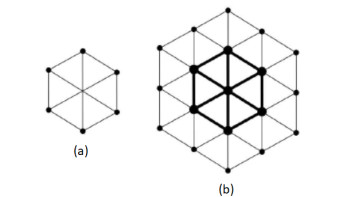
Current facial image manipulation techniques have caused public concerns while achieving impressive quality. However, these techniques are mostly bound to a single frame for synthesized videos and pay little attention to the most discriminatory temporal frequency artifacts between various frames. Detecting deepfake videos using temporal modeling still poses a challenge. To address this issue, we present a novel deepfake video detection framework in this paper that consists of two levels: temporal modeling and coherence analysis. At the first level, to fully capture temporal coherence over the entire video, we devise an efficient temporal facial pattern (TFP) mechanism that explores the color variations of forgery-sensitive facial areas by providing global and local-successive temporal views. The second level presents a temporal coherence analyzing network (TCAN), which consists of novel global temporal self-attention characteristics, high-resolution fine and low-resolution coarse feature extraction, and aggregation mechanisms, with the aims of long-range relationship modeling from a local-successive temporal perspective within a TFP and capturing the vital dynamic incoherence for robust detection. Thorough experiments on large-scale datasets, including FaceForensics++, DeepFakeDetection, DeepFake Detection Challenge, CelebDF-V2, and DeeperForensics, reveal that our paradigm surpasses current approaches and stays effective when detecting unseen sorts of deepfake videos.
Citation: Muhammad Ahmad Amin, Yongjian Hu, Jiankun Hu. Analyzing temporal coherence for deepfake video detection[J]. Electronic Research Archive, 2024, 32(4): 2621-2641. doi: 10.3934/era.2024119
[1] | Usman Babar, Haidar Ali, Shahid Hussain Arshad, Umber Sheikh . Multiplicative topological properties of graphs derived from honeycomb structure. AIMS Mathematics, 2020, 5(2): 1562-1587. doi: 10.3934/math.2020107 |
[2] | Ali Al Khabyah . Mathematical aspects and topological properties of two chemical networks. AIMS Mathematics, 2023, 8(2): 4666-4681. doi: 10.3934/math.2023230 |
[3] | R. Aguilar-Sánchez, J. A. Mendez-Bermudez, José M. Rodríguez, José M. Sigarreta . Multiplicative topological indices: Analytical properties and application to random networks. AIMS Mathematics, 2024, 9(2): 3646-3670. doi: 10.3934/math.2024179 |
[4] | Fei Yu, Hifza Iqbal, Saira Munir, Jia Bao Liu . M-polynomial and topological indices of some transformed networks. AIMS Mathematics, 2021, 6(12): 13887-13906. doi: 10.3934/math.2021804 |
[5] | Ali Al Khabyah, Haseeb Ahmad, Ali Ahmad, Ali N. A. Koam . A uniform interval-valued intuitionistic fuzzy environment: topological descriptors and their application in neural networks. AIMS Mathematics, 2024, 9(10): 28792-28812. doi: 10.3934/math.20241397 |
[6] | Sumiya Nasir, Nadeem ul Hassan Awan, Fozia Bashir Farooq, Saima Parveen . Topological indices of novel drugs used in blood cancer treatment and its QSPR modeling. AIMS Mathematics, 2022, 7(7): 11829-11850. doi: 10.3934/math.2022660 |
[7] | Fozia Bashir Farooq . Implementation of multi-criteria decision making for the ranking of drugs used to treat bone-cancer. AIMS Mathematics, 2024, 9(6): 15119-15131. doi: 10.3934/math.2024733 |
[8] | Jung-Chao Ban, Chih-Hung Chang . Entropy dimension of shifts of finite type on free groups. AIMS Mathematics, 2020, 5(5): 5121-5139. doi: 10.3934/math.2020329 |
[9] | Ali N. A. Koam, Ali Ahmad, Azeem Haider, Moin A. Ansari . Computation of eccentric topological indices of zero-divisor graphs based on their edges. AIMS Mathematics, 2022, 7(7): 11509-11518. doi: 10.3934/math.2022641 |
[10] | Yanjie Wang, Beibei Zhang, Bo Cao . On the number of zeros of Abelian integrals for a kind of quadratic reversible centers. AIMS Mathematics, 2023, 8(10): 23756-23770. doi: 10.3934/math.20231209 |
Current facial image manipulation techniques have caused public concerns while achieving impressive quality. However, these techniques are mostly bound to a single frame for synthesized videos and pay little attention to the most discriminatory temporal frequency artifacts between various frames. Detecting deepfake videos using temporal modeling still poses a challenge. To address this issue, we present a novel deepfake video detection framework in this paper that consists of two levels: temporal modeling and coherence analysis. At the first level, to fully capture temporal coherence over the entire video, we devise an efficient temporal facial pattern (TFP) mechanism that explores the color variations of forgery-sensitive facial areas by providing global and local-successive temporal views. The second level presents a temporal coherence analyzing network (TCAN), which consists of novel global temporal self-attention characteristics, high-resolution fine and low-resolution coarse feature extraction, and aggregation mechanisms, with the aims of long-range relationship modeling from a local-successive temporal perspective within a TFP and capturing the vital dynamic incoherence for robust detection. Thorough experiments on large-scale datasets, including FaceForensics++, DeepFakeDetection, DeepFake Detection Challenge, CelebDF-V2, and DeeperForensics, reveal that our paradigm surpasses current approaches and stays effective when detecting unseen sorts of deepfake videos.
Graph theory has provided the researcher with various useful tools, such as graph labeling, locating numbers and topological indices. Graph theory subject has many applications and implementations in different research subjects like chemistry, medicine and engineering. A graph can be recognized by a numeric value, a polynomial, a sequence of numbers or a matrix. The representation of the chemical compound in terms of diagram, known as its molecular graph, in which its atoms and the chemical bonding between them represent the nodes and edges, respectively. Recently, a new subject caught attention of the researchers was introduced, which is the combination of chemistry, information science and mathematics is called Cheminformatics, which studies QSAR/QSPR relationship, bioactivity and characterization of chemical compounds [1].
The topological index is a numeric value related with chemical compositions maintaining the correlation of chemical structures with many physico-chemical properties, chemical reactivity or biological activity. Topological indices are prepared on the grounds of the transformation of a chemical network into a number that describes the topology of the chemical network. Some of the main types of topological indices of graphs are distance-based topological indices, degree-based topological indices, and counting-related topological indices. Recently, numerous researchers have found topological indices for the study of fundamental properties of molecular graph or network. These networks have very motivating topological properties which have been considered in different characteristics in [2,3,4,5,6,7,8,9].
