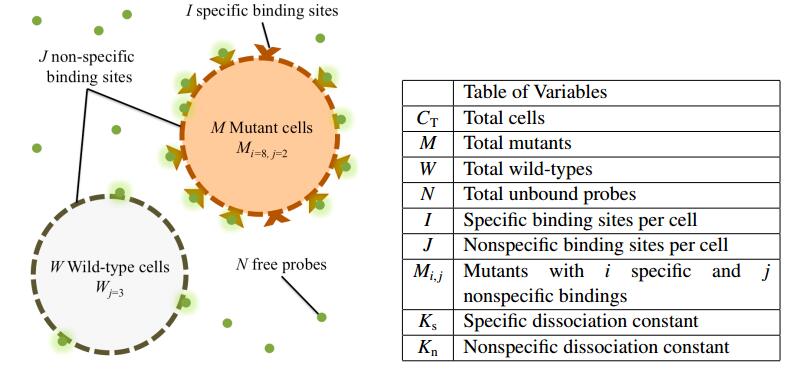
We consider an initial and boundary value problem for a nonlinear hyperbolic system with damping and diffusion. This system was derived from the Rayleigh–Benard equation. Based on a new observation of the structure of the system, two identities are found; then, the following results are proved by using the energy method. First, the well-posedness of the global large solution is established; then, the limit with a boundary layer as some diffusion coefficient tending to zero is justified. In addition, the L2 convergence rate in terms of the diffusion coefficient is obtained together with the estimation of the thickness of the boundary layer.
Citation: Xu Zhao, Wenshu Zhou. Vanishing diffusion limit and boundary layers for a nonlinear hyperbolic system with damping and diffusion[J]. Electronic Research Archive, 2023, 31(10): 6505-6524. doi: 10.3934/era.2023329
[1] | Junjing Cai, Qiwei Wang, Ce Wang, Yu Deng . Research on cell detection method for microfluidic single cell dispensing. Mathematical Biosciences and Engineering, 2023, 20(2): 3970-3982. doi: 10.3934/mbe.2023185 |
[2] | H. Thomas Banks, W. Clayton Thompson, Cristina Peligero, Sandra Giest, Jordi Argilaguet, Andreas Meyerhans . A division-dependent compartmental model for computing cell numbers in CFSE-based lymphocyte proliferation assays. Mathematical Biosciences and Engineering, 2012, 9(4): 699-736. doi: 10.3934/mbe.2012.9.699 |
[3] | Shuangshuang Wen, Peng Zhao, Siyu Chen, Bo Deng, Qin Fang, Jishi Wang . The impact of MCCK1, an inhibitor of IKBKE kinase, on acute B lymphocyte leukemia cells. Mathematical Biosciences and Engineering, 2024, 21(4): 5164-5180. doi: 10.3934/mbe.2024228 |
[4] | D. Mackey, E. Kelly, R. Nooney . Modelling random antibody adsorption and immunoassay activity. Mathematical Biosciences and Engineering, 2016, 13(6): 1159-1168. doi: 10.3934/mbe.2016036 |
[5] | Xuan Zhang, Huiqin Jin, Zhuoqin Yang, Jinzhi Lei . Effects of elongation delay in transcription dynamics. Mathematical Biosciences and Engineering, 2014, 11(6): 1431-1448. doi: 10.3934/mbe.2014.11.1431 |
[6] | Qirui Li, Baikun Zhang, Delong Cui, Zhiping Peng, Jieguang He . The research of recognition of peep door open state of ethylene cracking furnace based on deep learning. Mathematical Biosciences and Engineering, 2022, 19(4): 3472-3486. doi: 10.3934/mbe.2022160 |
[7] | Guohai Wang, Zhiyuan Hu . Icotinib inhibits proliferation and epithelial-mesenchymal transition of non-small cell lung cancer A549 cells. Mathematical Biosciences and Engineering, 2019, 16(6): 7707-7718. doi: 10.3934/mbe.2019386 |
[8] | Frederik Riis Mikkelsen . A model based rule for selecting spiking thresholds in neuron models. Mathematical Biosciences and Engineering, 2016, 13(3): 569-578. doi: 10.3934/mbe.2016008 |
[9] | Francesca Marcellini . The Riemann problem for a Two-Phase model for road traffic with fixed or moving constraints. Mathematical Biosciences and Engineering, 2020, 17(2): 1218-1232. doi: 10.3934/mbe.2020062 |
[10] | Sakorn Mekruksavanich, Anuchit Jitpattanakul . RNN-based deep learning for physical activity recognition using smartwatch sensors: A case study of simple and complex activity recognition. Mathematical Biosciences and Engineering, 2022, 19(6): 5671-5698. doi: 10.3934/mbe.2022265 |
We consider an initial and boundary value problem for a nonlinear hyperbolic system with damping and diffusion. This system was derived from the Rayleigh–Benard equation. Based on a new observation of the structure of the system, two identities are found; then, the following results are proved by using the energy method. First, the well-posedness of the global large solution is established; then, the limit with a boundary layer as some diffusion coefficient tending to zero is justified. In addition, the L2 convergence rate in terms of the diffusion coefficient is obtained together with the estimation of the thickness of the boundary layer.
