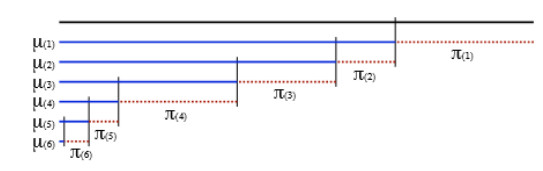
During a first or second course in number theory, students soon encounter several sets of "number theoretic interest". These include basic sets such as the rational numbers, algebraic numbers, transcendental numbers, and Liouville numbers, as well as more exotic sets such as the constructible numbers, normal numbers, computable numbers, badly approximable numbers, the Mahler sets S, T and U, and sets of irrationality exponent m, among others. Those exposed to some measure theory soon make a curious observation regarding a common property seemingly shared by all these sets: each of the sets has Lebesgue measure equal to zero, or its complement has Lebesgue measure equal to zero. In this expository note, we explain this phenomenon.
Citation: Taboka Prince Chalebgwa, Sidney A. Morris. Number theoretic subsets of the real line of full or null measure[J]. Electronic Research Archive, 2025, 33(2): 1037-1044. doi: 10.3934/era.2025046
[1] | Charlotte Christiansen, Christos S. Savva . Government bond market risk-return trade-off. Quantitative Finance and Economics, 2023, 7(2): 249-260. doi: 10.3934/QFE.2023013 |
[2] | Mara Madaleno, Elisabete Vieira . Volatility analysis of returns and risk: Family versus nonfamily firms. Quantitative Finance and Economics, 2018, 2(2): 348-372. doi: 10.3934/QFE.2018.2.348 |
[3] | Nitesha Dwarika . An innovative extended Bayesian analysis of the relationship between returns and different risk measures in South Africa. Quantitative Finance and Economics, 2022, 6(4): 570-603. doi: 10.3934/QFE.2022025 |
[4] | Steve Cook, Duncan Watson . Volatility in the Housing Market: Evidence on Risk and Return in theLondon Sub-market. Quantitative Finance and Economics, 2017, 1(3): 272-287. doi: 10.3934/QFE.2017.3.272 |
[5] | Dumisani Pamba, Sophia Mukorera, Peter Moores-Pitt . The asymmetric effects of cross-border equity flow volatility on equity market returns in SANEK countries. Quantitative Finance and Economics, 2025, 9(1): 40-75. doi: 10.3934/QFE.2025002 |
[6] | Chikashi Tsuji . The meaning of structural breaks for risk management: new evidence, mechanisms, and innovative views for the post-COVID-19 era. Quantitative Finance and Economics, 2022, 6(2): 270-302. doi: 10.3934/QFE.2022012 |
[7] | Elyas Elyasiani, Luca Gambarelli, Silvia Muzzioli . The Information Content of Corridor Volatility Measures During Calm and Turmoil Periods. Quantitative Finance and Economics, 2017, 1(4): 454-473. doi: 10.3934/QFE.2017.4.454 |
[8] | Didier Sornette, Peter Cauwels, Georgi Smilyanov . Can we use volatility to diagnose financial bubbles? lessons from 40 historical bubbles. Quantitative Finance and Economics, 2018, 2(1): 486-590. doi: 10.3934/QFE.2018.1.1 |
[9] | David Melkuev, Danqiao Guo, Tony S. Wirjanto . Applications of random-matrix theory and nonparametric change-point analysis to three notable systemic crises. Quantitative Finance and Economics, 2018, 2(2): 413-467. doi: 10.3934/QFE.2018.2.413 |
[10] | Samuel Asante Gyamerah . Modelling the volatility of Bitcoin returns using GARCH models. Quantitative Finance and Economics, 2019, 3(4): 739-753. doi: 10.3934/QFE.2019.4.739 |
During a first or second course in number theory, students soon encounter several sets of "number theoretic interest". These include basic sets such as the rational numbers, algebraic numbers, transcendental numbers, and Liouville numbers, as well as more exotic sets such as the constructible numbers, normal numbers, computable numbers, badly approximable numbers, the Mahler sets S, T and U, and sets of irrationality exponent m, among others. Those exposed to some measure theory soon make a curious observation regarding a common property seemingly shared by all these sets: each of the sets has Lebesgue measure equal to zero, or its complement has Lebesgue measure equal to zero. In this expository note, we explain this phenomenon.
Originating from the Modern Portfolio Theory by Markowitz (1952), the risk-return relationship is assumed to hold a positive and linear relationship—the higher the risk, the higher the return. However, there are many studies documented in literature that show a positive, or negative, or no relationship at all, as sufficed by Bekiros et al. (2017), Umutlu (2019) and Harris et al. (2019). Due to the magnitude of conflicting results over the years, this has caused an international and local debate to arise regarding the risk-return relationship (Jin, 2017). International studies have explored a number of theories and models in an attempt to resolve the inconclusive empirical backing of the risk-return relationship. For example, in recent studies by Jensen and Maheu (2018), Kim and Kim (2018) and Harris et al. (2019), it is noted that the risk-return relationship is positive, in line with theoretical expectations, when the volatility feedback (effect) is taken into account. Volatility feedback is considered a market characteristic of key interest because it takes longer to die out relative to regular volatility (Mancino and Sanfelici, 2020).
In comparison to international literature, the methods employed by South African studies and the volume of literature on the topic is limited (Manadimika and Chinzara, 2012; Steyn and Theart, 2019). Typically, higher levels of volatility are found in an emerging market (Adu et al., 2015). Therefore, it followed that the presence of volatility feedback, in an emerging market such as South Africa, can be expected to be more pronounced. Drawn from the National Treasury (2018), the presence of volatility feedback can pose a serious threat to the South African market and the business confidence of investors, leading to negative financial implications. This is because private investment contributes to around 60% of total investment, and South Africa relies heavily on foreign investment, because of a low rate of local savings.
Although volatility feedback is considered a useful market characteristic, this study focuses more on its impact on the risk-return relationship, following Jensen and Maheu (2018), Kim and Kim (2018) and Harris et al. (2019). The research problem of this study is that volatility feedback is an important source of asymmetry that should be taken into account when investigating the risk-return relationship. The primary aim is to investigate the magnitude of volatility feedback in the South African market. The secondary aim is to investigate the risk-return relationship provided volatility feedback is taken into account. This study followed Jensen and Maheu (2018), which is the first and only known study to apply the nonparametric Bayesian approach to the risk-return relationship and volatility feedback topic, to the best of the authors knowledge. The Bayesian approach is in contrast to conventional methods, such as regression analysis and the GARCH approach. Conventional quantitative finance methods, also known as the frequentist approach are based on classical statistics, where theory is either accepted or rejected based on empirical results.
Bayesian statistics is an extensive field of statistics built on Bayes (1763) theorem, which is defined as the probability estimation of a relationship given prior information. Specifically, where the theory and empirical model are closely related by means of the prior information. In this case, the relationship is between risk and return, and the prior information refers to volatility feedback, which is modelled from the historical price data. In the context of risk estimation, the probabilities of possible future outcomes can be estimated given prior information. This means that when an individual takes on some level of risk, they have some probability of knowledge, whereas in the case of uncertainty, they do not. In contrast to conventional methods, the Bayesian approach has the ability to effectively account for uncertainty as well as asymmetry, thus allowing for a more robust and informative estimation of the risk-return relationship (Jensen and Maheu, 2018; Karabatsos, 2017).
Following Jensen and Maheu (2018) and Karabatsos (2017), the Bayesian approach can be modelled in a parametric or nonparametric framework. A parametric model is defined as a finite model and is based on modelling assumptions, such as the normality assumption. Common examples include the regression analysis and the GARCH approach. However, the parametric Bayesian model is more robust than the conventional methods because of clustering analysis. Meaning that, although the model follows the normality assumption, it has the ability to take into account asymmetric forms of the risk-return relationship, to a greater extent than conventional models.
The nonparametric approach adjusts for model misspecifications, measurement errors and allows for a wide array of higher moment properties such as skewness, kurtosis and multiple modes (Jin, 2017). The nonparametric approach, also known as a "model free" approach, by Jensen and Maheu (2018), estimates the data free from predetermined constraints and assumptions, such as the normality assumption. Consequently, the use of the nonparametric Bayesian approach produces a powerful method for the estimation of the risk-return relationship. The model's ability to effectively fit nonlinear and asymmetric properties ensure a robust and reliable result.
In summary, by taking volatility feedback into account, this can assist the estimation of the risk-return relationship, following Jensen and Maheu (2018), Kim and Kim (2018) and Harris et al. (2019). According to Bekiros et al. (2017), the risk-return relationship is of paramount significance as it forms the basis of a number of strategies and investment ventures. A holistic view of the risk-return relationship and volatility feedback, can provide useful information to various market participants such as investors, portfolio managers and policymakers, with respect to their decision-making processes. The application of the novel Bayesian approach, by Jensen and Maheu (2018), ensures a more robust estimation of the risk-return relationship. In turn, this can contribute to advancements in stochastic volatility modelling and efficient risk estimation in the fields of portfolio and risk management. The main contribution of this study was to highlight the novel nonparametric Bayesian approach in South Africa, where literature mainly constituted of regression analysis and the GARCH approach. In addition, to provide reliable investor information for those who seek an opportunity to optimize their chance to earn a superior return.
This research article is made up of five parts: First, the risk-return relationship and volatility feedback topic are introduced. Second is the literature review which consists of the theoretical review and empirical review. The theoretical review covers the origination and theory of the volatility feedback phenomenon and how it is linked to the risk-return relationship. The empirical review covers local and international studies that investigate the risk-return relationship and volatility feedback. Third, the methodology covers the dataset and method procedure of the Bayesian approach employed in this study. Fourth, the empirical results show the data exploration and preliminary test of the parametric Bayesian approach. This study analyzes volatility feedback, by the parametric Bayesian approach followed by the nonparametric Bayesian approach, then the risk-return relationship. Finally, the key findings and implications of the test results are concluded.
