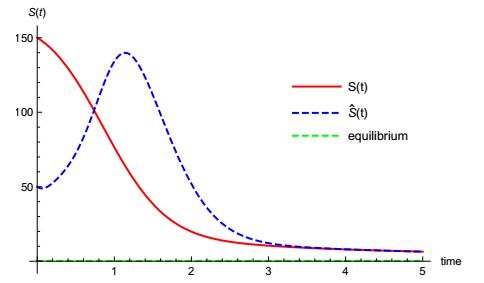
Citation: Mamadou L. Diouf, Abderrahman Iggidr, Max O. Souza. Stability and estimation problems related to a stage-structured epidemic model[J]. Mathematical Biosciences and Engineering, 2019, 16(5): 4415-4432. doi: 10.3934/mbe.2019220
[1] | Zhisheng Shuai, P. van den Driessche . Impact of heterogeneity on the dynamics of an SEIR epidemic model. Mathematical Biosciences and Engineering, 2012, 9(2): 393-411. doi: 10.3934/mbe.2012.9.393 |
[2] | Raquel Caballero-Águila, María J. García-Ligero, Aurora Hermoso-Carazo, Josefa Linares-Pérez . Unreliable networks with random parameter matrices and time-correlated noises: distributed estimation under deception attacks. Mathematical Biosciences and Engineering, 2023, 20(8): 14550-14577. doi: 10.3934/mbe.2023651 |
[3] | Jianxin Yang, Zhipeng Qiu, Xue-Zhi Li . Global stability of an age-structured cholera model. Mathematical Biosciences and Engineering, 2014, 11(3): 641-665. doi: 10.3934/mbe.2014.11.641 |
[4] | Bruno Buonomo, Deborah Lacitignola . On the stabilizing effect of cannibalism in stage-structured population models. Mathematical Biosciences and Engineering, 2006, 3(4): 717-731. doi: 10.3934/mbe.2006.3.717 |
[5] | Abdennasser Chekroun, Mohammed Nor Frioui, Toshikazu Kuniya, Tarik Mohammed Touaoula . Global stability of an age-structured epidemic model with general Lyapunov functional. Mathematical Biosciences and Engineering, 2019, 16(3): 1525-1553. doi: 10.3934/mbe.2019073 |
[6] | Gerasimos G. Rigatos, Efthymia G. Rigatou, Jean Daniel Djida . Change detection in the dynamics of an intracellular protein synthesis model using nonlinear Kalman filtering. Mathematical Biosciences and Engineering, 2015, 12(5): 1017-1035. doi: 10.3934/mbe.2015.12.1017 |
[7] | Jia Li . Malaria model with stage-structured mosquitoes. Mathematical Biosciences and Engineering, 2011, 8(3): 753-768. doi: 10.3934/mbe.2011.8.753 |
[8] | H. Thomas Banks, Shuhua Hu, Zackary R. Kenz, Hien T. Tran . A comparison of nonlinear filtering approaches in the context of an HIV model. Mathematical Biosciences and Engineering, 2010, 7(2): 213-236. doi: 10.3934/mbe.2010.7.213 |
[9] | Yi Zhang, Yue Song, Song Yang . T-S fuzzy observer-based adaptive tracking control for biological system with stage structure. Mathematical Biosciences and Engineering, 2022, 19(10): 9709-9729. doi: 10.3934/mbe.2022451 |
[10] | Jessica L. Hite, André M. de Roos . Pathogens stabilize or destabilize depending on host stage structure. Mathematical Biosciences and Engineering, 2023, 20(12): 20378-20404. doi: 10.3934/mbe.2023901 |
The dynamics of chronic diseases that are directly transmitted appears to be very simple at a first glance: in the presence of infectious individuals, susceptible ones get infected according to some assumed force of infection — in some cases there might be an exposed or latent stage. Thus, SI and SEI models (or their modified versions with more general incidence terms) seem to be very simple and appropriate descriptions of such dynamics. However, the development of such diseases is seldom as simple as described above. In many cases, an infected individual goes through different phases of disease severity, with possibly diverse forces of infection and disease-induced mortality rates – e.g. AIDS.
In this work, we analyse a class of stage-structured SI models with n infectious stages: Let x=(S,I1,…,In)T, and consider the following dynamics:
X(x)=(Λ−∑ni=1βiIiφ(S)−μ0S∑ni=1βiIiφ(S)−(μ1+γ1)I1γ1I1−(μ2+γ2)I2⋮γn−2In−2−(μn−1+γn−1)In−1γn−1In−1−μnIn). |
Most studies in the literature of mathematical epidemiology modelling focus on the understanding of both local and global stability features. The rationale behind this choice is that while local stability of the disease free equilibrium (DFE) characterises the invasibility dynamics of the disease, global stability describes the long term behaviour of the disease dynamics. In many models, these features depend only on the basic reproduction number of the model — the so-called R0— with the DFE being both locally and globally asymptotically stable when R0≤1, and an endemic equilibrium (usually unique) satisfying these properties when R0>1. This led Shuai and van den Driessche to introduce the concept of sharp threshold property (STP), cf. [1].
