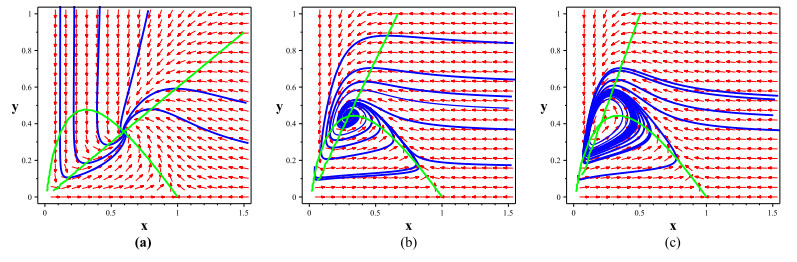
Citation: Yulia M. Panchenko, Andrey I. Marshakov, Ludmila A. Nikolaeva, Victoria V. Kovtanyuk. Prediction of first-year corrosion losses of copper and aluminum in continental regions[J]. AIMS Materials Science, 2018, 5(4): 624-649. doi: 10.3934/matersci.2018.4.624
[1] | Yuan Tian, Sanyi Tang . Dynamics of a density-dependent predator-prey biological system with nonlinear impulsive control. Mathematical Biosciences and Engineering, 2021, 18(6): 7318-7343. doi: 10.3934/mbe.2021362 |
[2] | Yuan Tian, Yan Gao, Kaibiao Sun . A fishery predator-prey model with anti-predator behavior and complex dynamics induced by weighted fishing strategies. Mathematical Biosciences and Engineering, 2023, 20(2): 1558-1579. doi: 10.3934/mbe.2023071 |
[3] | Qizhen Xiao, Binxiang Dai . Heteroclinic bifurcation for a general predator-prey model with Allee effect and state feedback impulsive control strategy. Mathematical Biosciences and Engineering, 2015, 12(5): 1065-1081. doi: 10.3934/mbe.2015.12.1065 |
[4] | Gianni Gilioli, Sara Pasquali, Fabrizio Ruggeri . Nonlinear functional response parameter estimation in a stochastic predator-prey model. Mathematical Biosciences and Engineering, 2012, 9(1): 75-96. doi: 10.3934/mbe.2012.9.75 |
[5] | Xiaoxiao Yan, Zhong Zhao, Yuanxian Hui, Jingen Yang . Dynamic analysis of a bacterial resistance model with impulsive state feedback control. Mathematical Biosciences and Engineering, 2023, 20(12): 20422-20436. doi: 10.3934/mbe.2023903 |
[6] | Yufei Wang, Huidong Cheng, Qingjian Li . Dynamic analysis of wild and sterile mosquito release model with Poincaré map. Mathematical Biosciences and Engineering, 2019, 16(6): 7688-7706. doi: 10.3934/mbe.2019385 |
[7] | Guirong Jiang, Qishao Lu, Linping Peng . Impulsive Ecological Control Of A Stage-Structured Pest Management System. Mathematical Biosciences and Engineering, 2005, 2(2): 329-344. doi: 10.3934/mbe.2005.2.329 |
[8] | Mengya Huang, Anji Yang, Sanling Yuan, Tonghua Zhang . Stochastic sensitivity analysis and feedback control of noise-induced transitions in a predator-prey model with anti-predator behavior. Mathematical Biosciences and Engineering, 2023, 20(2): 4219-4242. doi: 10.3934/mbe.2023197 |
[9] | Yi Yang, Lirong Liu, Changcheng Xiang, Wenjie Qin . Switching dynamics analysis of forest-pest model describing effects of external periodic disturbance. Mathematical Biosciences and Engineering, 2020, 17(4): 4328-4347. doi: 10.3934/mbe.2020239 |
[10] | Saheb Pal, Nikhil Pal, Sudip Samanta, Joydev Chattopadhyay . Fear effect in prey and hunting cooperation among predators in a Leslie-Gower model. Mathematical Biosciences and Engineering, 2019, 16(5): 5146-5179. doi: 10.3934/mbe.2019258 |
In the wide world, the threat of pests is growing to agriculture. How to control the pest quantity effectively in agricultural development has become one of the important problems. In the past decades, many researchers pay more attention to the methods of controlling pest populations, and there are some good results [1,2,3,4,5,6,7,8].
There are three main methods of controlling pests. One is the use of chemical control methods, namely spraying insecticide. Its disadvantage is that it pollutes the environment or products and also causes the ecological imbalance because of the massive death of predator evenly. The second is the use of biological control which can make full use of the role of natural control in the agricultural ecosystem, that is, to reduce the population density of pests effectively by artificially raising natural enemies and releasing them periodically. However this method is only applicable to the smaller density of pest populations and once the pest populations exceeds a certain number, the method will be invalid. The third method is the integrated control method of chemical control and biological control, which is the most widely used method in the agricultural and ecological resource management. If the density of pest populations is lower than the economic threshold, then it is not necessary to adopt any control strategy, but once the pest populations density reaches or exceeds the economic threshold, we should adopt a certain control strategy to avoid the imbalance of ecology. In this paper, we apply the state dependent impulsive differential equation to describe the implementation of the feedback control strategy.
In recent years, semi-continuous dynamical system theory has been applied in many fields such as mathematics, biological mathematics, ecology and economics [9,10,11,12,13,14,15,16,17]. In [18,19], the integrated control system with state pulse feedback control was considered, some sufficient conditions of the existence and uniqueness of the periodic solution were obtained by the successor functions method, and the stability of the periodic solution was proved. In [20], the related properties of the Leslie-Gower model without impulse were studied. In [21,22], the dynamic properties of periodic solutions of the predator-prey model were investigated. In [23], pei et al. applied the time pulse method to harvest the prey at fixed time. Jiao et al. [24] mainly considered the periodic pulsed predator-prey model during hibernation and obtained the global asymptotic stability criterion for the extinction boundary of the predator.
