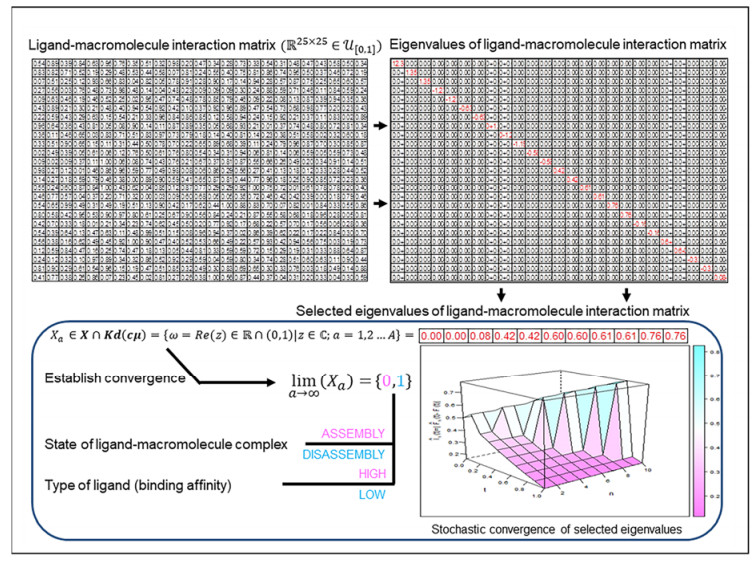
The objectives of this study were to assess the microbial quality of honey, evaluate the effect of microwaves on microbial survival, and assess the antibacterial activity of honey. Bacteria, yeast and mold were evaluated in samples before and after microwave treatment. Dominant bacterial contaminants were also identified. The antibacterial activity of honey was assessed against nine pathogens using an agar well diffusion assay. The minimum inhibitory concentration was determined for four honey samples that exhibited the highest antibacterial activity. In addition, one sample of Manuka honey was tested to compare its microbial load as well as its antibacterial activity to local honey samples. Sequencing using 16S rRNA gene was used for the identification of dominant bacteria. The average standard plate count, yeasts and molds were 286.5,161.0 and 25.5 CFU/g, respectively. Microwave treatment decreased microbial populations gradually with increasing power levels and exposure times. The present study indicated that raw honey had a significant antibacterial activity which decreased following microwave treatment. The identity of 125 isolates was confirmed with Bacillus being most frequently isolated.
Citation: Ziad Jaradat, Batool Khataybeh, Abdull Majid Al Ghzawi, Qutaiba Ababneh, Anas Al Nabusli. Molecular identification of major bacteria in honey and the effect of microwave treatment on its microbial quality and antibacterial activity[J]. AIMS Agriculture and Food, 2022, 7(3): 594-613. doi: 10.3934/agrfood.2022037
[1] | Christian Winkel, Simon Neumann, Christina Surulescu, Peter Scheurich . A minimal mathematical model for the initial molecular interactions of death receptor signalling. Mathematical Biosciences and Engineering, 2012, 9(3): 663-683. doi: 10.3934/mbe.2012.9.663 |
[2] | Hong Yuan, Jing Huang, Jin Li . Protein-ligand binding affinity prediction model based on graph attention network. Mathematical Biosciences and Engineering, 2021, 18(6): 9148-9162. doi: 10.3934/mbe.2021451 |
[3] | Yuewu Liu, Mengfang Zeng, Shengyong Liu, Chun Li . Dynamics analysis of building block synthesis reactions for virus assembly in vitro. Mathematical Biosciences and Engineering, 2023, 20(2): 4082-4102. doi: 10.3934/mbe.2023191 |
[4] | Ronald Lai, Trachette L. Jackson . A Mathematical Model of Receptor-Mediated Apoptosis: Dying to Know Why FasL is a Trimer. Mathematical Biosciences and Engineering, 2004, 1(2): 325-338. doi: 10.3934/mbe.2004.1.325 |
[5] | Max-Olivier Hongler, Roger Filliger, Olivier Gallay . Local versus nonlocal barycentric interactions in 1D agent dynamics. Mathematical Biosciences and Engineering, 2014, 11(2): 303-315. doi: 10.3934/mbe.2014.11.303 |
[6] | Feng Rao, Carlos Castillo-Chavez, Yun Kang . Dynamics of a stochastic delayed Harrison-type predation model: Effects of delay and stochastic components. Mathematical Biosciences and Engineering, 2018, 15(6): 1401-1423. doi: 10.3934/mbe.2018064 |
[7] | Yutong Man, Guangming Liu, Kuo Yang, Xuezhong Zhou . SNFM: A semi-supervised NMF algorithm for detecting biological functional modules. Mathematical Biosciences and Engineering, 2019, 16(4): 1933-1948. doi: 10.3934/mbe.2019094 |
[8] | O. E. Adebayo, S. Urcun, G. Rolin, S. P. A. Bordas, D. Trucu, R. Eftimie . Mathematical investigation of normal and abnormal wound healing dynamics: local and non-local models. Mathematical Biosciences and Engineering, 2023, 20(9): 17446-17498. doi: 10.3934/mbe.2023776 |
[9] | Zhenzhen Zheng, Ching-Shan Chou, Tau-Mu Yi, Qing Nie . Mathematical analysis of steady-state solutions in compartment and continuum models of cell polarization. Mathematical Biosciences and Engineering, 2011, 8(4): 1135-1168. doi: 10.3934/mbe.2011.8.1135 |
