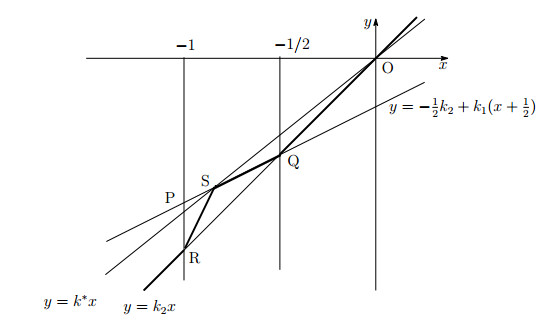
Vehicle routing problem (VRP) is a fundamental combinatorial optimization and integer programming problem with several important applications. The VRP is usually solved by using branch-and-bound techniques requiring solving a shortest path problem with resource constraints (SPPRC) and the determination of a lower bound, which can be computed by using column generation. The SPPRC entails finding the minimum cost elementary path in a valuated graph that is subject to constraints on resource consumption. The proposed exact solutions to this hard NP-hard problem require an excessive computation time which increases with the number of resources. In this paper, we propose a new approximate resolution of the SPPRC for acyclic and cyclic graphs. Our method is based on a Lagrangian relaxation of a subset of the constraints and using dominance only on a subset of the resources. This reduces the search space and allows users to efficiently compute solutions used to improve the column generation procedure. Extensive evaluation and comparison to the classical exact method show that the proposed algorithm achieves a good compromise between efficiency and quality of the SPPRC and the VRP solutions. Thus, our method can be used for practical large-scale VRP applications.
Citation: Abdelkader Lamamri, Mohammed Hachama. Approximate solution of the shortest path problem with resource constraints and applications to vehicle routing problems[J]. Electronic Research Archive, 2023, 31(2): 615-632. doi: 10.3934/era.2023030
[1] | Zhiyuan Wang, Chu Zhang, Shaopei Xue, Yinjie Luo, Jun Chen, Wei Wang, Xingchen Yan . Dynamic coordinated strategy for parking guidance in a mixed driving parking lot involving human-driven and autonomous vehicles. Electronic Research Archive, 2024, 32(1): 523-550. doi: 10.3934/era.2024026 |
[2] | Xiaoying Zheng, Jing Wu, Xiaofeng Li, Junjie Huang . UAV search coverage under priority of important targets based on multi-location domain decomposition. Electronic Research Archive, 2024, 32(4): 2491-2513. doi: 10.3934/era.2024115 |
[3] | Yu Shen, Hecheng Li . A multi-strategy genetic algorithm for solving multi-point dynamic aggregation problems with priority relationships of tasks. Electronic Research Archive, 2024, 32(1): 445-472. doi: 10.3934/era.2024022 |
[4] | Sida Lin, Lixia Meng, Jinlong Yuan, Changzhi Wu, An Li, Chongyang Liu, Jun Xie . Sequential adaptive switching time optimization technique for maximum hands-off control problems. Electronic Research Archive, 2024, 32(4): 2229-2250. doi: 10.3934/era.2024101 |
[5] | Ismail Ben Abdallah, Yassine Bouteraa, Saleh Mobayen, Omar Kahouli, Ali Aloui, Mouldi Ben Amara, Maher JEBALI . Fuzzy logic-based vehicle safety estimation using V2V communications and on-board embedded ROS-based architecture for safe traffic management system in hail city. Electronic Research Archive, 2023, 31(8): 5083-5103. doi: 10.3934/era.2023260 |
[6] | Jian Gong, Yuan Zhao, Jinde Cao, Wei Huang . Platoon-based collision-free control for connected and automated vehicles at non-signalized intersections. Electronic Research Archive, 2023, 31(4): 2149-2174. doi: 10.3934/era.2023111 |
[7] | Hao Li, Zhengwu Wang, Shuiwang Chen, Weiyao Xu, Lu Hu, Shuai Huang . Integrated optimization of planning and operation of a shared automated electric vehicle system considering the trip selection and opportunity cost. Electronic Research Archive, 2024, 32(1): 41-71. doi: 10.3934/era.2024003 |
[8] | Wenjie Wang, Suzhen Wen, Shen Gao, Pengyi Lin . A multi-objective dynamic vehicle routing optimization for fresh product distribution: A case study of Shenzhen. Electronic Research Archive, 2024, 32(4): 2897-2920. doi: 10.3934/era.2024132 |
[9] | Yineng Ouyang, Zhaotao Liang, Zhihui Ma, Lei Wang, Zhaohua Gong, Jun Xie, Kuikui Gao . A class of constrained optimal control problems arising in an immunotherapy cancer remission process. Electronic Research Archive, 2024, 32(10): 5868-5888. doi: 10.3934/era.2024271 |
[10] | Michael Barg, Amanda Mangum . Statistical analysis of numerical solutions to constrained phase separation problems. Electronic Research Archive, 2023, 31(1): 229-250. doi: 10.3934/era.2023012 |
Vehicle routing problem (VRP) is a fundamental combinatorial optimization and integer programming problem with several important applications. The VRP is usually solved by using branch-and-bound techniques requiring solving a shortest path problem with resource constraints (SPPRC) and the determination of a lower bound, which can be computed by using column generation. The SPPRC entails finding the minimum cost elementary path in a valuated graph that is subject to constraints on resource consumption. The proposed exact solutions to this hard NP-hard problem require an excessive computation time which increases with the number of resources. In this paper, we propose a new approximate resolution of the SPPRC for acyclic and cyclic graphs. Our method is based on a Lagrangian relaxation of a subset of the constraints and using dominance only on a subset of the resources. This reduces the search space and allows users to efficiently compute solutions used to improve the column generation procedure. Extensive evaluation and comparison to the classical exact method show that the proposed algorithm achieves a good compromise between efficiency and quality of the SPPRC and the VRP solutions. Thus, our method can be used for practical large-scale VRP applications.