Although there are fruitful results on mathematical models with state dependence on the pulse feedback control, but most of them are just based on the study of a single pulse control and few of them considered the feedback control strategy for two different influence of preys and predators at the same time. These researches were rare on that how to control pesticide spraying to get greater economic benefits [25,26,27]. Therefore, we construct a Leslie-Gower model with ratio-dependence and state pulse feedback control in this paper.
The structure of this paper is as follows: In Section 2, we build a Leslie-Gower predator-prey model with ratio-dependence and state pulse control. This is a generalisation of the original Leslie model with ratio-dependence by adding the state pulse feedback control. In Section 3, the existence, uniqueness and the asymptotic stability of the periodic solution are proved according to the relevant characteristics of the positive equilibrium point E∗. In Section 4, we formulate an optimal problem and obtain the economic threshold. In addition, some numerical simulation examples are carried out to verify our theoretical results. We give a short conclusion in the Section 5.
The Leslie model is one of the most classic prey-predator model, and it has attracted many scholars to modify and improve it in many aspects [28,29,30,31]. Especially, due to the laboratory experiments [32,33,34,35,36] and observation [37,38,39,40,41,42,43], a few of researchers focus on the ratio-dependence of the Leslie prey-predator model recently. Gupta et al constructed Leslie-Gower predator-prey model with Michaelis-Menten type prey-harvesting and investigated bifurcation of model [44]. In [45], Zhao et al established a Leslie-Gower predator-prey system with fixed time impulsive and discussed the persistence of system. However, state dependent pulse differential system is more in line with the actual biological system. Wei et al proposed a Leslie-Gower pest management model with impulsive state feedback control and discussed the conditions for existence and stability of periodic solution [46]. Flores and E. González-Olivares proposed a modified Leslie-Gower predator-prey model with ratio-dependent functional response and alternative food for the predator [47].
In [48], Liang et al established the following Leslie-Gower model with ratio-dependence:
{x′1(t1)=rx1(t1)(1−x1(t1)K)−kx1(t1)y1(t1)a1y1(t1)+x1(t1),y′1(t1)=y1(t1)a2(1−cy1(t1)x1(t1)). | (2.1) |
In System (2.1), x1(t1), y1(t1) represent the densities of preys and predators at time t1, respectively. a2 is the intrinsic rate of growth of the predator. Environmental carrying capacity is proportional to the maximum amount of food that the environment can hold, that means, K(x)=x/b. The predator's consumption of the prey depends on the ratio kx1a1y1+x1, where k is the maximum amount of the prey. The parameter c represents the maximum value of the average deficit reduction of y1 to x1, cy1x1 is named the Leslie-Gower pattern. In addition, the parameters r, a1, a2, k, c, K are all positive constants.
According to the Introduction, ET1 is denoted the pest slight harmful threshold. When the number of pest populations achieves ET1, we only need to adopt biological control strategies to reduce effectively the number of pests. Analogously, ET2 is denoted the pest economic injury threshold. When the number of pest populations achieves ET2, only by adopting chemical control strategy can the pest quantity be reduced effectively in a short time. However, in most cases, the control strategy we adopt is the integrated control strategy. In this paper, we apply the integrated control strategy to achieve effective control of pests when the number of pests satisfies x1∈[ET1, ET2]. To achieve this objective, we establish a model as follows:
{x′1(t1)=rx1(t1)−r(x1(t1))2K−kx1(t1)y1(t1)a1y1(t1)+x1(t1),y′1(t1)=a2y1(t1)−ca1y1(t1)x1(t1)y1(t1),} x1(t1)<ETΔx1(t1)=−p(x1)x1(t1),Δy1(t1)=−q(x1)y1(t1)+τ(x1),} x1(t1)=ET, | (2.2) |
where ET∈[ET1, ET2]. Consider the dynamic behavior of System(2.2), the proportionality coefficient of the amount of the killed pests and predators are defined as p(x1)(p(x1)∈(0,1)) and q(x1)(0<q(x1)<p(x1)), and τ(x1) is defined as the number of artificial natural enemies. To simplify, we nondimensionalize System (2.2) by using rt1=t, x1(t1)K=x(t), and ky1(t1)r=y(t). Then we get the following form:
{x′(t)=x(t)−x2(t)−x(t)y(t)α1y(t)+x(t),y′(t)=α2βy(t)−βy2(t)x(t),} x(t)<ξ,Δx(t)=−p(x)x(t),Δy(t)=−q(x)y(t)+τ(x),} x(t)=ξ, | (2.3) |
where α1=a1rkK, α2=a2kKrca1, β=a1ckK, and ξ=ETK.
{p(x)=pmaxx−ξminξmax−ξmin,τ(x)=τmax−(τmax−τmin)x−ξminξmax−ξmin,q(x)=qmaxx−ξminξmax−ξmin. | (2.4) |
The control parameters p(x), q(x), τ(x) are continuous functions defined on [ξmin,ξmax], ξmin and ξmax are the minimum value and maximum value respectively, which satisfy 0<ξmax≤ξ≤ξmax<K1−pmin. In addition, p(ξmax)=pmax, τ(ξmax)=τmin, τ(ξmin)=τmax and q(ξmax)=qmax. Denote pξ=p(ξ), τξ=τ(ξ) and qξ=q(ξ), we may see [49] for more details.
In the absence of impulses, the predator-prey model is as follows:
{x′(t)=x(t)−x2(t)−x(t)y(t)α1y(t)+x(t),y′(t)=α2βy(t)−βy2(t)x(t). | (2.5) |
The isoclinic line L1:1−x−1α1y+xy=0 has the following features: when the value range of x is (0,1), first, if the condition α1=1 holds, then the line L1 signifies a straight line; second, if the condition α1<1 holds, then the line L1 signifies an opening downward curve line; third, if the condition α1>1 holds, then the line L1 signifies an opening upward curve line. According to the practical significance, we only discuss the condition α1<1 in our research. It can be known from [48] that if α1α2+1>α2 holds, then System (2.5) exists a positive equilibrium E∗(x∗,y∗), where x∗=1−α2α1α2+1, y∗=α2x∗. The equilibrium E0(1,0) is a saddle point that the stable manifold is located on the positive x axis.