According to Bekiros et al. (2017), developed and emerging financial markets are subject to volatility, a strong persistence of risk which is asymmetric in nature. Two traditional theories documented to explain the phenomenon of asymmetric volatility are the leverage effect and volatility feedback, as sufficed by Jin (2017), Umutlu (2019) and Harris et al. (2019). Both theories describe a relationship between volatility and price movements; where the leverage effect is a nonlinear relationship, and volatility feedback is a positive and linear relationship (Jensen and Maheu, 2018). Asymmetric volatility and the leverage effect are treated synonymously by the GARCH school of models (Mandimika and Chinzara, 2012). However, Jin (2017) stated that asymmetric volatility is associated to a time-varying risk-premium which is linked to volatility feedback. In the context of this study, volatility feedback is specifically treated as a measure of volatility and asymmetry, in line with Jensen and Maheu (2018).
Volatility feedback, introduced by Pindyck (1984), describes the price movements in the market—for a volatility shock in the market, this causes an increase in expected risk, a rise in return and a drop in share price. The volatility feedback mechanism is defined as a measure of volatility that is strongly persistent over time and stochastic in nature (Mancino and Sanfelici, 2020). Kim and Kim (2018) described volatility feedback as a predictor of unanticipated errors, as a result of macroeconomic fundamentals, which affects returns. Moreover, that volatility feedback has the ability to counteract cumulative price movements, such as an increase or decrease in price, and thus account for asymmetry.
According to Umutlu (2019), volatility feedback is based on two assumptions, asymmetric volatility and the trade-off theory. Asymmetric volatility refers to the tendency of volatility to increase more for negative returns than positive returns, or vice versa, for the same magnitude (Jin, 2017). The trade-off theory is where an investor only takes on a high level of risk if compensated by a high level of return; hence, a positive risk-return relationship (Markowitz, 1952). Explained by Jensen and Maheu (2018), given that volatility is a priced factor in the market, meaning, for a price movement in shares, there is a relation to risk. For a volatility shock in the market, a strong persistence of risk, actual volatility persists and signals future volatility by asymmetric volatility. From that increase in volatility, results an increase in return by the trade-off theory which stems from the idea - the higher the risk, the higher the return. Hence, volatility feedback closely reflects the risk-return relationship by definition. This is why the volatility feedback mechanism can also be described as a risk premium that is an increasing function of volatility in the market (Jin, 2017).
Mancino and Sanfelici (2020) stated that volatility feedback is a useful tool in understanding market conditions as it indicates the level of liquidity of individual securities as well as the market. Consequently, it can be used as an indicator of market stability and assist investor decisions. The presence of strong volatility feedback suggests recessionary market conditions, whereas a weak presence indicates expansionary market conditions. This affects price movements and suggests the rising or falling of market prices, respectively. Forecasting this measure can allow an investor to strategize their investment venture to improve the probability of realizing a superior return. For example, in the presence of strong volatility feedback which indicates recessionary market conditions, prices are expected to fall. Therefore, an investor can immediately short sell their shares to minimize their future potential loss.
In summary, volatility feedback is a positive and linear relationship between price movements and volatility, by definition and the assumptions, in line with the theoretical risk-return relationship. Hence, volatility feedback is identified as an important source of asymmetry to take into account in determining a positive risk-return relationship, in line with theoretical expectations (Jensen and Maheu, 2018; Kim and Kim, 2018; Harris et al., 2019). Although the documented theoretical framework of volatility feedback is limited due to literature, the empirical review provides more insight to the significance of accounting for this measure when investigating the risk-return relationship.
Sultan (2018) investigated asymmetric volatility, in the context of volatility feedback, for twenty-four shares listed on the Finnish stock market. The study analyzed an updated sample period where the 2008 financial crisis and any newly listed companies within the time period were excluded. An EGARCH and ADCC-EGARCH model were applied to the data for the sample period of 1 January 2009 to 31 December 2017. The variables used were returns from the OMX Helsinki 25 stock index against conditional volatility and covariance. The study found that the presence of the leverage effect is high and that of volatility feedback is low. Further, that negative returns affected the risk premium more than positive returns, in line with the asymmetric volatility phenomenon.
Kim and Kim (2018) investigated volatility feedback by employing a unified framework which is a generalization of a number of sub-models to the United States (US) market. The monthly data sets were analyzed for a sample period from January 1959 to May 2014. The variables of interest were excess returns, and macroeconomic fundamentals to account for risk. The unified framework consisted of expected returns which were modelled as a linear function of expected variance, a probit model and a tailored factor called "volatility feedback news." The study explicitly took into account that volatility feedback is driven by news as a result of changes in macroeconomic fundamentals. Kim and Kim (2018) concluded a positive risk-return relationship for their study, in line with traditional expectations.
However, the main limitation in the study by Kim and Kim (2018), was the linear function of volatility. It presents an issue similar to one of the main drawbacks of the parametric GARCH approach, where the parameters are subject to nonnegativity constraints (Jin, 2017). If the parameters do not meet this restriction, then some adjustment has to be imposed to the data (Jensen and Maheu, 2018). Returns cannot be a linear function of volatility because empirically, there exists a magnitude of conflicting results regarding the relationship (Bekiros et al., 2017; Umutlu, 2019; Harris et al., 2019). In parametric approaches, it is common practice to impose assumptions and constraints on data (Jin, 2017). In contrast, for the Bayesian approach by Jensen and Maheu (2018), parameters are treated as random variables with no constraints imposed on the data when introduced into the model. This means that the parameters are treated in accordance to the probability of an outcome, based on the method used, and not adjusted to fit a certain or fixed result (Karabatsos, 2017; Kim and Kim, 2018).
Jensen and Maheu (2018) applied a nonparametric Bayesian approach to the US market for a sample period from January 1885 to December 2011. The study analyzed monthly excess returns, calculated as the difference between the S & P500 returns and risk-free rate for a specified sample of forty years followed by a 1-month US Treasury-bill (T-bill) rate. A bias-adjusted realized variance is calculated, following Hansen and Lunde (2006), where the bias-adjustment accounts for microstructure noise. Jensen and Maheu (2018) took into account volatility feedback which is considered an important source of asymmetry that affects risk estimation. The study recommended moving away from linearity and to include densities with higher moment properties. Results were graphically presented by plots of density estimation over a 90% credible interval. Volatility feedback was found to get stronger over time and once taken into account, the study found a positive and nonlinear risk-return relationship.
Harris et al. (2019) found that there was a strong presence of volatility feedback and the leverage effect due to the persistent volatile periods in the market of the United Kingdom. After accounting for both effects, a positive risk-return relationship was found at all states of volatility. The sample period of July 1962 to December 2005 for the monthly data were analyzed using a Value at Risk (VaR) model. A VaR model focused on the tails of a distribution where extreme events such as the 2008 financial crises occurred. A Markov switching model was further applied to account for the time-varying nature of volatility, for an extended period of July 1962 to December 2016. This was in order to account for the original period, the 2008 financial crisis and the remaining subsequent years. The addition of the Markov switching model is often used to account for the different regimes of volatility.
However, Chang et al. (2017) stated that the Markov switching model is considered impractical and unrealistic for two reasons: First, because the forecast of the model is based on the current state and not the underlying time series. Second, the Markov chain estimates the regimes in isolation of other parts of the model. This implied a frequentist or classical approach, where theory is either accepted or rejected, based on the empirical results. In contrast to the Bayesian approach, which has the ability to account for prior information before making an estimation. Hence, the Markov switching model is considered more effective when used in conjunction with the Bayesian approach, according to Chang et al. (2017).
In an international study by Jin (2017), a negative risk-return relationship was found for the majority of markets in its sample, including the Johannesburg Stock Exchange (JSE) of South Africa. The study analyzed the respective daily returns and variance for the sample period from January 2001 to October 2014 of sixteen stock markets. Jin (2017) highlighted the limitations of the GARCH approach as being prone to model misspecification as well as having a number of underlying assumptions and constraints due to being parametric. The study then made use of a nonparametric approach to overcome the limitations of the parametric approach. The nonparametric approach is a "model free" approach that estimates the data free from any predetermined assumptions (Jensen and Maheu, 2018). Jin (2017) applied the Hurst exponent to measure the long-term memory which referred to the persistence of risk over a long period of time. A detrended cross-correlation coefficient was then applied which has the ability to account for data on the verge of nonstationarity.
Results revealed a strong presence of long-term volatility and a negative risk-return relationship that got stronger over time. The finding of a negative risk-return relationship in South Africa was supported by Bekiros et al. (2017). Jin (2017) further investigated whether the result of the negative risk-return relationship was due to the leverage effect or volatility feedback. The majority of the markets showed very weak evidence of volatility feedback with the exception of South Africa, Brazil, India and Indonesia which were all emerging markets. Although both volatility feedback and the leverage effect were present, the negative risk-return relationship was found to be dominated by the leverage effect for the majority of markets, including South Africa. This finding was in contrast to the result of the BRICS study by Adu et al. (2015), who found the leverage effect present for all the markets except South Africa.
Steyn and Theart (2019) applied regression analysis of Jensen's alpha, the Sharpe ratio and paired t-tests. The aim of the study was to investigate whether South African investors were being compensated for taking on a higher level of risk. All the shares on the JSE were analyzed for the sample period from July 2004 to September 2018. The study concluded a negative risk-return relationship. With respect to the model, regression analysis consisted of basic statistical tests which were inadequate in fitting the financial data that was nonlinear and volatile in nature (Jensen and Maheu, 2018). Consequently, this should lead researchers to use more robust methods that are available to effectively model complex data such as the Bayesian approach (Karabatsos, 2017).