Let G=(V,E) be a simple connected graph, with V be the vertex set and E be the edge set of graph G, with order |V|=p, size |E|=q. The number of edges incident with a vertex ω is known as the degree of ω, denoted by ζ(ω). The reverse vertex degree (R(ω)) was introduced by Kulli [10] defined as: R(ω)=1−ζ(ω)+Δ, where Δ denoted the maximum degree of the given graph. Let ER(ω),R(μ) represents the edge partition of the given graph based on reverse degree of end vertices of an edge ωμ∈E and |ER(ω),R(μ)| represents its cardinality. There are detailed variations of topological indices mainly distance-based and degree-based indices, see [11,12,13,14,15,16]. Milan Randic [30] was the first who defined the degree-based indices and its reverse Randic index is defined as:
RRα(G)=∑ωμ∈E(G)(R(ω)×R(μ))α,α=12,−12,1,−1. | (1.1) |
Estrada et al. presented the atom bond connectivity (ABC) index in [18] and the reverse atom bond connectivity (RABC) is defined as:
RABC(G)=∑ωμ∈E(G)√R(ω)+R(μ)−2R(ω)×R(μ) | (1.2) |
Vukicevic and Furtula defined the geometric arithmetic (GA) index in [19] and the reverse geometric arithmetic (RGA) is presented as:
RGA(G)=∑ωμ∈E(G)2√R(ω)×R(μ)R(ω)+R(μ) | (1.3) |
Gutman et al. [20,21] defined the first and second Zagreb and its reverse indices as:
RM1(G)=∑ωμ∈E(G)(R(ω)+R(μ)) | (1.4) |
RM2(G)=∑ωμ∈E(G)(R(ω)×R(μ)) | (1.5) |
Shirdel et al. [22] introduced hyper Zagreb index. We defined the reverse hyper Zagreb index as:
RHM(G)=∑ωμ∈E(G)(R(ω)+R(μ))2 | (1.6) |
Furtula and Gutman [23] accomplished the forgotten index and its reverse forgotten index as:
RF(G)=∑ωμ∈E(G)((R(ω))2+(R(μ))2) | (1.7) |
Augmented Zagreb index was introduced by Furtula et al. [24] and the reverse augmented Zagreb index as:
RAZI(G)=∑ωμ∈E(G)(R(ω)×R(μ)R(ω)+R(μ)−2)3 | (1.8) |
Ranjini et al. [25] introduced the first redefined, second redefined and third redefined Zagreb indices. The reverse first redefined, second redefined and third redefined Zagreb indices are defined as:
RReZ1(G)=∑ωμ∈E(G)R(ω)+R(μ)R(ω)×R(μ) | (1.9) |
RReZ2(G)=∑ωμ∈E(G)R(ω)×R(μ)R(ω)+R(μ) | (1.10) |
RReZ3(G)=∑ωμ∈E(G)(R(ω)+R(μ))(R(ω)×R(μ)) | (1.11) |
For latest results on topological indices see [26,27,28,29,31,32,33,34,35]. In this paper, we compute the exact results for all the above reverse indices.
With the help of complete graphs of order 3 (K3), Chen et al. [36] assembled a hexagonal mesh. In terms of chemistry, these K3 graphs are also called oxide graphs. The Figure 1 is obtained by joining these K3 graphs. Two dimensional mesh graph HX(2) (see Figure 1 (a)), is obtained by joining six K3 graphs and three dimensional mesh graph HX(3) (see Figure 1 (b)) is obtained by putting K3 graphs around all side of HX(2). Furthermore, repeating the same process by putting the t K3 graph around each hexagon, we obtained the tth hexagonal mesh. To be noted that the one dimensional hexagonal mesh graph does not exist.
Simonraj et al. [37] created the new network which is named as third type of hex-derived networks. The graphically construction algorithm for third type of hexagonal hex-derived network HHDN3(t) (see Figure 2), triangular hex-derived network THDN3(t) (see Figure 3) and rectangular hex-derived network RHDN3(t) (see Figure 4) are defined in [38,39] and they determined some topological indices of these new derived networks. Some networks such as hexagonal, honeycomb, and grid networks, for instance, endure closeness to atomic or molecular lattice configurations. Related research that applies this theory and which could get additional advantages from the visions of the new research is found in [40,41,42,43,44,45,46].
Let Γ1=HHDN3(t) be the third type of hexagonal hex-derived network which is shown in Figure 2, where t≥4. The graph Γ1 has 21t2−39t+19 vertices from which 18t2−36t+18 vertices of reverse degree 15, 4 vertices of reverse degree 12, 6t−12 vertices of reverse degree 9 and 3t2−9t+9 vertices of reverse degree 1. There are 63t2−123t+60 number of edges of Γ1 is partitioned into nine classes based on their reverse degrees which are given in Eq (3.1).
|ER(ω),R(μ)(Γ1)|={9t2−33t+30,for; R(ω)=1,R(μ)=112t−24,for; R(ω)=9,R(μ)=16t−18,for; R(ω)=9,R(μ)=96,for; R(ω)=12,R(μ)=112,for; R(ω)=12,R(μ)=936t2−108t+84,for; R(ω)=15,R(μ)=136t−72,for; R(ω)=15,R(μ)=924,for; R(ω)=15,R(μ)=1218t2−36t+18,for; R(ω)=15,R(μ)=15 | (3.1) |
In the next theorem, we will calculate the reverse Randic index, reverse Zagreb indices, reverse forgotten index for Γ1 graph.
Theorem 3.1. Let Γ1 be the third type of hexagonal hex-derived network, then
● RRα(Γ1)=[9+36(15)α+18(225)α]t2+[−33+12(9)α+6(81)α−108(15)α+36(135)α−36(225)α]t+30−24(9)α−18(81)α+6(12)α+12(108)α+84(15)α−72(135)α+24(180)α+18(225)α
● RM1(Γ1)=1134t2−1782t+630
● RM2(Γ1)=4599t2−4299t−366
● RHM(Γ1)=25452t2−36300t+11922
● RF(Γ1)=16254t2−27702t+12654
Proof. Let Γ1 be the third type of hexagonal hex-derived network which is shown in Figure 2. The order of hexagonal hex derived network Γ1 is p=|Γ1|=21t2−39t+19 and size is q=63t2−123t+60. The edge partitioned of Γ1 based on their reverse degrees are shown in Eq (3.1). Reverse Randic index can be calculated by using Eq (3.1). Thus, from Eq (1.1), it follows,
RRα(Γ1)=(1)α|E1,1(Γ1)|+(9)α|E9,1(Γ1)|+(81)α|E9,9(Γ1)|+(12)α|E12,1(Γ1)|+(108)α|E12,9(Γ1)|+(15)α|E15,1(Γ1)|+(135)α|E15,9(Γ1)|+(180)α|E15,12(Γ1)|+(225)α|E15,15(Γ1)|.
