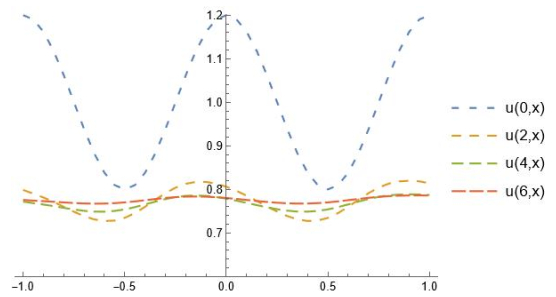
The projected increase of civil aviation activity, the degradation of air quality and the location of Beirut Airport embedded in a very urbanized area, in addition to the special geography and topography surrounding the airport which plays a significant role in drawing emissions to larger distances, demanded anassessment of the spatial impact of the airport activities on the air quality of Beirut and its suburbs. This is the first study in the Middle East region that model pollutant concentrations resulting from an international airport's activities using an advanced atmospheric dispersion modelling system in a country with no data. This followed validation campaigns showing very strong correlations (r = 0.85) at validation sites as close as possible to emission sources. The modelling results showed extremely high NO2 concentrations within the airport vicinity, i.e., up to 110 μg∙m-3 (which is greater than the World Health Organization annual guidelines) posing a health hazard to the workers in the ramp. The major contribution of Beirut–Rafic Hariri International Airport to the degradation of air quality was in the airport vicinity; however, it extended to Beirut and its suburbs in addition to affecting the seashore area due to emissions along the aircraft trajectory; this isan aspect rarely considered in previous studies. On the other hand, elevated volatile organic compound levels were observed near the fuel tanks and at the aerodrome center. This study provides (ⅰ) a methodology to assess pollutant concentrations resulting from airport emissions through the use of an advanced dispersion model in a country with no data; and (ⅱ) a tool for policy makers to better understand the contribution of the airport's operations to national pollutant emissions, which is vital for mitigation strategies and health impact assessments.
Citation: Tharwat Mokalled, Stéphane Le Calvé, Nada Badaro-Saliba, Maher Abboud, Rita Zaarour, Wehbeh Farah, Jocelyne Adjizian-Gérard. Atmospheric dispersion modelling of gaseous emissions from Beirutinternational airport activities[J]. AIMS Environmental Science, 2022, 9(5): 553-572. doi: 10.3934/environsci.2022033
[1] | Wenjing An, Xingdong Zhang . An implicit fully discrete compact finite difference scheme for time fractional diffusion-wave equation. Electronic Research Archive, 2024, 32(1): 354-369. doi: 10.3934/era.2024017 |
[2] | Yunxia Niu, Chaoran Qi, Yao Zhang, Wahidullah Niazi . Numerical analysis and simulation of the compact difference scheme for the pseudo-parabolic Burgers' equation. Electronic Research Archive, 2025, 33(3): 1763-1791. doi: 10.3934/era.2025080 |
[3] | Shasha Bian, Yitong Pei, Boling Guo . Numerical simulation of a generalized nonlinear derivative Schrödinger equation. Electronic Research Archive, 2022, 30(8): 3130-3152. doi: 10.3934/era.2022159 |
[4] | Leilei Wei, Xiaojing Wei, Bo Tang . Numerical analysis of variable-order fractional KdV-Burgers-Kuramoto equation. Electronic Research Archive, 2022, 30(4): 1263-1281. doi: 10.3934/era.2022066 |
[5] | Chang Hou, Hu Chen . Stability and pointwise-in-time convergence analysis of a finite difference scheme for a 2D nonlinear multi-term subdiffusion equation. Electronic Research Archive, 2025, 33(3): 1476-1489. doi: 10.3934/era.2025069 |
[6] | Li Tian, Ziqiang Wang, Junying Cao . A high-order numerical scheme for right Caputo fractional differential equations with uniform accuracy. Electronic Research Archive, 2022, 30(10): 3825-3854. doi: 10.3934/era.2022195 |
[7] | Jun Liu, Yue Liu, Xiaoge Yu, Xiao Ye . An efficient numerical method based on QSC for multi-term variable-order time fractional mobile-immobile diffusion equation with Neumann boundary condition. Electronic Research Archive, 2025, 33(2): 642-666. doi: 10.3934/era.2025030 |
[8] | Yao Yu, Guanyu Xue . A nonlinear correction finite volume scheme preserving maximum principle for diffusion equations with anisotropic and discontinuous coefficient. Electronic Research Archive, 2025, 33(3): 1589-1609. doi: 10.3934/era.2025075 |
[9] | Junseok Kim . Maximum principle preserving the unconditionally stable method for the Allen–Cahn equation with a high-order potential. Electronic Research Archive, 2025, 33(1): 433-446. doi: 10.3934/era.2025021 |
[10] | Mingfa Fei, Wenhao Li, Yulian Yi . Numerical analysis of a fourth-order linearized difference method for nonlinear time-space fractional Ginzburg-Landau equation. Electronic Research Archive, 2022, 30(10): 3635-3659. doi: 10.3934/era.2022186 |
The projected increase of civil aviation activity, the degradation of air quality and the location of Beirut Airport embedded in a very urbanized area, in addition to the special geography and topography surrounding the airport which plays a significant role in drawing emissions to larger distances, demanded anassessment of the spatial impact of the airport activities on the air quality of Beirut and its suburbs. This is the first study in the Middle East region that model pollutant concentrations resulting from an international airport's activities using an advanced atmospheric dispersion modelling system in a country with no data. This followed validation campaigns showing very strong correlations (r = 0.85) at validation sites as close as possible to emission sources. The modelling results showed extremely high NO2 concentrations within the airport vicinity, i.e., up to 110 μg∙m-3 (which is greater than the World Health Organization annual guidelines) posing a health hazard to the workers in the ramp. The major contribution of Beirut–Rafic Hariri International Airport to the degradation of air quality was in the airport vicinity; however, it extended to Beirut and its suburbs in addition to affecting the seashore area due to emissions along the aircraft trajectory; this isan aspect rarely considered in previous studies. On the other hand, elevated volatile organic compound levels were observed near the fuel tanks and at the aerodrome center. This study provides (ⅰ) a methodology to assess pollutant concentrations resulting from airport emissions through the use of an advanced dispersion model in a country with no data; and (ⅱ) a tool for policy makers to better understand the contribution of the airport's operations to national pollutant emissions, which is vital for mitigation strategies and health impact assessments.