Lemma 1 [48] If condition α1>1 holds, then system (5) is permanent.
Lemma 2 [48] The equilibrium E0(1,0) of system (5) is a saddle point with the positive x-axis as its stable manifold.
Lemma 3 [48] If conditions α1⋅α2+1>α2 and (α1α2+2)α2<(βα2+1)(α1⋅α2+1)2 hold, then the positive equilibrium E∗(x∗,y∗) of the system(2.5) is locally asymptotically stable node or focus. If conditions α1α2+1>α2 and (α1α2+2)α2>(βα2+1)(α1⋅α2+1)2 hold, then the positive equilibrium E∗(x∗,y∗) of the system (2.5) is an unstable node or focus. In addition, the system (2.5) has an unique limit cycle. The vector field of System (2.5) is shown in Figure 1.
By [50,Definition 2.1], System (2.3) is a semi-continuous power system. For System (2.3), the impulse set ∑M={(x,y)∈R2+|x=ξ,y≥0}, the pulse mapping φ:(x,y)∈∑M→((1−p(ξ))x,(1−q(ξ))y+τ)∈R2+, and the image set ∑N=φ(∑M)={(x,y)∈R2+|x=(1−pξ)ξ,y≥0}.
For any point T, xT and yT are denoted its horizontal coordinate and ordinate coordinate, respectively. If T∈∑M, then the impulse start on the point T, and the impulse function will run from T to ∑N. Considering the ecological practice, we apply System (2.3) to the space R2+.
In two aspects, we prove the existence, uniqueness and asymptotic stability of periodic solution: ξ≤min{x∗,ξmax} and max{x∗,ξmin}<ξ<1.
Theorem 3.1. If conditions α1⋅α2+1>α2, ξ≤min{x∗,ξmax} and (α1α2+2)α2<(βα2+1)(α1α2+1)2 hold, then System (2.3) possesses order-1 periodic solution.
Proof. According to Theorem 1, we can learn the positive equilibrium E∗(x∗,y∗) is stable.
The isoclinic line L1:1−x−yα1y+x=0 intersects with the image set ∑N at the point A. The trajectory with the initial point A intersects with the impulse set ∑M at point A1, then runs to the point A+1∈∑N with impulse effects. According to the location of point A+1, there may be three possible scenarios : (1) yA+1=yA, (2) yA+1>yA, (3) yA+1<yA.
Now, we prove each of these cases separately.
Case Ⅰ: yA+1=yA.
In this case, A+1 coincides with A, and the successor function of A is I(A)=yA+1−yA=0. According to [51,Definition 2.2], then we shall get that System (2.3) possesses order-1 periodic solution in case of yA+1=yA (see Figure 2(a)).
Case Ⅱ: yA+1>yA.
In this case, A+1 is above A, then the successor function of point A is I(A)=yA+1−yA>0. The trajectory, which has an initial point A+1, intersects with impulse set ∑M at the point A2, then jumps to the point A+2 with impulse effects. In view of vector field and disjointness of any two trajectories, we shall get that yA2<yA1, so we have yA+2<yA+1. Therefore, the successor function [51,Definition 2.1]of point A+1 is I(A+1)=yA+2−yA+1<0 (see Figure 2(b)).
Therefore, there must exist a point T which makes I(T)=0. It follows from [51,Lemma 2.3], between the point A and the point A+1, System (2.3) must have an order-1 periodic solution.
Case Ⅲ: yA+1<yA.
In this case, A+1 is below A, then the successor function of point A is I(A)=yA+1−yA<0. We select another point D which is the intersection point of phase ∑N and x axis. The trajectory, which has an initial point D, intersects with the impulse set ∑M at the point D1, then it runs to the point D+1 after impulse effects. We shall get yD+1=(1−q)yD1>0=yD, hence the successor function I(D)=yD+1−yD>0 is obtained (see Figure 2(c)).
Therefore, there must exist a point T, which makes I(T)=0. Considering [51,Lemma 2.3], System (2.3) has an order-1 periodic solution between the point D and the point A. This proof is completed.
Theorem 3.2. In case of ξ≤min{x∗,ξmax}, α1⋅α2+1>α2, (α1α2+2)α2<(βα2+1)(α1α2+1)2 and yA+1>yA, the order-1 periodic solution of System (2.3) is unique.
Proof. We arbitrarily select two points P and Q in the ¯AA+1, where yA<yP<yQ<yA+1. We denote function G(M)=M1 as the trajectory starting from the point M∈∑N and reaching the point M1∈∑M in the impulse set ∑M. Setting G(P)=P1∈∑M,G(Q)=Q1∈∑M, thus we have yQ1<yP1, and P1,Q1 are mapped to P+1,Q+1∈∑N after impulse effects, respectively, then the successor functions of P,Q satisfy:
I(P)−I(Q)=(yP+1−yP)−(yQ+1−yQ)=(yQ−yP)+(yP+1−yQ+1)=(yQ−yP)+(1−q)yP1+τ−(1−q)yQ1−τ=(yQ−yP)+(1−q)(yP1−yQ1)>0, |
The successor function is monotonically increasing in the ¯AA+1, thus there is only one point T∈(A,A1) which makes I(T)=0, i.e. System (2.3) has a unique order-1 periodic solution. This proof is completed.
Theorem 3.3. If conditions α1⋅α2+1>α2, (α1α2+2)α2<(βα2+1)(α1α2+1)2 and (1−q)∣Θ(f0,g0)∣<∣Θ(f1,g1)∣ hold, then the order-1 periodic solution of System (2.3) is orbitally asymptotically stable, where Θ(x,y)=1y(1−x−yα1y+x).