We consider the system of Hamilton-Jacobi equations
{λu1(x)+H1(Du1(x))+B1(u1(x),u2(x))=0 in Tn,λu2(x)+H2(Du2(x))+B2(u1(x),u2(x))=0 in Tn, | (1.1) |
where λ>0 is a given constant, the functions Hi:Rn→R and Bi:R2→R, with i=1,2, are given continuous functions, and Tn denotes the n-dimensional flat torus Rn/Zn.
In a recent paper [6], the authors have investigated the vanishing discount problem for a nonlinear monotone system of Hamilton-Jacobi equations
{λu1(x)+G1(x,Du1(x),u1(x),u2(x),…,um(x))=0 in Tn,λu1(x)+G1(x,Du1(x),u1(x),u2(x)⋮λum(x)+Gm(x,Dum(x),u1(x),u2(x),…,um(x))=0 in Tn, | (1.2) |
and established under some hypotheses on the Gi∈C(Tn×Rn×Rm) that, when uλ=(uλ,1,…,uλ,m)∈C(Tn)m denoting the (viscosity) solution of (1.2), the whole family {uλ}λ>0 converges in C(Tn)m to some u0∈C(Tn)m as λ→0+. The constant λ>0 in the above system is the so-called discount factor.
The hypotheses on the system are the convexity, coercivity, and monotonicity of the Gi as well as the solvability of (1.2), with λ=0. Here the convexity of Gi is meant that the functions Rn×Rm∋(p,u)↦Gi(x,p,u) are convex. We refer to [6] for the precise statement of the hypotheses.
Prior to work [6], there have been many contributions to the question about the whole family convergence (in other words, the full convergence) under the vanishing discount, which we refer to [1,3,4,6,8,9,10] and the references therein.
In the case of the scalar equation, B. Ziliotto [11] has recently shown an example of the Hamilton-Jacobi equation having non-convex Hamiltonian in the gradient variable for which the full convergence does not hold. In Ziliotto's approach, the first step is to find a system of two algebraic equations
{λu+f(u−v)=0,λv+g(v−u)=0, | (1.3) |
with two unknowns u,v∈R and with a parameter λ>0 as the discount factor, for which the solutions (uλ,vλ) stay bounded and fail to fully converge as λ→0+. Here, an "algebraic" equation is meant not to be a functional equation. The second step is to interpolate the two values uλ and vλ to get a function of x∈T1 which satisfies a scalar non-convex Hamilton-Jacobi equation in T1.
In the first step above, Ziliotto constructs f,g based on a game-theoretical and computational argument, and the formula for f,g is of the minimax type and not quite explicit. In [5], the author has reexamined the system given by Ziliotto, with a slight generality, as a counterexample for the full convergence in the vanishing discount.
Our purpose in this paper is to present a system (1.3), with an explicit formula for f,g, for which the solution (uλ,vλ) does not fully converge to a single point in R2. A straightforward consequence is that (1.1), with B1(u1,u2)=f(u1−u2) and B2(u1,u2)=g(u2−u1), has a solution given by
(uλ,1(x),uλ.2(x))=(uλ,vλ) for x∈Tn, |
under the assumption that Hi(x,0)=0 for all x∈Tn, and therefore, gives an example of a discounted system of Hamilton-Jacobi equations, the solution of which fails to satisfy the full convergence as the discount factor goes to zero.
The paper consists of two sections. This introduction is followed by Section 2, the final section, which is divided into three subsections. The main results are stated in the first subsection of Section 2, the functions f,g, the key elements of (1.3), are contstructed in the second subsection, and the final subsection provides the proof of the main results.
Our main focus is now the system
{λu+f(u−v)=0λv+g(v−u)=0, | (2.1) |
where f,g∈C(R,R) are nondecreasing functions, to be constructed, and λ>0 is a constant, to be sent to zero. Notice that (2.1) above is referred as (1.3) in the previous section.
We remark that, due to the monotonicity assumption on f,g, the mapping (u,v)↦(f(u−v),g(v−u)),R2→R2 is monotone. Recall that, by definition, a mapping (u,v)↦(B1(u,v),B2(u,v)),R2→R2 is monotone if, whenever (u1,v1),(u2,v2)∈R2 satisfy u1−u2≥v1−v2 (resp., v1−v2≥u1−u2), we have B1(u1,v1)≥B1(u2,v2) (resp., B2(u1,v1)≥B2(u2,v2)).
Our main results are stated as follows.
Theorem 1. There exist two increasing functions f,g∈C(R,R) having the properties (a)–(c):
(a) For any λ>0 there exists a unique solution (uλ,vλ)∈R2 to (2.1),
(b) the family of the solutions (uλ,vλ) to (2.1), with λ>0, is bounded in R2,
(c) the family {(uλ,vλ)}λ>0 does not converge as λ→0+.
It should be noted that, as mentioned in the introduction, the above theorem has been somewhat implicitly established by Ziliotto [11]. In this note, we are interested in a simple and easy approach to finding functions f,g having the properties (a)–(c) in Theorem 1.
The following is an immediate consequence of the above theorem.
