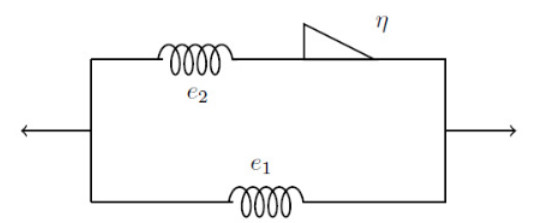
Steel is a versatile metal, got a wide range of applications in all the fields of engineering and technology. Generally, low carbon steels are tough and high alloy carbon steels are hard in nature. Certain applications demand both properties in the same steel. Carburization is one such technique that develops hard and wear resistant surfaces with a soft core. The objective of this work is to study the influence of post carburizing treatment (normalizing) on three grades of steels (EN 3, 20MnCr5, and EN 353). Post carburizing treatments are necessary to overcome the adverse effects of carburization alone. Here carburization was carried out in the propane atmosphere by heating the gas carburizing furnace to 930 ℃ for more than a day. Normalizing was carried out at 870 ℃ for 1 h and cooled in air. Tensile, hardness, Charpy impact tests along with SEM (scanning electron microscopy) and EDAX (energy dispersive X-ray analysis) were conducted to analyze the phase transformation, failure mode analysis in all the samples. Carburized steels displayed the formation of ferrite, pearlite, and sometimes bainite phases in the core and complete coarse pearlite in the case regions, whereas in the post carburized steels, increased amount of ferrite, fine pearlite, and bainite in the core and fine pearlite with traces of bainite in the case region was observed. Normalizing also refines the grain with increased UTS (ultimate tensile strength), hardness, and impact resistance. EN 353 showed higher UTS among the steels with 898 MPa after carburization and 1370 MPa after normalizing treatment. Maximum hardness of 48 HRC was observed in 20MnCr5 and toughness was superior in EN 3 with energy absorbed during test i.e., 8 and 12 J before and after normalizing treatment. Based on the fracture surface analysis, in EN 353 steel, a finer array of dimples with voids and elongated bigger clustered dimples containing ultrafine dimples array are observed in the core and case respectively during carburizing whereas, more density of river pattern and cleavage failure (brittle) are observed in the core and case respectively after post carburizing (normalizing) treatment. There is a reduction in the ductility of the steels after post carburizing treatment. It was observed that normalizing treatment produces superior mechanical properties in the carburized steels by grain refinement and strong microstructures like bainite. Normalizing as post carburizing treatment can be recommended for engineering applications where ductile core and hard surface are of great importance.
Citation: Pavan Hiremath, Gowrishankar M. C., Manjunath Shettar, Sathyashankara Sharma, Jayashree P. K., Suhas Kowshik. Influence of normalizing post carburizing treatment on microstructure, mechanical properties and fracture behavior of low alloy gear steels[J]. AIMS Materials Science, 2021, 8(5): 836-851. doi: 10.3934/matersci.2021051
[1] | Maurizio Verri, Giovanna Guidoboni, Lorena Bociu, Riccardo Sacco . The role of structural viscoelasticity in deformable porous media with incompressible constituents: Applications in biomechanics. Mathematical Biosciences and Engineering, 2018, 15(4): 933-959. doi: 10.3934/mbe.2018042 |
[2] | Fang Wang, Jiaming Wang, Mingxin Li, Jun Hu, Kehua Song, Jianguo Zhang, Yubo Fan . Biomechanical study of the effect of traction on elbow joint capsule contracture. Mathematical Biosciences and Engineering, 2023, 20(12): 21451-21466. doi: 10.3934/mbe.2023949 |
[3] | Oualid Kafi, Nader El Khatib, Jorge Tiago, Adélia Sequeira . Numerical simulations of a 3D fluid-structure interaction model for blood flow in an atherosclerotic artery. Mathematical Biosciences and Engineering, 2017, 14(1): 179-193. doi: 10.3934/mbe.2017012 |
[4] | Christophe Prud'homme, Lorenzo Sala, Marcela Szopos . Uncertainty propagation and sensitivity analysis: results from the Ocular Mathematical Virtual Simulator. Mathematical Biosciences and Engineering, 2021, 18(3): 2010-2032. doi: 10.3934/mbe.2021105 |
[5] | Tran Quang-Huy, Phuc Thinh Doan, Nguyen Thi Hoang Yen, Duc-Tan Tran . Shear wave imaging and classification using extended Kalman filter and decision tree algorithm. Mathematical Biosciences and Engineering, 2021, 18(6): 7631-7647. doi: 10.3934/mbe.2021378 |
[6] | Tuoi Vo, William Lee, Adam Peddle, Martin Meere . Modelling chemistry and biology after implantation of a drug-eluting stent. Part Ⅰ: Drug transport. Mathematical Biosciences and Engineering, 2017, 14(2): 491-509. doi: 10.3934/mbe.2017030 |
[7] | Li Cai, Qian Zhong, Juan Xu, Yuan Huang, Hao Gao . A lumped parameter model for evaluating coronary artery blood supply capacity. Mathematical Biosciences and Engineering, 2024, 21(4): 5838-5862. doi: 10.3934/mbe.2024258 |