[10] | Linlu Song, Shangbo Ning, Jinxuan Hou, Yunjie Zhao . Performance of protein-ligand docking with CDK4/6 inhibitors: a case study. Mathematical Biosciences and Engineering, 2021, 18(1): 456-470. doi: 10.3934/mbe.2021025 |
The objectives of this study were to assess the microbial quality of honey, evaluate the effect of microwaves on microbial survival, and assess the antibacterial activity of honey. Bacteria, yeast and mold were evaluated in samples before and after microwave treatment. Dominant bacterial contaminants were also identified. The antibacterial activity of honey was assessed against nine pathogens using an agar well diffusion assay. The minimum inhibitory concentration was determined for four honey samples that exhibited the highest antibacterial activity. In addition, one sample of Manuka honey was tested to compare its microbial load as well as its antibacterial activity to local honey samples. Sequencing using 16S rRNA gene was used for the identification of dominant bacteria. The average standard plate count, yeasts and molds were 286.5,161.0 and 25.5 CFU/g, respectively. Microwave treatment decreased microbial populations gradually with increasing power levels and exposure times. The present study indicated that raw honey had a significant antibacterial activity which decreased following microwave treatment. The identity of 125 isolates was confirmed with Bacillus being most frequently isolated.
Ligands, are biochemical modifiers of macromolecular structure and can impact biological function. These can be co-factors (iron, cobalt, copper, zinc), co-enzymes such as Nicotinamide- and Flavin- Adenine Dinucleotides (NAD, FAD) and full-length molecules with short binding sites [1,2]. Ligands, unlike substrates/co-substrates are either reversibly altered or not at all. The biochemical role of ligands, in vivo, is complex and can influence both, enzyme-mediated substrate catalysis and non-enzymatic association and dissociation interactions. Whilst, the interaction with competitive inhibitors, co-factors or co-enzymes involves definitive and direct modifications to the active site residues, the effect of a ligand can be allosteric and indirect [3,4,5]. The latter involves both long-distance conformational changes and non-covalent interactions (hydrogen, Van der Waals, hydrophobic, electrostatic) [6,7,8,9,10,11]. Empirical data suggests that the binding affinity or the strength-of-association of a macromolecule for its ligand is a critical determinant of function [7,8,9,10,11]. For example, 2, 3-Bisphophoglycerate is a potent modifier of Hemoglobin function and does so by shifting the oxygen-dissociation curve to the right. In its absence Hemoglobin retains high affinity for molecular oxygen (left-shift of the oxygen dissociation curve), an undesirable effect on its role as a transporter [10,11]. Similarly, Ascorbic acid maintains iron in its reduced state in the gastrointestinal tract and as part of the active site of non-haem iron (Ⅱ)- and 2-oxoglutarate-dependent dioxygenases [12,13]. Deficiency of Ascorbic acid is implicated in tardy iron absorption in the ileum as well as a range of collagen disorders such as scurvy (Prolyl- and Lysyl-hydroxylases) [14,15]. Conversely, proteins which are modified such as those that may originate from missense or amino acid substitutions and are secondary to genomic variants such as single nucleotide polymorphisms (SNPs) and insertions-deletions (indels), will also result in several clinical outcomes [16,17]. Here, too, enzyme catalysis is directly affected if these are present at the active site or is impacted indirectly (folding, stability, complex formation) when present elsewhere [16,17,18].
