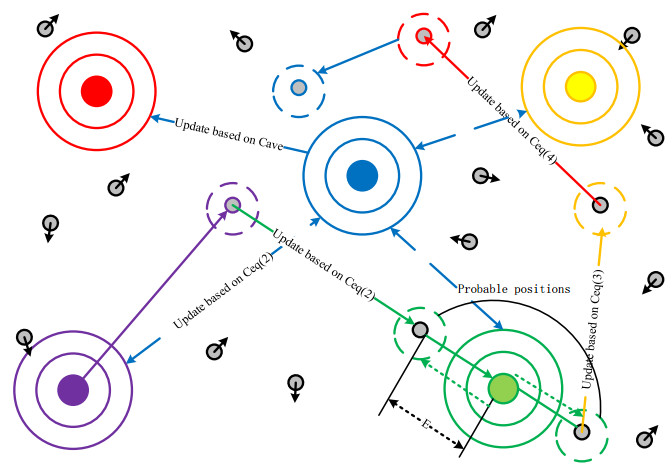
Citation: Chaitra Srikanth, Gattumane Motappa Madhu, Hemanth Bhamidipati, Siddarth Srinivas. The effect of CdO–ZnO nanoparticles addition on structural, electrical and mechanical properties of PVA films[J]. AIMS Materials Science, 2019, 6(6): 1107-1123. doi: 10.3934/matersci.2019.6.1107
[1] | Xiaoxu Peng, Heming Jia, Chunbo Lang . Modified dragonfly algorithm based multilevel thresholding method for color images segmentation. Mathematical Biosciences and Engineering, 2019, 16(6): 6467-6511. doi: 10.3934/mbe.2019324 |
[2] | Shikai Wang, Heming Jia, Xiaoxu Peng . Modified salp swarm algorithm based multilevel thresholding for color image segmentation. Mathematical Biosciences and Engineering, 2020, 17(1): 700-724. doi: 10.3934/mbe.2020036 |
[3] | Jiande Zhang, Chenrong Huang, Ying Huo, Zhan Shi, Tinghuai Ma . Multi-population cooperative evolution-based image segmentation algorithm for complex helical surface image. Mathematical Biosciences and Engineering, 2020, 17(6): 7544-7561. doi: 10.3934/mbe.2020385 |
[4] | Shenghan Li, Linlin Ye . Multi-level thresholding image segmentation for rubber tree secant using improved Otsu's method and snake optimizer. Mathematical Biosciences and Engineering, 2023, 20(6): 9645-9669. doi: 10.3934/mbe.2023423 |
[5] | Wenqi Ji, Xiaoguang He . Kapur's entropy for multilevel thresholding image segmentation based on moth-flame optimization. Mathematical Biosciences and Engineering, 2021, 18(6): 7110-7142. doi: 10.3934/mbe.2021353 |
[6] | Akansha Singh, Krishna Kant Singh, Michal Greguš, Ivan Izonin . CNGOD-An improved convolution neural network with grasshopper optimization for detection of COVID-19. Mathematical Biosciences and Engineering, 2022, 19(12): 12518-12531. doi: 10.3934/mbe.2022584 |
[7] | Mingzhu Li, Ping Li, Yao Liu . IDEFE algorithm: IDE algorithm optimizes the fuzzy entropy for the gland segmentation. Mathematical Biosciences and Engineering, 2023, 20(3): 4896-4911. doi: 10.3934/mbe.2023227 |
[8] | Nilkanth Mukund Deshpande, Shilpa Gite, Biswajeet Pradhan, Ketan Kotecha, Abdullah Alamri . Improved Otsu and Kapur approach for white blood cells segmentation based on LebTLBO optimization for the detection of Leukemia. Mathematical Biosciences and Engineering, 2022, 19(2): 1970-2001. doi: 10.3934/mbe.2022093 |
[9] | Yuting Liu, Hongwei Ding, Zongshan Wang, Gushen Jin, Bo Li, Zhijun Yang, Gaurav Dhiman . A chaos-based adaptive equilibrium optimizer algorithm for solving global optimization problems. Mathematical Biosciences and Engineering, 2023, 20(9): 17242-17271. doi: 10.3934/mbe.2023768 |
[10] | Yuan-Nan Young, Doron Levy . Registration-Based Morphing of Active Contours for Segmentation of CT Scans. Mathematical Biosciences and Engineering, 2005, 2(1): 79-96. doi: 10.3934/mbe.2005.2.79 |
Image segmentation is the basic work of image processing research. There are primarily four types of segmentation methods: thresholding [1,2,3], boundary-based [4,5], region-based [6,7,8], and hybrid techniques [9,10,11,12]. Several algorithmic techniques such as Artificial Neural Network [13], Convolutional neural Network [14], and K-nearest Neighbors [15] can also be applied in image segmentation.
Among all the methods of image segmentation, Otsu [16] method is the most popular one. Computational complexity of Otsu methods increases exponentially with the increasing number of thresholds due to exhaustive search. It is a difficult task to study how to improve the segmentation accuracy of Otsu with multi-thresholds method. The expansion of the actual Otsu's thresholding to multilevel thresholding is known as multi-Otsu thresholding [17]. Nevertheless, these methods are unable to obtain effective results for noisy images. To solve this problem, J. Zhuang [18] proposed a 2-D Otsu method that selects the optimal threshold on a 2-D histogram. Jing [19] proposed the maximum between-cluster variance by using a 3-D histogram approach and was named the 3-D Otsu method. In this method, the 3D histogram takes the median value of neighborhood pixels as the third feature of the existing features of the 2D OTSU method, that is, gray information and neighborhood mean value. L. Wang [20] derived a group of recurrence formula for 3-D Otsu's method and eliminated redundancy in the formula by introducing a look-up table. The method reduced the computation of the 3DOtsu. Therefore, in order to optimize the search process, a faster and automatic optimal threshold selection method is needed.
The traditional exhaustive methods take the large amount of computation. In this case, meta-heuristic methods have attracted much attention in recent years. L. Bian proposed a new multi-threshold MRI image segmentation algorithm based on mixed entropy using Curvelet transformation and Multi-Objective Particle Swarm Optimization [21]. This method could deal with the difficulties caused by noise disturbance, intensity inhomogeneity and edge blurring in Magnetic Resonance Imaging image segmentation. N. Muangkote proposed the nature-inspired meta-heuristic named multilevel thresholding moth-flame optimization algorithm (MTMFO) for multilevel thresholding [22]. This algorithm effectively solved the problem of satellite image segmentation. B. Surina proposed a multi-level thresholding model based on gray-level & local-average histogram (GLLA) and Tsallis–Havrda–Charvát entropy for RGB color image [23]. This method had the effectiveness and reasonability. A. Wunnava proposed an adaptive Harris Hawks optimization (AHHO) technique to solve the multi-level image segmentation [24]. The experimental results were beneficial to the segmentation field of image processing. The optimization algorithm is adopted to solve the threshold selection of the multi-threshold image segmentation method, which effectively improves the segmentation precision of the image segmentation method [25,26].
The optimization algorithm can solve practical engineering problems in recent years [27]. Different optimization algorithms adapt to different engineering problems and have different optimization capabilities [28,29]. These nature-inspired optimization algorithms were mainly classified into two classes recently which are evolutionary algorithm (EA) and biology-inspired or bio-inspired algorithms. EA imitated the Darwinian theory of evolution [30]. There were many good algorithms in this class. In 1975, GA was invented by John Holland [31], it used the binary representation of individuals. In 1997, Differential evolution was proposed Rainer stone, the essence was a multi - objective optimization algorithm [32]. The most popular class was the biology-inspired or bio-inspired algorithms right now. One of the most famous algorithms was the Particle Swarm Optimizer (PSO) which was developed based on the swarming behavior of fish and birds [33]. In 2015, the ant lion optimizer was proposed by Mirjalili [34]. In 2016, Askarzadeh proposed the crow search algorithm [35]. In 2017, the killer whale algorithm was proposed by Biyanto [36]. These algorithms were inspired from the predation behavior animal, so as to obtain better searching ability. There also some algorithms inspired from the physics and chemistry, these algorithms usually had simple mathematical models, but had good optimization effect. In 2001, the harmony search algorithm was proposed by Geem [37]. In 2015, Zheng Yu-Jun proposed water wave optimization algorithm [38]. In 2019, A. Faramarzi proposed a novel optimization algorithm, called Equilibrium optimizer (EO), which controlled volume mass balance models used to estimate both dynamic and equilibrium states [39]. No-Free-Lunch [40] proved that no algorithm can solve all optimization problems.
There is no perfect optimization algorithm and the optimization algorithm should be improved to better solve engineering problems. Many scholars study the hybrid optimization algorithm [41]. Pankaj U. proposed a new multistage hybrid optimization algorithm to optimize multilevel threshold [42]. The method had a good performance in test images. Amandeep proposed a fast SAR image segmentation method based on Particle Swarm Optimization-Gravitational Search Algorithm [43]. The method had good segmentation accuracy. D. Kole proposed a new approach to automatic unsupervised efficient image segmentation algorithm using hybrid technique based on Particle Swarm Optimization and Genetic Algorithm [44]. Gao H. presented a learning strategy-based particle swarm optimization algorithm with an exchange method [45]. The experiment shows that the method had a good result using the Berkeley images. So, the hybrid optimization algorithm could use the advantage of the different algorithms to enhance the search ability of the original algorithm.
This paper focuses on the segmentation of wood fiber images. The wood fiber images have the small target, it takes huge challenge for the segmentation method. We use 3DOtsu as a fitness function to segment wood fiber images. The threshold image method can overcome the difficulty of wood fiber image difference channel. The Equilibrium optimizer algorithm can find the threshold of the wood fiber images, however the segmentation accuracy is low and the CPU time is large. In order to improve the optimal ability of EOA, we use the GOA improve the EOA. The HEOA obtains the strong ability find the optimal threshold from the wood fiber images.
In this paper, the HEOA is proposed. The main contribution of this study is that the GOA improves the original EOA for multilevel threshold. Experiments are performed on CEC2015 data, classic images and wood fiber images. The proposed algorithm is compared with the original EOA and other six algorithms include CSA, FPA, PSO, HSOA and HWOA. The GOA algorithms can balance the exploration and exploitation of the EOA. The results show the superiority of the proposed algorithm in terms of the objective function value, image quality measures on both normal and high-level thresholding.
Otsu algorithm is a classical image segmentation method, and its segmentation results are excellent. This method can be extended to multi-threshold Otsu to obtain the maximum variance between two classes, so as to obtain the optimal threshold of the image [46].
Assuming that there are K thresholds, which divide the image into K+1 classes. The extended between-class variance is calculated by
f(t)=K∑i=0σi | (1) |
The sigma terms are determined by Eq. (6) and the mean levels are calculated by Eq. (7):
σ0=ϖ0(μ0−μT)2,σ1=ϖ1(μ1−μT)2,⋯,σK−1=ϖK−1(μK−1−μT)2 | (2) |
μ0=t∑i=1ipi/ϖ0,μ1=t2∑i=t1+1ipi/ϖ1,⋯,μK−1=L∑i=tM−1+1ipi/ϖK−1 | (3) |
The optimum thresholds are found by maximizing the between-class variance by Eq. (8):
t∗=argmax(K−1∑i=0σi) | (4) |
3D-Otsu, the histogram is constructed by taking gray values of pixels along with their spatial information including the neighborhood mean and median [47]. The Otsu only segment the single channel of the image, however the color images have three channels. There are more information in the three channels, so the 3DOtus can use the information of three channels and get the best threshold form the color images. An image I with K gray levels and N number of pixels is considered, where the intensity value of pixel at the location (x,y) denoted by f(x,y). The mean and median value of l×l neighborhood of pixel is denoted by g(x,y) and h(x,y), which is defined by Eq. (9) and Eq. (10).
g(x,y)=1l2l−12∑i=−l−12l−12∑j=−l−12f(x+i,y+j) | (5) |
h(x,y)=med{f(x+i,y+j);i,j=−l−12,...,l−12} | (6) |
Where, the value of l is taken as 3 in this paper. For every pixel in the image I, mean and median values are calculated in the l×l neighborhood.
Let (tf,tg,th) as the optimal threshold of the three histograms, and use the Eq. (8) to calculate the optimal threshold from the three histograms. The optimal threshold is expressed as:
t∗f=argmax{K−1∑i=0σi(tf)} | (7) |
t∗g=argmax{K−1∑i=0σi(tg)} | (8) |
t∗h=argmax{K−1∑i=0σi(th)} | (9) |
The final optimum thresholds are average of the result of t∗f, t∗g and t∗h, and can be defined by Eq.10:
t∗=(t∗f+t∗g+t∗h)/3 | (10) |
The 3DOtsu image segmentation method uses the information of three channels of color images, the optimal threshold can segment the image exactly.
The EO method is inspired by the simple well-mixed dynamic mass balance on the control body, in which the mass balance equation is used to describe the coordination of non-active components in the control body as a function of its various source and aggregation mechanisms. Similar to most meta-heuristic algorithms, EO uses the initial population to start the optimization process. The equilibrium candidates' collaboration can be seen from Figure1. The initial concentrations are constructed based on the number of particles and dimensions with uniform random initialization in the search space as follows:
Cinitiali=Cmin+rand×(Cmax−Cmin) | (11) |
Where, Cinitiali is the initial concentration vector of the ith particle, Cmax and Cmin denote the minimum and maximum values for the dimensions, rand is a random vector in interval of [0, 1].
The equilibrium state is the final convergence state of the algorithm, which is desired to be the global optimum. At the beginning of the optimization process, there is no knowledge about the equilibrium state and only equilibrium candidates are determined to provide a search pattern for the particles. According to the different experiments under different types of case problems, these candidate particles are the four optimal particles determined in the whole optimization process plus another particle. These particles are nominated as equilibrium candidates and are used to construct a vector called the equilibrium pool:
C→eq,pool={C→eq(1),C→eq(2),C→eq(3),C→eq(4),C→eq(ave)} | (12) |
Where, C→eq(1) is the first particle updates all of its concentrations, C→eq(ave)is the average of the particle.
The main concentration updating rule is the exponential term (F). In order to guarantee convergence by slowing down the search speed along with improving the exploration and exploitation ability of the algorithm, the version can be seen as:
→F=a1×sign(r−0.5)×(e−λ×t−1) | (13) |
Where:
t=(1−IterMax_iter)(a2IterMax_iter) | (14) |
Where, Iter and Max_iter present the current and the maximum number of iterations.
The generation rate is one of the most important terms in the proposed algorithm to provide the exact solution by improving the exploitation phase. The final set of generation rate equation is as follows:
G→=G→0e−λ×(t−t0)=G→0−F→ | (15) |
Where:
→G0=→GCP(→Ceq−λ→C) | (16) |
→GCP={0.5r1r2≥GP0r2<GP | (17) |
Where, r1 and r2 are random numbers in [0, 1] and GCP vector is constructed by the repetition of the same value resulted from Eq. (16).
Finally, the updating rule of EO will be as follows:
C→=C→eq+(C→−C→eq)×F→+G→λV(1−F→) | (18) |
Where, F is defined in Eq. (13), and V is considered as unit.
In 2017, Mirjalili Seyedali proposed the grasshopper optimization algorithm [48]. The grasshoppers are a genus of straight fins of insect, they are seen as pests, because they are in crops for food, to cause damage to agriculture. The growth cycle of grasshoppers is shown in Figure 2. The grasshoppers usually exist alone in nature, but they are one of the biggest swarm of all species. The grasshoppers are unique in that they crowd behavior in adults and larvae of between. Millions of larva foraging on the basis of jumping, they feed on almost all plants, and when they reach adulthood, they form a large group in the air, making long migrations, looking for the next food source.
In larvae stage, the main characteristic of grasshopper is moving slowly, small scale food. When they become adult, collective action has became the main activity characteristics of grasshopper. The natureinspired algorithms logically divide the search process into two tendencies: exploration and exploitation. So mathematical model of the gregarious grasshoppers is represented as follows:
Xi=Si+Gi+Ai | (19) |
where Xi defines the position of the i-th grasshopper, Si is the social interaction, Gi is the gravity force on the i-th grasshopper, and Ai shows the wind advection.
{Si=N∑j=1s(dij)→dijs(r)=fe−rl−e−r | (20) |
where dij is the distance between the i-th and the j-th grasshopper, calculated as dij=|xj−xi|, s is a function to define the strength of social forces, →dij is an unit vector from the i-th grasshopper to the j-th grasshopper.
Gi=−g→eg | (21) |
where g is the gravitational constant and →eg shows an unity vector towards the center of earth.
Ai=u→ew | (22) |
Where u is a constant drift and →ew is a unity vector in the direction of wind.
Substituting S, G, and A into Eq. (19), then this equation can be expanded as follows:
Xi=N∑j=1s(|xj−xi|)xj−xidij−g→eg+u→ew | (23) |
However, this mathematical model cannot be used directly to solve optimization problems, mainly because the grasshoppers quickly reach the comfort zone and the swarm does not converge to a specified point. A modified version of this equation is proposed as follows to solve optimization problems:
Xdi=c1(N∑j=1c2ubd−lbd2s(|xj−xi|)xj−xidij)+→Td | (24) |
Among them, ubd and lbd are a type of upper and lower limitation, →Td is the optimal value after each iteration, c1=c2=cmax−lcmax−cminL, c1 balances the global search and local search for the target area, c2 balances the relationship among the attraction between two grasshopper, cmax and cmin can set the maximum and minimum search ability, l represents the current iteration number, L is the largest number of iterations.
In this subsection, we describe the hybrid equilibrium optimizer algorithm in detail. The EOA fall into the local optimal easily. The algorithm cannot balance the exploitation and exploration. In order to solve this problem, we use the advantage of the GOA to improve the optimization ability of the GOA. We use the Eq. (19) to enhance the individual ability of the EOA. The Xi have the strong ability to avoid the EOA drop into the local optimal. The formula can be seen below:
→C=→Ceq+(→C−→Ceq)×→F+→GλV(1−→F)×Xi | (25) |
A comprehensive algorithm step of HEOA based multilevel color image segmentation is given in Algorithm 1.
Algorithm 1 Pseudo-code of HEOA algorithm |
Input: The color image Output: Segmentation color image Read the input and compute the histogram of three channels of color imageInitialize the parameters r1andr2 Initialize the random population Ci while L < Max_iter do Calculate the fitness values of EOA for (each hawk Ci) do Calculate Ceq, Xi Update the C using Eq. (25) Calculate the fitness function using Eq.(10) end for UpdateCbestif there is a better solution L=L+1 end while Get the best solution as the multilevel threshold K According the K segment the three channels of the images Get the segmentation images |
In order to use the information of the three channels of color images, we use the fitness functions Eq. (10) to calculate the histogram of the three channels. And then, we use the HEOA to optimize the fitness function. Finally, we can get the best threshold and segment the color images. The flowchart of the BMPA can be seen from the Figure 3.
The computational complexity of the proposed method depends on the number of each combination (L), the number of generations (g), the number of population (n) and the parameters dimensions (d). So, computational complexity on L combination isO(L). The computational complexity of population location update is O(n*d). The calculation of fitness function values of all seagull populations isO(n∗L). Therefore, the final computational complexity of the proposed method is:
O(MBE)≈O(g∗(n∗d+n∗L)) | (26) |
To verify the optimization performance of HEOA, its performance is compared with five other optimization methods including CSA, FPA, PSO, BA and basic EOA. The optimization algorithm compared in this section tests CEC2015 benchmark test functions. The detailed description of CEC 2015 benchmark test functions [49] is presented in Table 2. For fair comparison, the other parameters of all algorithms are set according to their original papers. The results obtained by the algorithms on benchmark functions are presented in Table 3. All parameters of the comparison optimization algorithm are shown in table 1. Figure 4 show the result of the CEC2015. The population size is 30 and the number of iterations is 100. The proposed DLNN are testing in Matlab 2018b.