[8] | Xu Bie, Yuanyuan Tang, Ming Zhao, Yingxi Liu, Shen Yu, Dong Sun, Jing Liu, Ying Wang, Jianing Zhang, Xiuzhen Sun . Pilot study of pressure-flow properties in a numerical model of the middle ear. Mathematical Biosciences and Engineering, 2020, 17(3): 2418-2431. doi: 10.3934/mbe.2020131 |
[9] | Subhadip Paul, Prasun Kumar Roy . The consequence of day-to-day stochastic dose deviation from the planned dose in fractionated radiation therapy. Mathematical Biosciences and Engineering, 2016, 13(1): 159-170. doi: 10.3934/mbe.2016.13.159 |
[10] | Rebecca Vandiver . Effect of residual stress on peak cap stress in arteries. Mathematical Biosciences and Engineering, 2014, 11(5): 1199-1214. doi: 10.3934/mbe.2014.11.1199 |
Steel is a versatile metal, got a wide range of applications in all the fields of engineering and technology. Generally, low carbon steels are tough and high alloy carbon steels are hard in nature. Certain applications demand both properties in the same steel. Carburization is one such technique that develops hard and wear resistant surfaces with a soft core. The objective of this work is to study the influence of post carburizing treatment (normalizing) on three grades of steels (EN 3, 20MnCr5, and EN 353). Post carburizing treatments are necessary to overcome the adverse effects of carburization alone. Here carburization was carried out in the propane atmosphere by heating the gas carburizing furnace to 930 ℃ for more than a day. Normalizing was carried out at 870 ℃ for 1 h and cooled in air. Tensile, hardness, Charpy impact tests along with SEM (scanning electron microscopy) and EDAX (energy dispersive X-ray analysis) were conducted to analyze the phase transformation, failure mode analysis in all the samples. Carburized steels displayed the formation of ferrite, pearlite, and sometimes bainite phases in the core and complete coarse pearlite in the case regions, whereas in the post carburized steels, increased amount of ferrite, fine pearlite, and bainite in the core and fine pearlite with traces of bainite in the case region was observed. Normalizing also refines the grain with increased UTS (ultimate tensile strength), hardness, and impact resistance. EN 353 showed higher UTS among the steels with 898 MPa after carburization and 1370 MPa after normalizing treatment. Maximum hardness of 48 HRC was observed in 20MnCr5 and toughness was superior in EN 3 with energy absorbed during test i.e., 8 and 12 J before and after normalizing treatment. Based on the fracture surface analysis, in EN 353 steel, a finer array of dimples with voids and elongated bigger clustered dimples containing ultrafine dimples array are observed in the core and case respectively during carburizing whereas, more density of river pattern and cleavage failure (brittle) are observed in the core and case respectively after post carburizing (normalizing) treatment. There is a reduction in the ductility of the steels after post carburizing treatment. It was observed that normalizing treatment produces superior mechanical properties in the carburized steels by grain refinement and strong microstructures like bainite. Normalizing as post carburizing treatment can be recommended for engineering applications where ductile core and hard surface are of great importance.
The biomechanical characterization of biological soft tissues was initially developed by Y.C. Fung on his classical biomechanical treatments [1,2]. He was one of the firsts, together with Fronek, to used a ``new kind'' of elasticity to describe the mechanical behavior of the soft tissues [3], they called this new behavior as pseudo-elasticity. A few years later a new generation of researchers continued on this sense, one of the most know is Holzapfel that together with Gasser and Ogden proposed a new constitutive framework for arterial wall mechanics behavior [4], basically based in a non linear elastic theory introduced by Ogden [5]. In 2004 this group of research realized a comparison of a multi-layer structural model for arterial walls applying a Fung type model, i.e. viscoelasticity. They explored the problematic that emerge on the material stability, in the convergence sense and others problems relatives to the viscoelastic formulation, then in 2010 Holzapfel and Ogden proposed a constitutive modeling of arteries [6] that originated a whole new constitutive frame, named hyperelasticity. Based on strain-energy functions and that represents a huge step on the task of arteries biomechanical characterization, and a great variety of progress was developed and published, like the modeling of biomechanical effects originated by an aneurysm [7,8], the 3D modelling of the human aorta [9,10] or the visco/hyperelastic model that simulate the nonlinear dynamics of atherosclerotic coronary arteries used to predict the initiation of heart attack [11,12].