In mathematical biology, predicting the time evolution of a biomass or a population over a spatial domain is a very important problem. Often, Lotka-Voterra-type equations are used to describe population dynamics, whether studying competition or predator-prey models. Such systems have been studied in many settings. Conditions under which two species can coexist have been treated theoretically. Numerical models have been developed that hope to mimic behavior of the system represented in the models. In the case that dispersion is occurring to each species, biomass or population studies over a domain ΩT=(0,T)×Ω for some Ω⊂Rd, generally with d=1,2, or 3 may take the general form
{ut=a1Δu+b1Lu+f1(u,v) in ΩTvt=a2Δv+b2Lv+f2(u,v) in ΩTu(0,x)=u0(x), v(0,x)=v0(x) in Ω | (1.1) |
where L is a linear operator on Ω, distinct from the Laplacian, that can take various forms. If a1,a2>0 in system (1.1), then some degree of local diffusion of each species is modeled by the equations. The b1=b2=0 case has been considered by multiple authors, such as in [1,2,3,4] and in their accompanying references.
In real world settings, populations in competition may demonstrate diffusion, dispersion, or some degree of both local and nonlocal behaviors. Competition for common resources may not only follow in their immediate neighborhood, but also in the entire spatial domain. In addition, this competition is not necessarily occurring only between individuals at the same location, but also between individuals at different locations; see [5] and references therein for an excellent motivation for and summary of models of nonlocal dispersion operators. Following similar reasoning, when b1=b2=1, the operator L given by
Lu(t,x)=∫ΩJ(x−y)u(t,y)dy−∫ΩJ(x−y) dy⋅u(t,x) | (1.2) |
has been motivated as an accurate reflection of dispersion between species for suitable J, and will be used here. The authors in [6] show the derivation of the nonlinear dispersion operator as J∗u−(J∗1)u, but then point out that it is unrealistic to use a convolution term to model biological species in bounded domains. Further, the restriction L in (1.2) of J∗u−(J∗1)u arises naturally in cases of hostile surroundings, a periodic environment, or under reflected boundary conditions. In [7], these assumptions are shown to be unnecessary, where the dispersion operator L in (1.2) is used to model nonlocal interaction. There, the authors show that for L, total internal energy is conserved and free energy decreases along trajectories so that L a suitable choice to reflect dispersion as a replacement for local dispersion modeled by the Laplacian for a symmetric interaction kernel J. Thus, operator L is also used both in nonlocal Allen-Cahn-type equations (c.f. [8]) or when describing population changes with nonlocal dispersion in biological systems (as in [9] and references therein).
Indeed, when restricted to ΩT⊂(0,T)×Rd, kernel function J in (1.2) becomes a measure of the probability that population members at all positions x affect those at y∈Rd, and vice-versa. Hence,
−ut(t,x)=−Lu(t,x) |
may be used to describe the rate at which members of a species are leaving x∈Ω at time t to travel to all other sites y∈Rd. Thus, for b1=b2=1, various forms of system (1.1) using L in (1.2) have also been studied in depth, including traveling wave solutions, spreading speed, stability of traveling waves, and of entire solutions as in [10,11,12], and references therein.
There has been a good deal of work on Lotka-Volterra models that include local diffusion, and more recently, nonlocal dispersion has been treated in such systems (see [1,2,3,4,13,14], along with references therein). In [15], an implicit approach to the numerical analysis of the system is introduced that mimics the dynamical properties of the true solution. In addition, it is proven that the scheme introduced there is uniquely solvable and unconditionally stable. The asymptotic behavior of the difference scheme is studied by constructing upper and lower solutions for the difference scheme. The convergence rate of the numerical solution to the true solution of the system is also given.
Following notation in (1.1) and (1.2) is
{ut=Lu+u(K1−u−av) in ΩTvt=Lu+v(K2−v−bu) in ΩTu(0,x)=u0(x), v(0,x)=v0(x) in Ω | (1.3) |
where Ω, ΩT, and L are defined previously. Here, u(t,x)≥0 and v(t,x)≥0 denote the population densities of the competing species for time t≥0 and x∈Ω⊂Rd for d=1,2. In addition, we assume that the system whose solutions describe the density of each species that began with (1.1) has been nondimensionalized, so that in (1.3), a,b,K1,K2>0 depend on the initial choice of constants in (1.1). To our knowledge, this system has not been analyzed with an unconditionally stable, nonstandard numerical method whose convergence rate can be given. This is the goal of this contribution.
As described previously, the system is used to model the two species competing with each other for the same prey, where both species are continuously distributed in time t throughout a region Ω, with each exhibiting free movement in the form of nonlocal dispersion. The method introduced to discretize (1.3) and the study of its properties will follow a similar development to the one used in [16], where properties of a single integro-differential equation that models an Ising spin system, with a convolution term that involves the Kac potential, are discussed in detail (see [17,18,19,20]).
