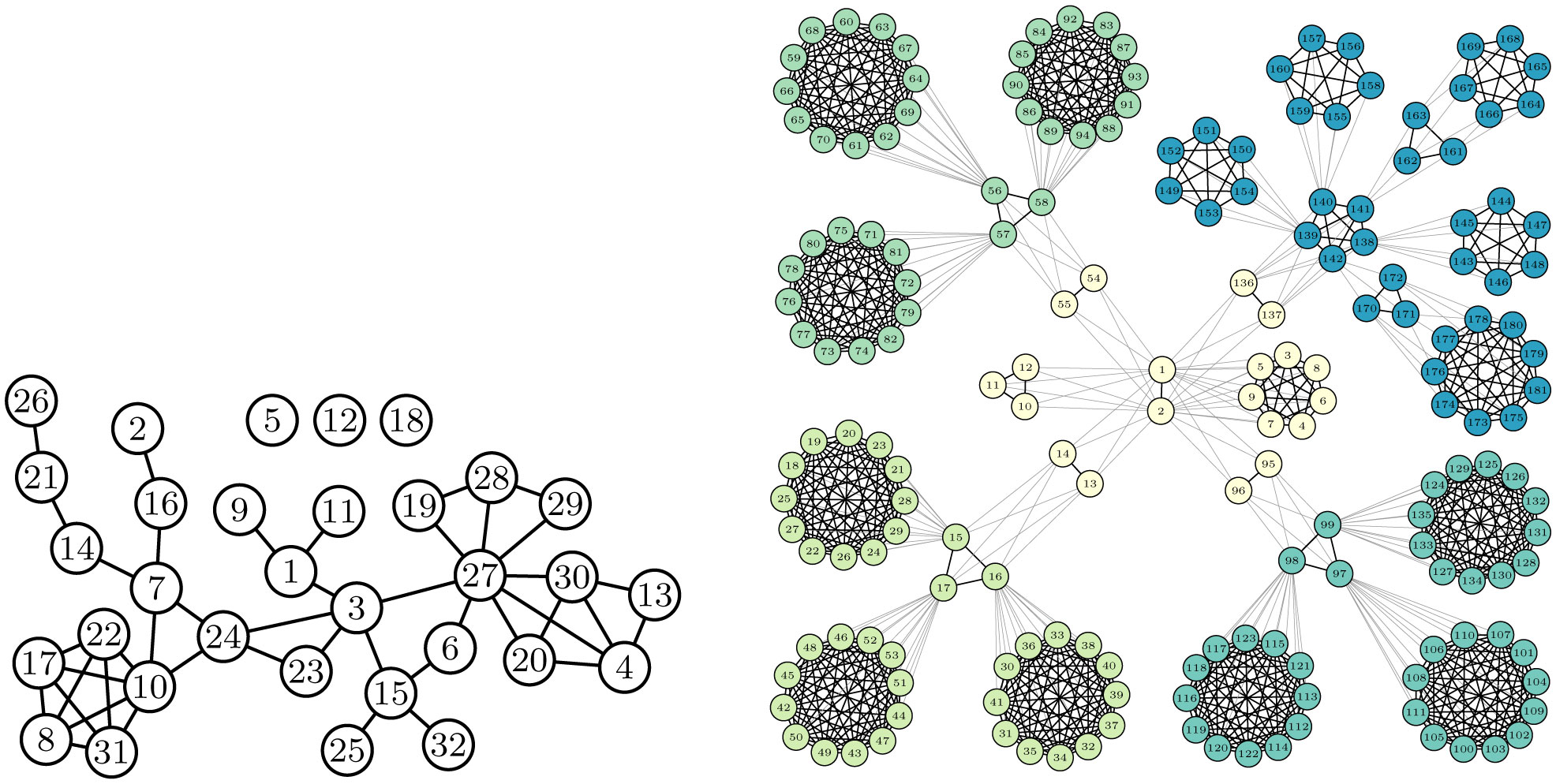
The modelling of epidemic spreading is essential in understanding the mechanisms of outbreaks and pandemics. Many models for different kinds of spreading have been proposed throughout the history of modelling, each suited for a specific scenario and parameters. On the other hand, models of information networks provide important tools for the analysis of the performance and reliability of such networks. We have previously presented a model for simulating the spreading of infectious disease throughout a social network and another one for simulating the connectivity of data traffic in an information network. We argue that these models are similar in that they produce equivalent results with appropriate parameters when run on the same network. We explain this by reasoning that the manners in which the models carry out their calculations, although devised from different assumptions, turn out to be equivalent. We also show empirical results of applying the models to calculate the spread of contagion and information connectivity on two complex networks suitable for the models. Based on the results, we calculate centrality metrics reflecting the outcome of the application, highlighting its important properties. We note that the centrality values obtained by running the epidemic model and the connectivity model turn out to be mutually equivalent, as predicted by their similar fashions of calculation. As the models were independently developed for their own applications, the equivalence in their calculation can not be explained by the models purposefully built similarly. Thus, not only are the two apparently completely separate areas of interest analysable with a single model but there appear to be inherent similarities in the mechanisms of epidemic spreading and determining network connectivity.
