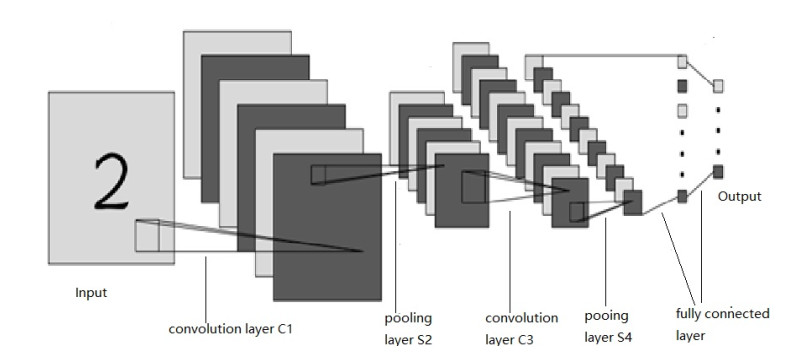
Citation: Chunmei He, Hongyu Kang, Tong Yao, Xiaorui Li. An effective classifier based on convolutional neural network and regularized extreme learning machine[J]. Mathematical Biosciences and Engineering, 2019, 16(6): 8309-8321. doi: 10.3934/mbe.2019420
[1] | Yufeng Li, Chengcheng Liu, Weiping Zhao, Yufeng Huang . Multi-spectral remote sensing images feature coverage classification based on improved convolutional neural network. Mathematical Biosciences and Engineering, 2020, 17(5): 4443-4456. doi: 10.3934/mbe.2020245 |
[2] | Ting Yao, Farong Gao, Qizhong Zhang, Yuliang Ma . Multi-feature gait recognition with DNN based on sEMG signals. Mathematical Biosciences and Engineering, 2021, 18(4): 3521-3542. doi: 10.3934/mbe.2021177 |
[3] | Tao Zhang, Hao Zhang, Ran Wang, Yunda Wu . A new JPEG image steganalysis technique combining rich model features and convolutional neural networks. Mathematical Biosciences and Engineering, 2019, 16(5): 4069-4081. doi: 10.3934/mbe.2019201 |
[4] | Zhangjie Wu, Minming Gu . A novel attention-guided ECA-CNN architecture for sEMG-based gait classification. Mathematical Biosciences and Engineering, 2023, 20(4): 7140-7153. doi: 10.3934/mbe.2023308 |
[5] | Xianli Liu, Yongquan Zhou, Weiping Meng, Qifang Luo . Functional extreme learning machine for regression and classification. Mathematical Biosciences and Engineering, 2023, 20(2): 3768-3792. doi: 10.3934/mbe.2023177 |
[6] | Sakorn Mekruksavanich, Wikanda Phaphan, Anuchit Jitpattanakul . Epileptic seizure detection in EEG signals via an enhanced hybrid CNN with an integrated attention mechanism. Mathematical Biosciences and Engineering, 2025, 22(1): 73-105. doi: 10.3934/mbe.2025004 |
[7] | Jia-Gang Qiu, Yi Li, Hao-Qi Liu, Shuang Lin, Lei Pang, Gang Sun, Ying-Zhe Song . Research on motion recognition based on multi-dimensional sensing data and deep learning algorithms. Mathematical Biosciences and Engineering, 2023, 20(8): 14578-14595. doi: 10.3934/mbe.2023652 |
[8] | Zhigao Zeng, Cheng Huang, Wenqiu Zhu, Zhiqiang Wen, Xinpan Yuan . Flower image classification based on an improved lightweight neural network with multi-scale feature fusion and attention mechanism. Mathematical Biosciences and Engineering, 2023, 20(8): 13900-13920. doi: 10.3934/mbe.2023619 |
[9] | Haifeng Song, Weiwei Yang, Songsong Dai, Haiyan Yuan . Multi-source remote sensing image classification based on two-channel densely connected convolutional networks. Mathematical Biosciences and Engineering, 2020, 17(6): 7353-7377. doi: 10.3934/mbe.2020376 |
[10] | Xiaoguang Liu, Yubo Wu, Meng Chen, Tie Liang, Fei Han, Xiuling Liu . A double-channel multiscale depthwise separable convolutional neural network for abnormal gait recognition. Mathematical Biosciences and Engineering, 2023, 20(5): 8049-8067. doi: 10.3934/mbe.2023349 |
Deep learning becomes one of the most important technologies in the field of artificial intelligence [1,2,3]. CNN is the most representative model of deep learning [4]. In 1989, Yann LeCun [5] proposes CNN model and applies CNN to handwritten character recognition. Yoshua Bengio presents the Probabilistic models of sequences and proposes generative adversarial networks [6]. Geoffrey Hinton et al. present a deep brief net [7] and apply CNN in Image Net game. The advantages of CNN against traditional methods can be summarized as follows [4].