There is a large volume of literature which describes macromolecules in terms of either residues (amino acids, nucleotides) interacting or an all atom-based interaction matrix. The proponent of the 2D approach is the Gaussian network model (GNM), while the Anisotropic network model (ANM) is representative of the 3D approach [19,20,21,22]. The fundamental premise of both these approaches is the elastic network model (ENM) [19]. Here, an atom or residue is modeled as an elastic mass and the interaction between a pair of atoms/residues is dependent on the selection of a pre-determined cut-off distance [22]. The force constant, although, not a parameter by definition, has also been studied and shows good correlation with B-factor data [23]. A major application of these studies is normal mode analysis (NMA), which has been used to glean valuable insights into the structural dynamics of the investigated macromolecule and into B-factor distribution [23,24]. Despite this success, there are significant limitations of this approach, including inadequate descriptors for the type of interactions computed by the Hessian matrix and data points that are dependent on a preselected cut-off distance (5–10 Ang, GNM; 10–15 Ang, ANM) [20,22,25]. Parameter-free versions of the ENM (pfENM), GNM (pfGNM) and ANM (pfANM), to resolve the latter, have been described and compared to establish B-factor distribution (isotropic, anisotropic) [25]. Additionally, many of these studies have focused on inferring biophysical characteristics such as cross-correlational fluctuations and mean square displacements. From a functional standpoint, however, it is not clear whether these data can be utilized to derive/study parameters such as the Michaelis-Menten constant (Km) or the association/dissociation (Ka/Kd). Since, these depend on the presence of an organic or inorganic modifier, i.e., ligand/substrate/co-factor/co-substrate, in addition to the modeled macromolecule, its exclusion is another major lacuna of these studies.
Despite the availability of clinical, empirical, analytical and computational data, a mathematically rigorous explanation for the heterogeneity in biochemical function, for a ligand-macromolecular complex, is missing. The work presented models a ligand and macromolecule as a homo- or hetero-dimer and subsumes a finite and equal number of atoms/residues per monomer. The pairwise interactions of the resulting square matrix will be chosen randomly from a standard uniform distribution. The resulting eigenvalues will be analyzed and modeled in accordance with known literature on biochemical reactions to generate biologically viable and usable dissociation constants. The theoretical results will be complemented by numerical studies where applicable. Additionally, and through various theorems, lemmas and corollaries, a schema to partition ligands into high- and low-affinity variants will also be discussed. The suitability of the transition-state dissociation constants as a model for ligand-macromolecular interactions will be inferentially assessed by analyzing the clinical outcomes of amino acid substitutions of selected enzyme homodimers. The relevance of the model to biochemical function will be discussed by examining the ligand-macromolecular complex for known ligands of non-haem iron (Ⅱ)- and 2-oxoglutarate-dependent dioxygenases (Fe2OG) and the major histocompatibility complex (Ⅰ) (MHC1).
The general outline of the manuscript includes an initial section where the system to be modeled, rationale for this study, and formal definitions are introduced (Section 2). The model is analyzed, formulated and presented as theorems, lemmas and corollaries (Section 3). This section also includes a numerical study to demonstrate and validate the theoretical assertions made. The biological relevance of these findings are discussed with an analysis of clinical outcomes of enzyme sequence variants and case studies of enzyme- and non-enzymatic complex formation (Section 4). A brief conclusion that summarizes the presented study, limitations and future directions is included at the end of the manuscript (Section 5). Details of all proofs are included after the conclusions (Section 6).