=(9t2−33t+30)+(9)α(12t−24)+(81)α(6t−18)+(12)α(6)+(108)α(12)+(15)α(36t2−108t+84)+(135)α(36t−72)+(180)α(24)+(225)α(18t2−36t+18).
=[9+36(15)α+18(225)α]t2+[−33+12(9)α+6(81)α−108(15)α+36(135)α−36(225)α]t+30−24(9)α−18(81)α+6(12)α+12(108)α+84(15)α−72(135)α+24(180)α+18(225)α. Put α=1 and after some calculation, we get reverse second Zagreb index RM2(Γ1) as:
RM2(Γ1)=4599t2−4299t−366. | (3.2) |
Using the Eq (1.4), we can determine the reverse first Zagreb index RM1(Γ1) as:
RM1(Γ1)=2×|E1,1(Γ1)|+10×|E9,1(Γ1)|+18×|E9,9(Γ1)|+13×|E12,1(Γ1)|+21×|E12,9(Γ1)|+16×|E15,1(Γ1)|+24×|E15,9(Γ1)|+27×|E15,12(Γ1)|+30×|E15,15(Γ1)|.
By putting the values of from equation (3.1) and after simplification, we obtain:
RM1(Γ1)=1134t2−1782t+630. | (3.3) |
Using the Eq (1.6), we can determine the reverse hyper Zagreb index RHM(Γ1) as:
RHM(Γ1)=4×|E1,1(Γ1)|+100×|E9,1(Γ1)|+324×|E9,9(Γ1)|+169×|E12,1(Γ1)|+441×|E12,9(Γ1)|+256×|E15,1(Γ1)|+576×|E15,9(Γ1)|+729×|E15,12(Γ1)|+900×|E15,15(Γ1)|.
After simplification, we get
RHM(Γ1)=25452t2−36300t+11922 |
Using the Eq (1.7), we can determine the reverse forgotten index RF(Γ1)) as:
RF(Γ1))=2×|E1,1(Γ1)|+82×|E9,1(Γ1)|+162×|E9,9(Γ1)|+145×|E12,1(Γ1)|+225×|E12,9(Γ1)|+226×|E15,1(Γ1)|+306×|E15,9(Γ1)|+369×|E15,12(Γ1)|+450×|E15,15(Γ1)|.
After simplification, we get
RF(Γ1)=16254t2−27702t+12654 |
In the next theorem, we will calculate the reverse atom bond connectivity index, reverse geometric arithmetic index for Γ1 graph.
Theorem 3.2. Let Γ1 be the third type of hexagonal hex-derived network, then
● RABC(Γ1)=(12√2105+12√75)t2+(8√2+83−36√2105+4√3305−24√75)t−8−16√2+√33+2√573+28√2105−8√3305+4√5+12√75
● RGA(Γ1)=(27+9√152)t2+(−2795−9√152)t+785+792√391−15√152+32√53.
Proof. The reverse atom bond connectivity (RABC(Γ1)), can be determined by using Eq (1.2) and Eq (3.1), as follows:
RABC(Γ1)=0×|E1,1(Γ1)|+√89×|E9,1(Γ1)|+√1681×|E9,9(Γ1)|+√1112×|E12,1(Γ1)|+√19108×|E12,9(Γ1)|+√1415×|E15,1(Γ1)|+√22135×|E15,9(Γ1)|+√25180×|E15,12(Γ1)|+√28225×|E15,15(Γ1)|.
After some simplification, we get
RABC(Γ1)=(12√2105+12√75)t2+(8√2+83−36√2105+4√3305−24√75)t−8−16√2+√33+2√573+28√2105−8√3305+4√5+12√75.
The reverse geometric arithmetic (RGA(Γ1)), can be determined by using Eq (1.3) and Eq (3.1), as follows:
RGA(Γ1)=|E1,1(Γ1)|+2√910×|E9,1(Γ1)|+2√8118×|E9,9(Γ1)|+2√1213×|E12,1(Γ1)|+2√10821×|E12,9(Γ1)|+2√1516×|E15,1(Γ1)|+2√13524×|E15,9(Γ1)|+2√18027×|E15,12(Γ1)|+2√22530×|E15,15(Γ1)|.
After some simplification, we get
RGA(Γ1)=(27+9√152)t2+(−2795−9√152)t+785+792√391−15√152+32√53. In the next theorems, we will calculate the reverse redefined Zagreb indices for Γ1 graph.
Theorem 3.3. Let Γ1 be the third type of hexagonal hex-derived network, then
● RReZ1(Γ1)=294t25−2474t15+362930
● RReZ2(Γ1)=693t24−2949t20−939071820
● RReZ3(Γ1)=130158t2−142518t+24828
Proof. Reverse redefined Zagreb indices can be calculated by using Eq (3.1), the RReZ1(Γ1) by using Eq (1.9) as follows:
RReZ1(Γ1)=2×|E1,1(Γ1)|+109×|E9,1(Γ1)|+1881×|E9,9(Γ1)|+1312×|E12,1(Γ1)|+21108×|E12,9(Γ1)|+1615×|E15,1(Γ1)|+24135×|E15,9(Γ1)|+27108×|E15,12(Γ1)|+30225×|E15,15(Γ1)|.
After some simplification, we get
RReZ1(Γ1)=294t25−2474t15+362930. |
The RReZ2(Γ1) can be determined by using Eq (1.10) as follows:
RReZ2(Γ1)=12×|E1,1(Γ1)|+910×|E9,1(Γ1)|+8118×|E9,9(Γ1)|+1213×|E12,1(Γ1)|+10821×|E12,9(Γ1)|+1516×|E15,1(Γ1)|+13524×|E15,9(Γ1)|+10827×|E15,12(Γ1)|+22530×|E15,15(Γ1)|.
After some simplification, we get
RReZ2(Γ1)=693t24−2949t20−939071820. |
The RReZ3(Γ1) can be calculated by using Eq (1.11) as follows:
RReZ3(Γ1)=2×|E1,1(Γ1)|+90×|E9,1(Γ1)|+1458×|E9,9(Γ1)|+156×|E12,1(Γ1)|+2268×|E12,9(Γ1)|+240×|E15,1(Γ1)|+3240×|E15,9(Γ1)|+2916×|E15,12(Γ1)|+6750×|E15,15(Γ1)|.