In summary, purely South African studies showed a limited modelling approach in the investigation of the risk-return relationship, as well as a nonpositive risk-return relationship, relative to the documented international literature. Although not empirically reviewed in detail in this study, in order to highlight the more unconventional approaches, it was noted that early South African studies by Mangani (2008), Mandimika and Chinzara (2012) and Adu et al. (2015) found no risk-return relationship. Mandimika and Chinzara (2012) recommended taking into account skewness and kurtosis when results are inconclusive regarding the risk-return relationship. From a chronological review of the aforementioned studies, it was noted that more significant evidence was found regarding volatility and asymmetric effects. However, the studies employed the GARCH approach which is considered misspecified and limited in accounting for asymmetry, by Jin (2017).
The more recent studies by Jin (2017), Bekiros et al. (2017) and Steyn and Theart (2019), found a negative risk-return relationship. These findings are still in contrast to the recent studies by international literature, such as Kim and Kim (2018), Harris et al. (2019) and Jensen and Maheu (2018), which found a positive risk-return relationship. A reason for the nonpositive risk-return relationship in the South African market could be due to the failure to account for volatility feedback when investigating the risk-return relationship or the choice of model (Jensen and Maheu, 2018; Kim and Kim, 2018). Given the result of high levels of volatility found in the studies by Mangani (2008), Mandimika and Chinzara (2012) and Adu et al. (2015), the investigation of volatility feedback and the risk-return relationship in the South African market is substantiated.
In the context of this study, only volatility feedback was explicitly investigated and not the leverage effect, since it was strongly associated to the risk-return relationship, as noted by Jensen and Maheu (2018). Moreover, in the BRICS study by Adu et al. (2015), the leverage effect was present for all the emerging markets, except South Africa.
This study focused on the largest South African market, the JSE, based on documented local literature. The secondary daily price data of the FTSE/ JSE ALSI was obtained from the Integrated Real-time Equity System (IRESS) database for the sample period from 15 October 2009 to 15 October 2019. Following Sultan (2018), daily data was analyzed because it provided a more precise estimate of the variables. An updated period of ten years was chosen, excluding both the financial crisis and apartheid era. The selection of this period was to limit the potential effects of structural breaks in the analysis. While it could be extended, it would become more susceptible to the influences of exogenous economic shocks. In addition, the sample included only listed companies; thus, newly listed and delisted companies were excluded.
Following Jensen and Maheu (2018), the variables excess returns and realized variance were calculated as follows. The daily ALSI closing price data was converted to ALSI market returns by the log transformation of Equation 1:
Rm=ln(PtPt−1) | (1) |
where Rm denoted market returns and Pt represented the share price for the current day t and Pt−1 was the share price for the previous day t−1, where t=1,…,2499. Thereafter, the calculation of excess returns was shown by Equation 2:
rt=Rm−Rf | (2) |
where rt denoted the market risk premium, equal to the difference between the market return Rm and the risk-free rate Rf. The annual risk-free rate was obtained from the South African Reserve Bank (SARB) and converted to a daily value from Equation 3, by Brooks (2014):
Daily Rf=(1+yearly Rf)(1365)−1 | (3) |
The South African T-bill was the proxy for the risk-free rate, primarily based on accessibility and availability, in line with Bekiros et al. (2017).
The calculation of the bias-adjusted realized variance by Hansen and Lunde (2006), was shown by Equation 4:
RVqt=ˆγ0+2q∑j=1(1−jq+1)ˆγj,where ˆγj=Nt−j∑t=1rt,irt,i+j | (4) |
where RVqt was equal to daily realized variance RVt where the bias-adjustment q was set to one.
Realized variance was equal to term one ˆγ0 which was equal to the first order of autocorrelation. Term two was the product of the bias-adjustment and ˆγj realized variance by definition. The latter was equal to the summation of returns squared r2t where Nt represented the number of daily returns in day t and j=0,…,q. The addition of kernel weights, not shown in Equation 4, ensured a positive bias-adjusted realized variance. In nonparametric Bayesian estimation, the application of kernels is often used, which acts as a type of weighting function. There are a number of different kernels; however, this study specified the Bartlett kernel, in line with Hansen and Lunde (2006).
According to Jensen and Maheu (2018), there are two specific opposing effects that act on excess returns. These effects are shown by the trade-off theory by Markowitz (1952) and volatility feedback by Pindyck (1984). The econometric model, given as Equation 5 by Jensen and Maheu (2018), shows how volatility feedback obscures any risk-return relationship. Note that throughout this study, the information set It−1 refers to all the possible values made up from the dataset, excess returns rt and realized variance RVT, represented by It−1={r1,RV1,…,rt,RVt} where t=1,…,T.
rt=E[rt|It−1]+α1(RVt−E[RVt|It−1])+et | (5) |
From Equation 5, E[rt|It−1] is defined as the expected conditional mean of excess returns, given the information set which is made up of excess returns and realized variance. This is an ex-ante (future) term and captures the positive risk-return relationship. The coefficient α1 of α1(RVt−E[RVt|It−1]) is a measure of the persistence of risk or a volatility shock on (RVt−E[RVt|It−1]). The latter is the difference between observed realized variance and expected realized variance. This is an ex-post (actual) term and captures volatility feedback. Finally, et is the innovation term of the model which captures the possible deviations between the observed and expected values (Brooks, 2014).
Provided volatility is a priced risk factor by Jensen and Maheu (2018), for a positive volatility shock α1, a strong persistence in risk; actual volatility persists and signals future volatility on expected returns. This results in a positive risk-return relationship by the trade-off theory which is captured by E[rt|It−1]. In turn, there is a demand for higher expected returns and in order to raise the expected returns; share prices decrease by volatility feedback, which is captured by the volatility innovation α1(RVt−E[RVt|It−1]).
In summary, for a positive volatility shock, there is an increase in E[rt|It−1] capturing the risk-return relationship and a decrease in α1(RVt−E[RVt|It−1]) capturing volatility feedback. Jensen and Maheu (2018) stated that this model mathematically shows how the two opposing effects act on excess returns. This is captured by the joint distribution of excess returns and realized variance. Once volatility feedback is established, the risk-return relationship is investigated. Note that this only occurs when the volatility shock is zero, as shown by Equation 6.
RVt=E[RVt|It−1] | (6) |
From Equation 6, in order for the volatility shock α1 of α1(RVt−E[RVt|It−1]) to be zero, the observed realized variance RVt needs to be equivalent to the expected realized variance E[RVt|It−1]. To aid understanding, this can be graphically represented as the intersection of the density lines of the observed and expected realized variance.
In the context of model implementation, the Bayesian approach consists of a prior which is an initial probability estimation and posterior which is an updated probability estimation (Bayes, 1763). Following Jensen and Maheu (2018), the prior is determined by the Dirichlet Process (DP) by Ferguson (1973), derived by the stick-breaking process by Sethuram (1994). The posterior is estimated by the slice sampling technique by Kalli et al. (2011) and Gibbs sampler.
The parametric Bayesian model follows the method procedure of the nonparametric Bayesian model by Jensen and Maheu (2018) with one exception—the model becomes a finite model and is further simplified by the author for computational ease. According to Karabatsos (2016), a nonparametric Bayesian model is often referred to being an infinite-mixture model. Essentially, a nonparametric Bayesian model assumes an infinite number of clusters, whereas a parametric Bayesian model assumes a finite number of clusters (Jensen and Maheu, 2018; Karabatsos, 2016). The parametric Bayesian model is a finite model, because by definition, a parametric model has a set number of parameters with respect to its sample size (Jin, 2017).
Therefore, although the parametric Bayesian model takes into account asymmetric properties, it does so, to a limited extent because the model is essentially parametric (Jin, 2017). Hence, the parametric model does not have the capacity to take into account an infinite number of possibilities and account for every possible risk-return relationship that could exist (Demirer et al., 2019). Consequently, there is no need to apply a prior to reduce an infinite model to a finite model since it is already finite. However, in order to derive the posterior parameter estimates, the posterior methods, the slice sampler by Kalli et al. (2011) and Gibbs sampler are still applied.
Following Jensen and Maheu (2018), in this studies procedure in uncovering the risk-return relationship, the first step is to model a joint distribution of excess returns and realized variance. This resulted in a number of bivariate density functions, which are the consequent possible densities of the joint model of the two variables, as shown in Equation 7:
p(rt,log(RVt)|It−1,Ω,Θ)=∞∑j=1wj∗f(rt,log(RVt)|θj,It−1) | (7) |
where the probability of excess returns and log realized variance is conditional on the information set, the mixture weights Ω=wj where ∑∞j=1wj=1 and mixture parameters Θ=θj where j=1,…,∞ which refers to the number of clusters of mixture parameters. This is equivalent to the sum of all the weights and functions of excess returns and log realized variance, given the parameters and information set.
The next step involves deriving a parametric version of the risk-return relationship by reducing the notation of Equation 7 to only the necessary components and having Equation 7 rewritten as Equation 8:
f(rt,log(RVt)|θj,It−1)≡f(rt|log(RVt),θj,It−1)∗f(log(RVt)|θj,It−1) | (8) |
where the latter is simply the product of the conditional distribution (term 1) and marginal distribution (term 2) by the law of total probability. Since excess returns and log realized variance theoretically tends to a normal distribution, Equation 8 allows for the representation of Equation 9 and 10, respectively.
Equation 9 and 10 follows a functional form of normality, like conventional parametric methods, such as basic regression analysis and the GARCH approach (Jin, 2017). However, the mixing of the finite number of cluster parameters allows for a wider array of joint densities, including higher moment asymmetric densities, in a finite sample space (Jensen and Maheu, 2018). Therefore, the parametric Bayesian model is more robust in accounting for asymmetry than the conventional aforementioned parametric models (Karabatsos, 2017; Jensen and Maheu, 2018).
f(rt|log(RVt),θj,It−1)=fN(rt|α0+α1RVt,η21RVt) | (9) |
From Equation 9 by Jensen and Maheu (2018), the conditional mean function of excess returns given log realized variance, the parameter and information set, is equivalent to the normal conditional mean function of excess returns. The latter is conditional on the coefficient α1 on RVt which represents the persistence of risk on realized variance and this term captures volatility feedback. The η21 on RVt indicates the systematic error on realized variance. This term refers to the error surrounding the stochastic measure of realized variance which is unavoidable regardless of the number of times the model is run (Beyhaghi et al., 2018).
f(log(RVt)|θj,It−1)=fN(log(RVt)|γ0+γ1log(RVt−1)+ |
+γ2log(RVt−1)+γ3rt−1√RVt−1+γ4|rt−1√RVt−1|,η22) | (10) |
From Equation 10 by Jensen and Maheu (2018), the conditional mean function of log realized variance, given the parameters and information set, is equivalent to the normal conditional mean function of log realized variance. The latter is conditional on the coefficients γ1, γ2, γ3 and γ4 which refers to the persistence of the variables. The first two terms cater for volatility feedback but the last two terms cater for the leverage effect. Although the latter two variables are taken into account, it is not within the scope of this study; thus, it is ignored.
