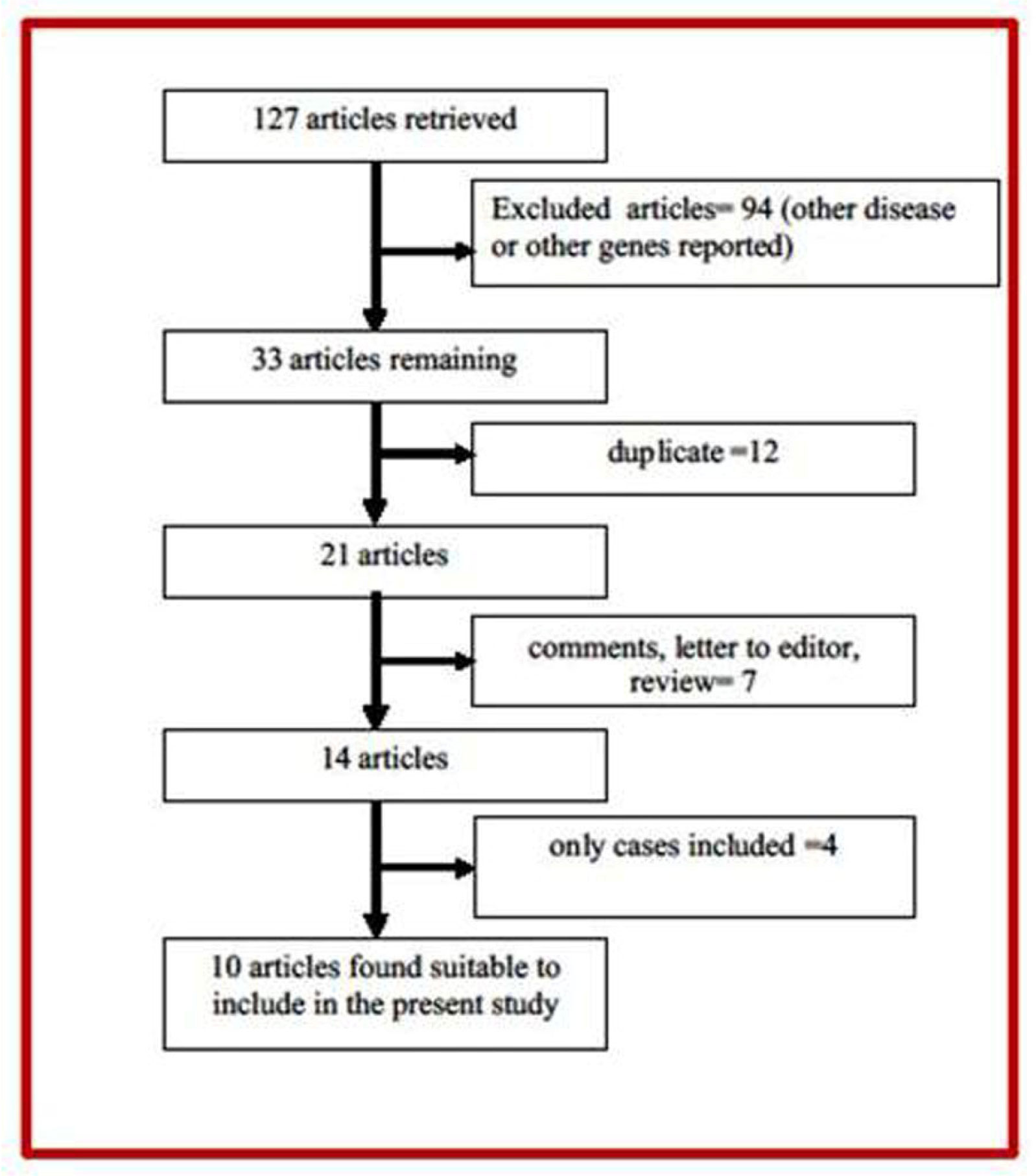
Transmission congestions are caused by electricity trading between generators and distribution companies in a deregulated environment. Power system operation and security in liberalized scenarios are maintained by removing branch overloads. A flexible alternating current transmission system (FACTS) controller is installed in a suitable location to redistribute the power flow among the transmission lines so that the power flows are brought within the capacity of the lines. In this work, series-connected thyristor-controlled switched compensators (TCSCs) and shunt-connected Volt-Ampere reactive (VAR) static compensators (SVCs) are installed in appropriate locations to alter the power flow patterns and to remove overloads. It is proposed to reduce the overload of transmission lines by locating series and shunt connected FACTS devices at proper locations. The size and location of TCSC and SVC devices greatly affect their ability to meet a congestion management goal. An optimization process optimizes the location and size of these devices to maximize the congestion mitigation benefits of the TCSC and SVC controllers. In this work, the whale optimization algorithm (WOA) is used to optimize the value of the objective function by appropriately choosing the location and size of the FACTS controllers. This algorithm has a few parameters that are tuned to give the best overall results. A WOA-based method is proposed to optimize the size and location of the FACTS devices and is implemented on the IEEE-30 bus test case. The results were compared and found to be improved with those of other algorithms such as the particle swarm optimization algorithm (PSO) and the firefly algorithm (FFA).