In Section 2, we introduce the difference scheme used for the approximation of (1.3) over ΩT⊂(0,T)×R. We prove existence of the numerical solution to the scheme and that this solution is stable, independent of the choice of Δt and Δx. We give the convergence rate of the numerical scheme to the true solution. In Section 3, we present some results of numerical experiments that confirm the stability and convergence of the proposed difference scheme in one- and two-dimensional spatial domains Ω. In Section 4, we provide a summary of the results.
Analysis will be carried out over a domain Ω in one-dimensional space. All results carry over naturally to higher-dimensional space. For t>0 we introduce time step tk=kΔt for k=0,1,2,…, where Δt is of fixed size to be determined later. On the interval Ω=(−L,L)⊂R we define the partition
Ωx={xi|xi=−L+i△x,i=1,2,…,N−1}, where Δx=2L/N. |
Using uki and vki to represent the numerical approximation to the true solutions u and v to (1.3) at (tk,xi), our choice of difference scheme for n=1 in (1.3) is nonstandard to invoke desirable properties that will be established later, namely
{uk+1i−uki△t=(J∗uk)i−(J∗1)iuk+1i+K1uki−ukiuk+1i−auk+1ivkivk+1i−vki△t=(J∗vk)i−(J∗1)ivk+1i+K2vki−vkivk+1i−bukivk+1i | (2.1) |
for k=0,1,2,… and for 0≤i≤N. Throughout, for convenience, the discretization of Lu as given in (1.2) for (2.1) will be denoted by
(J∗uk)i=Δx[12J(x0−xi)uk0+N−1∑m=1J(xm−xi)ukm+12J(xN−xi)ukN]; |
a similar expression is used for Lv. We also introduce the initial conditions in (1.3) as
u0i=u0(xi) and v0i=v0(xi) |
for i=0,1,2,…,N, where for all i, u0(xi),v0(xi)≥0.
Solving (2.1) for uk+1i and vk+1i gives the iteration scheme for n=1 as
{uk+1i=(J∗uk)iΔt+(1+K1Δt)uki1+(J∗1)iΔt+ukiΔt+avkiΔtvk+1i=(J∗vk)iΔt+(1+K2Δt)vki1+(J∗1)iΔt+vkiΔt+bukiΔt | (2.2) |
for k=0,1,2,… and i=0,1,2,…,N.
Although we present and prove theorems for n=1, it is useful for programming numerical models to show the method of approximating (1.3) for n=2 as well. In this case, we choose Ω=(−L,L)×(−W,W)⊂R2, and partitions
Ωxy={(xi,yj)|xi=−L+i△x,yj=−W+j△y,0≤i≤M,0≤j≤N} |
and
Ωt={tk|tk=k△t,0≤t≤K}, |
where △x=2L/M and △y=2W/N.
The difference scheme for (1.3) includes
u0i,j=u0(xi,yj) and v0i,j=v0(xi,yj), | (2.3) |
together with
{uk+1i,j−uki,j△t=(J∗uk)i,j−(J∗1)i,juk+1i,j+K1uki,j−uki,juk+1i,j−auk+1i,jvki,jvk+1i,j−vki,j△t=(J∗vk)i,j−(J∗1)i,jvk+1i,j+K2vki,j−vki,jvk+1i,j−bvk+1i,juki,j | (2.4) |
all for i=0,1,…,M and j=0,1,…,N, where in (2.4),
(J∗uk)i,j=△x△y[M−1∑m=1N−1∑n=1J(xm−xi,yn−yj)ukm,n+12M−1∑m=1(J(xm−xi,y0−yj)ukm,0+J(xm−xi,yN−yj)ukm,N)+12N−1∑n=1(J(x0−xi,yn−yj)uk0,n+J(xM−xi,yn−yj)ukM,n)+14(J(x0−xi,y0−yj)uk0,0+J(xM−xi,y0−yj)ukM,0+J(x0−xi,yN−yj)uk0,N+J(xM−xi,yN−yj)ukM,N)]. |
From (2.3) and (2.4), we arrive at the explicit finite difference scheme
{[1+(uki,j+avki,j+(J∗1)i,j)Δt]uk+1i,j=[(J∗uk)i,j+K1uki,j]Δt+uki,j[1+(vki,j+buki,j+(J∗1)i,j)Δt]vk+1i,j=[(J∗vk)i,j+K2vki,j]Δt+vki,j | (2.5) |
for i=0,1,…,M, j=0,1,…,N, and k=0,1,2,….
Theorem 2.1. For n=1 and for the initial conditions in (1.3), let m1=maxu0(x), m2=maxv0(x), M1=max{K1,m1}, and M2=max{K2,m2}. Then for all k=0,1,2,… and for i=0,1,2,…,N,
0≤uki≤M1and0≤vki≤M2. | (2.6) |
Hence, the numerical scheme (2.2) is unconditionally nonnegative and unconditionally stable.
Proof. We proceed by induction. For k=0,
0≤u0i≤m1≤M1 and 0≤v0i≤m2≤M2 |
for i=0,1,2,…,N, so that (2.6) holds.
Assume now that (2.6) holds for some k∈N. Then for k+1,
uk+1i=(J∗uk)iΔt+(1+K1Δt)uki1+(J∗1)iΔt+ukiΔt+avkiΔt≤(J∗1)iM1Δt+(uki+K1ukiΔt)1+(J∗1)iΔt+ukiΔt+avkiΔt≤(J∗1)iM1Δt+(M1+M1ukiΔt)1+(J∗1)iΔt+ukiΔt+avkiΔt≤M1[(J∗1)iΔt+1+ukiΔt]1+(J∗1)iΔt+ukiΔt+avkiΔt≤ M1. |
Similarly, vk+1i≤M2, so the result holds for k+1 if it holds for k. Therefore, by mathematical induction, (2.6) is valid for all k=0,1,2… and for i=0,1,2,…,N.