Equation 11 and 12 are of main interest for the parametric Bayesian model. Conditioning is dropped for convenience, following Jensen and Maheu (2018). The addition of the innovation terms εt and υt shown in both Equation 11 and 12 is to aid understanding. Further simplifications made by the author for computational ease are discussed.
rt=α0+α1RVt+εt | (11) |
log(RVt)=γ0+γ1log(RVt−1)+γ2124882488∑i=1log(RVt+1−i) +γ3rt−1√RVt−1+γ4|rt−1√RVt−1|+υt | (12) |
In Equation 11, the realized variance measure is not introduced into the innovation term. This is because the error variance in the model accounts for all unexplained variance that arise from sources such as uncertainty and measurement errors (Chakraborty and Lozano, 2019). This includes the systematic error on the realized variance measure which refers to the error surrounding the stochastic nature of the risk measure (Beyhaghi et al., 2018). Hence, both the innovation terms εt and υt of Equation 11 and 12 capture the possible deviations between the observed and expected values. It is accounted for and reflected through the σ21 and σ22 of both models (Karabatsos, 2017).
In the original Equation 10 by Jensen and Maheu (2018), the coefficient γ2 is supposed to cater for volatility feedback over a six-month period. However, it is not shown in this study because the entire sample period is taken into account instead, as shown by Equation 12. This is in order to essentially determine the presence and persistence of volatility over time, in the South African market, to provide an overall state and condition of the market.
Following Jensen and Maheu (2018), the posterior procedure consists of a number of steps. Initially, random samples are drawn from a joint distribution by means of a slice sampling technique. The slice sampler is applied to Equation 7, except an additional random variable represented by ut is introduced, as shown in Equation 13:
p(rt,log(RVt),ut|Ω,Θ,It−1)=∞∑j=11(ut<wj)∗f(rt,log(RVt)|(θj,It−1)) | (13) |
where the aim of variable ut is to ensure that only positive weights are retained and all weights of zero are "sliced away".
Thereafter, the following iteration method is applied which refers to the repetitive resampling process of a collection of steps. Firstly, a Gibbs sampling technique is applied, which is often used when the joint distribution is unknown and it is simpler to draw samples from the known conditional distribution. In this case, the conditional distribution contained the cluster mixture parameters and weights. Secondly, since the priors are strong, this allowed for the formation of a conjugate conditional posterior, meaning, a conditional posterior that shared similar model properties to the prior. Thirdly, consequently, each of the random variables tends to form a homogenous distribution provided the given weights and parametric space. Finally, if the cluster count is amended, there may be further prior draws.
This procedure would continue; however, the Gibbs sampling process is subject to a burn-in period of which samples from the earlier stages that are no longer accurately representative of the required distribution are discarded. The original base distribution is then updated to the posterior distribution. Hence, so are the coefficients and parameter estimates of Equation 11 and 12, from which the conclusive results are drawn with respect to volatility feedback and the risk-return relationship.
According to Jensen and Maheu (2018), the nonparametric Bayesian model follows the derivation of the joint model in 3.3.1.1, with one exception. The nonparametric model simply takes on a second subscript j which represents the cluster mixture parameters in an infinite sample space. It is this component j, the cluster mixture of parameters, which allows for the infinite asymmetric properties in the model. Hence, a greater number of possibilities, as in asymmetric densities, of the risk-return relationship in an infinite sample space. Without component j, the model is consequently limited and does not take into account the higher moment asymmetric properties such as skewness, kurtosis and multiple modes as effectively (Demirer et al., 2019; Jensen and Maheu, 2018). While the derivation and interpretation of the variables are identical to the parametric Bayesian model, they are recapped in this study for convenience.
Following Jensen and Maheu (2018), in this studies procedure in uncovering the risk-return relationship, the first step is to model a joint distribution of excess returns and realized variance. This resulted in a number of bivariate density functions which are the consequent possible densities of the joint model of the two variables shown in Equation 14:
p(rt,log(RVt)|It−1,Ω,Θ)=∞∑j=1wj∗f(rt,log(RVt)|θj,It−1) | (14) |
where the probability of excess returns and log realized variance is conditional on the information set, the mixture weights Ω=wj where ∑∞j=1wj=1 and mixture parameters Θ=θj where j=1,…,∞ which refers to the number of clusters of mixture parameters. This is equivalent to the sum of all the weights and functions of excess returns and log realized variance, given the parameters and information set.
The next step involved deriving a nonparametric version of the risk-return relationship by reducing the notation of Equation 14 to only the necessary components and rewriting Equation 14 as Equation 15:
f(rt,log(RVt)|θj,It−1)≡f(rt|log(RVt),θj,It−1)∗f(log(RVt)|θj,It−1) | (15) |
where the latter is simply the product of the conditional distribution (term 1) and marginal distribution (term 2) by the law of total probability. Since excess returns and log realized variance theoretically tends to a normal distribution, Equation 15 allows for the representation of Equation 16 and 17, respectively.
Equation 16 and 17 follows a functional form of normality like conventional methods such as regression analysis and the GARCH approach. However, the mixing of the infinite number of cluster parameters allows for a wide array of joint distributions, including asymmetric densities, in an infinite sample space (Karabatsos, 2017). The nonparametric approach allows for moving away from linearity and effectively includes densities with higher moment properties such as skewness, kurtosis and multiple modes (Jensen and Maheu, 2018). Equations 16 and 17 are the main equations of interest for the nonparametric Bayesian model.
f(rt|log(RVt),θj,It−1)=fN(rt|α0,j+α1,jRVt,η21,jRVt) | (16) |
From Equation 16 by Jensen and Maheu (2018), the conditional mean function of excess returns given log realized variance, the parameter and information set, is equivalent to the normal conditional mean function of excess returns. The latter is conditional on the coefficient α1,j on RVt which represented the persistence of risk on realized variance and this term captured volatility feedback. The η21,j on RVt indicates the systematic error on realized variance. This term referred to the error surrounding the stochastic measure of realized variance which is unavoidable regardless of the number of times the model is run (Beyhaghi et al., 2018).
f(log(RVt)|θj,It−1)=fN(log(RVt)|γ0,j+γ1,jlog(RVt−1)+ γ2,jlog(RVt−1)+γ3,jrt−1√RVt−1+γ4,j|rt−1√RVt−1|,η22,j) | (17) |
From Equation 17 by Jensen and Maheu (2018), the conditional mean function of log realized variance, given the parameters and information set, is equivalent to the normal conditional mean function of log realized variance. The latter is conditional on the coefficients γ1,j, γ2,j, γ3,j and γ4,j which refers to the persistence of the variables. The first two terms cater for volatility feedback but the last two terms cater for the leverage effect. Although the latter two variables are taken into account, it is not within the scope of this study; thus, it is ignored. Further, with respect to model implementation, conditioning is dropped for convenience for the equations of interest, Equations 16 and 17, and realized variance is not introduced into the error term by the author for computational ease.
The nonparametric Bayesian approach is also known as an infinite-mixture model by Karabatsos (2017), and it followed that every possible risk-return relationship that can hold is taken into account, allowing for an infinite number of possibilities. However, from an infinite number of possibilities arose a certain level of uncertainty attached to the variables due to their stochastic nature. This may induce model sensitivity and lead to problems such as overfitting, which can affect model fit and results. Therefore, following Jensen and Maheu (2018), the Bayesian Dirichlet Process (DP) is applied to reduce the uncertainty associated with an infinite model, by effectively reducing it to a finite means and ultimately improving the predictive ability of the model.
The Bayesian DP by Ferguson (1973), is defined as a probability estimation made up of a number of densities for a given sample space. In the context of nonparametric Bayesian problems, there are two conditions required to be met in order to ensure strong priors as it affects the form of the posterior. One, the prior is large, provided an infinite sample space to allow for conjugacy, that is, to allow for the sharing of model properties. Two, the shape of the prior is adequate. The Bayesian DP prior model addresses both conditions.
Conjugacy is a property of the DP for the prior as well as one of the steps in the Gibbs sampling technique for the posterior, by Jensen and Maheu (2018). This property is highlighted as one of significance because conjugacy refers to the sharing of model properties which affects the density form of the posterior. To clarify, if a prior had a density with asymmetric higher moment properties, then by conjugacy, the posterior is subject to those asymmetric higher moment properties when updating its parameters. Without the property of conjugacy, this can affect the model's convergence which describes the model's tendency towards a statistically sound result.
The DP can be derived by a number of ways; however, the stick-breaking process is foremost for practical application. The stick-breaking process by Sethuram (1994), involves the mixture weights being randomly drawn (from the joint distribution, in this case) by the successive breaking of intervals for a point of positive mass. Figure 1 shows a graphical representation of the stick-breaking process.
From Figure 1, each weight is represented as π for every jth cluster mixture and the μ represents the corresponding mean values of the joint densities. This is given by Equation 18:
πj=wjj−1∏i=1(1−wj),wj IID Beta(1,k) | (18) |
where the stick-breaking weights πj is equal to the weights, represented as wj for every jth cluster mixture, where wj is independent and identically distributed for a beta distribution with a mean of one and variance of k.