After some simplification, we get
RReZ3(Γ1)=130158t2−142518t+24828. |
Let Γ2=THDN3(t) be the third type of triangular hex-derived network which is shown in Figure 3, where t≥4. The graph Γ2 has 7t2−11t+62 vertices. There are 21t2−39t+182 number of edges of Γ2 is partitioned into six classes based on their reverse degrees which are given in Eq (4.1). Now we calculated reverse degree based indices such as: reverse Randic index RRα, reverse atom bond connectivity index RABC, reverse geometric arithmetic index RGA, first reverse Zagreb index RM1, second reverse Zagreb index RM2, reverse forgotten index RF, reverse hyper Zagreb index RHM and reverse redefined Zagreb indices for Γ2 graph.
|ER(ω),R(μ)(Γ2)|={3t22−21t2+18,for; R(ω)=1,R(μ)=16t−18,for; R(ω)=9,R(μ)=13t−6,for; R(ω)=9,R(μ)=96t2−30t+36,for; R(ω)=15,R(μ)=118t−30,for; R(ω)=15,R(μ)=93t2−6t+9,for; R(ω)=15,R(μ)=15 | (4.1) |
In the next theorem, we will calculate the reverse Randic index, reverse Zagreb indices, reverse forgotten index for Γ2 graph.
Theorem 4.1. Let Γ2 be the third type of triangular hex-derived network, then
● RRα(Γ2)=(32+6(15)α+3(225)α)t2+(−212+6(9)α+3(81)α−30(15)α+18(135)α−6(225)α)t+18−18(9)α−6(81)α+36(15)α−30(135)α+9(225)α.
● RM1(Γ2)=189t2−135t−126
● RM2(Γ2)=1533t22+1833t2−2115
● RHM(Γ2)=4242t2−1182t−3636
● RF(Γ2)=2709t2−3015t+594
Proof. Let Γ2 be the third type of triangular hex-derived network which is shown in Figure 3. The order of triangular hex derived network Γ2 is p=|Γ2|=7t2−11t+62 and size is q=21t2−39t+182. The edge partitioned of Γ2 based on their reverse degrees are shown in Eq (4.1). Reverse Randic index can be calculated by using Eq (4.1). Thus, from Eq (1.1), it follows,
RRα(Γ2)=(1)α|E1,1(Γ2)|+(9)α|E9,1(Γ2)|+(81)α|E9,9(Γ2)|+(15)α|E15,1(Γ2)|+(135)α|E15,9(Γ2)|+(225)α|E15,15(Γ2)|.
After simplification, we get
RRα(Γ2)=(32+6(15)α+3(225)α)t2+(−212+6(9)α+3(81)α−30(15)α+18(135)α−6(225)α)t+18−18(9)α−6(81)α+36(15)α−30(135)α+9(225)α.
Put α=1 and after some calculation, we get reverse second Zagreb index RM2(Γ2) as:
RM2(Γ2)=1533t22+1833t2−2115 | (4.2) |
Using the Eq (1.4), we can determine the reverse first Zagreb index RM1(Γ2) as:
RM1(Γ2)=2×|E1,1(Γ1)|+10×|E9,1(Γ1)|+18×|E9,9(Γ1)|+16×|E15,1(Γ1)|+24×|E15,9(Γ1)|+30×|E15,15(Γ1)|.
By putting the values of from Eq (4.1) and after simplification, we obtain:
RM1(Γ2)=189t2−135t−126 | (4.3) |
Using the Eq (1.6), we can determine the reverse hyper Zagreb index RHM(Γ2) as:
RHM(Γ2)=4×|E1,1(Γ2)|+100×|E9,1(Γ2)|+324×|E9,9(Γ2)|+256×|E15,1(Γ2)|+576×|E15,9(Γ2)|+900×|E15,15(Γ2)|.
After simplification, we get
RHM(Γ2)=4242t2−1182t−3636. |
Using the Eq (1.7), we can determine the reverse forgotten index RF(Γ2)) as:
RF(Γ2))=2×|E1,1(Γ2)|+82×|E9,1(Γ2)|+162×|E9,9(Γ2)|+226×|E15,1(Γ2)|+306×|E15,9(Γ2)|+450×|E15,15(Γ2)|.
After simplification, we get
RF(Γ2)=2709t2−3015t+594. |
In the next theorem, we will calculate the reverse atom bond connectivity index, reverse geometric arithmetic index for Γ2 graph.
Theorem 4.2. Let Γ2 be the third type of triangular hex-derived network, then
● RABC(Γ2)=(2√2105+2√75)t2+(4√2+43−2√210+2√3305−4√75)t−83−12√2+12√2105−2√3303+6√75
● RGA(Γ2)=(92+3√154)t2+(−9910+3√154)t+515−3√15.
Proof. The reverse atom bond connectivity (RABC(Γ2)), can be determined by using Eq (1.2) and Eq (4.1), as follows:
RABC(Γ2)=0×|E1,1(Γ2)|+√89×|E9,1(Γ2)|+√1681×|E9,9(Γ2)|+√1415×|E15,1(Γ2)|+√22135×|E15,9(Γ2)|+√28225×|E15,15(Γ2)|.
After some simplification, we get
RABC(Γ2)=(2√2105+2√75)t2+(4√2+43−2√210+2√3305−4√75)t−83−12√2+12√2105−2√3303+6√75.
The reverse geometric arithmetic (RGA(Γ2)), can be determined by using Eq (1.3) and Eq (4.1), as follows:
RGA(Γ2)=|E1,1(Γ2)|+2√910×|E9,1(Γ2)|+2√8118×|E9,9(Γ2)|+2√1516×|E15,1(Γ2)|+2√13524×|E15,9(Γ2)|+2√22530×|E15,15(Γ2)|.
After some simplification, we get
RGA(Γ2)=(92+3√154)t2+(−9910+3√154)t+515−3√15. |
In the next theorems, we will calculate the reverse redefined Zagreb indices for Γ2 graph.
Theorem 4.3. Let Γ2 be the third type of triangular hex-derived network, then
● RReZ1(Γ2)=49t25−649t15+73415
● RReZ2(Γ2)=231t28+1671t40−101710
● RReZ3(Γ2)=21693t2+15513t−38142.
Proof. Reverse redefined Zagreb indices can be calculated by using Eq (4.1), the RReZ1(Γ2) by using Eq (1.9) as follows:
RReZ1(Γ2)=2×|E1,1(Γ2)|+109×|E9,1(Γ2)|+1881×|E9,9(Γ2)|+1615×|E15,1(Γ2)|+24135×|E15,9(Γ2)|+30225×|E15,15(Γ2)|.