No often it is not the original Fung's propose for modeling arteries biomechanical behavior, he originally describe the mechanical behavior of the artery as a viscoelastic material. In general, this behavior may be imagined as a spectrum with elastic deformation in one limit case and viscous flow in the other, with varying combinations of the two spread over the range between. Thus, valid constitutive equations for viscoelastic behavior embody elastic deformation and viscous flow as special cases and at the same time provide for response patterns that characterize behavior blends of the two. Intrinsically, such equations will involve not only stress and strain, but time-rates of both stress and strain as well [13]. As mentioned before this kind of materials models, has a great inconvenient related with the convergence on the finite element method software, frequently used to solve this mathematical models [8,6,14,15]. To avoid this situation we can use a prony series and the relaxation function, but again to obtain an accurate solution we need to use a large number of prony series that elevates the computer time on the task of solution finding.
At recent times the fractional calculus theory has been used to modeling viscoelastic materials [16,17,18], consequently some researchers used to model biological soft tissues [19,20], like the use of Kelvin-Voigt fractional viscoelastic model employed to determinate the biomechanical properties of the human liver tissue or the pancreas by Wex [21,22], using stress relaxation test to articular cartilage [23], and even the human calcaneal fat pad [24] using fractional derivatives kernels. Recently this material models are used to estimate the biological changes of the mechanical behavior due to the presence of tumors [25]. Craiem et al [26,27,28] use a fractional viscoelastic constitutive model to describe the arterial biomechanics response, using uniaxial relaxation test.
One of the greatest advantages consist on that many of the basic viscoelastic ideas can be introduce within the context of a one-dimensional state of stress. Once the relaxation modulus, the creep compliance and the complex modulus are obtained, its functions can be included by a subroutine on a FEM software, with the necessary geometry restrictions [29] and the viscoelastic relaxation modifications, or by an finite element model specially develop for fractional differential and integral operators [30].
Viscoelastic fractional models have taken a recent boom in the task of modeling the mechanical behaviour of polymers and soft tissues. Due to the fact that the definition of the fractional derivative provide a new formulation to describe the mechanical behaviour of a material that exhibits a behavior that oscillates between the hooke solids model and the Newtonian fluids [31]. That is one of the principal characteristics of the soft tissues.
The circulatory system is basically composed of the heart and blood vessel system. At the time, the blood vessel system are composing of arteries, arterioles and veins. Arteries are basically conform of three internal layers, known as Tunica Intima, Tunica Media and Tunica Externa or Adventicia, with a semi-cylindrical form and mainly compose of collagen, elastin and muscular fibers [32]. In young humans, the intima is an extremely thin layer (80nm) like a membrane separate to the media for a lay of elastin, the media are form of soft muscular cells merge on a collagen and elastin cellular matrix, finally the externa is the thick layer compose of collagen and fibroblasts [33].
This particular conformation brings the artery a mixed mechanical material behavior know as viscoelasticity [1]. In general, viscoelastic behavior may be imagined as a spectrum with elastic deformation as one limiting case and viscous flow the other extreme case, with varying combinations of the two spread over the range between. Thus, valid constitutive equations for viscoelastic behavior embody elastic deformation and viscous flow as special cases and at the same time provide for response patterns that characterize behavior blends of the two.
Intrinsically, such equations will involve not only stress and strain, but time-rates of both stress and strain as well [13].
We first develop the mathematical and mechanical background that support the present research, with the finality that those readers interested on the topics be familiarized with the basic concepts.
Linear viscoelasticity is a common theory to approximate the time-dependent behaviour of polymers, and materials that exhibit similar characteristics at relatively low temperatures and stress.
The development of the mathematical theory of linear viscoelasticity is based on the principle that the mechanical stress on a certain period of time is directly proportional to the strain rate. In that way, if we have that stress and stress rate are infinitesimal and the stress-strain relation depend on time, that relationship can be expressed by a differential equation with constant coefficients.
The stress-strain relationship can be described, assuming that the Maxwell-Boltzmann principle are satisfied, by the constitutive equation:
σ(t)=t∫−∞G(t−ξ)dε(ξ)dξdξ | (2.1) |
or
ε(t)=t∫−∞J(t−ξ)dσ(ξ)dξdξ | (2.2) |
were G(t) and J(t) are the stress relaxation modulus and the creep compliance respectively. These important functions are commonly employing on material characterization, and are describing above.