On the other hand, except perhaps for the simplest models, even when the STP is satisfied, the time-scale between departure of the population state from the DFE and convergence to the EE is not necessarily short and the transient dynamics can be of significant interest. Therefore, while combined local-global analysis is mathematically correct, it can lead to an oversimplified view of the true dynamics.
This change of focus brings in additional issues: under the dichotomy of models with the STP, one expects to find the population state either near the DFE or near the EE. When considering the transient dynamics, this is no longer true. Moreover, when performing a long-term analysis the only information about the initial condition is that it has infectious individuals — for the transient dynamics, the knowledge of initial condition is important. Nevertheless, it is usually not possible to measure the whole state of the system at any time — in particular, the initial condition is usually unknown. Indeed, often one has access only to a partial information of the state.
These new issues lead quite naturally to the so-called observation problem: given some measurable information about a population, we want to estimate its complete state. More precisely, let x(t) and y(t) be the population state and the measurable information about this at time t — we will assume that y(t)=h(x(t)), where h is a smooth function. Let X(s) be a Lipschitz vector field that generates a global solution and consider the following problem:
{˙x=X(x)y=h(x) | (1.1) |
Give an initial condition, x(t0) say, the quantities x(t) and y(t) are uniquely determined. On the other hand, assume that only y(t) is known for t∈[t1,t2]. We are interested in identifying what kind of properties the vector field X, and the function h should satisfy in order that is possible to obtain an asymptotic estimate ˆx(t) of the state x(t). In addition, we also want to investigate how ˆx(t) can be computed using the vector field X and the measurable output y(t) — recall that we do not know the initial condition x(t0), and hence we cannot integrate the differential equation ˙x=X(x). We assume that the number of infected individuals in the last stage can be measured. Therefore, we have y=h(x)=C.x=(0,…,0,1).x=In.
Our goal is the following: knowing In(t) for all t≥0, how to derive estimates ˆS(t),ˆIi(t) satisfying limt→∞ˆS(t)−S(t)=0 and limt→∞ˆIi(t)−Ii(t)=0 and the convergence should be as fast as possible.
Outline
The remaining of the paper is organised as follows: Section 2 is devoted to a quick review of the asymptotic behaviour of the model: we give the expression of the basic reproduction number R0 and we study the stability properties of the equilibria.
In Section 3, we will show that the system with the output y=In is observable and we give some observers (state-estimators) that allow to give dynamical estimates of the unmeasured state variables S(T),I1(t),…,In−1(t). More precisely, we construct two different observer: the classical high-gain and the extended Kalman filter.
Next, in section 4, we present numerical experiments that indicate that convergence of the estimates ˆx(t) delivered by these observers towards the state x(t) is exponentially fast. However, the high-gain observer can display significant overshooting in the initial stages, while the Kalman filter observer does not present this behaviour. The latter also is robust when measurements are noisy, whereas the former might fail to converge in this case.
We finish with a discussion on the results in Section 5.
We consider the following epidemic model with multiple infectious stages:
{˙S=Λ−∑ni=1βiIiφ(S)−μ0S,˙I1=∑ni=1βiIiφ(S)−(μ1+γ1)I1,˙I2=γ1I1−(μ2+γ2)I2,⋮˙In−1=γn−2In−2−(μn−1+γn−1)In−1˙In=γn−1In−1−μnIn. | (2.1) |
where:
Λ is the recruitment, and βi is the per capita contact in the compartment Ii. The function φ is assumed to be continuous, positive and increasing that models the exposure of susceptible individuals to contacts with infectious ones. In particular, it allows for the specification of a more general incidence rate than the standard bilinear one — for instance, one can use φ(S)=Sp or φ(S)=S1+aS (with a>0) to take into account saturation effects. μ0 is the natural death rate of the susceptible individuals and μi is the death rate of the infected individuals in stage i, in general μi=μ0+di, di being the additional disease induced mortality rate. γi is the transition rate from stage i to stage i+1. The stability of this model with φ(S)=S has already been studied in ([2,3]).
It is easy to show that the set
Ω={(S,I1,…,In)∈Rn+1+:S+n∑i=1Ii≤Λμ0} since μ0=mini=0,…,nμi |
is a positively invariant set for the system.