After some simplification, we get
RReZ1(Γ2)=49t25−649t15+73415. |
The RReZ2(Γ2) can be determined by using Eq (1.10) as follows:
RReZ2(Γ2)=12×|E1,1(Γ2)|+910×|E9,1(Γ2)|+8118×|E9,9(Γ2)|+1516×|E15,1(Γ2)|+13524×|E15,9(Γ2)|+22530×|E15,15(Γ2)|.
After some simplification, we get
RReZ2(Γ2)=231t28+1671t40−101710. |
The RReZ3(Γ2) can be calculated by using Eq (1.11) as follows:
RReZ3(Γ2)=2×|E1,1(Γ2)|+90×|E9,1(Γ2)|+1458×|E9,9(Γ2)|+240×|E15,1(Γ2)|+3240×|E15,9(Γ2)|+6750×|E15,15(Γ2)|.
After some simplification, we get
RReZ3(Γ2)=21693t2+15513t−38142. |
In this section, we calculate certain reverse degree based topological indices of the third type of rectangular hex-derived network, RHDN3(t,w) of dimension t=w. Now we calculated reverse degree based indices such as: reverse Randic index RRα, reverse atom bond connectivity index RABC, reverse geometric arithmetic index RGA, first reverse Zagreb index RM1, second reverse Zagreb index RM2, reverse forgotten index RF, reverse augmented Zagreb index RAZI, reverse hyper Zagreb index RHM and reverse redefined Zagreb indices for Γ3 graph.
|ER(ω),R(μ)(Γ3)|={3t2−16t+21,for; R(ω)=1,R(μ)=18t−20,for; R(ω)=9,R(μ)=14t−10,for; R(ω)=9,R(μ)=92,for; R(ω)=12,R(μ)=14,for; R(ω)=12,R(μ)=912t2−48t+48,for; R(ω)=15,R(μ)=124t−44,for; R(ω)=15,R(μ)=98,for; R(ω)=15,R(μ)=126t2−12t+10,for; R(ω)=15,R(μ)=15 | (5.1) |
In the next theorem, we will calculate the reverse Randic index, reverse Zagreb indices, reverse forgotten index for Γ3 graph.
Theorem 5.1. Let Γ3 be the third type of rectangular hex-derived network, then
● RRα(Γ3)=(3+12(15)α+6(225)α)t2+(−16+8(9)α+4(81)α−48(15)α+24(135)α−12(225)α)t+21−20(9)α−10(81)α+2(12)α+4(108)α+48(15)α−44(135)α+8(180)α+10(225)α
● RM1(Γ3)=378t2−432t
● RM2(Γ3)=1533t2+200t−2043
● RHM(Γ3)=8484t2−7232t−1278
● RF(Γ3)=5418t2−7632t+2808.
Proof. Let Γ3 be the third type of rectangular hex-derived network which is shown in Figure 4. The order of hexagonal hex derived network Γ3 is p=|Γ1|=7t2−12t+6 and size is q=21t2−40t+19. The edge partitioned of Γ3 based on their reverse degrees are shown in Eq (5.1). Reverse Randic index can be calculated by using Eq (5.1). Thus, from Eq (1.1), it follows,
RRα(Γ3)=(1)α|E1,1(Γ3)|+(9)α|E9,1(Γ3)|+(81)α|E9,9(Γ3)|+(12)α|E12,1(Γ3)|+(108)α|E12,9(Γ3)|+(15)α|E15,1(Γ3)|+(135)α|E15,9(Γ3)|+(180)α|E15,12(Γ3)|+(225)α|E15,15(Γ3)|.
After Simplification, we get
RRα(Γ3)=(3+12(15)α+6(225)α)t2+(−16+8(9)α+4(81)α−48(15)α+24(135)α−12(225)α)t+21−20(9)α−10(81)α+2(12)α+4(108)α+48(15)α−44(135)α+8(180)α+10(225)α.
Put α=1 and after some calculation, we get reverse second Zagreb index RM2(Γ3) as:
RM2(Γ3)=1533t2+200t−2043. | (5.2) |
Using the Eq (1.4), we can determine the reverse first Zagreb index RM1(Γ3) as:
RM1(Γ3)=2×|E1,1(Γ3)|+10×|E9,1(Γ3)|+18×|E9,9(Γ3)|+13×|E12,1(Γ3)|+21×|E12,9(Γ3)|+16×|E15,1(Γ3)|+24×|E15,9(Γ3)|+27×|E15,12(Γ3)|+30×|E15,15(Γ3)|.
By putting the values of from Eq (5.1) and after simplification, we obtain:
RM1(Γ3)=378t2−432t. | (5.3) |
Using the Eq (1.6), we can determine the reverse hyper Zagreb index RHM(Γ3) as:
RHM(Γ3)=4×|E1,1(Γ3)|+100×|E9,1(Γ3)|+324×|E9,9(Γ3)|+169×|E12,1(Γ3)|+441×|E12,9(Γ3)|+256×|E15,1(Γ3)|+576×|E15,9(Γ3)|+729×|E15,12(Γ3)|+900×|E15,15(Γ3)|.
After simplification, we get
RHM(Γ3)=8484t2−7232t−1278. |
Using the Eq (1.7), we can determine the reverse forgotten index RF(Γ3)) as:
RF(Γ3))=2×|E1,1(Γ3)|+82×|E9,1(Γ3)|+162×|E9,9(Γ3)|+145×|E12,1(Γ3)|+225×|E12,9(Γ3)|+226×|E15,1(Γ3)|+306×|E15,9(Γ3)|+369×|E15,12(Γ3)|+450×|E15,15(Γ3)|.
After simplification, we get
RF(Γ3)=5418t2−7632t+2808. |
In the next theorem, we will calculate the reverse atom bond connectivity index, reverse geometric arithmetic index for Γ3 graph.
Theorem 5.2. Let Γ3 be the third type of rectangular hex-derived network, then
● RABC(Γ3)=(4√2105+4√75)t2+(16√23+169−16√2105+8√33015−8√75)t−409−40√23+√333+2√579+16√2105−44√33045+4√53+4√73.
● RGA(Γ3)=(9+3√152)t2−96t5+9+264√391−5√15+32√59.
Proof. The reverse atom bond connectivity (RABC(Γ3)), can be determined by using Eq (1.2) and Eq (5.1), as follows:
RABC(Γ3)=0×|E1,1(Γ3)|+√89×|E9,1(Γ3)|+√1681×|E9,9(Γ3)|+√1112×|E12,1(Γ3)|+√19108×|E12,9(Γ3)|+√1415×|E15,1(Γ3)|+√22135×|E15,9(Γ3)|+√25180×|E15,12(Γ3)|+√28225×|E15,15(Γ3)|.
After some simplification, we get
RABC(Γ3)=(4√2105+4√75)t2+(16√23+169−16√2105+8√33015−8√75)t−409−40√23+√333+2√579+16√2105−44√33045+4√53+4√73.
