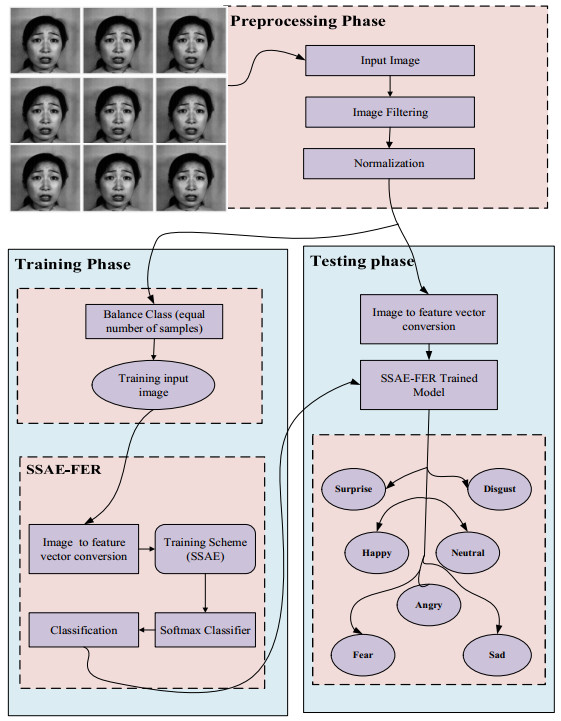
The development of urban digital inclusive finance has a complex network connection, and the characteristics of this network affect urban innovation and entrepreneurship. By using 287 cities from 2015 to 2019 in China, this paper constructs the network of Chinese digital inclusive finance, extracting two typical network characteristics. And it further studies the impact of the two network characteristics on innovation and entrepreneurship in various cities. The empirical results show that: first, the network centrality characteristics of digital inclusive finance have a significant promoting effect on urban innovation and entrepreneurship; second, the impact of the network centrality characteristics of digital inclusive finance is related to the speed of industrial structure transformation, but the impact mechanism is related to the node number instead of the node strength; third, the impact of the network centrality characteristics on innovation and entrepreneurship exists heterogeneity among cities and the moderately developed cities have the largest impact.
Citation: Zhenghui Li, Jinhui Zhu, Jiajia He. The effects of digital financial inclusion on innovation and entrepreneurship: A network perspective[J]. Electronic Research Archive, 2022, 30(12): 4697-4715. doi: 10.3934/era.2022238
[1] | Yanshou Dong, Junfang Zhao, Xu Miao, Ming Kang . Piecewise pseudo almost periodic solutions of interval general BAM neural networks with mixed time-varying delays and impulsive perturbations. AIMS Mathematics, 2023, 8(9): 21828-21855. doi: 10.3934/math.20231113 |
[2] | Ramazan Yazgan . An analysis for a special class of solution of a Duffing system with variable delays. AIMS Mathematics, 2021, 6(10): 11187-11199. doi: 10.3934/math.2021649 |
[3] | Sivajiganesan Sivasankar, Ramalingam Udhayakumar, Abd Elmotaleb A.M.A. Elamin, R. Samidurai, Sina Etemad, Muath Awadalla . Attractive solutions for Hilfer fractional neutral stochastic integro-differential equations with almost sectorial operators. AIMS Mathematics, 2024, 9(5): 11486-11510. doi: 10.3934/math.2024564 |
[4] | Chunli You, Linxin Shu, Xiao-bao Shu . Approximate controllability of second-order neutral stochastic differential evolution systems with random impulsive effect and state-dependent delay. AIMS Mathematics, 2024, 9(10): 28906-28930. doi: 10.3934/math.20241403 |
[5] | Velusamy Kavitha, Mani Mallika Arjunan, Dumitru Baleanu . Non-instantaneous impulsive fractional-order delay differential systems with Mittag-Leffler kernel. AIMS Mathematics, 2022, 7(5): 9353-9372. doi: 10.3934/math.2022519 |
[6] | Xin Liu, Yan Wang . Averaging principle on infinite intervals for stochastic ordinary differential equations with Lévy noise. AIMS Mathematics, 2021, 6(5): 5316-5350. doi: 10.3934/math.2021314 |
[7] | Hui Huang, Kaihong Zhao, Xiuduo Liu . On solvability of BVP for a coupled Hadamard fractional systems involving fractional derivative impulses. AIMS Mathematics, 2022, 7(10): 19221-19236. doi: 10.3934/math.20221055 |
[8] | Kulandhaivel Karthikeyan, Palanisamy Raja Sekar, Panjaiyan Karthikeyan, Anoop Kumar, Thongchai Botmart, Wajaree Weera . A study on controllability for Hilfer fractional differential equations with impulsive delay conditions. AIMS Mathematics, 2023, 8(2): 4202-4219. doi: 10.3934/math.2023209 |
[9] | Kanagaraj Muthuselvan, Baskar Sundaravadivoo, Kottakkaran Sooppy Nisar, Suliman Alsaeed . Discussion on iterative process of nonlocal controllability exploration for Hilfer neutral impulsive fractional integro-differential equation. AIMS Mathematics, 2023, 8(7): 16846-16863. doi: 10.3934/math.2023861 |
[10] | H. H. G. Hashem, A. M. A. El-Sayed, Maha A. Alenizi . Weak and pseudo-solutions of an arbitrary (fractional) orders differential equation in nonreflexive Banach space. AIMS Mathematics, 2021, 6(1): 52-65. doi: 10.3934/math.2021004 |
The development of urban digital inclusive finance has a complex network connection, and the characteristics of this network affect urban innovation and entrepreneurship. By using 287 cities from 2015 to 2019 in China, this paper constructs the network of Chinese digital inclusive finance, extracting two typical network characteristics. And it further studies the impact of the two network characteristics on innovation and entrepreneurship in various cities. The empirical results show that: first, the network centrality characteristics of digital inclusive finance have a significant promoting effect on urban innovation and entrepreneurship; second, the impact of the network centrality characteristics of digital inclusive finance is related to the speed of industrial structure transformation, but the impact mechanism is related to the node number instead of the node strength; third, the impact of the network centrality characteristics on innovation and entrepreneurship exists heterogeneity among cities and the moderately developed cities have the largest impact.
