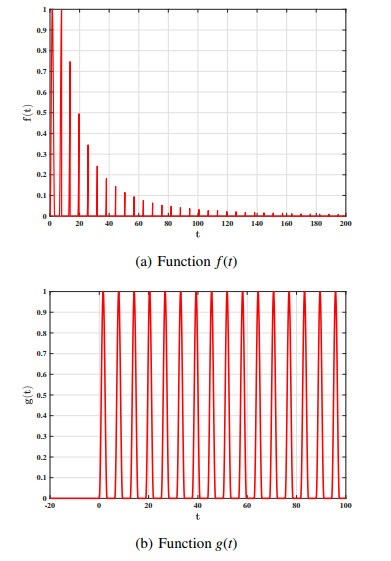
This study looks at the synthesis of innovative PEO/PVA/SrTiO3/NiO nanocomposites for piezoelectric sensors and gamma shielding applications that are low weight, elastic, affordable and have good gamma ray attenuation coefficients. The impact of SrTiO3/NiO on the structural characteristics of the PEO/PVA mixture is investigated. The polymer mixture PEO/PVA received additions of SrTiO3/NiO at concentrations of (0, 1, 2, 3 and 4) weight percent by the casting method. On the top surface of the films PEO/PVA/SrTiO3/NiO NCs, scanning electron microscopy reveals several randomly distributed aggregates or fragments that are consistent and coherent. An optical microscope image collection reveals that the blend*s additive distribution of NPs was homogenous. Gamma ray shielding application results show that the attenuation coefficient of PVA/PEO/SrTiO3/NiO NCs is increased by increasing concentration of SrTiO3/NiO nanoparticles. Radiation protection is another application for it. The pressure sensor application findings of NCs show that, when the applied pressure rises, electrical capacitance (Cp) increase.
Citation: Shaimaa Mazhar Mahdi, Majeed Ali Habeeb. Low-cost piezoelectric sensors and gamma ray attenuation fabricated from novel polymeric nanocomposites[J]. AIMS Materials Science, 2023, 10(2): 288-300. doi: 10.3934/matersci.2023015
[1] | Meiyu Sui, Yejuan Wang, Peter E. Kloeden . Pullback attractors for stochastic recurrent neural networks with discrete and distributed delays. Electronic Research Archive, 2021, 29(2): 2187-2221. doi: 10.3934/era.2020112 |
[2] | Wenlong Sun . The boundedness and upper semicontinuity of the pullback attractors for a 2D micropolar fluid flows with delay. Electronic Research Archive, 2020, 28(3): 1343-1356. doi: 10.3934/era.2020071 |
[3] | Xin-Guang Yang, Lu Li, Xingjie Yan, Ling Ding . The structure and stability of pullback attractors for 3D Brinkman-Forchheimer equation with delay. Electronic Research Archive, 2020, 28(4): 1395-1418. doi: 10.3934/era.2020074 |
[4] | Shu Wang, Mengmeng Si, Rong Yang . Dynamics of stochastic 3D Brinkman-Forchheimer equations on unbounded domains. Electronic Research Archive, 2023, 31(2): 904-927. doi: 10.3934/era.2023045 |
[5] | Guowei Liu, Hao Xu, Caidi Zhao . Upper semi-continuity of pullback attractors for bipolar fluids with delay. Electronic Research Archive, 2023, 31(10): 5996-6011. doi: 10.3934/era.2023305 |
[6] | Yangrong Li, Shuang Yang, Qiangheng Zhang . Odd random attractors for stochastic non-autonomous Kuramoto-Sivashinsky equations without dissipation. Electronic Research Archive, 2020, 28(4): 1529-1544. doi: 10.3934/era.2020080 |
[7] | Pan Zhang, Lan Huang, Rui Lu, Xin-Guang Yang . Pullback dynamics of a 3D modified Navier-Stokes equations with double delays. Electronic Research Archive, 2021, 29(6): 4137-4157. doi: 10.3934/era.2021076 |
[8] | Lingrui Zhang, Xue-zhi Li, Keqin Su . Dynamical behavior of Benjamin-Bona-Mahony system with finite distributed delay in 3D. Electronic Research Archive, 2023, 31(11): 6881-6897. doi: 10.3934/era.2023348 |
[9] | Lianbing She, Nan Liu, Xin Li, Renhai Wang . Three types of weak pullback attractors for lattice pseudo-parabolic equations driven by locally Lipschitz noise. Electronic Research Archive, 2021, 29(5): 3097-3119. doi: 10.3934/era.2021028 |
[10] | Yadan Shi, Yongqin Xie, Ke Li, Zhipiao Tang . Attractors for the nonclassical diffusion equations with the driving delay term in time-dependent spaces. Electronic Research Archive, 2024, 32(12): 6847-6868. doi: 10.3934/era.2024320 |
This study looks at the synthesis of innovative PEO/PVA/SrTiO3/NiO nanocomposites for piezoelectric sensors and gamma shielding applications that are low weight, elastic, affordable and have good gamma ray attenuation coefficients. The impact of SrTiO3/NiO on the structural characteristics of the PEO/PVA mixture is investigated. The polymer mixture PEO/PVA received additions of SrTiO3/NiO at concentrations of (0, 1, 2, 3 and 4) weight percent by the casting method. On the top surface of the films PEO/PVA/SrTiO3/NiO NCs, scanning electron microscopy reveals several randomly distributed aggregates or fragments that are consistent and coherent. An optical microscope image collection reveals that the blend*s additive distribution of NPs was homogenous. Gamma ray shielding application results show that the attenuation coefficient of PVA/PEO/SrTiO3/NiO NCs is increased by increasing concentration of SrTiO3/NiO nanoparticles. Radiation protection is another application for it. The pressure sensor application findings of NCs show that, when the applied pressure rises, electrical capacitance (Cp) increase.
In nature, the collective migration of bird flocks, the gathering migration of fish and cooperation of ant colonies are relatively common. The movement of biological groups has shown that, under conditions of limited environmental information and simple rules, the group system organizes itself into an orderly movement in which all individuals move at the same speed and maintain cohesion or geometric formation. Since the second half of the 20th century, the flocking behavior of multi-agent systems has attracted the attention of a large number of biologists, physicists and mathematicians. They take bird flocks as research objects and establish various flock models by observing and analyzing the activities of flock groups in real time (see [1,2,3,4]). The most influential and well-studied model is the Cucker–Smale model proposed by Cucker and Smale [1,2] in 2007. It is described as follows:
{˙xi=vi,˙vi=KNN∑j=1ϕ(‖xj−xi‖)(vj−vi),i=1,2,…,N, | (CS) |
where xi∈Rd denotes the position of the i-th particle and vi∈Rd stands for velocity. The parameter K>0 measures the alignment strength. The communication rate ϕ(⋅):[0,∞)→(0,∞) quantifies the influence between the i-th and j-th particles, and ‖⋅‖ denotes the Euclidean norm in Rd. The communication rate is represented as
ϕ(r)=1(1+r2)β. | (1.1) |
Note that unconditional flocking occurs if β<12, and conditional flocking occurs if β≥12. For more in-depth discussions, refer to [5,6]. Furthermore, unconditional flocking can also be obtained in the case of β=12 (see [7]). Recently, various modifications of the classical Cucker–Smale model have been proposed. For example, the Cucker–Smale model with hierarchical leadership (see [8,9,10,11,12,13], etc.), finite time and fixed time (see [14,15], etc.), random noise (see [9,10,11,12,16,17,18,19], etc.), communication failure (see [10,11,12,19,20,21], etc.), switching topologies (see [22,23], etc.) and time delay (see [5,17,24,25,26,27,28,29,30,31,32], etc.), and, for contributions in an overview, one can refer to [33,34].
In practical applications, time delays and communication failures are important factors that influence the dynamical behavior and stability of the system (see [35,36], etc.). This paper introduces some studies on the flocking of Cucker–Smale models with time delays. Liu and Wu first introduced the time-delay factor into the Cucker–Smale model in [30]. Based on this, Choi and Haškovec [27] studied the Cucker–Smale model with fixed transmission delay. However, their analysis is only valid for some re-normalized systems. Furthermore, the Cucker–Smale model with time-varying delays was studied in [31], where a strictly positive lower bound is assumed for ϕ. Erban, Haškovec and Sun [17] considered the randomness or imperfection of individual behavior and the delayed response of individuals to signals in the environment of the Cucker–Smale model. The numerical simulation results reveal that the introduction of time delays contributes to collective behaviors. Moreover, Haškovec and Markou [29] studied Cucker–Smale models with processing delays. They used the Lyapunov functional method, where the general communication function ϕ is considered and some assumptions are given. For other flocking results of delay Cucker–Smale systems, see [5,24,25,26,28,32], etc. In particular, Chen and Yin [25] studied the non-flocking and flocking behaviors of the Cucker–Smale model with time-varying delays and a short-range interaction rate. The model is as follows:
{˙xi=vi,˙vi=1N∑j≠iϕ(‖˜xj−˜xi‖)(˜vj−˜vi), | (1.2) |
where N∈N is the number of agents, (xi,vi)∈Rd×Rd denotes the phase-space coordinates of the i-th agent at the time t and d≥1 is the physical space dimension. Here, ˜xi:=xi(t−τ(t)) and ˜vi:=vi(t−τ(t)), and the variable time delay τ(t)≥0 is uniformly bounded, i.e., ˉτ:=supt≥0τ(t)<+∞. The authors of [25] generalized the results of [29] and obtained a flocking result for a more general communication rate, which also depends on the initial data in the short-range communication. This paper will present the application of some key inequalities and lemmas in [25] (see Lemma 2.1–2.2).
The agent's movement can be affected by obstacles, interference, noise, etc., so interaction failures are common. Martin et al. addressed interaction failures due to a limited communication range in [21]. The authors of [10] and [19] considered the hierarchical Cucker–Smale model under random interactions and the Cucker–Smale model with a random failure under root leadership. In these two studies, the random failures are independent and the probability of the interaction failure is fixed. Meanwhile, the overall leader or root leader of the group moves at a constant rate. Moreover, Mu and He presented a hierarchical Cucker–Smale model with time-varying failure probability under a random interaction and a Cucker–Smale model with random failures on a general graph in [11] and [12], respectively. Both [11] and [12] demonstrate that flocking behaviors often occur under certain conditions that depend exclusively on the initial state. However, the models with communication failures in the above literature are all discrete Cucker–Smale models and not continuous Cucker–Smale models. Recently, Bonnet and Flayac [20] studied continuous Cucker–Smale models with interaction failures. They emphasized that communication failures in the system are represented by time-varying interaction topologies, which differ from several other known contributions in the literature, such as [16,18,37]. The Cucker–Smale-type system with time-varying topological structures in [20] is shown below.
{˙xi=vi˙vi=1NN∑j=1ξij(t)ϕ(‖xi−xj‖)(vj−vi). | (1.3) |
where (x1,…,xN)∈(Rd)N and (v1,…,vN)∈(Rd)N respectively represent the positions and velocities of the agents, while ϕ(⋅) is a positive nonlinear kernel representing the extent of their mutual interactions. The functions ξij(⋅)∈L∞(R+,[0,1]) are communication weights that may lead to potential interaction failures in the system (e.g., when ξij(t)=0). The authors of [20] formulated a suitable persistence condition for the system (1.3), and they pointed out that a collection of weights (ξij(⋅)) satisfies the persistence condition (PE) (see Definition 2.3). The authors of [20] proved that the system (1.3) exhibits non-uniform exponential flocking with the strong fat tail condition (see Hypotheses (K)) in [20]) by constructing time-varying trajectory-based strict Lyapunov functions. In this paper, the strong fat tail condition of ϕ is improved, and the strong fat tail condition (1.6) is presented.