Algorithm | Parameters | Value |
EOA | c1 | 2 |
c2 | 2 | |
CSA [50] | AP | 0.5 |
FPA [51] | P | 0.5 |
PSO [52] | Swam size Cognitive, social acceleration Inertial weight | 2002, 20.95–0.4 |
BA [53] | β | (0, 1) |
HEOA | Levy | 1.5 |
No. | Functions | Related basic functions | Dim | fmin |
CEC-1 | Rotated Bent Cigar Function | Bent Cigar Function | 30 | 100 |
CEC-2 | Rotated Discus Function | Discus Function | 30 | 200 |
CEC-3 | Shifted and Rotated Weierstrass Function | Weierstrass Function | 30 | 300 |
CEC-4 | Shifted and Rotated Schwefel's Function | Schwefel's Function | 30 | 400 |
CEC-5 | Shifted and Rotated Katsuura Function | Katsuura Function | 30 | 500 |
CEC-6 | Shifted and Rotated HappyCat Function | HappyCat Function | 30 | 600 |
CEC-7 | Shifted and Rotated HGBat Function | HGBat Function | 30 | 700 |
CEC-8 | Shifted and Rotated Expanded Griewank's plus Rosenbrock's Function | Griewank's Function Rosenbrock's Function | 30 | 800 |
CEC-9 | Shifted and Rotated Expanded Scaer's F6 Function | Expanded Scaer's F6 Function | 30 | 900 |
CEC-10 | Hybrid Function 1 (N = 3) | Schwefel's FunctionRastrigin's FunctionHigh Conditioned Elliptic Function | 30 | 1000 |
CEC-11 | Hybrid Function 2 (N = 4) | Griewank's Function Weierstrass Function Rosenbrock's Function Scaer's F6 Function | 30 | 1100 |
CEC-12 | Hybrid Function 3 (N = 5) | Katsuura FunctionHappyCat FunctionExpanded Griewank's plus Rosenbrock's Function Schwefel's FunctionAckley's Function | 30 | 1200 |
CEC-13 | Composition Function 1 (N = 5) | Rosenbrock's FunctionHigh Conditioned Elliptic FunctionBent Cigar FunctionDiscus FunctionHigh Conditioned Elliptic Function | 30 | 1300 |
CEC-14 | Composition Function 2 (N = 3) | Schwefel's FunctionRastrigin's FunctionHigh Conditioned Elliptic Function | 30 | 1400 |
CEC-15 | Composition Function 3 (N = 5) | HGBat FunctionRastrigin's FunctionSchwefel's FunctionWeierstrass FunctionHigh Conditioned Elliptic Function | 30 | 1500 |
Func. | HEOA | CSA | PSO | FPA | BA | EOA | ||||||
Mean | Std. | Mean | Std. | Mean | Std. | Mean | Std. | Mean | Std. | Mean | Std. | |
CEC-1 | 1.05E+05 | 1.54E+07 | 4.21E+05 | 1.47E+08 | 2.17E+09 | 3.53E+07 | 1.76E+08 | 7.02E+07 | 4.76E+08 | 2.86E+08 | 1.07E+05 | 3.69E+07 |
CEC-2 | 6.70E+06 | 1.70E+09 | 2.06E+04 | 5.26E+09 | 7.01E+10 | 3.53E+09 | 3.04E+10 | 4.70E+09 | 3.36E+10 | 6.82E+09 | 6.70E+07 | 4.05E+09 |
CEC-3 | 3.20E+02 | 7.12E-02 | 3.20E+02 | 1.51E-01 | 3.22E+02 | 9.57E-02 | 3.21E+02 | 1.43E-01 | 3.21E+02 | 2.10E-01 | 3.55E+02 | 1.47E-01 |
CEC-4 | 4.10E+02 | 1.75E+00 | 4.05E+02 | 9.03E+00 | 5.46E+02 | 1.29E+01 | 5.30E+02 | 1.61E+01 | 5.21E+02 | 9.70E+00 | 4.50E+02 | 2.39E+01 |
CEC-5 | 9.81E+02 | 1.50E+02 | 1.22E+03 | 3.30E+02 | 4.74E+03 | 2.40E+02 | 3.80E+03 | 3.97E+02 | 3.65E+03 | 3.52E+02 | 9.91E+02 | 2.97E+02 |
CEC-6 | 2.05E+03 | 4.75E+06 | 2.14E+03 | 2.33E+07 | 3.79E+09 | 9.03E+06 | 4.52E+09 | 1.14E+07 | 1.28E+08 | 2.71E+07 | 2.10E+03 | 1.37E+07 |
CEC-7 | 7.02E+02 | 1.24E+01 | 7.03E+02 | 4.37E+01 | 1.81E+03 | 1.40E+01 | 1.78E+03 | 2.70E+01 | 1.78E+03 | 4.99E+01 | 8.82E+02 | 2.49E+01 |
CEC-8 | 1.47E+03 | 1.03E+06 | 1.41E+04 | 3.25E+06 | 2.21E+09 | 1.05E+06 | 1.34E+09 | 1.63E+06 | 1.34E+09 | 3.78E+06 | 1.47E+04 | 1.08E+06 |
CEC-9 | 1.00E+03 | 7.30E+00 | 1.00E+03 | 4.77E+01 | 1.33E+03 | 2.45E+01 | 1.33E+03 | 3.46E+01 | 1.62E+03 | 5.15E+01 | 1.00E+04 | 3.38E+01 |
CEC-10 | 1.23E+03 | 4.82E+04 | 2.05E+03 | 3.09E+06 | 2.58E+09 | 4.14E+05 | 2.97E+07 | 8.13E+05 | 4.41E+08 | 4.49E+06 | 1.36E+04 | 7.89E+05 |
CEC-11 | 1.35E+03 | 4.52E+01 | 1.40E+03 | 7.94E+01 | 2.00E+03 | 6.39E+01 | 1.77E+03 | 1.02E+02 | 1.77E+03 | 9.80E+01 | 1.32E+04 | 1.24E+02 |
CEC-12 | 1.30E+03 | 1.03E+01 | 1.30E+03 | 1.75E+01 | 1.76E+03 | 1.22E+01 | 1.49E+03 | 1.28E+01 | 1.51E+03 | 3.25E+01 | 1.34E+05 | 1.58E+01 |
CEC-13 | 1.30E+03 | 8.67E+00 | 1.34E+03 | 1.49E+00 | 5.44E+05 | 1.86E+01 | 1.54E+03 | 3.03E+01 | 1.56E+03 | 2.48E+00 | 1.55E+05 | 3.24E+01 |
CEC-14 | 3.22E+03 | 2.32E+03 | 8.88E+03 | 4.24E+03 | 2.23E+04 | 2.92E+03 | 2.23E+04 | 4.76E+03 | 2.23E+04 | 5.46E+03 | 3.22E+04 | 3.59E+03 |
CEC-15 | 1.60E+03 | 3.36E+02 | 1.60E+03 | 6.49E+02 | 1.28E+04 | 7.10E+02 | 9.01E+03 | 1.34E+03 | 4.09E+03 | 1.05E+03 | 1.80E+03 | 1.27E+03 |
As can be seen from table 3, the results of HEOA algorithm in processing all standard functions are better than those of other comparison algorithms, indicating that the HEOA algorithm can not only solve single-dimensional mathematical functions but also deal with multi-dimensional mathematical functions effectively. It shows that the optimization ability of HEOA algorithm is superior to other comparison algorithms. It can be seen from the Figure 4, the EOA and BA obtains the worst result and the HEOA gets the best result. Above the analysis, HEOA obtains the strong optimal ability, and in the next section we use the HEOA optimize the 3DOtsu.
In this section, HEOA algorithm is applied to optimize the 3DOtsu. In order to better verify the image segmentation ability of proposed algorithm, it is compared with the optimized 3DOtsu algorithm of CSA, PSO, FPA and BA. The color image has three color channels. In this paper, the images of the three channels are segmented, and then the three resulting images are fused to obtain the final segmentation result graph. Firstly, the segmentation effect and precision of HEOA algorithm are analyzed when the threshold value is increased. Then the segmentation ability, statistical analysis and stability analysis of the proposed HEOA algorithm and other optimization algorithms in 3DOtsu image segmentation are analyzed. All parameters of the comparison optimization algorithm are shown in table 3. The test images and the histogram of three channels of color images are as follows Figure 5. The test images included color natural images and satellite images. It can be seen from the histogram, the histogram of three channels has significant different and it take huge challenge for optimization algorithm to find the optimal threshold. Color image segmentation requires a higher threshold level, so it is more complex to use optimization technology to solve the problem. Therefore, the optimization algorithm has the characteristics of randomness.
In order to better observe the performance of the algorithm, we select K = 4, 8, 12 and 15. The evaluation index can better observe the performance of the algorithm. In order to comprehensively analyze the performance of the algorithm, we calculate the CPU time, Uniformity (U), Peak Signal-to-Noise Ratio (PSNR) and Feature Similarity Index (FSIM). The evaluation index of many object optimization methods can be seen table 4.
No. | Measures | Formulation | Reference |
1 | Uniformity measure | U=1−2×(k−1)×∑dj=1∑i∈Rj(fi−μj)2N×(fmax−fmin)2 | [54] |
2 | Peak Signal-to-Noise Ratio (PSNR) | PSNR=10×log10(2552MSE) MSE=1mnm−1∑i=1n−1∑j=1[I(i,j)−K(i,j)]2 |
[55] |
3 | Feature Similarity Index (FSIM) | FSIM=∑x=ηSL(x)⋅PCm(x)∑x=ηPCm(x) | [56] |
In this section, we compare the different segmentation method as fitness function. The EOA and HEOA optimize Otsu and 3DOtsu function. The FSIM of the compared algorithms can be seen from table 5. The Bar chart of the FSIM can be seen in Figure 6.
Image | K | EOA-Otsu | EOA-3DOtsu | HEOA-Otsu | HEOA-3DOtsu |
Test1 | 4 | 0.8628 | 0.8886 | 0.8973 | 0.9106 |
8 | 0.8657 | 0.8864 | 0.8957 | 0.9170 | |
12 | 0.9103 | 0.9332 | 0.9411 | 0.9580 | |
15 | 0.9293 | 0.9479 | 0.9565 | 0.9695 | |
Test2 | 4 | 0.8644 | 0.8799 | 0.8901 | 0.9015 |
8 | 0.8459 | 0.8674 | 0.8736 | 0.9135 | |
12 | 0.9298 | 0.9511 | 0.9569 | 0.9591 | |
15 | 0.9287 | 0.9474 | 0.9562 | 0.9671 | |
Test3 | 4 | 0.8586 | 0.8833 | 0.8919 | 0.9056 |
8 | 0.8599 | 0.8766 | 0.8859 | 0.9246 | |
12 | 0.9207 | 0.9370 | 0.9466 | 0.9661 | |
15 | 0.9049 | 0.9239 | 0.9358 | 0.9688 | |
Test4 | 4 | 0.8518 | 0.8660 | 0.8805 | 0.8963 |
8 | 0.8856 | 0.9060 | 0.9125 | 0.9221 | |
12 | 0.8823 | 0.9034 | 0.9143 | 0.9619 | |
15 | 0.9012 | 0.9260 | 0.9343 | 0.9742 | |
Test5 | 4 | 0.8379 | 0.8545 | 0.8645 | 0.8988 |
8 | 0.8648 | 0.8854 | 0.8992 | 0.9193 | |
12 | 0.9166 | 0.9305 | 0.9446 | 0.9659 | |
15 | 0.9055 | 0.9286 | 0.9345 | 0.9750 | |
Test6 | 4 | 0.8725 | 0.8902 | 0.9025 | 0.9157 |
8 | 0.8854 | 0.9040 | 0.9160 | 0.9189 | |
12 | 0.9267 | 0.9484 | 0.9566 | 0.9585 | |
15 | 0.8904 | 0.9095 | 0.9214 | 0.9663 | |
Test7 | 4 | 0.8708 | 0.8883 | 0.9009 | 0.9147 |
8 | 0.8845 | 0.9037 | 0.9155 | 0.9171 | |
12 | 0.9250 | 0.9478 | 0.9555 | 0.9581 | |
15 | 0.8898 | 0.9087 | 0.9202 | 0.9648 | |
Test8 | 4 | 0.8704 | 0.8871 | 0.9000 | 0.9143 |
8 | 0.8830 | 0.9017 | 0.9142 | 0.9155 | |
12 | 0.9232 | 0.9469 | 0.9535 | 0.9578 | |
15 | 0.8886 | 0.9079 | 0.9201 | 0.9629 | |
Test9 | 4 | 0.8716 | 0.8884 | 0.9011 | 0.9151 |
8 | 0.8844 | 0.9026 | 0.9157 | 0.9163 | |
12 | 0.9237 | 0.9470 | 0.9552 | 0.9596 | |
15 | 0.8897 | 0.9082 | 0.9217 | 0.9633 | |
Test10 | 4 | 0.8720 | 0.8896 | 0.9024 | 0.9171 |
8 | 0.8863 | 0.9027 | 0.9162 | 0.9179 | |
12 | 0.9247 | 0.9482 | 0.9561 | 0.9607 | |
15 | 0.8915 | 0.9089 | 0.9223 | 0.9645 |
It can be seen from table 5, the FSIM value of 3DOtsu is better than Otsu. The 3DOtsu can use the information of color image, and get the best segment result. And the result of HEOA is better than EOA, it means that the Hybrid algorithm improves the optimal ability of EOA and makes the algorithm find the best result. Above analyze, we use the 3DOtsu as the fitness function in the next experiment.
The Figure 6 show the FSIM result of the compared algorithms. The result of 3DOtsu is better than the result of Otsu. The 3DOtsu use the information of three channels and obtains the better threshold than Otsu. At the same time, the result of HEOA is better than EOA, it means that the GOA can improve the optimal ability of the EOA.
In this experiment, to show the merits of proposed technique, the results are compared with CSA, FPA, PSO, BA, HSOA [57] and HWOA [58] using 3DOtsu objective function. The parameters of the compared algorithms are set in the references.
The Figure 7 show the curve of Uniformity. It can be seen from figure, the HEOA obtains the best result in compared algorithms, the HSOA and HWOA gets the better result in compared algorithms, the CSA, FPA, PSO and BA obtains the worst result in all cases. It can be kwon that the hybrid optimization algorithms have the better optimal ability than original optimization algorithm. Most of all, the HEOA has the strongest ability in the compared algorithms.
From Table 6, it can be observed that for all the test images, HEOA is better and more reliable than CSA, FPA, PSO, and BA, because of its precise search capability, at a high threshold level (K). Performance of HSOA and HWOA has closely followed HEOA. The solution update strategy for FPA and PSO may have led to poor results. The comprehensive performance ranking of the comparison algorithm is as follows: HEOA > HSOA > HWOA > PSO > BA > CSA > FPA. So, the HEOA have a better performance than other algorithms.
Image | K | HEOA | HSOA | HWOA | CSA | FPA | PSO | BA |
Test1 | 4 | 0.9571 | 0.9415 | 0.9437 | 0.9245 | 0.9284 | 0.9262 | 0.9243 |
8 | 0.9674 | 0.9525 | 0.9594 | 0.9299 | 0.9309 | 0.9392 | 0.9373 | |
12 | 0.9744 | 0.9641 | 0.9654 | 0.9404 | 0.9456 | 0.9424 | 0.9457 | |
15 | 0.9795 | 0.9684 | 0.9617 | 0.9428 | 0.9441 | 0.9445 | 0.9458 | |
Test2 | 4 | 0.9544 | 0.9460 | 0.9460 | 0.9224 | 0.9167 | 0.9207 | 0.9216 |
8 | 0.9722 | 0.9583 | 0.9624 | 0.9442 | 0.9422 | 0.9425 | 0.9356 | |
12 | 0.9813 | 0.9717 | 0.9639 | 0.9437 | 0.9458 | 0.9526 | 0.9497 | |
15 | 0.9824 | 0.9698 | 0.9697 | 0.9499 | 0.9479 | 0.9468 | 0.9515 | |
Test3 | 4 | 0.9528 | 0.9437 | 0.9427 | 0.9244 | 0.9184 | 0.9184 | 0.9165 |
8 | 0.9626 | 0.9500 | 0.9482 | 0.9255 | 0.9274 | 0.9323 | 0.9290 | |
12 | 0.9782 | 0.9657 | 0.9625 | 0.9464 | 0.9453 | 0.9486 | 0.9406 | |
15 | 0.9812 | 0.9701 | 0.9683 | 0.9467 | 0.9450 | 0.9487 | 0.9513 | |
Test4 | 4 | 0.9518 | 0.9403 | 0.9435 | 0.9201 | 0.9171 | 0.9161 | 0.9187 |
8 | 0.9699 | 0.9589 | 0.9520 | 0.9327 | 0.9350 | 0.9331 | 0.9402 | |
12 | 0.9749 | 0.9623 | 0.9653 | 0.9385 | 0.9398 | 0.9409 | 0.9439 | |
15 | 0.9806 | 0.9717 | 0.9700 | 0.9486 | 0.9490 | 0.9457 | 0.9485 | |
Test5 | 4 | 0.9541 | 0.9410 | 0.9402 | 0.9257 | 0.9167 | 0.9206 | 0.9207 |
8 | 0.9679 | 0.9558 | 0.9560 | 0.9385 | 0.9306 | 0.9399 | 0.9392 | |
12 | 0.9769 | 0.9670 | 0.9618 | 0.9393 | 0.9441 | 0.9404 | 0.9455 | |
15 | 0.9808 | 0.9639 | 0.9678 | 0.9468 | 0.9508 | 0.9519 | 0.9467 | |
Test6 | 4 | 0.9507 | 0.9393 | 0.9373 | 0.9169 | 0.9197 | 0.9138 | 0.9154 |
8 | 0.9666 | 0.9504 | 0.9512 | 0.9383 | 0.9298 | 0.9355 | 0.9352 | |
12 | 0.9787 | 0.9644 | 0.9673 | 0.9492 | 0.9505 | 0.9474 | 0.9431 | |
15 | 0.9824 | 0.9713 | 0.9667 | 0.9470 | 0.9480 | 0.9498 | 0.9472 | |
Test7 | 4 | 0.9514 | 0.9397 | 0.9378 | 0.9183 | 0.9212 | 0.9150 | 0.9167 |
8 | 0.9667 | 0.9524 | 0.9529 | 0.9392 | 0.9300 | 0.9360 | 0.9366 | |
12 | 0.9802 | 0.9648 | 0.9675 | 0.9510 | 0.9521 | 0.9479 | 0.9433 | |
15 | 0.9841 | 0.9725 | 0.9680 | 0.9473 | 0.9483 | 0.9502 | 0.9487 | |
Test8 | 4 | 0.9508 | 0.9377 | 0.9365 | 0.9165 | 0.9196 | 0.9137 | 0.9161 |
8 | 0.9649 | 0.9522 | 0.9520 | 0.9383 | 0.9284 | 0.9349 | 0.9355 | |
12 | 0.9789 | 0.9642 | 0.9663 | 0.9507 | 0.9507 | 0.9479 | 0.9417 | |
15 | 0.9837 | 0.9715 | 0.9670 | 0.9468 | 0.9464 | 0.9497 | 0.9479 | |
Test9 | 4 | 0.9503 | 0.9374 | 0.9355 | 0.9158 | 0.9191 | 0.9118 | 0.9151 |
8 | 0.9633 | 0.9510 | 0.9500 | 0.9363 | 0.9272 | 0.9346 | 0.9351 | |
12 | 0.9772 | 0.9634 | 0.9655 | 0.9497 | 0.9488 | 0.9468 | 0.9402 | |
15 | 0.9820 | 0.9706 | 0.9655 | 0.9462 | 0.9455 | 0.9491 | 0.9460 | |
Test10 | 4 | 0.9505 | 0.9382 | 0.9375 | 0.9171 | 0.9207 | 0.9118 | 0.9167 |
8 | 0.9637 | 0.9519 | 0.9510 | 0.9367 | 0.9277 | 0.9360 | 0.9364 | |
12 | 0.9776 | 0.9653 | 0.9661 | 0.9511 | 0.9493 | 0.9472 | 0.9413 | |
15 | 0.9838 | 0.9710 | 0.9655 | 0.9481 | 0.9464 | 0.9504 | 0.9479 |
Table 7 and table 8 are PSNR and FSIM values of each algorithm respectively. As can be seen from the table, with the increase of the number of threshold values, the PSNR and FSIM values of the image have been significantly improved, indicating that the increase of the number of threshold values significantly improves the segmentation accuracy. The PSNR value of HEOA algorithm is better than other algorithms in all of the 24 groups. So, HEOA have a good competitive than other algorithms. Among all the data of FSIM, HEOA show an improvement of 1.42%, 1.35%, 4.21%, 4.02%, 4.19% and 4.09% over HSOA, HWOA, CSA, FPA, PSO and BA. This means that the segmentation results of HWOA are closer to the original image than other comparison algorithms.