(1) Hierarchical feature representation.
(2) Compared with traditional shallow models, a deeper architecture provides an exponentially increased expressive capability.
(3) The architecture of CNN provides an opportunity to optimize several related tasks together.
(4) Benefiting from the large learning capacity of CNNs, some classical computer vision challenges can be recast as high-dimensional data transform problems and solved from a different viewpoint.
Due to these advantages, CNN has been widely applied into many research fields [8,9,10,11,12,13,14]. However, the CNN has some disadvantages. Many Parameters need to be adjusted in CNN. It needs many training samples and the GPU is preferred for training CNN.
Extreme learning machine (ELM) [15] is a kind of feed-forward neural network in essence. ELM generates input layer weights and bias values at random, directly solves the least square solution of the output weights, and obtains the final training model at the same time. ELM has the advantages such as no iterative calculation, fast learning speed and strong generalization ability. Many scholars pay close attention to ELM and have achieved good results [16,17,18,19,20]. A fast kernel ELM combining the conjugate gradient method (CG-KELM) is presented in [16] and the kernel ELM is applied in image restoration. In [17], the paper further studies ELM for classification in the aspect of the standard optimization method and extends ELM to a specific type of "generalized" single-hidden layer feedforward networks-support vector network. An improved meta-learning model of ELM is proposed in [18]. Chunmei He et al. [19] propose a fast learning algorithm for the regular fuzzy neural network based on ELM which has good performance and approximation ability. Since ELM is based on the principle of empirical risk minimization which may lead to over-fitting problem. In [20], the scholars combine the structural risk minimization theory and weighted least square method and present RELM. RELM considers both the structural risk and the empirical risk and balances the two risks using the risk ratio parameter. RELM is widely used in the fields of classification, regression and prediction. RELM has the advantage of fast learning speed and further improves the generalization performance of ELM.
Motivated by the remarkable success of CNN and RELM, RELM is introduced into CNN and an effective classifier CNN-RELM is proposed in this paper. In CNN-RELM, CNN can extract the deep feature of the input, while RELM have fast learning speed and good generalized ability to acquire better recognized accuracy. The rest paper is organized as follows. Section 2 introduces the basic theory of CNN, ELM and RELM. The CNN-RELM model and learning algorithm are presented in section 3. The experiment simulations show the excellent performance of the CNN-RELM in section 4. Section 5 summarizes the study and discusses the future work.
A typical CNN is simply introduced here. The CNN topological model is shown in Figure 1.
In the CNN, the convolution layer and pooling layer extract the features and input into the fully connected layer to obtain the classification results. The feature extraction process is as follows.
In the convolution layer, convolution is performed on the input, and the output is
xlj=f(∑i∈Mjxl−1i∗wlij+blj) | (1) |
where
The gradient of the convolution layer is defined by
The output of the pooling layer is
xlj=f(βljdown(xl−1i)+blj) | (2) |
where
In the fully connected layer, the output of the l-th layer is
We simply introduce ELM and RELM. For more details, please refer to [15,20]. The topology of ELM is shown in Figure 2.
Different from other feed-forward neural networks, the input weight
‖H⋅ˆβ−Y‖=minβ‖H⋅β−Y‖ | (3) |
where
H(w1,⋯,wl,b1,⋯,bl,x1,⋯,xn)=[g(w1⋅x1+b1)⋯g(wl⋅x1+bl)⋮⋯⋮g(w1⋅xn+b1)⋯g(wl⋅xn+bl)]l×n. | (4) |
The solution of Eq (3) is the least normal square solution as follows.