The reverse geometric arithmetic (RGA(Γ3)), can be determined by using Eq (1.3) and Eq (5.1), as follows:
RGA(Γ3)=|E1,1(Γ3)|+2√910×|E9,1(Γ3)|+2√8118×|E9,9(Γ3)|+2√1213×|E12,1(Γ3)|+2√10821×|E12,9(Γ3)|+2√1516×|E15,1(Γ3)|+2√13524×|E15,9(Γ3)|+2√18027×|E15,12(Γ3)|+2√22530×|E15,15(Γ3)|.
After some simplification, we get
RGA(Γ3)=(9+3√152)t2−96t5+9+264√391−5√15+32√59.
In the next theorems, we will calculate the reverse redefined Zagreb indices for Γ3 graph.
Theorem 5.3. Let Γ3 be the third type of rectangular hex-derived network, then
● RReZ1(Γ3)=98t25−3184t45+597790
● RReZ2(Γ3)=231t24+86t5−28460273
● RReZ3(Γ3)=43386t2−8240t−31614.
Proof. Reverse redefined Zagreb indices can be calculated by using Eq (5.1), the RReZ1(Γ3) by using Eq (1.9) as follows:
RReZ1(Γ3)=2×|E1,1(Γ3)|+109×|E9,1(Γ3)|+1881×|E9,9(Γ3)|+1312×|E12,1(Γ3)|+21108×|E12,9(Γ3)|+1615×|E15,1(Γ3)|+24135×|E15,9(Γ3)|+27108×|E15,12(Γ3)|+30225×|E15,15(Γ3)|.
After some simplification, we get
RReZ1(Γ3)=98t25−3184t45+597790. |
The RReZ2(Γ1) can be determined by using equation (1.10) as follows:
RReZ2(Γ3)=12×|E1,1(Γ3)|+910×|E9,1(Γ3)|+8118×|E9,9(Γ3)|+1213×|E12,1(Γ3)|+10821×|E12,9(Γ3)|+1516×|E15,1(Γ3)|+13524×|E15,9(Γ3)|+10827×|E15,12(Γ3)|+22530×|E15,15(Γ3)|.
After some simplification, we get
RReZ2(Γ3)=231t24+86t5−28460273. |
The RReZ3(Γ3) can be calculated by using Eq (1.11) as follows:
RReZ3(Γ3)=2×|E1,1(Γ3)|+90×|E9,1(Γ3)|+1458×|E9,9(Γ3)|+156×|E12,1(Γ3)|+2268×|E12,9(Γ3)|+240×|E15,1(Γ3)|+3240×|E15,9(Γ3)|+2916×|E15,12(Γ3)|+6750×|E15,15(Γ3)|.
After some simplification, we get
RReZ3(Γ3)=43386t2−8240t−31614. |
In this article, we have calculated the exact solutions of reverse degree-based topological descriptors for hex-derived networks of third type. Hex-derived network has a variety of useful applications in pharmacy, electronics, and networking. We obtained the reverse degree-based indices such as reverse Randic index, reverse atom bond connectivity index, reverse geometric arithmetic index, reverse Zagreb indices, reverse redefined Zagreb indices for hex derived networks. These results may be helpful for people working in computer science and chemistry who encounter hex-derived networks.
The authors declare that there is no conflict of financial interests regarding the publication of this paper.
[1] | M. Kowalski, Deepfakes. Available from: https://www.github.com/MarekKowalski/FaceSwap/. |
[2] |
K. Liu, I. Perov, D. Gao, N. Chervoniy, W. Zhou, W. Zhang, Deepfacelab: integrated, flexible and extensible face-swapping framework, Pattern Recognit., 141 (2023), 109628. https://doi.org/10.1016/j.patcog.2023.109628 doi: 10.1016/j.patcog.2023.109628
![]() |
[3] | D. Afchar, V. Nozick, J. Yamagishi, I. Echizen, MesoNet: a compact facial video forgery detection network, in 2018 IEEE International Workshop on Information Forensics and Security (WIFS), (2018), 1–7. https://doi.org/10.1109/WIFS.2018.8630761 |
[4] | F. Matern, C. Riess, M. Stamminger, Exploiting visual artifacts to expose deepfakes and face manipulations, in 2019 IEEE Winter Applications of Computer Vision Workshops (WACVW), (2019), 83–92. https://doi.org/10.1109/WACVW.2019.00020 |
[5] | Y. Qian, G. Yin, L. Sheng, Z. Chen, J. Shao, Thinking in frequency: face forgery detection by mining frequency-aware clues, in ECCV 2020: Computer Vision – ECCV 2020, Springer-Verlag, (2020), 86–103. https://doi.org/10.1007/978-3-030-58610-2_6 |
[6] | H. Liu, X. Li, W. Zhou, Y. Chen, Y. He, H. Xue, et al., Spatial-phase shallow learning: rethinking face forgery detection in bfrequency domain, in Proceedings of the IEEE/CVF Conference on Computer Vision and Pattern Recognition (CVPR), (2021), 772–781. |
[7] | S. Chen, T. Yao, Y. Chen, S. Ding, J. Li, R. Ji, Local relation learning for face forgery detection, in Proceedings of the AAAI Conference on Artificial Intelligence, 35 (2021), 1081–1088. https://doi.org/10.1609/aaai.v35i2.16193 |
[8] | Q. Gu, S. Chen, T. Yao, Y. Chen, S. Ding, R. Yi, Exploiting fine-grained face forgery clues via progressive enhancement learning, in Proceedings of the AAAI Conference on Artificial Intelligence, 36 (2022), 735–743. https://doi.org/10.1609/aaai.v36i1.19954 |
[9] | X. Li, Y. Lang, Y. Chen, X. Mao, Y. He, S. Wang, et al., Sharp multiple instance learning for DeepFake video detection, in Proceedings of the 28th ACM International Conference on Multimedia, (2020), 1864–1872. https://doi.org/10.1145/3394171.3414034 |
[10] | Z. Gu, Y. Chen, T. Yao, S. Ding, J. Li, F. Huang, et al., Spatiotemporal inconsistency learning for DeepFake video detection, in Proceedings of the 29th ACM International Conference on Multimedia, (2021), 3473–3481. https://doi.org/10.1145/3474085.3475508 |
[11] | S. A. Khan, H. Dai, Video transformer for deepfake detection with incremental learning, in Proceedings of the 29th ACM International Conference on Multimedia, (2021), 1821–1828. http://doi.org/10.1145/3474085.3475332 |
[12] | D. H. Choi, H. J. Lee, S. Lee, J. U. Kim, Y. M. Ro, Fake video detection with certainty-based attention network, in 2020 IEEE International Conference on Image Processing (ICIP), (2020), 823–827. http://doi.org/10.1109/ICIP40778.2020.9190655 |