This paper focuses on the Cucker–Smale model with time-varying topological structures and reaction-type delay. Let xi(t)∈Rd and vi(t)∈Rd respectively be time-dependent position and velocity vectors of the i-th agent for i=1,2,…,N. Let ~ signify the value of a variable at time t−τ(t). For example, ˜xi=xi(t−τ(t)) and ˜vi:=vi(t−τ(t)), where the variable time delay τ(t)≥0 is uniformly bounded, i.e., ˉτ:=supt≥0τ(t)<+∞. Then, the delayed Cucker–Smale-type model studied in this paper is described by
{˙xi=vi,˙vi=1NN∑j=1˜ξij(t)ϕ(‖˜xj−˜xi‖)(˜vj−˜vi). | (1.4a) |
The initial position and velocity trajectories are prescribed for i=1,2,…,N:
(xi,vi)≡(x0i(t),v0i(t)),∀ t∈[−ˉτ,0], | (1.4b) |
with (x0i(t),v0i(t))∈C([−ˉτ,0];R2d). The communication rate ϕ quantifies the influence between the i-th particle and the j-th particle. The same as [20], the communication weight is no longer a fixed constant, but is represented by a time-varying communication weight ˜ξij(t). The communication weights ξij(t):[−ˉτ,+∞)→[0,1] are symmetric about the subscript, i.e., ξij(t)=ξji(t) for any i,j∈{1,2,…,N}. Obviously, ξij(t)=0 indicates that the communication between agent j and agent i is disconnected at time t.
This paper aims to provide sufficient conditions for the flocking behavior of Cucker–Smale systems with time-varying topological structures and reaction-type delay (see Theorem 3.1). For the case where no-delay occurs (i.e., ˉτ=0), the critical exponent of unconditional flocking in [20] is raised from β=14 to β=12 by replacing the strong fat tail condition with the weak light tail condition (1.6). For the case where no communication fails (i.e., ξij(t)≡1 for any i,j∈{1,2,…,N}), our result has a wider upper bound on the time delay than that in [25] (see Remark (3.1)). Finally, it is found that the introduction of time delay can deal with the problem of a low flocking convergence rate caused by the time-varying topology structure.
Below, the assumptions for ϕ=ϕ(r) and ξij(t) are presented.
Assumption 1.1. ϕ is bounded, positive, non-increasing and Lipschitz continuous on R+. Without loss of generality, we have
ϕ(r)≤1,∀ r≥0. | (1.5) |
Assumption 1.2. ϕ satisfies the {weak light tail} condition if there are two constants σ,κ>0 and a parameter η>12 such that
ϕ(r)≥κ(σ+r)2η,∀ r≥0. | (1.6) |
Assumption 1.3 (Haškovec and Markou [29]). Assume ϕ(r) is differentiable on R+; then, there is a constant α>0 such that
ϕ′(r)≥−αϕ(r),∀ r≥0. | (1.7) |
For the classical communication rate (1.1) in (CS), if σ=1,β≤η is chosen, Assumption 1.2 is verified; if α=2β, Assumption 1.3 is satisfied for all β≥0.
Remark 1.1. Assumption 1.2 implies two cases, namely, ϕ∈L1(R+,R+∗) if β=η>12 and ϕ∉L1(R+,R+∗) if β≤12, i.e., long-range communication weight and short-range communication weight.
Assumption 1.4. The communication weights (ξij(t)) are continuously differentiable over the interval [0,+∞), and there exists a constant γ>0 such that
ξ′ij(t)≥−γ | (1.8) |
for any t≥0 and i,j∈{1,2,…,N}.
Based on the above arguments, this paper mainly studies the asymptotic behavior of the model (1.4). The structure of the paper is as follows. In Section 2, some preliminary properties of the model (1.4) are established. Given important inequalities for position and velocity fluctuations, it is proved that the set of velocity fluctuations is uniformly bounded. In Section 3, the local time-dependent and global strict Lyapunov methods are respectively applied to prove the existence of a critical time delay for asymptotic flocking in the system (1.4). Finally, some numerical simulations are presented in Section 4.
In this section, the main tools and propositions used in this paper are introduced. For convenience, two notations of ϕ are given as follows:
ϕij(t):=ϕ(‖xi(t)−xj(t)‖),˜ϕij(t):=ϕ(‖˜xi(t)−˜xj(t)‖). |
Below, the model (1.4) is rewritten in a matrix form as
{˙x=v,˙v=−˜L(t,~x)~v,∀ t≥0. | (2.1a) |
(x(t),v(t))≡(x0(t),v0(t)),∀ t∈[−ˉτ,0], | (2.1b) |
where x=(x1,x2,…,xN) and v=(v1,v2,…,vN). ˜x and ˜v are similar. In addition, L:R×(Rd)N→RdN×dN is called the graph-Laplacian operator of the system (2.1). For almost every t≥0 and any v∈(Rd)N,
(L(t,x)v)i:=1NN∑j=1ξij(t)ϕij(t)(vi−vj), | (2.2) |
(˜L(t,~x)~v)i:=1NN∑j=1˜ξij(t)˜ϕij(t)(˜vi−˜vj). | (2.3) |
Further, for almost every t≥0 and any v∈(Rd)N, the partial graph-Laplacian operator Lξ:R→RdN×dN related to the weights (ξij(t)) is defined as
(Lξ(t)v)i=1NN∑j=1ξij(t)(vi−vj). | (2.4) |
This reformulation of multi-agent dynamics in terms of semi-linear ordinary differential equations in the phase space is fairly general and allows a comprehensive study of flocking problems via the Lyapunov method. With this goal, the so-called bilinear form of variance is introduced below.
Definition 2.1 (see section 1.2 in [38]). For any x,v∈(Rd)N, the variance bilinear form B:(Rd)N×(Rd)N→R is defined as:
B(x,v):=12N2N∑i,j=1⟨xi−xj,vi−vj⟩=1NN∑i=1⟨xi,vi⟩−⟨¯x,¯v⟩, |
where
¯x=1NN∑i=1xi,¯v=1NN∑i=1vi. |
Obviously, it is symmetric positive semi-definite and supports the following Cauchy-Schwarz inequality
B(x,v)≤√B(x,x)√B(v,v). | (2.5) |
For simplicity, this paper defines the position and velocity fluctuations as (t≥−ˉτ):
X(t):=√B(x(t),x(t));V(t):=B(v(t),v(t)), | (2.6) |
which can be evaluated based on the solutions of the system (2.1).
Note that any solution (x,v) of the model (2.1) satisfies
˙ˉx=ˉv,˙ˉv=0. |
This in turn implies that ˉv(t)=ˉv(0) and ˉx(t)=ˉx(0)+ˉv(0)t for any t≥0. Since the model (1.5a) is Galilean invariant, without loss of generality, it is assumed that ˉx=ˉv≡0; otherwise, xi−ˉx and vi−ˉv are replaced with xi and vi, respectively. The lead-up to this work has been to follow the above assumptions, no longer described. Note that it does not hold for t∈[−ˉτ,0) generally. Hence, for any t≥0,
X(t)=(1NN∑i=1‖xi(t)‖2)12,V(t)=1NN∑i=1‖vi(t)‖2. | (2.7) |
Now, this paper gives the definition of the asymptotic flocking behavior of the model (1.4) and our notion of presistence of excitation for the system (2.1), subject to multiplicative communication failures, respectively.
Definition 2.2. System (1.4) exhibits asymptotic flocking if the position and velocity fluctuations along the classical solution {(xi,vi)}Ni=1 of System (1.4) satisfy
supt≥0X(t)<∞,andlimt→∞V(t)=0. |
Definition 2.3 (see Definition 3 in [20]). The weight functions (ξij(⋅)) satisfy the presistence of excitation condition if there exists a pair (T,μ)∈R+∗×(0,1] such that, for all times t≥0 and every v∈(Rd)N,
B((1T∫t+TtLξ(s)ds)v,v)≥μB(v,v). | (PE) |
Remark 2.1. (PE) can be interpreted as a lower bound on the so-called algebraic connectivity (see [3,39,40]) of the average of the interaction graph with the weights (ξij(⋅)) over every time window of length T>0, because B(Lξv,v)B(v,v)>μ>0 cannot hold: set ξij=0; then, μ=0, which is a contradiction. The (2.8) requires the system to be persistently exciting in terms of the agents that have not yet reached flocking. Meanwhile, it only involves the communication weights (ξij(⋅)), not the kernel ϕ(⋅). For more characteristics and significance of the presistence of excitation condition, see Remark 1 and Section 4 in [20].
Next, two lemmas are introduced (see Lemma 2.3 and Remark 4 in [25]). To distinguish, this paper uses
V1(t):=(N∑i=1∑j≠i|vi(t)−vj(t)|2)12=√2N2V(t). |
For convenience, define that
k0:=maxs∈[−ˉτ,0]V1(s)V1(0),k=2‖ϕ‖∞k0. | (2.8) |
c=√4+4ˉτ3max{4,k20}. | (2.9) |
Lemma 2.1 (see Lemma 2.3 in [25]). Let 0≤ϕ∈Cb(R+) and V(0)≠0. Assume that ˉτ>0 satisfies ekˉτ≤2. Then, the classical solution {(xi,vi)}Ni=1 of the model (1.2) satisfies
V1(s)≤k0ek(t−s)V1(t),∀t>0,s∈[−ˉτ,0), |
and
V1(s)≤ek|t−s|V1(t),∀t,s≥0. |
Lemma 2.2 (see Remark 4 in [25]). Let 0≤ϕ∈Cb(R+) and V1(0)≠0. Assume that ˉτ>0 satisfies ekˉτ≤2; then, the classical solution {(xi,vi)}Ni=1 of the model (1.2) satisfies that
V1(t)≤cV1(0),∀t≥0. |
Lemma 2.3. Let V(0)≠0 and the communication ϕ satisfy Assumption 1. Assume that ˉτ>0 satisfies ekˉτ≤2; then, the classical solution {(xi,vi)}Ni=1 of the model (1.4) satisfies that
V(s)≤e2k|t−s|V(t),∀ t,s≥0; | (2.10a) |
V(s)≤k20e2k(t−s)V(t),∀ t≥0,s∈[−ˉτ,0); | (2.10b) |
and
˙X(t)a.e.≤√V(t)andV(t)≤c2V(0),∀ t≥0, | (2.11) |
where k0, k and c are defined as in (2.8) and (2.9), respectively.
Proof. This paper first proves the first part of (2.11). Taking the derivative of X2(t) along the solution curve of System (1.4), for almost every t≥0, according to (2.5), we have
2X(t)˙X(t)=dX2(t)dt=2B(x(t),v(t))≤2X(t)√V(t). |
Then, it is easy to obtain ˙X(t)≤√V(t) for almost every t≥0. Comparing our model (1.4) with the model (1.2), ξij(t)ϕ in the model (1.4) can be recorded as a whole, which satisfies the condition 0≤ϕ∈Cb(R+), and 2N2V(t)=V21(t). By Lemma 2.1 and Lemma 2.2, Lemma 2.3 is easy to be obtained.
For convenience, some notations are presented here. Most of them come from [20] and will be used in subsequent chapters.
