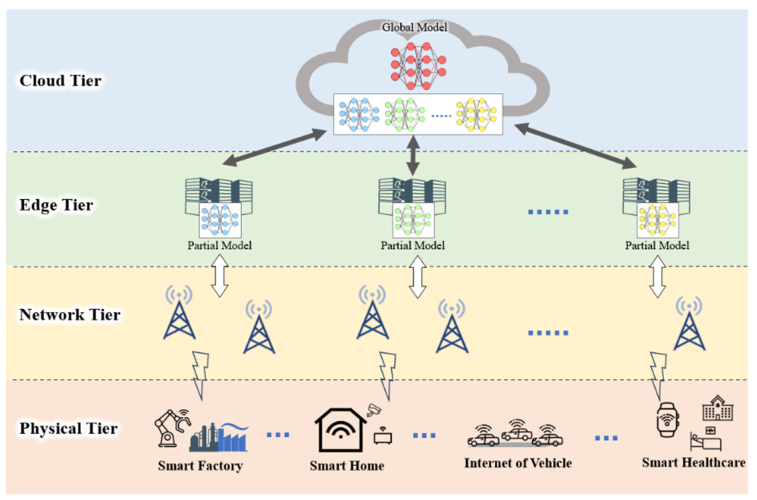
In this paper, we study the following Schrödinger-Poisson equations with double critical exponents:
{−Δu=|u|4u+ϕ|u|3u+λu,inΩ,−Δϕ=|u|5,inΩ,u=ϕ=0,on∂Ω,
where Ω is a bounded domain in R3 with Lipschitz boundary, λ is a real parameter satisfying suitable conditions. Using variational methods, we show the existence and nonexistence of nontrivial solutions for the Schrödinger-Poisson equations.
Citation: Li Cai, Fubao Zhang. The Brezis-Nirenberg type double critical problem for a class of Schrödinger-Poisson equations[J]. Electronic Research Archive, 2021, 29(3): 2475-2488. doi: 10.3934/era.2020125
[1] | Qun Dai, Zeheng Wang . SIRV fractional epidemic model of influenza with vaccine game theory and stability analysis. Electronic Research Archive, 2024, 32(12): 6792-6821. doi: 10.3934/era.2024318 |
[2] |
Zuliang Lu, Fei Huang, Xiankui Wu, Lin Li, Shang Liu .
Convergence and quasi-optimality of |
[3] | Chao Ma, Hang Gao, Wei Wu . Adaptive learning nonsynchronous control of nonlinear hidden Markov jump systems with limited mode information. Electronic Research Archive, 2023, 31(11): 6746-6762. doi: 10.3934/era.2023340 |
[4] | Qianqian Zhang, Mingye Mu, Heyuan Ji, Qiushi Wang, Xingyu Wang . An adaptive type-2 fuzzy sliding mode tracking controller for a robotic manipulator. Electronic Research Archive, 2023, 31(7): 3791-3813. doi: 10.3934/era.2023193 |
[5] | Xinling Li, Xueli Qin, Zhiwei Wan, Weipeng Tai . Chaos synchronization of stochastic time-delay Lur'e systems: An asynchronous and adaptive event-triggered control approach. Electronic Research Archive, 2023, 31(9): 5589-5608. doi: 10.3934/era.2023284 |
[6] | Sida Lin, Lixia Meng, Jinlong Yuan, Changzhi Wu, An Li, Chongyang Liu, Jun Xie . Sequential adaptive switching time optimization technique for maximum hands-off control problems. Electronic Research Archive, 2024, 32(4): 2229-2250. doi: 10.3934/era.2024101 |
[7] | Huan Luo . Heterogeneous anti-synchronization of stochastic complex dynamical networks involving uncertain dynamics: an approach of the space-time discretizations. Electronic Research Archive, 2025, 33(2): 613-641. doi: 10.3934/era.2025029 |
[8] | Ziang Chen, Chunguang Dai, Lei Shi, Gaofang Chen, Peng Wu, Liping Wang . Reaction-diffusion model of HIV infection of two target cells under optimal control strategy. Electronic Research Archive, 2024, 32(6): 4129-4163. doi: 10.3934/era.2024186 |
[9] | Xiangwen Yin . Promoting peer learning in education: Exploring continuous action iterated dilemma and team leader rotation mechanism in peer-led instruction. Electronic Research Archive, 2023, 31(11): 6552-6563. doi: 10.3934/era.2023331 |
[10] | Liping Fan, Pengju Yang . Load forecasting of microgrid based on an adaptive cuckoo search optimization improved neural network. Electronic Research Archive, 2024, 32(11): 6364-6378. doi: 10.3934/era.2024296 |
In this paper, we study the following Schrödinger-Poisson equations with double critical exponents:
{−Δu=|u|4u+ϕ|u|3u+λu,inΩ,−Δϕ=|u|5,inΩ,u=ϕ=0,on∂Ω,
where Ω is a bounded domain in R3 with Lipschitz boundary, λ is a real parameter satisfying suitable conditions. Using variational methods, we show the existence and nonexistence of nontrivial solutions for the Schrödinger-Poisson equations.
General data protection regulation (GDPR) has steered into a new era of data protection and privacy awareness [1,2]. As organizations and institutions engage with restricted data privacy requirements, the necessity to find solutions that oblige the regulation and protect sensitive information while still leveraging the potentiality of data-driven learning becomes highly significant. In the era of massive data and internet of things (IoT) heterogeneity, the handling of sensitive data is a complex objective for both businesses and researchers, which expands to a variety of services including healthcare systems, home security, wearable personal, payment systems, etc. [3,4,5,6]. The consequences of data breaches and privacy violations include not only legal but also ethical and reputational problems. Due to this circumstance, researchers and practitioners have pushed to explore new paradigms that can reconcile the functionality supports for both data-driven intelligence and robust data protection capability.
Decentralizing data from central servers to individual devices, federated learning (FL) unlocks the power of artificial intelligence (AI) for domains with sensitive data and diverse equipment [7]. It addresses data privacy concerns, enhances security, and improves model generalizability while reducing communication costs and server load [8]. Beyond these general benefits, FL holds enormous potential for network applications. First, its decentralized nature minimizes data transmission to central servers, alleviating network congestion and bandwidth demands [9]. Second, it enables personalized model training on individual devices, leading to contextually relevant models tailored to user behaviors and preferences. Additionally, by leveraging data diversity from various devices and locations, FL generates robust and generalized models that improve the overall accuracy and performance of network applications [10,11]. Ultimately, FL's decentralized approach not only protects data privacy but also optimizes network efficiency, empowers personalization, and boosts model performance in the networking landscape.
For researchers in the networking field, numerous challenges arise as they seek to balance the demands of optimizing network performance and preserving user privacy [12,13]. The exponential growth of data volumes, the increasing heterogeneity of networking taxonomies, and the intense sensitivity surrounding user data all contribute to these challenges. Consequently, researchers are driven to explore novel techniques that lead to a complementary technique, FL, which promises to upgrade the way networking systems are designed and optimized in a distributed and collaborative manner. In 2016, Google researchers released FL as a communication-efficient method of distributed learning between global servers and local participants through iterative global model broadcasting, local training, and model averaging [14]. Figure 1 illustrates the overview of FL that integrates in the networking field, including four main tiers, namely application, network, edge, and cloud. FL (edge) offers a comprehensive set of contributions to networking by introducing a framework where local devices can collectively train a shared model without exposing raw data, particularly sensitive information [15,16,17,18]. The framework feature not only enhances privacy preservation but also reduces the communication overhead that often troubles conventional data-sharing or high-volume uploading approaches.
Beyond privacy, FL (edge) also offers the potential for learning adaptation, low-latency operation, edge intelligence, personalization, and cost-effective resource utilization, all of which are significant for intelligent networking systems [19,20,21]. On the other hand, as researchers begin to explore the relationship between FL and networking, the selection of experimental simulation tools or platforms becomes a critical consideration. Therefore, in this survey, we aim to provide a comprehensive overview of the state-of-the-art experimental simulations for privacy-preserving FL in intelligent networking. Figure 2 presents the paper structure, and Table 1 gives the abbreviations used in this paper. Furthermore, to summarize our contributions, we categorize the main points as follows:
● Networking for FL: This section examines network simulation tools to ensure collaboration with FL frameworks. Simultaneously, network platforms can generate a precise dataset to obtain any specific applications. Typically, multiple simulation platforms can provide for several aspects (e.g., network topology acquisition, distributed computing putting capability, multi-round communication, and scalability deployment).
● FL for networking: Afterward, we reflect on the abovementioned platforms by reviewing how the FL framework resource can be utilized to complement networking simulation (e.g., data privacy, private preservation, bandwidth-efficient updates, latency reduction, learning robustness in edge intelligence, and converged round communication).
● Objectives of FL case studies: As we discussed, the collaboration between multiple simulation tools and the FL framework is eligible to accomplish several case studies in terms of learning performance, QoS, energy efficiency, and cost.
● Challenges and future directions: Simulation tools and the FL framework play a crucial role in realizing the full potential of various applications while addressing their inherent challenges in the critical areas of the research and development of 5G and beyond networks. However, in optimizing communication efficiency between the central server and decentralized clients, especially in constrained network resources, challenges include communication overhead, operational bandwidth cost, and expansion of multi-awareness learning (resources, delays, and energy). Additionally, ensuring the privacy of sensitive data during the FL process remains a paramount concern, prompting investigations into techniques such as secure multi-party computation and differential privacy. The scalability of FL in intelligent networking, mainly when dealing with a vast number of clients and diverse network conditions.
Acronym | Description |
API | Application programming interface |
CAPEX | Capital expenditure |
DDQN | Double deep Q-network |
DFQL | Deep federated Q-learning |
DL | Deep learning |
DNN | Deep neural network |
DRL | Deep reinforcement learning |
DSRA | Device selection and resource allocation |
DQL | Deep Q-learning |
EC | Edge cloud |
E2E | End-to-end |
FL | Federated learning |
HFL-VNE | Horizontal federated learning-virtual network embedding |
IID | Independent-and-identically-distributed |
IIoT | Industrial internet of things |
IoV | Internet of vehicles |
IoT | Internet of things |
MEC | Multi-access edge computing |
MFL | Multilevel federated learning |
ML | Machine learning |
MTFL | Multi-tentacle federated learning |
NFV | Network functions virtualization |
NFVeEC | NFV-enabled EC |
QoE | Quality of experience |
QoS | Quality of service |
RAN | Radio access network |
SDN | Software-defined networks |
VGAE | Variational graph autoencoder |
FL enables training on decentralized data sources while keeping the data localized. In practical FL scenarios, the active coordinator requires a critical focus on communication efficiency, model aggregation, and security evaluation to ensure its effectiveness. In Table 2, we gather the most relevant surveys analyzing in relation to FL simulation.
Survey paper | IoT networks | Overview of FL | Framework details | Key performance indicators | Simulation tools in networks | Ref. | Year |
W. Lim et al. | ✓ | ✓ | ✓ | ✘ | ✘ | [22] | 2020 |
D. Nguyen et al. | ✓ | ✓ | ✓ | ✘ | ✘ | [23] | 2021 |
R. Gupta et al. | ✘ | ✓ | ✓ | ✓ | ✘ | [24] | 2022 |
L. Witt et al. | ✘ | ✓ | ✓ | ✘ | ✘ | [25] | 2023 |
H. Chen et al. | ✘ | ✓ | ✓ | ✘ | ✘ | [26] | 2023 |
M. Al-Quraan et al. | ✘ | ✓ | ✓ | ✘ | ✘ | [27] | 2023 |
We started by listing all the processing steps of conventional FL and pointing out the main functions and literature reviews that are associated with intelligent networking. The execution can be defined as follows:
1) Initialization: FL begins with server setup as a global central server that is responsible for coordinating the overall FL process. Then, the coordinator selects the model by creating a model architecture suitable for the target application. Some use cases initially declare the model random or even pre-trained on public data. After deciding on the global model, the FL coordinator is required to identify the client batch in that round of communication, namely participant selection.
2) Participant selection: In mobile networks, participant selection is challenging due to bandwidth limitations and unstable availability or connectivity [28]. Suppose the coordinator sets a policy with random client selection in each round. In that case, the learning performance will return with unreliable accuracy, slow convergence, data imbalance, and unfairness [29], which heavily degrades the practicality in real-world scenarios. The selection approaches tackle the heterogeneity of device taxonomies in modern networks with diverse data type distributions and hardware-specific configurations. Table 3 summarizes proposed approaches in this domain, including targets on resource efficiency, convergence analysis, data heterogeneity handling, power-of-choice strategies, and unstable clients [30,31,32,33,34].