Image | K | HEOA | HSOA | HWOA | CSA | FPA | PSO | BA |
Test1 | 4 | 23.3863 | 23.2573 | 23.2526 | 23.0420 | 23.0405 | 23.0397 | 23.0539 |
8 | 29.2231 | 29.1025 | 29.0968 | 28.9021 | 28.9007 | 28.8989 | 28.9020 | |
12 | 32.5692 | 32.4393 | 32.4442 | 32.2300 | 32.2265 | 32.2282 | 32.2414 | |
15 | 33.3295 | 33.1947 | 33.1953 | 33.0002 | 32.9903 | 32.9903 | 32.9923 | |
Test2 | 4 | 24.4702 | 24.3443 | 24.3386 | 24.1472 | 24.1340 | 24.1342 | 24.1432 |
8 | 29.4260 | 29.3020 | 29.2936 | 29.0852 | 29.0987 | 29.0861 | 29.1020 | |
12 | 32.5786 | 32.4454 | 32.4470 | 32.2471 | 32.2464 | 32.2522 | 32.2424 | |
15 | 34.2153 | 34.0865 | 34.0884 | 33.8828 | 33.8824 | 33.8823 | 33.8887 | |
Test3 | 4 | 24.4769 | 24.3474 | 24.3453 | 24.1433 | 24.1406 | 24.1517 | 24.1495 |
8 | 29.9667 | 29.8287 | 29.8366 | 29.6330 | 29.6383 | 29.6298 | 29.6211 | |
12 | 32.6661 | 32.5412 | 32.5392 | 32.3369 | 32.3265 | 32.3288 | 32.3254 | |
15 | 34.7330 | 34.6031 | 34.6015 | 34.3994 | 34.3925 | 34.4084 | 34.3905 | |
Test4 | 4 | 24.7640 | 24.6386 | 24.6369 | 24.4284 | 24.4400 | 24.4423 | 24.4286 |
8 | 29.5467 | 29.4208 | 29.4154 | 29.2214 | 29.2193 | 29.2207 | 29.2104 | |
12 | 33.5823 | 33.4474 | 33.4548 | 33.2561 | 33.2435 | 33.2461 | 33.2509 | |
15 | 34.2159 | 34.0826 | 34.0820 | 33.8787 | 33.8740 | 33.8707 | 33.8853 | |
Test5 | 4 | 24.5319 | 24.3991 | 24.3994 | 24.2048 | 24.1918 | 24.1918 | 24.1924 |
8 | 29.5550 | 29.4198 | 29.4251 | 29.2137 | 29.2181 | 29.2237 | 29.2154 | |
12 | 34.3322 | 34.1972 | 34.1979 | 34.0010 | 33.9935 | 33.9870 | 33.9959 | |
15 | 35.0669 | 34.9409 | 34.9366 | 34.7387 | 34.7254 | 34.7407 | 34.7279 | |
Test6 | 4 | 25.2119 | 25.0871 | 25.0842 | 24.8695 | 24.8803 | 24.8734 | 24.8829 |
8 | 30.9087 | 30.7880 | 30.7821 | 30.5869 | 30.5821 | 30.5735 | 30.5700 | |
12 | 34.1912 | 34.0606 | 34.0591 | 33.8576 | 33.8505 | 33.8585 | 33.8626 | |
15 | 35.7843 | 35.6567 | 35.6607 | 35.4492 | 35.4505 | 35.4438 | 35.4423 | |
Test7 | 4 | 25.4053 | 25.1458 | 25.1450 | 24.8847 | 25.0748 | 24.9284 | 24.9402 |
8 | 31.0914 | 30.8883 | 30.8601 | 30.7788 | 30.5828 | 30.6162 | 30.6718 | |
12 | 34.3507 | 34.1142 | 34.1742 | 34.0164 | 33.9942 | 33.8766 | 33.8983 | |
15 | 35.9362 | 35.6969 | 35.7545 | 35.6007 | 35.4588 | 35.4937 | 35.6024 | |
Test8 | 4 | 25.5670 | 25.2034 | 25.2799 | 24.9384 | 25.1283 | 24.9787 | 25.0445 |
8 | 31.2698 | 31.0054 | 30.9921 | 30.8672 | 30.7368 | 30.6972 | 30.8372 | |
12 | 34.5283 | 34.2658 | 34.2137 | 34.2017 | 34.1031 | 33.9285 | 34.0632 | |
15 | 36.0846 | 35.7585 | 35.9118 | 35.6393 | 35.6021 | 35.4988 | 35.6352 | |
Test9 | 4 | 25.5622 | 25.0597 | 25.1265 | 24.7749 | 24.9583 | 24.9368 | 24.8720 |
8 | 31.1656 | 30.8841 | 30.8166 | 30.7155 | 30.6732 | 30.6568 | 30.8154 | |
12 | 34.4249 | 34.0884 | 34.1830 | 34.1183 | 33.9539 | 33.7553 | 33.9067 | |
15 | 36.0382 | 35.7553 | 35.7737 | 35.5952 | 35.5146 | 35.3593 | 35.5413 | |
Test10 | 4 | 25.4424 | 24.9891 | 25.1108 | 24.7329 | 24.7752 | 24.9286 | 24.8300 |
8 | 31.1315 | 30.7681 | 30.7427 | 30.5414 | 30.6599 | 30.5129 | 30.6245 | |
12 | 34.3961 | 33.9364 | 34.1416 | 33.9835 | 33.8675 | 33.7435 | 33.8129 | |
15 | 35.8866 | 35.7225 | 35.7457 | 35.4554 | 35.4165 | 35.2905 | 35.3448 |
Image | K | HEOA | HSOA | HWOA | CSA | FPA | PSO | BA |
Test1 | 4 | 0.9367 | 0.9236 | 0.9225 | 0.9094 | 0.9039 | 0.8944 | 0.9087 |
8 | 0.9517 | 0.9414 | 0.9390 | 0.9036 | 0.9077 | 0.9157 | 0.9084 | |
12 | 0.9587 | 0.9433 | 0.9473 | 0.9135 | 0.9149 | 0.9172 | 0.9135 | |
15 | 0.9668 | 0.9511 | 0.9540 | 0.9271 | 0.9331 | 0.9287 | 0.9284 | |
Test2 | 4 | 0.9356 | 0.9227 | 0.9262 | 0.9006 | 0.8943 | 0.8936 | 0.9065 |
8 | 0.9584 | 0.9422 | 0.9438 | 0.9221 | 0.9123 | 0.9122 | 0.9230 | |
12 | 0.9632 | 0.9548 | 0.9563 | 0.9283 | 0.9305 | 0.9331 | 0.9233 | |
15 | 0.9633 | 0.9553 | 0.9506 | 0.9242 | 0.9251 | 0.9255 | 0.9202 | |
Test3 | 4 | 0.9325 | 0.9197 | 0.9181 | 0.9003 | 0.9077 | 0.8898 | 0.9078 |
8 | 0.9477 | 0.9333 | 0.9362 | 0.9060 | 0.9062 | 0.9001 | 0.9048 | |
12 | 0.9599 | 0.9482 | 0.9483 | 0.9289 | 0.9289 | 0.9190 | 0.9162 | |
15 | 0.9607 | 0.9487 | 0.9468 | 0.9309 | 0.9251 | 0.9325 | 0.9358 | |
Test4 | 4 | 0.9385 | 0.9213 | 0.9207 | 0.9065 | 0.9063 | 0.8982 | 0.8954 |
8 | 0.9485 | 0.9362 | 0.9349 | 0.9057 | 0.9058 | 0.9115 | 0.9144 | |
12 | 0.9580 | 0.9487 | 0.9498 | 0.9240 | 0.9298 | 0.9154 | 0.9200 | |
15 | 0.9637 | 0.9457 | 0.9532 | 0.9229 | 0.9227 | 0.9321 | 0.9237 | |
Test5 | 4 | 0.9332 | 0.9213 | 0.9264 | 0.8894 | 0.9071 | 0.9082 | 0.8960 |
8 | 0.9469 | 0.9377 | 0.9361 | 0.9085 | 0.9141 | 0.9083 | 0.9106 | |
12 | 0.9642 | 0.9438 | 0.9503 | 0.9210 | 0.9254 | 0.9187 | 0.9270 | |
15 | 0.9687 | 0.9484 | 0.9489 | 0.9240 | 0.9174 | 0.9240 | 0.9267 | |
Test6 | 4 | 0.9304 | 0.9238 | 0.9186 | 0.8868 | 0.9055 | 0.8972 | 0.9054 |
8 | 0.9518 | 0.9317 | 0.9372 | 0.9027 | 0.9099 | 0.9117 | 0.9085 | |
12 | 0.9579 | 0.9492 | 0.9460 | 0.9280 | 0.9270 | 0.9258 | 0.9158 | |
15 | 0.9682 | 0.9517 | 0.9490 | 0.9289 | 0.9204 | 0.9315 | 0.9250 | |
Test7 | 4 | 0.9313 | 0.9252 | 0.9198 | 0.8882 | 0.9057 | 0.8984 | 0.9060 |
8 | 0.9536 | 0.9322 | 0.9384 | 0.9035 | 0.9109 | 0.9127 | 0.9087 | |
12 | 0.9590 | 0.9498 | 0.9477 | 0.9287 | 0.9280 | 0.9273 | 0.9177 | |
15 | 0.9699 | 0.9524 | 0.9510 | 0.9292 | 0.9222 | 0.9335 | 0.9258 | |
Test8 | 4 | 0.9294 | 0.9239 | 0.9181 | 0.8877 | 0.9044 | 0.8970 | 0.9058 |
8 | 0.9518 | 0.9309 | 0.9381 | 0.9029 | 0.9096 | 0.9116 | 0.9070 | |
12 | 0.9573 | 0.9487 | 0.9470 | 0.9276 | 0.9263 | 0.9263 | 0.9175 | |
15 | 0.9682 | 0.9513 | 0.9494 | 0.9280 | 0.9216 | 0.9324 | 0.9240 | |
Test9 | 4 | 0.9292 | 0.9234 | 0.9161 | 0.8866 | 0.9038 | 0.8956 | 0.9052 |
8 | 0.9506 | 0.9296 | 0.9368 | 0.9010 | 0.9089 | 0.9096 | 0.9064 | |
12 | 0.9569 | 0.9469 | 0.9470 | 0.9273 | 0.9250 | 0.9262 | 0.9160 | |
15 | 0.9676 | 0.9501 | 0.9474 | 0.9275 | 0.9216 | 0.9308 | 0.9236 | |
Test10 | 4 | 0.9296 | 0.9246 | 0.9170 | 0.8874 | 0.9046 | 0.8962 | 0.9057 |
8 | 0.9509 | 0.9306 | 0.9378 | 0.9025 | 0.9093 | 0.9098 | 0.9069 | |
12 | 0.9589 | 0.9483 | 0.9490 | 0.9275 | 0.9261 | 0.9277 | 0.9165 | |
15 | 0.9678 | 0.9501 | 0.9479 | 0.9287 | 0.9232 | 0.9316 | 0.9240 |
Table 9 shows the CPU time of each algorithm under different thresholds. When the threshold value K = 4, the results of each optimization algorithm differ little. At this point, the number of thresholds is small, the search space is small, and the optimization capability of each optimization algorithm is basically the same. When K = 15, the computational complexity of image segmentation increases and the CPU time increases absolutely. The average time of each algorithm in the test image is: HEOA < HSOA < HWOA < FPA < CSA < BA < PSO. So, the HEOA algorithm not only has the good performance in image segmentation, but also has the less CPU time than other compared algorithms.Table 6. The Uniformity measure for the comparison algorithms.
Image | K | HEOA | HSOA | HWOA | CSA | FPA | PSO | BA |
Test1 | 4 | 1.2145 | 1.2251 | 1.2247 | 1.2442 | 1.2405 | 1.2406 | 1.2389 |
8 | 1.3154 | 1.3254 | 1.3260 | 1.3452 | 1.3422 | 1.3386 | 1.3385 | |
12 | 1.5172 | 1.5274 | 1.5279 | 1.5448 | 1.5467 | 1.5451 | 1.5466 | |
15 | 1.8157 | 1.8260 | 1.8258 | 1.8359 | 1.8435 | 1.8376 | 1.8439 | |
Test2 | 4 | 1.2149 | 1.2259 | 1.2255 | 1.2363 | 1.2392 | 1.2436 | 1.2365 |
8 | 1.3154 | 1.3262 | 1.3263 | 1.3388 | 1.3411 | 1.3401 | 1.3371 | |
12 | 1.5181 | 1.5286 | 1.5288 | 1.5423 | 1.5449 | 1.5466 | 1.5389 | |
15 | 1.8158 | 1.8266 | 1.8266 | 1.8419 | 1.8398 | 1.8409 | 1.8424 | |
Test3 | 4 | 1.2151 | 1.2257 | 1.2258 | 1.2354 | 1.2367 | 1.2445 | 1.2367 |
8 | 1.3158 | 1.3268 | 1.3265 | 1.3402 | 1.3411 | 1.3456 | 1.3436 | |
12 | 1.5191 | 1.5292 | 1.5292 | 1.5394 | 1.5406 | 1.5474 | 1.5487 | |
15 | 1.8164 | 1.8270 | 1.8273 | 1.8462 | 1.8369 | 1.8437 | 1.8413 | |
Test4 | 4 | 1.2151 | 1.2254 | 1.2253 | 1.2444 | 1.2358 | 1.2358 | 1.2409 |
8 | 1.3166 | 1.3268 | 1.3271 | 1.3432 | 1.3398 | 1.3458 | 1.3461 | |
12 | 1.5192 | 1.5299 | 1.5301 | 1.5421 | 1.5476 | 1.5483 | 1.5465 | |
15 | 1.8166 | 1.8275 | 1.8269 | 1.8390 | 1.8466 | 1.8422 | 1.8425 | |
Test5 | 4 | 1.2161 | 1.2269 | 1.2269 | 1.2388 | 1.2447 | 1.2368 | 1.2401 |
8 | 1.3167 | 1.3273 | 1.3273 | 1.3456 | 1.3396 | 1.3382 | 1.3431 | |
12 | 1.5201 | 1.5304 | 1.5302 | 1.5471 | 1.5428 | 1.5454 | 1.5401 | |
15 | 1.8169 | 1.8274 | 1.8278 | 1.8431 | 1.8379 | 1.8456 | 1.8405 | |
Test6 | 4 | 1.2168 | 1.2271 | 1.2268 | 1.2372 | 1.2413 | 1.2412 | 1.2383 |
8 | 1.3172 | 1.3281 | 1.3273 | 1.3412 | 1.3407 | 1.3448 | 1.3379 | |
12 | 1.5206 | 1.5307 | 1.5313 | 1.5491 | 1.5408 | 1.5445 | 1.5493 | |
15 | 1.8169 | 1.8278 | 1.8270 | 1.8395 | 1.8463 | 1.8410 | 1.8428 | |
Test7 | 4 | 1.2183 | 1.2275 | 1.2282 | 1.2373 | 1.2421 | 1.2429 | 1.2399 |
8 | 1.3179 | 1.3284 | 1.3291 | 1.3429 | 1.3420 | 1.3463 | 1.3391 | |
12 | 1.5219 | 1.5326 | 1.5316 | 1.5510 | 1.5410 | 1.5462 | 1.5506 | |
15 | 1.8179 | 1.8293 | 1.8289 | 1.8408 | 1.8466 | 1.8410 | 1.8440 | |
Test8 | 4 | 1.2173 | 1.2270 | 1.2272 | 1.2371 | 1.2403 | 1.2424 | 1.2393 |
8 | 1.3167 | 1.3278 | 1.3282 | 1.3409 | 1.3401 | 1.3447 | 1.3386 | |
12 | 1.5217 | 1.5308 | 1.5303 | 1.5497 | 1.5398 | 1.5460 | 1.5498 | |
15 | 1.8172 | 1.8273 | 1.8284 | 1.8396 | 1.8446 | 1.8397 | 1.8424 | |
Test9 | 4 | 1.2154 | 1.2265 | 1.2258 | 1.2357 | 1.2391 | 1.2409 | 1.2376 |
8 | 1.3166 | 1.3268 | 1.3277 | 1.3398 | 1.3389 | 1.3428 | 1.3375 | |
12 | 1.5204 | 1.5304 | 1.5291 | 1.5482 | 1.5397 | 1.5453 | 1.5485 | |
15 | 1.8154 | 1.8272 | 1.8281 | 1.8395 | 1.8431 | 1.8385 | 1.8422 | |
Test10 | 4 | 1.2155 | 1.2282 | 1.2270 | 1.2377 | 1.2404 | 1.2419 | 1.2380 |
8 | 1.3172 | 1.3283 | 1.3279 | 1.3401 | 1.3399 | 1.3444 | 1.3391 | |
12 | 1.5207 | 1.5319 | 1.5305 | 1.5494 | 1.5397 | 1.5460 | 1.5495 | |
15 | 1.8160 | 1.8272 | 1.8297 | 1.8400 | 1.8440 | 1.8393 | 1.8442 |
The experimental results of each algorithm are the same, so statistical tests are needed. Parametric statistical tests are based on various assumptions [59]. The well-known non-parametric statistical tests, namely Friedman test [60] and Wilcoxon rank-sum test [61] are used in this section. For the average rank of Friedman's test given in table 10, the method presented in this paper takes the first place in all cases, and as the number of threshold levels increases, the rank value becomes smaller and smaller, which has greater advantages compared with other comparison methods. Through the above analysis, as the dimension of optimization problem increases, the optimization ability of the proposed algorithm HEOA becomes more and more obvious.
K | HEOA | HSOA | HWOA | CSA | FPA | PSO | BA |
4 | 3.1581 | 3.4458 | 3.5147 | 3.4581 | 3.6152 | 3.9951 | 3.8547 |
8 | 2.9315 | 3.5514 | 3.6891 | 3.5125 | 3.9156 | 3.9514 | 3.6984 |
12 | 1.9984 | 2.5589 | 3.6661 | 3.1285 | 3.9991 | 3.9541 | 3.8854 |
15 | 1.7415 | 2.6518 | 3.6581 | 3.1814 | 3.8147 | 3.8574 | 3.7781 |
Overall | 2.4574 | 3.0520 | 3.6320 | 3.3201 | 3.8362 | 3.9395 | 3.8042 |
The experimental statistical results are shown in Table 11 below. The null hypothesis is constructed as: there is no significant difference between the two algorithms. The alternative hypothesis states that there is a significant difference between the two algorithms. In the experiment, HEOA based method produces better result in 36 out of 40 cases when compared with HSOA based method and produces better result in 35 out of 40 cases when compared with HWOA based method and produces better result in 38 out of 40 cases when compared with CSA based method and produces better result in 38 out of 40 cases when compared with FPA based method and produces better result in 40 out of 40 cases when compared with the PSO based method. As can be seen from the results, there are significant differences among the six algorithms. In most cases, HEOA performs better than other algorithms.