Citation: Into Almiala, Vesa Kuikka. Similarity of epidemic spreading and information network connectivity mechanisms demonstrated by analysis of two probabilistic models[J]. AIMS Biophysics, 2023, 10(2): 173-183. doi: 10.3934/biophy.2023011
[1] | Muhammad Asif Zahoor Raja, Adeeba Haider, Kottakkaran Sooppy Nisar, Muhammad Shoaib . Intelligent computing knacks for infected media and time delay impacts on dynamical behaviors and control measures of rumor-spreading model. AIMS Biophysics, 2024, 11(1): 1-17. doi: 10.3934/biophy.2024001 |
[2] | Yong Zhou, Yiming Ding . Finding travel proportion under COVID-19. AIMS Biophysics, 2022, 9(3): 235-245. doi: 10.3934/biophy.2022020 |
[3] | C. Dal Lin, M. Falanga, E. De Lauro, S. De Martino, G. Vitiello . Biochemical and biophysical mechanisms underlying the heart and the brain dialog. AIMS Biophysics, 2021, 8(1): 1-33. doi: 10.3934/biophy.2021001 |
[4] | Kieran Greer . New ideas for brain modelling 6. AIMS Biophysics, 2020, 7(4): 308-322. doi: 10.3934/biophy.2020022 |
[5] | Mati ur Rahman, Mehmet Yavuz, Muhammad Arfan, Adnan Sami . Theoretical and numerical investigation of a modified ABC fractional operator for the spread of polio under the effect of vaccination. AIMS Biophysics, 2024, 11(1): 97-120. doi: 10.3934/biophy.2024007 |
[6] | Carlo Bianca . Interplay and multiscale modeling of complex biological systems. AIMS Biophysics, 2022, 9(1): 56-60. doi: 10.3934/biophy.2022005 |
[7] | Dafina Xhako, Niko Hyka, Elda Spahiu, Suela Hoxhaj . Medical image analysis using deep learning algorithms (DLA). AIMS Biophysics, 2025, 12(2): 121-143. doi: 10.3934/biophy.2025008 |
[8] | Alexander G. Volkov, Yuri B. Shtessel . Propagation of electrotonic potentials in plants: Experimental study and mathematical modeling. AIMS Biophysics, 2016, 3(3): 358-379. doi: 10.3934/biophy.2016.3.358 |
[9] | Omar El Deeb, Joseph El Khoury Edde . COVID19 vaccines as boosters or first doses: simulating scenarios to minimize infections and deaths. AIMS Biophysics, 2024, 11(2): 239-254. doi: 10.3934/biophy.2024014 |
[10] | Natarajan Mala, Arumugam Vinodkumar, Jehad Alzabut . Passivity analysis for Markovian jumping neutral type neural networks with leakage and mode-dependent delay. AIMS Biophysics, 2023, 10(2): 184-204. doi: 10.3934/biophy.2023012 |
The modelling of epidemic spreading is essential in understanding the mechanisms of outbreaks and pandemics. Many models for different kinds of spreading have been proposed throughout the history of modelling, each suited for a specific scenario and parameters. On the other hand, models of information networks provide important tools for the analysis of the performance and reliability of such networks. We have previously presented a model for simulating the spreading of infectious disease throughout a social network and another one for simulating the connectivity of data traffic in an information network. We argue that these models are similar in that they produce equivalent results with appropriate parameters when run on the same network. We explain this by reasoning that the manners in which the models carry out their calculations, although devised from different assumptions, turn out to be equivalent. We also show empirical results of applying the models to calculate the spread of contagion and information connectivity on two complex networks suitable for the models. Based on the results, we calculate centrality metrics reflecting the outcome of the application, highlighting its important properties. We note that the centrality values obtained by running the epidemic model and the connectivity model turn out to be mutually equivalent, as predicted by their similar fashions of calculation. As the models were independently developed for their own applications, the equivalence in their calculation can not be explained by the models purposefully built similarly. Thus, not only are the two apparently completely separate areas of interest analysable with a single model but there appear to be inherent similarities in the mechanisms of epidemic spreading and determining network connectivity.