Currently, FER is a major communication source which is the most important tool to interact with others without knowing their gender, race and national borders. FER comes under the category of non-verbal communication, which delivers a person's feelings in the form of gestures and body language [1] that allow people to infer others' feelings. Many of us can understand facial expressions from key emotions, but others cannot [2]. A robust emotion classification relies heavily on effective facial representations, so it is quite a challenging task to identify significant discriminative facial features that might demonstrate the appearances of each emotion because of the limitations and variability of facial expressions. In addition, the FER system has many challenges, such as difficulties occurring when the face is not in an accurate position, lighting problems while capturing the image, obstruction, noise and over-fitting [3]. Although there are several challenges in the existing FER system, still, it is a fascinating area that could help in different fields of life, such as online education, health management, audience analysis in the market and entertainment. It also helps in security, driver drowsiness detection, etc. S. H. Ma et al. [4] studied facial expressions and classified them into seven categories, which are happy, sad, angry, fearful, surprised, contemptuous and disgusted. During the last five years, different researchers have adopted different methods while working on FER. Some of them considered different types of data used as input to expression recognition systems. In light of perceptions, we observed that facial expressions are typically associated with emotions. For example, lifting the eyebrows indicates a sudden change that means the expression of fear or surprise. The image of human facial expressions is the standard and promising type of information, which gives many unexpected results, applying different algorithms on it such as convolutional neural network (CNN), artificial neural network (ANN), generative adversarial network (GAN), etc. These algorithms give results according to our desired expressions. However, it does not matter how an image is captured or which device is utilized for capturing the image, such as a digital camera, camcorder or DSLR. Human emotions can be perceived from numerous points of view, such as physiological and psychological signs. The FER might be a part of a telepresence bundle which provides information about the patient's mental condition to his doctor or counselor. When something is discovered to be wrong, the professional will choose to change his or her approach to patient care. According to [5], human facial expressions, visual contact, body language, voice tone and personal space are included in nonverbal communication. Since nonverbal communication is 55 to 94% of actual communication [6], positive nonverbal communication can help to boost interpersonal relationships and emphasize emotional bonds [7] proposed a deep learning method for facial recognition for distance learning that extracts features from the images and produced better results. Some recent deep learning methods have also been used for recognition in different fields like medical image classification and segmentation [8,9,10,11,12,13,14,15,16,17].
Over-fitting is the problem with existing FER systems which occurs when the model training has no issues, but they do not predict the indicated results at the time of testing. Many research authors are going to minimize the drawbacks of the prevailing systems, which tend to offer quick-expression classification in the same way. In the contemporary era, computer analysis of the face and facial expressions is the most commonly used issue among specialists. Facial expressions are one of the more significant aspects of human communication, with the face being responsible for communicating, not only for considerations but also for emotions. Over-fitting, due to a lack of training data, remains a major challenge that must be addressed by all deep learning FER systems to achieve high accuracy. The present study is conducted on the SSAE-FER framework for facial expression recognition. The main contributions are as follows.
ⅰ. The SSAE-FER model is used for the recognition of seven basic facial expressions. All the images are the same size for input into the model, which could give us better results than other comparison methods.
ⅱ. Our model is a two-layered stacked auto-encoder (SAE) which is lightweight, and the training and testing time is very much lower due to its simplicity.
ⅲ. The performance of the proposed system was assessed on two different datasets, JAFFE and CK+, and both datasets are publically available.
ⅳ. Enhanced and adequate results were attained in terms of accuracy, specificity and sensitivity.
The remaining portion of this paper is organized as follows: Section 2 discusses related work. Material and methods are discussed in Section 3, Section 4 presents the results and discussion, and the Section 5 concludes the paper.
There are many machine learning and deep learning algorithms which have a great impact on facial expression recognition (FER) systems. Deep learning has different types of algorithms which play a vital role in FER, like generative adversarial networks (GAN), deep belief networks (DBN), stacked sparse auto-encoder (SSAE), conventional neural networks (CNN), recurrent neural networks (CNN-RNN) architecture [18], etc. Similarly, machine learning has different methods and classifiers which are used for classification, like support vector machine (SVM), k-nearest neighbors (KNN), decision tree (DT), weighted hierarchical adaptive voting ensemble (WHAVE), logistic regression (LR), which are used for FER, analyzing a social interaction, intelligent transportation system, fruit identification and anomaly detection [19,20,21,22,23,24,25,26,27,28]. Some feature extraction techniques have been used in the past few years, such as the active shape model (ASM) [29], used to extract features based on expression contours. A deep network was used [30] which has two further different models, where the first model is a deep temporal appearance network (DTAN), and the other is a deep temporal geometry network (DTGN). Thus, DTAN extracts features based on temporal appearance, and another extracts deep temporal geometry network features using facial landmark points. The models are combined through a novel integration method to achieve the best accuracy for expression recognition. This network is known as the deep temporal appearance-geometry network (DTAGN). The selection of pairwise features and their classification is discussed in [31], and [32] introduced a peak-piloted deep network in which peak and non-peak expressions are involved. In this work, peak expressions supervise the recognition of non-peak expressions, but it can only distinguish the same expressions of the same subject. The process of non-peak-to-peak expression is indirectly inserted into the network to get the invariance to expression intensities. A back-propagation method, peak gradient suppression (PGS), is utilized for training the network.
Automatically recognizing facial expressions [33] is an interesting and important part of human-machine interaction, where [34] introduced the CNN and landmark feature technique for 3D facial expression recognition. [35] proposed a novel model for facial expression recognition (FER) using the color scheme and deep information through the Kinect sensor. The proposed system extracts the different features of facial expression and utilizes captured sensor information; it emphasizes vectors by face tracing algorithm and perceives the six facial emotions using the random forest (RF) algorithm. The implementation of RF is utilized for facial expression recognition execution for real-time scenarios. A novel deep-learning method for facial expression recognition is introduced in [36]. The training images were divided into seven groups, due to seven expressions, to train the sparse autoencoder network. Interestingly, a graph convolution neural network (GCNN) successfully recognized the object, text classification and human activities, so GCNN is used to represent the features. Euclidean distance is used to find out the shortest path between edges and joints; a convolutional neural network (CNN) deals with Euclidean data and performs further work on it. A spatial domain convolution kernel is stretched out to graph convolutional kernels to process the number of items over neighbor nodes. The application of pooling is used in the hidden layers of neighbor nodes to complete the data structure on incomplete nodes. The balance cuts and heavy edge matching (HEM) techniques are used for graph pooling. A graph may contain excess or blurring edges, so it utilizes the mechanism to notice the critical node [37].