Images | K | HSOA | HWOA | CSA | FPA | PSO |
Test1 | 4 | P < 0.05 | P < 0.05 | P < 0.05 | P < 0.05 | P < 0.05 |
8 | P > 0.05 | P < 0.05 | P < 0.05 | P < 0.05 | P < 0.05 | |
12 | P < 0.05 | P < 0.05 | P < 0.05 | P < 0.05 | P < 0.05 | |
15 | P < 0.05 | P > 0.05 | P < 0.05 | P < 0.05 | P < 0.05 | |
Test2 | 4 | P < 0.05 | P < 0.05 | P < 0.05 | P > 0.05 | P < 0.05 |
8 | P < 0.05 | P < 0.05 | P < 0.05 | P < 0.05 | P < 0.05 | |
12 | P < 0.05 | P < 0.05 | P < 0.05 | P < 0.05 | P < 0.05 | |
15 | P < 0.05 | P < 0.05 | P < 0.05 | P < 0.05 | P < 0.05 | |
Test3 | 4 | P < 0.05 | P < 0.05 | P < 0.05 | P < 0.05 | P < 0.05 |
8 | P < 0.05 | P < 0.05 | P < 0.05 | P < 0.05 | P < 0.05 | |
12 | P < 0.05 | P < 0.05 | P < 0.05 | P < 0.05 | P < 0.05 | |
15 | P < 0.05 | P < 0.05 | P < 0.05 | P < 0.05 | P < 0.05 | |
Test4 | 4 | P < 0.05 | P < 0.05 | P < 0.05 | P < 0.05 | P < 0.05 |
8 | P > 0.05 | P < 0.05 | P < 0.05 | P < 0.05 | P < 0.05 | |
12 | P < 0.05 | P > 0.05 | P < 0.05 | P < 0.05 | P < 0.05 | |
15 | P < 0.05 | P < 0.05 | P < 0.05 | P < 0.05 | P < 0.05 | |
Test5 | 4 | P < 0.05 | P < 0.05 | P < 0.05 | P < 0.05 | P < 0.05 |
8 | P < 0.05 | P < 0.05 | P < 0.05 | P < 0.05 | P < 0.05 | |
12 | P < 0.05 | P < 0.05 | P < 0.05 | P < 0.05 | P < 0.05 | |
15 | P < 0.05 | P < 0.05 | P < 0.05 | P < 0.05 | P < 0.05 | |
Test6 | 4 | P > 0.05 | P < 0.05 | P < 0.05 | P < 0.05 | P < 0.05 |
8 | P < 0.05 | P < 0.05 | P < 0.05 | P < 0.05 | P < 0.05 | |
12 | P < 0.05 | P < 0.05 | P < 0.05 | P < 0.05 | P < 0.05 | |
15 | P < 0.05 | P < 0.05 | P < 0.05 | P < 0.05 | P < 0.05 | |
Test7 | 4 | P < 0.05 | P < 0.05 | P > 0.05 | P < 0.05 | P < 0.05 |
8 | P < 0.05 | P < 0.05 | P < 0.05 | P < 0.05 | P < 0.05 | |
12 | P < 0.05 | P < 0.05 | P < 0.05 | P < 0.05 | P < 0.05 | |
15 | P < 0.05 | P < 0.05 | P < 0.05 | P < 0.05 | P < 0.05 | |
Test8 | 4 | P > 0.05 | P < 0.05 | P < 0.05 | P > 0.05 | P < 0.05 |
8 | P < 0.05 | P > 0.05 | P < 0.05 | P < 0.05 | P < 0.05 | |
12 | P < 0.05 | P < 0.05 | P < 0.05 | P < 0.05 | P < 0.05 | |
15 | P < 0.05 | P < 0.05 | P < 0.05 | P < 0.05 | P < 0.05 | |
Test9 | 4 | P < 0.05 | P < 0.05 | P < 0.05 | P < 0.05 | P < 0.05 |
8 | P < 0.05 | P > 0.05 | P < 0.05 | P < 0.05 | P < 0.05 | |
12 | P < 0.05 | P < 0.05 | P < 0.05 | P < 0.05 | P < 0.05 | |
15 | P < 0.05 | P < 0.05 | P < 0.05 | P < 0.05 | P < 0.05 | |
Test10 | 4 | P < 0.05 | P > 0.05 | P > 0.05 | P < 0.05 | P < 0.05 |
8 | P < 0.05 | P < 0.05 | P < 0.05 | P < 0.05 | P < 0.05 | |
12 | P < 0.05 | P < 0.05 | P < 0.05 | P < 0.05 | P < 0.05 | |
15 | P < 0.05 | P < 0.05 | P < 0.05 | P < 0.05 | P < 0.05 |
In this section, we compare with the novel image segmentation methods. The compared algorithms are PCNN [62], fuzzy c-means (FCM) [63], grayscale co-occurrence matrix (GLCM) [64]. In order to test the performance of the color image segmentation algorithms, we select the wood fibers which are taken under a high-powered microscope as the test images. The figures 8–11 show the wood fiber images and the segmentation result.
It can be seen from the figures 4–7, the result of PCNN has the over-segmentation phenomenon and the segmented images are the worst of the compared algorithms. The FCM and GLCM have the under-segmentation phenomenon and the results of the fiber images are not clear. Among the result of the fiber images, the HEOA-3DOtsu get the best result. The table 12 shows the HEOS obtains a large improvement than the others compared image segmentation methods. The recall and precision are 93.19% and 91.28% respectively. The results show that the performance of this method can provide the segmentation result of the wood fiber image. The Figure12 show the segmentation result of the proposed method. It can be seen from figure, the number of wood fiber can be seen clearly. This method obtains independent wood fiber area. So, the proposed method can segment the wood fiber images successfully and have strong robustness.
Method | R (%) | P (%) | AP (%) | VAL (%) |
FCM | 84.94 | 90.88 | 91.23 | 88.47 |
PCNN | 80.46 | 90.69 | 81.46 | 79.46 |
DLA | 70.12 | 92.95 | 78.32 | 77.57 |
BMPA | 93.19 | 91.28 | 91.45 | 90.38 |
The analysis of wood fiber graphics can understand the state of the fiber, so as to ensure the production of wood or paper that meets industrial requirements. The scholar Mainly analyze the shape and thickness of individual wood fibers. However, the wood fibers in the collected graphics are more responsible and similar to the background. Traditional segmentation methods cannot solve this problem. So, study the novel image segmentation method is necessary.
In the field of optimization algorithm, the proposed method has a good optimal ability solve the CEC2015. And the proposed method has a good competitiveness with the CSA, FPA, PSO, BA and basic EOA. Because of the hybrid optimization algorithm use the advantage of the two optimization algorithms to optimize the complex benchmark function. At the same time, the proposed method solves the threshold image segmentation and find the best threshold from the 3DOtsu. The algorithm gets the best result among CSA, FPA, PSO, BA, HSOA and HWOA. The hybrid optimization algorithms enhance the optimal ability of the original optimization algorithm.
In the field of the fiber wood image segmentation, the proposed method obtains the highest segmentation accuracy with PCNN, FCN and GLCM. The PCNN obtains the worst result in compared algorithms, the performance of PCNN rely on the set of parameters. The FCN and GLCM has strong ability to solve the grey image, however, these two methods take huge challenge to segment color image. The proposed method can overcome the difficult of color image. Finally, the proposed method obtains the wood fiber area exactly.
The limitations of the proposed method can be divided in two contents. First, similar to other optimization algorithms, this algorithm takes time to iterate to find the best solution, which is time-consuming. Second, this method has strong performance in the field of wood fiber image segmentation. In order to make the proposed method can adopt more real area, we will study the novel optimization algorithm.
The proposed method can solve the other problem of optimization. At the same time, it can be used in the field of medical image segmentation, forest fire image segmentation and so on. In the future, we will continue to study many object multi-level threshold methods and different optimization algorithms, so as to improve the image segmentation accuracy.
In this paper, the HEOA algorithm is used to optimize the 3DOtsu algorithm to obtain the optimal multi-threshold image. We use GOA to improve EOA algorithm and enhance the optimization ability of the algorithm. The CEC2015 is selected as benchmark function to test the performance of the hybrid optimization algorithms. The result shows that the hybrid algorithm can enhance the optimal ability of the EOA. And then, the HEOA is compared with other optimization algorithms to jointly optimize 3DOtsu algorithm for classic images and wood fiber images segmentation experiments. From U measure, PSNR and FSIM values, it can be seen that 3DOtsu-HEOA algorithm has the best segmentation accuracy. Finally, we compare the 3DOtsu-HEOA algorithm with the novel image segmentation method. It can be seen from the segmented result that HEOA can get the best segmented result. So, the 3DOtsu-HEOA algorithm proposed in this paper has better image segmentation accuracy and better stability.
This work was supported by the Fundamental Research Funds for the Central Universities under Grant No. 2572018BH08.
The authors have no conflict of interest.
[1] | Ching YC, Iskandar I (2011) Effect of polyurethane/nano-SiO2 composites coating on thermo-mechanical properties of polyethylene film. Adv Mater Proc 2010 161-164. |
[2] |
Ching YC, Yun CC, Iskandar Y (2012) Weathering resistance of solvent borne polyurethane/nanosilica composite. Adv Sci Lett 12: 165-169. doi: 10.1166/asl.2012.2739
![]() |
[3] | Arunkumar L, Venkataraman A (2005) Polymer nanocomposites. Resonance 10: 49-57. |
[4] |
Ching YC, Iskandar IY (2010) Influence of nanosilica/polyurethane composite coating on IR effectiveness and visible light transmission properties of polyethylene. Adv Mater Res 97-101: 1669-1672. doi: 10.4028/www.scientific.net/AMR.97-101.1669
![]() |
[5] | Li S, Shiling Z, Shuxue Z, et al. (2011) Physical and optical properties of silica/polymer nanocomposite inverse opal. Adv Sci Lett 11-12: 3445-3450. |
[6] | Ching YC, Iskandar IY (2009) Influence of nano-SiO2/polyamide composites coating on thermic effect and optical properties of polyethylene film. Int J Mod Phys B 23: 06n07. |
[7] |
Ranjith J, Harding I, Bowater I, et al. (2004) Preparation, surface modification and characterisation of solution cast starch PVA blended films. Polym test 23: 17-27. doi: 10.1016/S0142-9418(03)00049-7
![]() |
[8] |
Usharani K, Balu AR (2015) Structural, optical, and electrical properties of Zn-doped CdO polymer composite films fabricated by a simplified spray pyrolysis technique. Acta Metall Sin-Eng 28: 64-71. doi: 10.1007/s40195-014-0168-6
![]() |
[9] |
Pathak TK, Rajput JK, Kumar V, et al. (2017) Transparent conducting ZnO-CdO mixed oxide thin films grown by the sol-gel method. J colloid Interf Sci 487: 378-387. doi: 10.1016/j.jcis.2016.10.062
![]() |
[10] |
Trilok S, Pandya DK, Singh R, et al. (2011) Synthesis of cadmium oxide doped ZnO nanostructures using electrochemical deposition. J Alloy Comp 509: 5095-5098. doi: 10.1016/j.jallcom.2011.01.168
![]() |
[11] |
Fazhan W, Liu B, Zhijun Z, et al. (2009) Synthesis and properties of Cd-doped ZnO nanotubes. Physica E 41: 879-882. doi: 10.1016/j.physe.2008.12.026
![]() |
[12] |
Umar A, Akhtar MS, Al-Assiri MS, et al. (2018) Composite CdO-ZnO hexagonal nanocones: efficient materials for photovoltaic and sensing applications. Ceram Int 44: 5017-5024. doi: 10.1016/j.ceramint.2017.12.098
![]() |
[13] |
Jeevitesh KR, Trilok KP, Vinod K (2018) CdO:ZnO nanocomposite thin films for oxygen gas sensing at low temperature. Mater Sci Eng B-Adv 228: 241-248. doi: 10.1016/j.mseb.2017.12.002
![]() |
[14] |
Sharma AK, Potdar SS, Pakhare KS, et al. (2017) The selective ethanol gas sensing performance of CdO1-XZnOX nanocomposite. J Mater Sci-Mater E 28: 3752-3761. doi: 10.1007/s10854-016-5984-1
![]() |
[15] | Aruna ST, Alexander SM (2008) Combustion synthesis and nanomaterials. Curr Opin Solid St M 12: 44-50. |
[16] | Rashmi SH, Soumyashree S, Shrusti S, et al. (2018) Structural mechanical and electrical property evaluation of nano cadmium oxide polyvinyl alcohol composites. Int J Plast Technol 22: 41-55. |
[17] |
Rashmi SH, Raizada A, Madhu GM, et al. (2015) Influence of zinc oxide nanoparticles on structural and electrical properties of polyvinyl alcohol films. Plast Rubber Compos 44: 33-39. doi: 10.1179/1743289814Y.0000000115
![]() |
[18] |
Rao JK, Abhishek R, Debargha G, et al. (2015) Investigation of structural and electrical properties of novel CuO-PVA nanocomposite films. J Mater Sci 50: 7064-7074. doi: 10.1007/s10853-015-9261-0
![]() |
[19] | Cai X, Dan H, Shaojuan D, et al. (2014) Isopropanol sensing properties of coral-like ZnO-CdO composites by flash preparation via self-sustained decomposition of metal-organic complexes. Sensor Actuat B-Chem 198: 402-410. |
[20] |
Sathish DV, Ch RK, Ch VR, et al. (2012) Structural and optical investigations on ZnCdO nanopowder. Phys Scripta 86: 035708. doi: 10.1088/0031-8949/86/03/035708
![]() |
[21] | Bhadra J, Sarkar D (2010) Electrical and optical properties of polyaniline polyvinyl alcohol composite films. Indian J Pure Ap Phys 48: 425-428. |
[22] |
Suzan AK, Abaker M, Ahmad U, et al. (2012) Synthesis and characterizations of Cd-doped ZnO multipods for environmental remediation application. J Nanosci Nanotechno 12: 8453-8458. doi: 10.1166/jnn.2012.6801
![]() |
[23] |
Karthik K, Dhanuskodi S, Gobinath C, et al. (2015) Microwave-assisted synthesis of CdO-ZnO nanocomposite and its antibacterial activity against human pathogens. Spectrochim Acta A 139: 7-12. doi: 10.1016/j.saa.2014.11.079
![]() |