Corollary 2. Let Hi∈C(Rn,R), i=1,2, satisfy H1(0)=H2(0)=0. Let f,g∈C(R,R) be the functions given by Theorem 1, and set B1(u1,u2)=f(u1−u2) and B2(u1,u2)=g(u2−u1) for all (u1,u2)∈R2. For any λ>0, let (uλ,1,uλ,2) be the (viscosity) solution of (1.1). Then, the functions uλ,i are constants, the family of the points (uλ,1,uλ,2) in R2 is bounded, and it does not converge as λ→0+.
Notice that the convexity of Hi in the above corollary is irrelevant, and, for example, one may take Hi(p)=|p|2 for i∈I, which are convex functions.
We remark that a claim similar to Corollary 2 is valid when one replaces Hi(p) by degenerate elliptic operators Fi(x,p,M) as far as Fi(x,0,0)=0, where M is the variable corresponding to the Hessian matrices of unknown functions. (See [2] for an overview on the viscosity solution approach to fully nonlinear degenerate elliptic equations.)
If f,g are given and (u,v)∈R2 is a solution of (2.1), then w:=u−v satisfies
λw+f(w)−g(−w)=0. | (2.2) |
Set
h(r)=f(r)−g(−r) for r∈R, | (2.3) |
which defines a continuous and nondecreasing function on R.
To build a triple of functions f,g,h, we need to find two of them in view of the relation (2.3). We begin by defining function h.
For this, we discuss a simple geometry on xy-plane as depicted in Figure 1 below. Fix 0<k1<k2. The line y=−12k2+k1(x+12) has slope k1 and crosses the lines x=−1 and y=k2x at P:=(−1,−12(k1+k2)) and Q:=(−12,−12k2), respectively, while the line y=k2x meets the lines x=−1 and x=−12 at R:=(−1,−k2) and Q=(−12,−12k2), respectively.
Choose k∗>0 so that 12(k1+k2)<k∗<k2. The line y=k∗x crosses the line y=−12k2+k1(x+12) at a point S:=(x∗,y∗) in the open line segment between the points P=(−12,−12(k1+k2)) and Q=(−12,−12k2). The line connecting R=(−1,−k2) and S=(x∗,y∗) can be represented by y=−k2+k+(x+1), with k+:=y∗+k2x∗+1>k2.
We set
ψ(x)={k2x for x∈(−∞,−1]∪[−1/2,∞),min{−k2+k+(x+1),−12k2+k1(x+12)} for x∈(−1,−12). |
It is clear that ψ∈C(R) and increasing on R. The building blocks of the graph y=ψ(x) are three lines whose slopes are k1<k2<k+. Hence, if x1>x2, then ψ(x1)−ψ(x2)≥k1(x1−x2), that is, the function x↦ψ(x)−k1x is nondecreasing on R.
Next, we set for j∈N,
ψj(x)=2−jψ(2jx) for x∈R. |
It is clear that for all j∈N, ψj∈C(R), the function x↦ψj(x)−k1x is nondecreasing on R, and
ψj(x){>k2x for all x∈(−2−j,−2−j−1),=k2x otherwise. |
We set
η(x)=maxj∈Nψj(x) for x∈R. |
It is clear that η∈C(R) and x↦η(x)−k1x is nondecreasing on R. Moreover, we see that
η(x)=k2x for all x∈(−∞,−12]∪[0,∞), |
and that if −2−j<x<−2−j−1 and j∈N,
η(x)=ψj(x)>k2x. |
Note that the point S=(x∗,y∗) is on the graph y=ψ(x) and, hence, that for any j∈N, the point (2−jx∗,2−jy∗) is on the graph y=η(x). Similarly, since the point S=(x∗,y∗) is on the graph y=k∗x and for any j∈N, the point (2−jx∗,2−jy∗) is on the graph y=k∗x. Also, for any j∈N, the point (−2−j,−k22−j) lies on the graphs y=η(x) and y=k2x.
Fix any d≥1 and define h∈C(R) by
h(x)=η(x−d). |
For the function h defined above, we consider the problem
λz+h(z)=0. | (2.4) |
Lemma 3. For any λ≥0, there exists a unique solution zλ∈R of (2.4).
Proof. Fix λ≥0. The function x↦h(x)+λx is increasing on R and satisfies
limx→∞(h(x)+λx)=∞ and limx→−∞(h(x)+λx)=−∞. |
Hence, there is a unique solution of (2.4).
For any λ≥0, we denote by zλ the unique solution of (2.4). Since h(d)=0, it is clear that z0=d.
For later use, observe that if λ>0, k>0, and (z,w)∈R2 is the point of the intersection of two lines y=−λx and y=k(x−d), then w=−λz=k(z−d) and
z=kdk+λ. | (2.5) |
Lemma 4. There are sequences {μj} and {νj} of positive numbers converging to zero such that
zμj=k2dk2+μj and zνj=k∗dk∗+νj. |
Proof. Let j∈N. Since (−2−j,−k22−j) is on the intersection of the graphs y=k2x and y=η(x), it follows that (−2−j+d,−k22−j) is on the intersection of the graphs y=k2(x−d) and y=h(x). Set
μj=k22−jd−2−j, | (2.6) |
and note that μj>0 and that
−μj(d−2−j)=−k22−j, |
which says that the point (d−2−j,−k22−j) is on the line y=−μjx. Combining the above with
−k22−j=h(d−2−j) |
shows that d−2−j is the unique solution of (2.4). Also, since (d−2−j,−μj(d−2−j))=(d−2−j,−k22−j) is on the line y=k2(x−d), we find by (2.5) that
zμj=k2dk2+μj. |
Similarly, since (2−jx∗,2−jy∗) is on the intersection of the graphs y=k∗x and y=η(x), we deduce that if we set
νj:=−2−jy∗d+2−jx∗=2−j|y∗|d−2−j|x∗|, | (2.7) |
then
zνj=k∗dk∗+νj. |
It is obvious by (2.6) and (2.7) that the sequences {μj}j∈N and {νj}j∈N are decreasing and converge to zero.