The mean of one refers to the sum of cluster weights in order to represent the probability base distribution. The precision parameter, also known as the concentration parameter, given as k determines the extent of clustering and variance, in relation to the base distribution (Karabatsos, 2017). Explained by Jensen and Maheu (2018), as the concentration parameter increases, the spread of the positive cluster weights increases and there is no parameter mixing which leads to a continuous prior base distribution. If the concentration parameter decreases to zero, the spread decreases and the cluster weights concentrate at a single point. Therefore, a positive concentration parameter accompanied by a sufficient sample is desirable.
The posterior procedure consists of a number of steps. Initially, random samples are drawn from a joint distribution by means of a slice sampling technique, by Kalli et al. (2011). The slice sampler is applied to Equation 14, except an additional random variable represented by ut is introduced, as shown in Equation 19, by Jensen and Maheu (2018):
p(rt,log(RVt),ut|Ω,Θ,It−1)=∞∑j=11(ut<wj)∗f(rt,log(RVt)|θj,It−1) | (19) |
where the aim of variable ut is to ensure that only positive weights are retained and all weights of zero are "sliced away."
Thereafter, the following iteration method is applied which refers to the repetitive resampling process of a collection of steps. Firstly, a Gibbs sampling technique is applied, which is often used when the joint distribution is unknown and it is simpler to draw samples from the known conditional distribution. In this case, the conditional distribution contains the cluster mixture parameters and weights. Secondly, since the priors are strong, this allows for the formation of a conjugate conditional posterior, meaning, a conditional posterior that shares similar model properties to the prior. Thirdly, consequently, each of the random variables tends to form a homogenous distribution provided the given weights and nonparametric space. Finally, if the cluster count is amended, there may be further prior draws.
This procedure would continue; however, the Gibbs sampling process is subject to a burn-in period of which samples from the earlier stages that are no longer accurately representative of the required distribution are discarded. The prior base distribution is then updated to the posterior distribution. In this case, so are the coefficients and parameter estimates of Equation 16 and 17, from which conclusive results can be drawn with respect to the risk-return relationship and volatility feedback.
The parametric and nonparametric Bayesian model follows the methodology by Jensen and Maheu (2018), in terms of the choice of the prior and posteriors implemented in this study. The Dirichlet prior process by Ferguson (1973), is derived by the stick-breaking process by Sethuram (1994). The posterior Markov Chain Monte Carlo (MCMC) methods are based on the slice sampler by Kalli et al. (2011) and Gibbs sampling technique.
The test results of both the parametric and nonparametric Bayesian models are estimated by a specialized MATLAB software, 'Bayesian Regression: Nonparametric and Parametric Models' designed by George Karabatsos. This menu-driven software is used mainly due to its computational ease and efficiency (Karabatsos, 2017).
According to the study by Jensen and Maheu (2018), the results of the nonparametric Bayesian approach were presented by graphical plots of density estimation over a 90% credible interval. A density estimation refers to a probability estimation of an unknown distribution based on the given data. Following Karabatsos (2017), a credible or density interval is a Bayesian interval that accounts for conditional prior information, in contrast to a regular confidence interval. This study performs density estimation by means of a probability density function (pdf) plot over a 95% credible interval. The densities are plotted against the mean to essentially give the average form of the distribution.
Graphically, the peak of the density represents the mean value since it is a measure of central tendency. The magnitude and form of the density estimation are what is important and is further supported by the posterior parameter estimates. These posterior parameter estimates are determined over a 75% and 95% credible interval in the form of numerical analysis (Karabatsos, 2017).
For the parametric and nonparametric Bayesian model, the posterior point estimates of the parameters are analyzed over a 75% and 95% credible interval. Additionally, their robustness is determined by the Monte Carlo (MC) mixing value. For a 75% credible interval by Karabatsos (2017), if zero is excluded, then the parameter estimate is statistically significant. If zero is included, then the parameter estimate is statistically insignificant. For a 95% credible interval by Jensen and Maheu (2018), if the point estimate is found within the interval, it is concluded as statistically significant. The negative or positive signs indicate the direction in which the variable moves. If the point estimate does not lie within the interval, it is concluded as statistically insignificant. The MC mixing value by Karabatsos (2017), refers to how well all the parameters mix where the value is found between an interval of zero and one. A small value of 0.1 or 0.01 confirms adequate mixing, whereas an approximate value of 0.5 indicates optimal mixing. If the MC mixing value is inadequate, this indicates that the number of iterations is to be increased. Finally, for the analysis of volatility feedback and the risk-return relationship, the posterior parameter estimates are represented by ˆβ for the parametric Bayesian model and ˆd for the nonparametric Bayesian model.
Although not within the scope of this study, the model specifications are as follows. For the parametric Bayesian model, the prior variance of the slope parameters is specified as 1000 and the prior inverse gamma distribution of the error variance is specified as 0.001/2. For the nonparametric Bayesian model, the prior inverse gamma distribution of the error variance is specified as 5/2. For the DP, the intercept variance of the base distribution is specified as five. The prior gamma distribution, shape and rate, of the concentration parameter are both specified as one. Following Jensen and Maheu (2018), the posterior parameter estimates are determined by 20,000 MCMC sampling iterations, a burn-in period of 5000 and a thin number of 5. In this case, the latter thin number means that every fifth sampling iterate of the total 20,000 MCMC sampling iterations is collected to determine the posterior parameter estimates (Karabatsos, 2017).
Following standard literature, the ALSI index level data is differenced to log form before data exploration. This step is to ensure stationarity and avoid a spurious regression (Brooks, 2014). The data then undergoes common preliminary tests such as normality and heteroscedasticity, where the ALSI returns are found to have a volatile and asymmetric nature, in line with Adu et al. (2015). Data exhibiting such characteristics can effectively be accounted for by a parametric Bayesian model and more so, by a nonparametric Bayesian model, in line with the recommendations by Karabatsos (2017) and Jensen and Maheu (2018). Table 1 shows the basic descriptive statistics of the risk and return variables.
Variables | Mean | Variance | Kurtosis | Skewness | Minimum | Maximum |
rt | 0.0001 | 0.0001 | 1.277 | −0.167 | −0.037 | 0.042 |
r2t | 0.118 | 0.004 | −1.131 | −0.043 | 0.000 | 0.227 |
RVt | 0.981 | 0.000 | 3.185 | −1.567 | 0.946 | 0.988 |
log(RVt) | −0.019 | 0.000 | 3.323 | −1.593 | −0.056 | −0.012 |
rt/√RVt | 0.000 | 0.000 | 1.350 | −0.168 | −0.038 | 0.043 |
From Table 1, the summation of returns squared r2t is realized variance by definition (Jensen and Maheu, 2018). The RVt is a bias-adjusted measure of realized variance where the realized variance RVqt is equivalent to RVt since q is set to one, by Hansen and Lunde (2006). From the variance column, the realized variance by definition r2t contains more risk than the bias-adjusted realized variance RVt. The sum of squared returns r2t has negative excess kurtosis which indicates a thin tailed distribution. This finding is in contrast to the characteristics of an emerging market, which is subject to higher levels of volatility and is heavy-tailed, as noted by Adu et al. (2015).
The bias-adjusted realized variance RVt is heavy-tailed, as indicated by the positive excess kurtosis, implying that it contains more risk. However, it has a lower variance, in comparison to the realized variance by definition. This means that the bias-adjusted realized variance RVt is less risky because it has the ability to account for microstructure noise. The bias-adjustment accounts for the micro price movements in the market due to changes in supply and demand as well as stale prices which refers to when prices do not update to recent information (Hansen and Lunde, 2006). Thus, the bias-adjusted realized variance RVt provides a more robust estimate of risk, in line with Jensen and Maheu (2018).
On the other hand, returns rt has a positive excess kurtosis which indicates a heavy-tailed distribution. This finding is in line with the return characteristics of an emerging market, by Adu et al. (2015). With respect to skewness, all the variables reflect a negative value, where for returns, this indicates losses for investors. This is because negative skewness is associated to a negative payoff, by Mandimika and Chinzara (2012). Thus, it can be concluded that returns have an asymmetric nature since it follows an asymmetric distribution. Figure 2 shows a general relationship between excess returns and log realized variance by a basic scatter plot.
From Figure 2, the data of excess returns and log realized variance shows a nonlinear and asymmetric pattern. This result is in line with the South African study by Steyn and Theart (2019). The ends, in particular, show a number of outliers, in line with Jensen and Maheu (2018). Thus, in conclusion from Figure 1, the asymmetric properties and outliers shown by the data can be effectively accounted for by an asymmetric model, in line with Karabatsos (2017), Jensen and Maheu (2018) and Mandimika and Chinzara (2012).
Following Jensen and Maheu (2018), the summary statistics serve as a preliminary test to provide an overview of the parametric Bayesian model. Table 2 shows the results of the parameter estimates of the summary statistics.
Parameter Estimates | 75% Credible Interval | Mean | 95% Credible Interval | MC Mixing Value |
ˆα0 | (−0.118, −0.077) | −0.098 | (−0.156, −0.037) | 0.499 |
ˆα1 | (0.078, 0.121) | 0.100 | (0.038, 0.159) | 0.499 |
ˆσ21 | (0.000, 0.000) | 0.000 | (0.000, 0.000) | 0.492 |
ˆγ0 | (−0.019, −0.018) | −0.019 | (−0.019, −0.018) | 0.518 |
ˆγ1 | (−0.000, 0.000) | 0.000 | (−0.000, 0.000) | 0.512 |
ˆγ2 | (−0.096, 0.186) | 0.047 | (−0.370, 0.460) | 0.523 |
ˆγ3 | (0.062, 0.080) | 0.070 | (0.044, 0.098) | 0.490 |
ˆγ4 | (−0.126, −0.099) | −0.113 | (−0.152, −0.073) | 0.499 |
ˆσ22 | (0.000, 0.000) | 0.000 | (0.000, 0.000) | 0.500 |
From Table 2, the coefficient ˆα1 on RVt is the persistence of risk on realized variance and this term captures volatility feedback (Jensen and Maheu, 2018). The value is positive and statistically significant, confirming the presence of volatility feedback in the South African market, by both the 75 and 95% credible intervals. On the other hand, for the 95% credible interval, ˆγ1 has a mean value of zero which suggests an absence of volatility feedback. Further, ˆγ2 which in this case accounts for volatility feedback over the entire sample period is positive, has a high mean value and is statistically significant. However, for the 75% credible interval, both ˆγ1 and ˆγ2 are statistically insignificant, indicating no volatility feedback. Overall, these results indicate a weak presence of volatility feedback in the South African market.