3) Model distribution: After the server selects the clients that will participate in the training round, it distributes the current model to all the selected clients. The clients then download the model onto their local devices and train it on their own data.
4) Local model training: Local data is stored on each client device in the FL system, and the clients train on their local data without sending it to the server. However, there are also some challenges associated with using local data, including data heterogeneity, insufficient computation resources, and network connectivity. Due to the problems of local device capability, there are several studies proposing edge-assisted learning for handling FL local tasks, particularly over resource-constrained IoT devices.
5) Local model updates: After local training, each client generates the optimal model update for that round, which consists of the model parameters (e.g., weights). Table 4 presents the relevant literature that assists the local model training and updates.
6) Model updates aggregation and global update: Clients securely transmit their model parameter updates (e.g., weights) to the central server or even using edge-assisted methods. The central server aggregates the model updates into a global update. Conventional aggregation methods include federated averaging and secure multi-party computation. The central server integrates the aggregated global model update into the new-iteration central model. To improve this processing step, there are several studies that tackle different target objectives. Table 5 presents the literature reviews for this phase.
Summary of execution flows | Target objectives | Ref. | Year |
The proposed algorithm features two components: 1) An online learning component based on previous resource usage and training results for making fractional decisions, and 2) An internet-based rounding tool that transforms fractional choices into whole number selections. | Prioritization in cumulative utilization of computation and communication resources, while ensuring the convergence of both local and aggregated models. | [30] | 2020 |
The Power-of-Choice selection strategy works as follows: 1) The central server calculates the local losses of all clients, 2) The server sorts the clients in decreasing order of their local losses, and 3) The server selects the top set of clients to participate in the current training round. | Improvement of the convergence speed and accuracy of FL while reducing communication and computation overhead (target applications can be distributed machine learning (ML), mobile edge computing, and IoT). | [31] | 2022 |
"PyramidFL" is a fine-grained client selection method that first determines the utility-based client selection from the global view and then optimizes its utility profiling locally for further client selection. | Designing to speed up the FL training while achieving a higher final model performance, also prioritizing the use of clients with higher statistical and system utility. | [32] | 2020 |
1) Deadline-based aggregation: Aggregate client updates once a fixed deadline is met. 2) Joint optimization: two subproblems on client selection and parameter update. 3) E3CS: an exponential-weight algorithm for exploration and exploitation-based client selection. | Improvement of the training efficiency and final model accuracy in FL within a volatile training context, where clients are prone to failures. | [33] | 2022 |
"Newt" is an enhanced FL approach that includes a new client selection utility and fine-grained control on selection frequency. | Enabling efficient selection in intelligent transportation systems, which has challenges such as data and device heterogeneity and highly dynamic system. | [34] | 2022 |
Summary of execution flows | Target objectives | Ref. | Year |
"FL+HC" clusters clients are grouped based on how closely their local updates match the overall global model. After this grouping, the clusters are trained autonomously and simultaneously using dedicated models. | Improvement of the accuracy of the test set while minimizing the communication rounds needed to achieve convergence in FL, especially when dealing with non-independent and non-identically distributed (non-IID) data. | [35] | 2020 |
1) Bandwidth allocation: involves assigning additional bandwidth to devices that experience poorer channel conditions or possess less robust computational capabilities. 2) Device scheduling: suggests an approach that prioritizes selecting devices with the shortest model updating time until a favorable balance is struck between learning efficiency and round-trip latency. | Maximization of the convergence rate of FL training with respect to time rather than rounds, which can be achieved by minimizing the expected time for FL training to attain certain model accuracy. | [36] | 2020 |
Each client offloads partial data to the edge server for processing, and then, the clients update their local models using the remaining data (Meanwhile, the edge server updates the global model using the aggregated data). | Mitigation of the straggler effect in FL and improving the system efficiency (The method can be used in applications, such as mobile device training, IoT device training, or healthcare data training). | [37] | 2021 |
The optimal offloading strategy is derived by minimizing the FL loss function under the latency constraint and the Q-learning-based offloading strategy is proposed for the imperfect channel state information scenario. | Improvement of training efficiency and mitigating the straggler effect of FL in industrial IoT networks. | [38] | 2023 |
The greedy algorithm is proposed by iteratively assigning communication resources to the edge nodes that consume the least energy for local model training in each round, which proceeds until the communication resource budget is finished. | Training machine learning models for edge-assisted Internet of Agriculture Things applications, where data are both vertically and horizontally partitioned, and resources are limited. | [39] | 2022 |
Summary of execution flows | Target objectives | Ref. | Year |
1) Unbiased gradient aggregation: Evaluate the gradients against the global model parameters in the last local epoch, and 2) FedMeta: Perform meta updating on the global model parameters using a small set of data samples indicating the expected target distribution. | Improvement of the convergence speed and accuracy (Both unbiased gradient aggregation and FedMeta can be applied individually or together and can be integrated into existing FL). | [40] | 2020 |
1) Partition the network into groups and local model updates into segments, 2) Apply the aggregation protocol to segments with specific coordination between users, and 3) Aggregate the aggregated segments to obtain the global update. | Enabling secure model aggregation in FL systems, while allowing users to adjust the quantization proportional to their communication resources. | [41] | 2022 |
The method aggregates updates from scheduled devices using an age-aware weighting design, and the weights are assigned to each update based on the freshness of the data, with more recent updates receiving higher weights. | Development of an asynchronous FL framework with periodic aggregation that can support heterogeneous computation capabilities and training data distributions. | [42] | 2021 |
The method uses a feedback mechanism to communicate the estimated global update to each client, which then calculates the relevance of its local update to the estimated global update (If the relevance is lower than a predefined threshold, the client does not update). | Mitigation of communication overhead, which can be applied to a variety of FL applications, such as personalized healthcare, intelligent transportation systems, and distributed machine learning for financial services. | [43] | 2019 |
Participants apply linear transformation to the model update vector, then partially encrypt the transformed vector using multi-input functional encryption. | Design of an efficient secure aggregation scheme, which can protect the security of model updates without losing efficiency. | [44] | 2020 |
Each processing step helps improve the central model performance, deployed after the convergence evaluation and application performance requirements are satisfied. The privacy and security methods (e.g., encryption, secure communication protocols, and access controls) are converged to protect sensitive data and model interactions between entities [45]. Figure 3 illustrates the general view of FL's possible failures in networking. Throughout the overall execution flows, we point out the background of FL and its relationship with networking architecture. There are several challenges to handle, and from a researcher's perspective, complementary simulations of networking and FL are critical to gaining extensive experience before practically deploying their proposed approaches.
This section aims to gather comprehensive information related to FL frameworks and backgrounds studied in networking, especially the execution of FL frameworks in terms of initiate, participatory selection, model distribution, local model training, local mode updates, model update aggregate, and global update.
In this section, we primarily concentrate on illustrating the critical role of simulation performance by interacting network components, including user equipment (UE), radio access networks (RAN), core networks (CN), edge cloud (EC), software-defined networks (SDN), multi-access edge computing (MEC), and millimeter wave (mmWave). With several aspects given the intuitionistic abstract of relational network simulation tools, we can tackle representation for network performance in actual implementation and provide the design, optimization, and testing of 5G networking. Additionally, we can provide up-to-date trending networking to customized functions for evaluating and analyzing network performance and results. In this grading, we observe the state of simulation tools to accomplish scale-up, which assisted FL in ensuring the local data deserves security and privacy in many communication and computation aspects [46]. As in Table 6, we gather the trending networking simulation tools, which provide taxonomic and comprehensive information to involve within network protocol, standardization, management, orchestration, and network topology. Simulation tools can be specified depending on the use case and research objectives. The trend of network simulation tools and techniques are listed as follows: network simulation version 3 (NS-3), network simulation version 2 (NS-2), Mininet, Mininet-Wi-Fi, MATLAB, OMNet++, OpenDayLight, Floodlight, Ryu Controller, and OpenStack.
Platform | Summary | Primary focuses | Ref. | Year |
NS3 (C++, Python) | A simulator can provide an approach to enhance the comprehensive and flexible platform for computer network simulation. It also provides the ability to analyze and simulate network behaviors and several network standardization protocols, algorithms, scenarios, and topologies. Available: https://www.nsnam.org/ | - Customization and extension of its functionality - Supporting comprehensive network environment and network protocols - Execution of the simulation using an XML trace file - Support with OpenAI Gym |
[47,48,49] | 2008 |
NS2 (C++) | Designing to illustrate the behaviors of computer networks, which include wired and wireless communication, routing, and congest control protocol and transport (e.g., routing algorithm). Available: https://www.isi.edu/nsnam/ns/ |
- Routing - Switching - Extending to support IPv6 - Extending to support cloud computing |
[50] | 1997 |
Mininet (Python) | Open-source network emulator that provides to create, configure, and test network topologies, which supports large-scale network infrastructure and the integration with SDN controller using OpenFlow (simplifying the configuration on node, flow entry, and link). Available: http://mininet.org/ |
- Creation and design for a real-world SDN environment - Interconnecting API and AI - Exportation of network topology into Python script - Being mini-edit to visualize the topology |
[51] | 2010 |
Mininet-WiFi (Python) | Designing to evolve rapidly and develop to emulate wireless network controller environment that supports several wireless protocols such as Wi-Fi, ZigBee, and Bluetooth. Available: https://mininet-wifi.github.io |
- Development of a new scenario of the network wireless - Measurement of the performance of different Wi-Fi channels - Routing protocol for wireless connection mesh network - Providing a high degree of fidelity - Focusing on the security and scalability of mobile network |
[52] | 2021 |
MATLAB (Commercial) | Enabling cooperation with other programming languages and numerical computing environment, which provides more functions for modeling and simulating communication systems, wireless networks, cellular networks, and optical networks (enclosing several tools and libraries). Available: https://www.mathworks.com/help/index.html?s_tid=CRUX_lftnav |
- Simulation for network systems and protocols - Simulation for complex networks - Offering customization and development - Specification network function - areas or behaviors |
[53] | undefined |
OMNet++ (C++) | Designing a component architecture for models and providing a high degree of scaling to support an extensive network with millions of nodes, which is feasible to obtain a highly accurate result. Available: https://omnetpp.org/download/models-and-tools |
- Support model completeness - Simulation from LANs to WANs - Packet-switched and circuit-switched networks - Support for sensor networks and ad-hoc networks |
[54] | 1993 |
OpenDayLight (Java, Python, YAML) | Providing an open platform to focus on customizing and automating networks of network environment, which makes familiar with SDN controller scales up of the network device and network applications. Available: https://www.opendaylight.org |
- Combination of multiple service and protocol - Sustenance of various southbound APIs with network devices and protocol - Feasibly support of a wide range of standard networking protocols - Adaptation of NFV and SDN |
[55] | 2013 |
Floodlight (Java) | Offering greater flexibility, agility, and programmability in network management. Floodlight makes a rule of network policy and routing logic. Available: https://github.com/floodlight/floodlight |
- Support for multiple network protocols - Solution for flexible and scalable approaches |
[56] | 2014 |
Ryu-Controller (Python) | Supporting several protocols for managing network devices, such as OpenFlow, Netconf, and OF-config. Available: https://ryusdn.org/index.html |
- Creation flow table management - Traffic monitoring - Packet forwarding |
[57] | 2014 |
OpenStack | Open source offers a core network controller to accommodate network functions virtualization (NFV), it maintains the feature as a platform for network automation, orchestration, and management resources. Available: https://www.openstack.org |
- Network protocols and technologies - A private cloud platform - Enhancement of resources utilization and elastic hypervisors - RESTful API - Tools achieved with automation and integration |
[58] | 2010 |
In the 5G perspective, many simulation tools can offer advantages and leverage enhancements to indicate network performance and analysis. Due to differences in network settings, the platforms might gain fluctuation between QoS and QoE. Figure 4 shows the overview of simulations regarding setting scenarios, network environments, and differentiated network tiers; simulation tools can be performed depending on the characteristics of the network.
● NS3 gains interest from researchers in setting the network topologies to compromise the availability of RAN, mmWave, and handover processing. On the other hand, NS-3 has intensified by integrating an Open-AI gym into a collaboration capsule class that allows RL agents.