Notation. The original function of ϕ(⋅) in [X(ˉτ),X] is defined as
Φ(X):=∫XX(ˉτ)ϕ(r)dr. | (2.12) |
This paper uses the rescaled interaction kernel defined by
ϕT(r):=ϕ(√2N(r+cT√V(0))), | (2.13) |
where any r≥0. Define ΦT(⋅) by its uniquely determined primitive that vanishes at X(ˉτ), i.e.,
ΦT(X):=∫XX(ˉτ)ϕT(r)dr. | (2.14) |
This paper introduces the time-state dependent family of ψT(t):R+→RdN×dN, which is defined by
ψT(t):=2TId−IT(t), | (2.15) |
where
IT(t)=1T∫t+Tt∫stL(σ,x(σ))dσds |
and Id denotes the identity matrix of (Rd)N. Its pointwise derivative is given explicitly by
˙ψT(t)=L(t,x(t))−1T∫t+TtL(s,x(s))ds. |
This paper also gives some symbolic marks that will appear in some conditions and conclusions, i.e.,
a1:=1T+4ekˉτ+4ˉτ2e2kˉτ; | (2.16a) |
a2:=T+2ˉτ3Tk−20e−4kˉτ; | (2.16b) |
a3:=2T+2ˉτ3Tk20e4kˉτ. | (2.16c) |
At the end of this section, a critical lemma depending on the (PE) is introduced, which is derived from [20].
Lemma 2.4. Let (x(⋅),v(⋅)) be a solution of the system (2.1). Assume that ˉτ>0 satisfies ekˉτ≤2. If (2.8) holds with (T,μ)∈R+∗×(0,1], then, for any t≥0, we have
B((1T∫t+TtL(s,x(s))ds)v,v)≥μϕT(X(t))B(v,v), | (2.17) |
where ϕT(⋅) is defined by (2.13).
Proof. By the definition (2.2) of L:R+×(Rd)N→RdN×RdN, we have
B((1T∫t+TtL(s,x(s))ds)v,v)=12N2N∑i,j=1(1T∫t+Ttξij(s)ϕij(s)ds)‖vi−vj‖2≥12N2N∑i,j=1(1T∫t+Ttξij(s)ϕ(√2NX(s))ds)‖vi−vj‖2, | (2.18) |
where ϕ(⋅) is non-increasing. As a consequence of (2.11) in Lemma 2.3, for all s∈[t,t+T], it further holds that
X(s)=X(t)+∫st˙X(σ)dσ≤X(t)+cT√V(0). |
According to (2.18) and the fact that ϕ(⋅) is non-increasing, we have
B((1T∫t+TtL(s,x(s))ds)v,v)≥ϕT(X(t))2N2N∑i,j=1(1T∫t+Ttξij(s)ds)‖vi−vj‖2=ϕT(X(t))B((1T∫t+TtLξ(s)ds)v,v)≥μϕT(X(t))B(v,v), |
where the definitions of Lξ(⋅) in (2.4), ϕT(⋅) in (2.13) and (PE) in the last inequality are used.
Finally, some common inequalities that will be used in the proofs of subsequent contents are presented as follows:
0≤B(L(t,x(t))v,v)≤B(v,v); | (2.19a) |
0≤B(IT(t)v,v)≤TB(v,v); | (2.19b) |
TB(v,v)≤B(ψT(t)v,v)≤2TB(v,v); | (2.19c) |
0≤B(L(t,x(t))v,L(t,x(t))v)≤2B(L(t,x(t))v,v); | (2.19d) |
0≤B(IT(t)v,IT(t)v)≤2T2B(v,v); | (2.19e) |
where (x,v) is the solution of the system (2.1) and all t≥0.
Proof. The left-hand side of the inequality (2.19) obviously holds, and it will be proved below that the right-hand side also holds. By Definition (2.2) of L(t,x(t))v and Assumption 1.1, we have
B(L(t,x(t))v,v)=12N2N∑i,j=1ξij(t)ϕij(t)‖vj−vi‖2≤12N2N∑i,j=1‖vj−vi‖2=B(v,v), |
and, thereby, (2.19a) holds. Combining (2.19a) and the linearity of the integral, the estimates (2.19b) can be easily derived:
B(IT(t)v,v)=1T∫t+Tt∫stB(L(σ,x(σ))v,v)dσds≤TB(v,v). |
Then, according to the definition (2.15) of ψT(t), we have (2.19c). Similarly, our estimate for the inequality (2.19d) is as follows:
B(L(t,x(t))v,L(t,x(t))v)≤1N2N∑i=1N∑j=1ξij2(t)ϕ2ij(t)‖vi−vj‖2≤1N2N∑i,j=1ξij(t)ϕij(t)‖vi−vj‖2=2B(L(t,x(t))v,v), |
where the discrete Cauchy-Schwarz inequality is used in the first inequality, and 0≤ξij(t)ϕij(t)≤1 in the second inequality. Next, by applying the integral Cauchy-Schwartz inequality, we have
B(IT(t)v,IT(t)v)≤∫t+Tt∫stB(L(σ,x(σ))v,L(σ,x(σ))v)dσds≤∫t+Tt∫st2B(L(σ,x(σ))v,v)dσds≤2T2B(v,v), |
where the second inequality uses (2.19d) and the third inequality uses (2.19b).
The previous section has shown some preparatory work. In the following section, it will be argued that the asymptotic flocking behavior for System (1.4) with both long-range and short-range communication rates.
Theorem 3.1. Let V(0)≠0 and Assumptions 1.1–1.4 and (PE) be satisfied by the communication rate ϕ and the communication weights (ξij(⋅)), respectively. If
ekˉτ≤2 | (3.1a) |
and
c√V(0)<μa3ΦT(+∞), | (3.1b) |
then there exist a radius ˉXM>0 and two positive constants αM,γM given by (3.51) such that the classical solutions {(xi,vi)}Ni=1 of the system (1.4) satisfy
X(t)≤ˉXM,∀ t≥0,V(t)≤αMe−γMt,∀ t≥ˉτ, |
where k, c, a3 and ΦT(⋅) are defined by (2.8), (2.9), (2.16c) and (2.14), respectively.
Remark 3.1. It is worthy to note that there are very few restrictions on the time delays, and that the convergence rate of flocking is related to the communication weights (ξij(⋅)) and time delay τ(t). Besides, the (PE) condition is independent of the initial configuration. When ϕ(r)=(1+r2)−β with β∈[0,12], the second part of Condition (3.1) is equivalent to c√V(0)<+∞, which obviously holds for any initial data. When ϕ(r)=(1+r2)−β with β=η>12, the system (1.4) may exhibit flocking behavior if the initial data satisfy Condition (3.1).
If ξij(t)≡1 for any i,j∈{1,2,…,N}, then the system (1.4) degenerates into the system (1.3). However, the time-delay in Theorem 3.1 requires only ekˉτ≤2, which weakens the time delay requirement (see Theorem 3.1 in [25]). If ˉτ=0, then the system (1.4) exhibits unconditionnal flocking and β=12 is a critical exponent, which improves the results of [20] and is consistent with the observations of the classic Cucker–Smale model (CS). Therefore, it is easy to get the following corollary.
Corollary 3.1. Let V(0)≠0 and Assumptions 1.1–1.2 and (PE) be satisfied by the communication rate ϕ and the communication weights (ξij(⋅)), respectively. If the condition (3.1b) is satisfied, then there exist a radius ˉXM>0 and two positive constants αM,γM given by (3.51) such that the classical solutions {(xi,vi)}Ni=1 of the system (1.3) satisfy
X(t)≤ˉXM,V(t)≤αMe−γ M t |
for all times t≥0. Additionally, k, c, a3 and ΦT(⋅) are defined by (2.8), (2.9), (2.16c) and (2.14), respectively.
Remark 3.2. When ˉτ=0, Assumptions 1.3–1.4 are unnecessary because the inequalities (3.27)–(3.28) obviously hold.
To prove Theorem 3.1, it is split into a series of lemmas. Let ψT(t) be defined as in (2.15), and consider the candidate Lyapunov functional defined by
LT(t):=λ(t)B(ψT(t)v(t),v(t))+4ˉτTλ(t)∫tt−ˉτ∫tθ˜V(s)dsdθ, | (3.2) |
where t≥ˉτ and λ(t) is a smooth differentiable tuning curve. λ(t) monotonically decreases with the positive lower bound, i.e., ˙λ(t)<0, λ(t)≥1.
Lemma 3.1. Let V(0)≠0 and Assumption 1.1 and (PE) be satisfied by the communication rate ϕ and the communication weights (ξij(⋅)), respectively. If the condition (3.1a) is verified, then, for the solution (x(⋅),v(⋅)) of the system (2.1) and every real number λˉτ≥1, there exists a time horizon Tλˉτ>0 such that
˙LT(t)≤−μϕT(X(t))V(t), | (3.3) |
where, for almost every time t∈[ˉτ,ˉτ+Tλˉτ].
Proof. Taking the derivative of LT(t) along the solution curve of System (2.1) on (ˉτ,∞), we have
˙LT(t)≤˙λ(t)TV(t)+λ(t)V(t)−μλ(t)ϕT(X(t))V(t)+4ˉτ2Tλ(t)e2kˉτV(t)−4ˉτTλ(t)∫tt−ˉτ˜V(s)ds−2λ(t)B(ψT(t)v,˜L(t,˜x)˜v), | (3.4) |
where the first inequality holds because ˙λ(t)<0, and (2.10a) and (2.19a) are used in the second inequality. Then, go further to a more refined estimate. By (2.15) and the definition of ψT(t), we have
−2λ(t)B(ψT(t)v,˜L(t,˜x)˜v)=−4Tλ(t)B(˜L(t,˜x)˜v,v)+2λ(t)B(IT(t)v,˜L(t,˜x)˜v)= I1+I2. |
Estimate I1 first:
I1=−4Tλ(t)B(˜L(t,˜x)˜v,v)=−4Tλ(t)B(˜L(t,˜x)˜v,˜v)−4Tλ(t)B(˜L(t,˜x)˜v,v−˜v). |
For the last term, this work uses (2.5), Young's inequality and Assumption 1.1, in turn,
|4Tλ(t)B(˜L(t,˜x)˜v,v−˜v)|≤4Tλ(t)√B(˜L(t,˜x)˜v,˜L(t,˜x)˜v)√B(v−˜v,v−˜v)≤2Tλ(t)(B(˜L(t,˜x)˜v,˜L(t,˜x)˜v)+B(v−˜v,v−˜v))≤2Tλ(t)(2B(˜L(t,˜x)˜v,˜v)+B(v−˜v,v−˜v)), | (3.5) |
where, following (1.4a) and the H¨older inequality, we have
B(v−˜v,v−˜v)=1NN∑i=1‖vi−˜vi‖2=1NN∑i=1‖∫tt−τ(t)˙vi(s)ds‖2=1NN∑i=1‖∫tt−τ(t)1NN∑j=1ξij(s)˜ϕij(s)(˜vj(s)−˜vi(s))ds‖2≤ˉτN3N∑i=1∫tt−τ(t)(N∑j=1ξij(s)˜ϕij(s)‖˜vj(s)−˜vi(s)‖)2ds≤ˉτN2∫tt−ˉτ(N∑i,j=1‖˜vj(s)−˜vi(s)‖2)ds=2ˉτ∫tt−ˉτ˜V(s)ds. | (3.6) |
Hence, combining (3.6) and (3.5), we have
I1≤4ˉτTλ(t)∫tt−ˉτ˜V(s)ds. | (3.7) |
Next, estimate I2, and, following from (2.5) and (2.10a), we obtain that
I2=2λ(t)B(IT(t)v,˜L(t,˜x)˜v)≤2λ(t)√B(IT(t)v,IT(t)v)√B(˜L(t,˜x)˜v,˜L(t,˜x)˜v)≤4Tλ(t)√V(t)˜V(t)≤4Tekˉτλ(t)V(t), | (3.8) |
where (2.19d) and (2.19e) are used in the second inequality. Combining (3.4) with (3.7) and (3.8), we have
˙LT(t)≤(T˙λ(t)+λ(t)+4Tekˉτλ(t)+4ˉτ2Tλ(t)e2kˉτ −μλ(t)ϕT(X(t)))V(t), | (3.9) |
where t>ˉτ. Choose the curve λ(⋅) as a solution of the ODE for a given constant λˉτ≥1:
˙λ(t)=−a1λ(t),λ(ˉτ)=λˉτ, |
where a1 is defined as that in (2.16a). The latter is uniquely determined and can be written explicitly as
λ(t)=λˉτe−a1(t−ˉτ) | (3.10) |
for any t∈[ˉτ,ˉτ+lnλˉτa1]. Let Tλˉτ=lnλˉτa1, and substituting the analysis expression (3.10) for the curve λ(⋅) into (3.9) yields that (3.3) holds for almost every time t∈[ˉτ,ˉτ+Tλˉτ].