Citation: Vengadesan Alagapuri, Ashok Bakkiyaraj Radhakrishnan, S. Sakthivel Padaiyatchi. Optimization of position and rating of shunt and series connected FACTS devices for transmission congestion management in deregulated power networks[J]. AIMS Electronics and Electrical Engineering, 2024, 8(2): 165-186. doi: 10.3934/electreng.2024007
[1] | Qianqian Zhang, Mingye Mu, Heyuan Ji, Qiushi Wang, Xingyu Wang . An adaptive type-2 fuzzy sliding mode tracking controller for a robotic manipulator. Electronic Research Archive, 2023, 31(7): 3791-3813. doi: 10.3934/era.2023193 |
[2] | Lingling Zhang . Vibration analysis and multi-state feedback control of maglev vehicle-guideway coupling system. Electronic Research Archive, 2022, 30(10): 3887-3901. doi: 10.3934/era.2022198 |
[3] | Duhui Chang, Yan Geng . Distributed data-driven iterative learning control for multi-agent systems with unknown input-output coupled parameters. Electronic Research Archive, 2025, 33(2): 867-889. doi: 10.3934/era.2025039 |
[4] | Zhizhou Zhang, Yueliang Pan, Weilong Zhao, Jinchu Zhang, Zheng Zi, Yuan Xie, Hehong Zhang . Frequency analysis of a discrete-time fast nonlinear tracking differentiator algorithm based on isochronic region method. Electronic Research Archive, 2024, 32(9): 5157-5175. doi: 10.3934/era.2024238 |
[5] | Jian Gong, Yuan Zhao, Jinde Cao, Wei Huang . Platoon-based collision-free control for connected and automated vehicles at non-signalized intersections. Electronic Research Archive, 2023, 31(4): 2149-2174. doi: 10.3934/era.2023111 |
[6] | Chao Ma, Hang Gao, Wei Wu . Adaptive learning nonsynchronous control of nonlinear hidden Markov jump systems with limited mode information. Electronic Research Archive, 2023, 31(11): 6746-6762. doi: 10.3934/era.2023340 |
[7] | Tongtong Yu, Zhizhou Zhang, Yang Li, Weilong Zhao, Jinchu Zhang . Improved active disturbance rejection controller for rotor system of magnetic levitation turbomachinery. Electronic Research Archive, 2023, 31(3): 1570-1586. doi: 10.3934/era.2023080 |
[8] | Xingting Geng, Jianwen Feng, Yi Zhao, Na Li, Jingyi Wang . Fixed-time synchronization of nonlinear coupled memristive neural networks with time delays via sliding-mode control. Electronic Research Archive, 2023, 31(6): 3291-3308. doi: 10.3934/era.2023166 |
[9] | Ning Li, Yuequn Gao . Modified fractional order social media addiction modeling and sliding mode control considering a professionally operating population. Electronic Research Archive, 2024, 32(6): 4043-4073. doi: 10.3934/era.2024182 |
[10] | Chao Yang, Juntao Wu, Zhengyang Qiao . An improved fixed-time stabilization problem of delayed coupled memristor-based neural networks with pinning control and indefinite derivative approach. Electronic Research Archive, 2023, 31(5): 2428-2446. doi: 10.3934/era.2023123 |
Transmission congestions are caused by electricity trading between generators and distribution companies in a deregulated environment. Power system operation and security in liberalized scenarios are maintained by removing branch overloads. A flexible alternating current transmission system (FACTS) controller is installed in a suitable location to redistribute the power flow among the transmission lines so that the power flows are brought within the capacity of the lines. In this work, series-connected thyristor-controlled switched compensators (TCSCs) and shunt-connected Volt-Ampere reactive (VAR) static compensators (SVCs) are installed in appropriate locations to alter the power flow patterns and to remove overloads. It is proposed to reduce the overload of transmission lines by locating series and shunt connected FACTS devices at proper locations. The size and location of TCSC and SVC devices greatly affect their ability to meet a congestion management goal. An optimization process optimizes the location and size of these devices to maximize the congestion mitigation benefits of the TCSC and SVC controllers. In this work, the whale optimization algorithm (WOA) is used to optimize the value of the objective function by appropriately choosing the location and size of the FACTS controllers. This algorithm has a few parameters that are tuned to give the best overall results. A WOA-based method is proposed to optimize the size and location of the FACTS devices and is implemented on the IEEE-30 bus test case. The results were compared and found to be improved with those of other algorithms such as the particle swarm optimization algorithm (PSO) and the firefly algorithm (FFA).