[24] | Rahman MM, Sher BK, Hadi MM, et al. (2014) Facile synthesis of doped ZnO-CdO nanoblocks as solid-phase adsorbent and efficient solar photo-catalyst applications. J Ind Eng Chem 20: 2278-2286. |
[25] |
Rao JK, Abhishek R, Satyanarayana SV (2016) Influence of cadmium sulfide nanoparticles on structural and electrical properties of polyvinyl alcohol films. Express Polym Lett 10: 883-894 doi: 10.3144/expresspolymlett.2016.83
![]() |
[26] | Tanaka T (2005) Dielectric nanocomposites with insulating properties. IEEE T Dielect El In 12: 914-928. |
[27] | Zhang L, Wu P, Li Y, et al. (2014) Preparation process and dielectric properties of Ba0.5Sr0.5TiO3-P(VDF-CTFE) nanocomposites. Compos Part B-Eng 56: 284-289. |
[28] |
Dang ZM, Yuan JK, Zha JW, et al. (2012) Fundamentals, processes and applications of high-permittivity polymer-matrix composites. Prog Mater Sci 57: 660-723. doi: 10.1016/j.pmatsci.2011.08.001
![]() |
[29] |
Panda M, Srinivas V, Thakur AK (2008) On the question of percolation threshold in polyvinylidene fluoride/nanocrystalline nickel composites. Appl Phys Lett 92: 132905. doi: 10.1063/1.2900710
![]() |
[30] |
Yang C, Patricia CI, Karim Y (2004) The future of nanodielectrics in the electrical power industry. IEEE T Dielet El In 11: 797-807. doi: 10.1109/TDEI.2004.1349785
![]() |
[31] |
Dutta P, Biswas S, Subodh KD (2002) Dielectric relaxation in polyaniline-polyvinyl alcohol composites. Mater Res Bull 37: 193-200. doi: 10.1016/S0025-5408(01)00813-3
![]() |
[32] |
Ramadhar S, Tandon RP, Panwar VS, et al. (1991) Low-frequency ac conduction in lightly doped polypyrrole films. J Appl Phys 69: 2504-2511. doi: 10.1063/1.348688
![]() |
[33] |
Livi A, Levita G, Rolla PA (1993) Dielectric behavior at microwave frequencies of an epoxy resin during crosslinking. J Appl Polym Sci 50: 1583-1590. doi: 10.1002/app.1993.070500912
![]() |
[34] |
Eloundou JP (2002) Dipolar relaxations in an epoxy-amine system. Eur Polym J 38: 431-438. doi: 10.1016/S0014-3057(01)00200-2
![]() |
[35] | Vijaya BM, Padmasuvarna R (2016) Dielectric properties of the conducting polymers based on nr, nbr using two-point method at microwave frequencies. IJASTEMS 2: 106-112. |
[36] |
Tripathi R, Kumar A, Sinha TP (2009) Dielectric properties of CdS nanoparticles synthesized by soft chemical route. Pramana 72: 969-978. doi: 10.1007/s12043-009-0089-5
![]() |
[37] |
Bhajantri RF, Ravindrachary V, Harisha A, et al. (2007) Effect of barium chloride doping on PVA microstructure: positron annihilation study. Appl Phys A-Mater 87: 797-805. doi: 10.1007/s00339-007-3923-y
![]() |
[38] | Ho CH, Liu CD, Hsieh CH, et al. (2008) High dielectric constant polyaniline/poly (acrylic acid) composites prepared by in situ polymerization. Synthetic Met 158: 630-637. |
1. | K. Balakrishnan, R. Dhanalakshmi, M. Akila, Bam Bahadur Sinha, Improved equilibrium optimization based on Levy flight approach for feature selection, 2022, 1868-6478, 10.1007/s12530-022-09461-1 | |
2. | Krishna Gopal Dhal, Swarnajit Ray, Rebika Rai, Arunita Das, Archimedes Optimizer: Theory, Analysis, Improvements, and Applications, 2023, 1134-3060, 10.1007/s11831-022-09876-8 | |
3. | Jie Xing, Xinsen Zhou, Hanli Zhao, Huiling Chen, Ali Asghar Heidari, Elite levy spreading differential evolution via ABC shrink-wrap for multi-threshold segmentation of breast cancer images, 2023, 82, 17468094, 104592, 10.1016/j.bspc.2023.104592 | |
4. | Yudong Zhang, Juan Manuel Gorriz, Deepak Ranjan Nayak, Optimization Algorithms and Machine Learning Techniques in Medical Image Analysis, 2023, 20, 1551-0018, 5917, 10.3934/mbe.2023255 | |
5. | Rebika Rai, Krishna Gopal Dhal, Recent Developments in Equilibrium Optimizer Algorithm: Its Variants and Applications, 2023, 1134-3060, 10.1007/s11831-023-09923-y | |
6. | Heming Jia, Honghua Rao, Changsheng Wen, Seyedali Mirjalili, Crayfish optimization algorithm, 2023, 56, 0269-2821, 1919, 10.1007/s10462-023-10567-4 | |
7. | Leena Samantaray, Tanmaya Tapaswini Tripathy, Rutuparna Panda, A novel 3D differential entropy-based multi-class segmentation technique for brain MR image analysis, 2025, 100, 17468094, 107064, 10.1016/j.bspc.2024.107064 | |
8. | M. A. JiMing, Duan HongYu, Wang YuFan, Wang LiNa, Khan Bahadar Khan, Medical image segmentation based on simulated annealing and opposition-based learning island algorithm, 2024, 19, 1932-6203, e0307278, 10.1371/journal.pone.0307278 | |
9. | Mohammed Azmi Al-Betar, Iyad Abu Doush, Sharif Naser Makhadmeh, Ghazi Al-Naymat, Osama Ahmad Alomari, Mohammed A. Awadallah, Equilibrium optimizer: a comprehensive survey, 2023, 83, 1573-7721, 29617, 10.1007/s11042-023-16764-1 | |
10. | Huy-Du Dao, Thanh-Tung Nguyen, Ngoc-Kien Vu, Tuan-Linh Nguyen, 2024, Chapter 32, 978-3-031-62237-3, 284, 10.1007/978-3-031-62238-0_32 | |
11. | Huiying Jia, Design of fruit fly optimization algorithm based on Gaussian distribution and its application to image processing, 2024, 6, 27729419, 200090, 10.1016/j.sasc.2024.200090 |
Algorithm 1 Pseudo-code of HEOA algorithm |
Input: The color image Output: Segmentation color image Read the input and compute the histogram of three channels of color imageInitialize the parameters r1andr2 Initialize the random population Ci while L < Max_iter do Calculate the fitness values of EOA for (each hawk Ci) do Calculate Ceq, Xi Update the C using Eq. (25) Calculate the fitness function using Eq.(10) end for UpdateCbestif there is a better solution L=L+1 end while Get the best solution as the multilevel threshold K According the K segment the three channels of the images Get the segmentation images |
No. | Functions | Related basic functions | Dim | fmin |
CEC-1 | Rotated Bent Cigar Function | Bent Cigar Function | 30 | 100 |
CEC-2 | Rotated Discus Function | Discus Function | 30 | 200 |
CEC-3 | Shifted and Rotated Weierstrass Function | Weierstrass Function | 30 | 300 |
CEC-4 | Shifted and Rotated Schwefel's Function | Schwefel's Function | 30 | 400 |
CEC-5 | Shifted and Rotated Katsuura Function | Katsuura Function | 30 | 500 |
CEC-6 | Shifted and Rotated HappyCat Function | HappyCat Function | 30 | 600 |
CEC-7 | Shifted and Rotated HGBat Function | HGBat Function | 30 | 700 |
CEC-8 | Shifted and Rotated Expanded Griewank's plus Rosenbrock's Function | Griewank's Function Rosenbrock's Function | 30 | 800 |
CEC-9 | Shifted and Rotated Expanded Scaer's F6 Function | Expanded Scaer's F6 Function | 30 | 900 |
CEC-10 | Hybrid Function 1 (N = 3) | Schwefel's FunctionRastrigin's FunctionHigh Conditioned Elliptic Function | 30 | 1000 |
CEC-11 | Hybrid Function 2 (N = 4) | Griewank's Function Weierstrass Function Rosenbrock's Function Scaer's F6 Function | 30 | 1100 |
CEC-12 | Hybrid Function 3 (N = 5) | Katsuura FunctionHappyCat FunctionExpanded Griewank's plus Rosenbrock's Function Schwefel's FunctionAckley's Function | 30 | 1200 |
CEC-13 | Composition Function 1 (N = 5) | Rosenbrock's FunctionHigh Conditioned Elliptic FunctionBent Cigar FunctionDiscus FunctionHigh Conditioned Elliptic Function | 30 | 1300 |
CEC-14 | Composition Function 2 (N = 3) | Schwefel's FunctionRastrigin's FunctionHigh Conditioned Elliptic Function | 30 | 1400 |
CEC-15 | Composition Function 3 (N = 5) | HGBat FunctionRastrigin's FunctionSchwefel's FunctionWeierstrass FunctionHigh Conditioned Elliptic Function | 30 | 1500 |
Func. | HEOA | CSA | PSO | FPA | BA | EOA | ||||||
Mean | Std. | Mean | Std. | Mean | Std. | Mean | Std. | Mean | Std. | Mean | Std. | |
CEC-1 | 1.05E+05 | 1.54E+07 | 4.21E+05 | 1.47E+08 | 2.17E+09 | 3.53E+07 | 1.76E+08 | 7.02E+07 | 4.76E+08 | 2.86E+08 | 1.07E+05 | 3.69E+07 |
CEC-2 | 6.70E+06 | 1.70E+09 | 2.06E+04 | 5.26E+09 | 7.01E+10 | 3.53E+09 | 3.04E+10 | 4.70E+09 | 3.36E+10 | 6.82E+09 | 6.70E+07 | 4.05E+09 |
CEC-3 | 3.20E+02 | 7.12E-02 | 3.20E+02 | 1.51E-01 | 3.22E+02 | 9.57E-02 | 3.21E+02 | 1.43E-01 | 3.21E+02 | 2.10E-01 | 3.55E+02 | 1.47E-01 |
CEC-4 | 4.10E+02 | 1.75E+00 | 4.05E+02 | 9.03E+00 | 5.46E+02 | 1.29E+01 | 5.30E+02 | 1.61E+01 | 5.21E+02 | 9.70E+00 | 4.50E+02 | 2.39E+01 |
CEC-5 | 9.81E+02 | 1.50E+02 | 1.22E+03 | 3.30E+02 | 4.74E+03 | 2.40E+02 | 3.80E+03 | 3.97E+02 | 3.65E+03 | 3.52E+02 | 9.91E+02 | 2.97E+02 |
CEC-6 | 2.05E+03 | 4.75E+06 | 2.14E+03 | 2.33E+07 | 3.79E+09 | 9.03E+06 | 4.52E+09 | 1.14E+07 | 1.28E+08 | 2.71E+07 | 2.10E+03 | 1.37E+07 |
CEC-7 | 7.02E+02 | 1.24E+01 | 7.03E+02 | 4.37E+01 | 1.81E+03 | 1.40E+01 | 1.78E+03 | 2.70E+01 | 1.78E+03 | 4.99E+01 | 8.82E+02 | 2.49E+01 |
CEC-8 | 1.47E+03 | 1.03E+06 | 1.41E+04 | 3.25E+06 | 2.21E+09 | 1.05E+06 | 1.34E+09 | 1.63E+06 | 1.34E+09 | 3.78E+06 | 1.47E+04 | 1.08E+06 |
CEC-9 | 1.00E+03 | 7.30E+00 | 1.00E+03 | 4.77E+01 | 1.33E+03 | 2.45E+01 | 1.33E+03 | 3.46E+01 | 1.62E+03 | 5.15E+01 | 1.00E+04 | 3.38E+01 |
CEC-10 | 1.23E+03 | 4.82E+04 | 2.05E+03 | 3.09E+06 | 2.58E+09 | 4.14E+05 | 2.97E+07 | 8.13E+05 | 4.41E+08 | 4.49E+06 | 1.36E+04 | 7.89E+05 |
CEC-11 | 1.35E+03 | 4.52E+01 | 1.40E+03 | 7.94E+01 | 2.00E+03 | 6.39E+01 | 1.77E+03 | 1.02E+02 | 1.77E+03 | 9.80E+01 | 1.32E+04 | 1.24E+02 |
CEC-12 | 1.30E+03 | 1.03E+01 | 1.30E+03 | 1.75E+01 | 1.76E+03 | 1.22E+01 | 1.49E+03 | 1.28E+01 | 1.51E+03 | 3.25E+01 | 1.34E+05 | 1.58E+01 |
CEC-13 | 1.30E+03 | 8.67E+00 | 1.34E+03 | 1.49E+00 | 5.44E+05 | 1.86E+01 | 1.54E+03 | 3.03E+01 | 1.56E+03 | 2.48E+00 | 1.55E+05 | 3.24E+01 |
CEC-14 | 3.22E+03 | 2.32E+03 | 8.88E+03 | 4.24E+03 | 2.23E+04 | 2.92E+03 | 2.23E+04 | 4.76E+03 | 2.23E+04 | 5.46E+03 | 3.22E+04 | 3.59E+03 |
CEC-15 | 1.60E+03 | 3.36E+02 | 1.60E+03 | 6.49E+02 | 1.28E+04 | 7.10E+02 | 9.01E+03 | 1.34E+03 | 4.09E+03 | 1.05E+03 | 1.80E+03 | 1.27E+03 |
No. | Measures | Formulation | Reference |
1 | Uniformity measure | U=1−2×(k−1)×∑dj=1∑i∈Rj(fi−μj)2N×(fmax−fmin)2 | [54] |
2 | Peak Signal-to-Noise Ratio (PSNR) | PSNR=10×log10(2552MSE) MSE=1mnm−1∑i=1n−1∑j=1[I(i,j)−K(i,j)]2 |
[55] |
3 | Feature Similarity Index (FSIM) | FSIM=∑x=ηSL(x)⋅PCm(x)∑x=ηPCm(x) | [56] |
Image | K | EOA-Otsu | EOA-3DOtsu | HEOA-Otsu | HEOA-3DOtsu |
Test1 | 4 | 0.8628 | 0.8886 | 0.8973 | 0.9106 |
8 | 0.8657 | 0.8864 | 0.8957 | 0.9170 | |
12 | 0.9103 | 0.9332 | 0.9411 | 0.9580 | |
15 | 0.9293 | 0.9479 | 0.9565 | 0.9695 | |
Test2 | 4 | 0.8644 | 0.8799 | 0.8901 | 0.9015 |
8 | 0.8459 | 0.8674 | 0.8736 | 0.9135 | |
12 | 0.9298 | 0.9511 | 0.9569 | 0.9591 | |
15 | 0.9287 | 0.9474 | 0.9562 | 0.9671 | |
Test3 | 4 | 0.8586 | 0.8833 | 0.8919 | 0.9056 |
8 | 0.8599 | 0.8766 | 0.8859 | 0.9246 | |
12 | 0.9207 | 0.9370 | 0.9466 | 0.9661 | |
15 | 0.9049 | 0.9239 | 0.9358 | 0.9688 | |
Test4 | 4 | 0.8518 | 0.8660 | 0.8805 | 0.8963 |
8 | 0.8856 | 0.9060 | 0.9125 | 0.9221 | |
12 | 0.8823 | 0.9034 | 0.9143 | 0.9619 | |
15 | 0.9012 | 0.9260 | 0.9343 | 0.9742 | |
Test5 | 4 | 0.8379 | 0.8545 | 0.8645 | 0.8988 |
8 | 0.8648 | 0.8854 | 0.8992 | 0.9193 | |
12 | 0.9166 | 0.9305 | 0.9446 | 0.9659 | |
15 | 0.9055 | 0.9286 | 0.9345 | 0.9750 | |
Test6 | 4 | 0.8725 | 0.8902 | 0.9025 | 0.9157 |
8 | 0.8854 | 0.9040 | 0.9160 | 0.9189 | |
12 | 0.9267 | 0.9484 | 0.9566 | 0.9585 | |
15 | 0.8904 | 0.9095 | 0.9214 | 0.9663 | |
Test7 | 4 | 0.8708 | 0.8883 | 0.9009 | 0.9147 |
8 | 0.8845 | 0.9037 | 0.9155 | 0.9171 | |
12 | 0.9250 | 0.9478 | 0.9555 | 0.9581 | |
15 | 0.8898 | 0.9087 | 0.9202 | 0.9648 | |
Test8 | 4 | 0.8704 | 0.8871 | 0.9000 | 0.9143 |
8 | 0.8830 | 0.9017 | 0.9142 | 0.9155 | |
12 | 0.9232 | 0.9469 | 0.9535 | 0.9578 | |
15 | 0.8886 | 0.9079 | 0.9201 | 0.9629 | |
Test9 | 4 | 0.8716 | 0.8884 | 0.9011 | 0.9151 |
8 | 0.8844 | 0.9026 | 0.9157 | 0.9163 | |
12 | 0.9237 | 0.9470 | 0.9552 | 0.9596 | |
15 | 0.8897 | 0.9082 | 0.9217 | 0.9633 | |
Test10 | 4 | 0.8720 | 0.8896 | 0.9024 | 0.9171 |
8 | 0.8863 | 0.9027 | 0.9162 | 0.9179 | |
12 | 0.9247 | 0.9482 | 0.9561 | 0.9607 | |
15 | 0.8915 | 0.9089 | 0.9223 | 0.9645 |
Image | K | HEOA | HSOA | HWOA | CSA | FPA | PSO | BA |
Test1 | 4 | 0.9571 | 0.9415 | 0.9437 | 0.9245 | 0.9284 | 0.9262 | 0.9243 |
8 | 0.9674 | 0.9525 | 0.9594 | 0.9299 | 0.9309 | 0.9392 | 0.9373 | |
12 | 0.9744 | 0.9641 | 0.9654 | 0.9404 | 0.9456 | 0.9424 | 0.9457 | |
15 | 0.9795 | 0.9684 | 0.9617 | 0.9428 | 0.9441 | 0.9445 | 0.9458 | |
Test2 | 4 | 0.9544 | 0.9460 | 0.9460 | 0.9224 | 0.9167 | 0.9207 | 0.9216 |
8 | 0.9722 | 0.9583 | 0.9624 | 0.9442 | 0.9422 | 0.9425 | 0.9356 | |
12 | 0.9813 | 0.9717 | 0.9639 | 0.9437 | 0.9458 | 0.9526 | 0.9497 | |