The creep test consists of instantaneously subjecting the material to a simple shear stress of magnitude σ0 and maintaining that stress constant thereafter while measuring the shear strain as a function of time. The resulting strain is called the creep. In the stress relaxation test, and instantaneous shear strain of magnitude ε0 is imposed on the material sample and maintained at the value while the resulting stress, is recorded as a function of time. The decrease in the stress values over the duration of the test is referred to as the stress relaxation.
The behavior of viscoelastic materials when are subject to harmonic stress or strain is an important part of the theory of viscoelasticity and sustains a fundamental part of the research. Cyclic experiments are used to identify the mechanical behavior of the material and to determine the values of the elastic and viscous plots of this, maintaining a balance between complexity and simulation capacity of the phenomena. Data processing could be carried out in the same way for a non-cyclic signal, but it would have to be extensive in time to have enough information to fit the model and its processing would be complex.
Consider the response of the material, when is applying a harmonic shear strain of frequency ω as:
ε(t)=ε0sin(ωt) | (2.3) |
At the same time the strain rate changes with the same frequency ω with a translation ϕ with respect to the stress,
σ(t)=σ0sin(ωt+ϕ) | (2.4) |
replacing equation 2.4 on equation 2.1, will be able to obtain the constitutive equation:
σ(t)=ε0(G′sin(ωt)+G′′cos(ωt)) | (2.5) |
with
G′(ω)=ω∫∞0G(t−ξ)sin(ω(t−ξ)) d(t−ξ) | (2.6) |
and
G′′(ω)=ω∫∞0G(t−ξ)cos(ω(t−ξ)) d(t−ξ) | (2.7) |
where G′(ω), G′′(ω) are known as the storage and loss modulus respectively. Expressing the harmonic functions on the complex plane we have
σ∗ε∗=G∗=G′+iG′′ | (2.8) |
where G∗ are define as the complex modulus, and is simply the norm of the loss and storage modulus contributions.
At recent times the fractional calculus theory, has used to formulate a wide range of new models on the biomechanics and mechanobiology field [20], the fractional differential and integral equations have a great development specially in the task of characterize the mechanical behavior of soft tissues [19] like the brain [25], liver [21], arteries [27,28,34] and even the human calcaneal fat pad [24].
We now consider the fractional generalization of the Standard Linear Solid (FSLS), show on Figure 1. For this purpose, is sufficient to replace the first order derivative with the fractional Caputo [35] derivative of order ν∈(0,1) in their constitutive equations. We obtain the following stress-strain relationship and the corresponding material functions are described latter.
The equation 2.9 is basically the same that in integer order, but here we replace the first derivative with the Caputo fractional differential operator
∗0Dνtσ(t)+e2ησ(t)=(e1+e2) ∗0Dνtε(t)+e1e2ηε(t) | (2.9) |
applying the Laplace transform to both sides of the equation 2.9 we obtain,
[sν+e2η]ˉσ(s)=[(e1+e2)sν+(e1e2η)]ˉε(s) | (2.10) |
solving for ˉε(s)
ˉε(s)=sν+α(e1+e2)sν+βˉσ(s) | (2.11) |
where α=e2η and β=e1e2η, applying the Laplace inverse transform and the convolution law, we have the analytical solution for FSLS model,
ε(t)=[δ(t)e1+e2+1(e1+e2)t∞∑n=1(−ζtν)nΓ(nν)+αtν−1e1+e2∞∑n=0(−ζtν)nΓ(ν(n+1))]∗σ(t) | (2.12) |
where δ(t) is the traditional Dirac's delta, ∗ is a convolution and ζ=βe1.
Now we proceed to the implementation of the FSLS to the artery modeling process, first we describe the relaxation modulus, the creep compliance and the complex modulus, all necessary for the mechanical one dimensional characterization on the material [36]. Next we briefly shown the process to the creation of the vectorized image and the exportation to CAD software that aloud to be treating like a solid with the mechanical properties and restrictions.
The values of the constants used on the research are taking for experimental creep relaxation test realized on [27] and are e1=0.68, e2=0.39, η=2.14 and ν=0.23. Now we describe the material model functions like the relaxation modulus, creep compliance and complex modulus. The relaxation modulus for the FSLS has the form,
G(t)=e1+e2⋅Eν[−(e2ηtν)] | (3.1) |
where
Eν,φ[(−e2ηtν)]=∞∑n=0(−e2ηtν)nΓ(νn+φ) | (3.2) |
is the Mittag-Lleffler function [20] with ν,φ∈R+ and e1η∈R. On Figure 2 are plot the relaxation modulus function for four fractional order values, and the constants value mentioned before.
In the same way, we obtain and plot the creep compliance function, for different fractional values ν, the creep compliance function J(t) have the form:
J(t)=μ+(1e1−μ)[1−Eν[−(e1e2μηtν)]] | (3.3) |
where μ=1e1+e2.