The disease free equilibrium is DFE=(Λμ0,0,…,0).
The basic reproduction number represents the number of secondary cases produced by one infective host in entirely susceptible population. Following [4], we define by Fi(S,I) the rate of appearance of new infections in compartment i, and by Vi(S,I) the rate of transfer individuals in and out the compartment i by all others means. The matrix F and V are given by:
F=[∑ni=1βiIiφ(S)0⋯0] |
and
V=[−(μ1+γ1)I1γ1I1−(μ2+γ2)I2⋯γn−1In−1−μnIn]. |
Note that our V is the opposite of the same used in [4]. The Jacobian matrices at the disease free equilibrium are:
F=DF=(β1φ(Λ/μ0)β2φ(Λ/μ0)⋯βnφ(Λ/μ0)00⋯0⋮⋮⋱⋮00⋯0) |
and
V=DV=(−(μ1+γ1)000⋯00γ1−(μ2+γ2)00⋯000γ2−(μ3+γ3)0⋯00⋮⋮⋮⋮⋱⋮⋮0000⋯γn−1−μn). |
The basic reproduction number is then given by R0=ρ(−FV−1), and it is equal to:
R0=n−1∑i=1βiφ(Λ/μ0)∏ij=1γj−1∏ij=1(μj+γj)+βnφ(Λ/μ0)μnn−1∏j=1γjμj+γj with γ0=1=(n−1∑i=1βii∏j=1γj−1(μj+γj)+βnμnn−1∏j=1γjμj+γj)φ(Λ/μ0). |
Proposition 2.1. If R0>1, then system (2.1) has a unique endemic equilibrium.
An endemic equilibrium (S∗,I∗1,…,I∗n) satisfy :
I∗n=γn−1I∗n−1μnand fori=n−1downto2,I∗i=γi−1I∗i−1μi+γi. |
Then for i=2 to n−1
I∗i=i−1∏j=1γjμj+1+γj+1I∗1=i∏j=2γj−1μj+γjI∗1, | (2.2) |
and
I∗n=n−2∏j=1γjμj+1+γj+1×γn−1μnI∗1. | (2.3) |
Replacing the expressions of I∗i in the second equation of (2.1) gives:
(β1+n−1∑i=2βii∏j=2γj−1μj+γj+βnμn∏n−1j=1γj∏n−1j=2(μj+γj))φ(S∗)=μ1+γ1, |
(n−1∑i=1βii∏j=1γj−1(μj+γj)+βnμnn−1∏j=1γjμj+γj)φ(S∗)=1 |
R0φ(S∗)φ(Λ/μ0)=1 |
Thus,
φ(S∗)=φ(Λ/μ0)R0. | (2.4) |
Therefore, if R0>1 then φ(Λ/μ0)R0<φ(Λ/μ0), and since φ is a continuous and increasing function, it follows that there exists a unique S∗∈(0,Λ/μ0) satisfying equation (2.4), more precisely, S∗=φ−1(φ(Λ/μ0)R0).
Furthermore, by replacing S∗, φ(S∗) and I∗i for i=2 to n by their expressions, we get the expression of I∗1. After this, the expressions of I∗i,i∈{2,…,n} are also uniquely determined from (2.2) and (2.3).
Theorem 2.1. If R0≤1, the disease free equilibrium is globally asymptotically stable.
Proof. Let us consider:
V=∫SΛ/μ0(1−φ(Λ/μ0)φ(u))du+bI, |
where
I=(I1,…,In)T |
and
b=(1,μ1+γ1γ1−β1φ(Λμ0)γ1,…,…,n−1∏i=1(μi+γi)γi−n−1∑i=1βiφ(Λ/μ0)∏n−1j=i+1(μj+γj)∏n−1j=iγj). |
On the other hand:
R0=β1φ(Λ/μ0)μ1+γ1+β2φ(Λ/μ0)γ1(μ1+γ1)(μ2+γ2)+β3φ(Λ/μ0)γ1γ2(μ1+γ1)(μ2+γ2)(μ3+γ3)+…+βnφ(Λ/μ0)γ1…γn−1(μ1+γ1)…(μn−1+γn−1)μn |
Since R0≤1, we have μ1+γ1−β1φ(Λ/μ0)>0, and also
(μ1+γ1)(μ2+γ2)−β1φ(Λ/μ0)(μ2+γ2)−β2φ(Λ/μ0)γ1>0, |
and so on, until the last component of b,
n−1∏i=1(μi+γi)γi−n−1∑i=1βiφ(Λ/μ0)∏n−1j=i+1(μj+γj)∏n−1j=iγj>0. |
This shows that V is positive in Ω. Its derivative is:
˙V=(1−φ(Λ/μ0)φ(S))˙S+b˙I. |
The terms containing Ii, for i∈{1,…,n−1}, and βnInφ(S) cancel (thanks to the choice of b), and we obtain
˙V=(Λ−μS)(1−φ(Λ/μ0)φ(S))+(n∑i=1βiφ(Λ/μ0)∏n−1j=i+1(μj+γj)∏n−1j=iγj−n−1∏i=1(μi+γi)γi)In. |
This expression is equal to:
˙V=Λ(1−SΛ/μ0)(1−φ(Λ/μ0)φ(S))+n−1∏i=1(μi+γi)μnγi(R0−1)In. |
Since φ is an increasing positive function and R0≤1, we have ˙V≤0. By LaSalle Invariance Principle [5], the disease free equilibrium is globally asymptotically stable.