We now turn to the question of convergence of the difference equations (2.2) to the true solution of (1.3).
Theorem 2.2. If u,v∈C1,2([0,T]ׯΩ) are solutions to (1.3), then the solution of (2.2) converges to u and v as Δt,Δx→0, uniformly on [0,T], with rate O(Δt+Δx2).
Proof. Let (u(t,x),v(t,x)) represent the solution pair to (1.3), where u,v∈C1,2([0,T]ׯΩ). Set
U0i=u0(xi), V0i=v0(xi),Uki=u(tk,xi), and Vki=v(tk,xi). |
We will prove the convergence claim based on u, then the same will follow for v by the symmetry of equations in (1.3). Let Δt=T/K, so that tk=kΔt for k=0,1,2,…,K. From (1.3) and (2.1) we have
Uk+1i−UkiΔt=(J∗Uk)i−(J∗1)iUki+K1Uki−(Uik)2−aUkiVki+Ru(Δt,Δx), | (2.7) |
where Ru is a function with Ru(Δt,Δx)=O(Δt+Δx2). Let
Xki=Uki−uki, Yki=Vki−vki |
for k=0,1,2,…,K and i=0,1,2,…,N. Then X0i=0, Y0i=0, for i=0,1,2,…,N. Using (2.1) in conjunction with (2.7),
Xk+1i=Uki−uki+Δt[(J∗Uk)i−(J∗uk)i−(J∗1)i(Uki−uki)−(J∗1)i(uk+1i−uki)]+Δt[K1(Uki−uki)−((Uki)2−ukiuk+1i)−a(UkiVki−uk+1ivki)]+ΔtRu(Δt,Δx), | (2.8) |
so that from (2.8),
|Xk+1i|≤|Uki−uki|+Δt|(J∗Uk)i−(J∗uk)i|+Δt|(J∗1)i(Uki−uki)|+Δt|(J∗1)i(uk+1i−uki)|+K1Δt|Uki−uki|+Δt|(Uki)2−ukiuk+1i|+aΔt|UkiVki−uk+1ivki|+ΔtRu(Δt,Δx). | (2.9) |
We turn to upper bounds on each of the terms in (2.9). To accomplish this, for each k, k=0,1,2,…,K, we will use Wku=maxi|Uki−uki| and Wkv=maxi|Vki−vki|. Setting C1=maxi(J∗1)i,
|(J∗Uk)i−(J∗uk)i)|≤Δx2J(x0−xi)|Uk0−uk0|+ΔxN−1∑m=1J(xm−xi)|Ukm−ukm|+Δx2J(xN−xi)|UkN−ukN|≤Δx[12J(x0−xi)+N−1∑m=1J(xm−xi)+12J(xN−xi)]Wku≤C1Wku, | (2.10) |
|(J∗1)i(Uki−uki)|=(J∗1)i|Uki−uki|≤C1Wku, |
and
|(J∗1)i(uk+1i−uki)|≤C1|uk+1i−uki|. |
Now, since |uki| and |vki| are uniformly bounded by M1 and M2, from (2.1),
|uk+1i−uki|≤C(M1,M2,C1)△t, |
where C(M1,M2,C1) is a constant that depends only on M1, M2, and C1. Hence there exists C2 such that
|(J∗1)i(uk+1i−uki)|≤C1|uk+1i−uki|≤C1C(M1,M2,C1)△t=C2△t |
and
K1|Uki−uki|≤K1Wku, |
where K1 is that constant related to carrying capacity in (1.3), so that for some constants C3, C4, and C5,
|(Uki)2−ukiuk+1i|=|UkiUki−Ukiuki+Ukiuki−uk+1iUki+uk+1iUki−ukiuk+1i|≤|UkiUki−Ukiuki|+|Ukiuki−uk+1iUki|+|uk+1iUki−ukiuk+1i|≤|Uki||Uki−uki|+|Uki||uki−uk+1i|+|uk+1i||Uki−uki|≤C3Wku+C3C(M1,M2,C1)△t+C(M1,M2,C1)Wku= C4△t+C5Wku, |
and thus there exist constants C6, C7, and C8 with
a|UkiVki−uk+1ivki|= a|UkiVki−Vkiuki+Vkiuki−uk+1iVki+uk+1iVki−uk+1ivki|≤ a(|Vki||Uki−uki|+|Vki||uki−uk+1i|+|uk+1i||Vki−vki|)≤ C6Wku+C7△t+C8Wkv. | (2.11) |
Substituting (2.10) and (2.11) into (2.9), we obtain
|Xk+1i|≤(1+C8△t)Wku+C9△tWkv+ΔtRu(Δt,Δx) | (2.12) |
for i=0,1,…,N, where C8 and C9 are constants independent of i and k, and where △t2-terms are absorbed into ΔtRu(Δt,Δx). Therefore, for each k, k=0,1,2,…,K,
Wk+1u≤(1+C8△t)Wku+C9△tWkv+ΔtRu(Δt,Δx). | (2.13) |
Similarly, there exist C10 and C11 with
Wk+1v≤(1+C10△t)Wkv+C11△tWku+ΔtRv(Δt,Δx), | (2.14) |
where, as with Ru, Rv is a function with Rv(Δt,Δx)=O(Δt+Δx2). Now setting Zk=Wku+Wkv, from (2.13) and (2.14), there exists a constant C0 with
Zk+1≤(1+C0△t)Zk+ΔtRu(Δt,Δx) | (2.15) |
for k=0,1,2,…,K. Set
D=1+C0Δt≥1. | (2.16) |
Then since Z0=0, using (2.15) and (2.16) and iterating,
Zk+1≤Dk+1Z0+[1+D+D2+…+Dk]ΔtRu(Δt,Δx)≤Dk+1−1C0△tΔtRu(Δt,Δx) |
for k=0,1,2,…,K−1. Since ex≥1+x, it follows that eKx≥(1+x)K, so that for all k, k=0,1,2,…,K−1, and again using D from (2.16),
Dk+1−1≤DK−1≤eC0K△t−1=eC0T−1. |
Thus, for k=0,1,2,…,K−1,
Zk+1≤(eC0T−1)Ru(Δt,Δx), |
so that Zk→0 for k=0,1,2,…,K as Δt→0, Δx→0. This completes the proof.