Note that (3.3) involves both the variance V(⋅) and the Lyapunov functional LT(⋅). However, to prove Theorem 3.1, only the estimate of V(⋅) needs to be involved.
Lemma 3.2. Let V(0)≠0 and Assumption 1.1 and (PE) be satisfied by the communication rate ϕ and the communication weights (ξij(⋅)), respectively. If the conditions of (3.1) hold, then there is a mapping λˉτ∈[1,∞)↦XM(λˉτ)∈R+ along the solution (x(⋅),v(⋅)) of the system (2.1) such that
X(t)≤XM(λˉτ),∀t∈[ˉτ,ˉτ+Tλˉτ]. | (3.11) |
In particular, for every λˉτ≥1, the following local, strictly dissipative inequality holds:
V(ˉτ+Tλˉτ)≤a3λˉτa2V(ˉτ)exp(−μϕT(XM(λˉτ))Tλˉτλˉτa3), | (3.12) |
where a1,a2 and a3 are positive constants defined as those in (2.16), and Tλˉτ is defined in Lemma 3.1.
Proof. Choose λˉτ and let λ(⋅) denote the corresponding tuning functions given by (3.10). By (2.19c) and (2.10), for any solution (x(⋅),v(⋅)) of the system (2.1), we have
a2λ(t)V(t)≤LT(t)≤a3λ(t)V(t), |
where t∈[ˉτ,ˉτ+Tλˉτ]. Because 1≤λ(t)≤λˉτ, the following inequality holds:
a2V(t)≤LT(t)≤a3λˉτV(t), | (3.13) |
for any t∈[ˉτ,ˉτ+Tλˉτ]. Then, by (3.3), we have
˙LT(t)≤−μa3λˉτϕT(X(t))LT(t),∀ t∈(ˉτ,ˉτ+Tλˉτ). | (3.14) |
Now, this paper first proves the first part of Lemma 3.2. By integrating (3.3) on [ˉτ,t] for any t∈[ˉτ,ˉτ+Tλˉτ], we have
LT(t)≤LT(ˉτ)−μ∫tˉτϕT(X(s))V(s)ds, |
which, together with (3.13), yields
V(t)≤a3λˉτV(ˉτ)a2−μa2∫tˉτϕT(X(s))V(s)ds, | (3.15) |
where t∈[ˉτ,ˉτ+Tλˉτ]. Since ˙X(s)≤√V(s) and V(s)≤c2V(0) by (3.1a), applying the chance of variable r=X(s) in (3.15), the integral estimate can be obtained for all times t∈[ˉτ,ˉτ+Tλˉτ]:
V(t)≤a3λˉτV(ˉτ)a2−μc√V(0)a2∫X(t)X(ˉτ)ϕT(r)dr=a3λˉτV(ˉτ)a2−μc√V(0)a2ΦT(X(t)). | (3.16) |
Due to V(t)≥0, according to (3.16), for any t∈[ˉτ,ˉτ+Tλˉτ],
ΦT(X(t))≤a3λˉτμc√V(0)V(ˉτ). | (3.17) |
Since ϕ(⋅) is a non-increasing positive function, its primitive ΦT(⋅) is a strictly increasing map. Then, by (3.1b), there exists a constant XM(λˉτ) with XM(λˉτ)>X(ˉτ) such that
V(ˉτ)=μc√V(0)a3λˉτΦT(XM(λˉτ)). | (3.18) |
This paper claims that (3.11) holds. For contradiction, assume that there exists t∗∈[ˉτ,ˉτ+Tλˉτ] such that X(t∗)>XM(λˉτ). By the strict monotonically increasing property of ΦT(⋅), we have ΦT(X(t∗))>ΦT(XM(λˉτ)). Combining this with (3.17) and (3.18) implies
ΦT(XM(λˉτ))<ΦT(X(t∗))≤a3λˉτμc√V(0)V(ˉτ)=ΦT(XM(λˉτ)), |
which is a contradiction. Consequently, (3.11) holds.
Finally, this paper proves that (3.12) holds. Applying the Gronwall inequality to (3.14) with (3.11), we have
LT(t)≤LT(ˉτ)exp(−μa3λˉτϕT(XM(λˉτ))(t−ˉτ)) |
for all t \in \left[\bar{\tau}, \bar{\tau}+T_{\lambda_{\bar{\tau}}}\right]. This, together with (3.13), implies
\begin{gather} V(t)\le \frac{a_{3}\lambda_{\bar{\tau}}}{a_{2}} V(\bar{\tau})\exp \left( -\frac{\mu}{a_{3} \lambda_{\bar{\tau}}} \mathit{\boldsymbol{\phi}}_{T}(X_{M}(\lambda_{\bar{\tau}})) (t-{\bar{\tau}}) \right) \end{gather} | (3.19) |
for all t \in \left[\bar{\tau}, \bar{\tau}+T_{\lambda_{\bar{\tau}}}\right]. According to t = \bar{\tau}+T_{\lambda_{\bar{\tau}}} in (3.19), (3.12) holds immediately.
Using the dissipative inequality (3.12) obtained in Lemma 3.2, an upper bound on the standard deviation X(\cdot) can be obtained with respect to \lambda_{\bar{\tau}}\ge 1 .
Proposition 3.1. Let V(0) \neq 0 and Assumptions 1.1–1.2 and (PE) be satisfied by the communication rate \phi and the communication weights (\xi_{ij}(\cdot)) , respectively. If the conditions of (3.1) are satisfied, then there is a radius \bar{X}_{M} > 0 such that X(t) \le \bar{X}_{M} along the classical solution \left({{x\mathit{\boldsymbol{}}}}(\cdot), {{v\mathit{\boldsymbol{}}}}(\cdot)\right) of the system (2.1) for t \ge 0 .
Proof. From the expression of (3.18), we have
\mathit{\boldsymbol{\phi}}_{T}(X_{M}(\lambda_{\bar{\tau}})) = \mathit{\boldsymbol{\phi}}_{T} \circ {\boldsymbol \Phi}_{T}^{-1}\left(\frac{a_{3} \lambda_{\bar{\tau}}}{\mu c\sqrt{V(0)}} V(\bar{\tau}) \right), |
where " \circ " denotes the standard composition operation between functions. In addition, by integrating (1.7) with respect to r \in [X(\bar{\tau}), X] for some X > X(\bar{\tau}) , it also holds that
\Phi(X) \geq \frac{\kappa }{1-2\eta }\left((\sigma+X)^{1-\eta }-(\sigma+X(\bar{\tau}))^{1-2\eta }\right), |
which can be reformulated as
\begin{equation} X \leq \left(\frac{1-2\eta }{\kappa } \Phi(X)+(\sigma+X(\bar{\tau}))^{1-2\eta }\right)^{\frac{1}{1-2\eta }}-\sigma \end{equation} | (3.20) |
for every X \ge X(\bar{\tau}) . \Phi(\cdot) is defined in (2.12) to represent the primitive function of \phi(\cdot) . Next, applying variable substitution to (2.14) yields
\begin{align} \Phi(X) \le \sqrt{2}N {\boldsymbol \Phi}_{T}(X)+\Phi\left(\sqrt{2}N\left(X(\bar{\tau})+cT\sqrt{V(0)}\right)\right), \end{align} | (3.21) |
so that X: = X_{M}(\lambda_{\bar{\tau}}) = {\boldsymbol \Phi}_{T}^{-1}\left(\frac{a_{3} \lambda_{\bar{\tau}}}{\mu c\sqrt{V(0)}} V(\bar{\tau}) \right) . Recalling that {\mathit{\boldsymbol{\phi}}}_{T}(\cdot) is non-increasing, according to (3.20) and (3.21), and together with Assumption 1.2, we have
\begin{align} & \mathit{\boldsymbol{\phi}}_{T}(X_{M}(\lambda_{\bar{\tau}})) \\ \ge{}& \mathit{\boldsymbol{\phi}}_{T}\left(\left(\frac{1-2\eta }{\kappa } \Phi(X_{M}(\lambda_{\bar{\tau}}))+(\sigma+X(\bar{\tau}))^{1-2\eta }\right)^{\frac{1}{1-2\eta }}-\sigma\right) \\ \ge{}& \mathit{\boldsymbol{\phi}}_{T}\left(\frac{1-2\eta }{\kappa } \bigg(\sqrt{2}N{\boldsymbol\Phi}_{T}(X_{M}(\lambda_{\bar{\tau}}))+\Phi\bigg(\sqrt{2}N\left(X(\bar{\tau})\right.\right. \\ & \left.\left. +cT\sqrt{V(0)}\Big)\right) +\left((\sigma+X(\bar{\tau}))^{1-2\eta }\right)^{\frac{1}{1-2\eta }}-\sigma \right)\\ \ge{}& \mathit{\boldsymbol{\phi}}_{T}\left(\frac{1-2\eta }{\kappa } \bigg(\frac{\sqrt{2}N a_{3} \lambda_{\bar{\tau}}}{\mu c\sqrt{V(0)}} V(\bar{\tau})+\Phi\bigg(\sqrt{2}N\left(X(\bar{\tau})\right.\right. \\ & \left.\left. +cT\sqrt{V(0)}\Big)\right) +\left((\sigma+X(\bar{\tau}))^{1-2\eta }\right)^{\frac{1}{1-2\eta }}-\sigma \right)\\ \ge{}& \kappa\left(C_{1}+C_{2}\lambda_{\bar{\tau}}\right)^{\frac{2\eta }{2\eta -1}}, \end{align} | (3.22) |
where C_{1}, C_{2} < 0 only depend on \left(N, T, \mu, \bar{\tau}, k_{0}, \eta, \kappa \right). Substituting the inequality in (3.22) into (3.12) while recalling that T_{\lambda_{\bar{\tau}}} = \frac{\ln \lambda_{\bar{\tau}}}{a_{1}} , we have
\begin{align} &V(\bar{\tau}+T_{\lambda_{\bar{\tau}}}) \le V(\bar{\tau})\frac{a_{3} \lambda_{\bar{\tau}}}{a_{2}}\exp\left(-\frac{\mu\kappa T_{\lambda_{\bar{\tau}}}}{a_{3}\lambda_{\bar{\tau}}} \left(C_{1}+C_{2}\lambda_{\bar{\tau}}\right)^{\frac{2\eta }{2\eta -1}} \right) \\ \le{}& V(\bar{\tau})\frac{a_{3}}{a_{2}}\exp\left(a_{1}T_{\lambda_{\bar{\tau}}}-\frac{\mu\kappa T_{\lambda_{\bar{\tau}}}}{a_{3}}e^{-a_{1}T_{\lambda_{\bar{\tau}}}}\left(C_{1}+C_{2}\lambda_{\bar{\tau}}\right)^{\frac{2\eta }{2\eta -1}} \right) \\ \le{}& V(\bar{\tau})\frac{a_{3}}{a_{2}}\exp\left(\left(a_{1}-C_{3}e^{\frac{a_{1} T_{\lambda_{\bar{\tau}}}}{2\eta -1}} \right) T_{\lambda_{\bar{\tau}}} \right), \end{align} | (3.23) |
where C_{3} = \frac{\mu \kappa}{a_{3}}{C_{2}^{\frac{2\eta }{2\eta -1}}} > 0. Observe that \lambda_{\bar{\tau}} is free over the interval [1, +\infty) and T_{\lambda_{\bar{\tau}}} is a continuous monotone increasing function of \lambda_{\bar{\tau}} \in [1, +\infty) . Since T_{\lambda_{\bar{\tau}}} spans [0, +\infty) , a time reparametrization can be defined by using S: = \bar{\tau}+T_{\lambda_{\bar{\tau}}} . Then, by (3.23) and (2.11), this new time variable can be expressed as
\begin{align*} X(t) \leq &X(\bar{\tau})+\int_{\bar{\tau}}^{t}\sqrt{V(S)} \, \mathrm{d} S \\\leq &X(\bar{\tau})+\int_{0}^{t-\bar{\tau}}\sqrt{V(\bar{\tau})\frac{a_{3}}{a_{2}}}\exp\left(\left(a_{1}-C_{3}e^{\frac{a_{1}S}{2\eta -1}}\right) \frac{S}{2}\right) \, \mathrm{d} S \end{align*} |
for any t\ge\bar{\tau} . The integral on the right-hand side is uniformly bounded because of \eta > \frac{1}{2} . Thus, there exists a constant \bar{X}_{M} > 0 such that X(t) \le \bar{X}_{M} for all t \ge 0 , which proves our claim.