We fix k0∈(0,k1) and define f,g∈C(R) by f(x)=k0(x−d) and
g(x)=f(−x)−h(−x). |
It is easily checked that g(x)−(k1−k0)x is nondecreasing on R, which implies that g is increasing on R, and that h(x)=f(x)−g(−x) for all x∈R. We note that
f(d)=h(d)=g(−d)=0. | (2.8) |
We fix f,g,h as above, and consider the system (2.1).
Lemma 5. Let λ>0. There exists a unique solution of (2.1).
The validity of the above lemma is well-known, but for the reader's convenience, we provide a proof of the lemma above.
Proof. By choice of f,g, the functions f,g are nondecreasing on R. We show first the comparison claim: if (u1,v1),(u2,v2)∈R2 satisfy
λu1+f(u1−v1)≤0,λv1+g(v1−u1)≤0, | (2.9) |
λu2+f(u2−v2)≥0,λv2+g(v2−u2)≥0, | (2.10) |
then u1≤u2 and v1≤v2. Indeed, contrary to this, we suppose that max{u1−u2,v1−v2}>0. For instance, if max{u1−u2,v1−v2}=u1−u2, then we have u1−v1≥u2−v2 and u1>u2, and moreover
0≥λu1+f(u1−v1)≥λu1+f(u2−v2)>λu2+f(u2−v2), |
yielding a contradiction. The other case when max{u1−u2,v1−v2}=v1−v2, we find a contradiction, 0>λv2+g(v2−u2), proving the comparison.
From the comparison claim, the uniqueness of the solutions of (2.1) follows readily.
Next, we may choose a constant C>0 so large that (u1,v1)=(−C,−C) and (u2,v2)=(C,C) satisfy (2.9) and (2.10), respectively. We write S for the set of all (u1,u2)∈R2 such that (2.9) hold. Note that (−C,−C)∈S and that for any (u,v)∈S, u≤C and v≤C. We set
u∗=sup{u:(u,v)∈S for some v},v∗=sup{v:(u,v)∈S for some u}. |
It follows that −C≤u∗,v∗≤C. We can choose sequences
{(u1n,v1n)}n∈N,{(u2n,v2n)}n∈N⊂S |
such that {u1n},{v2n} are nondecreasing,
limn→∞u1n=u∗ and limn→∞v2n=v∗. |
Observe that for all n∈N, u2n≤u∗, v1n≤v∗, and
0≥λu1n+f(u1n−v1n)≥λu1n+f(u1n−v∗), |
which yields, in the limit as n→∞,
0≥λu∗+f(u∗−v∗). |
Similarly, we obtain 0≥λv∗+g(v∗−u∗). Hence, we find that (u∗,v∗)∈S.
We claim that (u∗,v∗) is a solution of (2.1). Otherwise, we have
0>λu∗+f(u∗−v∗) or 0>λv∗+g(v∗−u∗). |
For instance, if the former of the above inequalities holds, we can choose ε>0, by the continuity of f, so that
0>λ(u∗+ε)+f(u∗+ε−v∗). |
Since (u∗,v∗)∈S, we have
0≥λv∗+g(v∗−u∗)≥λv∗+g(v∗−u∗−ε). |
Accordingly, we find that (u∗+ε,v∗)∈S, which contradicts the definition of u∗. Similarly, if 0>λv∗+g(v∗−u∗), then we can choose δ>0 so that (u∗,v∗+δ)∈S, which is a contradiction. Thus, we conclude that (u∗,v∗) is a solution of (2.1).
Theorem 6. For any λ>0, let (uλ,vλ) denote the unique solution of (2.1). Let {μj},{νj} be the sequences of positive numbers from Lemma 2.4. Then
limj→∞uμj=k0dk2 and limj→∞uνj=k0dk∗. |
In particular,
lim infλ→0uλ≤k0dk2<k0dk∗≤lim supλ→0uλ. |
With our choice of f,g, the family of solutions (uλ,vλ) of (2.1), with λ>0, does not converge as λ→0.
Proof. If we set zλ=uλ−vλ, then zλ satisfies (2.4). By Lemma 4, we find that
zμj=k2dk2+μj and zνj=k∗dk∗+νj. |
Since uλ satisfies
0=λuλ+f(zλ)=λuλ+k0(zλ−d), |
we find that
uμj=−k0(zμj−d)μj=−k0dμj(k2k2+μj−1)=−k0dμj−μjk2+μj=k0dk2+μj, |
which shows that
limj→∞uμj=k0dk2. |
A parallel computation shows that
limj→∞uνj=k0dk∗. |
Recalling that 0<k∗<k2, we conclude that
lim infλ→0uλ≤k0dk2<k0dk∗≤lim supλ→0uλ. |
We remark that, since
limλ→0zλ=d and vλ=uλ−zλ, |
limj→∞vμj=k0dk2−d and limj→∞vνj=k0dk∗−d. |
We give the proof of Theorem 1.
Proof of Theorem 1. Assertions (a) and (c) are consequences of Lemma 5 and Theorem 6, respectively.