Remark. Similar results hold for n=2 in Theorems 2.1 and 2.2, and their proofs.
In this section we finish by presenting some results of computational experiments that verify the stability and convergence of the proposed difference scheme, confirming that the numerical solutions preserve the properties of the theoretical solution as well as those guaranteed by Theorem 2.2. Since there is no exact solution to compare with the approximation generated by the difference scheme, we use fix △x and compute for various △t values, then vice-versa. We compare the results in tables. We also present graphical results for dimensions n=1 in (2.2) and n=2 in (2.5).
CASEI. For n=1, we test method (2.1) for Ω=(−1,1), ϵ=0.1, a=0.4, b=0.6, K1=K2=1, u0(x)=0.2cos(2πx)+1, and v0(x)=0.3sin(2πx)+1, where J(x)=(ϵ√π)−1exp(−x2/ϵ2). We call these approximations (u(t,x),v(t,x)). Their convergence to steady state solutions is demonstrated in Figures 1 and 2 for Δt=Δx=0.05, as an example, since convergence is independent of time and space steps and graphs look much the same for any reasonable choices of small Δt and Δx.
CASEIA: Hold △x=0.05. Let (u(t,x),v(t,x)) denote the numerical solution under the parameters as chosen above corresponding to △t, while (u1(t,x),v1(t,x)) corresponds to △t1. Table 1 shows the maximum absolute errors, max|u(t,x)−u1(t,x)| and max|v(t,x)−v1(t,x)|, at t=5 across Ω.
△t | △t1 | max|u(5,x)−u1(5,x)| | max|v(5,x)−v1(5,x)| |
0.1 | 0.05 | 0.0040 | 0.00670 |
0.01 | 0.005 | 0.0004 | 0.00067 |
We note that the reduction of Δt by a factor of 0.5 reduces the error by O(Δt), as predicted by Theorem 2.2.
CASEIB: We fix △t=0.1 and vary △x. As before, we let (u(t,x),v(t,x)) represent the numerical solutions corresponding to △x, and let (u1(t,x),v1(t,x)) represent the numerical solutions corresponding to △x1. We compare the error differences max|u(t,x)−u1(t,x)| and max|v(t,x)−v1(t,x)| at t=5 across Ω.
△x | △x1 | max|u(5,x)−u1(5,x)| | max|v(5,x)−v1(5,x)| |
0.05 | 0.025 | 0.000181 | 0.000277 |
0.025 | 0.0125 | 0.000044 | 0.000068 |
We note that the reduction of Δx by a factor of 0.5 reduces the error by a factor of O(Δx2), or about 0.25, as stated in Theorem 2.2.
CASEII. For n=2, let Ω=(−1,1)×(−1,1), and let ϵ=0.1, a=0.4, b=0.6, u0(x,y)=0.4+0.2cos(2πx)cos(2πy), and v0(x,y)=0.5+0.3sin(2πx)sin(2πy), where Jϵ(x,y)=1ϵ2πexp(−x2+y2ϵ2).
We first show graphs of some approximate solutions generated by the two-dimensional method (2.5) for Δt=0.25, and Δx=Δy=0.2 in Figures 3 and 4. As in the one-dimensional case, since convergence is independent of time and space steps and graphs look much the same for any reasonable choices of small Δt and Δx, we have chosen these values as a representative of any such reasonable choice.
CASEIIA: We hold △x=△y=0.1 and compare accuracy for various △t-values in (2.5). Denote the numerical solution (u(t,x,y),v(t,x,y)) as the one generated by (2.5) corresponding to △t and (u1(t,x,y),v1(t,x,y)) corresponding to △t1. We compare the differences max|u(t,x,y)−u1(t,x,y)| and max|v(t,x,y)−v1(t,x,y)| at t=5 across Ω in Table 3.
△t | △t1 | max|u(5,x,y)−u1(5,x,y)| | max|v(5,x,y)−v1(5,x,y)| |
0.1 | 0.05 | 0.011975 | 0.009556 |
0.05 | 0.025 | 0.005879 | 0.004991 |
CASEIIB: Finally, we carry out the same accuracy test for fixed Δt=0.25 and various Δx=Δy and Δx1=Δy1 values at time t=5. The results are displayed in Table 4.
△x | △x1 | max|u(5,x,y)−u1(5,x,y)| | max|v(5,x,y)−v1(5,x,y)| |
0.1 | 0.05 | 0.0022898 | 0.0027063 |
0.05 | 0.025 | 0.0005250 | 0.0006352 |
All approximations in the tables show convergence at the rates predicted by Theorem 2.2.
The foregoing results have motivated the use of a Lotka-Volterra-type equation with operator L that reflects intra-species dispersion, or nonlocal interaction, with competition between species whose populations are given by u and v. A nonstandard numerical scheme was introduced that is stable, independent of the choice of time step, and that yields biologically sensible (nonnegative) numerical approximations to populations u and v of this system. Moreover, this nonstandard scheme was shown to be convergent to the solution of the proposed system and the order of convergence given. Because its convergence was established, it is possible to state with confidence that accurate solutions to the system are shown in the numerical experiments that were offered to confirm the results.
The authors declare they have not used Artificial Intelligence (AI) tools in the creation of this article.
The authors would like to thank the reviewers for their help and insightful suggestions for improvement of this paper.