A common problem in cell biology research is the desire to differentiate cells into categorical populations based on some defining molecular characteristic. Some examples include the presence or absence of a particular gene transcript [1,2], cells presenting viral epitopes to indicate infection [3,4], or expression of particular membrane proteins [5,6]. Flow cytometry is an effective tool to count the number of cells exhibiting the identifying characteristic. The process involves suspending cells of interest in a sheath fluid that is pressurized and extruded single file past a laser beam [7,8,9]. Each cell will scatter the laser's light towards optical sensors positioned around the stream. Sensors directly in front of the laser measure the forward scattering about a single cell, and is used to quantify the cell's surface area, volume, and shape. Alternatively, side scattering sensors measure photons emitted by fluorescent markers and dyes excited by the laser. The fluorescent probes are designed a priori to bind specifically to cell surface proteins and structures that characterize the cell species. Thus, a sufficiently high fluorescence intensity is an indication the cell is of the desired type.
However, details of the protocol arise that can confound the final count of cells. If we focus on the example of a population of "mutant" cells, characterized by the expression of a particular membrane surface receptor, mixed in with a population of "wild-type" cells, we can design a fluorescing probe that binds specifically to the receptor. If we suspend all cells in a solution containing an excess of probes, we expect all probes to bind to free receptors. However, each probe-receptor binding event is a reversible process, allowing some expected proportion of receptors to remain unbound at equilibrium. Furthermore, variation may exist in the number of receptors expressed, increasing the ways in which a mutant may escape binding [4,5]. To combat this measurement of false negatives, one can increase the probe concentration in the suspension, increasing its excess and driving the equilibrium towards more bound receptors. Unfortunately, although the probes are designed to bind specifically to the receptors, they will have a relatively small, but non-zero binding affinity to the rest of the cell membrane and its other embedded structures [10]. Increasing the probe concentration will result in a higher nonspecific binding to wild-type cell membranes, allowing false positive counts of mutants. The equilibrium configuration of probe bindings to all cells, whether specifically or nonspecifically, will produce a distribution of fluorescence data over a range of intensities. Typically, cells that exhibit levels of fluorescence below a threshold intensity are ignored in a process known as "gating." Setting that gating threshold is often a heuristic procedure, though some methods for automatic gating based on data clustering have been developed [9,11]. However, these methods do not incorporate the underlying chemical kinetics of probe binding and largely ignore the effects of nonspecific binding.
We develop a kinetic model for both specific and nonspecific binding of probes to cells. We employ a variant on the Langmuir adsorption model [12] with two competing types of binding sites: receptors and a discretization of the cell membrane. Here, the concentration of initially added probes applies the "partial pressure" driving probe binding to the cell surfaces. We will show the isotherm of fractional binding site occupancy to exhibit two regimes in which the receptor and membrane binding sites become saturated at different rates. We discuss how the interface between the two regimes is the ideal concentration of probes to include in the assay and how the model can inform optimal experimental design. We then present a probabilistic model for the expected number density of cells over possible numbers of probe bindings. Employing this model, we develop a variant on the expectation-maximization (EM) mixture model [13] to estimate the total number of mutant cells without heuristic gating. Furthermore, we propose a method for inferring the probe binding affinity and the receptor number distribution using a serial dilution protocol. Finally, we discuss potential applications and problems of using our method for the fluorescence activated cell sorting (FACS) assay. It should be noted that throughout this paper we continue using the example of "mutant cells" expressing surface receptors, but our models and analyses extend to all physiological applications of flow cytometry with fluorescing surface markers.
Let CT be the total number of cells in a suspension also containing NT probe molecules. We assume CT is precisely counted by the forward scattering measurement to differentiate cells from free probes or debris. Let M and W=CT−M be the number of mutant cells and wild-type cells, respectively. By definition, mutants are the only cells that express the surface receptors. Let N be the number of free, unbound fluorescing probes designed to specifically target the receptors with association and dissociation rates p+ and p−. Alternatively, for both mutants and wild-types, probes can bind and dissociate nonspecifically to the cell membrane itself with rates q+ and q−. Since, the on-rates p+ and q+ are likely to be comparable in magnitude, we expect probes bound nonspecifically to dissociate significantly more rapidly so that q−≫p−.
The total number of receptors I on a mutant cell varies across all cells with distribution f(I) and mean ⟨I⟩. The exact distribution f will depend on the details of the receptor and its expression and recycling pathways. A simple model for receptor expression is a production and degradation process with constant rates α and μ, respectively. The steady-state receptor number of such an immigration-death model follows a Poisson distribution
f(I)=⟨I⟩Ie−⟨I⟩I!, | (2.1) |
where ⟨I⟩=α/μ. We use this form of f(I) in the simulations we perform in this paper; however, the full models we derive are independent of the choice for f. Thus, we denote the total number of mutant cells that carry exactly I receptors as MI.