Proof. We denote that x=f(t),y=g(t) is a T-periodic solution of System (2.3) and f1=f(T)=ξ, g1=g(T); f0=f(0), g0=g(0); f+1=f(T+),g+1=g(T+), then we have
f+1=f0=(1−p)ξ,g+1=g0=(1−q)g1+τ. |
Let P(x,y)=x−x2−xyα1y+x, Q(x,y)=α2βy−βy2x, Φ(x,y)=−pξx, Ψ(x,y)=−qξy+τξ, Υ(x,y)=x−ξ.
Then ∂Φ∂x=−p, ∂Ψ∂x=0, ∂Φ∂y=0, ∂Ψ∂y=−q, ∂Υ∂x=1, ∂Υ∂y=0,
Δ1=P+(∂Ψ∂y∂Υ∂x−∂Ψ∂x∂Υ∂y+∂Υ∂x)P∂Υ∂x+Q∂Υ∂y+Q+(∂Φ∂x∂Υ∂y−∂Φ∂y∂Υ∂x+∂Υ∂y)P∂Υ∂x+Q∂Υ∂y=P(f+1,g+1)(−q×1−0×0+1)P(f1,g1)×1+Q(f1,g1)×0+Q(f+1,g+1)(−p×0−0×1+0)P(f1,g1)×1+Q(f1,g1)×0=(1−q)[f0−f20−f0g0α1g0+f0]f1−f21−f1g1α1g1+f1 |
and
∫T0(∂P∂x+∂Q∂y)dt=∫T0[1−x−yα1y+x+α2β−βyx]dt+∫T0[−x+xy(α1y+x)2−βyx]dt=∫T0[˙x(t)x(t)+˙y(t)y(t)]dt+∫T0[−x+xy(α1y+x)2−βyx]dt=lnx(T)y(T)x(0)y(0)+∫T0[−x+xy(α1y+x)2−βyx]dt=lnf1g1f0g0+∫T0[−x+xy(α1y+x)2−βyx]dt |
Furthermore,
μ2=Δ1exp∫T0[∂P∂x(f(t),g(t))+∂Q∂y(f(t),g(t))]dt,=(1−q)f0(1−f0−g0α1g0+f0)f1(1−f1−g1α1g1+f1)⋅exp[lnf1g1f0g0+∫T0[−x+xy(α1y+x)2−βyx]dt]=(1−q)g1(1−f0−g0α1g0+f0)g0(1−f1−g1α1g1+f1)⋅exp(∫T0[−x+xy(α1y+x)2−βyx]dt). |
According to biological significance, for any point (x,y) which satisfies 0<x<ξ<1, y<ξ(1−ξ)1−α1+α1ξ, then
−x+xy(α1y+x)2−βyx<0 |
and due to (1−q)∣Θ(f0,g0)∣<∣Θ(f1,g1)∣, thus we can get
|(1−q)g1(1−f0−g0α1g0+f0)g0(1−f1−g1α1g1+f1)|<1, |
Therefore the convergency ratio |μ2| is less than one. Following the analogue of the Poincaré criterion [52,Theorem 2.3], we know that the order-1 periodic solution of System (2.3) is orbitally asymptotically stable. According to the above analysis, the conclusion is obtained.
Learning from the fourth equations of System (2.3): Δy=−qy+τ, there exists three cases in Figure 3: (1)τ>γ, (2)τ=γ, (3)τ<γ, where γ=yA−(1−q)yA1 on the bases of value τ. Therefore, we can get following conclusions:
Theorem 3.4. In case of max{x∗,ξmin}<ξ<1, α1⋅α2+1>α2 and (α1α2+2)α2<(βα2+1)(α1α2+1)2, the System (2.3) has order-1 periodic solution.
Proof. We choose τ<γ as an example to prove(see Figure 3(b)).
In this case, yA+1=(1−q)yA1+τ<(1−q)yA1+yA−(1−q)yA1=yA. We denote the isoclinic line L1:1−x−yα1y+x=0 hitting the image set ∑N at point A. The trajectory starting from the point A intersects the impulse set ∑M at the point A1, then runs to the point A+1 with impulse effects. The point A+1 is the successor point of the point A, and A+1 lies under A, that is yA+1<yA, it is easy to verify that the successor function I(A)=yA+1−yA<0. Denote the intersection point of the image set ∑N and x axis as D. The trajectory, which starts from the initial point D, reaches the point D1 in the impulse set ∑M, then runs to the point D+1. The point D+1 is the successor point of the point D, and the point D+1 is above the point D, that means yD+1>yD, obviously, the successor function I(D)=yD+1−yD>0.
Therefore, there must exist a point T, which makes I(T)=0. Considering [51,Lemma 2.3], the System (2.3) has an order-1 periodic solution between the point D and the point A. This proof is done.
Now, let us consider the following case: if α1α2+1>α2 and (α1α2+2)>(βα2+1)(α1α2+1)2 hold, the positive equilibrium E∗ of System (2.5) is an unstable node or focus, then there must exist an unique limit cycle [51,Lemma 2.2] of System (2.5). In the following sections, we will mainly discuss the case that the positive equilibrium E∗=(x∗,y∗) is an unstable focus.
We denote the limit cycle as L0, then the limit cycle hits the isoclinic line L1:1−x−yα1y+x=0 at the point B0(ξ1,yB0) and the point B(ξ2,yB), where ξ1<ξ2. Obviously, ξ1<x∗<ξ2. We make two straight lines : l1:x=ξ1, l2:x=ξ2, and the limit cycle is sandwiched between l1 and l2.
Case Ⅰ: min{1,ξmax}>ξ>max{ξ2,ξmin} and (1−p)ξ>ξ2.