Modelling epidemic spreading is a central topic in the research of both contagion spreading and network science [1]. With the gravity of the impact on global health and economy of the recent COVID-19 pandemic [2], the field has gained an increasing amount of attention. Accordingly, models of epidemic spreading have been of great focus with new models constantly being proposed and built upon earlier ones. One approach to such models is compartmental models that divide the population into compartments based on their current role in the spreading process [3]. A simple example of a compartmental model is the SIR model which classifies each individual as either susceptible, infected, or recovered. Once recovered, the infection can no longer be transmitted to the recovered individual. The SIR model is well-studied and has been applied to complex networks in the literature [4]–[6]. Other types of compartmental models include SIS, SIRS, and SEIR, [3] in addition to more complex ones [7]. Models of contagion spreading can be used to quantitatively study effective ways of epidemic prevention [8], [9].
In this article, we highlight two models for different applications introduced in our earlier articles [9]–[11] and explain their equivalent results even when applied to each other's use cases. Both of the models are based on similar foundations for calculating the probabilities of spreading or connections between nodes in a network [12], where methods of probabilistic networks [13] are applied.
As mentioned, the models (referred to as the Spreading model and the Connectivity model) are designed for two separate applications. While the Spreading model is built for epidemic and behaviour spreading [10], the Connectivity model is constructed to simulate the reliability of connectivity in a service network [11]. Since the models are built for different use cases, we will introduce general vocabulary to talk about both models more easily. We call influence spreading the propagation of contagion, information, or a similar phenomenon through nodes of a network. The interpretation of contagion as influence spreading is rather natural: contagion spreads from one individual to another when they are in contact and, through these contacts, continues to spread further. Connectivity in information networks can also be thought of as information spreading through nodes and connections of the network. Where information can be transferred, a connection exists. Neither model simulates the spreading process through time—instead, only the final state of the spreading is calculated.
As the models produce equivalent results, they can both be applied to epidemic spreading. In particular, they are applicable to a situation where individuals gain full immunity to a disease after contagion, as a result of the models' principle to spread influence to a node only once, similarly to the SIR model [3]. In the case of information network connectivity, immunity can be equated to the inactivity of nodes in the network. Diseases resulting in full or near full immunisation include measles, mumps, rubella, and chickenpox [14], though many infections give no or only partial immunity. We will propose a model for partial immunity epidemic spreading in a future study of ours.
The Spreading model is built to be extendable and has the potential for additional parameters that the Connectivity model lacks. For example, parameters such as maximum spreading path length (Lmax) and breakthrough probability can be taken into account. This enables the calculation of novel forms of connectivity, based not only on the structure of the network but also on other possible phenomena arising from the new parameters.