According to the 75 and 95% credible intervals, the leverage effect is present, as indicated by the significance of ˆγ3 and ˆγ4. The presence of the leverage effect in the South African market is in line with Mandimika and Chinzara (2012) and Jin (2017), but in contrast to Adu et al. (2015). However, the mean values of 0.070 and -0.113 are weak, in comparison to volatility feedback which has a mean value of 0.100 as shown by ˆα1. The weak presence of the leverage effect in the South African market is in line with Mangani (2008). It can be concluded that volatility is weakly persistent, resulting in volatility feedback being absent in the South African market. This result is in contrast to the international study by Jin (2017), who found the presence of volatility feedback in the South African market. As a result, this motivated further testing of the volatility feedback mechanism.
The values of ˆσ21 and ˆσ22 are both zero which indicates that there is no error variance and all the MC mixing values are an approximate value of 0.5, indicating optimal mixing of the parameters. Hence, this confirmed the robustness of the posterior parameter estimates, in line with Karabatsos (2017).
Following Jensen and Maheu (2018), in order to truly capture the volatility feedback mechanism, log realized variance is determined in relation to three levels of volatility which are low, average and high volatility. Since this study analyzed daily data, the three periods are daily low, average and high volatility. The three periods are defined as 10 May 2010, 24 February 2011 and 16 March 2012, which were calculated as the minimum, average and maximum log realized variance values, respectively. Drawn from theory, log realized is expected to get stronger over time in relation to the three levels of volatility (Harris et al. 2019). Table 3 shows the results of the parametric Bayesian model for volatility feedback.
Parameter Estimates | 75% Credible Interval | Mean | 95% Credible Interval | MC Mixing Value |
ˆβ0 | (−0.028, −0.012) | −0.020 | (−0.044, 0.004) | 0.484 |
ˆβlow | (−0.137, 0.127) | −0.005 | (−0.412, 0.381) | 0.489 |
ˆβavg | (−0.144, 0.142) | 0.001 | (−0.394, 0.410) | 0.497 |
ˆβhigh | (−0.140, 0.140) | −0.004 | (−0.409, 0.387) | 0.502 |
ˆσ2 | (0.000, 0.000) | 0.000 | (0.000, 0.000) | 0.506 |
From Table 3, for a low level of volatility, the mean value is negative and statistically significant. The mean value then increased and reflected a positive value for an average level of volatility. However, although the mean value is significant for a high level of volatility, it decreased from an average level of volatility, indicating a dissipation of volatility. This finding is in direct contrast to the expected magnitude of volatility feedback, where it is characterized by tendencies to get stronger over time, by Harris et al. (2019) and Jensen and Maheu (2018). Further, for the 75% credible interval, all the mean values are found to be statistically insignificant. Thus, from the overall results of the parametric Bayesian model, it can be concluded that volatility feedback is absent in the South African market.
For the nonparametric Bayesian model, volatility feedback is analyzed by graphical analysis and numerical analysis. In the context of this study, for the probability density functions (pdfs), the plots display the spread and probability of occurrence of the log realized variance in relation to the specified level of volatility. Graphically, the peak of the density represents the mean value since it is a measure of central tendency.
Figures 3, 4 and 5 are density estimations of log realized variance for low, average and high levels of log realized variance, respectively. All three figures showed a distinct form for each plot. Figure 3 shows the density estimation for a period of low volatility.
From Figure 3, the spread is relatively high and the peak of the pdf is the highest in comparison to the plots for average and high levels of volatility. Specifically, the peak of the pdf is approximately 900. Meaning, the probability of occurrence of log realized variance is exceptionally high within the market condition of low volatility. Thus, the South African market is subject to relatively low volatility conditions. This finding is in contrast to the high levels of volatility characterized by an emerging market, by Adu et al. (2015).
This finding is further in contrast to the studies that found persistent levels of volatility in the South African market by Mangani (2008), Mandimika and Chinzara (2012) and Jin (2017). As a result, suggesting the presence of a stronger form of volatility—volatility feedback. The period of low volatility is representative of unusually stable conditions that does not necessarily facilitate an investor to achieve a higher return from taking on a higher level of risk. However, the result of low volatility is in line with the recent South African study by Steyn and Theart (2019). Figure 4 showed the density estimation for a period of average volatility.
In direct contrast to Figure 3, Figure 4 reflects a density estimation that has a very constricted spread. The form of Figure 4 strongly shows a horizontal and uniform shape along the y-axis. This relatively flat form suggests that volatility in relation to an average level of volatility is linear. The positive uniform and linear shape can be interpreted as a reflection of the traditional risk-return relationship, where an investor only takes on a high level of risk if compensated by a high level of return. This finding is in contrast to recent South African studies that document a negative relationship (Bekiros et al., 2017; Jin, 2017; Steyn and Theart, 2019) of which one would consequently expect a nonlinear shape. On that note, if the earlier South African studies were taken into account, which found an insignificant relationship (Mangani, 2008; Mandimika and Chinzara, 2012; Adu et al., 2015), one would expect no particular shape or form for the density estimation.
The probability estimation for the average level of volatility 13, is drastically low in comparison to a low level of volatility 900 in Figure 3. The result for the average level of volatility is unexpected as one would expect a higher probability estimation within this period. This is because it resembles the conditions of a typical market that promotes investment activity. That is, where investors are being compensated for their investment ventures. Hence, the low probability estimation is in contrast to the expectation of recent studies by Kim and Kim (2018) and Harris et al. (2019). All of which found that investors were being compensated in their respective markets. Figure 5 shows the density estimation for a period of high volatility.
Figure 5 is the most unique density estimation as it reflects the asymmetric property of multiple modes of which a nonparametric Bayesian model can effectively capture, according to Jensen and Maheu (2018) and Karabatsos (2017). The spread is constricted in two areas which can be as a result of the high level of volatility; thus, causing a distinct dispersion in the spread of the data. The probability estimation for log realized variance in relation to the highest level of volatility has the lowest value 11. Meaning, the probability of log realized variance in relation to a high level of volatility has the lowest chance of occurring in the South African market.
Specifically, its value is approximately about eighty times smaller than the pdf of the low specified level of volatility of 900 from Figure 3. This means that the probability of occurrence of log realized variance within a period of high volatility is very small, smaller than that for the low volatility period. Thus, log realized variance is strongly dissipating with respect to an increase in the level of volatility over time. This finding is in contrast to the high levels of volatility characterized by an emerging market and the South African studies which found persistent levels of volatility. More importantly, this finding contrasts to the expected result of volatility feedback which is characterized by tendencies to get stronger over time and take longer to die out, by Harris et al. (2019) and Jensen and Maheu (2018). Table 4 shows the results of the nonparametric Bayesian model for volatility feedback.
Parameter Estimates | 75% Credible Interval | Mean | 95% Credible Interval | MC Mixing Value |
ˆdlow | (−0.093, 0.066) | −0.311 | (−24.503, 26.842) | 0.496 |
ˆdavg | (−0.144, 0.127) | 0.406 | (−28.389, 37.115) | 0.499 |
ˆdhigh | (−0.091, 0.064) | 4.480 | (−35.850, 28.768) | 0.487 |
From Table 4, the mean values of the density estimations, increased from a low to average to a high level of volatility. This finding is in line with the volatility feedback definition, by Harris et al. (2019), and expected result by Jensen and Maheu (2018). However, the density estimation of log realized variance in relation to the three levels of volatility are all insignificant for the 75% credible interval but significant for the 95% credible interval.
Overall, from the results of the preliminary test, parametric Bayesian model and nonparametric Bayesian model, it is concluded that volatility feedback is absent in the South African market. This finding is in contrast to a number of studies such as Jin (2017), Kim and Kim (2018), Jensen and Maheu (2018) and Harris et al. (2019). Since all the MC mixing values are an approximate value of 0.5, this indicates optimal mixing of the parameters. Hence, this confirmed the robustness of the posterior parameter estimates, in line with Karabatsos (2017).
One would expect volatility feedback to be strongly prevalent in emerging markets since such markets are characterized by high levels of volatility. In contrast, the study by Sultan (2018), found a weak presence of volatility feedback in the emerging Finnish market. This study follows the sample period of Sultan (2018), which did not account for the 2008 financial crisis. As a result, this choice could be the main reason for the absence of volatility feedback in the South African market, as the high levels of volatility would have subdued after the 2008 financial crisis. In this context, the result of absent volatility feedback is theoretically and empirically expected since this study analyzed the post 2008 financial crisis period. Additionally, the risk-return relationship of South Africa is unaffected by the 2008 financial crisis in the study by Jin (2017), in support of its exclusion in this study. Thus, it can be concluded that the volatility feedback mechanism has a negligible effect in the investigation of the risk-return relationship in the South African market.
In turn, this result did not significantly impact the rising or falling of market prices, by Mancino and Sanfelici (2020). Since volatility feedback does not affect the dynamics of the South African financial market, it has a lesser impact with respect to an investor's decision-making process and investment strategies. That is, in terms of identifying and capitalizing from arbitrage opportunities and mispricing's in the South African stock market.
Since there is no significant evidence of volatility feedback in the market, the risk-return relationship is analyzed free from empirical distortions that resulted from volatility feedback. If only the risk premium is captured, it should be positive and linear over time, as suggested by traditional theoretical expectations. Table 5 shows the results of the parametric Bayesian model for the risk-return relationship.