When ξ>max{ξ2,ξmin} holds, then the limit cycle lies on the left of the impulse set ∑M, we denote function G(M)=M− as the trajectory starting from the point M∈∑N and hitting the impulse set ∑M at the point M−. Set G(C)=C−, where C− is the intersection of impulse set ∑M and isoclinic line L1. Denote C+ as the successor point of point C, there also exists three possible scenarios : yC+>yC, yC+=yC and yC+<yC (see Figure 4).
When yC+>yC, as is shown in Figure 4(a), then the motion trajectory of System (2.3) will go on for approaching the limit cycle by the several time pulse; when yC+=yC or yC+<yC hold (see Figure 4(b) and Figure 4(c), respectively), then System (2.3) has an order-1 periodic solution. Similar to the proof of Case Ⅰ and Case Ⅲ in Theorem 2, we can prove the existence of the order-1 periodic solution.
Therefore, we have the following conclusions:
Theorem 3.5. When α1α2+1>α2, (α1α2+2)α2>(βα2+1)(α1α2+1)2, ξ>max{ξ2,ξmin} and (1−p)ξ>ξ2 hold, if yC+ satisfies yC+>yC, then the trajectory of System (2.3) will approach to the limit cycle L0 in the end.
Case Ⅱ: ξ>max{ξ2,ξmin} and ξ1<(1−p)ξ<ξ2.
Theorem 3.6. When α1α2+1>α2, (α1α2+2)α2>(βα2+1)(α1α2+1)2, ξ>max{ξ2,ξmin} and ξ1<(1−p)ξ<ξ2 hold, then the trajectory of System (2.3) will approach to the limit cycle L0 in the end.
Proof. The trajectory starting from the point D either intersects with the impulse set ∑M or disjoins with the impulse set ∑M, therefore, we discuss the two cases separately in the following.
If the trajectory disjoins with the impulse set ∑M, the trajectory must approach to the limit cycle L0 ultimately (See Figure 5(b)).
Now, we will prove the case that the trajectory intersects with the impulse set ∑M. We define that P point is the intersection of the image set ∑N and the limit cycle L0. Take a point P−1∈∑M which causes P−1 runs to P after impulse effects, set G(P+1)=P−1∈∑M which makes P−2 runs to P+1 after the impulse effects, then yP>yP+1, yP−1>yP−2. This process is continuing until there exists a P+m+k∈∑N satisfying yP+m+k<τ. As a resault, we obtain a sequence {P+i}i=1,2,⋯,m+k of set ∑N satisfying G(P+m+k)=P−m+k and yP+m+k<yP+m+k−1. Due to the coincidence of P+m+k and D, we can get G(D)=P−m+k and yD<yP+m+k−1. In the following part, we will prove the trajectory of System (2.3) initiating any point of set will go on for approaching the limit cycle L0 ultimately.
From the vector field of System (2.5), we know the trajectory of System (2.5) initiating any point above P point of the image set ∑N will be free from impulse effect and approach the limit cycle L0 ultimately.
For any point is below P point, it must lie between P+m+k and P+i−1, where i=1,2,⋯,m+k and P+m+k=D. After i times' impulse effects, the trajectory initiating this point will arrive at some points of the image set ∑N and must be above P, and then approach the limit cycle L0 ultimately (See Figure 5(a)).
According above analysis, we know that the trajectory will tend to the limit cycle L0 ultimately. The proof has been done.
To prove the feasibility of this conclusion, some simple examples are given in this section.
Let α1=0.6, α2=0.8, β=1.2 and we shall get the internal equilibrium points of System (2.3) is E∗=(0.53,0.43), where E∗ is a stable node. Let p=0.65, q=0.15, τ=0.2 and we shall get a unique order-1 periodic solution and it is asymptotically stable (see Figure 6). What's more, we can get the period of order-1 periodic solution is T=5.081.
Let α1=0.75, α2=0.15, β=0.05, then we can get the positive equilibrium point is E∗=(0.294,0.441), and it is a stable focus. When ξ=0.32,p=0.4,q=0.2,τ=0.1, and the system (2.3) has an order-1 periodic solution and the solution is unique and asymptotically stable. What's more, we can get the period of order-1 periodic solution is T=3.9625 (see Figure 7).
Let α1=0.75, α2=2, and β=0.05, then we shall get the interval equilibrium point E∗=(0.2,0.4) is a unstable focus, and there exists an unique limit cycle.
(ⅰ) Let ξ=0.2,p=0.4,q=0.2,τ=0.1 and the initial value is (0.15,0.3), the system (2.3) possesses order-1 periodic solution (see Figure 8).
(ⅱ) Let ξ=0.6,p=0.3,q=0.2,τ=0.15 and the initial value is (0.1,0.18), then the trajectory Γ0 can not hit the impulse set ΣM, therefore system (2.3) does not possess order-1 periodic solution and the trajectory Γ0 tend to the limit cycle (see Figure 9).
The practical significance of the research of order-1 periodic solution is that it provides a possibility to determine the adding rate of beneficial insects and insect pest eradication rate, this makes the pulse control no longer the real-time monitor of agricultural production, but a cyclical. To maintain the balance of ecological agriculture, to further determine the optimal replenishment of beneficial insects rate and the rate of eliminating most pests, and to ensure the longest administer interval period, the lowest cost, we study the following optimization problem to find the optimal threshold ξ.
We define s1 as the unit cost of supplementary predator including the cost of processing agricultural environment, and denote s2 as the unit cost of insecticide spraying. Our goal is to minimize costs this process. Vcost is defined as the total cost in under the control of threshold ξ one period of model (2.3), and it is a function about the rate τ of predator replenishment and the rate p of killed prey, that means Vcost(ξ)=s1τ(ξ)+s2p(ξ). Therefore, we formulated the optimization model as
minVcost(ξ)T(ξ),
s.t. ξmin<ξ<ξmax.
