passband Filtering Applied to Viscoelasticity, 978-1-7281-6555-4, 1, 10.1109/MAPR49794.2020.9237774 | |
2. | Tran Quang-Huy, Nguyen Thi Hoang Yen, Truong Giang Le, Duc-Tan Tran, Two-dimensional viscoelastic imaging using an enhanced FDTD-AHI approach, 2021, 2446-4732, 10.1007/s42600-021-00130-x | |
3. | Tran Quang-Huy, Phuc Thinh Doan, Nguyen Thi Hoang Yen, Duc-Tan Tran, Shear wave imaging and classification using extended Kalman filter and decision tree algorithm, 2021, 18, 1551-0018, 7631, 10.3934/mbe.2021378 |
Algorithm 1: NLMS algorithm for signal improvement |
Step 1: Set up the parameters: step size β, filter order L, and noise variance.
Step 2: Start the simulation by assigning w(n)=0. Step 3: For n=0,1,2,…. 3.1. Calculate the output y(n) y(n)=w(n)∗x(n) 3.2. Calculate the error e(n) e(n) = vz(n) - y(n) 3.3. Update the filter's coefficient w(n+1) = w(n) + β e(n)x(n) 3.4. Extract the improved signal ˆvz(n) ˆvz(n) = e(n) 3.5. Change the value of β based on the amplitude of vz(n) |
Algorithm 2: NLMS/AHI algorithm for 2D-CSM imaging |
Step 1: Initiate the parameters for simulation.
Step 2: Vibrate the needle at a frequency of f0=150Hz. Step 3: Propagate the shear wave into the tissues. Step 4: Use the Doppler ultrasound device to measure vz at 120×120 spatial locations. Step 5: Extract the variance of noise and forward to the NLMS filter. Step 6: Employ NLMS filter (as shown in Algorithm 1) in order to improve the SNR. Step 7: Compute the temporal Fourier transform of the filtered signal. Step 8: Directly estimate CSM in each spatial location using (2.15). Step 9: Reconstruct the 2D-CSM image. |
Coordinate (mm, mm) |
Radius (mm) |
μ1 (Pa) | η (Pa.s) | |
Medium | 6000 | 1.2 | ||
Tumor | (40, 40) | 20 | 9000 | 1.8 |
Algorithm 1: NLMS algorithm for signal improvement |
Step 1: Set up the parameters: step size β, filter order L, and noise variance.
Step 2: Start the simulation by assigning w(n)=0. Step 3: For n=0,1,2,…. 3.1. Calculate the output y(n) y(n)=w(n)∗x(n) 3.2. Calculate the error e(n) e(n) = vz(n) - y(n) 3.3. Update the filter's coefficient w(n+1) = w(n) + β e(n)x(n) 3.4. Extract the improved signal ˆvz(n) ˆvz(n) = e(n) 3.5. Change the value of β based on the amplitude of vz(n) |
Algorithm 2: NLMS/AHI algorithm for 2D-CSM imaging |
Step 1: Initiate the parameters for simulation.
Step 2: Vibrate the needle at a frequency of f0=150Hz. Step 3: Propagate the shear wave into the tissues. Step 4: Use the Doppler ultrasound device to measure vz at 120×120 spatial locations. Step 5: Extract the variance of noise and forward to the NLMS filter. Step 6: Employ NLMS filter (as shown in Algorithm 1) in order to improve the SNR. Step 7: Compute the temporal Fourier transform of the filtered signal. Step 8: Directly estimate CSM in each spatial location using (2.15). Step 9: Reconstruct the 2D-CSM image. |
Coordinate (mm, mm) |
Radius (mm) |
μ1 (Pa) | η (Pa.s) | |
Medium | 6000 | 1.2 | ||
Tumor | (40, 40) | 20 | 9000 | 1.8 |