Furthermore, we consider the the total surface area A of the cell membrane and partition the binding region around a single receptor as As. We define this region as that which a probe fated to adsorb to the cell surface is more likely to bind to the associated receptor than directly to the membrane. We can partition the remaining cell surface into J=AAs−I discrete effective binding sites for the membrane. We expect the binding region of a receptor to be relatively small compared to the total surface area, making J≫I.
To accurately model the kinetic flows from one bound state of a mutant to another, it becomes necessary to track populations of cells indexed by both the number of probes bound specifically and nonspecifically. As shown in Figure 1, we define MIi,j as the number of mutant cells with exactly i probes bound to a maximum of I specific binding sites and exactly j probes adsorbed nonspecifically. For wild-type cells, probes can only attach nonspecifically, so we define Wj as the number of wild-type cells with exactly j adsorbed probes. We thus have the chemical rate equations
MIi,j+N(I−i)p+⇌(i+1)p−MIi+1,j,MIi,j+N(J−j)q+⇌(j+1)q−MIi,j+1,Wj+N(J−j)q+⇌(j+1)q−Wj+1. | (2.2) |
Let v be the volume of the cell suspension containing all cells and probes. Normalizing the cell and probe counts by v, we have the relevant concentrations [MIi,j], [Wj], and [N]. We can now derive the mass-action equations as
d[MIi,j]dt=−(I−i)p+[MIi,j][N]−(J−j)q+[MIi,j][N]+(i+1)p−[MIi+1,j]+(j+1)q−[MIi,j+1]+(I−i+1)p+[MIi−1,j][N]+(J−j+1)q+[MIi,j−1][N],d[Wj]dt=−(J−j)q+[Wi][N]+(j+1)q−[Wj+1]+(J−j+1)q+[Wj−1][N],d[N]dt=q−J∑j=1j[MIi,j]+p−I∑i=1i[MIi,j]−q+J−1∑j=0(J−j)[MIi,j]−p+I−1∑i=0(I−i)[MIi,j]. | (2.3) |
At equilibrium, we expect detailed balance in each of Eq 2.2. For specific and nonspecific binding respectively, we can define the dissociation constants
[N][Mi,j][Mi,j+1]=q−q+≡Knand[N][Mi,j][Mi+1,j]=p−p+≡Ks. | (2.4) |
One might interpret these constants as the probe concentration's resistance to binding and are parameters that will shape the entire dynamics of the model. Using inductive reasoning, we can characterize the mutant populations solely with the concentration of unbound cells:
[MIi,j]=[MI0,0](Ii)([N]Ks)i(Jj)([N]Kn)j. | (2.5) |
By the conservation of total mutant cells with I binding sites, we use the binomial expansion to derive
[MI]=I∑i=0J∑j=0[MIi,j]=[MI0,0](1+[N]Ks)I(1+[N]Kn)J. | (2.6) |
Using very similar arguments, we derive the concentration of unbound wild-types as
[W0]=[W](1+[N]Kn)−J. | (2.7) |
Next, we find the following result:
I∑i=0J∑j=0(i+j)[MIi,j]=I∑i=0J∑j=0(i+j)[MI0,0](Ii)([N]Ks)i(Jj)([N]Kn)j=[MI0,0][I([N]Ks)(1+[N]Ks)I−1(1+[N]Kn)J+J([N]Kn)(1+[N]Ks)I(1+[N]Kn)J−1]=[MI0,0](1+[N]Ks)I(1+[N]Kn)J[I[N](Kn+[N])+J[N](Ks+[N])(Ks+[N])(Kn+[N])]=[MI][I[N](Kn+[N])+J[N](Ks+[N])(Ks+[N])(Kn+[N])]. | (2.8) |
Using the conservation of the total concentration of initial probes [NT] and ⟨I⟩=∑I=0If(I), we derive
[NT]=[N]+J∑j=0j[Wj]+J∑I=0I∑i=0J∑j=0(i+j)[MIi,j]=[N]+J∑j=0j[W0](Jj)([N]Kn)j+J∑I=0[MI][I[N](Kn+[N])+J[N](Ks+[N])(Ks+[N])(Kn+[N])]=[N]+⟨I⟩[M][N][N]+Ks+J[CT][N][N]+Kn. | (2.9) |
It is possible to solve Eq 2.9 for [N] analytically as the roots of a cubic polynomial with [M], [W], [NT], [Ks], [Kn], [J], and ⟨I⟩ as parameters. However, in the next section, we will show how this kinetic model may be used to quantify the optimal total probe concentration [NT] to use when performing the assay.
If we define θT as the fractional occupancy of total binding sites across all cells, using Eq 2.9 we have
θT=[NT]−[N]J[CT]=(⟨I⟩J)([M][CT])[N]Ks1+[N]Ks+[N]Kn1+[N]Kn. | (2.10) |
Each of the two terms resemble a Langmuir isotherm which measures the fractional occupancy of binding sites on a surface substrate [12]. Framed in the Langmuir adsorption picture, the concentration of free probes [N] is directly analogous to the partial pressure of adsorbing gas. As shown in Figure 2(a), the fraction of occupied binding sites grows as you add more free probes, but eventually saturates.