For convenience, it is defined that
\begin{gather} D(t) = B\left(\mathit{\boldsymbol{L}}(t, x(t))v(t), v(t)\right), \end{gather} | (3.24) |
\begin{gather} \widetilde{D}(t) = B\left(\widetilde{\mathit{\boldsymbol{L}}}(t, \widetilde{x}(t))\widetilde{v}(t), \widetilde{v}(t)\right), \end{gather} | (3.25) |
\begin{gather} \hat{k} = \gamma +\alpha c N \sqrt{2V(0)} + 2ke^{k\bar{\tau}}. \end{gather} | (3.26) |
Here \alpha , \gamma and c are defined in (1.6), (1.8) and (2.11), respectively. Now, this paper provides a mutual-direction estimate for the quantum D(t) defined in (3.25).
Lemma 3.3. Let V(0)\neq 0 and Assumptions 1.1, 1.3 and 1.4 be satisfied by the communication rate \phi and the communication weights \xi_{ij}(t) , respectively. If (3.1a) holds, then the solution \left({{x\mathit{\boldsymbol{}}}}(\cdot), {{v\mathit{\boldsymbol{}}}}(\cdot)\right) of the system (2.1) satisfies that
\begin{align} D(t)+\frac{\hat{k}}{2k}\left(1-e^{2k(s-t)}\right)V(t) &\le D(s) \\ &\le D(t)+\frac{\hat{k}}{2k}\left(e^{2k(t-s)}-1\right)V(t) \end{align} | (3.27) |
for all t, s \ge 0 . In particular, we have
\begin{align} D(t) + \frac{\hat{k}}{2k}\left(1-e^{-2k\bar{\tau}}\right)V(t) & < \widetilde{D}(t)\\ & < D(t) + \frac{\hat{k}}{2k}\left(e^{2k\bar{\tau}}-1\right) V(t) \end{align} | (3.28) |
for all t \ge \bar{\tau} .
Proof. The proof is completed in two steps. In the first step, the inequality is proved:
\begin{align} \frac{\mathrm{d} D(t)}{\mathrm{d} t} \ge -\hat{k} V(t), \quad \forall\ t \ge 0. \end{align} | (3.29) |
For any t\ge 0 , D(t) is differentiated in time:
\begin{align} \frac{\mathrm{d} D(t)}{\mathrm{d} t} = &\frac{\mathrm{d}}{\mathrm{d} t} \left(\frac{1}{2N^{2}}\sum\limits_{i, j = 1}^{N} \xi_{ij}(t)\phi_{ij}(t)\left \|v_{i}-v_{j} \right \|^{2}\right)\\ = &\frac{1}{2N^{2}}\sum\limits_{i, j = 1}^{N} \xi_{ij}'(t)\phi_{ij}(t)\left \|v_{i}-v_{j} \right \|^{2}\\&+ \frac{1}{N^{2}}\sum\limits_{i, j = 1}^{N} \xi_{ij}(t) \phi_{ij}(t) \left \langle v_{i}-v_{j}, \dot{v}_{i}-\dot{v}_{j} \right \rangle\\&+ \frac{1}{2N^{2}}\sum\limits_{i, j = 1}^{N} \xi_{ij}(t)\phi_{ij}'(t) \left \langle \frac{x_{j}-x_{i}}{\|x_{j}-x_{i} \Vert }, v_{j}-v_{i} \right \rangle \left \|v_{i}-v_{j} \right \|^{2}. \end{align} | (3.30) |
By Assumption 1.1 and Assumption 1.4, \xi_{ij}'(t) \ge -\gamma for t \ge 0 ; for the first term on the right-hand side of (3.30), we have
\begin{equation} \frac{1}{2N^{2}}\sum\limits_{i, j = 1}^{N} \xi_{ij}'(t)\phi_{ij}(t)\left \|v_{i}-v_{j} \right \|^{2} \ge -\gamma V(t), \end{equation} | (3.31) |
where (2.6) is used in the last equality. For the second term on the right-hand side of (3.30), the symmetrization trick is applied:
\begin{align*} &\sum\limits_{i, j = 1}^{N} \xi_{ij}(t) \phi_{ij}(t) \left \langle v_{i}-v_{j}, \dot{v}_{i}-\dot{v}_{j} \right \rangle \\ = {}& 2\sum\limits_{i, j = 1}^{N} \xi_{ij}(t) \phi_{ij}(t) \left \langle v_{i}-v_{j}, \dot{v}_{i}\right \rangle. \end{align*} |
Then, the term is estimated by using the Cauchy-Schwartz inequality:
\begin{align} & \frac{2}{N^{2}}\sum\limits_{i, j = 1}^{N} \xi_{ij}(t) \phi_{ij}(t) \left \langle v_{i}-v_{j}, \dot{v}_{i}\right \rangle \\ = {}& -\frac{2}{N^{2}}\sum\limits_{i = 1}^{N} \left \langle \sum\limits_{j = 1}^{N}\xi_{ij}(t) \phi_{ij}(t)(v_{j}-v_{i}), \dot{v}_{i}\right \rangle \\ \ge{}& -\frac{2}{N^{3}} \sum\limits_{i = 1}^{N} \left\lVert \sum\limits_{j = 1}^{N} (v_{j}-v_{i})\right\rVert \left\lVert \sum\limits_{j = 1}^{N} (\widetilde{v}_{i}-\widetilde{v}_{j}) \right\rVert \\ \ge{}& -\frac{2}{N^{2}} \sum\limits_{i = 1}^{N}\left(\sqrt{\sum\limits_{j = 1}^{N} \| v_{i}-v_{j}\Vert ^{2}}\right) \left(\sqrt{\sum\limits_{j = 1}^{N}\| \widetilde{v}_{i}-\widetilde{v}_{j}\Vert ^{2}}\right) \\ \ge{}& -\frac{2}{N^{2}} \sqrt{\sum\limits_{i, j = 1}^{N} \| v_{i}-v_{j}\Vert ^{2}} \sqrt{\sum\limits_{i, j = 1}^{N}\| \widetilde{v}_{i}-\widetilde{v}_{j}\Vert ^{2}} \\ \ge{}& -4\sqrt{V(t)\widetilde{V}(t)} \ge -2ke^{k\bar{\tau}}V(t), \end{align} | (3.32) |
where \xi_{ij}(t) \le 1, \, \phi(t) \le 1 is used. In the last inequality, (2.10a) and (2.10b) in Lemma 2.3 are applied. By Assumption 1.3, for the third term on the right-hand side of (3.30), we have
\begin{align} &{}\frac{1}{2N^{2}}\sum\limits_{i, j = 1}^{N} \xi_{ij}(t)\phi_{ij}'(t) \left \langle \frac{x_{j}-x_{i}}{\|x_{j}-x_{i} \Vert }, v_{j}-v_{i} \right \rangle \left \|v_{i}-v_{j} \right \|^{2} \\ \ge{}& -\frac{\alpha}{2N^{2}} \sum\limits_{i, j = 1}^{N} \xi_{ij}(t)\phi_{ij}(t) \| v_{j}-v_{i}\Vert \left \|v_{i}-v_{j} \right \|^{2}\\ \ge{}& -\frac{\alpha c\sqrt{2V(0)}}{2N} \sum\limits_{i, j = 1}^{N} \left \|v_{i}-v_{j} \right \|^{2}\\ = {}& -\alpha cN \sqrt{2V(0)} V(t), \end{align} | (3.33) |
where, in the second inequality, the bound provided by (2.12) in Lemma 2.3 is used:
\| v_{j}-v_{i}\Vert \le \sqrt{2N^{2}V(t)} = N\sqrt{2V(t)}. |
By combining the estimates (3.31)–(3.33), we get (3.29).
In the second step, this paper proves (3.27) and (3.28). Integrating (3.29) from s to t yields
\begin{align} D(s) \le D(t)+\hat{k}\int_{s}^{t} V(\sigma ) \, \mathrm{d} \sigma, \quad \forall\ t, s \ge 0. \end{align} | (3.34) |
This paper claims that, for any t, s \ge 0 ,
\begin{align} \int_{s}^{t} V(\sigma ) \, \mathrm{d} \sigma \le \frac{1}{2k} \left(e^{2k(t-s)}-1\right)V(t), \end{align} | (3.35) |
\begin{align} \int_{s}^{t} V(\sigma ) \, \mathrm{d} \sigma \le \frac{1}{2k} \left(e^{2k(t-s)}-1\right)V(s). \end{align} | (3.36) |
In fact, by (2.10a), we have
\begin{align} \int_{s}^{t} V(\sigma ) \, \mathrm{d} \sigma \le \int_{s}^{t} e^{2k(t-\sigma )}V(t) \, \mathrm{d} \sigma \le \frac{1}{2k} \left(e^{2k(t-s)}-1\right)V(t), \\ \forall\, t \ge s \ge 0, \end{align} | (3.37) |
\begin{align} \int_{s}^{t} V(\sigma ) \, \mathrm{d} \sigma \le \int_{s}^{t} e^{-2k(\sigma-t )}V(t) \, \mathrm{d} \sigma \le \frac{1}{2k} \left(e^{2k(t-s)}-1\right)V(t), \\ \forall\, s \ge t \ge 0. \end{align} | (3.38) |
Combining (3.37) and (3.38) gives (3.35), and (3.36) can be obtained in the same way. According to (3.35) and (3.36), for any t, s \ge 0 , (3.34) can be scaled to
\begin{align} D(s) \le D(t) + \frac{\hat{k}}{2k} \left(e^{2k(t-s)}-1\right)V(t), \end{align} | (3.39) |
\begin{align} D(s) \le D(t) + \frac{\hat{k}}{2k} \left(e^{2k(t-s)}-1\right)V(s), \end{align} | (3.40) |
where (3.39) is the right-hand side of (3.27). Next, exchanging s with t in (3.40), we have
\begin{align} D(s) \ge D(t) + \frac{\hat{k}}{2k} \left(1-e^{2k(s-t)}\right)V(t), \quad \forall\, t, s \ge 0. \end{align} | (3.41) |
Combining (3.39) and (3.41), we obtain (3.27). In particular, let s = t-\tau(t) in (3.27), which gives (3.28).