Alcohol dependence (AD) or Alcoholism, also regarded as alcohol use disorder (AUD), is a complex and relapsing neuropsychiatric disorder [1],[2]. World Health Organization (WHO) reported that approximately 140 million individuals addicted to alcohol globally, resulting in to 2.5 million death each year [3]. AD is regarded as a “reward deficiency syndrome” that intemperately affects public health [4],[5]. It has been found to be influenced by both genetic and environmental factors [6],[7]. Exact patho-physiological and molecular mechanism of AD is not known yet. However, molecular genetic studies support that multiple genes determine an individual's predisposition to AD [8]. Heritability of AD likely plays an important role in its development and is determined to be moderate to high [9],[10]. It was reported frequently that alcohol consumption increased homocysteine (Hcy) concentration i.e hyperhomocysteinaemia [11]. However, inconsistent results of the combined effect of both positive and negative association have been reported between alcohol intake and Hcy [12]. Hyperhomocystenemia is already reported as risk factor for several diseases or disorders including neural tube defects, Alzheimer disease, schizophrenia, pregnancy complications, cardiovascular diseases, noninsulin dependent diabetes and end-stage renal disease as evidenced from several studies [13].
Homocysteine is a sulfur containing amino acid, several genetic and environmental risk factor are reported for higher plasma concentration of homocysteine [14]. Homocysteine (Hcy) is synthesized in methionine and folate cycle by demethylation of methionine. 5,10-methylenetetrahydrofolate reductase (MTHFR) enzyme of folate cycle plays an important role in homocysteine metabolism. MTHFR gene is present on chromosome 1p36.3. Numerous single nucleotide polymorphisms (SNP) are known in MTHFR gene like C677T and A1298C etc [15],[16]. The most clinically important and studies polymorphism is C677T (rs1801133), in which cytosine (C) is substituted with thymine (T) at 677 nucleotide position and consequently alanine is replaced by valine in MTHFR enzyme (Ala 222 Val) [17],[18]. The variant MTHFR enzyme is thermolabile with reduced activity (~70%) and it increased the plasma homocysteine concentrations [15] (Frosst et al., 1995). Globally, frequency of mutant T allele varies greatly [19]–[23]. Yadav et al. [23] have conducted a comprehensive C667T polymorphism study and reported the highest frequency in European populations ranging from 24.1% to 64.3% and, lowest frequency from African population. Several studies revealed association of MTHFR gene C677T polymorphism with AD. However, findings showed inconsistent results [24]–[26]. To derive a more precise estimation of the relationship, authors have performed a meta-analysis.
Present meta-analysis is carried out according to MOOSE (Meta-analysis of observational studies in epidemiology) guidelines.
Articles were retrieved through Pubmed, Google scholar, Springer Link, and Science Direct databases up to February 28, 2020, using following key words: “Methylenetetrahydrofolate reductase” or “MTHFR” or “C677T” or “rs1801133” or “polymorphism” and “Alcohol dependence” or “Alcoholism” or “AD” or “Addiction”.
Inclusion criteria were following: (1) MTHFR C677T polymorphism and alcohol dependence association was investigated in the study, (2) MTHFR C677T genotype/allele numbers in alcohol dependence cases and controls were given in the study, (3) sufficient information for calculating the odds ratio (OR) with 95% confidence interval (CI) and (4) Articles published in English language were only considered. Major reasons for studies exclusion were as follows: (1) no alcohol dependence cases analyzed, (2) the C677T polymorphism details information missing, and (3) duplicate article.
Name of first author, country name, number of cases and controls, number of genotypes in cases and controls and journal name with full reference from each article were extracted.