Consider the generic interaction between macromolecule (c) and ligand (μ),
cμrf→←rbc+μRXN(1) |
We can represent this interaction/reaction, at a steady state, with the rate equations [26],
Rd(cμ)=rf.[cμ]Lcμ≥0 | (1) |
Ra(cμ)=rb.[c]Lc≥0[μ]Lμ≥0 | (2) |
At steady state,
Rd(cμ)=Ra(cμ) | (3) |
⇒rfrb=[c]Lc≥0.[μ]Lμ≥0[cμ]Lcμ≥0 | (4) |
rfrb=Kd(cμ) | (5) |
Here,
c:=Macromolecule |
μ:=Ligand |
[.]:=Molarconcentrationofreactantinstandardform(M) |
Ra(cμ):=Rateofassociationofcomplex(Ms−1) |
Rd(cμ):=Rateofdissociationofcomplex(Ms−1) |
rf:=Rateconstantsofforwardreaction(s−1) |
rb:=Rateconstantsofreversereaction(M−1s−1) |
L:=Stoichiometryofreactant(s) |
Kd(cμ):=Dissociationconstantforligand−macromolecularcomplex(M) |
It is clear that a ligand-macromolecular complex may exist in one of three distinct states. These include: a) perfect association, b) perfect dissociation and c) an intermediate- or transition-state; and can be represented in terms of the dissociation constant,
Case (1) Perfectassociation Def. (1)
1rb→∞;rf→0 | (6, 7) |
⇒Kd(cμ)≈0 | (8) |
Case (2) Perfectdisassociation Def. (2)
rf>>>rb | (9) |
⇒Kd(cμ)≥1 | (10) |
Case (3) Transient−statedisassociationconstant Def. (3)
rf≶rb | (11) |
⇒Kd(cμ)∈R∩(0,1) | (12) |
Consider an atom/residue-based representation (amino acids/nucleotides) of a generic set of monomeric macromolecules, C={protein,DNA,RNA}, with z=1,2,….,Z components each with i-indexed (i=1,2,….,I) c-atoms/residues,
c≡Cz∈C|c=[c1c2….ci=I]T,I∈N | (13) |
The analogous model of a monomer ligand, L={smallmolecule,peptide,oligonucleotide}, with j-indexed (j=1,2,…,J) μ-atoms/residues is,
μ∈L|μμ=[μ1μ2….μj=J],J∈N | (14) |
It is also assumed that the ligand-macromolecular complex is a homo- or hetero-dimer with an equal number of atoms/residues (I=J) per monomer. The interaction matrix is,
⟨c|μ⟩=[c1c2..ci=I]T×[μ1μ2..μj=J]=Czμ=(ciμj)⊂RI×J | (15) |
The numerical values of this matrix are chosen randomly from the standard uniform distribution,
Czμ=(ciμj)∈U[0,1] | (16) |
The rationale for this choice is that each pairwise interaction is subsumed to be a function of an arbitrary number of non-bonded interactions (long- and short-range) and is therefore, unique. Clearly, this implies the existence of {I,J}-linear independent vectors,
rank(Czμ)={I,J} | (17) |
Since Czμ is diagonalizable there exists a diagonal matrix, KCzμ,
KCzμ=X−1CzμX | (18) |
zi=j=z∈diag(KCzμ)⊂C | (19) |
Czμ, is non-symmetric the computed eigenvalues of the modeled ligand-macromolecular interaction matrix can have positive and negative real parts,
{αij=Re(z)∈Kd(Czμ)⊂R|i=j,z∈C} | (20) |
{αij=Re(z)∈Kd(Czμ)⊂R|i=j,z∈C} | (20.1) |
A further subdivision can be made in accordance with established literature,
{αij=Re(z)∈Kd(Czμ)⊂R|i=j,z∈C}ω∞=Kd(cμ)≥1 | (21) |
Perfectassociation≡Reverseω0=Kd(cμ)=0 | (22) |
This selection generates the set,
ω=αij∈Kd(Czμ)⊂R∩(0,1) | (23) |
ω∈Kd(cμ)Def.(4) |
#Kd(cμ)=A | (23.1) |
We can combine these to get a preliminary definition of the transition-state disassociation constants. These are the strictly positive real part of all complex eigenvalues that characterize a ligand-macromolecular complex with an equal number of atoms/residues per monomer and belong to the open interval (0, 1),
{ω=Re(z)∈Kd(cμ)⊂Kd(Czμ)⊂R∩(0,1)|z∈C} | (Def.(5a)) |
Whilst, the states of perfect association and dissociation are key determinants of whether a reaction will occur or not, the transition-state dissociation constants may offer insights into the origins of threshold values, feedback mechanisms and other regulatory checkpoints. However, in order to ascribe biological relevance to these findings we must establish various bounds which can then be utilized to assess and thence assay the function of a ligand-macromolecular complex.