● MATLAB identifies tools used in many fields that can be simulated and analyzed by various network systems (e.g., communication networks, wireless networks, and network protocols). Network simulations can be performed on network performance analysis, which consists of analyzing network performance metrics such as latency, packet loss, packet drop ratio, packet size, throughput, and network capacity, by capturing the network routing and using a network protocol to simulate and analyze network routing algorithms such as border gateway protocol (BGP), open shortest path first (OSPF), and routing information protocol (RIP). Within this, MATLAB provides a built-in function and toolbox for simulation with transmission protocol control /internet protocol (TCP/IP), user data gram protocol/ internet protocol (UDP/IP), and Ethernet.
● Mininet is a widely used network emulator and simulator that provides fast simulation speed using OS-level virtualization function and thus has strengths in terms of scalability. It is a simulator that fully supports the OpenFlow protocol and works with various SDN devices. Mininet shows excellent compatibility with various currently released SDN controller open sources. However, the model diversity cannot simulate natural environments and multiple scenarios. The Mininet version includes standard switch and router models (the legacy model) alongside SDN-enabled switches and hosts. Mininet also offers a single link model that can configure detailed parameters like bandwidth, delay, and loss probability. Notably, Mininet lacks its own built-in traffic model and performance analysis capabilities. Instead, it relies on external traffic generation tools such as ping, iperf [59], and D-ITG [60]. Additionally, analyzing performance involves collecting and assessing packets or relying on other external tools. Consequently, there are limitations when using Mininet as a simulation tool for experiments to validate the stability and reliability of networking systems or those requiring a realistic network environment.
● Mininet-WiFi was established early with a wildly supported WiFi modules on the Mininet SDN network emulator and extended the function of the Mininet network by adding virtualized WiFi standard and access point (AP). The modules are based on Linux wireless drivers and 80211hwsim wireless simulation diver. Mininet-WiFi supports the use of spec mode.
● OMNet++ is an open source for academic, non-profit, and industrial purposes. OMNet++ is similar to NS3 and NS2 in the network simulation of both the wired network and wireless network, which holds capability to utilize for several objectives, such as queuing network modeling, mobility, and INET structure. OMNet++ also provides a use case under a graphical interface and limitation of network topology.
● OpenStack is an open source that allows for the building of a simplified platform. It provides flexible customization in implementation, fosters interoperability across deployment, and is easily accessible to meet the requirements of both users and operators (e.g., public and private cloud resource utilization). However, OpenStack can be considered to build a massively scalable operating system with elasticity and horizontal scalability in resources. OpenStack leads the several resource components and orchestration. On the other hand, a web-based application programmable interface (API) ensures that command and control aspects are computation, memory, storage, and networking.
● Floodlight is a controller platform that uses a Java-based OpenFlow controller to support orchestration virtualization and physical infrastructure and manages both OpenFlow and non-OpenFlow networks.
● OpenDayLight (ODL) was introduced by the open network foundation (ONF) in 2013 [61]. ODL controller offers enhanced reliability and clustering capabilities and resolves issues in older controllers. Numerous prominent companies have joined this initiative, aiming to create a resilient and effective system for large-scale industries. ODL significantly impacts SDN's business aspect, with most controllers choosing it as their foundation.
With several significant FL frameworks and network simulation tools, the primary purpose of conducting experimental simulations in networking for FL is to analyze, evaluate, and validate the performance, behavior, and feasibility of FL algorithms, protocols, and systems within network environments. Those simulations are vital for gaining into how FL operates in diverse network settings, considering factors like different device types, varying communication conditions, network heterogeneity, and privacy concerns. By simulating these scenarios, researchers can fine-tune algorithms, optimize parameters, and understand the implications of deploying FL in practical networking environments without real-world experimentation, accelerating research and development.
FL and networking complement each other by leveraging networking's data acquisition, edge and distributed computing capabilities, multi-round communications, and scalability deployment. At the same time, FL reinforces privacy preservation, model updates, latency reduction, learning robustness, and edge intelligence. Table 7 lists well-known platforms for conducting FL experiments. Academia and industry have created numerous platforms to facilitate the widespread adoption of FL, including Google AI, DeepMind, Facebook Research, WeBank+Linux Foundation, IBM, Microsoft, Intel, NVIDIA, etc. All platforms have the capability for the general FL with several dataset supports. We outline the summary specifications and functional focuses as follows:
Platform | Summary | Primary Focuses | Ref. | Year |
Flower (Python) |
Providing a unified approach to FL, federated evaluation, and federated analytics, which allows users to jointly federate different workloads, ML frameworks, and programming languages. Available: https://flower.dev |
- Offering large-scale experiments - Emphasis of heterogeneous participants - Transition to real-world devices - Integration of multiple ML training frameworks - Customizability and extensibility |
[62] | 2020 |
FedML (Python) |
Prioritization lightweight, cross-platform, and secure FL and federated analytics. Available: https://www.fedml.ai |
- Convergence of MLOps tools with decentralized learning - Vertical approaches to industries and applications - Scalability for data silos and rapid large model training |
[63] | 2020 |
FATE (Python) |
Designing to be industrial-grade with features such as scalability, security, and compliance (supports logistic regression, tree-based algorithms, deep learning, transfer learning, etc.). Available: https://fate.fedai.org |
- A wide range of secure computation protocols - FATE CLI, SDK, and dashboard - Design with distributed architecture, message-passing interface, and fault tolerance |
[64] | 2019 |
TFF (Python) |
Providing a flexible programming model for FL, as well as a variety of tools and libraries to support the development and deployment of FL applications. Available: https://www.tensorflow.org/federated |
- High-level interfaces (FL and FC API) including datasets, models, computation builders, client placement type, etc. - Integration with TensorFlow+Keras - Deployment to a variety of runtime environments (mobile devices, edge servers, and cloud platforms) |
[65] | 2019 |
PySyft (Python, Docker) |
Supporting a variety of techniques, such as differential privacy, secure multi-party computation, and homomorphic encryption. Available: https://openmined.github.io/PySyft/ |
- Extension to more privacy-preserving techniques - Support for multiple deployment environments - Security and private learning |
[66] | 2021 |
FLUTE (Python) |
Enabling rapid prototyping and simulation of new FL at scale with cloud integration and multi-GPU support, including novel optimization, privacy, and communications strategies. Available: https://github.com/microsoft/msrflute |
- A modular architecture for framework customization - A visualization tool for tracking the progress of FL - A possible integration with AzureML workspaces |
[67] | 2022 |
ns3-fl (C++, Python) |
Enhancement of the configuration of network settings and connecting to FL simulator, flsim, while implementation includes client-server ns3 and sync/async FL process communications. Available: https://github.com/eekaireb/ns3-fl |
- Emphasis of network settings - A power model of energy consuming while training clients - Enhancement of networking output reliability |
[68] | 2022 |
IBM FL (Python) |
Enabling distribution of machine learning in enterprise environments, prioritizing privacy, compliance, and data locality, and supporting various learning techniques and topologies. Available: https://ibmfl.res.ibm.com |
- Simplification of the adoption with infrastructure, coordination, and compatibility with common libraries and minimizes the learning curve - Deployment across various computing environments and designing custom fusion algorithms - A deep focus on the enterprise environment |
[69] | 2020 |
FFL-ERL (Erlang) |
Providing Erlang's suitability for FL, comparing its performance in two scenarios: a full Erlang implementation and a hybrid approach using C for numerical computations. Available: https://github.com/gregorulm/fcc_ffl_erl |
- Discussion of trade-off between C's high performance and Erlang's superior programmer productivity - Excellence in concurrency and distribution - Development and coordination capabilities |
[70] | 2018 |
OpenFL-XAI (Python, Docker) |
Emphasis privacy and explainability by focusing on the FL of Fuzzy Rule-Based Systems. Available: https://github.com/Unipisa/OpenFL-XAI |
- Intel's OpenFL framework - A solution for accurate, private, and interpretable AI applications - A trustworthy AI systems, privacy preservation, and transparency |
[71] | 2023 |
CrypTen (Python) |
Enabling privacy-preserving ML with secure multiparty computation with ML-centric features, a tensor library, and robust protocol implementations for real-world use. Available: https://crypten.ai |
abstractions for efficient and secure model evaluation - Simplification on secure ML for non-cryptography experts - Integration with PyTorch's familiar API |
[72] | 2021 |
FederatedScope (Python, Docker) |
Utilization of an event-based structure to grant users significant flexibility in autonomously defining the actions of distinct participants. Available: https://github.com/alibaba/FederatedScope |
- Support plug-in operations and components for enhanced privacy, attack simulation, and auto-tuning - Facilitation of the incorporation of diverse plug-in operations and components to enhance and streamline further development |
[73] | 2022 |
NVIDIA FLARE (Python) |
Providing an open-source SDK to ease building workflows with capabilities of scalable packaging, elastic, and lightweight. Available: https://github.com/NVIDIA/NVFlare |
- Multiple training and validation workflows - Federated analytics and lifecycle orchestration - Simplification on dashboard for management |
[74] | 2020 |
EasyFL (Python) | Targeting beginners with limited prior knowledge, while offering low-code platform, API design, and enhancing the deployment efficiency. Available: https://github.com/EasyFL-AI/EasyFL |
- Training outputs tracker - Simulation with statistical and system heterogeneity - Design on plug-in for training flow abstraction |
[75] | 2022 |
LEAF (Python) | Providing an adaptable benchmarking system designed for evaluating learning in federated settings with a collection of openly available federated datasets. Available: https://github.com/TalwalkarLab/leaf |
- Datasets: FEMNIST (Image classification), Sentiment140 (Sentiment analysis), Shakespeare (Next-character prediction), Synthetic (Classification), and Reddit (Next-word prediction) - Emphasis on learning in federated settings |
[76] | 2018 |
PaddleFL (Python, C++, Kubernetes) | Leveraging distributed training and Kubernetes-based job scheduling to offer scalable deployment, also easy replication. Available: https://github.com/PaddlePaddle/PaddleFL |
- Simplification of the deployment of FL systems on large-scale distributed clusters - A flexible framework with components for defining tasks, designing ML models, and handling distributed training configurations - Detail with run times on server, worker, and scheduler |
[77] | 2020 |
Figure 5 illustrates the overview of relations between FL for networking and networking for FL. These platforms provide comprehensive support for FL by offering various tools, libraries, and features tailored to different use cases and requirements. Flower stands out for its unified approach, emphasizing large-scale experiments, heterogeneous participant support, and multiple ML training frameworks. FedML prioritizes lightweight and secure FL, converging MLOps tools, and offering vertical approaches for various industries. FATE focuses on industrial-grade FL, supporting various algorithms, secure computation protocols, and a distributed architecture. TFF provides a flexible programming model, high-level interfaces, and easy deployment to diverse runtime environments. PySyft specializes in privacy-preserving techniques, offering support for multiple deployment environments. FLUTE enables rapid prototyping and simulation of FL at scale with modular architecture and cloud integration. ns3-fl enhances network settings and communications for FL simulation, while IBM FL focuses on enterprise environments, minimizing the learning curve and supporting custom fusion algorithms. FFL-ERL leverages Erlang concurrency and distribution capabilities for FL, OpenFL-XAI emphasizes privacy and explainability, and CrypTen bridges secure multiparty computation and ML. FederatedScope supports plug-in operations and components, NVIDIA FLARE simplifies workflows, EasyFL targets beginners with low-code and efficient deployment, and LEAF offers federated benchmarking with openly available datasets. Finally, PaddleFL simplifies deployment on large-scale clusters, providing flexibility for defining tasks and handling distributed training configurations, making these platforms valuable assets for the FL community. The lists of tools in Tables 6 and 7 complement each other in Table 8, which surveys articles about FL framework and simulation tools.
Paper | Performance matric | Aggregation approach | FL framework | Network simulation | Data acquisition | Ref. | Year |
L. Sami et al. | Throughput | FedAvg | Flower, FedScale, Flue | Unspecified | Google speech, OpenImage, Shakespeare | [78] | 2023 |
P. Tam et al. | Drop ratio, delivery ratio, delay, accuracy | FedAvg | TensorFlow Federated | Mininet, Ryu | MNIST and topology simulation | [79] | 2021 |
V. Balasubramanian et al. | Cache hit ratio, average delay | FedCo | PyTorch | Mininet | Simulation | [80] | 2021 |
R. Uddin et al. | Precision, recall, F1-score, accuracy | AWS OpenGrid | OpenMined | Mininet, Ryu | STIN SAT20, simulation (SDN) | [81] | 2023 |
V. Balasubramanian et al. | MNO revenue, cache hit ratio, average access latency, percentage error in placement | FedCo | Python | Mininet-Wifi | Simulation | [82] | 2021 |
In this section, we brought up five primary sector domains that are discussed about learning performance, QoS, energy consumption, and cost that recent studies have proposed using FL-based approaches for networking as Figure 6 and Table 9.