Recall (2.8). That is, we have f(d)=h(d)=g(−d)=0. Setting (u2,v2)=(d,0), we compute that for any λ>0,
λu2+f(u2−v2)>f(d)=0 and λv2+g(v2−u2)=g(−d)=0. |
By the comparison claim, proved in the proof of Lemma 5, we find that uλ≤d and vλ≤0 for any λ>0. Similarly, setting (u1,v1)=(0,−d), we find that for any λ>0,
λu1+f(u1−v1)=f(d)=0 and λv1+g(v1−u1)≤g(v1−u1)=g(−d)=0, |
which shows by the comparison claim that uλ≥0 and vλ≥−d for any λ>0. Thus, the sequence {(uλ,vλ)}λ>0 is bounded in R2, which proves assertion (b).
Proof of Corollary 2. For any λ>0, let (uλ,vλ)∈R2 be the unique solution of (2.1). Since H1(0)=H2(0)=0, it is clear that the constant function (uλ,1(x),uλ,2(x)):=(uλ,vλ) is a classical solution of (1.1). By a classical uniqueness result (see, for instance, [7,Theorem 4.7]), (uλ,1,uλ,2) is a unique viscosity solution of (1.1). The rest of the claims in Corollary 2 is an immediate consequence of Theorem 1.
Some remarks are in order. (ⅰ) Following [11], we may use Theorem 6 as the primary cornerstone for building a scalar Hamilton-Jacobi equation, for which the vanishing discount problem fails to have the full convergence as the discount factor goes to zero.
(ⅱ) In the construction of the functions f,g∈C(R,R) in Theorem 6, the author has chosen d to satisfy d≥1, but, in fact, one may choose any d>0. In the proof, the core step is to find the function h(x)=f(x)−g(−x), with the properties: (a) the function x↦h(x)−εx is nondecreasing on R for some ε>0 and (b) the curve y=h(x), with x<d, meets the lines y=p(x−d) and y=q(x−d), respectively, at Pj and Qj for all j∈N, where p,q,d are positive constants such that ε<p<q, and the sequences {Pj}j∈N,{Qj}j∈N converge to the point (d,0). Obviously, such a function h is never left-differentiable at x=d nor convex in any neighborhood of x=d. Because of this, it seems difficult to select f,g∈C(R,R) in Theorem 1, both smooth everywhere. In the proof of Theorem 6, we have chosen ε=k0, p=k∗, q=k2, Pj=(uνj,k∗(uνj−d)), and Qj=(uμj,k2(uμj−d))
Another possible choice of h among many other ways is the following. Define first η:R→R by η(x)=x(sin(log|x|)+2) if x≠0, and η(0)=0 (see Figure 2). Fix d>0 and set h(x)=η(x−d) for x∈R. we remark that η∈C∞(R∖{0}) and h∈C∞(R∖{d}). Note that if x≠0,
η′(x)=sin(log|x|)+cos(log|x|)+2∈[2−√2,2+√2], |
and that if we set xj=−exp(−2πj) and ξj=−exp(−2πj+π2), j∈N, then
η(xj)=2xj and η(ξj)=3ξj. |
The points Pj:=(xj+d,2xj) are on the intersection of two curves y=h(x) and y=2(x−d), while the points Qj:=(d+ξj,3ξj) are on the intersection of y=h(x) and y=3(x−d). Moreover, limPj=limQj=(d,0).
The author would like to thank the anonymous referees for their careful reading and useful suggestions. He was supported in part by the JSPS Grants KAKENHI No. 16H03948, No. 20K03688, No. 20H01817, and No. 21H00717.
The author declares no conflict of interest.
[1] |
G. B. Dantzig, J. H. Ramser, The truck dispatching problem, Manage. Sci., 6 (1959), 80–91. https://doi.org/10.1287/mnsc.6.1.80 doi: 10.1287/mnsc.6.1.80
![]() |
[2] |
M. Desrochers, J. Desrosiers, M. Solomon, A new optimization algorithm for the vehicle routing problem with time windows, Oper. Res., 40 (1982), 342–354. https://doi.org/10.1287/opre.40.2.342 doi: 10.1287/opre.40.2.342
![]() |
[3] |
J. Desrosiers, Y. Dumas, M. M. Solomon, F. Soumis, Time constrained routing and scheduling, Handbooks Oper. Res. Manage. Sci., 8 (1995), 35–139. https://doi.org/10.1016/S0927-0507(05)80106-9 doi: 10.1016/S0927-0507(05)80106-9
![]() |
[4] |
N. Kohl, J. Desrosiers, O. B. Madsen, M. M. Solomon, F. Soumis, 2-path cuts for the vehicle routing problem with time windows, Transp. Sci., 33 (1999), 101–116. https://doi.org/10.1287/trsc.33.1.101 doi: 10.1287/trsc.33.1.101
![]() |
[5] |
S. Irnich, D. Villeneuve, The shortest-path problem with resource constraints and k-cycle elimination for k ≥ 3, INFORMS J. Comput., 18 (2006), 391–406. https://doi.org/10.1287/ijoc.1040.0117 doi: 10.1287/ijoc.1040.0117
![]() |
[6] |
R. Sadykov, E. Uchoa, A. Pessoa, A bucket graph-based labeling algorithm with application to vehicle routing, Transp. Sci., 55 (2021), 4–28. https://doi.org/10.1287/trsc.2020.0985 doi: 10.1287/trsc.2020.0985
![]() |
[7] |
R. Baldacci, E. Bartolini, A. Mingozzi, R. Roberti, An exact solution framework for a broad class of vehicle routing problems, Comput. Manage. Sci., 7 (2010), 229–268. https://doi.org/10.1007/s10287-009-0118-3 doi: 10.1007/s10287-009-0118-3