Theorem 1 (T1): The linear map between the transition-state dissociation constants and the eigenvalues that characterize the interactions of the homo- or hetero-dimer form of the modeled ligand-macromolecular complex is the injection,
g:ω∈Kd(cμ)↦Kd(Czμ) | (24) |
Theorem 2 (T2): The transition-state dissociation constants that characterize the interactions of the homo- or hetero-dimer form of the modeled ligand-macromolecular complex is a monotonic and non-increasing sequence,
{ωa}a≤a+1|ω∈Kd(cμ) | (25) |
Theorem 3 (T3): The transition-state dissociation constants that characterize the interactions of the homo- or hetero-dimer form of the modeled ligand-macromolecule complex are monotonic, bounded and therefore, convergent,
lima→∞{ωa}a≤a+1={0,1}|ω∈Kd(cμ) | (26) |
Corollary 1 (C1): The transition-state dissociation constants that characterize the interactions of the homo- or hetero-dimer form of the modeled ligand-macromolecule complex is a sequence with defined greatest-lower and least-upper -bounds,
inf{ωa}a≤a+1<{ωa}a≤a+1<sup{ωa}a≤a+1 | (27) |
Corollary 2 (C2; without proof): The cardinality of the set of transition-state dissociation constants that characterize the interactions of the homo- or hetero-dimer form of the modeled ligand-macromolecule complex is finite,
#Kd(cμ)=A<#Kd(Czμ)={I,J} | (28) |
Using T1–T3 and C1, C2 we can refine our definition of the transition-state dissociation constants for the modeled ligand-macromolecular complex,
{ωa}a≤a+1|ω∈Kd(cμ);a=1,2…A | (Def.(5b)) |
where,
ω=Re(z)∈Kd(Czμ)⊂R∩(0,1)|z∈C |
It is clear from the above results that the eigenvalue-based transition-state dissociation constants are continuous and can potentially model the multiplicity of intermediate- or transient-states that a ligand-macromolecular complex may adopt. It should therefore, be possible to partition the transition-state dissociation constants into functionally distinct subsets and will be characteristic for a specific ligand-macromolecular complex.
Theorem 4 (T4): The transition-state dissociation constants that characterize the interactions of the homo- or hetero-dimer form of the modeled ligand-macromolecule complex is a proper subset of the complete set of the real part of all complex eigenvalues that comprise the interaction matrix,
Kd(cμ)⊂Kd(Czμ) | (29) |
Corollary 3 (C3): The distribution of the transition-state dissociation constants that characterize the interactions of the homo- or hetero-dimer form of the modeled ligand-macromolecule complex will result in a schema by which we can annotate the ligand as a high (μhigh)- or low (μlow)-affinity variant,
μ={μhigh,μlow} | (30) |
Biologically relevant macromolecular complexes are characterized by heterogeneity and high-order (Z≥2). This multimer-form of a macromolecule is formed around a primary molecule and its interactions. These may be protein-protein, DNA/RNA-protein or DNA-RNA-protein.
The multimer-form of ligand-macromolecular complex is easily modeled using the mathematical framework defined earlier. Here, the ligand-macromolecular complex is considered as a set of interacting monomers and the binding to a ligand occurs via a single unique monomer,
{Cz∈C|C1=C2⋯=Cz=Z|Z≥2} | (Def.(6a)) |
where,
Cz≡Czμ≡μCz | (Def.(6b)) |
Here,
μ:=Ligand |
Cz:=Uniquemonomerofamacromoleculethatassociateswithaligand |
μCz:=Ligand−macromolecularcomplex |
On the basis of these definitions we can re-index the remaining monomers, i.e., after excluding the unique monomer that binds to the ligand,
{Cy∈˜C=C∖{Cz}|y=1,2…Y;Y=Z−1} | (Def.(7)) |
We now derive an expression for the multimer (higher-order)-form of a ligand-macromolecular complex.
Theorem 5 (T5): The multimer-form of a ligand-macromolecular complex comprising identical monomer units and with an arbitrary unit associating with a ligand is,
∏y=Yy=1Cz.Cy=Cz.CyY|y=1,2…Y;Y=Z−1;Z≥2;z=1,2…Z | (31) |
Rewriting, this result in terms of the definition of the multimer form of a ligand-macromolecular complex,
Cz.CY≡μCz.CY | (Def.(8)) |
Theorem 6 (T6): The linear map between the transition-state dissociation constants that characterize the interactions of the monomer- and multimer-forms of a ligand-macromolecular complex is a bijection,
h−1∘h:ω∈Kd(cμ)↔u∈Kd(μCz) | (32) |
Theorem 7 (T7): The linear map between the transition-state dissociation constants and the eigenvalues that characterize the multimer-form of a ligand-macromolecular complex is a composition and an injection,
g∘h−1∘h:u∈Kd(μCz.CY)↦Kd(Czμ) | (33) |
The aforementioned theoretical results establish the mathematical rigor behind the definition and development of the transition-state dissociation constants as a model for ligand-macromolecular interactions (T1–T7, C1–C3). These assertions are complemented and numerically validated in R-4.1.2. Here, the R-packages, "ConvergenceConcepts" and "pracma" are utilized to investigate and analyze the stochastic convergence of the eigenvalues generated by the interaction matrix of a ligand-macromolecular complex (Supplementary Text 1) [27]. The R-scripts to establish convergence along with data processing are developed in-house (Supplementary Text 2). The stepwise algorithm to compute and numerically validate the transition-state dissociation constants is presented (Figure 1).