Note that the saturation is normalized according to the number of non-specific binding sites J as we expect it to be much larger than ⟨I⟩. Furthermore, the rate of adsorption is attenuated by the non-specific dissociation constant Kn. For small [N], when the cell membrane is far from saturation, we see the dynamics of the receptor binding site saturation in Figure 2(b). As Ks≫Kn, the total occupancy increases rapidly to saturation relative to that of the more dominant non-specific isotherm. Thus, there is a critical free probe concentration [N]∗ such that when [N] is below this threshold, each new probe introduced into the assay will contribute more to the occupancy of receptors than that of the nonspecific binding sites. This threshold can be obtained by differentiating the two terms in Eq 2.10 with respect to [N] and setting them equal to each other. This results in the following critical free probe concentration:
[N]∗=Kn√Ks⟨I⟩[M]KnJ[CT]−Ks1−√Ks⟨I⟩[M]KnJ[CT]. | (2.11) |
Note that [N]∗ is a monotonically increasing function of the concentration of mutant cells [M], which is typically unknown. Thus, a conservative recommended concentration [NT] to use in the cell/probe suspension would involve setting [M]=[CT] and evaluating Eq 2.10 with [N]∗. Furthermore, to ensure [N]∗ is strictly positive, the specific binding affinity of the probe is constrained by
Ks≤⟨I⟩JKn. | (2.12) |
In other words, for probes designed with weaker specific binding affinity than this threshold dictates, nonspecific binding sites are more likely to adsorb probes before any receptors for all values of [N] and [M]. Though it is clear that the lowest Ks possible is ideal for an assay, one may use this upper bound to decide if an available probe will at all be effective for a specific experiment.
In order to establish a connection between the equilibrium kinetic model and a typical output of a flow cytometry assay, we define the concentration of cells [Cr] with exactly r probes bound, regardless if they are bound specifically or nonspecifically, as
[Cr]=[Wr]+J∑I=0min(r,I)∑k=0[Mk,r−k]=([CT]−[M])(1+[N]Kn)−J(Jr)([N]Kn)r+[M]J∑I=0min(r,I)∑k=0f(I)(1+[N]Ks)−I(1+[N]Kn)−J(Ik)([N]Ks)k(Jr−k)([N]Kn)r−k. | (2.13) |
Equation 2.13 informs us of how the distribution of cell data will cluster, as illustrated in Figure 3(a). At relatively low concentrations of free probe [N], the binding of receptors can saturate, but leave the wild-type cells with only nonspecific binding to have significantly lower probe bindings. This effectively makes the two clusters qualitatively separable and imposing a gating threshold is straightforward. However, at high levels of free probe, the clusterings overlap and are thus difficult to differentiate heuristically, as demonstrated in Figure 3(b). Furthermore, these distributions are taken over the probe binding number r which is not directly measurable. We next show how r and [Cr] relate to the measured fluorescence intensity distribution.
As each cell passes through the cytometer, any bound probes will fluoresce with some strictly positive light intensity Fs. However, some variation in the fluorescence signal arises from molecular variability and instrumentation noise. We also expect each cell to have some relatively small amount of auto-fluorescence with intensity F0. Then if we define x as the total fluorescence intensity of a given cell and r as its corresponding number of bound probes, then we expect the probability density of x to follow
Pr(x|r)=1x√2πσ20exp[−(ln(x)−ln(F0+rFs))22σ20]. | (2.14) |
In a typical flow cytometry assay, however, we do not know how many probes are bound to each cell. Furthermore, we do not know the species b∈{0,1} of the cell, where 0 and 1 denote wild-type and mutant respectively. Using Eqs 2.13 and 2.14, we derive the marginal density of fluorescence intensity as
Pr(x)=Pr(b=0)J∑r=0Pr(x|r)Pr(r|b=0)+Pr(b=1)J∑r=0Pr(x|r)Pr(r=j|b=1)=1[CT]J∑r=0[Cr]x√2πσ20exp[−(ln(x)−ln(F0+rFs))22σ20]. | (2.15) |
Considering total number of probes r bound to a cell regardless if they are bound specifically or nonspecifically is sufficient if each probe fluoresces with an intensity independent of binding. However, for some probes, the fluorescence may be a product of a conformation change when binding to the designed target. This means that for nonspecifically bound probes, their conformation change may be partial and can result in a lower mean fluorescence intensity Fn. We must now consider how many probes are bound specifically and nonspecifically, making Eq 2.13 insufficient for computing the marginal density of x. Thus we derive the conditional densities
Pr(x|b=0)=(1−[M][CT])(1+[N]Kn)−JJ∑j=0(Jj)([N]Kn)j1x√2πσ20exp[−(ln(x)−ln(F0+jFn))22σ20], | (2.16) |
and
Pr(x|b=1)=[M][CT](1+[N]Kn)−J∑If(I)(1+[N]Ks)−II∑i=0J∑j=0(Ii)([N]Ks)i(Jj)([N]Kn)j×1x√2πσ20exp[−(ln(x)−ln(F0+iFs+jFn))22σ20]. | (2.17) |
In the next section we will show how these mathematical results can be used to iteratively estimate the size of the mutant population [M] from data and infer physical parameters such as Ks and ⟨I⟩.