We compare two simulation models built for two use cases: the epidemic spreading simulation model, and the connection reliability model. Both models consider a network as a set of weighted nodes and weighted directed edges between them. Undirected edges are modelled as two identical edges with the endpoints swapped. The output of the models is the two-dimensional probability matrix
The probability matrix C(s, t) produced by both of the models gives the conditional probabilities of influence spreading from node s to node t given that influence starts to spread from node s. If the probabilities, at which the nodes initially start to spread influence are known, the conditional probabilities of the probability matrix can be multiplied by them, producing unconditional probabilities.
The Spreading model works by simulating the spreading of influence from each node to the rest of the network separately. For each node as the source, the simulation is carried out a certain number of times: this is called the number of iterations. The probability of influence spreading to another node is calculated as the number of iterations where the node was influenced divided by the total number of iterations. The simulation itself works in steps, on which all newly influenced nodes (that is, nodes that became influenced on the step right before the current one) attempt to spread influence to all of their neighbouring nodes. This attempt automatically fails if the neighbouring node has been previously influenced. Otherwise, the spreading will succeed with a probability of we · Wt, where we is the weight of the edge connecting the nodes and Wt is the weight of the target node. If the spreading succeeds, the target node will be marked as influenced and will attempt to spread influence in the subsequent step. Another parameter, Lmax, is used to limit the maximum spreading path length: influence will only spread along paths at maximum Lmax edges long. Limiting Lmax can be used to shorten the execution times of the model at the cost of less precise results or to model a situation where spreading paths are limited by some factor, such as cutting spreading chains as a preventive measure against an epidemic. A more detailed description and pseudocode for the Simulation model are provided in our earlier study [10].
The calculation in the Connectivity model works similarly in that it simulates the connectivity multiple times, taking the average results. Instead of simulating the influence spreading from each node separately, for each iteration, the set of active nodes and edges is randomly determined: the probability at which each node and edge is active is its weight. For each active node, the nodes that can be reached by paths consisting of active nodes and edges are considered connected to it. C(s, t) is then the number of iterations where the active node s had an active path to node t divided by the total number of iterations. Unlike the Spreading model, the Connectivity model does not have the Lmax parameter, as the connectivity is not determined by stepping along paths. A more detailed description and pseudocode for the Connectivity model is provided in our earlier study [11].
For both models, the probability of influence spreading through a path with a specified starting node is the product of the weights of each edge and node on the path, excluding that of the starting node. In other words,
where P(ℒ) is the probability of spreading through the path ℒ of m edges and nodes excluding the starting node, and
As the probability of influence spreading through a path is given by the same equation for both of the models, the models' results should theoretically be equivalent. This equivalence only holds, however, when Lmax is not capped to some number in the Spreading model, in order to take into account all possible paths in the network as the Connectivity model does.
The probability matrix that both of the models generate can be used for various applications, as covered in our previous articles [9], [10]. At the core of these applications are different centrality measures that reflect how central each edge and node in the network is. These measures give insight into the structure of the network, helping to understand patterns and phenomena present therein. We give three examples of centrality measures: the in- and out-centralities and the betweenness centrality.
The in- and out-centrality measures are a natural way to approach node centrality [10]. They represent how much influence flows in and out of the node, respectively. We define the in-centrality of node t as
and, similarly, the out-centrality of node s as
where V is the set of nodes in the network [10].
In other words, the in-centrality of a node is the sum of the probabilities of spreading from all other nodes to the node in question, and the out-centrality of a node is the sum of the probabilities of spreading from the node in question to all other nodes. The in- and out-centralities directly translate to physical quantities of the network. As C(s, t) is the probability of influence spreading from node s to node t, the sum can be thought of as an expected value. In the case of in-centrality (Eq. 2.2), the sum represents the expected number of nodes that will spread influence to the specified node, and, for the out-centrality (Eq. 2.3), the sum represents the expected number of nodes that influence from the specified node will spread to. As a concrete example, the out-centrality of a node represents the expected number of infected people in a social network, when a contagious infection begins to spread from the starting node, and the in-centrality of an individual's vulnerability to being infected by different sources.