![]() |
[8] |
R. Fukasawa, Q. He, Y. Song, A branch-cut-and-price algorithm for the energy minimization vehicle routing problem, Transp. Sci., 50 (2016), 23–34. https://doi.org/10.1287/trsc.2015.0593 doi: 10.1287/trsc.2015.0593
![]() |
[9] |
D. Pecin, A. Pessoa, M. Poggi, E. Uchoa, Improved branch-cut-and-price for capacitated vehicle routing, Math. Program. Comput., 9 (2017), 61–100. https://doi.org/10.1007/s12532-016-0108-8 doi: 10.1007/s12532-016-0108-8
![]() |
[10] |
R. Fukasawa, H. Longo, J. Lysgaard, M. P. D. Aragão, M. Reis, E. Uchoa, et al., Robust branch-and-cut-and-price for the capacitated vehicle routing problem, Math. Program., 106 (2006), 491–511. https://doi.org/10.1007/s10107-005-0644-x doi: 10.1007/s10107-005-0644-x
![]() |
[11] |
R. Baldacci, N. Christofides, A. Mingozzi, An exact algorithm for the vehicle routing problem based on the set partitioning formulation with additional cuts, Math. Program., 115 (2008), 351–385. https://doi.org/10.1007/s10107-007-0178-5 doi: 10.1007/s10107-007-0178-5
![]() |
[12] |
R. Baldacci, A. Mingozzi, R. Roberti, New route relaxation and pricing strategies for the vehicle routing problem, Oper. Res., 59 (2011), 1269–1283. https://doi.org/10.1287/opre.1110.0975 doi: 10.1287/opre.1110.0975
![]() |
[13] |
S. Dabia, S. Ropke, T. V. Woensel, T. D. Kok, Branch and price for the time-dependent vehicle routing problem with time windows, Transp. Sci., 47 (2013), 380–396. https://doi.org/10.1287/trsc.1120.0445 doi: 10.1287/trsc.1120.0445
![]() |
[14] |
A. Nagih, F. Soumis, Nodal aggregation of resource constraints in a shortest path problem, Eur. J. Oper. Res., 172 (2006), 500–514. https://doi.org/10.1016/j.ejor.2004.09.052 doi: 10.1016/j.ejor.2004.09.052
![]() |
[15] |
I. Himmich, H. B. Amor, I. E. Hallaoui, F. Soumis, A primal adjacency-based algorithm for the shortest path problem with resource constraints, Transp. Sci., 54 (2020), 1153–1169. https://doi.org/10.1287/trsc.2019.0941 doi: 10.1287/trsc.2019.0941
![]() |
[16] |
M. Behnke, T. Kirschstein, C. Bierwirth, A column generation approach for an emission-oriented vehicle routing problem on a multigraph, Eur. J. Oper. Res., 288 (2021), 794–809. https://doi.org/10.1016/j.ejor.2020.06.035 doi: 10.1016/j.ejor.2020.06.035
![]() |
[17] | I. Mathlouthi, M. Gendreau, J. Y. Potvin, Branch-and-price for a multi-attribute technician routing and scheduling problem, in Operations Research Forum, Springer International Publishing, 2 (2021), 1–35. https://doi.org/10.1007/s43069-020-00044-x |
[18] |
S. Y. Tan, W. C. Yeh, The vehicle routing problem: State-of-the-art classification and review, Appl. Sci., 11 (2021), 10295. https://doi.org/10.3390/app112110295 doi: 10.3390/app112110295
![]() |
[19] | P. Toth, D. Vigo, Vehicle Routing: Problems, Methods, and Applications, SIAM, 2014. |
[20] |
L. Taccari, Integer programming formulations for the elementary shortest path problem, Eur. J. Oper. Res., 252 (2016), 122–130. https://doi.org/10.1016/j.ejor.2016.01.003 doi: 10.1016/j.ejor.2016.01.003
![]() |
[21] |
E. Manousakis, P. Repoussis, E. Zachariadis, C. Tarantilis, Improved branch-and-cut for the inventory routing problem based on a two-commodity flow formulation, Eur. J. Oper. Res., 290 (2021), 870–885. https://doi.org/10.1016/j.ejor.2020.08.047 doi: 10.1016/j.ejor.2020.08.047
![]() |
[22] |
G. Lera-Romero, J. J. Miranda-Bront, A branch and cut algorithm for the time-dependent profitable tour problem with resource constraints, Eur. J. Oper. Res., 289 (2021), 879–896. https://doi.org/10.1016/j.ejor.2019.07.014 doi: 10.1016/j.ejor.2019.07.014
![]() |
[23] |
C. M. Damião, J. M. P. Silva, E. Uchoa, A branch-cut-and-price algorithm for the cumulative capacitated vehicle routing problem, 4OR-Q. J. Oper. Res., 2021 (2021), 1–25. https://doi.org/10.1007/s10288-021-00498-7 doi: 10.1007/s10288-021-00498-7
![]() |
[24] |
H. B. Ticha, N. Absi, D. Feillet, A. Quilliot, Empirical analysis for the VRPTW with a multigraph representation for the road network, Comput. Oper. Res., 88 (2017), 103–116. https://doi.org/10.1016/j.cor.2017.06.024 doi: 10.1016/j.cor.2017.06.024
![]() |
[25] |
H. B. Ticha, N. Absi, D. Feillet, A. Quilliot, Vehicle routing problems with road-network information: State of the art, Networks, 72 (2018), 393–406. https://doi.org/10.1002/net.21808 doi: 10.1002/net.21808
![]() |
[26] |