ˆβ=H+Y, | (5) |
where
ELM only considers the empirical risk and doesn't consider the structural risk. ELM directly calculates the least squares solution, and users can't make fine-tuning according to the characteristics of the database, these results in poor control ability and over-fitting problems. Therefore, the structural risk minimization theory is introduced into ELM, and RELM is proposed [20]. The RELM model is as follows. When
argβminE(W)=argβminE(0.5‖β‖2+0.5γ‖ε‖2),s.t.∑li=1βig(wi⋅xj+bi)−yj=εj,j=1,⋯,n. | (6) |
where
ℓ(β,ε,α)=γ2‖ε‖2+12‖β‖2−∑nj=1αj(g(wi⋅xj+bj)−yj−εj)=γ2‖ε‖2+12‖β‖2−α(Hβ−Y−ε) | (7) |
where
β=(Iγ+HTH)+HTY. | (8) |
In this section, the CNN-RELM model and learning algorithm are presented. In CNN-RELM, the convolutional hidden layer and the pooling layer in CNN extract deep features from the original input and then RELM are used for feature classification. The CNN-RELM model is changed in the two steps of training period. And in testing period, there is no fully connected layer in CNN which is replaced by the RELM.A trained CNN-RELM used for testing is as in Figure 3. The training of CNN-RELM model is divided into two steps: The training of CNN and the merger of CNN and RELM. These two steps are presented in detail as follows.
In this step, the CNN model is trained. The CNN model in this step is as in Figure 4. The related parameters in CNN are adjusted by the gradient descent method according to the errors between the actual output and the expected output. The training of the CNN stops if the minimum error reaches or the maximum number of iterations reaches. Then the CNN is saved for the next step.
The feature map in the CNN is adjusted as follows.
(a) If the
xlj=f(∑xm−1i∈Mnxm−1i∗kmin+bmn) | (9) |
where
(b) If the
xmn=f(wmndown(xm−1n)+bmn) | (10) |
where
In this step, we firstly acquire the RELM which is optimized by genetic algorithm. And then the convolutional layers and pooling layers of the CNN is fixed while the full-connected layer of the CNN is replaced by the RELM. The topology of RELM is also shown as in Figure 3. The optimal risk ratio parameters
The learning algorithm of CNN-RELM is outlined in Algorithm 1. The learning algorithm flow chart of CNN-RELM is shown as in Figure 5.
Algorithm 1
Step 1. Initialize the CNN parameters, expected target and maximum iteration times.
Step 2. Compute the actual output of the network by Eqs (9), (10).
Step 3. If the predetermined target precision reaches or the maximum number of iterations reaches, then go to Step 5, else go to Step 4.
Step 4. Adjust the parameters of CNN by the gradient descent method and go to Step 2.
Step 5. Initialize RELM. Randomly initialize the weights and biases. Get the regularized parameters by GA.
Step 6. Let the feature vectors obtained by CNN into RELM and Compute
Step 7. Save the CNN-RELM and classify by the CNN-RELM.
In this section, we present the face recognition experiment to verify the feasibility of CNN-RELM and compare it with RELM and CNN. The impacts of different pooling methods and different number of training samples on the performance of CNN-RELM are also presented.
Two face databases: ORL and NUST are used in the simulations. The detailed database information is shown in Table 1. The ORL face database is founded by the Olivetti research laboratory in Cambridge, England. The ORL face database can be downloaded in the following website: http://www.cl.cam.ac.uk/research/dtg/attarchive/facedatabase.html. The NUST face database is made by Nanjing University of Science and Technology, China.
databases | classes | features | number of samples | remarks |
ORL | 40 | 10 | 400 | These include changes in facial expressions, minor changes in posture, and changes in scale within 20%. |
NUST | 96 | 10 | 216 | It mainly includes the change of face's different pose. |
The experimental execution environment is as follows. Software environment: Matlab-R2014a. Operation system: Windows 8. Hardware environment: Dell PC. CPU: Intel(R) Core(TM) i5-6500 CPU @ 3.20GHz. Hard disk: Seagate ST500DM002-1SB10A (500 GB /7200 rpm). Memory: 32 GB (Samsung DDR4 2666MHz).