We solve the optimization problem to obtain the optimal threshold ξo, and we get the optimal replenishment rate of prey τo=τ(ξo), , the optimal chemical control strength po=p(ξo) and the optimal impulse period To=T(τo,po).
Let α1=0.75, α2=1.5, β=0.05, ξmin=0.1, ξmax=0.5, τmin=0.1, τmax=1, pmax=0.75, qmax=0.3, taking these parameters into system (2.3) and system (2.4), we shall get the following equations
{x′(t)=x(t)−x2(t)−x(t)y(t)0.75y(t)+x(t),y′(t)=0.075y(t)−0.05y2(t)x(t). | (4.1) |
{p(x)=0.9375(x−0.1),τ(x)=0.8−x,q(x)=0.25(x−0.1). | (4.2) |
Bringing the values of parameter ξ into system (4.2) can calculate the values of parameters p, q, τ. The period T can be obtained by numerical simulation. Therefore, the relationship between impulse period T and the threshold ξ is obtained in Figure 10(a) (the relevant dates in figure are shown in Table 1). Set s1=2000, s2=2000, by calculation we can get the value of Vcost/T. The relationship between the cost per unit time Vcost/T and the threshold ξ is shown in Figure 10(b) (the relevant dates in figure are shown in Table 1). As is shown in Figure 10, we can obtain the optimal threshold ξo=0.33, the optimum yield of releases of the predator is τo=0.483, the optimal killing rate of predator is po=0.431, and the optimal impulse period is To=19.967. Therefore, the control measures are adopted when the density of pests ξ reaches 0.33. Furthermore, it should be noted that the optimum pest control level ξo is dependent on the ratio of ω=s2s1, as illustrated in Figure 11 (the relevant dates in figure are shown in Table 2).
ξ | p | q | τ | T | VcostT |
0.15 | 0.094 | 0.038 | 0.886 | 18.723 | 105.0 |
0.20 | 0.186 | 0.075 | 0.775 | 19.345 | 99.0 |
0.25 | 0.281 | 0.113 | 0.663 | 19.735 | 96.0 |
0.33 | 0.431 | 0.173 | 0.483 | 19.967 | 91.4 |
0.35 | 0.469 | 0.188 | 0.438 | 19.867 | 91.7 |
0.40 | 0.563 | 0.225 | 0.325 | 19.500 | 92.0 |
0.45 | 0.656 | 0.263 | 0.213 | 18.720 | 92.8 |
ξ | VcostT(s2s1=12) | VcostT(s2s1=1) | VcostT(s2s1=2) | VcostT(s2s1=5) |
0.15 | 99.7 | 105.0 | 57.0 | 72.4 |
0.2 | 89.7 | 99.0 | 59.3 | 88.1 |
0.25 | 81.4 | 96.0 | 62.1 | 104.8 |
0.33 | 70.0 | 91.4 | 67.4 | 132.1 |
0.35 | 67.7 | 91.7 | 69.3 | 140.0 |
0.4 | 62.2 | 92.0 | 74.0 | 161.0 |
0.45 | 57.8 | 92.8 | 81.5 | 187.0 |
This paper puts forward a kind of ratio dependence and double pulse control feeding system, which is more practical than continuous single pulse control strategy. At the same time, we prove the existence, uniqueness and stability of the periodic solution by using the theory of successor functions and the analogue of the Poincaré criterion. The numerical simulations are given to verify the feasibility of the pulse strategy. In addition, in order to maximize the economic benefits, the optimal threshold is obtained and the optimization strategy is provided to eliminate pests.
Compared with the literature [48], this paper adds impulse feedback control to the model, so this paper is a further study of [48]. Literature [46] and [51] proposed Leslie-Gower prey-predator models with different impulse feedback controls and discussed the existence, uniqueness and stability of order-1 periodic solution. Based on this, this paper further studies the optimal control strategy of pest management.
In real life, the optimization of plague control is also a hot topic for some scholars [53]. We will further improve the optimization control strategy to make our research work more realistic and meaningful.
The paper was supported by the National Natural Science Foundation of China (No.11371230), Shandong Provincial Natural Science Foundation, China (No. S2015SF002), SDUST Innovation Fund for Graduate Students(SDKDYC190351), SDUST Research Fund (2014TDJH102), and Joint Innovative Center for Safe and Effective Mining Technology and Equipment of Coal Resources, Shandong Province of China.
The authors declare that there is no conflict of interests regarding the publication of this paper.