Theorem 2.2. If R0>1 then the unique endemic equilibrium EE is globally asymptotically stable.
Proof. We consider the following candidate Lyapunov function
V=∫SS∗(1−φ(S∗)φ(u))du+cT(I1−I∗1lnI1,…,In−I∗nlnIn)T, |
where
cT=(1,μ1+γ1γ1−β1φ(S∗)γ1I∗1,(μ1+γ1)(μ2+γ2)γ1γ2−φ(S∗)γ2I∗2(β1I∗1+β2I∗2),…,…,∏n−1i=1μi+γiγi−φ(S∗)γn−1I∗n−1∑n−1i=1βiI∗i). |
We have
For i=2,…n:ci=∏i−1j=1μj+γjγj−φ(S∗)γi−1I∗i−1∑i−1j=1βjI∗j=μ1+γ1μi+γiI∗1I∗i−φ(S∗)(μi+γi)I∗i∑i−1j=1βjI∗j=1(μi+γi)I∗i((μ1+γ1)I∗1−φ(S∗)∑i−1j=1βjI∗j)=φ(S∗)∑nj=iβjI∗j(μi+γi)I∗i>0. |
The derivative of V along the solutions is:
˙V=(1−φ(S∗)φ(S))(Λ−∑ni=1βiIiφ(S)−μS)+(1−I∗1I1)(∑ni=1βiIiφ(S)−(μ1+γ1)I1)+∑ni=2ci(1−I∗iIi)(γi−1Ii−1−(μi+γi)Ii)( with γn=0)=(Λ−μS)−(Λφ(S∗)φ(S)−∑ni=1βiIiφ(S∗)−μSφ(S∗)φ(S))−(μ1+γ1)I1−(∑ni=1βiI∗1I1Iiφ(S)−(μ1+γ1)I∗1)+∑ni=2ci(1−I∗iIi)(γi−1Ii−1−(μi+γi)Ii)=(∑ni=1βiI∗iφ(S∗)+μS∗−μS)−((∑ni=1βiI∗iφ(S∗)+μS∗)φ(S∗)φ(S)−∑ni=1βiIiφ(S∗)−μSφ(S∗)φ(S))−(μ1+γ1)I1−(∑ni=1βiI∗1I1Iiφ(S)−(μ1+γ1)I∗1)+∑ni=2ci(1−I∗iIi)(γi−1Ii−1−(μi+γi)Ii) |
˙V=(∑ni=1βiI∗iφ(S∗)+μS∗−μS)−((∑ni=1βiI∗iφ(S∗)+μS∗)φ(S∗)φ(S)−∑ni=1βiIiφ(S∗)−μSφ(S∗)φ(S))−(μ1+γ1)I1−(∑ni=1βiI∗1I1Iiφ(S)−(μ1+γ1)I∗1)+∑ni=2ci(1−I∗iIi)(γi−1Ii−1−(μi+γi)Ii)=μ(S∗−S)(1−φ(S∗)φ(S))+β1I∗1φ(S∗)(2−φ(S∗)φ(S)−φ(S)φ(S∗))+∑ni=1βiIiφ(S∗)−(μ1+γ1)I1+∑ni=2ci(γi−1Ii−1−(μi+γi)Ii)+2∑ni=2βiI∗iφ(S∗)−∑ni=2βiI∗iφ(S∗)φ(S∗)φ(S)−∑ni=2βiI∗1I1Iiφ(S)−∑ni=2ci(γi−1I∗iIiIi−1−(μi+γi)I∗i) |
On one hand, we have
∑ni=1βiIiφ(S∗)−(μ1+γ1)I1+∑ni=2ci(γi−1Ii−1−(μi+γi)Ii)=∑ni=2ciγi−1Ii−1+∑ni=1(βiIiφ(S∗)−ci(μi+γi)Ii)=∑n−1i=1ci+1γiIi+∑ni=1(βiIiφ(S∗)−ci(μi+γi)Ii)=∑n−1i=1(ci+1γi+βiφ(S∗)−ci(μi+γi))Ii+(βnφ(S∗)−cnμn)In=0, since ci+1=ci(μi+γi)−βiφ(S∗)γi and cn=βnμnφ(S∗) |
On the other hand,
ci(μi+γi)I∗i=(μ1+γ1)I∗1−φ(S∗)i−1∑j=1βjI∗j and ciγi−1I∗iIiIi−1=((μ1+γ1)I∗1−i−1∑j=1βjI∗jφ(S∗))I∗iIi−1IiI∗i−1. |
Therefore,
˙V=μ(S∗−S)(1−φ(S∗)φ(S))+β1I∗1φ(S∗)(2−φ(S∗)φ(S)−φ(S)φ(S∗))+A |
with
A=φ(S∗)n∑i=2(2βiI∗i+n∑j=iβjI∗j−βiI∗iφ(S∗)φ(S)−βiI∗iI∗1Iiφ(S)I1I∗iφ(S∗)−n∑j=iβjI∗jI∗iIi−1IiI∗i−1)=n∑i=2βiI∗iφ(S∗)(i+1−φ(S∗)φ(S)−I∗1Iiφ(S)I1I∗iφ(S∗)−I∗2I1I2I∗1−I∗3I2I3I∗2−…−I∗iIi−1IiI∗i−1) |
Hence,
˙V=μ(S∗−S)(1−φ(S∗)φ(S))+β1I∗1φ(S∗)[2−φ(S∗)φ(S)−φ(S)φ(S∗)] |
+β2I∗2φ(S∗)[3−φ(S∗)φ(S)−I1I∗2I∗1I2−I∗1I2φ(S)I1I∗2φ(S∗)]+β3I∗3φ(S∗)[4−φ(S∗)φ(S)−I∗1I3φ(S)I1I∗3φ(S∗)−I1I∗2I∗1I2−I2I∗3I∗2I3] |
+…+βnI∗nφ(S∗)[n+1−φ(S∗)φ(S)−I∗1Inφ(S)I1I∗nφ(S∗)−I1I∗2I∗1I2−I2I∗3I∗2I3−…−In−1I∗nI∗n−1In]. |