Categorize | Methodology | Significance | Performance matric | Simulation/framework | Ref. | Year |
Learning performance | Edge client devices. | Accuracy and loss. | GNS3, OpenDayLight, NETCONF and RESTCONF/Flower, FedAvg | [83] | 2023 | |
TD-EPAD algorithm | Trustiness of training data in SD-IIoT. | Accuracy. | Mininet, Floodlight | [84] | 2023 | |
Quality of service | Supporting vector machine | Handling on allocating the network resource and controlling massive communication in backbone. | Delay, jitter, packet drop ratio, packet delivery ratio, throughput. | NS-3 | [87] | 2021 |
CUPE algorithm | Minimization of the content fetch delay for latency- sensitive of IoT. | Cach hit rate, content fetch delay. | Unspecified | [88] | 2023 | |
DDQN | Overcoming on the minimization of energy consumption, completion time, and round communication between local participant and node selection. | Packet drops ratio, throughput, overall accuracy, delay, and packet delivery ratio. | NS-3, Mininet, mini-nfv, OASIS TOSCA | [89] | 2022 | |
MultiFed, a multi-center FL framework | Acceleration for better convergence, heterogeneity handling, and improvement on scalability for privacy preservation in cloud-edge collaboration. | Total communication rounds, total communication delay, and total communication volume. | Unspecified | [90] | 2023 | |
Energy consumption | MIBLP, DDQN, FedRL algorithm | Minimization of the long-term delay and energy consumption of an IoT device. | Cost per user. | Unspecified | [92] | 2021 |
Cost | Fed-average algorithm | Approvement on client selection and optimizing model aggregation. | Averaging training accuracy, energy consumption of unit loss delay. | Unspecified | [95] | 2022 |
FL with networking is an extension of collaborative ML approaches that allows multiple decentralized devices or servers to collaborate to train shared models while keeping local and private data. FL has been increased to coordinate with learning performance in terms of computational capacity, amount of memory, and communication resources, which contribute to the learning process of privacy preservation for sensitive information.
In [83], authors proposed SDN support to the FL. Implementing the FL framework (FL client and FL server) is supported at the application level and network layer (SDN controller). SDN is handled with data empirically in the FL process, and this paper focuses on the less-explored issue of using FL for high-sensitive application applications. Hence, edge devices have experienced delays due to communication overload. Lastly, SDN maintains efficiency FL even in meeting heavy conditions. SDN-assisted FL can significantly reduce processing time with minimal signaling overhead to the form of the controller. Moreover, the authors aimed to enhance the security and trustworthiness of FL in the software-defined industrial internet of things (SD-IIoT) [84,85]. Implementing multi-tentacle FL (MTFL) frameworks assists in solutions to the growing prevalence of poisoning attacks. MTFL groups participants with similar training data and mode parameters into a "tentacle group". Along with impacting adaptive poisoning data, a stochastic tentacle data exchanging (STDE) protocol was utilized. The protocol involved adding Gaussian noises to exchange data, which differs from traditional defense mechanisms in that all exchanged data will be processed using differential privacy technology to safeguard tentacle privacy.
QoS is the major evaluation metrics for both aspects, namely E2E networking and FL multi-round communications. Each metric is essential for guaranteeing the delivery of services, especially in mission-critical applications. Several aspects of FL frameworks are to ensure that it can more effectively and precisely match the QoS requirements of the application. QoS indicates important things within the premises that as massive data gathering and data transmission [86]. Edge caching based on the fog computing network is considered a provisioning potential solution to tackle the latency and further content fetch delay and minimize the QoS of the end-to-end (E2E) delay. To prevent network congestion, bottlenecks, and the risk of data user privacy leakage, FL is ultimately used to enable E2E-assisted fog computing networks and edge virtualization [87,88,89]. In a novel multi-center FL framework for QoS prediction which utilized the central server from the cloud to the edge and trains global models in edge regions [90]. This framework employs two gradient aggregation strategies: 1) internal aggregation for regional users and 2) external aggregation among edge servers with cloud server assistance.
When referring to FL for network environment, energy and power consumption are important factors as recently demonstrated by the many reviewed studies. Because FL distributes the training process across devices on the network, interruptions such as power supply issues, network disconnections, and other disruptions can lead to a device pausing or discontinuing the learning process after a certain number of epochs. Moreover, when discussing the FL approach, it is essential to acknowledge the necessity of constructing ML models efficiently. These models should be capable of acquiring knowledge from historical IoT sensor data, which is employed to enhance energy efficiency and reduce costs [91]. Therefore, the significance of optimizing the energy consumption objective becomes more pronounced when taking the entire FL-IoT context. [92] presents the problem as one that centered on reducing both the delay and energy consumption of IoT devices within FL systems. The authors considered various factors, including the decision of whether to offload tasks to edge or cloud resources, the allocation of computation resources, local processing, and transmission power. Their primary objective is to minimize the long-term delay and energy usage. This problem was a multi-agent DRL challenge, which they tackled using a double-deep Q-learning (DQL) network through a DQL agent. To address the decision of whether to offload a task for processing. In the subsequent phase, they focused on allocating computation and communication resources [93]. Moreover, they employed the FedRL approach, where each IoT device trained its own DQL models, shared these models with a centralized controller, and updated the models in a central aggregation unit [94]. QoS for tasks and task queue length, exhibited fluctuations, selecting an efficient update frequency for the target network to stabilize the environment, leading to improved solutions for the agent. It is important to mention that the authors did not discuss the results related to cost minimization in their study.
When considering the goal of optimizing network costs, the primary focus is on two components: operational expenses (OPEX) associated with capital expenditure (CAPEX). For instance, in the context of FL implementations, communication often acts as a significant bottleneck, and thus, reducing communication costs involves optimizing connections [95]. Within this section, one can observe a variety of approaches to representing costs in the FL-IoT environment. Different research studies have been adopted for various metrics to achieve cost optimization. As a result, we encounter different terminologies used by different authors in this section. To enhance clarity, we have provided the specific cost metric for each study. [96] considers the battery-constrained federated edge learning problem for their operating CPU frequency for both prolonging the battery life and avoiding the untimely dropping from FL training. The primary objective was to reduce the overall system which was determined by both latency and energy consumption. To achieve this, the authors devised a resource allocation scheme to adjust the CPU frequency of devices and allocate wireless bandwidth. They introduced a DDPG-based allocation strategy to address this issue. Under this approach, clients were required to report their available resources and the server had to estimate the channel parameters for communication between users and devices. Additionally, the base station had to inform participants about the current CPU frequency and available wireless bandwidth. Consequently, the agent received varying rewards to meet both latency and energy consumption requirements. The proposed DDPG strategy outperformed E-DDPG (DDPG strategy with even bandwidth allocation) in terms of system cost. Mainly, it effectively utilized wireless bandwidth resources during the learning process, resulting in improved system performance. Moreover, DDPG performed better than alternative methods across various bandwidth values, as it efficiently leveraged system communication and computational resources.
Key performance indicators (KPIs) in FL in networking aim to measure the effectiveness and overall performance of collaborative model training of FL systems across decentralized devices while preserving user privacy. Here are the following key aspects:
● Speed and convergence: Introduces a novel framework that optimizes FL by employing multiple edge servers. This framework likely includes mechanisms for efficient communication, aggregation of model updates, and strategies to minimize latency during the learning process [97]. It also discusses empirical results and performance evaluations of FedMes, showcasing improvements in FL speed, convergence rates, and overall efficiency compared to traditional FL setups. [98] The primary focus is on improving the speed and convergence of FL algorithms, specifically by leveraging the momentum gradient descent (MGD) technique. MGD can be a valuable technique for accelerating FL, leading to faster model training, and potentially improving resource efficiency. The proposed adaptive FedMGD further enhances the performance by adapting to device heterogeneity.
● Delay: The majority of techniques must be solved to accelerate the model updates and parameters between the central server and participating devices. [99] The authors propose a dynamic sampling and adaptive resource allocation (DSARA) framework to minimize service delays in mobile FL. The algorithm incorporates the latest local model updates and resource constraints to select the optimal device subset for each round. It offers a valuable solution for enabling efficient and delay-aware FL on mobile devices, paving the way for practical applications in mobile edge computing and distributed ML.
● Communication round: The backbone of information exchange in FL, enabling collaborative model training while preserving data privacy on participating devices. 1) infrequent rounds with compressed updates achieved decent accuracy while minimizing bandwidth usage, 2) strategic selection of participating devices based on data relevance further improved efficiency, and 3) federated averaging with weights assigned based on update quality accelerated convergence towards the optimal model. Furthermore, it demonstrates the importance of optimizing communication rounds for resource-constrained networks in real-world FL applications. Moreover, [100] aims to decrease the size of models produced by both the server and clients while adjusting the FL procedure. Initially, the server creates a smaller sub-model with fewer parameters using federated dropout. Subsequently, the resulting model undergoes lossy compression on the server's end and transmits to the clients. The clients then decompress the model to begin training. After training, the updates are compressed and sent back to the server, which decompresses and combines the final model. The communication bottleneck in the federated averaging method is due to limited bandwidth, causing delays for clients in uploading their updates.
● Resource utilization: In FL-based IoT environments, we observe many heterogeneous IoT devices that are in nature and process limited resources. Hence, FL trains operated through efficient client selection and optimized resource utilization. In [101], it tackled the hurdles of unreliable wireless communication in FL. They built a framework that joins learning tasks with wireless communication on multiple devices. Recognizing issues like packet errors and limited bandwidth, they devised an optimization plan to minimize training errors while allocating resources and wisely choosing participating users. The framework accounts for how wireless channels affect learning, leading to a precise formula for predicting how well the model will train. This breakthrough could pave the way for reliable and efficient FL in resource-constrained settings like wireless networks.
This section focuses on utilizing FL case studies regarding learning performance, QoS, energy consumption, and cost. Meanwhile, KPIs are primarily impacted by FL to show the effectiveness of its ability to adapt to speed and convergence, delay, communication round, and resource utilization.
This section presents the main research challenges and future directions in this recent review within several issues to tackle for supporting FL with network simulation tools. However, this field also faces several challenges and has numerous future directions to explore, as follows:
● Communication overhead: To prevent communication overhead during communication between simulation tools and other tools for collaborating interactions, which is a critical phase to consider accurately in network environments of heterogeneous IoT. The primary purpose of maintaining communication and synchronization among various network components is the interface, nodes, or entities involved. For instance, where a client faces limited bandwidth, effective communication with the FL server during model training becomes challenging. Similarly, clients with insufficient processing capabilities find it impractical to execute assigned local computational tasks [102,103,104,105]. Additionally, when dealing with extensive data across the network, the resulting large model size poses difficulties for resource-constrained clients. Efficient training in such large data networks necessitates compressing client models, minimizing the burden on clients with constrained resources. If many FL clients grapple with resource limitations, the FL process demands increased server-client interactions to achieve target convergence. However, clients may find bearing the associated high communication costs prohibitive.
● Operational bandwidth cost: Offering the operational bandwidth cost is one of the concern cases in networking operations and a critical consideration as FL relies on the exchange of data and model between participants and global server. The following factors can involve operational bandwidth costs in FL: 1) exchanging data for local collaboration, 2) the frequency of participant-server round communications, 3) the distance/mobility between participants, 4) networks with lower bandwidth capacity [106] (cellular networks) will have higher bandwidth costs than networks with higher bandwidth capacity [107], and 5) the number of simulators/platforms involved in the deployment. Therefore, utilizing a (edge) cloud-based FL platform can optimize bandwidth cost by optimizing data transfer, edge aggregation, and caching capabilities.