The betweenness centrality measure is another approach to studying node centrality. It represents the significance of a node in transmitting influence between different parts of the network. Betweenness centrality can be easily defined for a set of nodes S, where the betweenness centrality of a single node s can be expressed as that for the set {s}. We first define the cohesion of a network as
and the partial cohesion of the network without the set of nodes S as
where V is the set of nodes in the network and CS is the probability matrix calculated with only nodes and edges between nodes in S taken into account. The probability matrix for partial cohesion has to be calculated independently from that for total cohesion, as the effects of removing nodes and edges can cut off paths between parts of the network and change the spreading probabilities.
With Eqs. 2.4 and 2.5, we define the betweenness centrality as the relative difference between the total and partial cohesion:
The cohesion portrays the total interconnectivity of the network. As the betweenness centrality is a relative difference in the cohesion (Eq. 2.6), the larger the betweenness centrality, the greater the effect of removing the specified nodes is on the interconnectivity. The betweenness centrality can be used to spot individuals who act in bridging roles between parts of the network, the isolation of which can help to contain the contagion to only a small part of the network.
To compare the models, we run them on two networks, the Student network [15] and the Organisation network [9] (Figure 1a,b). Precise descriptions of how the calculations in the models are carried out are presented in our earlier studies [9], [10]. The Student network is a small, 32-node network composed of empirical data on the relationships of Dutch university students [15]. We consider the network with all edge weights set to 0.5. The Organisation network, on the other hand, is a larger, 181-node network that represents a real-world organisation structure. The network consists of five departments with multiple groups forming each of them as well as hierarchical leadership relations. The Organisation network was first introduced in [9], along with different classes of preventive measures that simulate epidemic prevention by decreasing the weights of certain edges. In this article, we study the case where preventive measures are in use on all edges except on those representing leadership relations, which means that only edges representing leadership and group relations are present. Edges representing group relations have a weight of 0.5, and edges representing leadership relations have a weight of 0.3. Both of the networks are considered to be undirected with node weights equal to 1. The models work and give equivalent results for directed networks with varying node weights as well. Further study into the performance of the models and their application to other networks is presented in our earlier studies [9]–[11].
As both of the networks are real-life social networks, they are well suited for modelling epidemic spreading. Using the two networks, we can compare the models on networks exhibiting different properties: the Student network is small and sparse, whereas the Organisation network is larger and much denser. Together, the networks represent a multitude of different situations for our models to perform on. It is worth noting, however, that as the edges of the networks are undirected, the in- and out-centrality (Eqs. 2.2 and 2.3) for a node will always be equal due to the symmetry of spreading from and to the node. This is not the case for scenarios where a node can be influenced more than once, such as an epidemic of an infectious disease, the contraction of which does not lead to full immunity [2]. In practice, the probability of an infection spreading from one person to another is often different than the probability of spreading in the other direction, represented by different weights in the directed edges between them.
The models calculate the probabilities of spreading between all pairs of nodes given the layout and weight parameters of the network. The results can be used to calculate important quantities, specific to the network and its weights. One example of such quantity is the basic reproduction number for epidemics, which is a measure of contagiousness defined as the number of new infections a single infection on average leads to [16]. We have calculated the basic reproduction number for simulations run on the Organisation network as a function of edge weights in our previous study [9]. It is important to note that quantities such as the basic reproduction number are not specific to the models and must be independently calculated for any network and parameters that the models are run on. As each set of parameters and the input network define a unique spreading scenario, measures such as the basic reproduction number can always be calculated for any combination thereof. An example of such a calculation is provided in [9].
For both networks, we calculate the total cohesion (Eq. 2.4) and mean betweenness centrality (Eq. 2.6) using both models and varying Lmax for the Spreading model. We normalise the total cohesion by the number of values constituting its sum, N(N – 1), where N is the number of nodes in the network, to get the node-wise average in- and out-centrality. This scaling factor is attained from the probability matrix being of shape N × N with the diagonal consisting of N missing values. The sum of the in- and out-centralities (Eqs. 2.2 and 2.3) are equal to the cohesion:
From Eq. 3.1, the averages of the in- and out-centralities are also equal. This means that the normalised cohesion represents both the average node-wise in- and out-centralities.