Parameter Estimates | 75% Credible Interval | Mean | 95% Credible Interval | MC Mixing Value |
ˆβ0 | (0.002, 0.002) | 0.002 | (0.001, 0.003) | 0.494 |
ˆβlog(rv) | (0.078, 0.117) | 0.098 | (0.040, 0.157) | 0.498 |
ˆσ2 | (0.000, 0.000) | 0.000 | (0.000, 0.000) | 0.494 |
From Table 5, for the 75% credible interval, the relationship between risk and return is significant. This result is supported by the 95% credible interval which indicated a positive and significant risk-return relationship. Since all the MC mixing values are an approximate value of 0.5, this indicates optimal mixing of the parameters, hence, robust results by Karabatsos (2017). Figure 6 is a plot that shows the mean (M) and 95% quantiles of excess returns as a function of log realized variance.
From Figure 6, a positive and linear relationship between risk and return is shown over time, in line with expectations from conventional theory by Markowitz (1952), and international literature by Kim and Kim (2018) and Harris et al. (2019). However, this result is in contrast to the majority of purely South African studies who found a negative (Steyn and Theart, 2019) or no relationship at all (Mangani, 2008; Mandimika and Chinzara, 2012; Adu et al., 2015). The implications of a positive risk-return relationship means that risk-taking investors can optimize their chance of earning a superior return in the South African market.
From a methodological perspective, it is noteworthy that the positive and linear test result of the risk-return relationship is from a parametric Bayesian model. Meaning, a parametric model covering a finite sample space, that is limited to accounting for every possible higher moment asymmetric form of the risk-return relationship. Therefore, a nonparametric Bayesian model would be more effective in capturing the risk-return relationship, as suggested by Karabatsos (2017) and Jensen and Maheu (2018).
From the nonparametric Bayesian model, the density of the risk-return relationship is plotted against the mean to essentially give the average form of the distribution. Figure 7 shows a plot of the density estimation for the risk-return relationship.
On the flat surface of Figure 7 encompassing the x and y-axes, a distinct nonlinear relationship between risk and return is exhibited by the pdf. This suggests that the risk-return relationship is nonlinear in the South African market. However, according to the pdf, the probability estimation of this occurrence is exceptionally low, specifically 0.000004 ≈ 0. Meaning, the risk-return relationship is insignificant as it has a zero probability, which translates to an event that has no chance of occurrence. The numerical result of the density estimation provides more insight to this finding. Table 6 shows the results of the risk-return relationship.
Parameter Estimates | 75% Credible Interval | Mean | 95% Credible Interval | MC Mixing Value |
ˆdrr | (−0.229, 0.215) | 3.540 | (−1.892, 1.371) | 0.510 |
From Table 6, according to the 75 and 95% credible interval, the risk-return relationship is insignificant in the South African market. Since all the MC mixing values are an approximate value of 0.5, this indicates optimal mixing of the parameters, hence, robust results. Thus, the final result is that there is no relationship between risk and return in the South African market, in support of the pdf plot in Figure 7.
Drawn from Umutlu (2019), often when a model has estimated a theoretically expected result, this suggests a robust modelling approach. This study showed such a case when a positive risk-return relationship was found by the parametric Bayesian model. However, a model designed to capture nonlinear and asymmetric properties is more likely to effectively capture these properties and estimate a nonlinear risk-return relationship, as suggested by Jin (2017), Karabatsos (2017) and Jensen and Maheu (2018) and Demirer et al. (2019). Specifically, a nonparametric approach that relaxes modelling assumptions, such as normality is considered more robust.
Since the nonparametric Bayesian approach is more robust than the parametric Bayesian approach, this study concluded that there is no risk-return relationship. Therefore, an investor does not earn a higher compensation in the form of return when taking on any level of risk in the South African market. This finding is in line with the early South African studies by Mangani (2008), Mandimika and Chinzara (2012) and Adu et al. (2015). Investors can include South Africa in their investment portfolio with higher risk countries in order to spread their risk and derive diversification benefits. In addition, risk averse investors can find a safe environment within the South African market and earn a return in accordance to their risk tolerance.
The aim of this study was to investigate the risk-return relationship, provided that volatility feedback was taken into account. It was found that recent studies that took into account volatility feedback found a positive risk-return relationship, in line with theoretical expectations, whereas South African literature found either a negative or no risk-return relationship. This was possibly due to the failure to account for volatility feedback or the model choice. Therefore, this study was the first to apply the novel and robust Bayesian approach in the South African market, to investigate the risk-return relationship and volatility feedback topic. This study modelled the Bayesian approach in a parametric and nonparametric framework for comparative purposes.
Both the parametric and nonparametric Bayesian model found that volatility feedback was absent in the South African market. This was contrary to expectations, especially since emerging markets are subject to high levels of volatility. The risk-return relationship was then analyzed free from empirical distortions that resulted from volatility feedback. From the parametric Bayesian model, the risk-return relationship was found to be positive and linear. This finding was in line with theoretical expectations and the international studies by Kim and Kim (2018) and Harris et al. (2019).
In contrast, the nonparametric Bayesian model revealed no relationship between risk and return. Given that the nonparametric Bayesian model is more robust, this study concluded that there is no risk-return relationship. The absence of volatility feedback and no risk-return relationship in the South African market was still a source of useful information. Although investors and arbitrageurs cannot capitalize on mispricing's, they can still be guided in terms of their investment strategies and decisions. An investor can include South Africa in their investment portfolio with higher risk countries in order to spread their risk and derive diversification benefits. In addition, risk averse investors can find a safe environment within the market of South Africa and earn a return in accordance to their risk tolerance.
This study has two recommendations for future research purposes. The use of high frequency data has become popular given the rise in high frequency trading. Thus, daily data provides a more precise estimate of variables (Jin, 2017). Further, the presence of volatility feedback is more pronounced when using daily data, according to Sultan (2018). Consequently, this may increase the magnitude of the volatility feedback mechanism and improve the estimation of the risk-return relationship, once it is taken into account. Therefore, the first recommendation is to use higher frequency data such as tick data, in line with Jensen and Maheu (2018), provided data availability and accessibility.
In this study, the absence of volatility feedback indicated the conditions of an economic recovery, which made sense, because the sample period analyzed was the post financial crisis period. Therefore, the second recommendation of this study is to analyze a sample period before, including and excluding the 2007/ 2008 financial crisis, because it can provide useful information to researchers and investors alike. First, to investigate if the result of volatility feedback lines up with theory drawn from Mancino and Sanfelici (2020), as it did in this study. Second, the different levels of volatility in each period are likely to affect risk estimation; thus, the empirical result of the risk-return relationship. Third, information on the magnitude volatility feedback alone or in relation to the risk-return relationship can assist an investor in strategizing their investment to improve their probability of realizing a superior return.
The secondary price dataset used in this study can be obtained from the IRESS database.
The author would like to thank Prof. John Maheu for his expert academic advice.
The author declares no conflicts of interest in this paper.
[1] | D. Angell, Irrationality and Transcendence in Number Theory, CRC Press, 2022. https://doi.org/10.1201/9781003111207 |
[2] | S. A. Morris, A. Jones, K. R. Pearson, Abstract and Famous Impossibilities, Springer Nature, 2022. https://doi.org/10.1007/978-3-031-05698-7 |