Step 1: A ligand and macromolecule with an equal number of atoms/residues (n=25) is chosen. Whilst, the complex can be modeled as a perfect homodimer, imperfect forms such as alternatively spliced isoenzymes are prevalent and commonly observed. Alternatively, the ligand can be modeled as a different macromolecule altogether.
Step 2: Populate the square interaction matrix with values randomly chosen from a uniform distribution, U[0,1]. These will represent one of three potential states for each interacting pair of atoms/residues of the modeled ligand-macromolecular complex (association, complete disassembly, transition-state).
Step 3: Compute the complex eigenvalues of this matrix and extract the real part of each.
Step 4: Form a sequence of the subset comprising those values that are strictly positive and belong to the open interval (0,1).
Step 5: Establish the stochastic convergence in distribution and/or probability of the terms of this sequence to the expected upper (tsup)- and lower (tinf)-bounds, i.e., 0 and 1.
Step 5.1: Construct a sequence of random numbers, X, whose elements are uniquely mapped to the eigenvalue-based transition-state dissociation constants and represent intermediate- or transition-states of the modeled ligand-macromolecular complex,
{Xa∈X∩Kd(cμ)⊂R∩(0,1)|a=1,2…A} | (Def.(9)) |
Here,
A=#(X∩Kd(cμ)) | (34) |
Step 5.2: Establish convergence of this set of random numbers. Here, weak convergence will suffice (distribution, probability),
lima→∞(Xa)→{tinf,tsup}={0,1} | (35) |
The parameters to accomplish this numerically are,
nmax:=NumberofvaluestoanalyseM:=Numberofpathsε:=Thresholdvaluetinf:=Lowerlimitofintervaltoestablishconvergencetsup:=Upperlimitofintervaltoestablishconvergence |
The values of these parameters for the numerically studied example are,
nmax=A=11 | (35.1) |
M=500 | (35.2) |
ε=0.01 | (35.3) |
tinf=0 | (35.4) |
tsup=1 | (35.5) |
The eigenvalue-based model of transition-state dissociation constants of a ligand-macromolecular complex asserts that there are several intermediate- or transition-states of a complex and that each of these has the potential to modify the biochemical process that the complex participates in.
Ligand-macromolecular complexes, in vivo, possess a finite and in most cases, an incomparable number of atoms/residues. The theoretical results establish definition(s), bounds and metrics to assess biochemical function for both, monomer (T1–T4, C1–C3)- and multimer (T5–T7)-forms. The numerical data suggests that the set of transition-state dissociation constants can be finite, converge and retain statistical relevance (Figure 1).
Proposition (P): The transition-state dissociation constants for the monomer (z=Z=1)- and multimer (Z≥2)-forms of a ligand-macromolecular complex with a finite number of atoms/residues of each (I,J) per monomer,
{u∈Kd(μCz.CY)|Cz∈C,μ∈L;z=1,2…Z;A={I,J}} | (Def.(10)) |
is the finite set,
Kd(μCz.CY)⊂Kd(Czμ) |
Here,
I=#Cz|Cz∈C | (36) |
J=#μ|μ∈L | (37) |
where,
Kd(.):=Setofconstrainedeigenvalue−basedtransition−statedissociationconstants |
I:=Finitenumberofatomsorresiduesofmacromolecule |
J:=Finitenumberofatomsorresiduesofligand |
Cμz.CY:=Multimerformofligand−macromolecularcomplex |