All analysis were done according to the method of Rai et al. [27]. Odds ratio (ORs) with 95% confidence intervals (CIs) were calculated using fixed effect and random effect models [28],[29]. A five genetic models viz. allele contrast, co-dominant, homozygote, dominant and recessive models were calculated. Heterogeneity was investigated and quantified by I2 statistic [30]. Chi-squared analysis was used to determine whether the genotype distribution of control group was in Hardy–Weinberg equilibrium or not. Subetaoup analysis was conducted by ethnicity. In included articles, case samples were not categorized on the basis of gender, so the subetaoup analysis based on gender did not performed in present meta-analysis. Publication bias was investigated by Egger's regression intercept test [31]. P value <0.05 was considered statistically significant. All calculations were done by softwares MIX version 1.7 [32] and MetaAnalyst [33] program.
Selection of studies is given in fow diagram (Figure 1). Following the exclusion criteria, 10 individual case-control studies with a total of 1676 cases and 1594 controls were included into this meta-analysis [24]–[26],[34]–[40]. One author [38] reported their data in to two categories, we included both set of data as different studies. Hence, total number of included studies in present meta-analysis is eleven (Table 1).
Study | Ethnicity | Control | Case | Case Genotype |
Control Genotype |
HWE p-value of controls | ||||
CC | CT | TT | CC | CT | TT | |||||
Bonsch, 2006 | Caucasian | 115 | 134 | 64 | 56 | 14 | 60 | 41 | 14 | 0.10 |
Lutz, 2006 | Caucasian | 102 | 221 | 95 | 94 | 32 | 53 | 41 | 8 | 0.98 |
Lutz, 2007 | Caucasian | 101 | 142 | 65 | 58 | 19 | 53 | 40 | 8 | 0.90 |
Saffroy, 2008 | Caucasian | 93 | 242 | 108 | 113 | 21 | 35 | 41 | 17 | 0.41 |
Benyamina, 2009 | Caucasian | 93 | 120 | 56 | 53 | 11 | 35 | 41 | 17 | 0.41 |
Fabris, 2009 | Caucasian | 236 | 63 | 17 | 35 | 11 | 69 | 113 | 54 | 0.55 |
Shin, 2010 | Asian | 232 | 68 | 11 | 39 | 18 | 42 | 129 | 61 | 0.07 |
Supic, 2010, Heavy Alcoholic | Caucasian | 57 | 32 | 13 | 9 | 10 | 27 | 24 | 6 | 0.84 |
Supic, 2010, Non heavy Alcoholic | Caucasian | 105 | 64 | 37 | 23 | 4 | 53 | 42 | 10 | 0.69 |
Singh, 2014 | Asian | 313 | 139 | 91 | 44 | 4 | 228 | 78 | 7 | 0.91 |
Singh, 2015 | Asian | 147 | 451 | 312 | 125 | 14 | 107 | 35 | 5 | 0.32 |
Overall, eleven studies provided 1676/1594 cases/controls for MTHFR C677T polymorphism. The prevalence of C and T alleles in AD cases was 71.22% and 28.79% respectively. The percentage frequency of TT genotype among cases and controls was 9.43% and 12.98%, respectively whereas prevalence of CT heterozygote among AD cases was 38.72% and 39.21% in controls. The prevalence of CC homozygote among AD cases and controls was 51.85% and 47.80%, respectively. Genotypes were in Hardy-Weinberg equilibrium in all controls. In control group the percentage of C and T allele frequencies was 67.41% and 32.59% respectively (Figure 2).
No significant association was observed between the MTHFR C677T polymorphism and the susceptibility to AD in all the genetic models using random effect model (for T vs. C (allele contrast): OR = 1.04, 95% CI = 0.88–1.24; CT vs. CC (co-dominant): OR = 1.02, 95% CI = 0.62–1.68; for TT+CT vs. CC (dominant): OR = 1.10, 95% CI = 0.94–1.29; for TT vs. CC (homozygote): OR = 1.01, 95% CI = 0.66–1.51; for TT vs. CT + CC (recessive): OR = 0.97, 95% CI = 0.66–1.40) (Table 2; Figures 3).