A convolutional neural network (CNN) combined with bag of words is used. It was successful in object detection, while for further improvement in its results, supervised and unsupervised methods are used. It has been observed that there are different objects in an image that define feature descriptors that are used for forming histograms. By creating histograms of different images that form a bag of words (BoW) that can be learned by a classifier. For the new experimentation of the model, the features were extracted from images using CNN, and then spatial pyramid matching (SPM) was applied to this information to localize the objects. They used CaffeNet, which is similar to Alex-Net, where pooling is done before normalization. It used the t-distribution stochastic neighbor embedding (t-SNE) algorithm to visualize different obtained features from the last layer of CaffeNet in a high-dimensional histogram for each image, which also allows clustering. Although t-SNE is an unsupervised method to cluster data, it is used to see how it classifies suggested data by applying the K-means clustering algorithm on top of t-SNE. Therefore, this is useful in human actions recognition and accomplished the best outcomes using contrasting, where various classification algorithms are used, like k-nearest neighbor (KNN), support vector machine (SVM), relational neighbor (RN) [38] and particle swarm optimization (PSO) [39]. Different other methods have been used in a very efficient way using optical coherence tomography (OCT) in vivo imaging [40,41,42,43].
In video-based action recognition the understanding of actions, pose, estimation, and retrieval of images from different perspectives [44]. Many types of research on the FER system have been directed at both posed and natural expressions under various imaging conditions that include a few head poses, imaging resolutions, illumination factors and occlusion [45]. Conditional generative adversarial networks (cGANs) [46] are applied to gain the images from the neutral face. However, the model has many intermediate layers in which filters remain unchanged, and different layers of the same size are concatenated and combined with the last and fully connected layer for the classification of expression that includes a display of happy, angry, fearful, natural, sad, surprise and disgust [47].
Convolutional neural networks (CNN) are used for feature extraction and classification purposes. A method known as amalgam fusion consists of two levels; the prior level is a feature and the post level is a decision. Both are implemented in a way to pool the features in one place and observe its decision at different stages. As CNN model has trained with a different voice sample of the Ryerson Audio-Visual Dataset of Emotional Speech and Song (RAVDESS) dataset [48] and then joined with an output of an image classifier by utilizing the fusion results. The attained results through decision-level which further proceed towards the final decision [49]. In this study [50] the author proposed a novel technique of color channel-wise recurrent learning which obtained an accuracy rate of 85.74% on facial expression. All the above studies formed the basis of the significant results of their own proposed methods of facial expression, but there is still a need for a simple and attractive piece of facial expressions recognition. We are going to introduce the new technique for the FER framework using SSAE-FER, which will grab the user's attention and will help them to solve their challenges regarding facial expression recognition systems.
In this section, we present our proposed method, which consists of pre-processing, training and testing. A flow diagram of our proposed method is shown in Figure 1.
Image preprocessing is performed on the input dataset on a 2D facial image. The purpose of preprocessing is to enhance the images, where 2 datasets are used in this experiment. The same preprocessing is applied to both datasets. In the first step, we converted the images into grayscale, and a Gaussian smoothing filter is applied due to the noise in the original image. We set the sigma value of σ = 0.5, which is helpful for noise removal. The results of the preprocessing step are illustrated in Figure 2.
Normalizing the whole image is a good idea rather than normalizing some specific parts of the face such as the eye, lips, nose and eyebrows [51]. Normalization has a great influence on images because of different intensities, so we normalize the data using zero mean unit variance. After the normalization, we adjust the images to 0 and 1 values [52]. All the images are normalized, and we performed the class balancing method, where all the classes have the same images in the training set. This is an important step to cure overfitting [53], and our method gained effective results to prepare the input images for the training phase. Due to the preprocessing techniques, our images are more enhanced and prepared for training on the SSAE-FER model.
After the preprocessing step, we are ready to train our data on SSAE-FER. An SSAE is based on two phases, encoder and decoder, to extract high-level feature learning in an unsupervised manner in the first step. The original sizes of the input images were 48 × 48 and 256 × 256 pixels for the 2 individually trained datasets, and we flattened the original images to 2304 and 65,536 for further steps. There are 2 main steps in the training, which are pre-training and fine-tuning. In the pre-training step, we provided the data without ground truth, and in the second step, we trained the whole dataset with ground truths. The SSAE-FER comprises many layers of sparse autoencoder, where the output of each hidden layer is connected to the input of the successive hidden layer. The hidden layers are trained in an unsupervised algorithm and then are fine-tuned in a supervised fashion by using the stochastic gradient descent (SGD) algorithm. We train the autoencoder using input data of 48 × 48 size or 256 × 256 and acquire the learned data from it. The learned data from the previous layer is utilized as input for the next layer, and this continues until the training is completed. Once all the hidden layers are trained, the model uses the backpropagation between hidden layers by using SGD. In the proposed method, we worked on two SSAE layers. The working of SSAE in our work is shown in Figure 3.
The model performs the greedy layer-wise pre-training of data, considering a stacked autoencoder composed of n layers. The suggested model can be greedily pre-trained to initialize the parameters of the deep network, to train the first layer using the input to obtain the parameters for the first autoencoder in the stack, whereas all other parameters in the remainder of the network remain fixed. By initializing the parameters, the input can be transformed into a vector consisting of the activations (learned features) of the hidden units. The autoencoder can map the input directly to the hidden layer using a parameter called an encoder [54]. The encoding step can transform the high-dimensional input data into lower dimensions. The decoding step involves mapping these learned features from the hidden space back to the reconstruction of that input. We have demonstrated the structure of the SSAE1 hidden layers above in Figure 3, where the SSAE2 layers are stacked together. The output of the first layer of SSAE becomes the input of the next layer of SSAE. Data is compressed at latent layers, which become the input of further layers for better performance. The stacked layered network is connected to the Softmax layer, which performs the prediction of the features attained by the SSAE2. As our proposed method uses a novel technique for facial expression recognition, the autoencoder works in an unsupervised manner in pre-training. It comprises encoder and decoder: The encoder maps the input data and represents it in a new form, whereas the new form of data is then decoded at the output to regenerate the input x' as given in Eqs (1) and (2), where x is the input, and z is the new representation of the input.