15 | 0.9824 | 0.9698 | 0.9697 | 0.9499 | 0.9479 | 0.9468 | 0.9515 | |
Test3 | 4 | 0.9528 | 0.9437 | 0.9427 | 0.9244 | 0.9184 | 0.9184 | 0.9165 |
8 | 0.9626 | 0.9500 | 0.9482 | 0.9255 | 0.9274 | 0.9323 | 0.9290 | |
12 | 0.9782 | 0.9657 | 0.9625 | 0.9464 | 0.9453 | 0.9486 | 0.9406 | |
15 | 0.9812 | 0.9701 | 0.9683 | 0.9467 | 0.9450 | 0.9487 | 0.9513 | |
Test4 | 4 | 0.9518 | 0.9403 | 0.9435 | 0.9201 | 0.9171 | 0.9161 | 0.9187 |
8 | 0.9699 | 0.9589 | 0.9520 | 0.9327 | 0.9350 | 0.9331 | 0.9402 | |
12 | 0.9749 | 0.9623 | 0.9653 | 0.9385 | 0.9398 | 0.9409 | 0.9439 | |
15 | 0.9806 | 0.9717 | 0.9700 | 0.9486 | 0.9490 | 0.9457 | 0.9485 | |
Test5 | 4 | 0.9541 | 0.9410 | 0.9402 | 0.9257 | 0.9167 | 0.9206 | 0.9207 |
8 | 0.9679 | 0.9558 | 0.9560 | 0.9385 | 0.9306 | 0.9399 | 0.9392 | |
12 | 0.9769 | 0.9670 | 0.9618 | 0.9393 | 0.9441 | 0.9404 | 0.9455 | |
15 | 0.9808 | 0.9639 | 0.9678 | 0.9468 | 0.9508 | 0.9519 | 0.9467 | |
Test6 | 4 | 0.9507 | 0.9393 | 0.9373 | 0.9169 | 0.9197 | 0.9138 | 0.9154 |
8 | 0.9666 | 0.9504 | 0.9512 | 0.9383 | 0.9298 | 0.9355 | 0.9352 | |
12 | 0.9787 | 0.9644 | 0.9673 | 0.9492 | 0.9505 | 0.9474 | 0.9431 | |
15 | 0.9824 | 0.9713 | 0.9667 | 0.9470 | 0.9480 | 0.9498 | 0.9472 | |
Test7 | 4 | 0.9514 | 0.9397 | 0.9378 | 0.9183 | 0.9212 | 0.9150 | 0.9167 |
8 | 0.9667 | 0.9524 | 0.9529 | 0.9392 | 0.9300 | 0.9360 | 0.9366 | |
12 | 0.9802 | 0.9648 | 0.9675 | 0.9510 | 0.9521 | 0.9479 | 0.9433 | |
15 | 0.9841 | 0.9725 | 0.9680 | 0.9473 | 0.9483 | 0.9502 | 0.9487 | |
Test8 | 4 | 0.9508 | 0.9377 | 0.9365 | 0.9165 | 0.9196 | 0.9137 | 0.9161 |
8 | 0.9649 | 0.9522 | 0.9520 | 0.9383 | 0.9284 | 0.9349 | 0.9355 | |
12 | 0.9789 | 0.9642 | 0.9663 | 0.9507 | 0.9507 | 0.9479 | 0.9417 | |
15 | 0.9837 | 0.9715 | 0.9670 | 0.9468 | 0.9464 | 0.9497 | 0.9479 | |
Test9 | 4 | 0.9503 | 0.9374 | 0.9355 | 0.9158 | 0.9191 | 0.9118 | 0.9151 |
8 | 0.9633 | 0.9510 | 0.9500 | 0.9363 | 0.9272 | 0.9346 | 0.9351 | |
12 | 0.9772 | 0.9634 | 0.9655 | 0.9497 | 0.9488 | 0.9468 | 0.9402 | |
15 | 0.9820 | 0.9706 | 0.9655 | 0.9462 | 0.9455 | 0.9491 | 0.9460 | |
Test10 | 4 | 0.9505 | 0.9382 | 0.9375 | 0.9171 | 0.9207 | 0.9118 | 0.9167 |
8 | 0.9637 | 0.9519 | 0.9510 | 0.9367 | 0.9277 | 0.9360 | 0.9364 | |
12 | 0.9776 | 0.9653 | 0.9661 | 0.9511 | 0.9493 | 0.9472 | 0.9413 | |
15 | 0.9838 | 0.9710 | 0.9655 | 0.9481 | 0.9464 | 0.9504 | 0.9479 |
Image | K | HEOA | HSOA | HWOA | CSA | FPA | PSO | BA |
Test1 | 4 | 23.3863 | 23.2573 | 23.2526 | 23.0420 | 23.0405 | 23.0397 | 23.0539 |
8 | 29.2231 | 29.1025 | 29.0968 | 28.9021 | 28.9007 | 28.8989 | 28.9020 | |
12 | 32.5692 | 32.4393 | 32.4442 | 32.2300 | 32.2265 | 32.2282 | 32.2414 | |
15 | 33.3295 | 33.1947 | 33.1953 | 33.0002 | 32.9903 | 32.9903 | 32.9923 | |
Test2 | 4 | 24.4702 | 24.3443 | 24.3386 | 24.1472 | 24.1340 | 24.1342 | 24.1432 |
8 | 29.4260 | 29.3020 | 29.2936 | 29.0852 | 29.0987 | 29.0861 | 29.1020 | |
12 | 32.5786 | 32.4454 | 32.4470 | 32.2471 | 32.2464 | 32.2522 | 32.2424 | |
15 | 34.2153 | 34.0865 | 34.0884 | 33.8828 | 33.8824 | 33.8823 | 33.8887 | |
Test3 | 4 | 24.4769 | 24.3474 | 24.3453 | 24.1433 | 24.1406 | 24.1517 | 24.1495 |
8 | 29.9667 | 29.8287 | 29.8366 | 29.6330 | 29.6383 | 29.6298 | 29.6211 | |
12 | 32.6661 | 32.5412 | 32.5392 | 32.3369 | 32.3265 | 32.3288 | 32.3254 | |
15 | 34.7330 | 34.6031 | 34.6015 | 34.3994 | 34.3925 | 34.4084 | 34.3905 | |
Test4 | 4 | 24.7640 | 24.6386 | 24.6369 | 24.4284 | 24.4400 | 24.4423 | 24.4286 |
8 | 29.5467 | 29.4208 | 29.4154 | 29.2214 | 29.2193 | 29.2207 | 29.2104 | |
12 | 33.5823 | 33.4474 | 33.4548 | 33.2561 | 33.2435 | 33.2461 | 33.2509 | |
15 | 34.2159 | 34.0826 | 34.0820 | 33.8787 | 33.8740 | 33.8707 | 33.8853 | |
Test5 | 4 | 24.5319 | 24.3991 | 24.3994 | 24.2048 | 24.1918 | 24.1918 | 24.1924 |
8 | 29.5550 | 29.4198 | 29.4251 | 29.2137 | 29.2181 | 29.2237 | 29.2154 | |
12 | 34.3322 | 34.1972 | 34.1979 | 34.0010 | 33.9935 | 33.9870 | 33.9959 | |
15 | 35.0669 | 34.9409 | 34.9366 | 34.7387 | 34.7254 | 34.7407 | 34.7279 | |
Test6 | 4 | 25.2119 | 25.0871 | 25.0842 | 24.8695 | 24.8803 | 24.8734 | 24.8829 |
8 | 30.9087 | 30.7880 | 30.7821 | 30.5869 | 30.5821 | 30.5735 | 30.5700 | |
12 | 34.1912 | 34.0606 | 34.0591 | 33.8576 | 33.8505 | 33.8585 | 33.8626 | |
15 | 35.7843 | 35.6567 | 35.6607 | 35.4492 | 35.4505 | 35.4438 | 35.4423 | |
Test7 | 4 | 25.4053 | 25.1458 | 25.1450 | 24.8847 | 25.0748 | 24.9284 | 24.9402 |
8 | 31.0914 | 30.8883 | 30.8601 | 30.7788 | 30.5828 | 30.6162 | 30.6718 | |
12 | 34.3507 | 34.1142 | 34.1742 | 34.0164 | 33.9942 | 33.8766 | 33.8983 | |
15 | 35.9362 | 35.6969 | 35.7545 | 35.6007 | 35.4588 | 35.4937 | 35.6024 | |
Test8 | 4 | 25.5670 | 25.2034 | 25.2799 | 24.9384 | 25.1283 | 24.9787 | 25.0445 |
8 | 31.2698 | 31.0054 | 30.9921 | 30.8672 | 30.7368 | 30.6972 | 30.8372 | |
12 | 34.5283 | 34.2658 | 34.2137 | 34.2017 | 34.1031 | 33.9285 | 34.0632 | |
15 | 36.0846 | 35.7585 | 35.9118 | 35.6393 | 35.6021 | 35.4988 | 35.6352 | |
Test9 | 4 | 25.5622 | 25.0597 | 25.1265 | 24.7749 | 24.9583 | 24.9368 | 24.8720 |
8 | 31.1656 | 30.8841 | 30.8166 | 30.7155 | 30.6732 | 30.6568 | 30.8154 | |
12 | 34.4249 | 34.0884 | 34.1830 | 34.1183 | 33.9539 | 33.7553 | 33.9067 | |
15 | 36.0382 | 35.7553 | 35.7737 | 35.5952 | 35.5146 | 35.3593 | 35.5413 | |
Test10 | 4 | 25.4424 | 24.9891 | 25.1108 | 24.7329 | 24.7752 | 24.9286 | 24.8300 |
8 | 31.1315 | 30.7681 | 30.7427 | 30.5414 | 30.6599 | 30.5129 | 30.6245 | |
12 | 34.3961 | 33.9364 | 34.1416 | 33.9835 | 33.8675 | 33.7435 | 33.8129 | |
15 | 35.8866 | 35.7225 | 35.7457 | 35.4554 | 35.4165 | 35.2905 | 35.3448 |
Image | K | HEOA | HSOA | HWOA | CSA | FPA | PSO | BA |
Test1 | 4 | 0.9367 | 0.9236 | 0.9225 | 0.9094 | 0.9039 | 0.8944 | 0.9087 |
8 | 0.9517 | 0.9414 | 0.9390 | 0.9036 | 0.9077 | 0.9157 | 0.9084 | |
12 | 0.9587 | 0.9433 | 0.9473 | 0.9135 | 0.9149 | 0.9172 | 0.9135 | |
15 | 0.9668 | 0.9511 | 0.9540 | 0.9271 | 0.9331 | 0.9287 | 0.9284 | |
Test2 | 4 | 0.9356 | 0.9227 | 0.9262 | 0.9006 | 0.8943 | 0.8936 | 0.9065 |
8 | 0.9584 | 0.9422 | 0.9438 | 0.9221 | 0.9123 | 0.9122 | 0.9230 | |
12 | 0.9632 | 0.9548 | 0.9563 | 0.9283 | 0.9305 | 0.9331 | 0.9233 | |
15 | 0.9633 | 0.9553 | 0.9506 | 0.9242 | 0.9251 | 0.9255 | 0.9202 | |
Test3 | 4 | 0.9325 | 0.9197 | 0.9181 | 0.9003 | 0.9077 | 0.8898 | 0.9078 |
8 | 0.9477 | 0.9333 | 0.9362 | 0.9060 | 0.9062 | 0.9001 | 0.9048 | |
12 | 0.9599 | 0.9482 | 0.9483 | 0.9289 | 0.9289 | 0.9190 | 0.9162 | |
15 | 0.9607 | 0.9487 | 0.9468 | 0.9309 | 0.9251 | 0.9325 | 0.9358 | |
Test4 | 4 | 0.9385 | 0.9213 | 0.9207 | 0.9065 | 0.9063 | 0.8982 | 0.8954 |
8 | 0.9485 | 0.9362 | 0.9349 | 0.9057 | 0.9058 | 0.9115 | 0.9144 | |
12 | 0.9580 | 0.9487 | 0.9498 | 0.9240 | 0.9298 | 0.9154 | 0.9200 | |
15 | 0.9637 | 0.9457 | 0.9532 | 0.9229 | 0.9227 | 0.9321 | 0.9237 | |
Test5 | 4 | 0.9332 | 0.9213 | 0.9264 | 0.8894 | 0.9071 | 0.9082 | 0.8960 |
8 | 0.9469 | 0.9377 | 0.9361 | 0.9085 | 0.9141 | 0.9083 | 0.9106 | |
12 | 0.9642 | 0.9438 | 0.9503 | 0.9210 | 0.9254 | 0.9187 | 0.9270 | |
15 | 0.9687 | 0.9484 | 0.9489 | 0.9240 | 0.9174 | 0.9240 | 0.9267 | |
Test6 | 4 | 0.9304 | 0.9238 | 0.9186 | 0.8868 | 0.9055 | 0.8972 | 0.9054 |
8 | 0.9518 | 0.9317 | 0.9372 | 0.9027 | 0.9099 | 0.9117 | 0.9085 | |
12 | 0.9579 | 0.9492 | 0.9460 | 0.9280 | 0.9270 | 0.9258 | 0.9158 | |
15 | 0.9682 | 0.9517 | 0.9490 | 0.9289 | 0.9204 | 0.9315 | 0.9250 | |
Test7 | 4 | 0.9313 | 0.9252 | 0.9198 | 0.8882 | 0.9057 | 0.8984 | 0.9060 |
8 | 0.9536 | 0.9322 | 0.9384 | 0.9035 | 0.9109 | 0.9127 | 0.9087 | |
12 | 0.9590 | 0.9498 | 0.9477 | 0.9287 | 0.9280 | 0.9273 | 0.9177 | |
15 | 0.9699 | 0.9524 | 0.9510 | 0.9292 | 0.9222 | 0.9335 | 0.9258 | |
Test8 | 4 | 0.9294 | 0.9239 | 0.9181 | 0.8877 | 0.9044 | 0.8970 | 0.9058 |
8 | 0.9518 | 0.9309 | 0.9381 | 0.9029 | 0.9096 | 0.9116 | 0.9070 | |
12 | 0.9573 | 0.9487 | 0.9470 | 0.9276 | 0.9263 | 0.9263 | 0.9175 | |
15 | 0.9682 | 0.9513 | 0.9494 | 0.9280 | 0.9216 | 0.9324 | 0.9240 | |
Test9 | 4 | 0.9292 | 0.9234 | 0.9161 | 0.8866 | 0.9038 | 0.8956 | 0.9052 |
8 | 0.9506 | 0.9296 | 0.9368 | 0.9010 | 0.9089 | 0.9096 | 0.9064 | |
12 | 0.9569 | 0.9469 | 0.9470 | 0.9273 | 0.9250 | 0.9262 | 0.9160 | |
15 | 0.9676 | 0.9501 | 0.9474 | 0.9275 | 0.9216 | 0.9308 | 0.9236 | |
Test10 | 4 | 0.9296 | 0.9246 | 0.9170 | 0.8874 | 0.9046 | 0.8962 | 0.9057 |
8 | 0.9509 | 0.9306 | 0.9378 | 0.9025 | 0.9093 | 0.9098 | 0.9069 | |
12 | 0.9589 | 0.9483 | 0.9490 | 0.9275 | 0.9261 | 0.9277 | 0.9165 | |
15 | 0.9678 | 0.9501 | 0.9479 | 0.9287 | 0.9232 | 0.9316 | 0.9240 |
Image | K | HEOA | HSOA | HWOA | CSA | FPA | PSO | BA |
Test1 | 4 | 1.2145 | 1.2251 | 1.2247 | 1.2442 | 1.2405 | 1.2406 | 1.2389 |
8 | 1.3154 | 1.3254 | 1.3260 | 1.3452 | 1.3422 | 1.3386 | 1.3385 | |
12 | 1.5172 | 1.5274 | 1.5279 | 1.5448 | 1.5467 | 1.5451 | 1.5466 | |
15 | 1.8157 | 1.8260 | 1.8258 | 1.8359 | 1.8435 | 1.8376 | 1.8439 | |
Test2 | 4 | 1.2149 | 1.2259 | 1.2255 | 1.2363 | 1.2392 | 1.2436 | 1.2365 |
8 | 1.3154 | 1.3262 | 1.3263 | 1.3388 | 1.3411 | 1.3401 | 1.3371 | |
12 | 1.5181 | 1.5286 | 1.5288 | 1.5423 | 1.5449 | 1.5466 | 1.5389 | |
15 | 1.8158 | 1.8266 | 1.8266 | 1.8419 | 1.8398 | 1.8409 | 1.8424 | |
Test3 | 4 | 1.2151 | 1.2257 | 1.2258 | 1.2354 | 1.2367 | 1.2445 | 1.2367 |
8 | 1.3158 | 1.3268 | 1.3265 | 1.3402 | 1.3411 | 1.3456 | 1.3436 | |
12 | 1.5191 | 1.5292 | 1.5292 | 1.5394 | 1.5406 | 1.5474 | 1.5487 | |
15 | 1.8164 | 1.8270 | 1.8273 | 1.8462 | 1.8369 | 1.8437 | 1.8413 | |
Test4 | 4 | 1.2151 | 1.2254 | 1.2253 | 1.2444 | 1.2358 | 1.2358 | 1.2409 |
8 | 1.3166 | 1.3268 | 1.3271 | 1.3432 | 1.3398 | 1.3458 | 1.3461 | |
12 | 1.5192 | 1.5299 | 1.5301 | 1.5421 | 1.5476 | 1.5483 | 1.5465 | |
15 | 1.8166 | 1.8275 | 1.8269 | 1.8390 | 1.8466 | 1.8422 | 1.8425 | |
Test5 | 4 | 1.2161 | 1.2269 | 1.2269 | 1.2388 | 1.2447 | 1.2368 | 1.2401 |
8 | 1.3167 | 1.3273 | 1.3273 | 1.3456 | 1.3396 | 1.3382 | 1.3431 | |
12 | 1.5201 | 1.5304 | 1.5302 | 1.5471 | 1.5428 | 1.5454 | 1.5401 | |
15 | 1.8169 | 1.8274 | 1.8278 | 1.8431 | 1.8379 | 1.8456 | 1.8405 | |
Test6 | 4 | 1.2168 | 1.2271 | 1.2268 | 1.2372 | 1.2413 | 1.2412 | 1.2383 |
8 | 1.3172 | 1.3281 | 1.3273 | 1.3412 | 1.3407 | 1.3448 | 1.3379 | |
12 | 1.5206 | 1.5307 | 1.5313 | 1.5491 | 1.5408 | 1.5445 | 1.5493 | |
15 | 1.8169 | 1.8278 | 1.8270 | 1.8395 | 1.8463 | 1.8410 | 1.8428 | |
Test7 | 4 | 1.2183 | 1.2275 | 1.2282 | 1.2373 | 1.2421 | 1.2429 | 1.2399 |
8 | 1.3179 | 1.3284 | 1.3291 | 1.3429 | 1.3420 | 1.3463 | 1.3391 | |
12 | 1.5219 | 1.5326 | 1.5316 | 1.5510 | 1.5410 | 1.5462 | 1.5506 | |
15 | 1.8179 | 1.8293 | 1.8289 | 1.8408 | 1.8466 | 1.8410 | 1.8440 | |
Test8 | 4 | 1.2173 | 1.2270 | 1.2272 | 1.2371 | 1.2403 | 1.2424 | 1.2393 |
8 | 1.3167 | 1.3278 | 1.3282 | 1.3409 | 1.3401 | 1.3447 | 1.3386 | |
12 | 1.5217 | 1.5308 | 1.5303 | 1.5497 | 1.5398 | 1.5460 | 1.5498 | |
15 | 1.8172 | 1.8273 | 1.8284 | 1.8396 | 1.8446 | 1.8397 | 1.8424 | |
Test9 | 4 | 1.2154 | 1.2265 | 1.2258 | 1.2357 | 1.2391 | 1.2409 | 1.2376 |
8 | 1.3166 | 1.3268 | 1.3277 | 1.3398 | 1.3389 | 1.3428 | 1.3375 | |
12 | 1.5204 | 1.5304 | 1.5291 | 1.5482 | 1.5397 | 1.5453 | 1.5485 | |
15 | 1.8154 | 1.8272 | 1.8281 | 1.8395 | 1.8431 | 1.8385 | 1.8422 | |
Test10 | 4 | 1.2155 | 1.2282 | 1.2270 | 1.2377 | 1.2404 | 1.2419 | 1.2380 |
8 | 1.3172 | 1.3283 | 1.3279 | 1.3401 | 1.3399 | 1.3444 | 1.3391 | |
12 | 1.5207 | 1.5319 | 1.5305 | 1.5494 | 1.5397 | 1.5460 | 1.5495 | |
15 | 1.8160 | 1.8272 | 1.8297 | 1.8400 | 1.8440 | 1.8393 | 1.8442 |
K | HEOA | HSOA | HWOA | CSA | FPA | PSO | BA |
4 | 3.1581 | 3.4458 | 3.5147 | 3.4581 | 3.6152 | 3.9951 | 3.8547 |
8 | 2.9315 | 3.5514 | 3.6891 | 3.5125 | 3.9156 | 3.9514 | 3.6984 |
12 | 1.9984 | 2.5589 | 3.6661 | 3.1285 | 3.9991 | 3.9541 | 3.8854 |
15 | 1.7415 | 2.6518 | 3.6581 | 3.1814 | 3.8147 | 3.8574 | 3.7781 |
Overall | 2.4574 | 3.0520 | 3.6320 | 3.3201 | 3.8362 | 3.9395 | 3.8042 |
Images | K | HSOA | HWOA | CSA | FPA | PSO |
Test1 | 4 | P < 0.05 | P < 0.05 | P < 0.05 | P < 0.05 | P < 0.05 |
8 | P > 0.05 | P < 0.05 | P < 0.05 | P < 0.05 | P < 0.05 | |
12 | P < 0.05 | P < 0.05 | P < 0.05 | P < 0.05 | P < 0.05 | |
15 | P < 0.05 | P > 0.05 | P < 0.05 | P < 0.05 | P < 0.05 | |
Test2 | 4 | P < 0.05 | P < 0.05 | P < 0.05 | P > 0.05 | P < 0.05 |
8 | P < 0.05 | P < 0.05 | P < 0.05 | P < 0.05 | P < 0.05 | |