To evaluate the performance of CNN-RELM, some simulations on the ORL face databases are given. In training of the CNN, there are two convolution layers:
After the CNN is trained, the fully connected layer is replaced by RELM for classification. The feature images obtained by the pooling layer
In this section, we compare the performances of CNN-RELM, RELM and CNN in face recognition experiments. The parameters in the CNN and the risk ratio parameter of RELM are similar to that in [21]. Two standard face databases: ORL and NUST as in Table 1 are used in the comparative experiments. In the experiment, we select seven images of each person as training samples, and the remaining three images as test samples. All hidden layer node number of neural networks is set to 200. The recognition results of the three algorithms are shown in Table 2.
databases | methods | division of sample sets | classification accuracy (%) | |
training samples | test samples | |||
ORL | RELM CNN CNN-RELM |
280 280 280 |
120 120 120 |
91.67 90.00 96.67 |
NUST | RELM CNN CNN-RELM |
672 672 672 |
288 288 288 |
89.58 88.54 96.88 |
As seen from Table 2, the CNN-RELM model proposed in this paper has the best recognition accuracy. In next section, an experiment is given to study the influence of different pooling methods and number of the training sample on CNN-RELM.
In order to evaluate how different pooling methods and different training samples impact on the performance of the CNN-RELM, the experiment is conducted in this section. The ORL face database is selected here. The parameters of the CNN-RELM model are selected as the same as those in section 4.2. The pooling method respectively uses Max-pooling, Average-pooling, Stochastic-pooling and Lp-pooling. Figures 4–7 are selected for each class's training samples and the rest pictures are taken as test samples. The experimental results are shown in Table 3 and Figure 8.
methods | training samples | |||
4 | 5 | 6 | 7 | |
Max-pooling | 83.75 | 88.00 | 94.37 | 96.67 |
Average-pooling | 84.17 | 92.00 | 96.25 | 97.50 |
Stochastic-pooling | 83.75 | 89.50 | 95.00 | 96.67 |
Lp-pooling | 84.58 | 92.50 | 96.88 | 98.33 |
By the experiment in Figures 6 and 7, it's known that the CNN-RELM is feasible in classification. From Table 2, we can concluded that the CNN-RELM outperform CNN and RELM in classification. The CNN-RELM model combines CNN with RELM and overcomes the deficiency of the two models. The CNN-RELM can also be used in other application tasks such as remote sensing and object shape reconstruction and the process is similar to that of classification.
From Table 3 and Figure 8, we can see that the recognition rate increase at the same pooling strategy as the selected training samples increase. When the same number of training samples is selected, the
Seen from Figure 8, the selection of different pooling methods has an impact on the performance of CNN-RELM. In practical applications, the selection of appropriate pooling methods according to the actual situation of data is conducive to achieving better application results.
An effective classifier CNN-RELM is proposed in this paper. Firstly, the CNN-RELM trains the convolutional neural network using the gradient descent method until the learning target accuracy reaches. Then the fully connected layer of CNN is replaced by RELM optimized by genetic algorithm and the rest layers of the CNN remain unchanged. A series of experiments conducted on ORL and NUST databases show that the CNN-RELM outperforms CNN and RELM in classification and demonstrate the efficiency and accuracy of the proposed CNN-RELM model. Meanwhile, we also verify that the selection of different pooling methods has an impact on the performance of CNN-RELM. When the same number of training samples is selected, the pooling strategy has the highest recognition rate. In practical applications, the selection of appropriate pooling methods according to the actual situation of data is conducive to achieving better application results. Due to the uniting of CNN and RELM, CNN-RELM have the advantages of CNN and RELM and it is easier to learn and faster in testing. The future work includes improve the generalized ability and further reduce the training time.
This work is supported by the National Natural Science Foundation of China (Grant No. 61402227), the Natural Science Foundation of Hunan Province (No.2019JJ50618) and the project of Xiangtan University (Grant No. 11kz/kz08055). This work is also supported by the key discipline of computer science and technology in Hunan province, China.
The authors declare there is no conflict of interest.