● Expansion of multi-awareness learning (MAL): FL framework enables the ML model to learn from distributed data while preserving privacy and reducing communication costs. Network simulation offers exciting opportunities for enhancing network performance through innovative methods. For instance, MAL models can dynamically fine-tune routing algorithms, congestion control protocols, and power management strategies, improving network throughput, decreasing latency, and energy conservation. One approach for extending MAL to network simulation is to adopt a reinforcement learning methodology. In this method, the MAL model is trained to interact with a simulated network environment, learning to take actions that maximize a predefined reward. Reward functions can be tailored to represent specific network performance objectives, such as achieving higher throughput, lower latency, or reduced energy consumption. Another approach for integrating MAL into network simulation involves using supervised learning. The MAL model was trained using a historical network data dataset annotated with desired network performance metrics. The expansion of MAL into network simulation presents a promising avenue of research with the potential to transform how networks are conceived and administered. MAL can create more efficient, dependable, and sustainable network infrastructures by empowering ML models to glean insights from distributed data and adapt to evolving network conditions. The specification terms of how MAL could optimize network performance are routing optimization, congestion control, and power management. The limitation emerges when a network characteristic relies on the models of its components. These models tend to oversimplify the real-world scenario and the performance of real-world networks is impacted by the variability in conditions, including traffic loads, hardware failures, and software bugs. These factors can be challenging to model accurately in a simulator.
● Quantum computing for FL: Quantum computing presents the opportunity for substantial benefits compared to classical machine learning algorithms [108]. By harnessing quantum superposition and entanglement, quantum algorithms can conduct computations in parallel, potentially leading to faster processing and heightened efficiency. Quantum ML (QML) algorithms exhibit superior effectiveness in managing extensive datasets and intricate patterns, enhancing learning capabilities. Moreover, quantum algorithms can extract information from quantum state transformations and interference, resulting in more accurate predictions. While the realization of quantum advantage in QFL is an ongoing area of research and development, the distinctive features of quantum computing show potential for addressing complex computational tasks and introducing novel possibilities in data analysis and pattern recognition. It challenges the following tantalizing options: 1) collaborative learning across a vast network, 2) data privacy, 3) communication, 4) gradient leakage, 5) compromised client, and 6) compromised server.
● Security vulnerabilities: The distributed training method in FL provides an avenue for engaging a substantial client base, potentially extending to millions of clients. Within the FL framework, clients are not inherently trustworthy and each client may possess varying degrees of malicious or adversarial capabilities. [109] The server employs a random selection process for clients, allowing them to participate in FL training iterations. Identifying malicious clients in a training session becomes challenging when dealing with thousands or even millions of clients. Consequently, malicious clients may exploit the system to gain access to and learn about the privacy of other clients participating in the same iteration.
● Differential privacy: DP may lead to exposing sensitive data information. FL can preserve privacy across various applications, including social media, innovative city applications, healthcare [110], and traffic management. In these applications, sensitive data is stored locally on mobile or edge devices [111]. Only the model parameters derived from these devices are transmitted to the global FL to train the overarching machine-learning model. The subsequent discussion delves into recent studies and efforts to utilize FL to preserve privacy in these contexts.
At the end of this section, FL demonstrates its increasingly significant role in IoT networks and applications. We also raise several critical research challenges and current issue trends to be considered for further FL-networking system implementation. We list several challenges concerning FL with network simulation, such as communication overhead, operation bandwidth cost, expansion of multi-awareness learning, security vulnerabilities, and differential privacy.
In this paper, we presented a survey of experimental simulations for privacy-preserving FL in intelligent networking. We examined the potential relationship between the network simulation tool and the FL framework. An introduction with a motivational statement from networking and FL is gathered comprehensive terms in novelty concepts and technologies recently. Then, we described the preliminary studies for FL frameworks that can be leveraged to achieve learning approaches with intelligence networks. Afterward, we provided networking environments that provide critical support for data acquisition, edge computing capabilities, round communication, connectivity, and scalable topologies. Moreover, FL can leverage capabilities to achieve learning adaptation, low-latency operation, edge intelligence, personalization, and privacy preservation. Additionally, we brought up case studies of FL potential in terms of learning performance, QoS, energy consumption, and cost. Finally, we discussed potential challenges and future directions that could provide valuable ways for researchers in the recently trending field development to enhance the application in practical, real-world systems.
The authors declare they have not used Artificial Intelligence (AI) tools in the creation of this article.
This work was supported by Institute of Information & communications Technology Planning & Evaluation (IITP) grant funded by the Korea government (MSIT) (No. RS-2022-00167197, Development of Intelligent 5G/6G Infrastructure Technology for The Smart City), in part by the National Research Foundation of Korea (NRF), Ministry of Education, through Basic Science Research Program under Grant NRF-2020R1I1A3066543, in part by BK21 FOUR (Fostering Outstanding Universities for Research) under Grant 5199990914048, and in part by the Soonchunhyang University Research Fund.
The authors declare there is no conflict of interest.
[1] |
C. O. Alves, A. B. Nóbrega and M. Yang, Multi-bump solutions for Choquard equation with deepening potential well, Calc. Var. Partial Differ. Equ., 55 (2016), Art. 48, 28 pp. doi: 10.1007/s00526-016-0984-9
![]() |
[2] |
Existence of semiclassical ground state solutions for a generalized Choquard equation. J. Differ. Equations. (2014) 257: 4133-4164. ![]() |
[3] |
Multiplicity and concentration of solutions for a quasilinear Choquard equation. J. Math. Phys. (2014) 55: 423-443. ![]() |
[4] |
Y. Ao, Existence of solutions for a class of nonlinear Choquard equations with critical growth, Appl. Anal., (2019), 1–17. doi: 10.1080/00036811.2019.1608961
![]() |
[5] |
On a system involving a critically growing nonlinearity. J. Math. Anal. Appl. (2012) 387: 433-438. ![]() |
[6] |
Positive solutions of nonlinear elliptic equations involving critical Sobolev exponents. Commun. Pur. Appl. Math. (1983) 36: 437-477. ![]() |
[7] |
L. Cai and F. Zhang, The Brezis-Nirenberg type double critical problem for the Choquard equation, SN Partial Differential Equations and Applications, 1 (2020). doi: 10.1007/s42985-020-00032-0
![]() |
[8] |
An existence result for nonlinear elliptic problems involving critical Sobolev exponent. Ann. Inst. H. Poincaré Anal. Non Linéaire (1985) 2: 463-470. ![]() |
[9] |
Some existence results for superlinear elliptic boundary value problems involving critical exponents. J. Funct. Anal. (1986) 69: 289-306. ![]() |
[10] |
Multiple solutions to a magnetic nonlinear Choquard equation. Z. Angew. Math. Phys. (2012) 63: 233-248. ![]() |
[11] |
Xiaojing Feng, Ground state solution for a class of Schrödinger-Poisson-type systems with partial potential, Z. Angew. Math. Phys., 71 (2020), Paper No. 37, 16 pp. doi: 10.1007/s00033-020-1254-4
![]() |
[12] |
The Brezis-Nirenberg type critical problem for the nonlinear Choquard equation. Sci. China Math. (2018) 61: 1219-1242. ![]() |
[13] |
C. Y. Lei, G. S. Liu and H. M. Suo, Positive solutions for a Schrödinger-Poisson system with singularity and critical exponent, J. Math. Anal. Appl., 483 (2020), 123647, 21 pp. doi: 10.1016/j.jmaa.2019.123647
![]() |
[14] |
F. Li, Y. Li and J. Shi, Existence of positive solutions to Schrödinger-Poisson type systems with critical exponent, Commun. Contemp. Math., 16 (2014), 1450036, 28 pp. doi: 10.1142/S0219199714500369
![]() |
[15] |
F. Li, L. Long, Y. Huang and Z. Liang, Ground state for Choquard equation with doubly critical growth nonlinearity, J. Qual. Theory Differ. Equ., (2019), 1–15. doi: 10.14232/ejqtde.2019.1.33
![]() |
[16] |
Ground states for Choquard equations with doubly critical exponents. Rocky Mountain J. Math. (2019) 49: 153-170. ![]() |
[17] |
Existence of a ground state solution for Choquard equation with the upper critical exponent. Comput. Math. Appl. (2018) 76: 2635-2647. ![]() |
[18] |
Existence and uniqueness of the minimizing solution of Choquard's nonlinear equation. Studies in Appl. Math. (1976/77) 57: 93-105. ![]() |
[19] |
The Choquard equation and related questions. Nonlinear Anal. (1980) 4: 1063-1072. ![]() |
[20] |
Classification of positive solitary solutions of the nonlinear Choquard equation. Arch. Ration. Mech. Anal. (2010) 195: 455-467. ![]() |
[21] |
Groundstates of nonlinear Choquard equations: Existence, qualitative properties and decay asymptotics. J. Funct Anal. (2013) 265: 153-184. ![]() |
[22] |
P. H. Rabinowitz, Minimax Methods in Critical Point Theory with Applications to Differential Equations, Published for the Conference Board of the Mathematical Sciences by the American Mathematical Society, Providence, RI, 1986. doi: 10.1090/cbms/065
![]() |
[23] |
Nonlinear Choquard equations: Doubly critical case. Appl. Math. Lett. (2018) 76: 148-156. ![]() |
[24] |
M. Struwe, Variational Methods, Springer-Verlag, Berlin, 1990. doi: 10.1007/978-3-662-02624-3
![]() |
[25] |
Groundstates for a local nonlinear perturbation of the Choquard equations with lower critical exponent. J. Math. Anal. Appl. (2018) 464: 1184-1202. ![]() |
[26] |
M. Willem, Minimax Theorems, Birkhäuser Boston, 1996. doi: 10.1007/978-1-4612-4146-1
![]() |
[27] |
M. Willem, Functional Analysis, Springer New York, 2013. doi: 10.1007/978-1-4614-7004-5
![]() |
[28] |
On the critical cases of linearly coupled Choquard systems. Appl. Math. Lett. (2019) 91: 1-8. ![]() |
[29] |
Existence, uniqueness and multiplicity of positive solutions for schrödinger-Poisson system with singularity. J. Math. Anal. Appl. (2016) 437: 160-180. ![]() |
[30] |
Existence and asymptotic behavior of positive solutions for Kirchhoff type problems with steep potential well. J. Differ. Equations (2020) 269: 10085-10106. ![]() |
1. | Prohim Tam, Seyha Ros, Inseok Song, Seungwoo Kang, Seokhoon Kim, A Survey of Intelligent End-to-End Networking Solutions: Integrating Graph Neural Networks and Deep Reinforcement Learning Approaches, 2024, 13, 2079-9292, 994, 10.3390/electronics13050994 | |
2. | Alaelddin F. Y. Mohammed, Joohyung Lee, Sangdon Park, Dynamic Bandwidth Slicing in Passive Optical Networks to Empower Federated Learning, 2024, 24, 1424-8220, 5000, 10.3390/s24155000 | |
3. | Makara Mao, Ahyoung Lee, Min Hong, Efficient Fabric Classification and Object Detection Using YOLOv10, 2024, 13, 2079-9292, 3840, 10.3390/electronics13193840 | |
4. | Seyha Ros, Prohim Tam, Inseok Song, Seungwoo Kang, Seokhoon Kim, Handling Efficient VNF Placement with Graph-Based Reinforcement Learning for SFC Fault Tolerance, 2024, 13, 2079-9292, 2552, 10.3390/electronics13132552 | |
5. | Seyha Ros, Seungwoo Kang, Inseok Song, Geonho Cha, Prohim Tam, Seokhoon Kim, Priority/Demand-Based Resource Management with Intelligent O-RAN for Energy-Aware Industrial Internet of Things, 2024, 12, 2227-9717, 2674, 10.3390/pr12122674 |
Acronym | Description |
API | Application programming interface |
CAPEX | Capital expenditure |
DDQN | Double deep Q-network |
DFQL | Deep federated Q-learning |
DL | Deep learning |
DNN | Deep neural network |
DRL | Deep reinforcement learning |
DSRA | Device selection and resource allocation |
DQL | Deep Q-learning |
EC | Edge cloud |
E2E | End-to-end |
FL | Federated learning |
HFL-VNE | Horizontal federated learning-virtual network embedding |
IID | Independent-and-identically-distributed |
IIoT | Industrial internet of things |
IoV | Internet of vehicles |
IoT | Internet of things |
MEC | Multi-access edge computing |
MFL | Multilevel federated learning |
ML | Machine learning |
MTFL | Multi-tentacle federated learning |
NFV | Network functions virtualization |
NFVeEC | NFV-enabled EC |
QoE | Quality of experience |
QoS | Quality of service |
RAN | Radio access network |
SDN | Software-defined networks |
VGAE | Variational graph autoencoder |
Survey paper | IoT networks | Overview of FL | Framework details | Key performance indicators | Simulation tools in networks | Ref. | Year |
W. Lim et al. | ✓ | ✓ | ✓ | ✘ | ✘ | [22] | 2020 |
D. Nguyen et al. | ✓ | ✓ | ✓ | ✘ | ✘ | [23] | 2021 |
R. Gupta et al. | ✘ | ✓ | ✓ | ✓ | ✘ | [24] | 2022 |
L. Witt et al. | ✘ | ✓ | ✓ | ✘ | ✘ | [25] | 2023 |
H. Chen et al. | ✘ | ✓ | ✓ | ✘ | ✘ | [26] | 2023 |
M. Al-Quraan et al. | ✘ | ✓ | ✓ | ✘ | ✘ | [27] | 2023 |
Summary of execution flows | Target objectives | Ref. | Year |