Z=H(Wx+b) | (1) |
X'=H(W'x+b') | (2) |
In the above Eq (1), h represents the activation function of neurons of the hidden layer. In Eq (2), g represents the neurons of the output layer, W and W' represent the weight matrices, and b and b' represent the bias vectors for encoder and decoder, respectively. SSAE layers have some weights W and biases b, which help to produce better results. Further parameters of the model are utilized to improve the performance of the network. Fine-tuning reduces the error rate observed from the previous epoch which is performed in a supervised manner. After the backpropagation is utilized to fine-tune the whole network, this process minimizes the error rate and refines the model enough to deal with the new samples of datasets.
After the completion of fine-tuning, we trained the FER-SSAE model and applied the classifier Softmax on the last layer. The utilization of the Softmax function is best for multiclass data classification because it maps and predicts the values to probabilities against each expression present in the data. There are seven nodes in the last or output layer of the model that facilitate the network to choose the most desirable features for the representation of each image. Therefore, with the Softmax classifier used for the classification of expressions, this function returns the probability of each class. In our case, it gives the best recognition result against seven expressions. The equation for the Softmax activation function is given below [55].
Softmax(zi)=exp(zi)∑iexp(zi) | (3) |
In Eq (3), the z is the neuron value, which it presents, in the output, and exp is the non-linear function. Later, the sum of exponential finds is used to divide the values of neurons to perform normalization and subsequently convert them into different probabilities when Softmax activation is applied on the final or last layer of each neuron to recognize the expression successfully. Figure 4 shows how two hidden layers work during the pre-training and fine-tuning stages for the classification of expressions.
After the completion of training the same data, input is provided for testing for the trained model. In this step, we also tested the balanced class for better test results. Some of each class sample are equally validated on our SSAE-FER model for classifications of expressions. The structure of the proposed model is given below in Figure 5, which illustrates the complete overview.
In this section, we present the datasets used in this experiment, the parametric settings for the SSAE-FER model and the details of the results.
JAFFE [56,57] and CK+ [58] were used for the experiment on the SSAE-FER model. The JAFFE dataset contains 10 Japanese female expressions that have seven poses: happy, sad, fear, anger, surprise, neutral and disgust. Several images of each expression are available in the dataset having 256 × 256 pixels resolution. We used 213 2D grayscale images from the JAFFE dataset with different classes: anger containing 30 images, disgust in 29 images, fear in 33 images, happiness in 31 images, neutral in 30 images, sadness in 31 images, and 29 images containing surprise expressions. Similarly, the CK+ dataset contains 8 expressions, seven primary expressions, and contempt expressions. The dataset comprises a total of 981 images of different classes were used in our experiment: The happy class contains 207 images, sad 84 images, anger 135 images, fear 75 images, surprise 249 images, disgust 177 images, and the contempt class contains 54 images in our proposed work. The resolution of the CK+ dataset images is 48 × 48 pixels. In [59], the JFEE dataset is used in facial expression recognition which is publically available at the following link: https://zenodo.org/record/3451524#.YSSx1I4zaM8. CK+ dataset is also available publically at the following link: https://www.kaggle.com/shawon10/ckplus. A sample of CK+ & JAFFE dataset images is given below in Figure 6. This work was performed on MATLAB 2021a with a Core i7 processor (3.6 GHz CPU) and 32 GB of RAM. In this study, our FER-SSAE model was based on the MATLAB library "Deep learning Toolbox" [60].
In this section, we provide our results on the basis of our SSAE-FER model. 70% of the data was utilized to train the model, while the rest of the data was used for testing and validation. There were two hidden layers, with 100 neurons at the SSAE1 and similarly 100 neurons on the second layer, SSAE2. The final layer contains 7 neurons to find the most similar features, which help to recognize the expression in each testing image. For pre-training, we set up 200 epochs, and for fine-tuning 6000 epochs with a minimum batch size of 32 were used. The learning rate for pre-training and fine-tuning was 0.0001 with a sparsity of 0.05 and momentum of 0.9. Table 1 shows the parametric settings for our experiment. Our model took 3 hours on CK+ and 13 hours on the JAFFE dataset for fine-tuning. Table 1 shows the parameters which were used to train the SSAE-FER model. Mean Square Error (MSE) was noted at 0.06 on CK+, and the error rate was 0.02 on the JAFFE dataset during the training. The training and validation graphs are given in Figure 7 for both datasets.
Parametric name | Values |
Hidden layers Number of neurons at each layer Number of epochs Learning rate Momentum Mini batch size Sparsity |
2 Layer1 & Layer2 = 100 200 for pre-training and 6000 for fine-tuning 0.0001 0.9 32 0.5 |
The following boundaries are given to assess the exhibition of our planned model.
Accuracy: the proportion of the total number of right expectations. It consists of the prediction of seven human expression samples. We can calculate it by using the following equation:
TP+TNTP+TN+FP+FN | (4) |
Error rate: the total number of predicted cases that were not correct. It consists of both positive and negative samples of seven expressions. We can calculate it by using the following equation:
FP+FNTP+TN+FP+FN | (5) |
Sensitivity or Recall: the proportion of genuine positive occasions effectively recognized is called the true positive rate. We can calculate it by using the following equation:
TPTP+FN | (6) |
Precision: Precision is the ratio of true positives to the total of false positives and true positives. We can calculate it by using the following equation:
TPTP+FP | (7) |
Specificity: This is the proportion of negative occasions accurately distinguished and is also known as the negative rate. We can calculate it by using the following equation:
TNTN+FN | (8) |
True positives (TP) represent those expressions that are correctly identified. False positives (FP) represent the expressions that do not belong to their respective class but the model identifies them as a part of it. True negatives (TN) represent the images that do not belong to another class and are correctly identified as belonging to other classes. False negatives (FN) represent the expressions that belong to a class itself but are identified as another class expression. The results of our model are presented in Tables 2 and 3 for CK+ and JAFFE datasets, respectively.