[1] | Y. Bengio, Learning deep architectures for AI, Found. Trends Mach. Learn., 1 (2009), 1-71. |
[2] | F. Schroff, D. Kalenichenko and J. Philbin, FaceNet: A Unified Embedding for Face Recognition and Clustering, 2015 IEEE Conference on Computer Vision and Pattern Recognition (CVPR), United States of America, 2015, 815-823. Available from: https://arxiv.org/pdf/1503.03832.pdf. |
[3] | G. Huang, Z. Liu, L. van der Maaten, et al., Densely Connected Convolutional Networks, 2017 IEEE Conference on Computer Vision and Pattern Recognition (CVPR), United States of America, 2017, 4700-4708. Available from: https://arxiv.org/abs/1608.06993. |
[4] | Z. Q. Zhao, P. Zheng, S. T. Xu, et al., Object Detection with Deep Learning: A Review, IEEE Trans. Neural Networks Learn. Syst., (2019), 1-21. |
[5] | Y. L. Cun, L. D. Jackel, B. Boser, et al., Handwritten digit recognition: Applications of neural network chips and automatic learning, IEEE Commun. Mag., 11 (1989), 41-46. |
[6] | I. J. Goodfellow, J. P. Abadie, M. Mirza, et al., Generative Adversarial Networks, Advances in neural information processing systems, 2014, 2672-2680. Available from: https://arxiv.org/abs/1406.2661. |
[7] | G. E. Hinton, S. Osindero and Y. W. Teh, A fast learning algorithm for deep belief nets, Neural Comput., 18 (2006), 1527-1554. |
[8] | Y. Z. Xu, X. J. Yao, X. Li, et al, Target detection in high resolution remote sensing images based on full convolution network, Bull. Surv. Mapp., 1 (2018), 77-82. |
[9] | M. S. Hasan, An application of pre-trained CNN for image classification, 2017 20th International Conference of Computer and Information Technology (ICCIT), 2017. Available from: https://ieeexplore.ieee.org/stamp/stamp.jsp?tp=&arnumber=8281779. |
[10] | X. Qin, Y. Zhou, Z. He, et al., A Faster R-CNN Based Method for Comic Characters Face Detection, 2017 14th IAPR International Conference on Document Analysis and Recognition (ICDAR), 2017, 1074-1080. Available from: https://ieeexplore.ieee.org/stamp/stamp.jsp?tp=&arnumber=8270109. |
[11] | R. Ranjan, V. M. Patel and R. Chellappa, HyperFace: A Deep Multi-Task Learning Framework for Face Detection, Landmark Localization, Pose Estimation, and Gender Recognition, IEEE Trans. Pattern Anal. Mach. Intell., 41 (2019), 121-135. |
[12] | S. Xie and H. Hu, Facial Expression Recognition Using Hierarchical Features with Deep Comprehensive Multipatches Aggregation Convolutional Neural Networks, IEEE Trans. Multimedia, 21 (2019), 211-220. |
[13] | G. Chen, C. Li, W. Wei, et al., Fully convolutional neural network with augmented atrous spatial pyramid pool and fully connected fusion path for high resolution remote sensing image segmentation, Appl. Sci., 9 (2019), 1816. |
[14] | A. Kulikajevas, R. Maskeliūnas, R. Damaševičius, et al., Reconstruction of 3D Object Shape Using Hybrid Modular Neural Network Architecture Trained on 3D Models from ShapeNetCore Dataset, Sensors, 19 (2019), 1553. |
[15] | G. B. Huang, Q. Y. Zhu and C. K. Siew, Extreme learning machine: A new learning scheme of feedforward neural networks, Neural Networks, 2 (2004), 985-990. |
[16] | C. He, F. Xu, Y. Liu, et al., A fast kernel extreme learning machine based on conjugate gradient, Network Comput. Neural Syst., 29 (2018), 70-80. |
[17] | G. B. Huang, X. Ding and H. Zhou, Optimization method based extreme learning machine for classification, Neurocomputing, 74 (2010), 155-163. |