[3] | I. Niven, Irrational Numbers, Mathematical Association of America, 1967. https://doi.org/10.5948/9781614440116 |
[4] | Y. Bugeaud, Distribution Modulo one and Diophantine Approximation, Cambridge University Press, 2012. https://doi.org/10.1017/CBO9781139017732 |
[5] | K. Weihrauch, Computable Analysis: An Introduction, Springer Science & Business Media, 2000. https://dl.acm.org/doi/book/10.5555/2601642 |
[6] | A. Baker, Transcendental Number Theory, Cambridge University Press, 1975. https://doi.org/10.1017/CBO9780511565977 |
[7] | J. M. Borwein, P. B. Borwein, Pi and the AGM: A Study in Analytic Number Theory and Computational Complexity, Wiley–Blackwell, 1987. |
[8] | P. Walters, An Introduction to Ergodic Theory, Springer Verlag, 1982. |
[9] | R. L. Zimmer, Ergodic Theory and Semisimple Groups, Birkhäuser, 1984. https://link.springer.com/book/10.1007/978-1-4684-9488-4 |
[10] |
V. A. Androsenko, Irrationality measure of the number π√3, Izv. Math., 79 (2015), 1–17. https://doi.org/10.1070/IM2015v079n01ABEH002731 doi: 10.1070/IM2015v079n01ABEH002731
![]() |
[11] |
V. Becher, Y. Bugeaud, T. A. Slaman, The irrationality exponent of computable numbers, Proc. Am. Math. Soc., 144 (2016), 1509–1521. DOI: https://doi.org/10.1090/proc/12841 doi: 10.1090/proc/12841
![]() |
[12] |
V. Becher, J. Reimann, T. A. Slaman, Irrationality exponent, Hausdorff, dimension and effectivization, Monatsh. Math., 144 (2018), 167–188. https://doi.org/10.1007/s00605-017-1094-2 doi: 10.1007/s00605-017-1094-2
![]() |
[13] |
Y. Guo, W. Wu, Z. Wen, On the irrationality exponent of the regular paperfolding numbers, Linear Algebra Appl., 446 (2014), 237–264. https://doi.org/10.1016/j.laa.2013.12.023 doi: 10.1016/j.laa.2013.12.023
![]() |
[14] | V. Jarnik, Zur Metrischen theorie der diophantischen approximation, Prace Matematyczno-Fizyczne, 36 (1929), 91–106. https://bibliotekanauki.pl/articles/2011815 |
[15] |
A. Polyanskii, On the irrationality measures of certain numbers, Moscow J. Comb. Number Theory, 1 (2011), 81–91. https://doi.org/10.1134/S0001434618030306 doi: 10.1134/S0001434618030306
![]() |
[16] |
A. Polyanskii, On the irrationality measures of certain numbers II, Math. Notes, 103 (2018), 626–634. https://doi.org/10.1134/S0001434618030306 doi: 10.1134/S0001434618030306
![]() |
[17] | J. Beck, Inevitable Randomness in Discrete Mathematics, American Mathematical Society, 2009. |
[18] | G. Hardy, E. M. Wright, An Introduction to The Theory of Numbers, Oxford University Press, 1954. https://doi.org/10.1093/oso/9780199219858.001.0001 |
[19] | M. Kac, Statistical Independence in Probability, Analysis and Number Theory, Mathematical Association of America, 1964. : https://doi.org/10.5948/UPO9781614440123 |
[20] |
G. Martin, Absolutely abnormal numbers, Am. Math. Mon., 108 (2001), 746–754. https://doi.org/DOI:10.2307/2695618 doi: 10.2307/2695618
![]() |
[21] |
R. V. Nillsen, Normal numbers without measure theory, Am. Math. Mon., 107 (2000), 639–644. https://doi.org/10.2307/2589120 doi: 10.2307/2589120
![]() |
[22] | D. D. Wall, On Normal Numbers, Ph.D thesis, University of California, Berkeley, 1949. |
[23] |
E. Borel, Les probabilit'es dénombrables et leurs applications arithmétiques, Rend. Circ. Mat. Palermo, 27 (1909), 247–271. https://doi.org/10.1007/BF03019651 doi: 10.1007/BF03019651
![]() |
[24] |
P. Szüsz, B. Volkmann, On numbers with given digit distributions, Arch. Math., 52 (1989), 237–-244. https://doi.org/10.1007/BF01194386 doi: 10.1007/BF01194386
![]() |
[25] | P. Erdős, Representations of real numbers as sums and products of Liouville numbers, Michigan Math. J., 446 (1962), 59–60. |
[26] |
K. Mahler, Zur approximation der exponential function un des logarithmus I, J. Reine Angew. Math., 166 (1932), 118–136. https://doi.org/10.1515/crll.1932.166.118 doi: 10.1515/crll.1932.166.118
![]() |
[27] |
K. Mahler, On the approximation of logarithms of algebraic numbers, Philos. Trans. R. Soc. London Ser. A, 245 (1953), 371–398. https://doi.org/10.1098/rsta.1953.0001 doi: 10.1098/rsta.1953.0001
![]() |
[28] | K. Mahler, On the approximation of π, in Pi: A Source Book, Springer, New York, 56 (1953), 30–42. https://doi.org/10.1007/978-1-4757-4217-6 |
[29] |
D. Kleinbock, T. Ly, Badly approximable S-numbers and absolute Schmidt games, J. Number Theory, 164 (2016), 13–41. https://doi.org/10.1016/j.jnt.2015.12.014 doi: 10.1016/j.jnt.2015.12.014
![]() |
1. | Meng Pang, Zhe Li, A novel profit-based validity index approach for feature selection in credit risk prediction, 2024, 9, 2473-6988, 974, 10.3934/math.2024049 | |
2. | Li Peng, Yujie Yang, Cheng Yang, Zejun Li, Ngai Cheong, HRGCNLDA: Forecasting of lncRNA-disease association based on hierarchical refinement graph convolutional neural network, 2024, 21, 1551-0018, 4814, 10.3934/mbe.2024212 |
Variables | Mean | Variance | Kurtosis | Skewness | Minimum | Maximum |
rt | 0.0001 | 0.0001 | 1.277 | −0.167 | −0.037 | 0.042 |
r2t | 0.118 | 0.004 | −1.131 | −0.043 | 0.000 | 0.227 |
RVt | 0.981 | 0.000 | 3.185 | −1.567 | 0.946 | 0.988 |
log(RVt) | −0.019 | 0.000 | 3.323 | −1.593 | −0.056 | −0.012 |
rt/√RVt | 0.000 | 0.000 | 1.350 | −0.168 | −0.038 | 0.043 |
Parameter Estimates | 75% Credible Interval | Mean | 95% Credible Interval | MC Mixing Value |
ˆα0 | (−0.118, −0.077) | −0.098 | (−0.156, −0.037) | 0.499 |
ˆα1 | (0.078, 0.121) | 0.100 | (0.038, 0.159) | 0.499 |
ˆσ21 | (0.000, 0.000) | 0.000 | (0.000, 0.000) | 0.492 |
ˆγ0 | (−0.019, −0.018) | −0.019 | (−0.019, −0.018) | 0.518 |
ˆγ1 | (−0.000, 0.000) | 0.000 | (−0.000, 0.000) | 0.512 |
ˆγ2 | (−0.096, 0.186) | 0.047 | (−0.370, 0.460) | 0.523 |
ˆγ3 | (0.062, 0.080) | 0.070 | (0.044, 0.098) | 0.490 |
ˆγ4 | (−0.126, −0.099) | −0.113 | (−0.152, −0.073) | 0.499 |
ˆσ22 | (0.000, 0.000) | 0.000 | (0.000, 0.000) | 0.500 |
Parameter Estimates | 75% Credible Interval | Mean | 95% Credible Interval | MC Mixing Value |
ˆβ0 | (−0.028, −0.012) | −0.020 | (−0.044, 0.004) | 0.484 |
ˆβlow | (−0.137, 0.127) | −0.005 | (−0.412, 0.381) | 0.489 |
ˆβavg | (−0.144, 0.142) | 0.001 | (−0.394, 0.410) | 0.497 |
ˆβhigh | (−0.140, 0.140) | −0.004 | (−0.409, 0.387) | 0.502 |
ˆσ2 | (0.000, 0.000) | 0.000 | (0.000, 0.000) | 0.506 |
Parameter Estimates | 75% Credible Interval | Mean | 95% Credible Interval | MC Mixing Value |
ˆdlow | (−0.093, 0.066) | −0.311 | (−24.503, 26.842) | 0.496 |
ˆdavg | (−0.144, 0.127) | 0.406 | (−28.389, 37.115) | 0.499 |
ˆdhigh | (−0.091, 0.064) | 4.480 | (−35.850, 28.768) | 0.487 |
Parameter Estimates | 75% Credible Interval | Mean | 95% Credible Interval | MC Mixing Value |
ˆβ0 | (0.002, 0.002) | 0.002 | (0.001, 0.003) | 0.494 |
ˆβlog(rv) | (0.078, 0.117) | 0.098 | (0.040, 0.157) | 0.498 |
ˆσ2 | (0.000, 0.000) | 0.000 | (0.000, 0.000) | 0.494 |
Parameter Estimates | 75% Credible Interval | Mean | 95% Credible Interval | MC Mixing Value |
ˆdrr | (−0.229, 0.215) | 3.540 | (−1.892, 1.371) | 0.510 |
Variables | Mean | Variance | Kurtosis | Skewness | Minimum | Maximum |
rt | 0.0001 | 0.0001 | 1.277 | −0.167 | −0.037 | 0.042 |
r2t | 0.118 | 0.004 | −1.131 | −0.043 | 0.000 | 0.227 |
RVt | 0.981 | 0.000 | 3.185 | −1.567 | 0.946 | 0.988 |
log(RVt) | −0.019 | 0.000 | 3.323 | −1.593 | −0.056 | −0.012 |
rt/√RVt | 0.000 | 0.000 | 1.350 | −0.168 | −0.038 | 0.043 |
Parameter Estimates | 75% Credible Interval | Mean | 95% Credible Interval | MC Mixing Value |
ˆα0 | (−0.118, −0.077) | −0.098 | (−0.156, −0.037) | 0.499 |
ˆα1 | (0.078, 0.121) | 0.100 | (0.038, 0.159) | 0.499 |
ˆσ21 | (0.000, 0.000) | 0.000 | (0.000, 0.000) | 0.492 |
ˆγ0 | (−0.019, −0.018) | −0.019 | (−0.019, −0.018) | 0.518 |
ˆγ1 | (−0.000, 0.000) | 0.000 | (−0.000, 0.000) | 0.512 |
ˆγ2 | (−0.096, 0.186) | 0.047 | (−0.370, 0.460) | 0.523 |
ˆγ3 | (0.062, 0.080) | 0.070 | (0.044, 0.098) | 0.490 |
ˆγ4 | (−0.126, −0.099) | −0.113 | (−0.152, −0.073) | 0.499 |
ˆσ22 | (0.000, 0.000) | 0.000 | (0.000, 0.000) | 0.500 |
Parameter Estimates | 75% Credible Interval | Mean | 95% Credible Interval | MC Mixing Value |
ˆβ0 | (−0.028, −0.012) | −0.020 | (−0.044, 0.004) | 0.484 |
ˆβlow | (−0.137, 0.127) | −0.005 | (−0.412, 0.381) | 0.489 |
ˆβavg | (−0.144, 0.142) | 0.001 | (−0.394, 0.410) | 0.497 |
ˆβhigh | (−0.140, 0.140) | −0.004 | (−0.409, 0.387) | 0.502 |
ˆσ2 | (0.000, 0.000) | 0.000 | (0.000, 0.000) | 0.506 |
Parameter Estimates | 75% Credible Interval | Mean | 95% Credible Interval | MC Mixing Value |
ˆdlow | (−0.093, 0.066) | −0.311 | (−24.503, 26.842) | 0.496 |
ˆdavg | (−0.144, 0.127) | 0.406 | (−28.389, 37.115) | 0.499 |
ˆdhigh | (−0.091, 0.064) | 4.480 | (−35.850, 28.768) | 0.487 |
Parameter Estimates | 75% Credible Interval | Mean | 95% Credible Interval | MC Mixing Value |
ˆβ0 | (0.002, 0.002) | 0.002 | (0.001, 0.003) | 0.494 |
ˆβlog(rv) | (0.078, 0.117) | 0.098 | (0.040, 0.157) | 0.498 |
ˆσ2 | (0.000, 0.000) | 0.000 | (0.000, 0.000) | 0.494 |
Parameter Estimates | 75% Credible Interval | Mean | 95% Credible Interval | MC Mixing Value |
ˆdrr | (−0.229, 0.215) | 3.540 | (−1.892, 1.371) | 0.510 |