H. B. Ticha, N. Absi, D. Feillet, A. Quilliot, T. V. Woensel, A branch-and-price algorithm for the vehicle routing problem with time windows on a road network, Networks, 73 (2019), 401–417. https://doi.org/10.1002/net.21852 doi: 10.1002/net.21852
![]() |
[27] |
C. Archetti, M. G. Speranza, A survey on matheuristics for routing problems, EURO J. Comput. Optim., 2 (2014), 223–246. https://doi.org/10.1007/s13675-014-0030-7 doi: 10.1007/s13675-014-0030-7
![]() |
[28] |
D. Pecin, C. Contardo, G. Desaulniers, E. Uchoa, New enhancements for the exact solution of the vehicle routing problem with time windows, INFORMS J. Comput., 29 (2017), 489–502. https://doi.org/10.1287/ijoc.2016.0744 doi: 10.1287/ijoc.2016.0744
![]() |
[29] | G. Desaulniers, J. Desrosiers, M. M. Solomon, Column Generation, Springer Science & Business Media, 2006. |
[30] |
M. E. Lübbecke, J. Desrosiers, Selected topics in column generation, Oper. Res., 53 (2005), 1007–1023. https://doi.org/10.1287/opre.1050.0234 doi: 10.1287/opre.1050.0234
![]() |
[31] | M. Desrochers, La fabrication d'horaires de travail pour les conducteurs d'autobus par une méthode de génération de colonnes, Université de Montréal, Centre de recherche sur les transports, 1986. |
[32] |
M. Desrochers, F. Soumis, A reoptimization algorithm for the shortest path problem with time windows, Eur. J. Oper. Res., 35 (1988), 242–254. https://doi.org/10.1016/0377-2217(88)90034-3 doi: 10.1016/0377-2217(88)90034-3
![]() |
[33] |
G. Desaulniers, D. Villeneuve, The shortest path problem with time windows and linear waiting costs, Transp. Sci., 34 (2000), 312–319. https://doi.org/10.1287/trsc.34.3.312.12298 doi: 10.1287/trsc.34.3.312.12298
![]() |
[34] |
D. Feillet, P. Dejax, M. Gendreau, C. Gueguen, An exact algorithm for the elementary shortest path problem with resource constraints: Application to some vehicle routing problems, Networks: Int. J., 44 (2004), 216–229. https://doi.org/10.1002/net.20033 doi: 10.1002/net.20033
![]() |
[35] |
G. Righini, M. Salani, Symmetry helps: Bounded bi-directional dynamic programming for the elementary shortest path problem with resource constraints, Discrete Optim., 3 (2006), 255–273. https://doi.org/10.1016/j.disopt.2006.05.007 doi: 10.1016/j.disopt.2006.05.007
![]() |
[36] |
A. Chabrier, Vehicle routing problem with elementary shortest path based column generation, Comput. Oper. Res., 33 (2006), 2972–2990. https://doi.org/10.1016/j.cor.2005.02.029 doi: 10.1016/j.cor.2005.02.029
![]() |
[37] |
D. Feillet, M. Gendreau, L. M. Rousseau, New refinements for the solution of vehicle routing problems with branch and price, INFOR: Inf. Syst. Oper. Res., 45 (2007), 239–256. https://doi.org/10.3138/infor.45.4.239 doi: 10.3138/infor.45.4.239
![]() |
[38] |
M. Tagmouti, M. Gendreau, J. Y. Potvin, Arc routing problems with time-dependent service costs, Eur. J. Oper. Res., 181 (2007), 30–39. https://doi.org/10.1016/j.ejor.2006.06.028 doi: 10.1016/j.ejor.2006.06.028
![]() |
[39] |
M. Jepsen, B. Petersen, S. Spoorendonk, D. Pisinger, Subset-row inequalities applied to the vehicle-routing problem with time windows, Oper. Res., 56 (2008), 497–511. https://doi.org/10.1287/opre.1070.0449 doi: 10.1287/opre.1070.0449
![]() |
[40] |
G. Righini, M. Salani, New dynamic programming algorithms for the resource constrained elementary shortest path problem, Networks: Int. J., 51 (2008), 155–170. https://doi.org/10.1002/net.20212 doi: 10.1002/net.20212
![]() |
[41] |
G. Desaulniers, F. Lessard, A. Hadjar, Tabu search, partial elementarity, and generalized k-path inequalities for the vehicle routing problem with time windows, Transp. Sci., 42 (2008), 387–404. https://doi.org/10.1287/trsc.1070.0223 doi: 10.1287/trsc.1070.0223
![]() |
[42] |
A. Qureshi, E. Taniguchi, T. Yamada, An exact solution approach for vehicle routing and scheduling problems with soft time windows, Transp. Res. Part E Logist. Transp. Rev., 45 (2009), 960–977. https://doi.org/10.1016/j.tre.2009.04.007 doi: 10.1016/j.tre.2009.04.007
![]() |
[43] |
A. Bettinelli, A. Ceselli, G. Righini, A branch-and-cut-and-price algorithm for the multi-depot heterogeneous vehicle routing problem with time windows, Transp. Res. Part C Emerging Technol., 19 (2011), 723–740. https://doi.org/10.1016/j.trc.2010.07.008 doi: 10.1016/j.trc.2010.07.008
![]() |
[44] |
F. Liberatore, G. Righini, M. Salani, A column generation algorithm for the vehicle routing problem with soft time windows, 4OR, 9 (2011), 49–82. https://doi.org/10.1007/s10288-010-0136-6 doi: 10.1007/s10288-010-0136-6