No. | Expression | Precision % | Sensitivity % | Specificity % | Accuracy % |
1 | Anger | 100.00 | 82.00 | 100.00 | 99.00 |
2 | Contempt | 100.00 | 100.00 | 100.00 | 100.00 |
3 | Disgust | 100.00 | 100.00 | 100.00 | 100.00 |
4 | Fear | 100.00 | 100.00 | 100.00 | 100.00 |
5 | Happy | 100.00 | 100.00 | 100.00 | 100.00 |
6 | Sadness | 95.00 | 95.10 | 100.00 | 96.00 |
7 | Surprise | 100.00 | 100.00 | 100.00 | 100.00 |
Mean | 99.28 | 96.72 | 100.00 | 99.30 |
No. | Expression | Precision % | Sensitivity % | Specificity % | Accuracy % |
1 | Anger | 96.30 | 96.30 | 99.30 | 98.00 |
2 | Disgust | 85.70 | 92.30 | 97.50 | 96.70 |
3 | Fear | 91.30 | 100.00 | 97.50 | 99.40 |
4 | Happy | 100.00 | 92.30 | 100.00 | 98.90 |
5 | Neutral | 86.50 | 83.90 | 97.50 | 95.20 |
6 | Sadness | 89.70 | 89.70 | 98.10 | 96.80 |
7 | Surprise | 100.00 | 96.20 | 100.00 | 99.40 |
Mean | 92.90 | 92.96 | 98.60 | 92.50 |
The CK+ dataset gives us much better results using SSAE-FER on 7 standard expressions. Precision, sensitivity, specificity and accuracy were noted at 99.28, 96.72,100 and 99.30%, respectively. On the other hand, the JAFEE dataset gives us lower results due to the complexity of the dataset, where precision, sensitivity, specificity and accuracy were noted at 92.90, 92.96, 98.60 and 92.50%, respectively.
In this section, we compare our results with other techniques which are given in Table 4.
Methodology | Precision % | Sensitivity % | Specificity % | Error rate % | Accuracy % |
[58] | - | - | - | 10.55 | 89.45 |
[59] | - | - | - | 17.90 | 82.10 |
[49] | 88.00 | 86.00 | - | 13.64 | 86.36 |
[60] | - | - | - | 26.20 | 73.80 |
Our model on the CK+ dataset Our model on the JAFFE dataset |
99.28 92.90 |
96.72 92.96 |
100.00 98.60 |
0.70 7.50 |
99.30 92.50 |
The error rate and accuracy presented in [61] were 10.55 and 89.45% with a novel technique of FER that has a modified classification and regression tree (M-CRT) to deal with the problem in the classification of expressions. The supervised descent and local binary method involve forgetting the global and local features. In [62] a projective complex matrix factorization (proCMF) is introduced, high-dimensional images are used for input, and these are converted into lower dimension subspace. It deals with the complex domain through the optimization problem where the error rate and accuracy were 17.9 and 82.10%. Another novel technique to recognize the expressions is through multimodal automatic emotion recognition (AER) network, which is highly capable in recognizing the expressions with reasonable accuracy. The model achieved 86.36% accuracy with 88% precision [49]. In [63], the author proposed a technique for the FER system to reduce the parameters. A deep learning neural network with a fully connected layer and the global average pooling (GAP) method is applied to achieve 73.80% accuracy. Our proposed method shows a comparatively high recognition rate of 99.30% accuracy on CK+ and 92.50% on JAFFE dataset. Our model on the JAFFE dataset did not perform well due to the complex nature of the dataset, but on CK+, it performed well.
As is quite evident after plenty of research and deliberation, gaining insight into what a person may be feeling is very valuable for many reasons by identifying human feelings from their facial expressions. We have adopted a unique approach, the SSAE-FER model, for the classification of facial expressions. Our model learns the features automatically when input images are given to the model. The pre-training of datasets is achieved in an unsupervised manner and then fine-tuned in a supervised manner. After that, the probability estimation matrix showed the most effective results in the classification of seven basic facial expressions.
Our work was limited to training on CPU-based machines, which is why it took a longer time for training. In the future, we will use a framework that could support GPU, which will improve the training time. Several possible research directions of our proposed model can be utilized for the binary classification of images, such as tumor classification and segmentation. The performance of the proposed model could be enhanced by providing a larger dataset; moreover, it can be used for color-based datasets and real-time scenarios.
This research work was supported by Zayed University in Abu Dhabi with research fund #R20102.
The authors declare that they have no conflict of interest.