The monotonicity of φ together with the inequality between the arithmetic and geometric means show that ˙V is negative definite with respect to EE. It follows that the endemic equilibrium EE is globally asymptotically stable on the positive orthant Rn+1+ minus the stable manifold of the DFE.
The state of System (2.1) will be denoted x(t)=(S(t),I1(t),…,In(t))T. We suppose that only the number of infected in the last stage is available for measurement, i.e, the measurable output of the system is y(t)=In(t). Therefore we are interested in the observability properties of the following system
{˙x=f(x),y=h(x). | (3.1) |
where the expression of f is given in (2.1) and h(x)=x.(0,…,1).
To study the observability of (3.1), we introduce (as usual) the following map using the successive time derivatives of the output function along the trajectories of the system:
ψ:x⟼(y˙y⋮y(n)) |
Lemma 3.1. The map ψ is a diffeomorphism from from ∘Ω (the interior of Ω) onto ψ(∘Ω), and hence system (3.1) is observable.
Proof. For k∈{1,…,n−1}, the kth derivative of y is given by the following expression:
y(k)=(−1)kμknIn+(−1)k−1γn−1∑k−1i=0(μn−1+γn−1)k−1−iμinIn−1+(−1)k−2γn−1γn−2[∑k−2i=0(μn−2+i+γn−2+i)k−2−i((μn−1+i+γn−1+i)i+μin)+∑i,j,l≠0,i+j+l=k−2(μn−2+γn−2)i(μn−1+γn−1)jμln]In−2+……+(−1)k−p∏k−pi=1γn−i[∑k−pi=0(μn−p+i+γn−p+i)k−p−i((μn−p+1+i+γn−p+1+i)i+…+(μn−1+γn−1)i+μin)+∑il≠0,i1+…+ip+1=k−p(μn−p+γn−p)i1…(μn−1+γn−1)ipμip+1n]In−p+……−∏k−1i=1γn−i((μn−k+1+γn−k+1)+(μn−k+2+γn−k+2)+…+(μn−1+γn−1)+μn)In−k+1+∏ki=1γn−iIn−k. |
Denote by ckIn−p the coefficient corresponding to the variable In−p. The expression for y(k) can be rewritten as:
y(k)=k∑p=0ckIn−pIn−p,k∈{1,…,n−1}. |
Moreover, the expression of y(n) can be easily deduced from the expression of y(n−1):
y(n)=n−2∑p=0cn−1In−p(γn−p−1In−p−1−(μn−p+γn−p)In−p)+cn−1I1(n∑i=1βiIiφ(S)−(μ1+γ1)I1). |
The Jacobian matrix of ψ at x=(S,I1,I2,…,In) is:
∂ψ∂x=(0⋯0010⋯0c1In−1c1In0⋯c2In−2c2In−1c2In⋮...⋮⋮⋮cnS⋯⋯cnIn−1cnIn) |
An inductive computation shows that every ciIn−i, for i=1 to n−1, is uniquely determined, constant and not equal to zero. The last coefficient cnS, corresponding to the variable S in x, is given by:
cnS=cn−1I1n∑i=1βiIiφ′(S). |