The proposed algorithm features two components: 1) An online learning component based on previous resource usage and training results for making fractional decisions, and 2) An internet-based rounding tool that transforms fractional choices into whole number selections. | Prioritization in cumulative utilization of computation and communication resources, while ensuring the convergence of both local and aggregated models. | [30] | 2020 |
The Power-of-Choice selection strategy works as follows: 1) The central server calculates the local losses of all clients, 2) The server sorts the clients in decreasing order of their local losses, and 3) The server selects the top set of clients to participate in the current training round. | Improvement of the convergence speed and accuracy of FL while reducing communication and computation overhead (target applications can be distributed machine learning (ML), mobile edge computing, and IoT). | [31] | 2022 |
"PyramidFL" is a fine-grained client selection method that first determines the utility-based client selection from the global view and then optimizes its utility profiling locally for further client selection. | Designing to speed up the FL training while achieving a higher final model performance, also prioritizing the use of clients with higher statistical and system utility. | [32] | 2020 |
1) Deadline-based aggregation: Aggregate client updates once a fixed deadline is met. 2) Joint optimization: two subproblems on client selection and parameter update. 3) E3CS: an exponential-weight algorithm for exploration and exploitation-based client selection. | Improvement of the training efficiency and final model accuracy in FL within a volatile training context, where clients are prone to failures. | [33] | 2022 |
"Newt" is an enhanced FL approach that includes a new client selection utility and fine-grained control on selection frequency. | Enabling efficient selection in intelligent transportation systems, which has challenges such as data and device heterogeneity and highly dynamic system. | [34] | 2022 |
Summary of execution flows | Target objectives | Ref. | Year |
"FL+HC" clusters clients are grouped based on how closely their local updates match the overall global model. After this grouping, the clusters are trained autonomously and simultaneously using dedicated models. | Improvement of the accuracy of the test set while minimizing the communication rounds needed to achieve convergence in FL, especially when dealing with non-independent and non-identically distributed (non-IID) data. | [35] | 2020 |
1) Bandwidth allocation: involves assigning additional bandwidth to devices that experience poorer channel conditions or possess less robust computational capabilities. 2) Device scheduling: suggests an approach that prioritizes selecting devices with the shortest model updating time until a favorable balance is struck between learning efficiency and round-trip latency. | Maximization of the convergence rate of FL training with respect to time rather than rounds, which can be achieved by minimizing the expected time for FL training to attain certain model accuracy. | [36] | 2020 |
Each client offloads partial data to the edge server for processing, and then, the clients update their local models using the remaining data (Meanwhile, the edge server updates the global model using the aggregated data). | Mitigation of the straggler effect in FL and improving the system efficiency (The method can be used in applications, such as mobile device training, IoT device training, or healthcare data training). | [37] | 2021 |
The optimal offloading strategy is derived by minimizing the FL loss function under the latency constraint and the Q-learning-based offloading strategy is proposed for the imperfect channel state information scenario. | Improvement of training efficiency and mitigating the straggler effect of FL in industrial IoT networks. | [38] | 2023 |
The greedy algorithm is proposed by iteratively assigning communication resources to the edge nodes that consume the least energy for local model training in each round, which proceeds until the communication resource budget is finished. | Training machine learning models for edge-assisted Internet of Agriculture Things applications, where data are both vertically and horizontally partitioned, and resources are limited. | [39] | 2022 |
Summary of execution flows | Target objectives | Ref. | Year |
1) Unbiased gradient aggregation: Evaluate the gradients against the global model parameters in the last local epoch, and 2) FedMeta: Perform meta updating on the global model parameters using a small set of data samples indicating the expected target distribution. | Improvement of the convergence speed and accuracy (Both unbiased gradient aggregation and FedMeta can be applied individually or together and can be integrated into existing FL). | [40] | 2020 |
1) Partition the network into groups and local model updates into segments, 2) Apply the aggregation protocol to segments with specific coordination between users, and 3) Aggregate the aggregated segments to obtain the global update. | Enabling secure model aggregation in FL systems, while allowing users to adjust the quantization proportional to their communication resources. | [41] | 2022 |
The method aggregates updates from scheduled devices using an age-aware weighting design, and the weights are assigned to each update based on the freshness of the data, with more recent updates receiving higher weights. | Development of an asynchronous FL framework with periodic aggregation that can support heterogeneous computation capabilities and training data distributions. | [42] | 2021 |
The method uses a feedback mechanism to communicate the estimated global update to each client, which then calculates the relevance of its local update to the estimated global update (If the relevance is lower than a predefined threshold, the client does not update). | Mitigation of communication overhead, which can be applied to a variety of FL applications, such as personalized healthcare, intelligent transportation systems, and distributed machine learning for financial services. | [43] | 2019 |
Participants apply linear transformation to the model update vector, then partially encrypt the transformed vector using multi-input functional encryption. | Design of an efficient secure aggregation scheme, which can protect the security of model updates without losing efficiency. | [44] | 2020 |
Platform | Summary | Primary focuses | Ref. | Year |
NS3 (C++, Python) | A simulator can provide an approach to enhance the comprehensive and flexible platform for computer network simulation. It also provides the ability to analyze and simulate network behaviors and several network standardization protocols, algorithms, scenarios, and topologies. Available: https://www.nsnam.org/ | - Customization and extension of its functionality - Supporting comprehensive network environment and network protocols - Execution of the simulation using an XML trace file - Support with OpenAI Gym |
[47,48,49] | 2008 |
NS2 (C++) | Designing to illustrate the behaviors of computer networks, which include wired and wireless communication, routing, and congest control protocol and transport (e.g., routing algorithm). Available: https://www.isi.edu/nsnam/ns/ |
- Routing - Switching - Extending to support IPv6 - Extending to support cloud computing |
[50] | 1997 |
Mininet (Python) | Open-source network emulator that provides to create, configure, and test network topologies, which supports large-scale network infrastructure and the integration with SDN controller using OpenFlow (simplifying the configuration on node, flow entry, and link). Available: http://mininet.org/ |
- Creation and design for a real-world SDN environment - Interconnecting API and AI - Exportation of network topology into Python script - Being mini-edit to visualize the topology |
[51] | 2010 |
Mininet-WiFi (Python) | Designing to evolve rapidly and develop to emulate wireless network controller environment that supports several wireless protocols such as Wi-Fi, ZigBee, and Bluetooth. Available: https://mininet-wifi.github.io |
- Development of a new scenario of the network wireless - Measurement of the performance of different Wi-Fi channels - Routing protocol for wireless connection mesh network - Providing a high degree of fidelity - Focusing on the security and scalability of mobile network |
[52] | 2021 |
MATLAB (Commercial) | Enabling cooperation with other programming languages and numerical computing environment, which provides more functions for modeling and simulating communication systems, wireless networks, cellular networks, and optical networks (enclosing several tools and libraries). Available: https://www.mathworks.com/help/index.html?s_tid=CRUX_lftnav |
- Simulation for network systems and protocols - Simulation for complex networks - Offering customization and development - Specification network function - areas or behaviors |
[53] | undefined |
OMNet++ (C++) | Designing a component architecture for models and providing a high degree of scaling to support an extensive network with millions of nodes, which is feasible to obtain a highly accurate result. Available: https://omnetpp.org/download/models-and-tools |
- Support model completeness - Simulation from LANs to WANs - Packet-switched and circuit-switched networks - Support for sensor networks and ad-hoc networks |
[54] | 1993 |
OpenDayLight (Java, Python, YAML) | Providing an open platform to focus on customizing and automating networks of network environment, which makes familiar with SDN controller scales up of the network device and network applications. Available: https://www.opendaylight.org |
- Combination of multiple service and protocol - Sustenance of various southbound APIs with network devices and protocol - Feasibly support of a wide range of standard networking protocols - Adaptation of NFV and SDN |
[55] | 2013 |
Floodlight (Java) | Offering greater flexibility, agility, and programmability in network management. Floodlight makes a rule of network policy and routing logic. Available: https://github.com/floodlight/floodlight |
- Support for multiple network protocols - Solution for flexible and scalable approaches |
[56] | 2014 |
Ryu-Controller (Python) | Supporting several protocols for managing network devices, such as OpenFlow, Netconf, and OF-config. Available: https://ryusdn.org/index.html |
- Creation flow table management - Traffic monitoring - Packet forwarding |
[57] | 2014 |
OpenStack | Open source offers a core network controller to accommodate network functions virtualization (NFV), it maintains the feature as a platform for network automation, orchestration, and management resources. Available: https://www.openstack.org |
- Network protocols and technologies - A private cloud platform - Enhancement of resources utilization and elastic hypervisors - RESTful API - Tools achieved with automation and integration |
[58] | 2010 |
Platform | Summary | Primary Focuses | Ref. | Year |
Flower (Python) |
Providing a unified approach to FL, federated evaluation, and federated analytics, which allows users to jointly federate different workloads, ML frameworks, and programming languages. Available: https://flower.dev |
- Offering large-scale experiments - Emphasis of heterogeneous participants - Transition to real-world devices - Integration of multiple ML training frameworks - Customizability and extensibility |
[62] | 2020 |
FedML (Python) |
Prioritization lightweight, cross-platform, and secure FL and federated analytics. Available: https://www.fedml.ai |
- Convergence of MLOps tools with decentralized learning - Vertical approaches to industries and applications - Scalability for data silos and rapid large model training |
[63] | 2020 |
FATE (Python) |
Designing to be industrial-grade with features such as scalability, security, and compliance (supports logistic regression, tree-based algorithms, deep learning, transfer learning, etc.). Available: https://fate.fedai.org |
- A wide range of secure computation protocols - FATE CLI, SDK, and dashboard - Design with distributed architecture, message-passing interface, and fault tolerance |
[64] | 2019 |
TFF (Python) |
Providing a flexible programming model for FL, as well as a variety of tools and libraries to support the development and deployment of FL applications. Available: https://www.tensorflow.org/federated |
- High-level interfaces (FL and FC API) including datasets, models, computation builders, client placement type, etc. - Integration with TensorFlow+Keras - Deployment to a variety of runtime environments (mobile devices, edge servers, and cloud platforms) |
[65] | 2019 |
PySyft (Python, Docker) |
Supporting a variety of techniques, such as differential privacy, secure multi-party computation, and homomorphic encryption. Available: https://openmined.github.io/PySyft/ |
- Extension to more privacy-preserving techniques - Support for multiple deployment environments - Security and private learning |
[66] | 2021 |
FLUTE (Python) |
Enabling rapid prototyping and simulation of new FL at scale with cloud integration and multi-GPU support, including novel optimization, privacy, and communications strategies. Available: https://github.com/microsoft/msrflute |
- A modular architecture for framework customization - A visualization tool for tracking the progress of FL - A possible integration with AzureML workspaces |
[67] | 2022 |
ns3-fl (C++, Python) |
Enhancement of the configuration of network settings and connecting to FL simulator, flsim, while implementation includes client-server ns3 and sync/async FL process communications. Available: https://github.com/eekaireb/ns3-fl |
- Emphasis of network settings - A power model of energy consuming while training clients - Enhancement of networking output reliability |
[68] | 2022 |
IBM FL (Python) |
Enabling distribution of machine learning in enterprise environments, prioritizing privacy, compliance, and data locality, and supporting various learning techniques and topologies. Available: https://ibmfl.res.ibm.com |