The complex modulus, present on Figure 2, complete the set of basic functions required for the mechanical characterization.
The axial dicom images are used to obtain a 2D geometry for every one of the slices, taking care on identify properly witch points generate each one of the segments, to do that its necessary to establish a consistent metric respect the patient's measure and an appropriate Hounsfield scale. The coordinates are localized and saved on a csv file. Now a spline curve can be generated through the geometric pattern, this way the cloud of points on each slice are limit by a close contour. This processes are repeat for the creation of each one of the slices and each one of the respective segments of the artery, the intima, the media and the adventicia. This procedure is illustrated in the left side of Figure 3.
Once the geometric patterns are due in all the set of axial images. Again a spline is applied to the set of slices, now to generate the 3D artery segment representation. Therefore this geometry is save on.iges format and export to CAD software, for the three layer solid representation. Now this is able to function properly in finite element method analysis software as see on the right side of Figure 3.
Like in all the others numerical methods, the precision of the method consist basically on the size of the step. If the element is the sufficient small the method converge to the require solution with minimal error. For that reason we need to do the finest mesh that can be possible in function that the processor is able to work.
The artery have a total volume of 0.2865 cm3 and are meshed with 654,977 eight node brick elements, i.e. the mesh consist on 2,000,000 element for cubic centimeter. This size of the mesh it's necessary to are secure that the finite element method converge to the require solution, because in other way the software can enter on a infinity loop or brings an non sense solution, from this number of elements the convergence is the same. The mechanical properties of the three segments are shown in table 1.
Omega g∗ | Omega g∗ | Omega k∗ | Omega k∗ | Frecuency |
real | imag | real | imag | Hz |
2.02E-11 | 4.32E-06 | 2.18E-11 | 3.94E-06 | 0.001 |
2.02E-09 | 4.32E-05 | 2.18E-09 | 0.000038 | 0.01 |
2.02E-07 | 0.000432 | 2.18E-07 | 0.000389 | 0.1 |
2.01E-05 | 0.004323 | 2.17E-05 | 0.003897 | 1.0 |
-0.056137 | 0.166779 | 0.116504 | -0.018474 | 23.0 |
0.113203 | 0.267329 | 0.313763 | 0.479758 | 100.0 |
0.989947 | 0.032453 | 0.514424 | -0.426401 | 350.0 |
The finite element method software are configured to realize the viscoelastic material routine by the property implementation of the frequency data test. Once the data are introduced on the software, we need to apply a load on the internal intima surface, simulating the pressure caused by a blood flow rate of 120/80 mmHg as shown on Figure 4 [37], and a constant load pressure on the exterior externa surface due to muscular compression originated by the muscles that round the artery, the temperature of the body will be consider constant, the extremes of the aorta are fixed by a constraint option and for last the interaction between the layers is set as a tie restriction.
The result of the solid's deformation is shown on top of Figure 5, where the simulation exhibit a tendency or pattern of the deformation route and not necessarily the real deformation, the behavior showed by the artery concord with those founded and predicted previously by [24,19].
In Figure 5 the efforts of von Mises also known as equivalent efforts are show since these are obtained from a relationship that combines the main efforts in an equivalent effort that can be used to compare with the effort of transfer of the analyzed material. The values of the von Mises stress obtained in this research are consistent with previously developed investigations in the experimental field where values of 0.213 MPa for blood pressure of 120mmHg are reported, which coincides with the results obtained by simulation using the viscoelastic fractional method.
In previous research it is founded that the area where the maximum stress values are presented, is distributed in the intimate layer of the artery and is located in the place where it changes its geometry, that is, where there is a change in artery curvature [40].
Finally, Figure 6 shows the distribution of internal pressure caused by blood flow where 0.03MPa pressure zones are identified in general and some 0.098MP maximum pressure zones, which is consistent with the results previously published by Holzapfel [41]
The results obtained were compared with experiments carried out in 2014, where fractional models have been used to characterize various soft tissues, showing that the parameters determined in the research are within the range of those previously found [24,25,19].
The stress distribution and the maximum values funded in the research concords with those previously reported by Holzapfel [40,41], using an hyperelastic model with prony series.
First we obtained the reconstruction of a segment of the aortic artery based on medical images obtained from a computerized axial tomography scanner, using the Hounsfield scale we could identify each of its three constituent layers (intima, media and adventitia). In addition, the process of exporting the medical image in a vectorized geometry was carried out with which it was possible to export to a solid form, that could be manipulated in a finite element software.