Using Eqs 2.16 and 2.17, we propose an iterative algorithm to automatically infer the concentration of mutant cells [M] without heuristic gating. Let xk be a set of data, where xk is the fluorescence intensity measured for cell k and let bk∈{0,1} be its corresponding species assignment. Because this is an iterative method, we will index each numerical step with t∈{0,1,2,⋯}. Using Bayes rule, we can compute the probability cell k is a mutant as
Pr(bk=1|xk,[M](t))=[M](t)Pr(xk|bk=1,[M](t))([CT]−[M](t))Pr(xk|bk=0,[M](t))+[M](t)Pr(xk|bk=1,[M](t)), | (3.1) |
where [M](t) is the current mutant concentration estimate. The iterative procedure starts with an initial guess at the concentration of mutant cells [M](0) which is used to calculate the probability in Eq 3.1. The next estimate [M](t+1) is then given by
[M](t+1)=CT∑k=1Pr(bk=1|xk,[M](t)). | (3.2) |
This process is repeated until [M](t) converges. Note that, though calculating Eq 3.1 for a single xk can technically be a O(J3) computational operation, some asymptotic arguments can be made to concatenate summations to terms that are sufficiently close to zero. More importantly, the only value that changes over all iterations is [M](t). Thus, the more computationally heavy summations in Eqs 2.16 and 2.17 can be done once and stored in a matrix, making all subsequent iterations compute linearly with the number of cells CT.
An example using our algorithm for estimating the mutant cell count from simulated data is shown in Figure 4(a) where CT=100 and M=W=50. Immediately evident is the wild-type cells' propensity to be clustered close to the mutants when as little as one probe is bound. When a reasonable gate threshold is drawn as demonstrated, the 87 cells to the right are counted as mutants, resulting in 27 false positives. Our algorithm accounts for the probability of wild-types having high fluorescence, resulting in the closer estimate of M=51. Even for parameter regimes where probe binding to wild-types are rare, for large numbers of cells CT, the occasional nonspecific binding event will result in the gating process invariably over-counting mutants.
In typical flow cytometry assays, probes designed to bind specifically to the receptors of interest are often prepared elsewhere. Thus it is not uncommon for an experimentalist to test the affinity of a probe prior to an assay in order to insure it is sufficiently effective for the planned experiment [5]. This is typically done by preparing a homogeneous suspension of mutant cells with the probes, so that [M]=[CT]. The experimentalist will then perform cytometry with a sufficiently high concentration of free probes [N] and quantify the number of cells that contain any fluorescing probes. The solution of probes is subsequently diluted by some factor D and the assay is repeated dmax number of times. This process, known as serial dilution, arises in many applications from testing antibacterial agents [15] to quantifying viral infectivity [16]. In this context, it is used to find the characteristic dilution number dc such that all cells are still bound to at least one probe. The experimentalist can then use the corresponding probe concentration for the flow cytometry assay. However, having provided a kinetic model, we can employ this process to infer physical parameters of interest. To do so, we start by using Eq 2.13 to derive the concentration of the number of cells C∗ with one or more probes attached as
[C∗]=[CT]−[C0]=[CT]−[CT]∑I⟨I⟩Iexp(−⟨I⟩)I!(1+[N]KsDd)−I(1+[N]KnDd)−J=[CT][1−(1+[N]KnDd)−Jexp(−⟨I⟩[N]KsDd1+[N]KsDd)], | (3.3) |
where d is the dilution number. If we normalize [C∗] by the total concentration of cells [CT], then we can treat the expression as a probability that a given cell will fluoresce as a function of d, as shown in Figure 4(b). The placement of the characteristic drop in probability is dictated by the parameters Ks and ⟨I⟩. If we consider the data point C∗d as the number of cells that fluoresce at dilution d, then we expect its value to be binomial distributed and we can derive the likelihood function
L({C∗d})=dmax∏d=dmin(CTC∗d)[1−(1+[N]KnDd)−Jexp(−⟨I⟩[N]KsDd+[N]Ks)]C∗d[(1+[N]KnDd)−Jexp(−⟨I⟩[N]KsDd+[N]Ks)]CT−C∗d. | (3.4) |
For a given set of data {C∗d}, the log of the likelihood is a function of the parameters and can be maximized to solve for maximum likelihood estimates (MLE) of these parameters. As the original intent of the serial dilution procedure is to quantify the affinity of specific probe binding, Ks would be the desired inferred parameter. However, depending on the underlying experiment, one can envision estimating the expression of surface receptors ⟨I⟩ and its change under differing experimental environments.