Enzyme-mediated catalysis, or lack thereof, results in metabolic enzyme disorders and may be inherited (inborn errors of metabolism) or acquired [28]. In order to assess the biomedical relevance of modeling ligand-macromolecule interactions as transition-state dissociation constants, the clinical outcomes of amino acid substitutions of selected enzyme homo- or hetero-dimers are examined (Table 1). These outcomes, i.e., benign, likely benign, pathologic, likely pathologic, conflicting, uncertain significance, are defined in accordance with the prevalent nomenclature of the ClinVar database [16]. Here, the data annotated as "uncertain significance" are those sequence variants with a high likelihood ( \approx 90–95\% ) of being "benign" or "pathogenic" [17]. This means they are likely to classified as "true positive", and if ignored will result in a "false negative". On the other hand, an outcome designated as with a "conflicting interpretation" is likely to be due to unresolved contradictory findings in the presence or absence of confounding factors. If we assume perfect contradiction, i.e., 50%, and couple this with the previous result, we get a \approx 70–73\% possibility that the variant of interest is a "true positive". This means, that here too, if missed a "false negative" will result. The metric of choice is the Recall (R) percentage,
R = \frac{TP}{TP+FN}\times 100 | (38) |
R: = Recall |
\begin{array}{l} TP: = Known\;positives(benign, likelybenign,\\ pathogenic, likely\;pathogenic) \end{array} | (Def. \; {\mathit{(12)}}) |
\begin{array}{l} FN: = Likely\;positives(conflicting\\ \;data, uncertain\;significance) \end{array} | (Def. \; {\mathit{(13)}}) |
Enzyme | EC | CV | SNP | M | Co | US | B | LB | P | LP | FN | TP | R (%) | |
1 | Glucokinase | 2.7.1.2 | 778 | 619 | 370 | 49 | 157 | 4 | 4 | 65 | 113 | 206 | 186 | 47.45 |
2 | Pyruvate kinase | 2.7.1.40 | 1239 | 629 | 209 | 14 | 135 | 7 | 7 | 30 | 20 | 149 | 64 | 30.05 |
3 | Cathepsin A | 3.4.16.x | 1776 | 1270 | 501 | 20 | 382 | 26 | 23 | 26 | 34 | 402 | 109 | 21.33 |
4 | Pyruvate dehydrogenase | 1.2.4.1 | 2317 | 1591 | 584 | 25 | 391 | 35 | 46 | 62 | 43 | 416 | 186 | 30.9 |
5 | Phosphofructokinase 1 | 2.7.1.11 | 165 | 32 | 10 | 2 | 4 | 2 | 1 | 1 | 1 | 6 | 5 | 45.45 |
6 | Phosphofructokinase 2 | 2.7.1.105 | 206 | 76 | 23 | 1 | 17 | 1 | 1 | 2 | 1 | 18 | 5 | 21.74 |
7 | Cystathione beta-synthase | 4.2.1.22 | 930 | 744 | 255 | 21 | 172 | 3 | 4 | 46 | 32 | 193 | 85 | 30.58 |
8 | DNA topoisomerase Ⅱ | 5.6.2.2 | 466 | 319 | 152 | 0 | 122 | 11 | 14 | 3 | 1 | 122 | 29 | 19.21 |
9 | Guanylate cyclase 1 | 4.6.1.2 | 1572 | 1117 | 576 | 29 | 417 | 11 | 10 | 34 | 59 | 446 | 114 | 20.36 |
10 | Phenylalanine hydroxylase | 1.14.16.1 | 1314 | 1102 | 631 | 5 | 182 | 0 | 3 | 161 | 254 | 187 | 418 | 69.09 |
Note: EC: Enzyme commission number; CV: Number of clinical variants; SNP: Single nucleotide polymorphisms; M: Missense mutations; Co: Conflicting data; US: Uncertain significance; B: Benign; LB: Likely; P: Pathogenic; LP: Likely pathogenic; FN: False negative (Co + US); TP: True positive (B + LB + P + LP); R: Recall ( \frac{TP}{TP+FN}\times 100 ). |
It is clear from these data that amino acid substitutions (nature, type), either alone or in combination, comprise distinct transition-states and are significant contributors to the biochemical function of each enzyme dimer ( Recall\approx 19–70\% ). Since each of these states will result in a distinct Kd , it is easily inferred that the transition-state dissociation constants, for a complex, may be more representative of biochemical function (T1–T4, C1–C3).
The discussion, vide supra, presents and highlights the biomedical relevance of modeling ligand-macromolecular interactions as transition-state dissociation constants. The results for monomer- and multimer-forms of ligand-macromolecular complexes are mathematically rigorous and have been validated, in silico. The results are now examined in context of biochemical function (enzyme, non-enzyme) for selected cases.