The authors declare there are no conflicts of interest.
[1] | ICAO (2019) Aviation Benefits Report, Montreal, Global aviation Industry High-level Group. Available from https://www.icao.int/sustainability/Documents/AVIATION-BENEFITS-2019-web.pdf. |
[2] | CAEP 9 (2013) Doc 10012, Committee on Aviation Environmental Protection, Ninth Meeting, 999 University Street, Montréal, Quebec, Canada H3C 5H7, ICAO 10012 CAEP. |
[3] | Brasseur GP, Gupta M, Anderson BE, et al. (2015) Impact of Aviation on Climate: FAA's Aviation Climate Change Research Initiative (ACCRI) Phase Ⅱ. Bull Am Meteorol Soc. |
[4] | FAA (2015) Aviation Emissions, Impacts & Mitigation A Primer, Office of Environment and Energy. |
[5] |
Mahashabde A, Wolfe P, Ashok A, et al. (2011) Assessing the environmental impacts of aircraft noise and emissions. Prog Aerosp Sci 47: 15–52. https://doi.org/10.1016/j.paerosci.2010.04.003 doi: 10.1016/j.paerosci.2010.04.003
![]() |
[6] |
Bendtsen KM, Bengtsen E, Saber AT, et al. (2021) A review of health effects associated with exposure to jet engine emissions in and around airports. Environ Health 20: 10. https://doi.org/10.1186/s12940-020-00690-y doi: 10.1186/s12940-020-00690-y
![]() |
[7] |
Jung K-H, Artigas F, Shin JY (2011) Personal, indoor, and outdoor exposure to VOCs in the immediate vicinity of a local airport. Environ Monit Assess 173: 555–567. https://doi.org/10.1007/s10661-010-1404-9 doi: 10.1007/s10661-010-1404-9
![]() |
[8] |
Levy JI, Woody M, Baek BH, et al. (2012) Current and future particulate-matter-related mortality risks in the United States from aviation emissions during landing and takeoff. Risk Anal Off Publ Soc Risk Anal 32: 237–249. https://doi.org/10.1111/j.1539-6924.2011.01660.x doi: 10.1111/j.1539-6924.2011.01660.x
![]() |
[9] |
Schindler BK, Weiss T, Schütze A, et al. (2013) Occupational exposure of air crews to tricresyl phosphate isomers and organophosphate flame retardants after fume events. Arch Toxicol 87: 645–648. https://doi.org/10.1007/s00204-012-0978-0 doi: 10.1007/s00204-012-0978-0
![]() |
[10] |
Yim SHL, Stettler MEJ, Barrett SRH (2013) Air quality and public health impacts of UK airports. Part Ⅱ: Impacts and policy assessment. Atmos Environ 67: 184–192. https://doi.org/10.1016/j.atmosenv.2012.10.017 doi: 10.1016/j.atmosenv.2012.10.017
![]() |
[11] |
Yim SHL, Lee GL, Lee IH, et al. (2015) Global, regional and local health impacts of civil aviation emissions. Environ Res Lett 10: 034001. https://doi.org/10.1088/1748-9326/10/3/034001 doi: 10.1088/1748-9326/10/3/034001
![]() |
[12] | Kim BY (2012) Guidance for Quantifying the Contribution of Airport Emissions to Local Air Quality, Transportation Research Board. https://doi.org/10.17226/22757 |
[13] | Kim BY (2015) Understanding Airport Air Quality and Public Health Studies Related to Airports, Transportation Research Board. https://doi.org/10.17226/22119 |
[14] | Wood E (2008) Aircraft and Airport-related Hazardous Air Pollutants: Research Needs and Analysis, Transportation Research Board. |
[15] | RIDEM (2008) Characterization of Ambient Air Toxics in Neighborhoods Abutting T.F. Green Airport and Comparison Sites: Final Report, Rhode Island Department of Environmental Management. |
[16] |
Zhu Y, Fanning E, Yu RC, et al. (2011) Aircraft emissions and local air quality impacts from takeoff activities at a large International Airport. Atmos Environ 45: 6526–6533. https://doi.org/10.1016/j.atmosenv.2011.08.062 doi: 10.1016/j.atmosenv.2011.08.062
![]() |
[17] |
Carslaw DC, Beevers SD, Ropkins K, et al. (2006) Detecting and quantifying aircraft and other on-airport contributions to ambient nitrogen oxides in the vicinity of a large international airport. Atmos Environ 40: 5424–5434. https://doi.org/10.1016/j.atmosenv.2006.04.062 doi: 10.1016/j.atmosenv.2006.04.062
![]() |
[18] |
Peace H, Maughan J, Owen B, et al. (2006) Identifying the contribution of different airport related sources to local urban air quality. Environ Model Softw 21: 532–538. https://doi.org/10.1016/j.envsoft.2004.07.014 doi: 10.1016/j.envsoft.2004.07.014
![]() |
[19] |
Farias F, ApSimon H (2006) Relative contributions from traffic and aircraft NOx emissions to exposure in West London. Environ Model Softw 21: 477–485. https://doi.org/10.1016/j.envsoft.2004.07.010 doi: 10.1016/j.envsoft.2004.07.010
![]() |
[20] |
Westerdahl D, Fruin SA, Fine PL, et al. (2008) The Los Angeles International Airport as a source of ultrafine particles and other pollutants to nearby communities. Atmos Environ 42: 3143–3155. https://doi.org/10.1016/j.atmosenv.2007.09.006 doi: 10.1016/j.atmosenv.2007.09.006