A very common use of flow cytometry is in fluorescence activated cell sorting (FACS) in which cells are physically sorted into bins based on their species type [8,17]. As each cell is sent past the laser, the intensity measurement informs the computer in real-time which category the cell falls into. The droplet containing the cell exits an electrically charged ring that induces an electric charge in the droplet. An electric field controlled by the computer is then used to propel the extruded cell into the appropriate bin based on the fluorescence measurement. However, the confounding factors previously discussed can cause incorrect sorting of cells due to non-specific binding and other background fluorescence. If all parameters are a priori known, then using Eq 3.1 can technically be used to determine the cell species as the expression quantifies the probability that a cell is a mutant over a wild-type cell given its fluorescence. A resulting probability larger than 0.5 will indicate a mutant, making Eq 3.1 a decision function. However, there are two complications. One is that the evaluations of Eq 3.1 are relatively computationally intensive, especially if the expected number of receptors ⟨I⟩ is large. The real-time nature of the physical process of FACS requires rapid evaluation, though increasing computational resources can alleviate the problem. The second, more pertinent issue is that, though we are assuming all parameters are known, it is unlikely that the concentration of mutants [M] is a priori known. Biologists typically use cytometry assays after some experiment and the quantification of [M] is often the primary desired quantity still undetermined. Furthermore, our method of estimating [M] is a model-based clustering technique that leverages all data collectively, making real-time analysis problematic.
One potential solution for both problems is to use a two-pass cytometry method. One pass through the cytometer would be used to quantify the concentration of mutants [M] while also storing the evaluations of Eq 3.1. All cells would be collected together and reintroduced to the sheath fluid for a second pass for the FACS step. Though it would be improbable to exactly match each cell with their stored evaluation in the first pass, this extra data will act as a prior for more informed statistical sorting of the cells. Though previously discussed applications of our model use protocols already practiced by biologists, the potential overhead of using a two-pass cytometry process would be subject to the specific requirements of each experiment employing the method.
In this paper, we have created a full kinetic model of the specific and nonspecific binding dynamics of a cell/probe suspension at chemical equilibrium. Using a mass-action approach, we derived expressions for important equilibrium quantities as functions of physical and experimental parameters of the flow cytometry assay. The total number of afflicted cells, which we refer to as mutants, is often the primary desired quantity of the protocol as the probes are assumed to attach only to those cells. However, we show quantitatively how the nonspecific binding of probes to the membranes of both mutants and wild-type cells can confound the results. Furthermore, using the analogous Langmuir adsorption isotherm, we demonstrated how to choose probe concentration that will minimize these confounding effects. For the estimation of the total number of mutants in flow cytometry output, which is often subject to heuristic gating, we provided an iterative algorithm to obtain this number without input from the experimentalist. We claim that having a fundamental model for which the algorithm is based will increase the accuracy over other clustering attempts. Furthermore, we extract further utility from a serial dilution process often employed to measure the affinity of probes to infer true physical parameters of the cells. Lastly, we discuss the potential applications and issues with using our method for fluorescence activated cell sorting (FACS) while proposing a two-pass cytometry process to alleviate some of the problems.
Our model and analysis approach can be readily extended to include multiple probes, multiple specifically binding receptors, and more general distribution functions for receptor expression by the mutant cells. We expect that in such more complex, higher dimensional discrimination assays, our more systematic and quantitative analysis methods should provide more accurate results. Finally, we are developing a web based tool that fully implements our flow cytometry analysis procedure so that it can be applied to experimentally measured data. This will increase the accessibility of our model and enable quantitative comparisons with existing methods, including heuristic gating.
This research was supported by the National Science Foundation through grants DMS-1516675 and DMS-1814364 and the NIH through grant R01HL146552. We are especially grateful to Anupama Dimashkie and Dr. Saravanan Karumbayaram for insightful discussions.
The authors declare no conflict of interest in this manuscript.