A true heterogeneity existed between studies for allele contrast (Pheterogeneity = 0.02, Q = 20.64, I2 = 51.56%, t2 = 0.04, z = 0.69), co-dominant genotype (Pheterogeneity < 0.0001, Q = 86.64, I2 = 88.46%, t2 = 0.61, z = 4.29), homozygote genotype (Pheterogeneity = 0.02, Q = 20.93, I2 = 52.24%, t2 = 0.24, z = 0.1), and recessive genotype (Pheterogeneity = 0.02, Q = 21.00, I2 = 52.40%, t2 = 0.20, z = 0.47) comparisons. The “I2” value of more than 50% shows high level of true heterogeneity.
Genetic Models | Fixed effect OR (95% CI), p | Random effect OR (95% CI), p | Heterogeneity p-value (Q test) | I2 (%) | Publication Bias (p of Egger's test) |
Allele Contrast (T vs. C) | 1.04 (0.92–1.17), 0.48 | 1.04 (0.88–1.24), 0.60 | 0.02 | 51.56 | 0.62 |
Dominant (TT+CT vs. CC) | 1.41 (1.20–1.65), <0.0001 | 1.02 (0.62–1.68), 0.92 | <0.0001 | 88.46 | 0.06 |
Homozygote (TT vs. CC) | 0.98 (0.75–1.29), 0.92 | 1.01 (0.66–1.51), 0.97 | 0.02 | 52.24 | 0.26 |
Co-dominant (CT vs. CC) | 1.10 (0.94–1.29), 0.21 | 1.10 (0.94–1.29), 0.21 | 0.43 | 0 | 0.48 |
Recessive (CC+CT vs. TT) | 0.94 (0.73–1.20), 0.63 | 0.97 (0.66–1.40), 0.86 | 0.02 | 52.4 | 0.28 |
Out of 11 studies included in the present meta-analysis, 3 studies were carried out in Asian countries, and 8 studies were carried out on Caucasian (Table 1). The subetaoup analysis by ethnicity did not reveal any significant association between MTHFR C677T polymorphism and AD in Asian population (T vs. C: OR = 1.16; 95% CI = 0.93–1.44; p = 0.17; I2 = 3.1%; Pheterogeneity = 0.65; TT vs. CC: OR = 1.16; 95% CI = 0.62–2.02; p = 0.69; I2 = 3.1%; Pheterogeneity = 0.89; and TT+CT vs. CC: OR = 1.26; 95% CI = 0.96–1.67; p = 0.09; I2 = 3.1%; Pheterogeneity = 0.81); and Caucasian population (T vs. C: OR = 0.99; 95% CI = 0.86–1.14; p = 0.93; I2 = 61.75%; Pheterogeneity = 0.01; TT vs. CC: OR= 0.95; 95% CI = 0.70–1.29; p = 0.75; I2 = 65.63%; Pheterogeneity = 0.004; and TT+CT vs. CC: OR = 1.03; 95% CI = 0.85–1.25; p = 0.75; I2 = 13.93%; Pheterogeneity = 0.32) (Figures 4 and 5).
Symmetrical shape of Funnel plots' revealed absence of publication bias. P values of Egger's test were more than 0.05, also provided statistical evidence for the funnel plots' symmetry (p = 0.62 for T vs. C; p = 0.48 for TT vs. CC; p = 0.26 for CT vs. CC; p = 0.48 for TT+AC vs. CC; p = 0.28 for TT vs. CT+CC) (Table 2; Figure 6).
In vivo and in vitro studies has demonstrated that homocysteine has neurotoxic effects especially on dopamine neurons of reward pathway [11]. In addition, hyperhomocysteinemia is also reported in AD [11],[41]. In MTHFR gene several polymorphisms are reported but according to deficit hypothesis of addiction, only C677T polymorphism-dependent alteration of the reward system possibly leads to alcohol addiction. Further, homovanillic acid (HVA) is a potential indicator of central dopaminergic neuronal activity [42] and experimentally, it was demonstrated that higher concentration of homocysteine lowers the level of HVA in rat striatum region [43]. On the basis of 11 studies providing data on MTHFR C677T genotype and AD risk in two ethnic populations, including over 3,205 subjects, our meta-analysis provides an evidence that TT and CT genotypes or T allele are not associated with AD risk. Hence the present meta-analysis indicated that C677T is not a risk factor of AD.
Meta-analysis is a statistical tool to combine the information of independent case-control studies with similar target [44]. Several meta-analysis are published, which evaluated effects of folate pathway genes polymorphisms in susceptibility of diseases/disorders- cleft lip and palate [45], down syndrome [46]–[48], male infertility [49], bipolar disorder [50], schizophrenia [51],[52], depression [53], obsessive compulsive disorder [54], hyperurecemia [55], epilepsy [56], Alzheimers disease [57], esophageal cancer [58], and ovary cancer [59].