[13] | E. Sabir, J. Cheng, A. Jaiswal, W. Abdalmageed, I. Masi, P. Natarajan, Recurrent convolutional strategies for face manipulation detection in videos, Interfaces (GUI), (2019), 80–87. |
[14] |
A. Chintha, B. Thai, S. J. Sohrawardi, K. Bhatt, A. Hickerson, M. Wright, et al., Recurrent convolutional structures for audio spoof and video deepfake detection, IEEE J. Sel. Top. Signal Process., 14 (2020), 1024–1037. http://doi.org/10.1109/JSTSP.2020.2999185 doi: 10.1109/JSTSP.2020.2999185
![]() |
[15] | A. Haliassos, K. Vougioukas, S. Petridis, M. Pantic, Lips don't lie: a generalisable and robust approach to face forgery detection, in Proceedings of the IEEE/CVF Conference on Computer Vision and Pattern Recognition (CVPR), (2021), 5039–5049. |
[16] | Y. Zheng, J. Bao, D. Chen, M. Zeng, F. Wen, Exploring temporal coherence for more general video face forgery detection, in Proceedings of the IEEE/CVF International Conference on Computer Vision (ICCV), (2021), 15044–15054. |
[17] | Z. Gu, Y. Chen, T. Yao, S. Ding, J. Li, L. Ma, Delving into the local: dynamic inconsistency learning for DeepFake video detection, in Proceedings of the AAAI Conference on Artificial Intelligence, 36 (2022), 744–752. http://doi.org/10.1609/aaai.v36i1.19955 |
[18] | X. Zhao, Y. Yu, R. Ni, Y. Zhao, Exploring complementarity of global and local spatiotemporal information for fake face video detection, in ICASSP 2022 - 2022 IEEE International Conference on Acoustics, Speech and Signal Processing (ICASSP), (2022), 2884–2888. http://doi.org/10.1109/ICASSP43922.2022.9746061 |
[19] | R. Shao, T. Wu, Z. Liu, Detecting and recovering sequential DeepFake manipulation, in ECCV 2022: Computer Vision – ECCV 2022, Springer-Verlag, (2022), 712–728. http://doi.org/10.1007/978-3-031-19778-9_41 |
[20] | A. Dosovitskiy, L. Beyer, A. Kolesnikov, D. Weissenborn, X. Zhai, T. Unterthiner, et al., An image is worth 16 x 16 words: transformers for image recognition at scale, preprint, arXiv: 2010.11929. |
[21] | A. Arnab, M. Dehghani, G. Heigold, C. Sun, M. Lučić, C. Schmid, ViViT: a video vision transformer, in Proceedings of the IEEE/CVF International Conference on Computer Vision (ICCV), (2021), 6836–6846. |
[22] | Y. Zhang, X. Li, C. Liu, B. Shuai, Y. Zhu, B. Brattoli, et al., VidTr: Video transformer without convolutions, in Proceedings of the IEEE/CVF International Conference on Computer Vision (ICCV), (2021), 13577–13587. |
[23] | L. He, Q. Zhou, X. Li, L. Niu, G. Cheng, X. Li, et al., End-to-end video object detection with spatial-temporal transformers, in Proceedings of the 29th ACM International Conference on Multimedia, (2021), 1507–1516. http://doi.org/10.1145/3474085.3475285 |
[24] |
Z. Xu, D. Chen, K. Wei, C. Deng, H. Xue, HiSA: Hierarchically semantic associating for video temporal grounding, IEEE Trans. Image Process., 31 (2022), 5178–5188. http://doi.org/10.1109/TIP.2022.3191841 doi: 10.1109/TIP.2022.3191841
![]() |
[25] | O. de Lima, S. Franklin, S. Basu, B. Karwoski, A. George, Deepfake detection using spatiotemporal convolutional networks, preprint, arXiv: 2006.14749. |
[26] | D. Güera, E. J. Delp, Deepfake video detection using recurrent neural networks, in 2018 15th IEEE International Conference on Advanced Video and Signal Based Surveillance (AVSS), (2018), 1–6. http://doi.org/10.1109/AVSS.2018.8639163 |
[27] | I. Masi, A. Killekar, R. M. Mascarenhas, S. P. Gurudatt, W. AbdAlmageed, Two-branch recurrent network for isolating deepfakes in videos, in ECCV 2020: Computer Vision – ECCV 2020, Springer-Verlag, (2020), 667–684. http://doi.org/10.1007/978-3-030-58571-6_39 |
[28] |
Y. Yu, R. Ni, Y. Zhao, S. Yang, F. Xia, N. Jiang, et al., MSVT: Multiple spatiotemporal views transformer for DeepFake video detection, IEEE Trans. Circuits Syst. Video Technol., 33 (2023), 4462–4471. http://doi.org/10.1109/TCSVT.2023.3281448 doi: 10.1109/TCSVT.2023.3281448
![]() |
[29] |
H. Cheng, Y. Guo, T. Wang, Q. Li, X. Chang, L. Nie, Voice-face homogeneity tells deepfake, ACM Trans. Multimedia Comput. Commun. Appl., 20 (2023), 1–22. http://doi.org/10.1145/3625231 doi: 10.1145/3625231
![]() |
[30] |
W. Yang, X. Zhou, Z. Chen, B. Guo, Z. Ba, Z. Xia, et al., AVoiD-DF: Audio-visual joint learning for detecting deepfake, IEEE Trans. Inf. Forensics Secur., 18 (2023), 2015–2029. http://doi.org/10.1109/TIFS.2023.3262148 doi: 10.1109/TIFS.2023.3262148
![]() |
[31] | M. Liu, J. Wang, X. Qian, H. Li, Audio-visual temporal forgery detection using embedding-level fusion and multi-dimensional contrastive loss, IEEE Trans. Circuits Syst. Video Technol., 2023. http://doi.org/10.1109/TCSVT.2023.3326694 |
[32] |
Q. Yin, W. Lu, B. Li, J. Huang, Dynamic difference learning with spatio–temporal correlation for deepfake video detection, IEEE Trans. Inf. Forensics Secur., 18 (2023), 4046–4058. http://doi.org/10.1109/TIFS.2023.3290752 doi: 10.1109/TIFS.2023.3290752
![]() |
[33] |
Y. Wang, C. Peng, D. Liu, N. Wang, X. Gao, Spatial-temporal frequency forgery clue for video forgery detection in VIS and NIR scenario, IEEE Trans. Circuits Syst. Video Technol., 33 (2023), 7943–7956. http://doi.org/10.1109/TCSVT.2023.3281475 doi: 10.1109/TCSVT.2023.3281475
![]() |