The absolute value of the determinant of the Jacobian matrix is |det(∂ψ∂x)|=cnS∏n−1i=1ciIn−i. Since ciIn−i≠0 for all i∈{1,…,n−1}, it follows that det(∂ψ∂x)=0 if and only if (I1,…,In)=(0,…,0). Therefore, the rank of ∂ψ∂x is then n+1 on ∘Ω the interior of Ω. Now, consider x and x′ such that ψ(x)=ψ(x′). Then the equality of the first component of the vectors gives In=I′n, and therefore the equality of the second component of ψ(x) and ψ(x′) gives that In−1=I′n−1. By repeating this reasoning until the nth−1 component, we get Ik=I′k, for k∈{1,…,n}. Using these equalities in the last equation and the fact that φ is strictly increasing and continuous, we obtain that S=S′. Then x=x′, which prove that ψ is injective. The injectivity of ψ and the full rank of ∂ψ∂x show that ψ is a diffeomorphism from ∘Ω onto ψ(∘Ω).
We are now able to construct an observer for (3.1).
We begin by performing variable change z=ψ(x), and we determine ψ−1. Through the identification zi=ψi(x), we obtain that:
In=z1,In−1=1γn−1z2−μnγn−1z1, |
In−2=1γn−1γn−2z3+(μn−1+γn−1)+μnγn−1γn−2z2−(μn−1+γn−1)μn+2μ2nγn−1γn−2z1, |
repeating this until the (n−1)-th component, we show that:
In−k=(dk1,…,dkk+1)(z1,…,zk+1), |
and the n+1 th component gives that:
S=φ−1(zn+1+(dn+11,…,dn+1n)(z1,…,zn)(d1,…,dn)(z1,…,zn)), |
recall that φ is continuous and strictly increasing.
With the new coordinate z, system (3.1) is given by the following:
{˙z=[010⋯0001⋯0...⋯0000⋯1000⋯0]z+(00.0Ψ(z(t))),h=z1=(1,…,0)z. | (3.2) |
We denote the matrix in (3.2) by A and C=(1,…,0). It is easily shown that Ψ(z)=y(n+1)(ψ−1(z)) is k-Lipschitzian on Ω. The observer of (3.2) is given by ([7,6]):
˙ˆz=Aˆz+(0,…,Ψ(ˆz(t)))T+Σ−1θCT(y−Cˆz), |
where Σθ is the solution of the equation:
θΣθ+ATΣθ+ΣθA−CTC=0. |
The resolution of the equation leads to:
Σθ(i,j)=(−1)i+j(i+j−2)!(i−1)!(j−1)!1θi+j−1. |
The system
˙ˆx=f(ˆx)+[∂ψ∂x]−1ˆx×Σ−1θCT(y−h(ˆx)) |
is an observer for (3.1).
Application: We apply the result for n=2 and φ(x)=x. The corresponding system is:
{˙S=Λ−(β1I1+β2I2)S−μ0S,˙I1=(β1I1+β2I2)S−(μ1+γ)I1,˙I2=γI1−μ2I2. | (3.3) |
The output is y=I2 and
ψ(S,I1,I2)=(y˙y¨y) = (I2γI1−μ2I2γ(β1I1+β2I2)S−γ(μ1+μ2+γ)I1+μ22I2).
Its Jacobian can be written as:
∂ψ∂x=(0010γ−μ2γ(β1I1+β2I2)γβ1S−γ(μ1+μ2+γ)γβ2S+μ22). |
The determinant of the Jacobian is equal to det(∂ψ∂x)=γ2(β1I1+β2I2), which is positive in the positively invariant open set Ω0={S>0,I1>0,I2>0,S+I1+I2<Λμ0}.