Based on the uniform estimate obtained in Proposition 3.1, we shall prove the second part of Theorem 3.1.
Proof of Theorem 3.1. Since X(\cdot) is uniformly bounded in Proposition 3.1, this paper only needs to prove that the exponential convergence of V(\cdot) is toward 0. Combining (2.17) and Proposition 3.1, recalling that {\mathit{\boldsymbol{\phi}}}_{T}(\cdot) is non-increasing, we have
\begin{equation} B \left( \left( \frac{1}{T}\int_t^{t + T} {\mathit{\boldsymbol{L}}}(s, {{x\mathit{\boldsymbol{}}}}(s))ds \right){{v\mathit{\boldsymbol{}}} }, {{v\mathit{\boldsymbol{}}}} \right) \ge \mu{\mathit{\boldsymbol{\phi}}_{T}}(\bar{X}_{M}) B({v\mathit{\boldsymbol{}}} , {v\mathit{\boldsymbol{}}} ) \end{equation} | (3.42) |
for any t \ge 0 , where \mathit{\boldsymbol{\phi}}_{T}(\cdot) is defined by (2.13).
Let \psi_{T}(t) be defined as that in (2.15), and consider the Lyapunov functional defined by
\begin{equation} \begin{aligned} \mathfrak{L}_{T}(t) : = & {\lambda} V(t) + B\left(\psi_{T}(t)\mathit{\boldsymbol{v}}, \mathit{\boldsymbol{v}} \right) \\ &+ \left(4\lambda + 2T\right)\bar{\tau} \int_{t-\bar{\tau}}^{t} \int_{\theta }^{t} \widetilde{D}(s) \, \mathrm{d} s \, \mathrm{d} \theta \end{aligned} \end{equation} | (3.43) |
for all times t \ge \bar{\tau} , where \lambda > 0 is a tuning parameter and (\mathit{\boldsymbol{x}}(t), \mathit{\boldsymbol{v}}(t)) solves (2.1). Note that, by (2.19) and (2.10), we have
\begin{gather} a_{4}V(t) \le \mathfrak{L}_{T}(t) \le a_{5}V(t) \end{gather} | (3.44) |
for all times t \ge \bar{\tau} , where a_{4} = \lambda + T and a_{5} = \lambda+2T+(2\lambda+T)\bar{\tau}^{3}e^{4k\bar{\tau}} . Now, this paper proves that the following strictly dissipative inequality holds for t \ge \bar{\tau} :
\dot{\mathfrak{L}}_{T}(t) \le -\gamma_{M} {\mathfrak{L}}_{T}(t), |
where \gamma_{M} > 0 is a given constant. With this goal in mind, this paper takes the derivative of \mathfrak{L}_{T}(t) along the solution curve of the system (2.1) on \left(\bar{\tau}, \infty \right) :
\begin{equation} \begin{aligned} \dot{\mathfrak{L}}_{T}(t) \le&-(2\lambda+4T)B \left (\widetilde{\mathit{\boldsymbol{L}}}(t, \widetilde{\mathit{\boldsymbol{x}}}) \widetilde{\mathit{\boldsymbol{v}}}, \mathit{\boldsymbol{v}} \right)-\mu{\mathit{\boldsymbol{\phi}}_{T}}(\bar{X}_{M})V(t)\\ &+ 2B \left ( \mathit{\boldsymbol{\mathcal{I}}} _{T}(t) \mathit{\boldsymbol{v}} , \widetilde{\mathit{\boldsymbol{L}}}(t, \widetilde{\mathit{\boldsymbol{x}}}) \widetilde{\mathit{\boldsymbol{v}}} \right)+(4\lambda +8T)\bar{\tau}^{2} \widetilde{D}(t)\\ &+D(t)-(4\lambda +8T)\bar{\tau} \int_{t-\bar{\tau}}^{t} \widetilde{D}(s) \, \mathrm{d} s, \end{aligned} \end{equation} | (3.45) |
where (3.42) is used. This work uses Young's inequality with \delta = 2 and the inequality (2.19d):
\begin{align} &{}B \left(\widetilde{\mathit{\boldsymbol{L}}}(t, \widetilde{\mathit{\boldsymbol{x}}}) \widetilde{\mathit{\boldsymbol{v}}}, \mathit{\boldsymbol{v}} \right) \\ \ge{}& \widetilde{D}(t)-\left| B \left(\widetilde{\mathit{\boldsymbol{L}}}(t, \widetilde{\mathit{\boldsymbol{x}}}) \widetilde{\mathit{\boldsymbol{v}}}, \mathit{\boldsymbol{v}}-\widetilde{\mathit{\boldsymbol{v}}} \right) \right\vert \\ \ge{}& \widetilde{D}(t)- \sqrt{B \left (\widetilde{\mathit{\boldsymbol{L}}}(t, \widetilde{\mathit{\boldsymbol{x}}}) \widetilde{\mathit{\boldsymbol{v}}}, \widetilde{\mathit{\boldsymbol{L}}}(t, \widetilde{\mathit{\boldsymbol{x}}}) \widetilde{\mathit{\boldsymbol{v}}} \right)} \sqrt{B \left ({\mathit{\boldsymbol{v}}}-\widetilde{\mathit{\boldsymbol{v}}}, {\mathit{\boldsymbol{v}}}-\widetilde{\mathit{\boldsymbol{v}}}\right)}\\ \ge{}& \widetilde{D}(t)- \frac{1}{4}B \left (\widetilde{\mathit{\boldsymbol{L}}}(t, \widetilde{\mathit{\boldsymbol{x}}}) \widetilde{\mathit{\boldsymbol{v}}}, \widetilde{\mathit{\boldsymbol{L}}}(t, \widetilde{\mathit{\boldsymbol{x}}}) \widetilde{\mathit{\boldsymbol{v}}} \right) - B \left ({\mathit{\boldsymbol{v}}}-\widetilde{\mathit{\boldsymbol{v}}}, {\mathit{\boldsymbol{v}}}-\widetilde{\mathit{\boldsymbol{v}}} \right)\\ \ge{}& \frac{1}{2}\widetilde{D}(t) - 2 \bar{\tau}\int_{t-\bar{\tau}}^{t} \widetilde{D}(s) \, \mathrm{d} s. \end{align} | (3.46) |
The second inequality in (3.46) is the Cauchy-Schwartz inequality (2.5), and the last term is given by (3.6). According to (3.8), we have
\begin{equation} \begin{aligned} &{}B \left ( \mathit{\boldsymbol{\mathcal{I}}} _{T}(t) \mathit{\boldsymbol{v}} , \widetilde{\mathit{\boldsymbol{L}}}(t, \widetilde{\mathit{\boldsymbol{x}}}) \widetilde{\mathit{\boldsymbol{v}}} \right)\\ \le{}& \sqrt{B \left ( \mathit{\boldsymbol{\mathcal{I}}} _{T}(t)\mathit{\boldsymbol{v}} , \mathit{\boldsymbol{\mathcal{I}}} _{T}(t) \mathit{\boldsymbol{v}} \right) } \sqrt{B \left ( \widetilde{\mathit{\boldsymbol{L}}}(t, \widetilde{\mathit{\boldsymbol{x}}}) \widetilde{\mathit{\boldsymbol{v}}}, \widetilde{\mathit{\boldsymbol{L}}}(t, \widetilde{\mathit{\boldsymbol{x}}}) \widetilde{\mathit{\boldsymbol{v}}} \right) }\\ \le{}& 2 T \sqrt{V(t) \widetilde{D}(t)} \le T\epsilon V(t)+\frac{T}{\epsilon}\widetilde{D}(t) , \end{aligned} \end{equation} | (3.47) |
where (2.19d) is used in the second inequality and Young's inequality with \epsilon > 0 is used in the last inequality. Substituting (3.46) and (3.47) into the inequality (3.45), we have
\begin{equation} \begin{aligned} \dot{\mathfrak{L}}_{T}(t) \le& -\left(\mu{\mathit{\boldsymbol{\phi}}_{T}}(\bar{X}_{M})-2T\epsilon \right)V(t) + D(t)\\ &+ \left(\frac{2T}{\epsilon}+4\lambda \bar{\tau}^{2}-\lambda \right)\widetilde{D}(t) \end{aligned} \end{equation} | (3.48) |
for any t \ge \bar{\tau} and given \lambda, \epsilon > 0 . Below, for the right side of (3.48), this paper uses (3.1a) and (3.28):
\begin{align*} \dot{\mathfrak{L}}_{T}(t) \le& -\left(\mu{\mathit{\boldsymbol{\phi}}_{T}}(\bar{X}_{M})-2T\epsilon+\frac{\hat{k}}{2k}\left(1-e^{-2k\bar{\tau}}\right) \right)V(t) \\ &+ \left(\frac{2T}{\epsilon}+4\lambda \bar{\tau}^{2}-\lambda + 1\right)\widetilde{D}(t). \end{align*} |
Therefore, the following parameters are chosen:
\begin{align} \epsilon = \frac{\hat{k}\left(1-e^{-2k\bar{\tau}}\right)}{4Tk}+\frac{\mu{\mathit{\boldsymbol{\phi}}_{T}}(\bar{X}_{M})}{4T} \quad \text{and} \quad \lambda = \frac{\frac{2T}{\epsilon}+1}{1-4{\bar{\tau}}^{2}}. \end{align} | (3.49) |
According to the condition (3.1a) in Theorem 3.1, we have \epsilon, \lambda > 0 . Using the inequality (3.44), the following inequality can be obtained:
\begin{align*} \dot{\mathfrak{L}}_{T}(t) \le -\frac{\mu{\mathit{\boldsymbol{\phi}}_{T}}(\bar{X}_{M})}{2}V(t) \le -\frac{\mu{\mathit{\boldsymbol{\phi}}_{T}}(\bar{X}_{M})}{2a_{5}}{\mathfrak{L}}_{T}(t), \end{align*} |
for any t \ge \bar{\tau} . By applying the Gronwall inequality together with (3.44), we have
\begin{align} V(t) \le \alpha_{M}e^{-\gamma_{M}t} \end{align} | (3.50) |
for all times t\ge\bar{\tau} , where \alpha_{M}, \gamma_{M} > 0 are given by
\begin{gather} \alpha_{M} = \frac{a_{5}}{a_{4}}V(\bar{\tau})\exp\left({\frac{\mu\bar{\tau}{\mathit{\boldsymbol{\phi}}_{T}}(\bar{X}_{M})}{2a_{5}}}\right), \quad \gamma_{M} = \frac{\mu{\mathit{\boldsymbol{\phi}}_{T}}(\bar{X}_{M})}{2a_{5}}, \end{gather} | (3.51) |
with \lambda > 0 as that in (3.49). By combining Proposition 3.1 and (3.50), it is concluded that the solution \left({{x\mathit{\boldsymbol{}}}}(\cdot), {{v\mathit{\boldsymbol{}}}}(\cdot)\right) of the system (2.1) converges to flocking with an exponential rate in the velocity variable.