[18] | W. Zou, F. Yao, B. Zhang, et al., Back Propagation Convex Extreme Learning Machine, Proc. ELM, 9 (2016), 259-272. |
[19] | C. He, Y. Liu, T. Yao, et al., A fast learning algorithm based on extreme learning machine for regular fuzzy neural network, J. Intell. Fuzzy Syst., 36 (2019), 3263-3269. |
[20] | W. Deng, Q. Zheng and L. Chen, Regularized Extreme Learning Machine, 2009 IEEE Symposium on Computational Intelligence and Data Mining, 2009, 389-395. Available from: https://ieeexplore.ieee.org/abstract/document/4938676. |
[21] | W. Y. Deng, Q. H. Zheng, L. Chen, et al., Study on extreme learning method of neural network, Chin. J. Comput., 33 (2010), 279-287. |
1. | Saqib Ali, Jianqiang Li, Yan Pei, Muhammad Saqlain Aslam, Zeeshan Shaukat, Muhammad Azeem, An Effective and Improved CNN-ELM Classifier for Handwritten Digits Recognition and Classification, 2020, 12, 2073-8994, 1742, 10.3390/sym12101742 | |
2. | Zhongze Wu, Chunmei He, Liwen Yang, Fangjun Kuang, Attentive evolutionary generative adversarial network, 2021, 51, 0924-669X, 1747, 10.1007/s10489-020-01917-8 | |
3. | Jinghan Shang, Fei Shao, Jun Liu, Design of the Music Intelligent Management System Based on a Deep CNN, 2022, 2022, 1939-0122, 1, 10.1155/2022/1559726 | |
4. | Xiufen Luo, Pan Zheng, Analysis the Innovation Path on Psychological Ideological with Political Teaching in Universities by Big Data in New Era, 2022, 2022, 1748-6718, 1, 10.1155/2022/4305886 | |
5. | G. D. Praveenkumar, Dr. R. Nagaraj, Deep Convolutional Neural Network Based Extreme Learning Machine Image Classification, 2021, 2394-4099, 30, 10.32628/IJSRSET1218475 | |
6. | Hui Deng, Zhibin Ou, Yichuan Deng, Multi-Angle Fusion-Based Safety Status Analysis of Construction Workers, 2021, 18, 1660-4601, 11815, 10.3390/ijerph182211815 | |
7. | Songjian Dan, 2022, Chapter 7, 978-3-030-89507-5, 50, 10.1007/978-3-030-89508-2_7 | |
8. | Li Lin, Wen Gan, Yuchen Li, 3D Simulation Design and Application of Traditional Hanfu Based on Internet of Things, 2022, 2022, 1607-887X, 1, 10.1155/2022/6977485 | |
9. | Chunmei He, Lanqing Zheng, Taifeng Tan, Xianjun Fan, Zhengchun Ye, Manifold discrimination partial adversarial domain adaptation, 2022, 252, 09507051, 109320, 10.1016/j.knosys.2022.109320 | |
10. | Lijun Zhou, Wei Liao, Dongyang Wang, Dong Wang, Guinan Zhang, Yi Cui, Junyi Cai, A High-Precision Diagnosis Method for Damp Status of OIP Bushing, 2021, 70, 0018-9456, 1, 10.1109/TIM.2020.3047194 | |
11. | Ya'nan Wang, Sen Liu, Haijun Jia, Xintao Deng, Chunpu Li, Aiguo Wang, Cuiwei Yang, A two-step method for paroxysmal atrial fibrillation event detection based on machine learning, 2022, 19, 1551-0018, 9877, 10.3934/mbe.2022460 | |
12. | Zhiping Song, 2022, Mathematical Modeling Method based on Neural Network and Computer Multi-Dimensional Space, 978-1-7281-8115-8, 1080, 10.1109/ICETCI55101.2022.9832088 | |
13. | Shaojing Liu, Analysis on the literature communication path of new media integrating public mental health, 2022, 13, 1664-1078, 10.3389/fpsyg.2022.997558 | |
14. | Haiyao Wang, Bolin Dai, Xiaolei Li, Naiwen Yu, Jingyang Wang, A Novel Hybrid Model of CNN-SA-NGU for Silver Closing Price Prediction, 2023, 11, 2227-9717, 862, 10.3390/pr11030862 | |