[1] | Knotkova D, Kreislova K, Dean SW (2010) ISOCORRAG International Atmospheric Exposure Program: Summary of Results, ASTM Series 71, West Conshohocken, PA: ASTM International. |
[2] | Tidblad J, Kucera V, Mikhailov AA (1998) Statistical analysis of 8 year materials exposure and acceptable deterioration and pollution levels, In: UN/ECE ICP on Effects on Materials, Report No. 30, Stockholm, Sweden: Swedish Corrosion Institute, 1–49. |
[3] |
Tidblad J, Kucera V, Mikhailov AA, et al. (2001) UN ECE ICP Materials: Dose-Response Functions on Dry and Wet Acid Deposition Effects After 8 Years of Exposure. Water Air Soil Poll 130: 1457–1462. doi: 10.1023/A:1013965030909
![]() |
[4] | Morcillo M, Almeida EM, Rosales BM, et al. (1998) Functiones de Dano (Dosis/Respuesta) de la Corrosion Atmospherica en Iberoamerica, In: Corrosion y Proteccion de Metales en las Atmosferas de Iberoamerica, Madrid, Spain: Programma CYTED, 629–660. |
[5] | Morcillo M (1995) Atmospheric corrosion in Ibero-America: The MICAT project, In: Kirk WW, Lawson HH, Atmospheric corrosion, ASTM STP 1239, Philadelphia, PA: American Society for Testing and Materials, 257–275. |
[6] | Panchenko YM, Shuvakhina LN, Mikhailovsky YN (1982) Atmospheric corrosion of metals in Far Eastern regions. Zashchita metallov 18: 575–582 (in Russian). |
[7] | ISO 9223:2012(E) (2012) Corrosion of metals and alloys—Corrosivity of atmospheres—Classification, determination and estimation. International Standards Organization, Geneva. |
[8] | Syed S (2006) Atmospheric corrosion of materials. Emir J Eng Res 11: 1–24. |
[9] |
De la Fuente D, Castano JG, Morcillo M (2007) Long-term atmospheric corrosion of zinc. Corros Sci 49: 1420–1436. doi: 10.1016/j.corsci.2006.08.003
![]() |
[10] |
Landolfo R, Cascini L, Portioli F (2010) Modeling of metal structure corrosion damage: A state of the art report. Sustainability 2: 2163–2175. doi: 10.3390/su2072163
![]() |
[11] |
Morcillo M, De la Fuente D, Diaz I, et al. (2011) Atmospheric corrosion of mild steel. Rev Metal 47: 426–444. doi: 10.3989/revmetalm.1125
![]() |
[12] |
De la Fuente D, Diaz I, Simancas J, et al. (2011) Long-term atmospheric corrosion of mild steel. Corros Sci 53: 604–617. doi: 10.1016/j.corsci.2010.10.007
![]() |
[13] |
Morcillo M, Chico B, Diaz I, et al. (2013) Atmospheric corrosion data of weathering steels: A review. Corros Sci 77: 6–24. doi: 10.1016/j.corsci.2013.08.021
![]() |
[14] |
Surnam BYR, Chiu CW, Xiao HP, et al. (2015) Long-term atmospheric corrosion in Mauritius. Corros Eng Sci Techn 50: 155–159. doi: 10.1179/1743278214Y.0000000240
![]() |
[15] |
Panchenko YM, Marshakov AI, Igonin TN, et al. (2014) Long-term forecast of corrosion mass losses of technically important metals in various world regions using a power function. Corros Sci 88: 306–316. doi: 10.1016/j.corsci.2014.07.049
![]() |
[16] |
Panchenko YM, Marshakov AI (2016) Long-term prediction of metal corrosion losses in atmosphere using a power-linear function. Corros Sci 109: 217–229. doi: 10.1016/j.corsci.2016.04.002
![]() |
[17] | ISO 9224:2012(E) (2012) Corrosion of metals and alloys—Corrosivity of atmospheres—Guiding values for the corrosivity categories. International Standards Organization, Geneva. |
[18] | Tidblad J, Mikhailov AA, Kucera V (1999) Unified Dose-Response Functions after 8 Years of Exposure. Quantification of Effects of Air Pollutants on Materials,UN ECE Workshop Proceedings, Umweltbundesamt, Berlin, 77–86. |
[19] | Tidblad J, Mikhailov AA, Kucera V (2000) Acid deposition effects on materials in subtropical and tropical climates. Data compilation and temperate climate comparison, KI Report, Stockholm, Sweden: Swedish Corrosion Institute, 1–33. |
[20] |
Chico B, De la Fuente D, Díaz I, et al. (2017) Annual atmospheric corrosion of carbon steel worldwide. An integration of ISOCORRAG, ICP/UNECE and MICAT databases. Materials 10: 601. doi: 10.3390/ma10060601
![]() |
[21] |
Panchenko YM, Marshakov AI (2017) Prediction of first-year corrosion losses of carbon steel and zinc in continental regions. Materials 10: 422. doi: 10.3390/ma10040422
![]() |
[22] |
Tidblad J, Mikhailov AA, Kucera V (2000) Model for the prediction of the time of wetness from average annual data on relative air humidity and air temperature. Prot Metal 36: 533–540. doi: 10.1023/A:1026621009635
![]() |
[23] |
Feliu S, Morcillo M, Feliu Jr S (1993) The prediction of atmospheric corrosion from meteorological and pollution parameters—I. Annual corrosion. Corros Sci 34: 403–414. doi: 10.1016/0010-938X(93)90112-T
![]() |
[24] | Mikhailov AA, Panchenko YM, Kuznetsov YI (2016) Atmospheric corrosion and protection of metals, Tambov: Pershin, Inc. (in Russian). |
[25] |
Zakipour S, Tidblad J, Leygraf C (1995) Atmospheric Corrosion Effects of SO2 and O3 on Laboratory‐Exposed Copper. J Electrochem Soc 142: 757–760. doi: 10.1149/1.2048530
![]() |