[1] |
D. Y. Hsieh, On partial differential equations related to Lorenz system, J. Math. Phys., 28 (1987), 1589–1597. https://doi.org/10.1063/1.527465 doi: 10.1063/1.527465
![]() |
[2] |
Y. Kuramoto, T. Tsuzuki, On the formation of dissipative structures in reaction-diffusion systems, Prog. Theoret. Phys., 54 (1975), 687–699. https://doi.org/10.1143/PTP.54.687 doi: 10.1143/PTP.54.687
![]() |
[3] | S. Q. Tang, Dissipative Nonlinear Evolution Equations and Chaos, Ph.D Thesis, The Hong Kong University of Science and Technology in Hong Kong, 1995. |
[4] |
E. N. Lorenz, Deterministic nonperiodic flows, J. Atom. Sci., 20 (1963), 130–141. https://doi.org/10.1175/1520-0469(1963)020%3C0130:DNF%3E2.0.CO;2 doi: 10.1175/1520-0469(1963)020%3C0130:DNF%3E2.0.CO;2
![]() |
[5] |
D. Y. Hsieh, S. Q. Tang, Y. P Wang, L. X. Wu, Dissipative nonlinear evolution equations and chaos, Stud. Appl. Math., 101 (1998), 233–266. https://doi.org/10.1063/1.527465 doi: 10.1063/1.527465
![]() |
[6] |
S. Q. Tang, H. J. Zhao, Nonlinear stability for dissipative nonlinear evolution equations with ellipticity, J. Math. Anal. Appl., 233 (1999), 336–358. https://doi.org/10.1006/jmaa.1999.6316 doi: 10.1006/jmaa.1999.6316
![]() |
[7] |
R. J. Duan, C. J. Zhu, Asymptotics of dissipative nonlinear evolution equations with ellipticity: different end states, J. Math. Anal. Appl., 303 (2005), 15–35. https://doi.org/10.1016/j.jmaa.2004.06.007 doi: 10.1016/j.jmaa.2004.06.007
![]() |
[8] |
C. J. Zhu, Z. A. Wang, Decay rates of solutions to dissipative nonlinear equations with ellipticity, Z. Angew. Math. Phys., 55 (2004), 994–1014. https://doi.org/10.1007/s00033-004-3117-9 doi: 10.1007/s00033-004-3117-9
![]() |
[9] |
R. J. Duan, S. Q. Tang, C. J. Zhu, Asymptotics in nonlinear evolution system with dissipation and ellipticity on quadrant, J. Math. Anal. Appl., 323 (2006), 1152–1170. https://doi.org/10.1016/j.jmaa.2005.11.002 doi: 10.1016/j.jmaa.2005.11.002
![]() |
[10] |
L. Z. Ruan, C. J. Zhu, Boundary layer for nonlinear evolution equations with damping and diffusion, Disc. Cont. Dyna. Sys., 32 (2012), 331–352. https://doi.org/10.3934/dcds.2012.32.331 doi: 10.3934/dcds.2012.32.331
![]() |
[11] |
H. Y. Peng, L. Z. Ruan, J. L. Xiang, A note on boundary layer of a nonlinear evolution system with damping and diffusions, J. Math. Anal. Appl., 426 (2015), 1099–1129. https://doi.org/10.1016/j.jmaa.2015.01.053 doi: 10.1016/j.jmaa.2015.01.053
![]() |
[12] |
K. M. Chen, C. J. Zhu, The zero diffusion limit for nonlinear hyperbolic system with damping and diffusion, J. Hyper. Diff. Equation, 5 (2008), 767–783. https://doi.org/10.1142/S0219891608001696 doi: 10.1142/S0219891608001696
![]() |
[13] |
L. Z. Ruan, H. Y. Yin, Convergence rates of vanishing diffusion limit on nonlinear hyperbolic system with damping and diffusion, J. Math. Phys., 53 (2012), 103703. https://doi.org/10.1063/1.4751283 doi: 10.1063/1.4751283
![]() |
[14] |
L. Z. Ruan, Z. Y. Zhang, Global decaying solution to dissipative nonlinear evolution equations with ellipticity, Appl. Math. Comput., 217 (2011), 6054–6066. https://doi.org/10.1016/j.amc.2010.12.066 doi: 10.1016/j.amc.2010.12.066
![]() |
[15] |
K. Nishihara, Asymptotic profile of solutions to nonlinear dissipative evolution system with ellipticity, Z. Angew. Math. Phys., 57 (2006), 604–614. https://doi.org/10.1007/s00033-006-0062-9 doi: 10.1007/s00033-006-0062-9
![]() |
[16] |
Z. A. Wang, Optimal decay rates of solutions to dissipative nonlinear evolution equations with ellipticity, Z. Angew. Math. Phys., 57 (2006), 399-418. https://doi.org/10.1007/s00033-005-0030-9 doi: 10.1007/s00033-005-0030-9
![]() |
[17] | H. Schlichting, J. Kestin, R. L. Street, Boundary Layer Theory, 7th Edition, McGraw-Hill, London, 1979. |
[18] |
H. Frid, V. Shelukhin, Boundary layers for the Navier–Stokes equations of compressible fluids, Comm. Math. Phys., 208 (1999), 309–330. https://doi.org/10.1007/s002200050760 doi: 10.1007/s002200050760
![]() |
[19] |
X. L. Qin, T. Yang, Z. A. Yao, W. S. Zhou, Vanishing shear viscosity and boundary layer for the Navier–Stokes equations with cylindrical symmetry, Arch. Rational Mech. Anal., 216 (2015), 1049–1086. https://doi.org/10.1007/s00205-014-0826-x doi: 10.1007/s00205-014-0826-x
![]() |
[20] |