12 | P < 0.05 | P < 0.05 | P < 0.05 | P < 0.05 | P < 0.05 | |
15 | P < 0.05 | P < 0.05 | P < 0.05 | P < 0.05 | P < 0.05 | |
Test3 | 4 | P < 0.05 | P < 0.05 | P < 0.05 | P < 0.05 | P < 0.05 |
8 | P < 0.05 | P < 0.05 | P < 0.05 | P < 0.05 | P < 0.05 | |
12 | P < 0.05 | P < 0.05 | P < 0.05 | P < 0.05 | P < 0.05 | |
15 | P < 0.05 | P < 0.05 | P < 0.05 | P < 0.05 | P < 0.05 | |
Test4 | 4 | P < 0.05 | P < 0.05 | P < 0.05 | P < 0.05 | P < 0.05 |
8 | P > 0.05 | P < 0.05 | P < 0.05 | P < 0.05 | P < 0.05 | |
12 | P < 0.05 | P > 0.05 | P < 0.05 | P < 0.05 | P < 0.05 | |
15 | P < 0.05 | P < 0.05 | P < 0.05 | P < 0.05 | P < 0.05 | |
Test5 | 4 | P < 0.05 | P < 0.05 | P < 0.05 | P < 0.05 | P < 0.05 |
8 | P < 0.05 | P < 0.05 | P < 0.05 | P < 0.05 | P < 0.05 | |
12 | P < 0.05 | P < 0.05 | P < 0.05 | P < 0.05 | P < 0.05 | |
15 | P < 0.05 | P < 0.05 | P < 0.05 | P < 0.05 | P < 0.05 | |
Test6 | 4 | P > 0.05 | P < 0.05 | P < 0.05 | P < 0.05 | P < 0.05 |
8 | P < 0.05 | P < 0.05 | P < 0.05 | P < 0.05 | P < 0.05 | |
12 | P < 0.05 | P < 0.05 | P < 0.05 | P < 0.05 | P < 0.05 | |
15 | P < 0.05 | P < 0.05 | P < 0.05 | P < 0.05 | P < 0.05 | |
Test7 | 4 | P < 0.05 | P < 0.05 | P > 0.05 | P < 0.05 | P < 0.05 |
8 | P < 0.05 | P < 0.05 | P < 0.05 | P < 0.05 | P < 0.05 | |
12 | P < 0.05 | P < 0.05 | P < 0.05 | P < 0.05 | P < 0.05 | |
15 | P < 0.05 | P < 0.05 | P < 0.05 | P < 0.05 | P < 0.05 | |
Test8 | 4 | P > 0.05 | P < 0.05 | P < 0.05 | P > 0.05 | P < 0.05 |
8 | P < 0.05 | P > 0.05 | P < 0.05 | P < 0.05 | P < 0.05 | |
12 | P < 0.05 | P < 0.05 | P < 0.05 | P < 0.05 | P < 0.05 | |
15 | P < 0.05 | P < 0.05 | P < 0.05 | P < 0.05 | P < 0.05 | |
Test9 | 4 | P < 0.05 | P < 0.05 | P < 0.05 | P < 0.05 | P < 0.05 |
8 | P < 0.05 | P > 0.05 | P < 0.05 | P < 0.05 | P < 0.05 | |
12 | P < 0.05 | P < 0.05 | P < 0.05 | P < 0.05 | P < 0.05 | |
15 | P < 0.05 | P < 0.05 | P < 0.05 | P < 0.05 | P < 0.05 | |
Test10 | 4 | P < 0.05 | P > 0.05 | P > 0.05 | P < 0.05 | P < 0.05 |
8 | P < 0.05 | P < 0.05 | P < 0.05 | P < 0.05 | P < 0.05 | |
12 | P < 0.05 | P < 0.05 | P < 0.05 | P < 0.05 | P < 0.05 | |
15 | P < 0.05 | P < 0.05 | P < 0.05 | P < 0.05 | P < 0.05 |
Method | R (%) | P (%) | AP (%) | VAL (%) |
FCM | 84.94 | 90.88 | 91.23 | 88.47 |
PCNN | 80.46 | 90.69 | 81.46 | 79.46 |
DLA | 70.12 | 92.95 | 78.32 | 77.57 |
BMPA | 93.19 | 91.28 | 91.45 | 90.38 |
Algorithm 1 Pseudo-code of HEOA algorithm |
Input: The color image Output: Segmentation color image Read the input and compute the histogram of three channels of color imageInitialize the parameters r1andr2 Initialize the random population Ci while L < Max_iter do Calculate the fitness values of EOA for (each hawk Ci) do Calculate Ceq, Xi Update the C using Eq. (25) Calculate the fitness function using Eq.(10) end for UpdateCbestif there is a better solution L=L+1 end while Get the best solution as the multilevel threshold K According the K segment the three channels of the images Get the segmentation images |
Algorithm | Parameters | Value |
EOA | c1 | 2 |
c2 | 2 | |
CSA [50] | AP | 0.5 |
FPA [51] | P | 0.5 |
PSO [52] | Swam size Cognitive, social acceleration Inertial weight | 2002, 20.95–0.4 |
BA [53] | β | (0, 1) |
HEOA | Levy | 1.5 |
No. | Functions | Related basic functions | Dim | fmin |
CEC-1 | Rotated Bent Cigar Function | Bent Cigar Function | 30 | 100 |
CEC-2 | Rotated Discus Function | Discus Function | 30 | 200 |
CEC-3 | Shifted and Rotated Weierstrass Function | Weierstrass Function | 30 | 300 |
CEC-4 | Shifted and Rotated Schwefel's Function | Schwefel's Function | 30 | 400 |
CEC-5 | Shifted and Rotated Katsuura Function | Katsuura Function | 30 | 500 |
CEC-6 | Shifted and Rotated HappyCat Function | HappyCat Function | 30 | 600 |
CEC-7 | Shifted and Rotated HGBat Function | HGBat Function | 30 | 700 |
CEC-8 | Shifted and Rotated Expanded Griewank's plus Rosenbrock's Function | Griewank's Function Rosenbrock's Function | 30 | 800 |
CEC-9 | Shifted and Rotated Expanded Scaer's F6 Function | Expanded Scaer's F6 Function | 30 | 900 |
CEC-10 | Hybrid Function 1 (N = 3) | Schwefel's FunctionRastrigin's FunctionHigh Conditioned Elliptic Function | 30 | 1000 |
CEC-11 | Hybrid Function 2 (N = 4) | Griewank's Function Weierstrass Function Rosenbrock's Function Scaer's F6 Function | 30 | 1100 |
CEC-12 | Hybrid Function 3 (N = 5) | Katsuura FunctionHappyCat FunctionExpanded Griewank's plus Rosenbrock's Function Schwefel's FunctionAckley's Function | 30 | 1200 |
CEC-13 | Composition Function 1 (N = 5) | Rosenbrock's FunctionHigh Conditioned Elliptic FunctionBent Cigar FunctionDiscus FunctionHigh Conditioned Elliptic Function | 30 | 1300 |
CEC-14 | Composition Function 2 (N = 3) | Schwefel's FunctionRastrigin's FunctionHigh Conditioned Elliptic Function | 30 | 1400 |
CEC-15 | Composition Function 3 (N = 5) | HGBat FunctionRastrigin's FunctionSchwefel's FunctionWeierstrass FunctionHigh Conditioned Elliptic Function | 30 | 1500 |
Func. | HEOA | CSA | PSO | FPA | BA | EOA | ||||||
Mean | Std. | Mean | Std. | Mean | Std. | Mean | Std. | Mean | Std. | Mean | Std. | |
CEC-1 | 1.05E+05 | 1.54E+07 | 4.21E+05 | 1.47E+08 | 2.17E+09 | 3.53E+07 | 1.76E+08 | 7.02E+07 | 4.76E+08 | 2.86E+08 | 1.07E+05 | 3.69E+07 |
CEC-2 | 6.70E+06 | 1.70E+09 | 2.06E+04 | 5.26E+09 | 7.01E+10 | 3.53E+09 | 3.04E+10 | 4.70E+09 | 3.36E+10 | 6.82E+09 | 6.70E+07 | 4.05E+09 |
CEC-3 | 3.20E+02 | 7.12E-02 | 3.20E+02 | 1.51E-01 | 3.22E+02 | 9.57E-02 | 3.21E+02 | 1.43E-01 | 3.21E+02 | 2.10E-01 | 3.55E+02 | 1.47E-01 |
CEC-4 | 4.10E+02 | 1.75E+00 | 4.05E+02 | 9.03E+00 | 5.46E+02 | 1.29E+01 | 5.30E+02 | 1.61E+01 | 5.21E+02 | 9.70E+00 | 4.50E+02 | 2.39E+01 |
CEC-5 | 9.81E+02 | 1.50E+02 | 1.22E+03 | 3.30E+02 | 4.74E+03 | 2.40E+02 | 3.80E+03 | 3.97E+02 | 3.65E+03 | 3.52E+02 | 9.91E+02 | 2.97E+02 |
CEC-6 | 2.05E+03 | 4.75E+06 | 2.14E+03 | 2.33E+07 | 3.79E+09 | 9.03E+06 | 4.52E+09 | 1.14E+07 | 1.28E+08 | 2.71E+07 | 2.10E+03 | 1.37E+07 |
CEC-7 | 7.02E+02 | 1.24E+01 | 7.03E+02 | 4.37E+01 | 1.81E+03 | 1.40E+01 | 1.78E+03 | 2.70E+01 | 1.78E+03 | 4.99E+01 | 8.82E+02 | 2.49E+01 |
CEC-8 | 1.47E+03 | 1.03E+06 | 1.41E+04 | 3.25E+06 | 2.21E+09 | 1.05E+06 | 1.34E+09 | 1.63E+06 | 1.34E+09 | 3.78E+06 | 1.47E+04 | 1.08E+06 |
CEC-9 | 1.00E+03 | 7.30E+00 | 1.00E+03 | 4.77E+01 | 1.33E+03 | 2.45E+01 | 1.33E+03 | 3.46E+01 | 1.62E+03 | 5.15E+01 | 1.00E+04 | 3.38E+01 |
CEC-10 | 1.23E+03 | 4.82E+04 | 2.05E+03 | 3.09E+06 | 2.58E+09 | 4.14E+05 | 2.97E+07 | 8.13E+05 | 4.41E+08 | 4.49E+06 | 1.36E+04 | 7.89E+05 |
CEC-11 | 1.35E+03 | 4.52E+01 | 1.40E+03 | 7.94E+01 | 2.00E+03 | 6.39E+01 | 1.77E+03 | 1.02E+02 | 1.77E+03 | 9.80E+01 | 1.32E+04 | 1.24E+02 |
CEC-12 | 1.30E+03 | 1.03E+01 | 1.30E+03 | 1.75E+01 | 1.76E+03 | 1.22E+01 | 1.49E+03 | 1.28E+01 | 1.51E+03 | 3.25E+01 | 1.34E+05 | 1.58E+01 |
CEC-13 | 1.30E+03 | 8.67E+00 | 1.34E+03 | 1.49E+00 | 5.44E+05 | 1.86E+01 | 1.54E+03 | 3.03E+01 | 1.56E+03 | 2.48E+00 | 1.55E+05 | 3.24E+01 |
CEC-14 | 3.22E+03 | 2.32E+03 | 8.88E+03 | 4.24E+03 | 2.23E+04 | 2.92E+03 | 2.23E+04 | 4.76E+03 | 2.23E+04 | 5.46E+03 | 3.22E+04 | 3.59E+03 |
CEC-15 | 1.60E+03 | 3.36E+02 | 1.60E+03 | 6.49E+02 | 1.28E+04 | 7.10E+02 | 9.01E+03 | 1.34E+03 | 4.09E+03 | 1.05E+03 | 1.80E+03 | 1.27E+03 |
No. | Measures | Formulation | Reference |
1 | Uniformity measure | U=1−2×(k−1)×∑dj=1∑i∈Rj(fi−μj)2N×(fmax−fmin)2 | [54] |
2 | Peak Signal-to-Noise Ratio (PSNR) | PSNR=10×log10(2552MSE) MSE=1mnm−1∑i=1n−1∑j=1[I(i,j)−K(i,j)]2 |
[55] |
3 | Feature Similarity Index (FSIM) | FSIM=∑x=ηSL(x)⋅PCm(x)∑x=ηPCm(x) | [56] |
Image | K | EOA-Otsu | EOA-3DOtsu | HEOA-Otsu | HEOA-3DOtsu |
Test1 | 4 | 0.8628 | 0.8886 | 0.8973 | 0.9106 |
8 | 0.8657 | 0.8864 | 0.8957 | 0.9170 | |
12 | 0.9103 | 0.9332 | 0.9411 | 0.9580 | |
15 | 0.9293 | 0.9479 | 0.9565 | 0.9695 | |
Test2 | 4 | 0.8644 | 0.8799 | 0.8901 | 0.9015 |
8 | 0.8459 | 0.8674 | 0.8736 | 0.9135 | |
12 | 0.9298 | 0.9511 | 0.9569 | 0.9591 | |
15 | 0.9287 | 0.9474 | 0.9562 | 0.9671 | |
Test3 | 4 | 0.8586 | 0.8833 | 0.8919 | 0.9056 |
8 | 0.8599 | 0.8766 | 0.8859 | 0.9246 | |
12 | 0.9207 | 0.9370 | 0.9466 | 0.9661 | |
15 | 0.9049 | 0.9239 | 0.9358 | 0.9688 | |
Test4 | 4 | 0.8518 | 0.8660 | 0.8805 | 0.8963 |
8 | 0.8856 | 0.9060 | 0.9125 | 0.9221 | |
12 | 0.8823 | 0.9034 | 0.9143 | 0.9619 | |
15 | 0.9012 | 0.9260 | 0.9343 | 0.9742 | |
Test5 | 4 | 0.8379 | 0.8545 | 0.8645 | 0.8988 |
8 | 0.8648 | 0.8854 | 0.8992 | 0.9193 | |
12 | 0.9166 | 0.9305 | 0.9446 | 0.9659 | |
15 | 0.9055 | 0.9286 | 0.9345 | 0.9750 | |
Test6 | 4 | 0.8725 | 0.8902 | 0.9025 | 0.9157 |
8 | 0.8854 | 0.9040 | 0.9160 | 0.9189 | |
12 | 0.9267 | 0.9484 | 0.9566 | 0.9585 | |
15 | 0.8904 | 0.9095 | 0.9214 | 0.9663 | |
Test7 | 4 | 0.8708 | 0.8883 | 0.9009 | 0.9147 |
8 | 0.8845 | 0.9037 | 0.9155 | 0.9171 | |
12 | 0.9250 | 0.9478 | 0.9555 | 0.9581 | |
15 | 0.8898 | 0.9087 | 0.9202 | 0.9648 | |
Test8 | 4 | 0.8704 | 0.8871 | 0.9000 | 0.9143 |
8 | 0.8830 | 0.9017 | 0.9142 | 0.9155 | |
12 | 0.9232 | 0.9469 | 0.9535 | 0.9578 | |
15 | 0.8886 | 0.9079 | 0.9201 | 0.9629 | |
Test9 | 4 | 0.8716 | 0.8884 | 0.9011 | 0.9151 |
8 | 0.8844 | 0.9026 | 0.9157 | 0.9163 | |
12 | 0.9237 | 0.9470 | 0.9552 | 0.9596 | |
15 | 0.8897 | 0.9082 | 0.9217 | 0.9633 | |
Test10 | 4 | 0.8720 | 0.8896 | 0.9024 | 0.9171 |
8 | 0.8863 | 0.9027 | 0.9162 | 0.9179 | |
12 | 0.9247 | 0.9482 | 0.9561 | 0.9607 | |
15 | 0.8915 | 0.9089 | 0.9223 | 0.9645 |
Image | K | HEOA | HSOA | HWOA | CSA | FPA | PSO | BA |
Test1 | 4 | 0.9571 | 0.9415 | 0.9437 | 0.9245 | 0.9284 | 0.9262 | 0.9243 |
8 | 0.9674 | 0.9525 | 0.9594 | 0.9299 | 0.9309 | 0.9392 | 0.9373 | |
12 | 0.9744 | 0.9641 | 0.9654 | 0.9404 | 0.9456 | 0.9424 | 0.9457 | |
15 | 0.9795 | 0.9684 | 0.9617 | 0.9428 | 0.9441 | 0.9445 | 0.9458 | |
Test2 | 4 | 0.9544 | 0.9460 | 0.9460 | 0.9224 | 0.9167 | 0.9207 | 0.9216 |
8 | 0.9722 | 0.9583 | 0.9624 | 0.9442 | 0.9422 | 0.9425 | 0.9356 | |
12 | 0.9813 | 0.9717 | 0.9639 | 0.9437 | 0.9458 | 0.9526 | 0.9497 | |
15 | 0.9824 | 0.9698 | 0.9697 | 0.9499 | 0.9479 | 0.9468 | 0.9515 | |
Test3 | 4 | 0.9528 | 0.9437 | 0.9427 | 0.9244 | 0.9184 | 0.9184 | 0.9165 |
8 | 0.9626 | 0.9500 | 0.9482 | 0.9255 | 0.9274 | 0.9323 | 0.9290 | |
12 | 0.9782 | 0.9657 | 0.9625 | 0.9464 | 0.9453 | 0.9486 | 0.9406 | |
15 | 0.9812 | 0.9701 | 0.9683 | 0.9467 | 0.9450 | 0.9487 | 0.9513 | |
Test4 | 4 | 0.9518 | 0.9403 | 0.9435 | 0.9201 | 0.9171 | 0.9161 | 0.9187 |
8 | 0.9699 | 0.9589 | 0.9520 | 0.9327 | 0.9350 | 0.9331 | 0.9402 | |
12 | 0.9749 | 0.9623 | 0.9653 | 0.9385 | 0.9398 | 0.9409 | 0.9439 | |
15 | 0.9806 | 0.9717 | 0.9700 | 0.9486 | 0.9490 | 0.9457 | 0.9485 | |
Test5 | 4 | 0.9541 | 0.9410 | 0.9402 | 0.9257 | 0.9167 | 0.9206 | 0.9207 |
8 | 0.9679 | 0.9558 | 0.9560 | 0.9385 | 0.9306 | 0.9399 | 0.9392 | |
12 | 0.9769 | 0.9670 | 0.9618 | 0.9393 | 0.9441 | 0.9404 | 0.9455 | |
15 | 0.9808 | 0.9639 | 0.9678 | 0.9468 | 0.9508 | 0.9519 | 0.9467 | |
Test6 | 4 | 0.9507 | 0.9393 | 0.9373 | 0.9169 | 0.9197 | 0.9138 | 0.9154 |
8 | 0.9666 | 0.9504 | 0.9512 | 0.9383 | 0.9298 | 0.9355 | 0.9352 | |
12 | 0.9787 | 0.9644 | 0.9673 | 0.9492 | 0.9505 | 0.9474 | 0.9431 | |
15 | 0.9824 | 0.9713 | 0.9667 | 0.9470 | 0.9480 | 0.9498 | 0.9472 | |
Test7 | 4 | 0.9514 | 0.9397 | 0.9378 | 0.9183 | 0.9212 | 0.9150 | 0.9167 |
8 | 0.9667 | 0.9524 | 0.9529 | 0.9392 | 0.9300 | 0.9360 | 0.9366 | |
12 | 0.9802 | 0.9648 | 0.9675 | 0.9510 | 0.9521 | 0.9479 | 0.9433 | |
15 | 0.9841 | 0.9725 | 0.9680 | 0.9473 | 0.9483 | 0.9502 | 0.9487 | |
Test8 | 4 | 0.9508 | 0.9377 | 0.9365 | 0.9165 | 0.9196 | 0.9137 | 0.9161 |
8 | 0.9649 | 0.9522 | 0.9520 | 0.9383 | 0.9284 | 0.9349 | 0.9355 | |
12 | 0.9789 | 0.9642 | 0.9663 | 0.9507 | 0.9507 | 0.9479 | 0.9417 | |
15 | 0.9837 | 0.9715 | 0.9670 | 0.9468 | 0.9464 | 0.9497 | 0.9479 | |
Test9 | 4 | 0.9503 | 0.9374 | 0.9355 | 0.9158 | 0.9191 | 0.9118 | 0.9151 |
8 | 0.9633 | 0.9510 | 0.9500 | 0.9363 | 0.9272 | 0.9346 | 0.9351 | |
12 | 0.9772 | 0.9634 | 0.9655 | 0.9497 | 0.9488 | 0.9468 | 0.9402 | |
15 | 0.9820 | 0.9706 | 0.9655 | 0.9462 | 0.9455 | 0.9491 | 0.9460 | |
Test10 | 4 | 0.9505 | 0.9382 | 0.9375 | 0.9171 | 0.9207 | 0.9118 | 0.9167 |
8 | 0.9637 | 0.9519 | 0.9510 | 0.9367 | 0.9277 | 0.9360 | 0.9364 | |
12 | 0.9776 | 0.9653 | 0.9661 | 0.9511 | 0.9493 | 0.9472 | 0.9413 | |