[26] | Tidblad J, Kucera V (1993) The role of NOx and O3 in the corrosion and degradation of materials, Report 1993:6Е, Stockholm, Sweden: Swedish Corrosion Institute, 1–46. |
1. | Ping Han, Zhengbo Chang, Xinzhu Meng, Asymptotic Dynamics of a Stochastic SIR Epidemic System Affected by Mixed Nonlinear Incidence Rates, 2020, 2020, 1076-2787, 1, 10.1155/2020/8596371 | |
2. | Zhenzhen Shi, Qingjian Li, Weiming Li, Huidong Cheng, Poincaré Map Approach to Global Dynamics of the Integrated Pest Management Prey-Predator Model, 2020, 2020, 1076-2787, 1, 10.1155/2020/2376374 | |
3. | Zheng Li, Fang Wang, Jianhui Wang, Adaptive Finite-Time Neural Control for a Class of Stochastic Nonlinear Systems With Known Hysteresis, 2020, 8, 2169-3536, 123639, 10.1109/ACCESS.2020.2974871 | |
4. | Yufei Wang, Huidong Cheng, Qingjian Li, Dynamical Properties of a Herbivore-Plankton Impulsive Semidynamic System with Eating Behavior, 2020, 2020, 1076-2787, 1, 10.1155/2020/5726895 | |
5. | Dezhao Li, Yu Liu, Huidong Cheng, Dynamic Complexity of a Phytoplankton-Fish Model with the Impulsive Feedback Control by means of Poincaré Map, 2020, 2020, 1076-2787, 1, 10.1155/2020/8974763 | |
6. | Jing Xu, Mingzhan Huang, Xinyu Song, Dynamical analysis of a two-species competitive system with state feedback impulsive control, 2020, 13, 1793-5245, 2050007, 10.1142/S1793524520500072 | |
7. | Dezhao Li, Huidong Cheng, Yu Liu, Dynamic Analysis of Beddington–DeAngelis Predator-Prey System with Nonlinear Impulse Feedback Control, 2019, 2019, 1076-2787, 1, 10.1155/2019/5308014 | |
8. | Zhouhong Li, Tianwei Zhang, Permanence for Leslie-Gower predator-prey system with feedback controls on time scales, 2020, 1607-3606, 1, 10.2989/16073606.2020.1799256 | |
9. | Ryoto Himo, Masaki Ogura, Naoki Wakamiya, Iterative shepherding control for agents with heterogeneous responsivity, 2022, 19, 1551-0018, 3509, 10.3934/mbe.2022162 | |
10. | Yuan Tian, Yan Gao, Kaibiao Sun, A fishery predator-prey model with anti-predator behavior and complex dynamics induced by weighted fishing strategies, 2023, 20, 1551-0018, 1558, 10.3934/mbe.2023071 | |
11. | Wei Li, Tonghua Zhang, Yufei Wang, Huidong Cheng, Dynamic analysis of a plankton–herbivore state-dependent impulsive model with action threshold depending on the density and its changing rate, 2022, 107, 0924-090X, 2951, 10.1007/s11071-021-07022-w | |
12. | Yazhi Wu, Guangyao Tang, Changcheng Xiang, Dynamic analysis of a predator-prey state-dependent impulsive model with fear effect in which action threshold depending on the prey density and its changing rate, 2022, 19, 1551-0018, 13152, 10.3934/mbe.2022615 | |
13. | Jitsuro Sugie, Kazuki Ishibashi, The only limit cycle that appears in damped harmonic oscillators affected by state-dependent impulses, 2024, 531, 0022247X, 127886, 10.1016/j.jmaa.2023.127886 | |
14. | Xiangjun Dai, Jianjun Jiao, Qi Quan, Airen Zhou, Dynamics of a predator–prey system with sublethal effects of pesticides on pests and natural enemies, 2024, 17, 1793-5245, 10.1142/S1793524523500079 | |
15. | Yongfeng Li, Song Huang, Zhongyi Xiang, A state-dependent impulsive system with ratio-dependent action threshold for investigating SIR model, 2024, 9, 2473-6988, 4781, 10.3934/math.2024231 | |
16. | Liping Wu, Zhongyi Xiang, Dynamic analysis of a predator-prey impulse model with action threshold depending on the density of the predator and its rate of change, 2024, 9, 2473-6988, 10659, 10.3934/math.2024520 | |
17. | Xiangjun Dai, Jianjun Jiao, Qi Quan, Dynamic Analysis of an Instantaneous/Non‐Instantaneous Unilateral Diffusion Prey–Predator Model With Impulsive Control Strategy, 2025, 0170-4214, 10.1002/mma.10930 |
ξ | p | q | τ | T | VcostT |
0.15 | 0.094 | 0.038 | 0.886 | 18.723 | 105.0 |
0.20 | 0.186 | 0.075 | 0.775 | 19.345 | 99.0 |
0.25 | 0.281 | 0.113 | 0.663 | 19.735 | 96.0 |
0.33 | 0.431 | 0.173 | 0.483 | 19.967 | 91.4 |
0.35 | 0.469 | 0.188 | 0.438 | 19.867 | 91.7 |
0.40 | 0.563 | 0.225 | 0.325 | 19.500 | 92.0 |
0.45 | 0.656 | 0.263 | 0.213 | 18.720 | 92.8 |
ξ | VcostT(s2s1=12) | VcostT(s2s1=1) | VcostT(s2s1=2) | VcostT(s2s1=5) |
0.15 | 99.7 | 105.0 | 57.0 | 72.4 |
0.2 | 89.7 | 99.0 | 59.3 | 88.1 |
0.25 | 81.4 | 96.0 | 62.1 | 104.8 |
0.33 | 70.0 | 91.4 | 67.4 | 132.1 |
0.35 | 67.7 | 91.7 | 69.3 | 140.0 |
0.4 | 62.2 | 92.0 | 74.0 | 161.0 |
0.45 | 57.8 | 92.8 | 81.5 | 187.0 |
ξ | p | q | τ | T | VcostT |
0.15 | 0.094 | 0.038 | 0.886 | 18.723 | 105.0 |
0.20 | 0.186 | 0.075 | 0.775 | 19.345 | 99.0 |
0.25 | 0.281 | 0.113 | 0.663 | 19.735 | 96.0 |
0.33 | 0.431 | 0.173 | 0.483 | 19.967 | 91.4 |
0.35 | 0.469 | 0.188 | 0.438 | 19.867 | 91.7 |
0.40 | 0.563 | 0.225 | 0.325 | 19.500 | 92.0 |
0.45 | 0.656 | 0.263 | 0.213 | 18.720 | 92.8 |
ξ | VcostT(s2s1=12) | VcostT(s2s1=1) | VcostT(s2s1=2) | VcostT(s2s1=5) |
0.15 | 99.7 | 105.0 | 57.0 | 72.4 |
0.2 | 89.7 | 99.0 | 59.3 | 88.1 |
0.25 | 81.4 | 96.0 | 62.1 | 104.8 |
0.33 | 70.0 | 91.4 | 67.4 | 132.1 |
0.35 | 67.7 | 91.7 | 69.3 | 140.0 |
0.4 | 62.2 | 92.0 | 74.0 | 161.0 |
0.45 | 57.8 | 92.8 | 81.5 | 187.0 |