![]() |
[21] |
Koulidis AG, Progiou AG, Ziomas IC (2020) Air Quality Levels in the Vicinity of Three Major Greek Airports. Environ Model Assess 25: 749–760. https://doi.org/10.1007/s10666-020-09699-6 doi: 10.1007/s10666-020-09699-6
![]() |
[22] |
Hudda N, Gould T, Hartin K, et al. (2014) Emissions from an International Airport Increase Particle Number Concentrations 4-fold at 10 km Downwind. Environ Sci Technol 48: 6628–6635. https://doi.org/10.1021/es5001566 doi: 10.1021/es5001566
![]() |
[23] |
Mokalled T, Le Calvé S, Badaro-Saliba N, et al. (2018) Identifying the impact of Beirut Airport's activities on local air quality - Part Ⅰ: Emissions inventory of NO2 and VOCs. Atmos Environ 187: 435–444. https://doi.org/10.1016/j.atmosenv.2018.04.036 doi: 10.1016/j.atmosenv.2018.04.036
![]() |
[24] | CERC (2015) EMIT: Atmospheric Emissions Inventory Toolkit User Guide. Version 3.4, 3 King's Parade. Carmbridge CB2 1SJ, Cambridge Environmental Research Consultant Ltd. |
[25] |
Mokalled T, Adjizian Gérard J, Abboud M, et al. (2019) An assessment of indoor air quality in the maintenance room at Beirut-Rafic Hariri International Airport. Atmospheric Pollut Res 10: 701–711. https://doi.org/10.1016/j.apr.2018.11.008 doi: 10.1016/j.apr.2018.11.008
![]() |
[26] |
Mokalled T, Adjizian Gérard J, Abboud M, et al. (2021) An Assessment of Indoor Air Quality in the Arrivals Hall of Beirut–Rafic Hariri International Airport: Monitoring of VOCs and NO2. Atmosphere 12: 400. https://doi.org/10.3390/atmos12030400 doi: 10.3390/atmos12030400
![]() |
[27] |
Baayoun A, Itani W, El Helou J, et al. (2019) Emission inventory of key sources of air pollution in Lebanon. Atmos Environ 215: 116871. https://doi.org/10.1016/j.atmosenv.2019.116871 doi: 10.1016/j.atmosenv.2019.116871
![]() |
[28] |
Salameh T, Afif C, Sauvage S, et al. (2014) Speciation of non-methane hydrocarbons (NMHCs) from anthropogenic sources in Beirut, Lebanon. Environ Sci Pollut Res 21: 10867–10877. https://doi.org/10.1007/s11356-014-2978-5 doi: 10.1007/s11356-014-2978-5
![]() |
[29] |
Waked A, Afif C (2012) Emissions of air pollutants from road transport in Lebanon and other countries in the Middle East region. Atmos Environ 61: 446–452. https://doi.org/10.1016/j.atmosenv.2012.07.064 doi: 10.1016/j.atmosenv.2012.07.064
![]() |
[30] |
Mokalled T, Adjizian Gérard J, Abboud M, et al. (2018) VOC tracers from aircraft activities at Beirut Rafic Hariri International Airport. Atmospheric Pollut Res. https://doi.org/10.1016/j.apr.2018.09.009 doi: 10.1016/j.apr.2018.09.009
![]() |
[31] | ICAO (2008) Report of the independent experts on the LTTG NOx review and medium and long term technology goals for NOx., Montréal :, International Civil Aviation Organization. |
[32] |
Badaro-Saliba N, Adjizian-Gerard J, Zaarour R, et al. (2013) A geostatistical approach for assessing population exposure to NO2 in a complex urban area (Beirut, Lebanon). Stoch Environ Res Risk Assess 28: 467–474. https://doi.org/10.1007/s00477-013-0765-3 doi: 10.1007/s00477-013-0765-3
![]() |
[33] | US EPA O (2021) US EPA, Volatile Organic Compounds' Impact on Indoor Air Quality, 2021. Available from: https://www.epa.gov/indoor-air-quality-iaq/volatile-organic-compounds-impact-indoor-air-quality. |
[34] | Lebanese DGCA (2015) Statistics Department Daily Transactions. |
[35] | Lebanese DGCA (2016) Statistics - Beirut Rafic Hariri International Airport, 2016. Available from: https://www.beirutairport.gov.lb/_statistic.php. |
[36] | Lebanese DGCA (2017) eAIS Package for Lebanon, 2017. Available from: https://eaip.austrocontrol.at/all/ol/170105/2017-01-05-AIRAC/index.html. |
[37] | CERC (2017) ADMS-AIrport User Guide. version 4.1.1, 3 King's Parade. Carmbridge CB2 1SJ, Cambridge Environmental Research Consultant Ltd. |
[38] | CERC (2021) CERC > Environmental software > ADMS-Airport > Input and output, 2021. Available from: https://www.cerc.co.uk/environmental-software/ADMS-Airport-model/data.html. |
[39] | ICAO (2011) Airport Air Quality Manual (Doc 9889). |
[40] | Azzi M, Johnson (1992) An introduction to the generic reaction set photochemical smog mechanism, Proc. 11th Clean Air Conference, Brisbane, Australia, Best, P., Bofinger, N. and Cliff, D., 451–462. |
[41] |
Venkatram A, Karamchandani P, Pai P, et al. (1994) The development and application of a simplified ozone modeling system (SOMS). Atmos Environ 28: 3665–3678. https://doi.org/10.1016/1352-2310(94)00190-V doi: 10.1016/1352-2310(94)00190-V