Several limitations that should be acknowledged like (i) calculated crude Odds ratio, (ii) included the less number of available studies (10 studies) and the limited sample size of each included study, (iii) observed higher between study heterogeneity, (iv) considered only one gene polymorphism and (v) not considered other confounding factors like diet, gender etc. In addition to limitations, current meta-analysis has several strength also such as—higher study power and larger sample size in comparison to individual case control studies, and absence of publication bias etc.
In conclusion, pooled analysis of data from 11 separate articles indicates that the MTHFR 677TT genotype is not a risk factor for AD. The results of present meta-analysis should be interpreted with certain cautions due to presence of higher heterogeneity and small number of included studies. Future large-scale, population-based association studies from different regions of the world are required to investigate potential gene-gene and gene-environment interactions involving the MTHFR C677T polymorphism in determining AD risk.
[1] |
Fang RS, David AK (1999) Optimal Dispatch under transmission contracts. IEEE T Power Syst 14: 732–737. https://doi.org/10.1109/59.761905 doi: 10.1109/59.761905
![]() |
[2] |
Choudekar P, Asija D (2021) Transmission congestion management using multi objective hybrid flower pollination and particle swarm optimization algorithm by optimal placement of TCSC. Metaheuristic and Evolutionary Computation: Algorithms and Applications, 569–583. https://doi.org/10.1007/978-981-15-7571-6_26 doi: 10.1007/978-981-15-7571-6_26
![]() |
[3] |
Patel NK, Suthar BN, Thakkar J (2021) Transmission congestion management considering voltage stability margin. SN Applied Sciences 3: 261. https://doi.org/10.1007/s42452-020-04132-9 doi: 10.1007/s42452-020-04132-9
![]() |
[4] | Gad M, Shinde P, Kulkarni SU (2012) Optimal location of TCSC by sensitivity methods. Int J Comput Eng Res 2: 162–168. |
[5] |
Leung HC, Chung TS (2000) Optimal power flow with a versatile FACTS controller by genetic algorithm approach. Proc. Power engineering society winter meeting 4: 2806–2811. https://doi.org/10.1049/cp:20000387 doi: 10.1049/cp:20000387
![]() |
[6] | Hingorani NG, Gyugyi L (1998) Understanding FACTS. The Institution of Electrical and Electronics Engineers, 1998. |
[7] |
Gyugyi L, Schauder CD, Williams SL, Rietman TR, Torgerson DR, Edris A (1995) The unified power flow controller: a new approach to power transmission control. IEEE T Power Deliver 10: 1085–1097. https://doi.org/10.1109/61.400878 doi: 10.1109/61.400878
![]() |
[8] |
Larsen E, Millers N, Nilsson S, Lindgren S (1992) Benefits of GTO-based compensation systems for electric utility applications. IEEE T Power Deliver 7: 2056–2064. https://doi.org/10.1109/61.157009 doi: 10.1109/61.157009
![]() |
[9] |
Preedavinchit P, Srivastava SC (1998) Optimal reactive power dispatch considering FACTS devices. Electric Power Syst Res 46: 251–257. https://doi.org/10.1016/S0378-7796(98)00075-3 doi: 10.1016/S0378-7796(98)00075-3
![]() |
[10] | Momoh JA, Zhu JZ (1998) A new approach to optimal power flow with phase shifter. SMC'98 Conference Proceedings. 1998 IEEE International Conference on Systems, Man, and Cybernetics (Cat. No. 98CH36218) 5: 4794–4799. |
[11] |