Remark 3.3. Assumptions 1.3–1.4 cannot be removed because it provides a key inequality (3.28) that is needed in the time-delay system (1.4), which is a restriction of Theorem 3.1. However, if \bar{\tau} = 0 , then Assumptions 1.3–1.4 can be removed because the inequalities (3.27)–(3.28) obviously hold.
In this section, numerical simulation examples of the dynamic behavior are provided for the system (1.4) with regard to particle positions and velocities under Condition (3.1). The flocking behavior in Section 3 is simulated and the effect of interaction failure and time lag on the flocking convergence rate is analyzed.
First, for the convenience of the following discussion, two functions and their respective images are given. These two functions are inspired by the forced harmonic vibration equation for any k \in \mathbb{N} :
\begin{align} f(t)& = \begin{cases} \sin ^{2} t, & \text{ if } t \in [2k\pi , (2k+\frac{1}{k+1})\pi); \notag\\ 0, & \text{ if } other. \end{cases} \end{align} | (4.1) |
\begin{align} g(t)& = \begin{cases} \sin ^{2} t, & \text{ if } t \in [2k\pi , (2k+1)\pi); \notag\\ 0, & \text{ if } other. \end{cases} \end{align} | (4.2) |
The images are shown in Fig. 1, which shows that the continuous zero set E_{k}\left(t:f(t) = 0, t \in [2k\pi, 2(k+1)\pi)\right) increases over time, while the continuous zero set F_{k}\left(t: g(t) = 0, t \in [2k\pi, 2(k+1)\pi)\right) maintains \pi units over time.
Example 4.1. Let N = 10 , \phi(r) = \frac{1}{(1+r)^{\beta}} , \tau(t) = \frac{1}{4} \sin t and \xi_{ij}(t) = g(t) for any i, j \in \{ 1, 2, \ldots, N\} , which satisfies the (PE) condition because T = 2\pi and \mu \leq \frac{1}{4} are chosen; we have
\begin{align*} \frac{1}{T} \int_{t}^{t+T} \xi_{ij}(s) \, ds = \frac{1}{T} \int_{0}^{\pi} \sin^{2} s \, ds \geq \mu \end{align*} |
for any t \ge 0 and i, j \in \{ 1, 2, \ldots, N\} . For the initial data, {{x\mathit{\boldsymbol{}}}}(t) and {{v\mathit{\boldsymbol{}}}}(t) are set to be constant for t \in [-\bar{\tau}, 0] . By a direct calculation, \bar{\tau} = \frac{1}{4} , k_0 = 1 , c = \sqrt{17} / {2} , a_3 = 4\pi+\frac{\pi e^2}{16} and e^{k\bar{\tau}} = e^{\frac{1}{2}}\thickapprox 1.65 < 2 are obtained. Moreover, if \beta = \frac{1}{2} , then \mathit{\boldsymbol{\Phi}}_{T}(+\infty) = +\infty , which implies that Condition (3.1) in Theorem 3.1 holds. So, according to Theorem 3.1, the flocking occurs. We set the initial position
{{x\mathit{\boldsymbol{}}}}(0)\equiv (0.1, -0.2, 0.3, -0.4, 0.5, -0.6, 0.7, -0.8, 0.9, -1), |
and the initial velocity
{{v\mathit{\boldsymbol{}}}}(0)\equiv (-0.1, 0.2, -0.3, 0.4, -0.5, 0.6, -0.7, 0.8, -0.9, 1). |
As described in Fig. 2, numerical simulations confirm that position fluctuations are uniformly bounded and velocity fluctuations converge exponentially to zero.
If \beta = 1 , we set the initial position
{{x\mathit{\boldsymbol{}}}}(0)\equiv (1, -2, 3, -4, 5, -6, 7, -8, 9, -10) \times 10^{-4}, |
and the initial velocity
{{v\mathit{\boldsymbol{}}}}(0)\equiv (-1, 2, -3, 4, -5, 6, -7, 8, -9, 10)\times 10^{-4}. |
Then, V(0) = X(0) = 3.825 \times 10^{-7} and X(0) > X(\bar{\tau}) , which can be seen from Fig. 3(a). Thus, we have
c\sqrt{V(0)}\approx 0.00127 < 0.00184 \approx \int_{X(0)}^{+\infty} \mathit{\boldsymbol{\phi}}_{T}(r) \, dr < \mathit{\boldsymbol{\Phi}}_{T}(+\infty), |
which implies that Condition (3.1) in Theorem 3.1 holds. The numerical simulations are shown in Fig. 3. The results confirm that position fluctuations are uniformly bounded, and that velocity fluctuations converge exponentially to zero, indicating that the system 1.4 exhibits flocking behavior.
It can be seen in Figs. 2 and 3 that the relative velocity between particles is stepped attenuation, and that it finally converges to zero, which is guaranteed by the selection of communication weights satisfying the (PE) condition. Next, another communication weight function is selected, which does not meet the (PE) condition, to analyze the flocking behavior of the system (1.4).
Example 4.2. Let \xi_{ij}(t) = f(t) for any i, j \in \{ 1, 2, \ldots, N\} ; the remaining parameters and variables are the same as those in Example 1. Obviously, \xi_{ij}(t) does not satisfy the (2.8) condition since \lim_{t \to \infty} f(t) = 0. The numerical simulations are shown in Figs. 4 and 5. It can be seen that the relative positions between the particles are unbounded. Although the relative velocity also decreases in a step-like manner, it does not converge to zero in the end but maintains a uniform velocity. Therefore, when the system 1.4 encounters a communication fault with the communication weight f(t) , the flocking behavior will fail.
Example 4.3. To analyze the influence of the time-varying topological structure and time lag \tau(t) on the flocking convergence rate, this paper investigates the evolution of velocity fluctuations with time for four different situations. \xi_{ij}(t) = g(t) for any i, j \in \{ 1, 2, \ldots, N\} and \tau(t) = \frac{1}{4} \sin t is the first case; \xi_{ij}(t) \equiv 1 and \tau(t) = \frac{1}{4} \sin t is the second case; \xi_{ij}(t) = g(t) for any i, j \in \{ 1, 2, \ldots, N\} and \tau(t) = 0 is the third case; \xi_{ij}(t) \equiv 1 and \tau(t) = 0 is the fourth case. They are respectively represented in Fig. 6 by a red solid line, a blue dashed line, a magenta dashed line and a green dashed line. Other configurations were chosen in the same way as in Example 4.1. Figure 6 illustrates the numerical simulation, where (a) and (b) represent the flocking convergence rate for long-range communication and short-range communication, respectively. It can be seen that the communication failure caused by the time-varying topological structure slows down the flocking convergence in both situations, whereas the small delay speeds up the flocking convergence. So, it is advantageous to introduce time delays to the general Cucker–Smale system with the time-varying topological structure, which allows the system to initiate flocking more quickly.
The phenomena of communication failure and time delay are often observed in nature. This paper mainly studies the influence of communication failures caused by a time-varying topology and reaction-type delay on the flocking behavior of multi-particle systems. Two main problems are discussed in this paper. One is as follows: what conditions do time-varying topologies need to meet to avoid communication failure without affecting the final flocking behavior? The other is the question of how the influence of time delay affects the flocking behavior of time-varying systems? This paper gives the corresponding (PE) condition for the first problem. Under this condition, the flocking behavior of the Cucker–Smale system (1.3) with a time-varying topological structure will occur, which proves that, when there is long-range interaction, the system will have unconditional flocking behavior, and when there is short-range interaction, the system will have flocking behavior when the initial value satisfies certain conditions. But, inevitably, communication failure slows down the convergence rate of flocking behavior. In addition, it was found in the numerical simulation that, if the (PE) condition given in this paper is not satisfied, the flocking behavior of the system will fail. For the second problem, this paper gives the corresponding delay condition (3.1) to maintain the stability of the system (1.4) flocking behavior, and through numerical simulation analysis, it was found that the delay is conducive to accelerating the convergence rate of the flocking behavior, which improves the slow convergence rate problem caused by communication failure. In addition, it is worth mentioning that the Lyapunov functional constructed in this paper is more refined than that in [20], and that the conclusion obtained includes and extends the conclusion in [20]. The assumption of communication weight selected in this work is a fixed function, but, in reality, the change of communication weight is often random, which cannot be described by a function. At this time, we need to introduce random time variables and establish corresponding random persistent excitation conditions, which is our next focus of study.
The authors would like to thank all of the reviewers who participated in the review and MJEditor (www.mjeditor.com) for its linguistic assistance during the preparation of this manuscript. This research was supported by the National Natural Science Foundation of China (11671011) and Natural Science Foundation for General Program of Hunan Province (2022JJ30655).
The authors have no conflict of interest to declare that is relevant to the content of this paper.