15 | 0.9838 | 0.9710 | 0.9655 | 0.9481 | 0.9464 | 0.9504 | 0.9479 |
Image | K | HEOA | HSOA | HWOA | CSA | FPA | PSO | BA |
Test1 | 4 | 23.3863 | 23.2573 | 23.2526 | 23.0420 | 23.0405 | 23.0397 | 23.0539 |
8 | 29.2231 | 29.1025 | 29.0968 | 28.9021 | 28.9007 | 28.8989 | 28.9020 | |
12 | 32.5692 | 32.4393 | 32.4442 | 32.2300 | 32.2265 | 32.2282 | 32.2414 | |
15 | 33.3295 | 33.1947 | 33.1953 | 33.0002 | 32.9903 | 32.9903 | 32.9923 | |
Test2 | 4 | 24.4702 | 24.3443 | 24.3386 | 24.1472 | 24.1340 | 24.1342 | 24.1432 |
8 | 29.4260 | 29.3020 | 29.2936 | 29.0852 | 29.0987 | 29.0861 | 29.1020 | |
12 | 32.5786 | 32.4454 | 32.4470 | 32.2471 | 32.2464 | 32.2522 | 32.2424 | |
15 | 34.2153 | 34.0865 | 34.0884 | 33.8828 | 33.8824 | 33.8823 | 33.8887 | |
Test3 | 4 | 24.4769 | 24.3474 | 24.3453 | 24.1433 | 24.1406 | 24.1517 | 24.1495 |
8 | 29.9667 | 29.8287 | 29.8366 | 29.6330 | 29.6383 | 29.6298 | 29.6211 | |
12 | 32.6661 | 32.5412 | 32.5392 | 32.3369 | 32.3265 | 32.3288 | 32.3254 | |
15 | 34.7330 | 34.6031 | 34.6015 | 34.3994 | 34.3925 | 34.4084 | 34.3905 | |
Test4 | 4 | 24.7640 | 24.6386 | 24.6369 | 24.4284 | 24.4400 | 24.4423 | 24.4286 |
8 | 29.5467 | 29.4208 | 29.4154 | 29.2214 | 29.2193 | 29.2207 | 29.2104 | |
12 | 33.5823 | 33.4474 | 33.4548 | 33.2561 | 33.2435 | 33.2461 | 33.2509 | |
15 | 34.2159 | 34.0826 | 34.0820 | 33.8787 | 33.8740 | 33.8707 | 33.8853 | |
Test5 | 4 | 24.5319 | 24.3991 | 24.3994 | 24.2048 | 24.1918 | 24.1918 | 24.1924 |
8 | 29.5550 | 29.4198 | 29.4251 | 29.2137 | 29.2181 | 29.2237 | 29.2154 | |
12 | 34.3322 | 34.1972 | 34.1979 | 34.0010 | 33.9935 | 33.9870 | 33.9959 | |
15 | 35.0669 | 34.9409 | 34.9366 | 34.7387 | 34.7254 | 34.7407 | 34.7279 | |
Test6 | 4 | 25.2119 | 25.0871 | 25.0842 | 24.8695 | 24.8803 | 24.8734 | 24.8829 |
8 | 30.9087 | 30.7880 | 30.7821 | 30.5869 | 30.5821 | 30.5735 | 30.5700 | |
12 | 34.1912 | 34.0606 | 34.0591 | 33.8576 | 33.8505 | 33.8585 | 33.8626 | |
15 | 35.7843 | 35.6567 | 35.6607 | 35.4492 | 35.4505 | 35.4438 | 35.4423 | |
Test7 | 4 | 25.4053 | 25.1458 | 25.1450 | 24.8847 | 25.0748 | 24.9284 | 24.9402 |
8 | 31.0914 | 30.8883 | 30.8601 | 30.7788 | 30.5828 | 30.6162 | 30.6718 | |
12 | 34.3507 | 34.1142 | 34.1742 | 34.0164 | 33.9942 | 33.8766 | 33.8983 | |
15 | 35.9362 | 35.6969 | 35.7545 | 35.6007 | 35.4588 | 35.4937 | 35.6024 | |
Test8 | 4 | 25.5670 | 25.2034 | 25.2799 | 24.9384 | 25.1283 | 24.9787 | 25.0445 |
8 | 31.2698 | 31.0054 | 30.9921 | 30.8672 | 30.7368 | 30.6972 | 30.8372 | |
12 | 34.5283 | 34.2658 | 34.2137 | 34.2017 | 34.1031 | 33.9285 | 34.0632 | |
15 | 36.0846 | 35.7585 | 35.9118 | 35.6393 | 35.6021 | 35.4988 | 35.6352 | |
Test9 | 4 | 25.5622 | 25.0597 | 25.1265 | 24.7749 | 24.9583 | 24.9368 | 24.8720 |
8 | 31.1656 | 30.8841 | 30.8166 | 30.7155 | 30.6732 | 30.6568 | 30.8154 | |
12 | 34.4249 | 34.0884 | 34.1830 | 34.1183 | 33.9539 | 33.7553 | 33.9067 | |
15 | 36.0382 | 35.7553 | 35.7737 | 35.5952 | 35.5146 | 35.3593 | 35.5413 | |
Test10 | 4 | 25.4424 | 24.9891 | 25.1108 | 24.7329 | 24.7752 | 24.9286 | 24.8300 |
8 | 31.1315 | 30.7681 | 30.7427 | 30.5414 | 30.6599 | 30.5129 | 30.6245 | |
12 | 34.3961 | 33.9364 | 34.1416 | 33.9835 | 33.8675 | 33.7435 | 33.8129 | |
15 | 35.8866 | 35.7225 | 35.7457 | 35.4554 | 35.4165 | 35.2905 | 35.3448 |
Image | K | HEOA | HSOA | HWOA | CSA | FPA | PSO | BA |
Test1 | 4 | 0.9367 | 0.9236 | 0.9225 | 0.9094 | 0.9039 | 0.8944 | 0.9087 |
8 | 0.9517 | 0.9414 | 0.9390 | 0.9036 | 0.9077 | 0.9157 | 0.9084 | |
12 | 0.9587 | 0.9433 | 0.9473 | 0.9135 | 0.9149 | 0.9172 | 0.9135 | |
15 | 0.9668 | 0.9511 | 0.9540 | 0.9271 | 0.9331 | 0.9287 | 0.9284 | |
Test2 | 4 | 0.9356 | 0.9227 | 0.9262 | 0.9006 | 0.8943 | 0.8936 | 0.9065 |
8 | 0.9584 | 0.9422 | 0.9438 | 0.9221 | 0.9123 | 0.9122 | 0.9230 | |
12 | 0.9632 | 0.9548 | 0.9563 | 0.9283 | 0.9305 | 0.9331 | 0.9233 | |
15 | 0.9633 | 0.9553 | 0.9506 | 0.9242 | 0.9251 | 0.9255 | 0.9202 | |
Test3 | 4 | 0.9325 | 0.9197 | 0.9181 | 0.9003 | 0.9077 | 0.8898 | 0.9078 |
8 | 0.9477 | 0.9333 | 0.9362 | 0.9060 | 0.9062 | 0.9001 | 0.9048 | |
12 | 0.9599 | 0.9482 | 0.9483 | 0.9289 | 0.9289 | 0.9190 | 0.9162 | |
15 | 0.9607 | 0.9487 | 0.9468 | 0.9309 | 0.9251 | 0.9325 | 0.9358 | |
Test4 | 4 | 0.9385 | 0.9213 | 0.9207 | 0.9065 | 0.9063 | 0.8982 | 0.8954 |
8 | 0.9485 | 0.9362 | 0.9349 | 0.9057 | 0.9058 | 0.9115 | 0.9144 | |
12 | 0.9580 | 0.9487 | 0.9498 | 0.9240 | 0.9298 | 0.9154 | 0.9200 | |
15 | 0.9637 | 0.9457 | 0.9532 | 0.9229 | 0.9227 | 0.9321 | 0.9237 | |
Test5 | 4 | 0.9332 | 0.9213 | 0.9264 | 0.8894 | 0.9071 | 0.9082 | 0.8960 |
8 | 0.9469 | 0.9377 | 0.9361 | 0.9085 | 0.9141 | 0.9083 | 0.9106 | |
12 | 0.9642 | 0.9438 | 0.9503 | 0.9210 | 0.9254 | 0.9187 | 0.9270 | |
15 | 0.9687 | 0.9484 | 0.9489 | 0.9240 | 0.9174 | 0.9240 | 0.9267 | |
Test6 | 4 | 0.9304 | 0.9238 | 0.9186 | 0.8868 | 0.9055 | 0.8972 | 0.9054 |
8 | 0.9518 | 0.9317 | 0.9372 | 0.9027 | 0.9099 | 0.9117 | 0.9085 | |
12 | 0.9579 | 0.9492 | 0.9460 | 0.9280 | 0.9270 | 0.9258 | 0.9158 | |
15 | 0.9682 | 0.9517 | 0.9490 | 0.9289 | 0.9204 | 0.9315 | 0.9250 | |
Test7 | 4 | 0.9313 | 0.9252 | 0.9198 | 0.8882 | 0.9057 | 0.8984 | 0.9060 |
8 | 0.9536 | 0.9322 | 0.9384 | 0.9035 | 0.9109 | 0.9127 | 0.9087 | |
12 | 0.9590 | 0.9498 | 0.9477 | 0.9287 | 0.9280 | 0.9273 | 0.9177 | |
15 | 0.9699 | 0.9524 | 0.9510 | 0.9292 | 0.9222 | 0.9335 | 0.9258 | |
Test8 | 4 | 0.9294 | 0.9239 | 0.9181 | 0.8877 | 0.9044 | 0.8970 | 0.9058 |
8 | 0.9518 | 0.9309 | 0.9381 | 0.9029 | 0.9096 | 0.9116 | 0.9070 | |
12 | 0.9573 | 0.9487 | 0.9470 | 0.9276 | 0.9263 | 0.9263 | 0.9175 | |
15 | 0.9682 | 0.9513 | 0.9494 | 0.9280 | 0.9216 | 0.9324 | 0.9240 | |
Test9 | 4 | 0.9292 | 0.9234 | 0.9161 | 0.8866 | 0.9038 | 0.8956 | 0.9052 |
8 | 0.9506 | 0.9296 | 0.9368 | 0.9010 | 0.9089 | 0.9096 | 0.9064 | |
12 | 0.9569 | 0.9469 | 0.9470 | 0.9273 | 0.9250 | 0.9262 | 0.9160 | |
15 | 0.9676 | 0.9501 | 0.9474 | 0.9275 | 0.9216 | 0.9308 | 0.9236 | |
Test10 | 4 | 0.9296 | 0.9246 | 0.9170 | 0.8874 | 0.9046 | 0.8962 | 0.9057 |
8 | 0.9509 | 0.9306 | 0.9378 | 0.9025 | 0.9093 | 0.9098 | 0.9069 | |
12 | 0.9589 | 0.9483 | 0.9490 | 0.9275 | 0.9261 | 0.9277 | 0.9165 | |
15 | 0.9678 | 0.9501 | 0.9479 | 0.9287 | 0.9232 | 0.9316 | 0.9240 |
Image | K | HEOA | HSOA | HWOA | CSA | FPA | PSO | BA |
Test1 | 4 | 1.2145 | 1.2251 | 1.2247 | 1.2442 | 1.2405 | 1.2406 | 1.2389 |
8 | 1.3154 | 1.3254 | 1.3260 | 1.3452 | 1.3422 | 1.3386 | 1.3385 | |
12 | 1.5172 | 1.5274 | 1.5279 | 1.5448 | 1.5467 | 1.5451 | 1.5466 | |
15 | 1.8157 | 1.8260 | 1.8258 | 1.8359 | 1.8435 | 1.8376 | 1.8439 | |
Test2 | 4 | 1.2149 | 1.2259 | 1.2255 | 1.2363 | 1.2392 | 1.2436 | 1.2365 |
8 | 1.3154 | 1.3262 | 1.3263 | 1.3388 | 1.3411 | 1.3401 | 1.3371 | |
12 | 1.5181 | 1.5286 | 1.5288 | 1.5423 | 1.5449 | 1.5466 | 1.5389 | |
15 | 1.8158 | 1.8266 | 1.8266 | 1.8419 | 1.8398 | 1.8409 | 1.8424 | |
Test3 | 4 | 1.2151 | 1.2257 | 1.2258 | 1.2354 | 1.2367 | 1.2445 | 1.2367 |
8 | 1.3158 | 1.3268 | 1.3265 | 1.3402 | 1.3411 | 1.3456 | 1.3436 | |
12 | 1.5191 | 1.5292 | 1.5292 | 1.5394 | 1.5406 | 1.5474 | 1.5487 | |
15 | 1.8164 | 1.8270 | 1.8273 | 1.8462 | 1.8369 | 1.8437 | 1.8413 | |
Test4 | 4 | 1.2151 | 1.2254 | 1.2253 | 1.2444 | 1.2358 | 1.2358 | 1.2409 |
8 | 1.3166 | 1.3268 | 1.3271 | 1.3432 | 1.3398 | 1.3458 | 1.3461 | |
12 | 1.5192 | 1.5299 | 1.5301 | 1.5421 | 1.5476 | 1.5483 | 1.5465 | |
15 | 1.8166 | 1.8275 | 1.8269 | 1.8390 | 1.8466 | 1.8422 | 1.8425 | |
Test5 | 4 | 1.2161 | 1.2269 | 1.2269 | 1.2388 | 1.2447 | 1.2368 | 1.2401 |
8 | 1.3167 | 1.3273 | 1.3273 | 1.3456 | 1.3396 | 1.3382 | 1.3431 | |
12 | 1.5201 | 1.5304 | 1.5302 | 1.5471 | 1.5428 | 1.5454 | 1.5401 | |
15 | 1.8169 | 1.8274 | 1.8278 | 1.8431 | 1.8379 | 1.8456 | 1.8405 | |
Test6 | 4 | 1.2168 | 1.2271 | 1.2268 | 1.2372 | 1.2413 | 1.2412 | 1.2383 |
8 | 1.3172 | 1.3281 | 1.3273 | 1.3412 | 1.3407 | 1.3448 | 1.3379 | |
12 | 1.5206 | 1.5307 | 1.5313 | 1.5491 | 1.5408 | 1.5445 | 1.5493 | |
15 | 1.8169 | 1.8278 | 1.8270 | 1.8395 | 1.8463 | 1.8410 | 1.8428 | |
Test7 | 4 | 1.2183 | 1.2275 | 1.2282 | 1.2373 | 1.2421 | 1.2429 | 1.2399 |
8 | 1.3179 | 1.3284 | 1.3291 | 1.3429 | 1.3420 | 1.3463 | 1.3391 | |
12 | 1.5219 | 1.5326 | 1.5316 | 1.5510 | 1.5410 | 1.5462 | 1.5506 | |
15 | 1.8179 | 1.8293 | 1.8289 | 1.8408 | 1.8466 | 1.8410 | 1.8440 | |
Test8 | 4 | 1.2173 | 1.2270 | 1.2272 | 1.2371 | 1.2403 | 1.2424 | 1.2393 |
8 | 1.3167 | 1.3278 | 1.3282 | 1.3409 | 1.3401 | 1.3447 | 1.3386 | |
12 | 1.5217 | 1.5308 | 1.5303 | 1.5497 | 1.5398 | 1.5460 | 1.5498 | |
15 | 1.8172 | 1.8273 | 1.8284 | 1.8396 | 1.8446 | 1.8397 | 1.8424 | |
Test9 | 4 | 1.2154 | 1.2265 | 1.2258 | 1.2357 | 1.2391 | 1.2409 | 1.2376 |
8 | 1.3166 | 1.3268 | 1.3277 | 1.3398 | 1.3389 | 1.3428 | 1.3375 | |
12 | 1.5204 | 1.5304 | 1.5291 | 1.5482 | 1.5397 | 1.5453 | 1.5485 | |
15 | 1.8154 | 1.8272 | 1.8281 | 1.8395 | 1.8431 | 1.8385 | 1.8422 | |
Test10 | 4 | 1.2155 | 1.2282 | 1.2270 | 1.2377 | 1.2404 | 1.2419 | 1.2380 |
8 | 1.3172 | 1.3283 | 1.3279 | 1.3401 | 1.3399 | 1.3444 | 1.3391 | |
12 | 1.5207 | 1.5319 | 1.5305 | 1.5494 | 1.5397 | 1.5460 | 1.5495 | |
15 | 1.8160 | 1.8272 | 1.8297 | 1.8400 | 1.8440 | 1.8393 | 1.8442 |
K | HEOA | HSOA | HWOA | CSA | FPA | PSO | BA |
4 | 3.1581 | 3.4458 | 3.5147 | 3.4581 | 3.6152 | 3.9951 | 3.8547 |
8 | 2.9315 | 3.5514 | 3.6891 | 3.5125 | 3.9156 | 3.9514 | 3.6984 |
12 | 1.9984 | 2.5589 | 3.6661 | 3.1285 | 3.9991 | 3.9541 | 3.8854 |
15 | 1.7415 | 2.6518 | 3.6581 | 3.1814 | 3.8147 | 3.8574 | 3.7781 |
Overall | 2.4574 | 3.0520 | 3.6320 | 3.3201 | 3.8362 | 3.9395 | 3.8042 |
Images | K | HSOA | HWOA | CSA | FPA | PSO |
Test1 | 4 | P < 0.05 | P < 0.05 | P < 0.05 | P < 0.05 | P < 0.05 |
8 | P > 0.05 | P < 0.05 | P < 0.05 | P < 0.05 | P < 0.05 | |
12 | P < 0.05 | P < 0.05 | P < 0.05 | P < 0.05 | P < 0.05 | |
15 | P < 0.05 | P > 0.05 | P < 0.05 | P < 0.05 | P < 0.05 | |
Test2 | 4 | P < 0.05 | P < 0.05 | P < 0.05 | P > 0.05 | P < 0.05 |
8 | P < 0.05 | P < 0.05 | P < 0.05 | P < 0.05 | P < 0.05 | |
12 | P < 0.05 | P < 0.05 | P < 0.05 | P < 0.05 | P < 0.05 | |
15 | P < 0.05 | P < 0.05 | P < 0.05 | P < 0.05 | P < 0.05 | |
Test3 | 4 | P < 0.05 | P < 0.05 | P < 0.05 | P < 0.05 | P < 0.05 |
8 | P < 0.05 | P < 0.05 | P < 0.05 | P < 0.05 | P < 0.05 | |
12 | P < 0.05 | P < 0.05 | P < 0.05 | P < 0.05 | P < 0.05 | |
15 | P < 0.05 | P < 0.05 | P < 0.05 | P < 0.05 | P < 0.05 | |
Test4 | 4 | P < 0.05 | P < 0.05 | P < 0.05 | P < 0.05 | P < 0.05 |
8 | P > 0.05 | P < 0.05 | P < 0.05 | P < 0.05 | P < 0.05 | |
12 | P < 0.05 | P > 0.05 | P < 0.05 | P < 0.05 | P < 0.05 | |
15 | P < 0.05 | P < 0.05 | P < 0.05 | P < 0.05 | P < 0.05 | |
Test5 | 4 | P < 0.05 | P < 0.05 | P < 0.05 | P < 0.05 | P < 0.05 |
8 | P < 0.05 | P < 0.05 | P < 0.05 | P < 0.05 | P < 0.05 | |
12 | P < 0.05 | P < 0.05 | P < 0.05 | P < 0.05 | P < 0.05 | |
15 | P < 0.05 | P < 0.05 | P < 0.05 | P < 0.05 | P < 0.05 | |
Test6 | 4 | P > 0.05 | P < 0.05 | P < 0.05 | P < 0.05 | P < 0.05 |
8 | P < 0.05 | P < 0.05 | P < 0.05 | P < 0.05 | P < 0.05 | |
12 | P < 0.05 | P < 0.05 | P < 0.05 | P < 0.05 | P < 0.05 | |
15 | P < 0.05 | P < 0.05 | P < 0.05 | P < 0.05 | P < 0.05 | |
Test7 | 4 | P < 0.05 | P < 0.05 | P > 0.05 | P < 0.05 | P < 0.05 |
8 | P < 0.05 | P < 0.05 | P < 0.05 | P < 0.05 | P < 0.05 | |
12 | P < 0.05 | P < 0.05 | P < 0.05 | P < 0.05 | P < 0.05 | |
15 | P < 0.05 | P < 0.05 | P < 0.05 | P < 0.05 | P < 0.05 | |
Test8 | 4 | P > 0.05 | P < 0.05 | P < 0.05 | P > 0.05 | P < 0.05 |
8 | P < 0.05 | P > 0.05 | P < 0.05 | P < 0.05 | P < 0.05 | |
12 | P < 0.05 | P < 0.05 | P < 0.05 | P < 0.05 | P < 0.05 | |
15 | P < 0.05 | P < 0.05 | P < 0.05 | P < 0.05 | P < 0.05 | |
Test9 | 4 | P < 0.05 | P < 0.05 | P < 0.05 | P < 0.05 | P < 0.05 |
8 | P < 0.05 | P > 0.05 | P < 0.05 | P < 0.05 | P < 0.05 | |
12 | P < 0.05 | P < 0.05 | P < 0.05 | P < 0.05 | P < 0.05 | |
15 | P < 0.05 | P < 0.05 | P < 0.05 | P < 0.05 | P < 0.05 | |
Test10 | 4 | P < 0.05 | P > 0.05 | P > 0.05 | P < 0.05 | P < 0.05 |
8 | P < 0.05 | P < 0.05 | P < 0.05 | P < 0.05 | P < 0.05 | |
12 | P < 0.05 | P < 0.05 | P < 0.05 | P < 0.05 | P < 0.05 | |
15 | P < 0.05 | P < 0.05 | P < 0.05 | P < 0.05 | P < 0.05 |
Method | R (%) | P (%) | AP (%) | VAL (%) |
FCM | 84.94 | 90.88 | 91.23 | 88.47 |
PCNN | 80.46 | 90.69 | 81.46 | 79.46 |
DLA | 70.12 | 92.95 | 78.32 | 77.57 |
BMPA | 93.19 | 91.28 | 91.45 | 90.38 |