Liu JY, Song YH (1999) Comparison studies of unified power flow controller with static var compensators and phase shifters. Electric Machines & Power Systems 27: 237–251. https://doi.org/10.1080/073135699269271 doi: 10.1080/073135699269271
![]() |
[12] |
Besharat H, Taher SA (2008) Congestion management by determining optimal location of TCSC in deregulated power systems. Int J Elec Power 30: 563–568. http://dx.doi.org/10.1016/j.ijepes.2008.08.007 doi: 10.1016/j.ijepes.2008.08.007
![]() |
[13] |
Singh SN, David AK (2001) Optimal location of FACTS devices for congestion management. Electr Power Syst Res 58: 71–79. https://doi.org/10.1016/S0378-7796(01)00087-6 doi: 10.1016/S0378-7796(01)00087-6
![]() |
[14] |
Amjady N, Hakimi M (2012) Dynamic voltage stability constrained congestion management framework for deregulated electricity markets. Energy Convers Manag 58: 66–75. https://doi.org/10.1016/j.enconman.2012.01.006 doi: 10.1016/j.enconman.2012.01.006
![]() |
[15] | Gerbex S, Cherkaoui R, Germond AJ (2003) Optimal location of FACTS devices to enhance power system security. 2003 IEEE Bologna Power Tech Conference Proceedings 3: 7-pp. IEEE. |
[16] |
Rashed GI, Sun Y (2011) Optimal placement of Thyristor controlled series compensation for enhancing power system security based on computational intelligence techniques. Procedia Engineering 15: 908–914. https://doi.org/10.1016/j.proeng.2011.08.168 doi: 10.1016/j.proeng.2011.08.168
![]() |
[17] |
Rahim MA, Musirin I, Abidin IZ, Othman MM (2010) Contingency based congestion management and cost minimization using bee colony optimization technique. PECon2010 - 2010 IEEE International Conference on Power and Energy 891–896. https://doi.org/10.1109/PECON.2010.5697705 doi: 10.1109/PECON.2010.5697705
![]() |
[18] |
Shrestha GB, Feng W (2005) Effects of series compensation on spot price power markets. Int J Elec Power 27: 428–436. https://doi.org/10.1016/j.ijepes.2005.03.001 doi: 10.1016/j.ijepes.2005.03.001
![]() |
[19] |
Hosseinipoor N, Nabavi SMH (2010) Optimal locating and sizing of TCSC using genetic Algorithm for congestion management in deregualted power markets. 2010 9th International Conference on Environment and Electrical Engineering 136–139. https://doi.org/10.1109/EEEIC.2010.5489968 doi: 10.1109/EEEIC.2010.5489968
![]() |
[20] |
Biswas PP, Arora P, Mallipeddi R, Suganthan PN, Panigrahi BK (2021) Optimal placement and sizing of FACTS devices for optimal power flow in a wind power integrated electrical network. Neural Comput Appl 33: 6753–6774. https://doi.org/10.1007/s00521-020-05453-x doi: 10.1007/s00521-020-05453-x
![]() |
[21] | Verma KS, Singh SN, Gupta HO (2001) FACTS devices location for enhancement of total transfer capability. 2001 IEEE Power Engineering Society Winter Meeting. Conference Proceedings (Cat. No. 01CH37194) 2: 522–527. |
[22] |
Jordehi AR (2015) Brainstorm optimisation algorithm (BSOA): An efficient algorithm for finding optimal location and setting of FACTS devices in electric power systems. Int J Elec Power 69: 48–57. https://doi.org/10.1016/j.ijepes.2014.12.083 doi: 10.1016/j.ijepes.2014.12.083
![]() |
[23] |
Mirjalili S, Lewis A (2016) The whale optimization algorithm. Adv Eng Softw 95: 51-67. https://doi.org/10.1016/j.advengsoft.2016.01.008 doi: 10.1016/j.advengsoft.2016.01.008
![]() |