Compared with previous works where simulations of the biomechanical effects of the artery were performed using geometric idealizations, considering the layers of the artery as perfect cylinders, it was observed that when doing this what is had for the state of stress consists of a distribution perfectly symmetrical of the stresses, and in the case of the state of deformations in the same way there is a constant deformation in all directions of the solid. However, the geometry of the artery does not consist of a series of cylinders, so it was found in the development of the investigation that the distribution of stress has its local maximum, speaking of von Mises stress, at the point where the artery presents a change in curvature that generates a great deformation at that point, unlike to a uniform deformation, will end up affecting more to one end of the artery.
In this paper we shown that viscoelastic fractional models represents properly the mechanical behavior of the aortic artery, based on a uniaxial simple model.
In addition, it has been proven that with the viscoelastic fractional model, values similar to those previously provided in the literature are obtained without the use of prony series, which considerably reduces the computation time required.
We want to thankful the institutions that supported the present research project, Tecnológico Nacional de México / Instituto Tecnológico Superior de Cajeme, the Biomechanics Investigation Group from Universidad Tecnológica de la Habana, La Habana, Cuba and the Pontificia Universidad Católica de Valparaíso, Chile.
All authors declare no conflicts of interest in this paper.
[1] |
Kajihara M (2009) Numerical analysis for migration of austenite/ferrite interface during carburization of Fe. J Mater Sci 44: 2109-2118. doi: 10.1007/s10853-009-3299-9
![]() |
[2] |
Christ HJ (1998) Experimental characterization and computerbased description of the carburization behaviour of the austenitic stainless steel AISI 304L. Mater Corros 49: 258-265. doi: 10.1002/(SICI)1521-4176(199804)49:4<258::AID-MACO258>3.0.CO;2-U
![]() |
[3] |
Sudha C, Bharasi NS, Anand R, et al. (2010) Carburization behavior of AISI 316LN austenitic stainless steel—Experimental studies and modeling. J Nucl Mater 402: 186-195. doi: 10.1016/j.jnucmat.2010.05.023
![]() |
[4] |
Akiyama M, Oki Y, Nagai M (2012) Steam reforming of ethanol over carburized alkali-doped nickel on zirconia and various supports for hydrogen production. Catal Today 181:4-13. doi: 10.1016/j.cattod.2011.09.043
![]() |
[5] |
Boniatti R, Bandeira AL, Crespi  E, et al. (2013) The influence of surface microstructure and chemical composition on corrosion behaviour in fuel-grade bio-ethanol of low-alloy steel modified by plasma nitro-carburizing and post-oxidizing. Appl Surf Sci 280: 156-163. doi: 10.1016/j.apsusc.2013.04.116
![]() |
[6] |
Yin L, Ma X, Tang G, et al. (2019) Characterization of carburized 14Cr14Co13Mo4 stainless steel by low pressure carburizing. Surf Coat Technol 358: 654-660. doi: 10.1016/j.surfcoat.2018.11.090
![]() |
[7] |
Roy S, Sundararajan S (2019) Effect of retained austenite on spalling behavior of carburized AISI 8620 steel under boundary lubrication. Int J Fatigue 119: 238-246. doi: 10.1016/j.ijfatigue.2018.10.001
![]() |
[8] |
Lødeng R, Ranga C, Rajkhowa T, et al. (2017) Hydrodeoxygenation of phenolics in liquid phase over supported MoO3 and carburized analogues. Biomass Convers Biorefin 7: 343-359. doi: 10.1007/s13399-017-0252-z
![]() |
[9] |
Kvryan A, Efaw C, Higginbotham K, et al. (2019) Corrosion initiation and propagation on carburized martensitic stainless steel surfaces studied via advanced scanning probe microscopy. Materials 12: 940. doi: 10.3390/ma12060940
![]() |
[10] |
McLeod AC, Bishop CM, Stevens KJ, et al. (2015) Microstructure and carburization detection in HP alloy pyrolysis tubes. Metallogr Microstruct Anal 4: 273-285. doi: 10.1007/s13632-015-0210-8