![]() |
[42] |
Carruthers DJ, McHugh C, Jackson M, et al. (2011) Developments in ADMS-Airport to take account of near field dispersion and applications to Heathrow Airport. Int J Environ Pollut 44: 332. https://doi.org/10.1504/IJEP.2011.038434 doi: 10.1504/IJEP.2011.038434
![]() |
[43] | CAEP (2008) LAQ Candidate Models Capabilities and Inter-Comparison Study. |
[44] | CERC (2021) CERC > Environmental software > ADMS-Airport model, 2021. Available from: https://www.cerc.co.uk/environmental-software/ADMS-Airport-model.html. |
[45] | DfT (2006) Project for the Sustainable Development of Heathow - Report of the Air Quality Technical Panels. |
[46] | McHugh C (2007) Air Quality Studies for Heathrow: Base Case, Segregated Mode, Mixed Mode and Third Runway Scenarios modelled using ADMS-Airport. |
[47] | CERC (2022) CERC > Environmental software > ADMS-Airport > Model options, 2022. Available from: https://www.cerc.co.uk/environmental-software/ADMS-Airport-model/options.html. |
[48] | FAA (2014) Aviation Emissions and Air Quality Handbook Version 3. |
[49] |
Saltzman BE (1954) Colorimetric Microdetermination of Nitrogen Dioxide in Atmosphere. Anal Chem 26: 1949–1955. https://doi.org/10.1021/ac60096a025 doi: 10.1021/ac60096a025
![]() |
[50] |
Glasius M, Funch Carlsen M, Stroyer Hansen T, et al. (1999) Measurements of nitrogen dioxide on Funen using diffusion tubes. Atmos Environ 33: 1177–1185. https://doi.org/10.1016/S1352-2310(98)00285-4 doi: 10.1016/S1352-2310(98)00285-4
![]() |
[51] | Passam (2017) Nitrogen Dioxide – passam, 2017. Available from: http://www.passam.ch/wp/nitrogen-dioxide/. |
[52] | Carruthers DJ, McHugh C, Church S, et al. (2008) ADMS-AIRPORT: MODEL INTER-COMPARISIONS AND MODEL VALIDATION, CERC, 3 King's Parade, Cambridge CB2 1SJ, UK. |
[53] | Carruthers DJ, Gray S, Johnson K, et al. (2007) Intercomparison of five modelling approaches including ADMS-Airport and EDMS/AERMOD for predicting air quality in the vicinity of London Heathrow Airport, Air and Waste Management Association—Guideline on Air Quality Models: Applications and FLAG Developments 2006. A & WMA, 247–258. |
[54] | Evans JD (1996) Straightforward statistics for the behavioral sciences, Pacific Grove, Brooks/Cole Pub. Co. |
[55] |
Kowang TO, Long CS, Rasli A (2015) Innovation Management and Performance Framework for Research University in Malaysia. Int Educ Stud 8: 32. https://doi.org/10.5539/ies.v8n6p32 doi: 10.5539/ies.v8n6p32
![]() |
[56] |
Masiol M, Harrison RM (2014) Aircraft engine exhaust emissions and other airport-related contributions to ambient air pollution: A review. Atmos Environ 95: 409–455. https://doi.org/10.1016/j.atmosenv.2014.05.070 doi: 10.1016/j.atmosenv.2014.05.070
![]() |
[57] | Yaman SH (2001) Aircraft and vehicle induced emissions at the Beirut International Airport a characterization and exposure assessment - by Sarah Hassan Yaman. |
[58] |
Anderson BE, Chen G, Blake DR (2006) Hydrocarbon emissions from a modern commercial airliner. Atmos Environ 40: 3601–3612. https://doi.org/10.1016/j.atmosenv.2005.09.072 doi: 10.1016/j.atmosenv.2005.09.072
![]() |
[59] |
Adamkiewicz G, Hsu H-H, Vallarino J, et al. (2010) Nitrogen dioxide concentrations in neighborhoods adjacent to a commercial airport: a land use regression modeling study. Environ Health Glob Access Sci Source 9: 73. https://doi.org/10.1186/1476-069X-9-73 doi: 10.1186/1476-069X-9-73
![]() |
△t | △t1 | max|u(5,x)−u1(5,x)| | max|v(5,x)−v1(5,x)| |
0.1 | 0.05 | 0.0040 | 0.00670 |
0.01 | 0.005 | 0.0004 | 0.00067 |
△x | △x1 | max|u(5,x)−u1(5,x)| | max|v(5,x)−v1(5,x)| |
0.05 | 0.025 | 0.000181 | 0.000277 |
0.025 | 0.0125 | 0.000044 | 0.000068 |
△t | △t1 | max|u(5,x,y)−u1(5,x,y)| | max|v(5,x,y)−v1(5,x,y)| |
0.1 | 0.05 | 0.011975 | 0.009556 |
0.05 | 0.025 | 0.005879 | 0.004991 |
△x | △x1 | max|u(5,x,y)−u1(5,x,y)| | max|v(5,x,y)−v1(5,x,y)| |
0.1 | 0.05 | 0.0022898 | 0.0027063 |
0.05 | 0.025 | 0.0005250 | 0.0006352 |
△t | △t1 | max|u(5,x)−u1(5,x)| | max|v(5,x)−v1(5,x)| |
0.1 | 0.05 | 0.0040 | 0.00670 |
0.01 | 0.005 | 0.0004 | 0.00067 |
△x | △x1 | max|u(5,x)−u1(5,x)| | max|v(5,x)−v1(5,x)| |
0.05 | 0.025 | 0.000181 | 0.000277 |
0.025 | 0.0125 | 0.000044 | 0.000068 |
△t | △t1 | max|u(5,x,y)−u1(5,x,y)| | max|v(5,x,y)−v1(5,x,y)| |
0.1 | 0.05 | 0.011975 | 0.009556 |
0.05 | 0.025 | 0.005879 | 0.004991 |
△x | △x1 | max|u(5,x,y)−u1(5,x,y)| | max|v(5,x,y)−v1(5,x,y)| |
0.1 | 0.05 | 0.0022898 | 0.0027063 |
0.05 | 0.025 | 0.0005250 | 0.0006352 |