[24] | The IEEE 30-Bus Test System. Available from: http://www.ee.washington.edu/research/pstca/pf30/pg$_$tca30bus.htm. |
Study | Ethnicity | Control | Case | Case Genotype |
Control Genotype |
HWE p-value of controls | ||||
CC | CT | TT | CC | CT | TT | |||||
Bonsch, 2006 | Caucasian | 115 | 134 | 64 | 56 | 14 | 60 | 41 | 14 | 0.10 |
Lutz, 2006 | Caucasian | 102 | 221 | 95 | 94 | 32 | 53 | 41 | 8 | 0.98 |
Lutz, 2007 | Caucasian | 101 | 142 | 65 | 58 | 19 | 53 | 40 | 8 | 0.90 |
Saffroy, 2008 | Caucasian | 93 | 242 | 108 | 113 | 21 | 35 | 41 | 17 | 0.41 |
Benyamina, 2009 | Caucasian | 93 | 120 | 56 | 53 | 11 | 35 | 41 | 17 | 0.41 |
Fabris, 2009 | Caucasian | 236 | 63 | 17 | 35 | 11 | 69 | 113 | 54 | 0.55 |
Shin, 2010 | Asian | 232 | 68 | 11 | 39 | 18 | 42 | 129 | 61 | 0.07 |
Supic, 2010, Heavy Alcoholic | Caucasian | 57 | 32 | 13 | 9 | 10 | 27 | 24 | 6 | 0.84 |
Supic, 2010, Non heavy Alcoholic | Caucasian | 105 | 64 | 37 | 23 | 4 | 53 | 42 | 10 | 0.69 |
Singh, 2014 | Asian | 313 | 139 | 91 | 44 | 4 | 228 | 78 | 7 | 0.91 |
Singh, 2015 | Asian | 147 | 451 | 312 | 125 | 14 | 107 | 35 | 5 | 0.32 |
Genetic Models | Fixed effect OR (95% CI), p | Random effect OR (95% CI), p | Heterogeneity p-value (Q test) | I2 (%) | Publication Bias (p of Egger's test) |
Allele Contrast (T vs. C) | 1.04 (0.92–1.17), 0.48 | 1.04 (0.88–1.24), 0.60 | 0.02 | 51.56 | 0.62 |
Dominant (TT+CT vs. CC) | 1.41 (1.20–1.65), <0.0001 | 1.02 (0.62–1.68), 0.92 | <0.0001 | 88.46 | 0.06 |
Homozygote (TT vs. CC) | 0.98 (0.75–1.29), 0.92 | 1.01 (0.66–1.51), 0.97 | 0.02 | 52.24 | 0.26 |
Co-dominant (CT vs. CC) | 1.10 (0.94–1.29), 0.21 | 1.10 (0.94–1.29), 0.21 | 0.43 | 0 | 0.48 |
Recessive (CC+CT vs. TT) | 0.94 (0.73–1.20), 0.63 | 0.97 (0.66–1.40), 0.86 | 0.02 | 52.4 | 0.28 |
Study | Ethnicity | Control | Case | Case Genotype |
Control Genotype |
HWE p-value of controls | ||||
CC | CT | TT | CC | CT | TT | |||||
Bonsch, 2006 | Caucasian | 115 | 134 | 64 | 56 | 14 | 60 | 41 | 14 | 0.10 |
Lutz, 2006 | Caucasian | 102 | 221 | 95 | 94 | 32 | 53 | 41 | 8 | 0.98 |
Lutz, 2007 | Caucasian | 101 | 142 | 65 | 58 | 19 | 53 | 40 | 8 | 0.90 |
Saffroy, 2008 | Caucasian | 93 | 242 | 108 | 113 | 21 | 35 | 41 | 17 | 0.41 |
Benyamina, 2009 | Caucasian | 93 | 120 | 56 | 53 | 11 | 35 | 41 | 17 | 0.41 |
Fabris, 2009 | Caucasian | 236 | 63 | 17 | 35 | 11 | 69 | 113 | 54 | 0.55 |
Shin, 2010 | Asian | 232 | 68 | 11 | 39 | 18 | 42 | 129 | 61 | 0.07 |
Supic, 2010, Heavy Alcoholic | Caucasian | 57 | 32 | 13 | 9 | 10 | 27 | 24 | 6 | 0.84 |
Supic, 2010, Non heavy Alcoholic | Caucasian | 105 | 64 | 37 | 23 | 4 | 53 | 42 | 10 | 0.69 |
Singh, 2014 | Asian | 313 | 139 | 91 | 44 | 4 | 228 | 78 | 7 | 0.91 |
Singh, 2015 | Asian | 147 | 451 | 312 | 125 | 14 | 107 | 35 | 5 | 0.32 |
Genetic Models | Fixed effect OR (95% CI), p | Random effect OR (95% CI), p | Heterogeneity p-value (Q test) | I2 (%) | Publication Bias (p of Egger's test) |
Allele Contrast (T vs. C) | 1.04 (0.92–1.17), 0.48 | 1.04 (0.88–1.24), 0.60 | 0.02 | 51.56 | 0.62 |
Dominant (TT+CT vs. CC) | 1.41 (1.20–1.65), <0.0001 | 1.02 (0.62–1.68), 0.92 | <0.0001 | 88.46 | 0.06 |
Homozygote (TT vs. CC) | 0.98 (0.75–1.29), 0.92 | 1.01 (0.66–1.51), 0.97 | 0.02 | 52.24 | 0.26 |
Co-dominant (CT vs. CC) | 1.10 (0.94–1.29), 0.21 | 1.10 (0.94–1.29), 0.21 | 0.43 | 0 | 0.48 |
Recessive (CC+CT vs. TT) | 0.94 (0.73–1.20), 0.63 | 0.97 (0.66–1.40), 0.86 | 0.02 | 52.4 | 0.28 |