[1] |
Z. H. Li, L. M. Chen, H. Dong, What are bitcoin market reactions to its-related events, Int. Rev. Econ. Finance, 73 (2021), 1–10. https://doi.org/10.1016/j.iref.2020.12.020 doi: 10.1016/j.iref.2020.12.020
![]() |
[2] |
Z. H. Li, H. Dong, C. Floros, A. Charemis, P. Failler, Re-examining Bitcoin volatility: A CAViaR-based approach, Emerging Mark. Finance Trade, 58 (2022), 1320–1338. https://doi.org/10.1080/1540496x.2021.1873127 doi: 10.1080/1540496x.2021.1873127
![]() |
[3] |
T. R. Cui, H. Ye, H. H. Teo, J. Z. Li, Information technology and open innovation: A strategic alignment perspective, Inf. Manage., 52 (2015), 348–358. https://doi.org/10.1016/j.im.2014.12.005 doi: 10.1016/j.im.2014.12.005
![]() |
[4] |
M. Dakhli, D. D. Clercq, Human capital, social capital, and innovation: a multi-country study, Entrepreneurship Reg. Dev., 16 (2004), 107–128. https://doi.org/10.1080/08985620410001677835 doi: 10.1080/08985620410001677835
![]() |
[5] |
D. Cumming, S. Johan, Venture's economic impact in Australia, J. Technol. Transfer, 41 (2016), 25–59. https://doi.org/10.1007/s10961-014-9378-3 doi: 10.1007/s10961-014-9378-3
![]() |
[6] |
Z. H. Li, Z. H. Huang, H. Dong, The influential factors on outward foreign direct investment: evidence from the "The Belt and Road", Emerging Mark. Finance Trade, 55 (2019), 3211–3226. https://doi.org/10.1080/1540496x.2019.1569512 doi: 10.1080/1540496x.2019.1569512
![]() |
[7] |
Z. H. Li, F. Q. Zou, B. Mo, Does mandatory CSR disclosure affect enterprise total factor productivity, Econ. Res. Ekonomska Istrazivanja, 35 (2021), 4902–4921. https://doi.org/10.1080/1331677x.2021.2019596 doi: 10.1080/1331677x.2021.2019596
![]() |
[8] |
T. H. Li, X. Li, G. K. Liao, Business cycles and energy intensity. Evidence from Emerging Economies, Borsa Istanbul Rev., 22 (2021), 560–570. https://doi.org/10.1016/j.bir.2021.07.005 doi: 10.1016/j.bir.2021.07.005
![]() |
[9] |
S. P. Borgatti, P. C. Foster, The network paradigm in organizational research: A review and typology, J. Manage., 29 (2003), 991–1013. https://doi.org/10.1016/S0149-2063(03)00087-4 doi: 10.1016/S0149-2063(03)00087-4
![]() |
[10] | W. B. Arthur, The Economy As An Evolving Complex System II, CRC Press, 2018. |
[11] |
Y. Pierrakis, L. Collins, Crowdfunding: A new innovative model of providing funding to projects and businesses, Social Sci. Res., 2013 (2013). http://dx.doi.org/10.2139/ssrn.2395226 doi: 10.2139/ssrn.2395226
![]() |
[12] |
S. C. Moenninghoff, A. Wieandt, The future of peer-to-peer finance, Schmalenbach's J. Bus. Res., 65 (2013), 466–487. https://doi.org/10.1007/BF03372882 doi: 10.1007/BF03372882
![]() |
[13] |
F. H. Wen, J. H. Cao, Z. Liu, X. Wang, Dynamic volatility spillovers and investment strategies between the Chinese stock market and commodity markets, Int. Rev. Financ. Anal., 76 (2021). https://doi.org/10.1016/j.irfa.2021.101772 doi: 10.1016/j.irfa.2021.101772
![]() |
[14] |
S. Jiang, S. Qiu, H. Zhou, Will digital financial development affect the effectiveness of monetary policy in emerging market countries, Econ. Res. Ekonomska Istrazivanja, 35 (2021), 3437–3472. https://doi.org/10.1080/1331677x.2021.1997619 doi: 10.1080/1331677x.2021.1997619
![]() |
[15] |
F. Guo, J. Y. Wang, F. Wang, T. Kong, X. Zhang, Z. Y. Cheng, Measuring China's digital inclusive financial development: Index compilation and spatial characteristics, China Econ. Q., 19 (2020), 1401–1418. https://doi.org/10.13821/j.cnki.ceq.2020.03.12 doi: 10.13821/j.cnki.ceq.2020.03.12
![]() |
[16] |
D. Ahlstrom, G. D. Bruton, Rapid institutional shifts and the co-evolution of entrepreneurial firms in transition economies, Entrepreneurship Theory Pract., 34 (2010), 531–554. https://doi.org/10.1111/j.1540-6520.2010.00373.x doi: 10.1111/j.1540-6520.2010.00373.x
![]() |
[17] |
B. Armendariz, J. Morduch, The economics of microfinance, J. Int. Econ., 70 (2006), 328–333. https://doi.org/10.1016/j.jinteco.2006.02.001 doi: 10.1016/j.jinteco.2006.02.001
![]() |
[18] |
P. Aghion, T. Fally, S. Scarpetta, Credit constraints as a barrier to the entry and post-entry growth of firms, Econ. Policy, 52 (2007), 731–779. https://doi.org/10.1111/j.1468-0327.2007.00190.x doi: 10.1111/j.1468-0327.2007.00190.x
![]() |
[19] |
Z. H. Li, Z. M. Ao, B. Mo, Revisiting the valuable roles of global financial assets for international stock markets: Quantile coherence and causality-in-quantiles approaches, Mathematics, 9 (2021), 1750. https://doi.org/10.3390/math9151750 doi: 10.3390/math9151750
![]() |
[20] |
Z. H. Li, J. H. Zhong, Impact of economic policy uncertainty shocks on China's financial conditions, Finance Res. Lett., 35 (2020), 101303. https://doi.org/10.1016/j.frl.2019.101303 doi: 10.1016/j.frl.2019.101303
![]() |
[21] |
S. Agarwal, R. B. H. Hauswald, The choice between arm's-length and relationship debt: Evidence from eLoans, FRB of Chicago Working Paper, 10 (2008), 488–500. http://dx.doi.org/10.2139/ssrn.1306455 doi: 10.2139/ssrn.1306455
![]() |
[22] | B. H. Hall, J. Lerner, Chapter 14-The financing of R & D and innovation, in Handbook of the Economics of Innovation, 1 (2010), 609–639. https://doi.org/10.1016/S0169-7218(10)01014-2 |