[34] | D. E. King, Dlib-ml: A machine learning toolkit, J. Mach. Learn. Res., 10 (2009), 1755–1758. Available from: http://www.jmlr.org/papers/volume10/king09a/king09a.pdf. |
[35] | A. Rossler, D. Cozzolino, L. Verdoliva, C. Riess, J. Thies, M. Niessner, FaceForensics++: Learning to detect manipulated facial images, in 2019 IEEE/CVF International Conference on Computer Vision (ICCV), (2019), 1–11. http://doi.org/10.1109/ICCV.2019.00009 |
[36] | E. Xie, W. Wang, Z. Yu, A. Anandkumar, J. M. Alvarez, P. Luo, SegFormer: Simple and efficient design for semantic segmentation with transformers, in Advances in Neural Information Processing Systems, 34 (2021), 12077–12090. |
[37] | W. Wang, E. Xie, X. Li, D. P. Fan, K. Song, D. Liang, et al., Pyramid vision transformer: a versatile backbone for dense prediction without convolutions, in Proceedings of the IEEE/CVF International Conference on Computer Vision (ICCV), (2021), 568–578. |
[38] | X. Chu, Z. Tian, B. Zhang, X. Wan, C. Shen, Conditional positional encodings for vision transformers, preprint, arXiv: 2102.10882. |
[39] | M. A. Islam, S. Jia, N. D. B. Bruce, How much position information do convolutional neural networks encode? preprint, arXiv: 2001.08248. |
[40] | N. Dufour, A. Gully, P. Karlsson, A. V. Vorbyov, T. Leung, J. Childs, et al., Contributing data to Deepfake detection research by Google Research & Jigsaw, 2019. Available from: http://blog.research.google/2019/09/contributing-data-to-deepfake-detection.html. |
[41] | B. Dolhansky, J. Bitton, B. Pflaum, J. Lu, R. Howes, M. Wang, et al., The DeepFake detection challenge (DFDC) dataset, preprint, arXiv: 2006.07397. |
[42] | Y. Li, X. Yang, P. Sun, H. Qi, S. Lyu, Celeb-DF: A large-scale challenging dataset for DeepFake forensics, in Proceedings of the IEEE/CVF Conference on Computer Vision and Pattern Recognition (CVPR), (2020), 3207–3216. |
[43] | L. Jiang, R. Li, W. Wu, C. Qian, C. C. Loy, DeeperForensics-1.0: A large-scale dataset for real-world face forgery detection, in Proceedings of the IEEE/CVF Conference on Computer Vision and Pattern Recognition (CVPR), (2020), 2889–2898. |
[44] |
A. P. Bradley, The use of the area under the ROC curve in the evaluation of machine learning algorithms, Pattern Recognit., 30 (1997), 1145–1159. http://doi.org/10.1016/S0031-3203(96)00142-2 doi: 10.1016/S0031-3203(96)00142-2
![]() |
[45] | P. Micikevicius, S. Narang, J. Alben, G. F. Diamos, E. Elsen, D. Garcia, et al., Mixed precision training, preprint, arXiv: 1710.03740. |
[46] | Z. Zhang, M. R. Sabuncu, Generalized cross entropy loss for training deep neural networks with noisy labels, in Advances in Neural Information Processing Systems, 31 (2018). |
[47] | L. van der Maaten, G. Hinton, Visualizing data using t-SNE, J. Mach. Learn. Res., 9 (2008), 2579–2605. |
[48] | I. Radosavovic, R. P. Kosaraju, R. Girshick, K. He, P. Dollár, Designing network design spaces, in 2020 IEEE/CVF Conference on Computer Vision and Pattern Recognition (CVPR), (2020), 10425–10433. http://doi.org/10.1109/CVPR42600.2020.01044 |
[49] | Z. Liu, H. Hu, Y. Lin, Z. Yao, Z. Xie, Y. Wei, et al., Swin transformer v2: Scaling up capacity and resolution, in Proceedings of the IEEE/CVF Conference on Computer Vision and Pattern Recognition (CVPR), (2022), 12009–12019. |
[50] | H. Bao, L. Dong, S. Piao, F. Wei, BEiT: BERT pre-training of image transformers, preprint, arXiv: 2106.08254. |
[51] | W. Yu, M. Luo, P. Zhou, C. Si, Y. Zhou, X. Wang, et al., Metaformer is actually what you need for vision, in Proceedings of the IEEE/CVF Conference on Computer Vision and Pattern Recognition (CVPR), (2022), 10819–10829. |
1. | Saima Parveen, Fozia Bashir Farooq, Nadeem Ul Hassan Awan, Rakotondrajao Fanja, Muhammad Farooq Choudhary, Muhammad Kamran Jamil, Topological Indices of Drugs Used in Rheumatoid Arthritis Treatment and Its QSPR Modeling, 2022, 2022, 2314-4785, 1, 10.1155/2022/1562125 | |
2. | Ali N.A. Koam, Moin A. Ansari, Azeem Haider, Ali Ahmad, Muhammad Azeem, Topological properties of reverse-degree-based indices for sodalite materials network, 2022, 15, 18785352, 104160, 10.1016/j.arabjc.2022.104160 | |
3. | Ali N. A. Koam, Ali Ahmad, Ashfaq Ahmed Qummer, Gohar Ali, On the Study of Reverse Degree-Based Topological Properties for the Third Type of p th Chain Hex-Derived Network, 2021, 2021, 2314-4785, 1, 10.1155/2021/4540276 | |
4. | Usman Babar, Asim Naseem, Hani Shaker, Mian Muhammad Zobair, Haidar Ali, Andrea Penoni, Eccentricity-Based Topological Descriptors of First Type of Hex-Derived Network, 2022, 2022, 2090-9071, 1, 10.1155/2022/3340057 | |
5. | Vignesh Ravi, QSPR analysis of drugs used for treatment of hepatitis via reduced reverse degree-based topological descriptors, 2024, 99, 0031-8949, 105236, 10.1088/1402-4896/ad729d | |
6. | Muhammad Mudassar Hassan, Topological Descriptors of Molecular Networks via Reverse Degree, 2023, 1040-6638, 1, 10.1080/10406638.2023.2274473 | |
7. | Qasem M. Tawhari, Muhammad Naeem, Abdul Rauf, Muhammad Kamran Siddiqui, Oladele Oyelakin, Modeling and estimation of physiochemical properties of cancer drugs using entropy measures, 2025, 15, 2045-2322, 10.1038/s41598-025-87755-5 |