- Simplification of the adoption with infrastructure, coordination, and compatibility with common libraries and minimizes the learning curve - Deployment across various computing environments and designing custom fusion algorithms - A deep focus on the enterprise environment |
[69] | 2020 |
FFL-ERL (Erlang) |
Providing Erlang's suitability for FL, comparing its performance in two scenarios: a full Erlang implementation and a hybrid approach using C for numerical computations. Available: https://github.com/gregorulm/fcc_ffl_erl |
- Discussion of trade-off between C's high performance and Erlang's superior programmer productivity - Excellence in concurrency and distribution - Development and coordination capabilities |
[70] | 2018 |
OpenFL-XAI (Python, Docker) |
Emphasis privacy and explainability by focusing on the FL of Fuzzy Rule-Based Systems. Available: https://github.com/Unipisa/OpenFL-XAI |
- Intel's OpenFL framework - A solution for accurate, private, and interpretable AI applications - A trustworthy AI systems, privacy preservation, and transparency |
[71] | 2023 |
CrypTen (Python) |
Enabling privacy-preserving ML with secure multiparty computation with ML-centric features, a tensor library, and robust protocol implementations for real-world use. Available: https://crypten.ai |
abstractions for efficient and secure model evaluation - Simplification on secure ML for non-cryptography experts - Integration with PyTorch's familiar API |
[72] | 2021 |
FederatedScope (Python, Docker) |
Utilization of an event-based structure to grant users significant flexibility in autonomously defining the actions of distinct participants. Available: https://github.com/alibaba/FederatedScope |
- Support plug-in operations and components for enhanced privacy, attack simulation, and auto-tuning - Facilitation of the incorporation of diverse plug-in operations and components to enhance and streamline further development |
[73] | 2022 |
NVIDIA FLARE (Python) |
Providing an open-source SDK to ease building workflows with capabilities of scalable packaging, elastic, and lightweight. Available: https://github.com/NVIDIA/NVFlare |
- Multiple training and validation workflows - Federated analytics and lifecycle orchestration - Simplification on dashboard for management |
[74] | 2020 |
EasyFL (Python) | Targeting beginners with limited prior knowledge, while offering low-code platform, API design, and enhancing the deployment efficiency. Available: https://github.com/EasyFL-AI/EasyFL |
- Training outputs tracker - Simulation with statistical and system heterogeneity - Design on plug-in for training flow abstraction |
[75] | 2022 |
LEAF (Python) | Providing an adaptable benchmarking system designed for evaluating learning in federated settings with a collection of openly available federated datasets. Available: https://github.com/TalwalkarLab/leaf |
- Datasets: FEMNIST (Image classification), Sentiment140 (Sentiment analysis), Shakespeare (Next-character prediction), Synthetic (Classification), and Reddit (Next-word prediction) - Emphasis on learning in federated settings |
[76] | 2018 |
PaddleFL (Python, C++, Kubernetes) | Leveraging distributed training and Kubernetes-based job scheduling to offer scalable deployment, also easy replication. Available: https://github.com/PaddlePaddle/PaddleFL |
- Simplification of the deployment of FL systems on large-scale distributed clusters - A flexible framework with components for defining tasks, designing ML models, and handling distributed training configurations - Detail with run times on server, worker, and scheduler |
[77] | 2020 |
Paper | Performance matric | Aggregation approach | FL framework | Network simulation | Data acquisition | Ref. | Year |
L. Sami et al. | Throughput | FedAvg | Flower, FedScale, Flue | Unspecified | Google speech, OpenImage, Shakespeare | [78] | 2023 |
P. Tam et al. | Drop ratio, delivery ratio, delay, accuracy | FedAvg | TensorFlow Federated | Mininet, Ryu | MNIST and topology simulation | [79] | 2021 |
V. Balasubramanian et al. | Cache hit ratio, average delay | FedCo | PyTorch | Mininet | Simulation | [80] | 2021 |
R. Uddin et al. | Precision, recall, F1-score, accuracy | AWS OpenGrid | OpenMined | Mininet, Ryu | STIN SAT20, simulation (SDN) | [81] | 2023 |
V. Balasubramanian et al. | MNO revenue, cache hit ratio, average access latency, percentage error in placement | FedCo | Python | Mininet-Wifi | Simulation | [82] | 2021 |
Categorize | Methodology | Significance | Performance matric | Simulation/framework | Ref. | Year |
Learning performance | Edge client devices. | Accuracy and loss. | GNS3, OpenDayLight, NETCONF and RESTCONF/Flower, FedAvg | [83] | 2023 | |
TD-EPAD algorithm | Trustiness of training data in SD-IIoT. | Accuracy. | Mininet, Floodlight | [84] | 2023 | |
Quality of service | Supporting vector machine | Handling on allocating the network resource and controlling massive communication in backbone. | Delay, jitter, packet drop ratio, packet delivery ratio, throughput. | NS-3 | [87] | 2021 |
CUPE algorithm | Minimization of the content fetch delay for latency- sensitive of IoT. | Cach hit rate, content fetch delay. | Unspecified | [88] | 2023 | |
DDQN | Overcoming on the minimization of energy consumption, completion time, and round communication between local participant and node selection. | Packet drops ratio, throughput, overall accuracy, delay, and packet delivery ratio. | NS-3, Mininet, mini-nfv, OASIS TOSCA | [89] | 2022 | |
MultiFed, a multi-center FL framework | Acceleration for better convergence, heterogeneity handling, and improvement on scalability for privacy preservation in cloud-edge collaboration. | Total communication rounds, total communication delay, and total communication volume. | Unspecified | [90] | 2023 | |
Energy consumption | MIBLP, DDQN, FedRL algorithm | Minimization of the long-term delay and energy consumption of an IoT device. | Cost per user. | Unspecified | [92] | 2021 |
Cost | Fed-average algorithm | Approvement on client selection and optimizing model aggregation. | Averaging training accuracy, energy consumption of unit loss delay. | Unspecified | [95] | 2022 |
Acronym | Description |
API | Application programming interface |
CAPEX | Capital expenditure |
DDQN | Double deep Q-network |
DFQL | Deep federated Q-learning |
DL | Deep learning |
DNN | Deep neural network |
DRL | Deep reinforcement learning |
DSRA | Device selection and resource allocation |
DQL | Deep Q-learning |
EC | Edge cloud |
E2E | End-to-end |
FL | Federated learning |
HFL-VNE | Horizontal federated learning-virtual network embedding |
IID | Independent-and-identically-distributed |
IIoT | Industrial internet of things |
IoV | Internet of vehicles |
IoT | Internet of things |
MEC | Multi-access edge computing |
MFL | Multilevel federated learning |
ML | Machine learning |
MTFL | Multi-tentacle federated learning |
NFV | Network functions virtualization |
NFVeEC | NFV-enabled EC |
QoE | Quality of experience |
QoS | Quality of service |
RAN | Radio access network |
SDN | Software-defined networks |
VGAE | Variational graph autoencoder |
Survey paper | IoT networks | Overview of FL | Framework details | Key performance indicators | Simulation tools in networks | Ref. | Year |
W. Lim et al. | ✓ | ✓ | ✓ | ✘ | ✘ | [22] | 2020 |
D. Nguyen et al. | ✓ | ✓ | ✓ | ✘ | ✘ | [23] | 2021 |
R. Gupta et al. | ✘ | ✓ | ✓ | ✓ | ✘ | [24] | 2022 |
L. Witt et al. | ✘ | ✓ | ✓ | ✘ | ✘ | [25] | 2023 |
H. Chen et al. | ✘ | ✓ | ✓ | ✘ | ✘ | [26] | 2023 |
M. Al-Quraan et al. | ✘ | ✓ | ✓ | ✘ | ✘ | [27] | 2023 |
Summary of execution flows | Target objectives | Ref. | Year |
The proposed algorithm features two components: 1) An online learning component based on previous resource usage and training results for making fractional decisions, and 2) An internet-based rounding tool that transforms fractional choices into whole number selections. | Prioritization in cumulative utilization of computation and communication resources, while ensuring the convergence of both local and aggregated models. | [30] | 2020 |
The Power-of-Choice selection strategy works as follows: 1) The central server calculates the local losses of all clients, 2) The server sorts the clients in decreasing order of their local losses, and 3) The server selects the top set of clients to participate in the current training round. | Improvement of the convergence speed and accuracy of FL while reducing communication and computation overhead (target applications can be distributed machine learning (ML), mobile edge computing, and IoT). | [31] | 2022 |
"PyramidFL" is a fine-grained client selection method that first determines the utility-based client selection from the global view and then optimizes its utility profiling locally for further client selection. | Designing to speed up the FL training while achieving a higher final model performance, also prioritizing the use of clients with higher statistical and system utility. | [32] | 2020 |
1) Deadline-based aggregation: Aggregate client updates once a fixed deadline is met. 2) Joint optimization: two subproblems on client selection and parameter update. 3) E3CS: an exponential-weight algorithm for exploration and exploitation-based client selection. | Improvement of the training efficiency and final model accuracy in FL within a volatile training context, where clients are prone to failures. | [33] | 2022 |
"Newt" is an enhanced FL approach that includes a new client selection utility and fine-grained control on selection frequency. | Enabling efficient selection in intelligent transportation systems, which has challenges such as data and device heterogeneity and highly dynamic system. | [34] | 2022 |
Summary of execution flows | Target objectives | Ref. | Year |
"FL+HC" clusters clients are grouped based on how closely their local updates match the overall global model. After this grouping, the clusters are trained autonomously and simultaneously using dedicated models. | Improvement of the accuracy of the test set while minimizing the communication rounds needed to achieve convergence in FL, especially when dealing with non-independent and non-identically distributed (non-IID) data. | [35] | 2020 |
1) Bandwidth allocation: involves assigning additional bandwidth to devices that experience poorer channel conditions or possess less robust computational capabilities. 2) Device scheduling: suggests an approach that prioritizes selecting devices with the shortest model updating time until a favorable balance is struck between learning efficiency and round-trip latency. | Maximization of the convergence rate of FL training with respect to time rather than rounds, which can be achieved by minimizing the expected time for FL training to attain certain model accuracy. | [36] | 2020 |
Each client offloads partial data to the edge server for processing, and then, the clients update their local models using the remaining data (Meanwhile, the edge server updates the global model using the aggregated data). | Mitigation of the straggler effect in FL and improving the system efficiency (The method can be used in applications, such as mobile device training, IoT device training, or healthcare data training). | [37] | 2021 |
The optimal offloading strategy is derived by minimizing the FL loss function under the latency constraint and the Q-learning-based offloading strategy is proposed for the imperfect channel state information scenario. | Improvement of training efficiency and mitigating the straggler effect of FL in industrial IoT networks. | [38] | 2023 |
The greedy algorithm is proposed by iteratively assigning communication resources to the edge nodes that consume the least energy for local model training in each round, which proceeds until the communication resource budget is finished. | Training machine learning models for edge-assisted Internet of Agriculture Things applications, where data are both vertically and horizontally partitioned, and resources are limited. | [39] | 2022 |
Summary of execution flows | Target objectives | Ref. | Year |
1) Unbiased gradient aggregation: Evaluate the gradients against the global model parameters in the last local epoch, and 2) FedMeta: Perform meta updating on the global model parameters using a small set of data samples indicating the expected target distribution. | Improvement of the convergence speed and accuracy (Both unbiased gradient aggregation and FedMeta can be applied individually or together and can be integrated into existing FL). | [40] | 2020 |
1) Partition the network into groups and local model updates into segments, 2) Apply the aggregation protocol to segments with specific coordination between users, and 3) Aggregate the aggregated segments to obtain the global update. | Enabling secure model aggregation in FL systems, while allowing users to adjust the quantization proportional to their communication resources. | [41] | 2022 |
The method aggregates updates from scheduled devices using an age-aware weighting design, and the weights are assigned to each update based on the freshness of the data, with more recent updates receiving higher weights. | Development of an asynchronous FL framework with periodic aggregation that can support heterogeneous computation capabilities and training data distributions. | [42] | 2021 |
The method uses a feedback mechanism to communicate the estimated global update to each client, which then calculates the relevance of its local update to the estimated global update (If the relevance is lower than a predefined threshold, the client does not update). | Mitigation of communication overhead, which can be applied to a variety of FL applications, such as personalized healthcare, intelligent transportation systems, and distributed machine learning for financial services. | [43] | 2019 |