[23] |
M. Shahrokhi, E-finance: status, innovations, resources and future challenges, Managerial Finance, 34 (2008), 365–398. https://doi.org/10.1108/03074350810872787 doi: 10.1108/03074350810872787
![]() |
[24] |
P. H. Hsu, X. Tian, Y. Xu, Financial development and innovation: Cross-country evidence, J. Financ. Econ., 112 (2014), 116–135. https://doi.org/10.1016/j.jfineco.2013.12.002 doi: 10.1016/j.jfineco.2013.12.002
![]() |
[25] |
M. Usman, M. S. A. Makhdum, R. Kousar, Does financial inclusion, renewable and non-renewable energy utilization accelerate ecological footprints and economic growth? Fresh evidence from 15 highest emitting countries, Sustainable Cities Soc., 65 (2021). https://doi.org/10.1016/j.scs.2020.102590 doi: 10.1016/j.scs.2020.102590
![]() |
[26] |
S. L. Chen, J. H. Zhong, P. Failler, Does China transmit financial cycle spillover effects to the G7 countries, Econ. Res. Ekonomska Istrazivanja, 35 (2022), 5184–5201. https://doi.org/10.1080/1331677x.2021.2025123 doi: 10.1080/1331677x.2021.2025123
![]() |
[27] |
Y. Liu, Z. H. Li, M. R. Xu, The influential factors of financial cycle spillover: Evidence from China, Emerging Mark. Finance Trade, 56 (2020), 1336–1350. https://doi.org/10.1080/1540496x.2019.1658076 doi: 10.1080/1540496x.2019.1658076
![]() |
[28] | B. Venet, F. Peltrault, Intra-industry trade and economic distance: causality tests using panel data, 2005. |
[29] | X. Zhang, China innovation and entrepreneurship index, 2019 (2019). https://doi.org/10.18170/DVN/PEFDAS |
[30] |
R. Burt, Structural holes: The social structure of competition, Adm. Sci. Q., 40 (1995), 345–349. https://doi.org/10.2307/2393642 doi: 10.2307/2393642
![]() |
[31] | S. Wasserman, F. Katherine, Social Network Analysis: Methods and Applications (Structural Analysis in the Social Sciences), Cambridge University Press, 1994. |
[32] |
L. C. Freeman, Centrality in social networks: Conceptual clarification, Social Networks, 1 (1979), 215–239. https://doi.org/10.1016/0378-8733(78)90021-7 doi: 10.1016/0378-8733(78)90021-7
![]() |
[33] |
Z. H. Li, F. Q. Zou, Y. Tan, J. H. Zhu, Does financial excess support land urbanization-An empirical study of cities in China, Land, 10 (2021), 635. https://doi.org/10.3390/land10060635 doi: 10.3390/land10060635
![]() |
[34] |
F. Li, C. Y. Yang, Z. H. Li, P. Failler, Does geopolitics have an impact on energy ttrade? Empirical research on emerging countries, Sustainability, 13 (2021), 5199. https://doi.org/10.3390/su13095199 doi: 10.3390/su13095199
![]() |
[35] |
C. Y. Yang, T. H. Li, K. Albitar, Does energy efficiency affect ambient PM2.5? The moderating role of energy investment, Front. Environ. Sci., 9 (2021), 707751. https://doi.org/10.3389/fenvs.2021.707751 doi: 10.3389/fenvs.2021.707751
![]() |
[36] |
I. S. Farouq, N. U. Sambo, A. U. Ahmad, A. H. Jakada, I. A. Danmaraya, Does financial globalization uncertainty affect CO2 emissions? Empirical evidence from some selected SSA countries, Quant. Finance Econ., 5 (2021), 247–263. https://doi.org/10.3934/qfe.2021011 doi: 10.3934/qfe.2021011
![]() |
[37] |
Y. Pan, L. Ma, Y. Wang, How and what kind of cities benefit from the development of digital inclusive finance? Evidence from the upgrading of export in Chinese cities, Econ. Res. Ekonomska Istrazivanja, 35 (2021), 3979–4007. https://doi.org/10.1080/1331677x.2021.2007414 doi: 10.1080/1331677x.2021.2007414
![]() |
[38] |
Y. Luo, Y. C. Peng, L. Y. Zeng, Digital financial capability and entrepreneurial performance, Int. Rev. Econ. Finance, 76 (2021), 55–74. https://doi.org/10.1016/j.iref.2021.05.010 doi: 10.1016/j.iref.2021.05.010
![]() |
[39] |
Z. H. Li, Z. H. Huang, P. Failler, Dynamic correlation between crude oil price and investor sentiment in China: Heterogeneous and asymmetric effect, Energies, 15 (2022), 687. https://doi.org/10.3390/en15030687 doi: 10.3390/en15030687
![]() |
[40] |
T. H. Li, X. Li, K. Albitar, Threshold effects of financialization on enterprise R & D innovation: a comparison research on heterogeneity, Quant. Finance Econ., 5 (2021), 496–515. https://doi.org/10.3934/qfe.2021022 doi: 10.3934/qfe.2021022
![]() |
[41] |
X. H. Lu, X. Jiang, M. Q. Gong, How land transfer marketization influence on green total factor productivity from the approach of industrial structure? Evidence from China, Land Use Policy, 95 (2020), 104610. https://doi.org/10.1016/j.landusepol.2020.104610 doi: 10.1016/j.landusepol.2020.104610
![]() |
[42] |
M. Bruhn, I. Love, The real impact of improved access to finance: Evidence from Mexico, J. Finance, 69 (2014), 1347–1376. https://doi.org/10.1111/jofi.12091 doi: 10.1111/jofi.12091
![]() |
[43] | R. M. Baron, D. A. Kenny, The moderator-mediator variable distinction in social psychological research: conceptual, strategic, and statistical considerations, J. Pers. Social Psychol., 51 (1986), 1173–1182. |
[44] |
J. Atta-Mensah, Commodity-linked bonds as an innovative financing instrument for African countries to build back better, Quant. Finance Econ., 5 (2021), 516–541. https://doi.org/10.3934/qfe.2021023 doi: 10.3934/qfe.2021023