We give also the expression of Σθ:
Σθ=(θ−θ−2θ−3−θ−22θ−3−3θ−4θ−3−3θ−46θ−5). |
With the variable change, we have: z=ψ(S,I1,I2), which leads to:
z1=I2,z2=γI1−μ2I2,I1=μ2γz1+1γz2, |
z3=γ(β1I1+β2I2)S−γ(μ1+μ2+γ)I1+μ22I2, |
S=z3+γ(μ1+μ2+γ)I1−μ22I2γ(β1I1+β2I2), |
and when we replace I1 and I2 by their expression, we get:
S=μ2(μ1+γ)z1+(μ1+μ2+γ)z2+z3(μ2β1+γβ2)z1+β1z2. |
The expression of ψ−1 is:
ψ−1:z→{μ2(μ1+γ)z1+(μ1+μ2+γ)z2+z3(μ2β1+γβ2)z1+β1z2,μ2γz1+1γz2,z1. |
According to the expression of ψ−1 we deduce Ψ(z):
Ψ(z)=((β1μ2+β2γ)z2+β1z3)μ2(μ1+γ)z1+(μ1+μ2+γ)z2+z3(β1μ2+β2γ)z1+β1z2 |
+((β1μ2+β2γ)z1+β1z2)(Λ−(μ1+γ)μ2γz1−μ1+μ2+γγz2−z3γ |
−μ0μ2(μ1+γ)z1+(μ1+μ2+γ)z2+z3(β1μ2+β2γ)z1+β1z2)−(μ1+γ)z2−(μ1+μ2+γ)z3. |
With the variable z, system (3.3) is given by:
˙z=F(z)=(z2z3Ψ(z)) | (3.4) |
Let ˜Ψ be a globally Lipschitz map on the whole R3 whose restriction to Ω0 is Ψ, and ˜ψ an extension of the diffeomorphism ψ to the whole R3.
The observer of (3.4) is simply:
{˙ˆz1=ˆz2+3θ(z1−ˆz1),˙ˆz2=ˆz3+3θ2(z1−ˆz1),˙ˆz3=˜Ψ(ˆz)+θ3(z1−ˆz1). | (3.5) |
In the original coordinates, the expression of the observer is:
˙ˆx=f(ˆx)+[∂˜ψ∂x]−1ˆx×Σ−1θCT(y−h(ˆx)) |
This can be written:
{˙ˆS=Λ−(β1^I1+β2^I2)ˆS−μ0ˆS−(3θ2(μ1+μ2+γ−β1ˆS)γ(β1^I1+β2^I2)+θ3γ(β1^I1+β2^I2))(^I2−I2)−3θ(μ2(γ+μ1)−(β1μ2+β2γ)ˆS)γ(β1^I1+β2^I2)(^I2−I2),˙^I1=(β1^I1+β2^I2)ˆS−(γ+μ1)^I1−3μ2θ+θ2γ(^I2−I2),˙^I2=γ^I1−μ2^I2−3θ(^I2−I2). | (3.6) |
For System (3.4), it is possible to construct another type of observer called extended Kalman filter (see, for instance, [8]):
{˙ˆz=F(ˆz)−1rΣ(t)CT(Cˆz−y),˙Σ=Qθ+[A⋆(ˆz)]TΣ+ΣA⋆(ˆz)−1rΣCTCΣ, | (3.7) |
where r, θ are positive real numbers, Qθ=θΔ−1θQΔ−1θ, Q is a given symmetric positive definite n×n matrix, and Δθ=diag(1,1θ,…1θn−1). A⋆(ˆz) is the Jacobian matrix of F(z) evaluated at z=ˆz, i.e, A⋆(ˆz)=∂∂z(F(z))|z=ˆz.
Once again, for θ≥1 and large enough, the system (3.7) is an exponential observer for the system (3.4). The difference with the Luenberger extended observer (3.5) is that the gain 1rΣ(t)CT used in the correction term is not constant but it is dynamically computed as a solution of a Riccati matrix differential equation.
In the original coordinates, the observer is (with Σ(t) given by (3.7))
{˙ˆS=Λ−(β1^I1+β2^I2)ˆS−μ0ˆS−(Σ2,1(t)(μ1+μ2+γ−β1ˆS)γ(β1^I1+β2^I2)+Σ3,1(t)γ(β1^I1+β2^I2))(^I2−I2)−Σ1,1(t)(μ2(γ+μ1)−(β1μ2+β2γ)ˆS)γ(β1^I1+β2^I2)(^I2−I2),˙^I1=(β1^I1+β2^I2)ˆS−(μ1+γ)^I1−μ2Σ1,1(t)+Σ2,1(t)γ(^I2−I2),˙^I2=γ^I1−μ2^I2−Σ1,1(t)(^I2−I2). | (3.8) |
This observer works very efficiently and, unlike observer (3.6), there is no initial overshoot of the estimate.