![]() |
[45] |
D. Duque, L. Lozano, A. L. Medaglia, Solving the orienteering problem with time windows via the pulse framework, Comput. Oper. Res., 54 (2015), 168–176. https://doi.org/10.1016/j.cor.2014.08.019 doi: 10.1016/j.cor.2014.08.019
![]() |
[46] |
L. Lozano, D. Duque, A. L. Medaglia, An exact algorithm for the elementary shortest path problem with resource constraints, Transp. Sci., 50 (2016), 348–357. https://doi.org/10.1287/trsc.2014.0582 doi: 10.1287/trsc.2014.0582
![]() |
[47] |
G. Lera-Romero, J. J. Miranda-Bront, Integer programming formulations for the time-dependent elementary shortest path problem with resource constraints, Electron. Notes Discrete Math., 69 (2018), 53–60. https://doi.org/10.1016/j.endm.2018.07.008 doi: 10.1016/j.endm.2018.07.008
![]() |
[48] |
K. Dalmeijer, G. Desaulniers, Addressing orientation symmetry in the time window assignment vehicle routing problem, INFORMS J. Comput., 33 (2021), 495–510. https://doi.org/10.1287/ijoc.2020.0974 doi: 10.1287/ijoc.2020.0974
![]() |
[49] |
D. Taş, Electric vehicle routing with flexible time windows: a column generation solution approach, Transp. Lett., 13 (2021), 97–103. https://doi.org/10.1080/19427867.2020.1711581 doi: 10.1080/19427867.2020.1711581
![]() |
[50] | M. Gendreau, J. Y. Potvin, O. Bräumlaysy, G. Hasle, A. Løkketangen, {Metaheuristics for the vehicle routing problem and its extensions: A categorized bibliography}, in The Vehicle Routing Problem: Latest Advances and New Challenges, Springer US, Boston, MA, (2008), 143–169. https://doi.org/10.1007/978-0-387-77778-8_7 |
[51] |
J. Pasha, A. L. Nwodu, A. M. Fathollahi-Fard, G. Tian, Z. Li, H. Wang, et al., Exact and metaheuristic algorithms for the vehicle routing problem with a factory-in-a-box in multi-objective settings, Adv. Eng. Inf., 52 (2022), 101623. https://doi.org/10.1016/j.aei.2022.101623 doi: 10.1016/j.aei.2022.101623
![]() |
[52] |
H. Park, D. Son, B. Koo, B. Jeong, Waiting strategy for the vehicle routing problem with simultaneous pickup and delivery using genetic algorithm, Expert Syst. Appl., 165 (2021), 113959. https://doi.org/10.1016/j.eswa.2020.113959 doi: 10.1016/j.eswa.2020.113959
![]() |
[53] |
H. Fan, Y. Zhang, P. Tian, Y. Lv, H. Fan, Time-dependent multi-depot green vehicle routing problem with time windows considering temporal-spatial distance, Comput. Oper. Res., 129 (2021), 105211. https://doi.org/10.1016/j.cor.2021.105211 doi: 10.1016/j.cor.2021.105211
![]() |
[54] |
G. Srivastava, A. Singh, R. Mallipeddi, Nsga-ii with objective-specific variation operators for multiobjective vehicle routing problem with time windows, Expert Syst. Appl., 176 (2021), 114779. https://doi.org/10.1016/j.eswa.2021.114779 doi: 10.1016/j.eswa.2021.114779
![]() |
[55] |
W. C. Yeh, S. Y. Tan, Simplified swarm optimization for the heterogeneous fleet vehicle routing problem with time-varying continuous speed function, Electronics, 10 (2021). https://doi.org/10.3390/electronics10151775 doi: 10.3390/electronics10151775
![]() |
[56] |
M. A. Nguyen, G. T. Dang, M. H. Hà, M. T. Pham, The min-cost parallel drone scheduling vehicle routing problem, Eur. J. Oper. Res., 299 (2022), 910–930. https://doi.org/10.1016/j.ejor.2021.07.008 doi: 10.1016/j.ejor.2021.07.008
![]() |
[57] |
P. Sun, L. P. Veelenturf, S. Dabia, T. V. Woensel, The time-dependent capacitated profitable tour problem with time windows and precedence constraints, Eur. J. Oper. Res., 264 (2018), 1058–1073. https://doi.org/10.1016/j.ejor.2017.07.004 doi: 10.1016/j.ejor.2017.07.004
![]() |
[58] |
P. Sun, L. P. Veelenturf, M. Hewitt, T. V. Woensel, The time-dependent pickup and delivery problem with time windows, Transp. Res. Part B Methodol., 116 (2018), 1–24. https://doi.org/10.1016/j.trb.2018.07.002 doi: 10.1016/j.trb.2018.07.002
![]() |
[59] | M. M. Solomon, Vehicle Routing and Scheduling with Time Window Constraints: Models and Algorithms (Heuristics), PhD thesis, University of Pennsylvania, 1984. |
[60] |
G. Clarke, J. W. Wright, Scheduling of vehicles from a central depot to a number of delivery points, Oper. Res., 12 (1964), 568–581. https://doi.org/10.1287/opre.12.4.568 doi: 10.1287/opre.12.4.568
![]() |
1. | Yuandong Chen, Jinhao Pang, Yuchen Gou, Zhiming Lin, Shaofeng Zheng, Dewang Chen, Research on the A* Algorithm for Automatic Guided Vehicles in Large-Scale Maps, 2024, 14, 2076-3417, 10097, 10.3390/app142210097 |