![]() |
[11] |
Gupta A, Singla G, Pandey OP (2016) Effect of synthesis parameters on structural and thermal properties of NbC/C nano composite synthesized via in-situ carburization reduction route at low temperature. Ceram Int 42: 13024-13034. doi: 10.1016/j.ceramint.2016.05.081
![]() |
[12] |
Niu L, Liu X, Liu X, et al. (2017) In situ XRD study on promotional effect of potassium on carburization of spray-dried precipitated Fe2O3 catalysts. ChemCatChem 9: 1691-1700. doi: 10.1002/cctc.201601665
![]() |
[13] |
Hegde A, Sharma S, Sadanand RV (2019) Mechanical characterization and optimization of heat treatment parameters of manganese alloyed austempered ductile iron. J Mech Eng Sci 13: 4356-4367. doi: 10.15282/jmes.13.1.2019.01.0371
![]() |
[14] |
Ahmed HM, Seetharaman S (2010) Reduction-carburization of NiO-WO3 under isothermal conditions using H2-CH4 gas mixture. Metall Mater Trans B 41: 173-181. doi: 10.1007/s11663-009-9307-2
![]() |
[15] |
Gutierrez-Urrutia I, Zaefferer S, Raabe D (2010) The effect of grain size and grain orientation on deformation twinning in a Fe-22wt.% Mn-0.6wt.% C TWIP steel. Mat Sci Eng A-Struct 527: 3552-3560. doi: 10.1016/j.msea.2010.02.041
![]() |
[16] |
Gramlich A, Schmiedl T, Schönborn S, et al. (2020) Development of air-hardening martensitic forging steels. Mat Sci Eng A-Struct 784: 139321. doi: 10.1016/j.msea.2020.139321
![]() |
[17] |
Faccoli M, Ghidini A, Mazzù A (2019) Changes in the microstructure and mechanical properties of railway wheel steels as a result of the thermal load caused by shoe braking. Metall Mater Trans A 50: 1701-1714. doi: 10.1007/s11661-019-05135-x
![]() |
[18] |
Medyński D, Janus A (2018) Effect of heat treatment parameters on abrasive wear and corrosion resistance of austenitic nodular cast iron Ni-Mn-Cu. Arch Civ Mech Eng 18: 515-521. doi: 10.1016/j.acme.2017.08.001
![]() |
[19] |
Zheng B, Shu G, Jiang Q (2019) Experimental study on residual stresses in cold rolled austenitic stainless steel hollow sections. J Constr Steel Res 152: 94-104. doi: 10.1016/j.jcsr.2018.04.021
![]() |
[20] |
Sajid HU, Kiran R (2018) Influence of stress concentration and cooling methods on post-fire mechanical behavior of ASTM A36 steels. Constr Build Mater 186: 920-945. doi: 10.1016/j.conbuildmat.2018.08.006
![]() |
[21] |
An JH, Lee J, Kim YS, et al. (2019) Effects of post weld heat treatment on mechanical and electrochemical properties of welded carbon steel pipe. Met Mater Int 25: 304-312. doi: 10.1007/s12540-018-0201-9
![]() |
[22] |
Pandey C, Mahapatra MM, Kumar P, et al. (2018) Homogenization of P91 weldments using varying normalizing and tempering treatment. Mat Sci Eng A-Struct 710: 86-101. doi: 10.1016/j.msea.2017.10.086
![]() |
[23] |
Kusakin P, Belyakov A, Haase C, et al. (2014) Microstructure evolution and strengthening mechanisms of Fe-23Mn-0.3C-1.5Al TWIP steel during cold rolling. Mat Sci Eng A-Struct 617: 52-60. doi: 10.1016/j.msea.2014.08.051
![]() |
[24] |
Senopati G, Sutowo C, Kartika I, et al. (2019) The effect of solution treatment on microstructure and mechanical properties of Ti-6Mo-6Nb-8Sn alloy. Mater Today Proc 13: 224-228. doi: 10.1016/j.matpr.2019.03.218
![]() |
[25] |
Bakhtiari R, Ekrami A (2009) The effect of bainite morphology on the mechanical properties of a high bainite dual phase (HBDP) steel. Mat Sci Eng A-Struct 525: 159-165. doi: 10.1016/j.msea.2009.07.042
![]() |
[26] |
van Bohemen SMC (2012) Bainite and martensite start temperature calculated with exponential carbon dependence. Mater Sci Tech-Lond 28: 487-495. doi: 10.1179/1743284711Y.0000000097
![]() |
[27] |
Yang J, Yu H, Yin J, et al. (2016) Formation and control of martensite in Ti-6Al-4V alloy produced by selective laser melting. Mater Design 108: 308-318. doi: 10.1016/j.matdes.2016.06.117
![]() |
[28] |