Participants apply linear transformation to the model update vector, then partially encrypt the transformed vector using multi-input functional encryption. | Design of an efficient secure aggregation scheme, which can protect the security of model updates without losing efficiency. | [44] | 2020 |
Platform | Summary | Primary focuses | Ref. | Year |
NS3 (C++, Python) | A simulator can provide an approach to enhance the comprehensive and flexible platform for computer network simulation. It also provides the ability to analyze and simulate network behaviors and several network standardization protocols, algorithms, scenarios, and topologies. Available: https://www.nsnam.org/ | - Customization and extension of its functionality - Supporting comprehensive network environment and network protocols - Execution of the simulation using an XML trace file - Support with OpenAI Gym |
[47,48,49] | 2008 |
NS2 (C++) | Designing to illustrate the behaviors of computer networks, which include wired and wireless communication, routing, and congest control protocol and transport (e.g., routing algorithm). Available: https://www.isi.edu/nsnam/ns/ |
- Routing - Switching - Extending to support IPv6 - Extending to support cloud computing |
[50] | 1997 |
Mininet (Python) | Open-source network emulator that provides to create, configure, and test network topologies, which supports large-scale network infrastructure and the integration with SDN controller using OpenFlow (simplifying the configuration on node, flow entry, and link). Available: http://mininet.org/ |
- Creation and design for a real-world SDN environment - Interconnecting API and AI - Exportation of network topology into Python script - Being mini-edit to visualize the topology |
[51] | 2010 |
Mininet-WiFi (Python) | Designing to evolve rapidly and develop to emulate wireless network controller environment that supports several wireless protocols such as Wi-Fi, ZigBee, and Bluetooth. Available: https://mininet-wifi.github.io |
- Development of a new scenario of the network wireless - Measurement of the performance of different Wi-Fi channels - Routing protocol for wireless connection mesh network - Providing a high degree of fidelity - Focusing on the security and scalability of mobile network |
[52] | 2021 |
MATLAB (Commercial) | Enabling cooperation with other programming languages and numerical computing environment, which provides more functions for modeling and simulating communication systems, wireless networks, cellular networks, and optical networks (enclosing several tools and libraries). Available: https://www.mathworks.com/help/index.html?s_tid=CRUX_lftnav |
- Simulation for network systems and protocols - Simulation for complex networks - Offering customization and development - Specification network function - areas or behaviors |
[53] | undefined |
OMNet++ (C++) | Designing a component architecture for models and providing a high degree of scaling to support an extensive network with millions of nodes, which is feasible to obtain a highly accurate result. Available: https://omnetpp.org/download/models-and-tools |
- Support model completeness - Simulation from LANs to WANs - Packet-switched and circuit-switched networks - Support for sensor networks and ad-hoc networks |
[54] | 1993 |
OpenDayLight (Java, Python, YAML) | Providing an open platform to focus on customizing and automating networks of network environment, which makes familiar with SDN controller scales up of the network device and network applications. Available: https://www.opendaylight.org |
- Combination of multiple service and protocol - Sustenance of various southbound APIs with network devices and protocol - Feasibly support of a wide range of standard networking protocols - Adaptation of NFV and SDN |
[55] | 2013 |
Floodlight (Java) | Offering greater flexibility, agility, and programmability in network management. Floodlight makes a rule of network policy and routing logic. Available: https://github.com/floodlight/floodlight |
- Support for multiple network protocols - Solution for flexible and scalable approaches |
[56] | 2014 |
Ryu-Controller (Python) | Supporting several protocols for managing network devices, such as OpenFlow, Netconf, and OF-config. Available: https://ryusdn.org/index.html |
- Creation flow table management - Traffic monitoring - Packet forwarding |
[57] | 2014 |
OpenStack | Open source offers a core network controller to accommodate network functions virtualization (NFV), it maintains the feature as a platform for network automation, orchestration, and management resources. Available: https://www.openstack.org |
- Network protocols and technologies - A private cloud platform - Enhancement of resources utilization and elastic hypervisors - RESTful API - Tools achieved with automation and integration |
[58] | 2010 |
Platform | Summary | Primary Focuses | Ref. | Year |
Flower (Python) |
Providing a unified approach to FL, federated evaluation, and federated analytics, which allows users to jointly federate different workloads, ML frameworks, and programming languages. Available: https://flower.dev |
- Offering large-scale experiments - Emphasis of heterogeneous participants - Transition to real-world devices - Integration of multiple ML training frameworks - Customizability and extensibility |
[62] | 2020 |
FedML (Python) |
Prioritization lightweight, cross-platform, and secure FL and federated analytics. Available: https://www.fedml.ai |
- Convergence of MLOps tools with decentralized learning - Vertical approaches to industries and applications - Scalability for data silos and rapid large model training |
[63] | 2020 |
FATE (Python) |
Designing to be industrial-grade with features such as scalability, security, and compliance (supports logistic regression, tree-based algorithms, deep learning, transfer learning, etc.). Available: https://fate.fedai.org |
- A wide range of secure computation protocols - FATE CLI, SDK, and dashboard - Design with distributed architecture, message-passing interface, and fault tolerance |
[64] | 2019 |
TFF (Python) |
Providing a flexible programming model for FL, as well as a variety of tools and libraries to support the development and deployment of FL applications. Available: https://www.tensorflow.org/federated |
- High-level interfaces (FL and FC API) including datasets, models, computation builders, client placement type, etc. - Integration with TensorFlow+Keras - Deployment to a variety of runtime environments (mobile devices, edge servers, and cloud platforms) |
[65] | 2019 |
PySyft (Python, Docker) |
Supporting a variety of techniques, such as differential privacy, secure multi-party computation, and homomorphic encryption. Available: https://openmined.github.io/PySyft/ |
- Extension to more privacy-preserving techniques - Support for multiple deployment environments - Security and private learning |
[66] | 2021 |
FLUTE (Python) |
Enabling rapid prototyping and simulation of new FL at scale with cloud integration and multi-GPU support, including novel optimization, privacy, and communications strategies. Available: https://github.com/microsoft/msrflute |
- A modular architecture for framework customization - A visualization tool for tracking the progress of FL - A possible integration with AzureML workspaces |
[67] | 2022 |
ns3-fl (C++, Python) |
Enhancement of the configuration of network settings and connecting to FL simulator, flsim, while implementation includes client-server ns3 and sync/async FL process communications. Available: https://github.com/eekaireb/ns3-fl |
- Emphasis of network settings - A power model of energy consuming while training clients - Enhancement of networking output reliability |
[68] | 2022 |
IBM FL (Python) |
Enabling distribution of machine learning in enterprise environments, prioritizing privacy, compliance, and data locality, and supporting various learning techniques and topologies. Available: https://ibmfl.res.ibm.com |
- Simplification of the adoption with infrastructure, coordination, and compatibility with common libraries and minimizes the learning curve - Deployment across various computing environments and designing custom fusion algorithms - A deep focus on the enterprise environment |
[69] | 2020 |
FFL-ERL (Erlang) |
Providing Erlang's suitability for FL, comparing its performance in two scenarios: a full Erlang implementation and a hybrid approach using C for numerical computations. Available: https://github.com/gregorulm/fcc_ffl_erl |
- Discussion of trade-off between C's high performance and Erlang's superior programmer productivity - Excellence in concurrency and distribution - Development and coordination capabilities |
[70] | 2018 |
OpenFL-XAI (Python, Docker) |
Emphasis privacy and explainability by focusing on the FL of Fuzzy Rule-Based Systems. Available: https://github.com/Unipisa/OpenFL-XAI |
- Intel's OpenFL framework - A solution for accurate, private, and interpretable AI applications - A trustworthy AI systems, privacy preservation, and transparency |
[71] | 2023 |
CrypTen (Python) |
Enabling privacy-preserving ML with secure multiparty computation with ML-centric features, a tensor library, and robust protocol implementations for real-world use. Available: https://crypten.ai |
abstractions for efficient and secure model evaluation - Simplification on secure ML for non-cryptography experts - Integration with PyTorch's familiar API |
[72] | 2021 |
FederatedScope (Python, Docker) |
Utilization of an event-based structure to grant users significant flexibility in autonomously defining the actions of distinct participants. Available: https://github.com/alibaba/FederatedScope |
- Support plug-in operations and components for enhanced privacy, attack simulation, and auto-tuning - Facilitation of the incorporation of diverse plug-in operations and components to enhance and streamline further development |
[73] | 2022 |
NVIDIA FLARE (Python) |
Providing an open-source SDK to ease building workflows with capabilities of scalable packaging, elastic, and lightweight. Available: https://github.com/NVIDIA/NVFlare |
- Multiple training and validation workflows - Federated analytics and lifecycle orchestration - Simplification on dashboard for management |
[74] | 2020 |
EasyFL (Python) | Targeting beginners with limited prior knowledge, while offering low-code platform, API design, and enhancing the deployment efficiency. Available: https://github.com/EasyFL-AI/EasyFL |
- Training outputs tracker - Simulation with statistical and system heterogeneity - Design on plug-in for training flow abstraction |
[75] | 2022 |
LEAF (Python) | Providing an adaptable benchmarking system designed for evaluating learning in federated settings with a collection of openly available federated datasets. Available: https://github.com/TalwalkarLab/leaf |
- Datasets: FEMNIST (Image classification), Sentiment140 (Sentiment analysis), Shakespeare (Next-character prediction), Synthetic (Classification), and Reddit (Next-word prediction) - Emphasis on learning in federated settings |
[76] | 2018 |
PaddleFL (Python, C++, Kubernetes) | Leveraging distributed training and Kubernetes-based job scheduling to offer scalable deployment, also easy replication. Available: https://github.com/PaddlePaddle/PaddleFL |
- Simplification of the deployment of FL systems on large-scale distributed clusters - A flexible framework with components for defining tasks, designing ML models, and handling distributed training configurations - Detail with run times on server, worker, and scheduler |
[77] | 2020 |
Paper | Performance matric | Aggregation approach | FL framework | Network simulation | Data acquisition | Ref. | Year |
L. Sami et al. | Throughput | FedAvg | Flower, FedScale, Flue | Unspecified | Google speech, OpenImage, Shakespeare | [78] | 2023 |
P. Tam et al. | Drop ratio, delivery ratio, delay, accuracy | FedAvg | TensorFlow Federated | Mininet, Ryu | MNIST and topology simulation | [79] | 2021 |
V. Balasubramanian et al. | Cache hit ratio, average delay | FedCo | PyTorch | Mininet | Simulation | [80] | 2021 |
R. Uddin et al. | Precision, recall, F1-score, accuracy | AWS OpenGrid | OpenMined | Mininet, Ryu | STIN SAT20, simulation (SDN) | [81] | 2023 |
V. Balasubramanian et al. | MNO revenue, cache hit ratio, average access latency, percentage error in placement | FedCo | Python | Mininet-Wifi | Simulation | [82] | 2021 |
Categorize | Methodology | Significance | Performance matric | Simulation/framework | Ref. | Year |
Learning performance | Edge client devices. | Accuracy and loss. | GNS3, OpenDayLight, NETCONF and RESTCONF/Flower, FedAvg | [83] | 2023 | |
TD-EPAD algorithm | Trustiness of training data in SD-IIoT. | Accuracy. | Mininet, Floodlight | [84] | 2023 | |
Quality of service | Supporting vector machine | Handling on allocating the network resource and controlling massive communication in backbone. | Delay, jitter, packet drop ratio, packet delivery ratio, throughput. | NS-3 | [87] | 2021 |
CUPE algorithm | Minimization of the content fetch delay for latency- sensitive of IoT. | Cach hit rate, content fetch delay. | Unspecified | [88] | 2023 | |
DDQN | Overcoming on the minimization of energy consumption, completion time, and round communication between local participant and node selection. | Packet drops ratio, throughput, overall accuracy, delay, and packet delivery ratio. | NS-3, Mininet, mini-nfv, OASIS TOSCA | [89] | 2022 | |
MultiFed, a multi-center FL framework | Acceleration for better convergence, heterogeneity handling, and improvement on scalability for privacy preservation in cloud-edge collaboration. | Total communication rounds, total communication delay, and total communication volume. | Unspecified | [90] | 2023 | |
Energy consumption | MIBLP, DDQN, FedRL algorithm | Minimization of the long-term delay and energy consumption of an IoT device. | Cost per user. | Unspecified | [92] | 2021 |
Cost | Fed-average algorithm | Approvement on client selection and optimizing model aggregation. | Averaging training accuracy, energy consumption of unit loss delay. | Unspecified | [95] | 2022 |