The mean betweenness centrality is calculated as the average of the node-wise betweenness centralities. The minimum Lmax value, for which the results of the models are ideally equal (assuming arbitrary precision), is the maximum length, for which a self-avoiding path exists in the network, since then the Spreading model is able to simulate spreading throughout the whole network. A self-avoiding path is never longer than the number of nodes in the network, which gives a trivial upper bound. Thus, as Lmax increases, the results of the Spreading model approach those of the Connectivity model. In practice, this happens with Lmax much lower than N (Figures 2 and 3). This is due to the probability of spreading through a path decreasing exponentially with the path length.
The cohesions of the Student and Organisation networks are around 19% and 40%, respectively (Figures 2a and 3a). The Organisation network achieves a higher cohesion due to its much denser nature. The difference in density can also be seen in Figures 2b and 3b, where the Student and Organisation networks' betweenness centralities (Eq. 2.6) are around 0.12 and 0.03, respectively. Nodes in the Student network are connected by much fewer paths than in the Organisation network, and therefore each node has a more prominent role in allowing connections between other nodes.
As the results given by the Spreading model converge already with lower values of Lmax, epidemic simulation can be performed more efficiently by not taking longer spreading paths into account. With a low Lmax, however, the results differ, which allows the simulation of scenarios where spreading paths are limited by factors such as consistent isolation of patients. Since the two models produce identical results with high Lmax, both models can be used for the intended purpose of the other. The equivalence of the results indicates the similarities in the mechanisms of epidemic spreading [9] and information network connectivity [11]: where contagion spreads from node to node with immunity inhibiting it in epidemic spreading, in connectivity, information has to similarly travel from node to node with inactive nodes as a limiting factor. These similarities in the mechanisms might extend to other types of network modelling, which is a subject for future research.
The models can be run on any network with any edge and node weights but are capable of modelling only scenarios where any one node is influenced at most once. In our earlier studies, we have also presented a model where all nodes can get influenced any number of times. We will present a model capable of dealing with situations where getting influenced decreases a node's probability of getting influenced again by a given breakthrough probability in our future studies.
Modelling breakthrough influence is an example of how the models can be applied to the purposes of each other. Namely, many epidemics [9] and influence spreading [10] processes are based on similar spreading mechanisms. Both processes can have significant breakthrough probabilities depending on the specific characteristics of virus species or information types. For example, COVID-19 variants [2] can have different breakthrough probabilities despite being very close in nature. Rumours and propaganda have a higher breakthrough probability, as they circulate between people and change form more effectively than facts and knowledge.
We have compared the calculation methods and results of two models designed for epidemic spreading and connectivity simulation introduced in our earlier research. We call these models the Spreading model and Connectivity model, respectively. Even though the models are designed for very different applications, the manner in which they calculate their results is mathematically equivalent. Accordingly, the two models produce equivalent results with high enough spreading path length, Lmax, for the Spreading model. As the models were independently developed for their different purposes, their equivalence highlights the similarities in the mechanisms of epidemic spreading and information network connectivity. The similar results also enable the application of the models to each others' intended purposes, allowing different parameters to be taken into account.
In this study, we have highlighted the opportunities for using interdisciplinary modelling and simulation methods in the research of epidemic spreading, resilience in communication networks, and influence spreading in social networks.