L. Yao, T. Zhang, C. J. Zhu, Boundary layers for compressible Navier–Stokes equations with density–dependent viscosity and cylindrical symmetry, Ann. Inst. H. Poincaré Anal. NonLinéaire., 28 (2011), 677–709. https://doi.org/10.1016/j.anihpc.2011.04.006 doi: 10.1016/j.anihpc.2011.04.006
![]() |
[21] |
S. Jiang, J. W. Zhang, Boundary layers for the Navier–Stokes equations of compressible heat–conducting flows with cylindrical symmetry, SIAM J. Math. Anal. 41 (2009), 237–268. https://doi.org/10.1137/07070005X doi: 10.1137/07070005X
![]() |
[22] |
Q. Q. Hou, Z. A. Wang, K. Zhao, Boundary layer problem on a hyperbolic system arising from chemotaxis, J. Differ. Equations, 261 (2016), 5035–5070. https://doi.org/10.1016/j.jde.2016.07.018 doi: 10.1016/j.jde.2016.07.018
![]() |
[23] |
M. Sammartino, R. Caflisch, Zero viscosity limit for analytic solutions of the Navier–Stokes equations on a half-space, I. Existence for Euler and Prandtl equations, Comm. Math. Phys., 192 (1998), 433–461. https://doi.org/10.1007/s002200050304 doi: 10.1007/s002200050304
![]() |
[24] | V. V. Shelukhin, A shock layer in parabolic perturbations of a scalar conservation law, in Proceedings of the Edinburgh Mathematical Society, 46 (2003), 315–328. https://doi.org/10.1017/S0013091502000548 |
[25] |
V. V. Shelukhin, The limit of zero shear viscosity for compressible fluids, Arch. Rational Mech. Anal., 143 (1998), 357–374. https://doi.org/10.1007/s002050050109 doi: 10.1007/s002050050109
![]() |
[26] |
H. Y. Wen, T. Yang, C. J. Zhu, Optimal convergence rate of the vanishing shear viscosity limit for compressible Navier–Stokes equations with cylindrical symmetry, J. Math. Pures Appl., 146 (2021), 99–126. https://doi.org/10.1016/j.matpur.2020.09.003 doi: 10.1016/j.matpur.2020.09.003
![]() |
[27] |
E. Grenier, O. Guès, Boundary layers for viscous perturbations of noncharacteristic quasilinear problems, J. Differ. Equations, 143 (1998), 110–146. https://doi.org/10.1006/jdeq.1997.3364 doi: 10.1006/jdeq.1997.3364
![]() |
[28] |
W. S. Zhou, X. L. Qin, C. Y. Qu, Zero shear viscosity limit and boundary layer for the Navier–Stokes equations of compressible fluids between two horizontal parallel plates, Nonlinearity, 28 (2015), 1721–1743. https://10.1088/0951-7715/28/6/1721 doi: 10.1088/0951-7715/28/6/1721
![]() |
[29] |
L. Hsiao, H. Y. Jian, Global smooth solutions to the spatially periodic Cauchy problem for dissipative nonlinear evolution equations, J. Math. Anal. Appl., 213 (1997), 262–274. https://doi.org/10.1006/jmaa.1997.5535 doi: 10.1006/jmaa.1997.5535
![]() |
[30] | D. L. Powers, Boundary Value Problems: and Partial Differential Equations, Academic Press, 2005. |
[31] |
H. Y. Jian, D. G. Chen, On the Cauchy problem for certain system of semilinear parabolic equation, Acta Math. Sin., 14 (1998), 27–34. https://doi.org/10.1007/BF02563880 doi: 10.1007/BF02563880
![]() |
[32] |
Z. A. Wang, Large time profile of solutions for a dissipative nonlinear evolution system with conservational form, J. Phys. A: Math. Gen., 38 (2005), 10955–10969. 10.1088/0305-4470/38/50/006 doi: 10.1088/0305-4470/38/50/006
![]() |
[33] |
W. Allegretto, Y. P. Lin, Z. Y. Zhang, Properties of global decaying solutions to the Cauchy problem of nonlinear evolution equations, Z. Angew. Math. Phys., 59 (2008), 848–868. https://doi.org/10.1007/s00033-008-7026-1 doi: 10.1007/s00033-008-7026-1
![]() |
[34] |
C. C. Chou, Y. F. Deng, Numerical solutions of a nonlinear evolution system with small dissipation on parallel processors, J. Sci. Comput., 13 (1998), 405–417. https://doi.org/10.1023/A:1023237317673 doi: 10.1023/A:1023237317673
![]() |
[35] |
L. Fan, N. T. Trouba, Convergence rates of vanishing diffusion limit on conservative form of Hsieh's equation, Stud. Appl. Math., 146 (2021), 753–776. https://doi.org/10.1111/sapm.12366 doi: 10.1111/sapm.12366
![]() |
[36] |
C. M. Dafermos, L. Hsiao, Global smooth thermomechanical processes in one-dimensional nonlinear thermoviscoelasticity, Nonlinear Anal., 6 (1982), 435–454. https://doi.org/10.1016/0362-546X(82)90058-X doi: 10.1016/0362-546X(82)90058-X
![]() |
[37] |
J. Simon, Nonhomogeneous viscous incompressible fluids: existence of velocity, density and pressure, SIAM J. Math. Anal., 21 (1990), 1093–1117. https://doi.org/10.1137/0521061 doi: 10.1137/0521061
![]() |
1. | Yue Wang, Bhaven A. Mistry, Tom Chou, Discrete stochastic models of SELEX: Aptamer capture probabilities and protocol optimization, 2022, 156, 0021-9606, 244103, 10.1063/5.0094307 |