Adnan F, Sajuri Z, Omar MZ (2019) Effect of uniaxial load on microstructure and mechanical properties of Thixo-joint AISI D2 tool steel. J Mech Eng Sci 13: 5006-5020. doi: 10.15282/jmes.13.2.2019.17.0414
![]() |
[29] |
Dmitrieva O, Ponge D, Inden G, et al. (2011) Chemical gradients across phase boundaries between martensite and austenite in steel studied by atom probe tomography and simulation. Acta Mater 59: 364-374. doi: 10.1016/j.actamat.2010.09.042
![]() |
[30] |
Odusote K, Adekunle AS, Rabiu AB (2015) Effect of vegetable oil quenchants on the properties of aluminum during solution heat treatment. J Mech Eng Sci 8: 1343-1350. doi: 10.15282/jmes.8.2015.9.0131
![]() |
[31] |
Pandey C, Mahapatra MM, Kumar P, et al. (2017) Microstructure characterization and charpy toughness of P91 weldment for as-welded, post-weld heat treatment and normalizing & tempering heat treatment. Met Mater Int 23: 900-914. doi: 10.1007/s12540-017-6850-2
![]() |
[32] |
Majid MSA, Daud R, Afendi M, et al. (2014) Stress-strain response modelling of glass fibre reinforced epoxy composite pipes under multiaxial loadings. J Mech Eng Sci 6: 916-928. doi: 10.15282/jmes.6.2014.18.0088
![]() |
[33] |
Ghadimi H, Nedjhad Sh, Eghbali B (2013) Enhanced grain refinement of cast aluminum alloy by thermal and mechanical treatment of Al-5Ti-B master alloy. Trans Nonferrous Met Soc China 23: 1563-1569. doi: 10.1016/S1003-6326(13)62631-X
![]() |
[34] |
Wang T, Cao F, Chen Z, et al. (2015) Three dimensional microstructures and wear resistance of Al-Bi immiscible alloys with different grain refiners. Sci China Technol Sci 58: 870-875. doi: 10.1007/s11431-015-5816-1
![]() |
[35] |
Rosip NIM, Ahmad S, Jamaludin KR, et al. (2013) Production of 316L stainless steel (SS316L) foam via slurry method. J Mech Eng Sci 5: 707-712. doi: 10.15282/jmes.5.2013.17.0068
![]() |
[36] |
Crupi V, Epasto G, Guglielmino E, et al. (2015) Analysis of temperature and fracture surface of AISI4140 steel in very high cycle fatigue regime. Theor Appl Fract Mec 80: 22-30. doi: 10.1016/j.tafmec.2015.07.007
![]() |
[37] |
Casati R, Lemke J, Vedani M (2016) Microstructure and fracture behavior of 316L austenitic stainless steel produced by selective laser melting. J Mater Sci Technol 32: 738-744. doi: 10.1016/j.jmst.2016.06.016
![]() |
[38] |
Rafi HK, Starr TL, Stucker BE (2013) A comparison of the tensile, fatigue, and fracture behavior of Ti-6Al-4V and 15-5 PH stainless steel parts made by selective laser melting. Int J Adv Manuf Tech 69: 1299-1309. doi: 10.1007/s00170-013-5106-7
![]() |
[39] |
Pandey C, Saini N, Mahapatra MM, et al. (2017) Study of the fracture surface morphology of impact and tensile tested cast and forged (C & F) Grade 91 steel at room temperature for different heat treatment regimes. Eng Fail Anal 71: 131-147. doi: 10.1016/j.engfailanal.2016.06.012
![]() |
1. | Qiyin Lv, Yuan Zhang, Ping He, Yao Chen, Yiwen Wang, Negative Poisson’s ratio artificial blood vessel skeleton multi-objective optimization design and numerical modelling, 2025, 0954-4062, 10.1177/09544062241312888 |
Omega g∗ | Omega g∗ | Omega k∗ | Omega k∗ | Frecuency |
real | imag | real | imag | Hz |
2.02E-11 | 4.32E-06 | 2.18E-11 | 3.94E-06 | 0.001 |
2.02E-09 | 4.32E-05 | 2.18E-09 | 0.000038 | 0.01 |
2.02E-07 | 0.000432 | 2.18E-07 | 0.000389 | 0.1 |
2.01E-05 | 0.004323 | 2.17E-05 | 0.003897 | 1.0 |
-0.056137 | 0.166779 | 0.116504 | -0.018474 | 23.0 |
0.113203 | 0.267329 | 0.313763 | 0.479758 | 100.0 |
0.989947 | 0.032453 | 0.514424 | -0.426401 | 350.0 |
Omega g∗ | Omega g∗ | Omega k∗ | Omega k∗ | Frecuency |
real | imag | real | imag | Hz |
2.02E-11 | 4.32E-06 | 2.18E-11 | 3.94E-06 | 0.001 |
2.02E-09 | 4.32E-05 | 2.18E-09 | 0.000038 | 0.01 |
2.02E-07 | 0.000432 | 2.18E-07 | 0.000389 | 0.1 |
2.01E-05 | 0.004323 | 2.17E-05 | 0.003897 | 1.0 |
-0.056137 | 0.166779 | 0.116504 | -0.018474 | 23.0 |
0.113203 | 0.267329 | 0.313763 | 0.479758 | 100.0 |
0.989947 | 0.032453 | 0.514424 | -0.426401 | 350.0 |