[1] |
Tommasini FJ, Ferreira LdC, Tienne LGP, et al. (2018) Poly(Methyl Methacrylate)-SiC nanocomposites prepared through in situ polymerization. Mat Res 21: e20180086. https://doi.org/10.1590/1980-5373-MR-2018-0086 doi: 10.1590/1980-5373-MR-2018-0086
![]() |
[2] | Habeeb MA (2011) Effect of rate of deposition on the optical parameters of GaAs films. Eur J Sci Res 57: 478–484. |
[3] |
Choi B, Park S, Kim S (2015) Preparation of polyethylene oxide composite electrolytes containing imidazoliumcation salt-attached titanium oxides and their conducing behavior. J Ind Eng Chem 31: 352–359. https://doi.org/10.1016/j.jiec.2015.07.009 doi: 10.1016/j.jiec.2015.07.009
![]() |
[4] |
Kumar K, Ravi M, Pavani Y, et al. (2012) Electrical conduction mechanism in NaCl completed PEO/PVP polymer blend electrolytes. J. Non-Cryst Solids 358: 3205–3211. https://doi.org/10.1016/j.jnoncrysol.2012.08.022 doi: 10.1016/j.jnoncrysol.2012.08.022
![]() |
[5] |
Mohammed AH, Habeeb MA (2022) CO2O3 nanofiller-based polymer blend nanocomposites for enhanced optical properties for optoelectronics devices and as a model of antibacterial. HIV Nurs 22: 1167–1172. https://doi.org/10.31838/hiv22.02.225 doi: 10.31838/hiv22.02.225
![]() |
[6] |
Habeeb MA (2014) Dielectric and optical properties of (PVAc-PEG-Ber) biocomposites. J Eng Appl Sci 9: 102–108. https://doi.org/10.36478/jeasci.2014.102.108 doi: 10.36478/jeasci.2014.102.108
![]() |
[7] |
Dooley KM, Chen SY, Ross JR (1994) Stable nickel-containing catalysts for the oxidative chupling of methane. J Catal 145: 402–408. https://doi.org/10.1006/jcat.1994.1050 doi: 10.1006/jcat.1994.1050
![]() |
[8] |
Hosseini MA, Malekie S, Kazemi F (2022) Experimental evaluation of gamma radiation shielding characteristics of Polyvinyl Alcohol/Tungsten oxide composite: A comparison study of micro and nano sizes of the fillers. Nucl Instrum Methods Phys Res 1026: 166214. https://doi.org/10.1016/j.nima.2021.166214 doi: 10.1016/j.nima.2021.166214
![]() |
[9] |
Hosseini MA, Zareb H, Malekie S (2023) Raman spectroscopy of electron irradiated Multi-Walled Carbon Nanotube for dosimetry purposes. Radiat Phys Chem 202: 110535. https://doi.org/10.1016/j.radphyschem.2022.110535 doi: 10.1016/j.radphyschem.2022.110535
![]() |
[10] |
Safdari MS, Malekie S, Kashian S, et al. (2022) Introducing a novel beta-ray sensor based on polycarbonate/bismuth oxide nanocomposite. Sci Rep 12: 2496. https://doi.org/10.1038/s41598-022-06544-6 doi: 10.1038/s41598-022-06544-6
![]() |
[11] |
Ebrahimi N, Hosseini MA, Malekie S (2020) Preliminary study of linearity response of γ-irradiated graphene oxide as a novel dosimeter using the Raman spectroscopy. Bull Mater Sci 43: 233. https://doi.org/10.1007/s12034-020-02177-5 doi: 10.1007/s12034-020-02177-5
![]() |
[12] |
Zike ZI, Habeeb MA (2022) Role of BaTiO3 /TiO2 nanofillers on the optical characteristics of biopolymer for optics and photonics fields. HIV Nurs 22: 1185–1189. https://doi.org/10.31838/hiv22.02.229 doi: 10.31838/hiv22.02.229
![]() |
[13] |
Shirinov AV, Schomburg WK (2008) Pressure sensor from a PVDF film. Sens Actuators A Phys 142: 48–55. https://doi.org/10.1016/j.sna.2007.04.002 doi: 10.1016/j.sna.2007.04.002
![]() |
[14] |
Janczak D, Słoma M, Wroblewski G, et al. (2014) Screen-printed resistive pressure sensors containing graphene nanoplatelets and carbon nanotubes. Sensors 14: 17304–17312. https://doi.org/10.3390/s140917304 doi: 10.3390/s140917304
![]() |
[15] |
Obaid HN, Habeeb MA, Rashid FL, et al. (2013) Thermal energy storage by nanofluids. J Eng Appl Sci 8: 143–145. https://doi.org/10.36478/jeasci.2013.143.145 doi: 10.36478/jeasci.2013.143.145
![]() |
[16] |
Liao YC, Xu DG, Zhang PC (2018) Preparation and characterization of Bi2O3/XNBR flexible films for attenuating gamma rays. Nucl Sci Tech 29: 99. https://doi.org/10.1007/s41365-018-0436-7 doi: 10.1007/s41365-018-0436-7
![]() |
[17] |
Habeeb MA, Jaber ZS (2022) Enhancement of structural and optical properties of CMC/PAA blend by addition of zirconium carbide nanoparticles for optics and photonics applications. Eur J Phys 4: 176–182. https://doi.org/10.26565/2312-4334-2022-4-18 doi: 10.26565/2312-4334-2022-4-18
![]() |
[18] |
Jebur QM, Hashim A, Habeeb MA (2020) Fabrication, structural and optical properties for (Polyvinyl alcohol-polyethylene oxide iron oxide) nanocomposites. Egypt J Chem 63: 611–623. https://dx.doi.org/10.21608/ejchem.2019.10197.1669 doi: 10.21608/ejchem.2019.10197.1669
![]() |
[19] |
Erdem M, Baykara O, Dogru M, et al. (2010) A novel shielding material prepared from solid waste containing lead for gamma ray. Radiat Phys Chem 79: 917–922. https://doi.org/10.1016/j.radphyschem.2010.04.009 doi: 10.1016/j.radphyschem.2010.04.009
![]() |
[20] |
Mahdi SM (2022) Evaluation of the influence of SrTiO3 and CoO nanofillers on the structural and electrical polymer blend characteristics for electronic devices. Dig J Nanomater Biostruct 17: 941–948. https://doi.org/10.15251/DJNB.2022.173.941 doi: 10.15251/DJNB.2022.173.941
![]() |
[21] |
Habeeb MA, Hashim A, Hayder N (2020) Structural and optical properties of novel (PS-Cr2O3/ZnCoFe2O4) nanocomposites for UV and microwave shielding. Egypt J Chem 63: 697–708. https://dx.doi.org/10.21608/ejchem.2019.12439.1774 doi: 10.21608/ejchem.2019.12439.1774
![]() |
[22] | Atta ER, Zakaria KM, Madbouly AM (2015) Study on polymer clay layered nanocomposites as shielding materials for ionizing radiation. Int J Recent Sci Res 6: 4263–4269. |
[23] |
Abbas NK, Habeeb MA, Algidsawi AJK (2015) Preparation of chloro penta amine cobalit(Ⅲ) chloride and study of its influence on the structural and some optical properties of polyvinyl acetate. Int J of Polym Sci 2015: 926789. https://doi.org/10.1155/2015/926789 doi: 10.1155/2015/926789
![]() |
[24] |
Hayder N, Habeeb MA, Hashim A (2020) Structural, optical and dielectric properties of (PS-In2O3/ZnCoFe2O4) nanocomposites. Egypt J Chem 63: 577–592. https://doi.org/10.21608/ejchem.2019.14646.18874 doi: 10.21608/ejchem.2019.14646.18874
![]() |
[25] |
Mahdi SM, Habeeb MA (2022) Synthesis and augmented optical characteristics of PEO-PVA-SrTiO3-NiO hybrid nanocomposites for optoelectronics and antibacterial applications. Opt Quantum Electron 54: 854. https://doi.org/10.1007/s11082-022-04267-6 doi: 10.1007/s11082-022-04267-6
![]() |
[26] |
Chandra K, Ipsita C, Debdulal S, et al. (2015) Modified clad optical fibre coated with PVA/TiO2 nanocomposite for humidity sensing application. Int J Smart Sens Intell Syst 8: 1424–1442. https://doi.org/10.21307/ijssis-2017-813 doi: 10.21307/ijssis-2017-813
![]() |
[27] |
Jebur QM, Hashim A, Habeeb MA (2020) Structural, AC electrical and optical properties of (polyvinyl alcohol-polyethylene oxide-aluminum oxide) nanocomposites for piezoelectric devices. Egypt J Chem 63: 719–734. https://dx.doi.org/10.21608/ejchem.2019.14847.1900 doi: 10.21608/ejchem.2019.14847.1900
![]() |
[28] |
Habeeb MA, Hashim A, Hayder N (2020) Fabrication of (PS-Cr2O3/ZnCoFe2O4) nanocomposites and studying their dielectric and fluorescence properties for IR sensors. Egypt J Chem 63: 709–717. https://dx.doi.org/10.21608/ejchem.2019.13333 doi: 10.21608/ejchem.2019.13333
![]() |
[29] |
Habeeb MA, Abdul Hamza RS (2018) Novel of (biopolymer blend-MgO) nanocomposites: Fabrication and characterization for humidity sensors. J Bionanosci 12: 328–335. https://doi.org/10.1166/jbns.2018.1535 doi: 10.1166/jbns.2018.1535
![]() |
[30] |
George FF, Leon MC, Ayo A, et al. (2010) Metal oxide semi-conductor gas sensors in environmental monitoring. J Sens 10: 5469–5502. https://doi.org/10.3390/s100605469 doi: 10.3390/s100605469
![]() |
[31] |
Habeeb MA, Kadhim WK (2014) Study the optical properties of (PVA-PVAC-Ti) nanocomposites. J Eng Appl Sci 9: 109–113. https://doi.org/10.36478/jeasci.2014.109.113 doi: 10.36478/jeasci.2014.109.113
![]() |
[32] |
Narsimha P, Shilpa J, Syed K, et al. (2006) Electrical and humidity sensing properties of polyaniline/WO3 composites. Sens Actuators B Chem 114: 599–603. https://doi.org/10.1016/j.snb.2005.06.057 doi: 10.1016/j.snb.2005.06.057
![]() |
[33] |
Geng WC, Li N, Li XT, et al. (2007) Effect of polymerization time on the humidity sensing properties of polypyrrole. Sens Actuators B Chem 125: 114–119. https://doi.org/10.1016/j.snb.2007.01.041 doi: 10.1016/j.snb.2007.01.041
![]() |
[34] | Hadi AH, Habeeb MA (2021) Effect of CdS nanoparticles on the optical properties of (PVA-PVP) blends. J Mech Eng Res Developments 44: 265–274. https//jmerd.net/03-2021-265-274 |
[35] |
Roy MK, Mahloniya RG, Bajpai J, et al. (2012) Spectroscopic and morphological evaluation of gamma radiation irradiated polypyrole based nanocomposites. J Adv Mater Lett 3: 426–432. https://doi.org/10.5185/amlett.2012.6373 doi: 10.5185/amlett.2012.6373
![]() |
[36] |
Akkurt I, Akyıldırım H, Mavi B, et al. (2010) Gamma-ray shielding properties of concrete including barite at different energies. Prog Nucl Energy 52: 620–623. https://doi.org/10.1016/j.pnucene.2010.04.006 doi: 10.1016/j.pnucene.2010.04.006
![]() |
[37] |
Habeeb MA, Mahdi WS (2019) Characterization of (CMC-PVP-Fe2O3) nanocomposites for gamma shielding application. Int J Emerging Trends Eng Res 7: 247–255. https://doi.org/10.30534/ijeter/2019/06792019 doi: 10.30534/ijeter/2019/06792019
![]() |
[38] |
Habeeb MA, Hamza RSA (2018) Synthesis of (Polymer blend-MgO) nanocomposites and studying electrical properties for piezoelectric application. Indones J Electr Eng Inf 6: 428–435. https://doi.org/10.11591/ijeei.v6i1.511 doi: 10.11591/ijeei.v6i1.511
![]() |
[39] | Kaur S, Singh KJ (2013) Comparative study of lead borate and lead silicate glass systems doped with aluminum oxide as gamma-ray shielding materials. Int J Innov Technol Explor Eng 25: 172–175. |
[40] |
Hashim A, Habeeb MA, Jebur QM (2019) Structural, dielectric and optical properties for (Polyvinyl alcohol-polyethylene oxide manganese oxide) nanocomposites. Egypt J Chem 63: 735–749. https://dx.doi.org/10.21608/ejchem.2019.14849.1901 doi: 10.21608/ejchem.2019.14849.1901
![]() |
[41] |
Zhang J, Yu J, Jaroniec M, et al. (2012) Noble metal-free reduced graphene oxide-ZnxCd1-xS nanocomposite with enhanced solar photocatalytic H2-production performance. Nano Lett 12: 4584–4589. https://doi.org/10.1021/nl301831h doi: 10.1021/nl301831h
![]() |
[42] |
Hadi AH, Habeeb MA (2021) The dielectric properties of (PVA-PVP-CdS) nanocomposites for gamma shielding applications. J Phys Conf Ser 1973: 012063. https://doi.org/10.1088/1742-6596/1973/1/012063 doi: 10.1088/1742-6596/1973/1/012063
![]() |
[43] |
Mahdi SM, Habeeb MA (2022) Fabrication and tailored structural and dielectric characteristics of (SrTiO3/NiO) nanostructure doped (PEO/PVA) polymeric blend for electronics fields. Phys Chem Solid State 23: 785–792. https://doi.org/10.15330/pcss.23.4.785-792 doi: 10.15330/pcss.23.4.785-792
![]() |
[44] | Mehrara R, Malekie S, Kotahi SMS, et al. (2021) Introducing a novel low energy gamma ray shield utilizing Polycarbonate Bismuth Oxide composite. Sci Rep 11: 10614. https://doi.org/10.1038/s41598-021-89773-5 |