Remark 3.1. It must be emphasized that use of the globally Lipschitz prolongations ˜Ψ and ˜ψ is not only a mathematical delicacy, but it is also essential for applications. Absence of such global Lipschitz prolongations can prevent convergence of the observer, as it is illustrated in [9]. However, it is not in general an easy task to construct explicitly such globally Lipschitz prolongations.
We simulated the various observers in the example case of a two-stage infectious class – the model given by System (3.3). The parameters used in these simulations were as follows: β1=0.01;β2=0.15;γ=0.02;Λ=40;μ0=μ1=0.01;μ2=0.025.
The evolution of S(t) and the corresponding estimates using the Kalman observer — Equation (3.8) — can be seen in Figure 1. These estimates are robust as it can be seen from the simulations in Figure 2, where the measurable output y(t)=I2(t) was corrupted with noise.
In Figure 4 we plotted the evolution of I1(t) together with the Kalman observer estimates. Figure 4 displays the corresponding noisy simulations.
The estimates of S(t) and I1(t) provided by the High Gain observer are displayed in Figures 5 and 6. In this case there is an initial overshoot of the estimate. When the measurable output, y(t), was corrupted by noise, we were not always able to verify the convergence of this observer, and hence we do not display any noisy results for the high gain observer.
In this work we considered and analysed a class of stage-structured SI models, which included a number of previously studied models in the literature — cf. [6,8].
We begin by identifying an expression for the basic reproductive number (R0) for these models, and we also show that they satisfy the Sharp Threshold Property [1]. We then proceed to present some preliminary results on the observability of these models, and after that we derive two state-estimators.
These estimators are then put to work in Section 4 where various numerical experiments are presented. Both estimators show exponential convergence, but the extended Luenberger estimator usually displays some initial overshooting in contradistinction to the extended Kalman filter. Indeed, the behaviour of the latter is very accurate, even when the measurable output y(t)=I2(t) is corrupted by noise. On the other hand, the high-gain Luenberger observer is very sensitive to noisy measurements, and it might fail to converge in this case.
We are grateful to two anonymous referees for valuable comments and suggestions that led to an improvement of this paper.
This work was partially funded by Inria and CAPES-Brazil in the framework of the program STIC AmSud (project MOSTICAW). MOS was partially supported by CNPq under grant # 309079/2015-2 and by CAPES — Finance Code 001.
All authors declare no conflicts of interest in this paper.
[1] | Z. Shuai and P. van den Driessche, Global stability of infectious disease models using Lyapunov functions, SIAM J. Appl. Math., 73 (2013), 1513-1532. |
[2] | A. Iggidr, J. Mbang, G. Sallet, et al., Multi-compartment models. Discrete Contin. Dyn. Syst. Supplements, suppl. volume(Dynamical Systems and Differential Equations. Proceedings of the 6th AIMS International Conference), 2007, 506–519. |
[3] | H. Guo and M. Y. Li, Global dynamics of a staged progression model for infectious diseases, Math. Biosci. Eng., 3 (2006), 513–525. |
[4] | P. van den Driessche and J. Watmough, Reproduction numbers and sub-threshold endemic equi- libria for compartmental models of disease transmission, Math. Biosci., 180 (2002), 29–48. |
[5] | J.-P. LaSalle, Stability theory for ordinary differential equations. J. Differ. Equations, 4 (1968), 57–65. |
[6] | J.-P. Gauthier, H. Hammouri and S. Othman, A simple observer for nonlinear systems applications to bioreactors. IEEE Trans. Autom. Control, 37 (1992), 875–880. |
[7] | G. Bornard and H. Hammouri, A high gain observer for a class of uniformly observable systems. In Proceedings of the 30th IEEE Conference on Decision and Control, 1991, 1494–1496. |
[8] | F. Deza, E. Busvelle, J.-P. Gauthier, et al., High gain estimation for nonlinear systems, Syst. Control Lett., 18 (1992), 295–299. |
[9] | A. Guiro, A. Iggidr, D. Ngom, et al., On the stock estimation for some fishery systems, Rev. Fish Biol. Fisher., 19 (2009), 313–327. |
1. | Rahul Bansal, Amit Kumar, Amit Kumar Singh, Sandeep Kumar, Stochastic filtering based transmissibility estimation of novel coronavirus, 2021, 112, 10512004, 103001, 10.1016/j.dsp.2021.103001 | |
2. | Rahul Bansal, Stochastic filtering in fractional-order circuits, 2021, 103, 0924-090X, 1117, 10.1007/s11071-020-06152-x | |
3. | Ali Zemouche, Rajesh Rajamani, Observer Design for Non-Globally Lipschitz Nonlinear Systems Using Hilbert Projection Theorem, 2022, 6, 2475-1456, 2581, 10.1109/LCSYS.2022.3170534 |