15. | Subramanian. M, Md. Abul Ala Walid, Dr. Sarada Prasanna Mallick, Ravi Rastogi, Amit Chauhan, A. Vidya, 2023, Melanoma Skin Cancer Detection using a CNN-Regularized Extreme Learning Machine (RELM) based Model, 979-8-3503-4664-0, 1239, 10.1109/ICEARS56392.2023.10085489 | |
16. | Yiyun Zhang, Sheng'an Zhou, 2022, Face Feature Extraction Algorithm Based on Wavelet Transform and CNN, 978-1-6654-9721-3, 111, 10.1109/ICITBS55627.2022.00032 | |
17. | Shahad Altamimi, Qasem Abu Al-Haija, Maximizing intrusion detection efficiency for IoT networks using extreme learning machine, 2024, 4, 2730-7239, 10.1007/s43926-024-00060-x | |
18. | Lansa Ding, Xiaoyi Wei, Dezheng Wang, Congyan Chen, Construction of multi-features comprehensive indicator for machinery health state assessment, 2024, 35, 0957-0233, 066202, 10.1088/1361-6501/ad2bcb | |
19. | Qi Shi, Yanlei Li, Fan Zhang, Qianyun Ma, Jianfeng Sun, Yaqiong Liu, Jianlou Mu, Wenxiu Wang, Yiwei Tang, Whale optimization algorithm-based multi-task convolutional neural network for predicting quality traits of multi-variety pears using near-infrared spectroscopy, 2024, 215, 09255214, 113018, 10.1016/j.postharvbio.2024.113018 | |
20. | Zhenhao Zhu, Qiushuang Zheng, Hongbing Liu, Jingyang Zhang, Tong Wu, Xianqiang Qu, Prediction Model for Pipeline Pitting Corrosion Based on Multiple Feature Selection and Residual Correction, 2024, 1671-9433, 10.1007/s11804-024-00468-5 | |
21. | Zhicheng Liu, Long Zhao, Guanru Wen, Peng Yuan, Qiu Jin, A Monitoring Method for Transmission Tower Foots Displacement Based on Wind-Induced Vibration Response, 2023, 17, 1930-2991, 541, 10.32604/sdhm.2023.029760 | |
22. | Manjula Prabakaran, Jesmin Zakaria, S Kannan, Santhiya P, Rajasekar P, M V Rathnamma, 2025, Enhancing Kidney Stone Detection in CT scans with Image Processing and the DCNN-RELM Model, 979-8-3315-0967-5, 1317, 10.1109/ICEARS64219.2025.10940943 |
databases | classes | features | number of samples | remarks |
ORL | 40 | 10 | 400 | These include changes in facial expressions, minor changes in posture, and changes in scale within 20%. |
NUST | 96 | 10 | 216 | It mainly includes the change of face's different pose. |
databases | methods | division of sample sets | classification accuracy (%) | |
training samples | test samples | |||
ORL | RELM CNN CNN-RELM |
280 280 280 |
120 120 120 |
91.67 90.00 96.67 |
NUST | RELM CNN CNN-RELM |
672 672 672 |
288 288 288 |
89.58 88.54 96.88 |
methods | training samples | |||
4 | 5 | 6 | 7 | |
Max-pooling | 83.75 | 88.00 | 94.37 | 96.67 |
Average-pooling | 84.17 | 92.00 | 96.25 | 97.50 |
Stochastic-pooling | 83.75 | 89.50 | 95.00 | 96.67 |
Lp-pooling | 84.58 | 92.50 | 96.88 | 98.33 |
databases | classes | features | number of samples | remarks |
ORL | 40 | 10 | 400 | These include changes in facial expressions, minor changes in posture, and changes in scale within 20%. |
NUST | 96 | 10 | 216 | It mainly includes the change of face's different pose. |
databases | methods | division of sample sets | classification accuracy (%) | |
training samples | test samples | |||
ORL | RELM CNN CNN-RELM |
280 280 280 |
120 120 120 |
91.67 90.00 96.67 |
NUST | RELM CNN CNN-RELM |
672 672 672 |
288 288 288 |
89.58 88.54 96.88 |
methods | training samples | |||
4 | 5 | 6 | 7 | |
Max-pooling | 83.75 | 88.00 | 94.37 | 96.67 |
Average-pooling | 84.17 | 92.00 | 96.25 | 97.50 |
Stochastic-pooling | 83.75 | 89.50 | 95.00 | 96.67 |
Lp-pooling | 84.58 | 92.50 | 96.88 | 98.33 |