![]() |
[45] |
Z. H. Li, C. Y. Yang, Z. H. Huang, How does the fintech sector react to signals from central bank digital currencies, Finance Res. Lett., 50 (2022), 103308. https://doi.org/10.1016/j.frl.2022.103308 doi: 10.1016/j.frl.2022.103308
![]() |
[46] |
Y. Tan, Z. H. Li, S. M. Liu, M. I. Nazir, M. Haris, Competitions in different banking markets and shadow banking: evidence from China, Int. J. Emerging Mark., 17 (2022), 1465–1483. https://doi.org/10.1108/ijoem-04-2020-0401 doi: 10.1108/ijoem-04-2020-0401
![]() |
[47] |
R. Haas, A. Ajanovic, J. Ramsebner, T. Perger, J. Knapek, J. W. Bleyl, Financing the future infrastructure of sustainable energy systems, Green Finance, 3 (2021), 90–118. https://doi.org/10.3934/gf.2021006 doi: 10.3934/gf.2021006
![]() |
[48] |
M. Kanwal, H. Khan, Does carbon asset add value to clean energy market? Evidence from EU, Green Finance, 3 (2021), 495–507. https://doi.org/10.3934/gf.2021023 doi: 10.3934/gf.2021023
![]() |
[49] |
J. H. Zhu, Z. H. Huang, Z. H. Li, K. Albitar, The impact of urbanization on energy intensity-An empirical study on OECD countries, Green Finance, 3 (2021), 508–526. https://doi.org/10.3934/gf.2021024 doi: 10.3934/gf.2021024
![]() |
[50] |
J. H. Zhu, Z. H. Li, Can digital financial inclusion effectively stimulate technological Innovation of agricultural enterprises?—A case study on China, Natl. Accounting Rev., 3 (2021), 398–421. https://doi.org/10.3934/NAR.2021021Z. doi: 10.3934/NAR.2021021Z
![]() |
[51] |
H. Li, L. M. Chen, H. Dong, What are bitcoin market reactions to its-related events, Int. Rev. Econ. Finance, 73 (2021), 1–10. https://doi.org/10.1016/j.iref.2020.12.020 doi: 10.1016/j.iref.2020.12.020
![]() |
[52] |
Z. Li, H. Chen, B. Mo, Can digital finance promote urban innovation? Evidence from China, Borsa Istanbul Rev., 2022 (2022). https://doi.org/10.1016/j.bir.2022.10.006. doi: 10.1016/j.bir.2022.10.006
![]() |
1. | Hehe Yang, Qiang Feng, Xiaoxia Wang, Didar Urynbassarova, Aajaz A. Teali, Reduced Biquaternion Windowed Linear Canonical Transform: Properties and Applications, 2024, 12, 2227-7390, 743, 10.3390/math12050743 |
Parametric name | Values |
Hidden layers Number of neurons at each layer Number of epochs Learning rate Momentum Mini batch size Sparsity |
2 Layer1 & Layer2 = 100 200 for pre-training and 6000 for fine-tuning 0.0001 0.9 32 0.5 |
No. | Expression | Precision % | Sensitivity % | Specificity % | Accuracy % |
1 | Anger | 100.00 | 82.00 | 100.00 | 99.00 |
2 | Contempt | 100.00 | 100.00 | 100.00 | 100.00 |
3 | Disgust | 100.00 | 100.00 | 100.00 | 100.00 |
4 | Fear | 100.00 | 100.00 | 100.00 | 100.00 |
5 | Happy | 100.00 | 100.00 | 100.00 | 100.00 |
6 | Sadness | 95.00 | 95.10 | 100.00 | 96.00 |
7 | Surprise | 100.00 | 100.00 | 100.00 | 100.00 |
Mean | 99.28 | 96.72 | 100.00 | 99.30 |
No. | Expression | Precision % | Sensitivity % | Specificity % | Accuracy % |
1 | Anger | 96.30 | 96.30 | 99.30 | 98.00 |
2 | Disgust | 85.70 | 92.30 | 97.50 | 96.70 |
3 | Fear | 91.30 | 100.00 | 97.50 | 99.40 |
4 | Happy | 100.00 | 92.30 | 100.00 | 98.90 |
5 | Neutral | 86.50 | 83.90 | 97.50 | 95.20 |
6 | Sadness | 89.70 | 89.70 | 98.10 | 96.80 |
7 | Surprise | 100.00 | 96.20 | 100.00 | 99.40 |
Mean | 92.90 | 92.96 | 98.60 | 92.50 |
Methodology | Precision % | Sensitivity % | Specificity % | Error rate % | Accuracy % |
[58] | - | - | - | 10.55 | 89.45 |
[59] | - | - | - | 17.90 | 82.10 |
[49] | 88.00 | 86.00 | - | 13.64 | 86.36 |
[60] | - | - | - | 26.20 | 73.80 |
Our model on the CK+ dataset Our model on the JAFFE dataset |
99.28 92.90 |
96.72 92.96 |
100.00 98.60 |
0.70 7.50 |
99.30 92.50 |
Parametric name | Values |
Hidden layers Number of neurons at each layer Number of epochs Learning rate Momentum Mini batch size Sparsity |
2 Layer1 & Layer2 = 100 200 for pre-training and 6000 for fine-tuning 0.0001 0.9 32 0.5 |
No. | Expression | Precision % | Sensitivity % | Specificity % | Accuracy % |
1 | Anger | 100.00 | 82.00 | 100.00 | 99.00 |
2 | Contempt | 100.00 | 100.00 | 100.00 | 100.00 |
3 | Disgust | 100.00 | 100.00 | 100.00 | 100.00 |
4 | Fear | 100.00 | 100.00 | 100.00 | 100.00 |
5 | Happy | 100.00 | 100.00 | 100.00 | 100.00 |
6 | Sadness | 95.00 | 95.10 | 100.00 | 96.00 |
7 | Surprise | 100.00 | 100.00 | 100.00 | 100.00 |
Mean | 99.28 | 96.72 | 100.00 | 99.30 |
No. | Expression | Precision % | Sensitivity % | Specificity % | Accuracy % |
1 | Anger | 96.30 | 96.30 | 99.30 | 98.00 |
2 | Disgust | 85.70 | 92.30 | 97.50 | 96.70 |
3 | Fear | 91.30 | 100.00 | 97.50 | 99.40 |
4 | Happy | 100.00 | 92.30 | 100.00 | 98.90 |
5 | Neutral | 86.50 | 83.90 | 97.50 | 95.20 |
6 | Sadness | 89.70 | 89.70 | 98.10 | 96.80 |
7 | Surprise | 100.00 | 96.20 | 100.00 | 99.40 |
Mean | 92.90 | 92.96 | 98.60 | 92.50 |
Methodology | Precision % | Sensitivity % | Specificity % | Error rate % | Accuracy % |
[58] | - | - | - | 10.55 | 89.45 |
[59] | - | - | - | 17.90 | 82.10 |
[49] | 88.00 | 86.00 | - | 13.64 | 86.36 |
[60] | - | - | - | 26.20 | 73.80 |
Our model on the CK+ dataset Our model on the JAFFE dataset |
99.28 92.90 |
96.72 92.96 |
100.00 98.60 |
0.70 7.50 |
99.30 92.50 |