[1] | (2005) National Research CouncilNetwork Science. Washington: The National Academies Press. https://doi.org/10.17226/11516 |
[2] |
Hu B, Guo H, Zhou P, et al. (2021) Characteristics of SARS-CoV-2 and COVID-19. Nat Rev Microbiol 19: 141-154. https://doi.org/10.1038/s41579-020-00459-7 ![]() |
[3] |
Pastor-Satorras R, Castellano C, van Mieghem P, et al. (2015) Epidemic processes in complex networks. Rev Mod Phys 87: 925-979. https://doi.org/10.1103/RevModPhys.87.925 ![]() |
[4] |
Valdez LD, Braunstein LA, Havlin S (2020) Epidemic spreading on modular networks: the fear to declare a pandemic. Phys Rev E 101: 032309. https://doi.org/10.1103/PhysRevE.101.032309 ![]() |
[5] |
de Arruda GF, Rodrigues FA, Moreno Y (2018) Fundamentals of spreading processes in single and multilayer complex networks. Phys Rep 756: 1-59. https://doi.org/10.1016/j.physrep.2018.06.007 ![]() |
[6] | Nowzari C, Preciado VM, Pappas GJ (2016) Analysis and control of epidemics: a survey of spreading processes on complex networks. IEEE Contr Syst Mag 36: 26-46. https://doi.org/10.1109/MCS.2015.2495000 |
[7] | Arenas Alex, Cota W, Gómez-Gardeñes J, et al. (2020) Derivation of the effective reproduction number ℛ for COVID-19 in relation to mobility restrictions and confinement. medRxiv . https://doi.org/10.1101/2020.04.06.20054320 |
[8] |
Matamalas JT, Arenas A, Gómez S (2018) Effective approach to epidemic containment using link equations in complex networks. Sci Adv 4: eaau4212. https://doi.org/10.1126/sciadv.aau4212 ![]() |
[9] |
Kuikka V (2022) Modelling epidemic spreading in structured organisations. Physica A 592: 126875. https://doi.org/10.1016/j.physa.2022.126875 ![]() |
[10] | Kuikka V, Pham MAA (2022) Models of influence spreading on social networks. Complex Networks & Their Applications X. Cham: Springer International Publishing 112-123. https://doi.org/10.1007/978-3-030-93413-2_10 |
[11] | Kuikka V, Syrjänen M (2019) Modelling utility of networked services in military environments. In: 2019 International Conference on Military Communications and Information Systems (ICMCIS) . https://doi.org/10.1109/ICMCIS.2019.8842757 |
[12] |
Ball MO, Colbourn CJ, Rovan JS (1995) Chapter 11 Network Reliability. Handbooks in Operations Research and Management Science.Elsevier 673-762. https://doi.org/10.1016/S0927-0507(05)80128-8 ![]() |
[13] |
Interdonato R, Atzmueller M, Gaito S, et al. (2019) Feature-rich networks: going beyond complex network topologies. Appl Netw Sci 2019: 4. https://doi.org/10.1007/s41109-019-0111-x ![]() |
[14] |
Amanna IJ, Carlson NE, Slifka MK (2007) Duration of humoral immunity to common viral and vaccine antigens. New Engl J Med 357: 1903-1915. https://doi.org/10.1056/NEJMoa066092 ![]() |
[15] | van de Bunt GG (1999) Friends by choice. An actor-oriented statistical network model for friendship networks through time. University of Groningen, Thela Thesis . |
[16] |
Delamater PL, Street EJ, Leslie TF, et al. (2019) Complexity of the basic reproduction number (R0). Emerg Infect Dis 25: 1-4. https://doi.org/10.3201/eid2501.171901 ![]() |
1. | Into Almiala, Henrik Aalto, Vesa Kuikka, Influence spreading model for partial breakthrough effects on complex networks, 2023, 630, 03784371, 129244, 10.1016/j.physa.2023.129244 | |
2. | Vesa Kuikka, Detecting Overlapping Communities Based on Influence-Spreading Matrix and Local Maxima of a Quality Function, 2024, 12, 2079-3197, 85, 10.3390/computation12040085 | |
3. | Vesa Kuikka, Kimmo K. Kaski, Detailed-level modelling of influence spreading on complex networks, 2024, 14, 2045-2322, 10.1038/s41598-024-79182-9 | |
4. | Vesa Kuikka, Opinion Formation on Social Networks—The Effects of Recurrent and Circular Influence, 2023, 11, 2079-3197, 103, 10.3390/computation11050103 |