Case 1: Oxygen sensitive variants of non-haem iron (II)- and 2-oxoglutarate-dependent dioxygenases
The non-haem iron (Ⅱ)- and 2-oxoglutarate-dependent dioxygenases \left(EC1.14.x.y\right) , comprise a large superfamily of enzymes, are present in all kingdoms of life and is chemically diverse (variable reaction chemistry, multiple substrates) [12,13,29]. Clinically relevant members include Phytanoyl-CoA dioxygenase (PHYT), Lysine Hydroxylases, and the Proline 4-Hydroxylases (P4H) amongst several others [12,13,14,15,29]. These enzymes have important roles in Phytanic acid metabolism and collagen maturation, with sub-optimal activities contributing to diseases such as Refsum and the Ehlers-Danlos (ED)-syndrome [14,15,30]. Here, too, the outcomes (clinical, non-clinical) associated with substitution mutations for PHYT suggest that modeling ligand-macromolecular interactions as transition-state dissociation constants may be a better index of biochemical function (T1–T4, C1–C3, P) [31].
P4Hs, are classified as being either hypoxia-sensitive \left(H-P4H\equiv HP4H;EC\mathrm{1.14.11.29}\right) or collagen transforming \left(C-P4H\equiv CP4H;EC\mathrm{1.14.11.2}\right) [28]. These reactions may be written,
\begin{array}{rr}HP4H-HIF+{O}_{2}+2OG+Proline\begin{array}{c}\stackrel{{r}_{f}}{\to }\\ \underset{{r}_{b}}{\leftarrow }\end{array}Hydroxyproline+SA+{CO}_{2}& RXN\left(2\right)\\ CP4H+{O}_{2}+2OG+Proline\begin{array}{c}\stackrel{{r}_{f}}{\to }\\ \underset{{r}_{b}}{\leftarrow }\end{array}Hydroxyproline+SA+{CO}_{2}& RXN\left(3\right)\end{array} |
\begin{array}{rrr}{r}_{f}, {r}_{b}& : = & Rate\;constants\;for\;forward\;and\;backward\;reactions\;at\;steady\;state\\ HP4H& : = & Hypoxia\;inducible\;factor-dependent\;Proline\;4-Hydroxylase\\ HIF\equiv {\mu }_{high}& : = & Hypoxia-inducible\;factor(high-affinity\;modifier)\\ 2OG& : = & 2-oxoglutarate\\ SA& : = & Succinic\;acid\\ C{O}_{2}& : = & Carbon\;dioxide\end{array} |
The amino acid identity between HP4H and CP4H notwithstanding, there are significant differences between the molecular biology that they exhibit. This implies that despite the similarity of co-factor \left(iron\left(II\right)\right) , substrate \left(L-Proline\right) and co-substrate \left(2-oxoglutarate\right) , the binding affinities for molecular dioxygen vary considerably [7,32],
{Km}_{HP4H} = 0.1-0.76\;mM | (39) |
{Km}_{CP4H} = 0.03-1.5\;mM | (40) |
The turnover numbers for the cognate substrate, too, differ significantly [7],
{Kcat}_{HP4H} = 0.015-0.733{s}^{-1} | (41) |
{Kcat}_{CP4H} = 0.0188-0.02{s}^{-1} | (42) |
Clearly, a plausible explanation for these disparate empirical observations is the binding of the hypoxia-inducible factors (HIF) to P4H. The hypoxia-inducible factors (HIFs), are a family (n = 3) of transcription factors which sense hypoxia and trigger the upregulation of hypoxia-dependent genes [32,33,34]. Here, although hypoxia-inducible factor, is a full length protein, the actual binding site is the C-terminal end of HP4H [7,35].
Kd\approx 0.000016-0.023\;mM | (43) |
Some of these observations may be inferred from the partitioning of the transition-state dissociation constants into distinct subsets (Table 2):
Case 1 | Case 2 | |
Ligand \left(\boldsymbol{\mu }\in {\boldsymbol{\mathcal{L}}}\right) | Hypoxia-inducible factor | Peptide |
Macromolecule \left(\boldsymbol{c}\equiv {\boldsymbol{\mathcal{C}}}_{\boldsymbol{z}}\in \boldsymbol{\mathcal{C}}\right) | HP4H, CP4H | M1\beta |
Primary complex \left\langle {} \right.\boldsymbol{c}|\boldsymbol{\mu }\left. {} \right\rangle High-affinity variant :=\boldsymbol{K}\boldsymbol{d}\left({\boldsymbol{\mu }}_{\boldsymbol{h}\boldsymbol{i}\boldsymbol{g}\boldsymbol{h}}{\boldsymbol{\mathcal{C}}}_{\boldsymbol{z}}\right) Low-affinity variant :=\boldsymbol{K}\boldsymbol{d}\left({\boldsymbol{\mu }}_{\boldsymbol{h}\boldsymbol{i}\boldsymbol{g}\boldsymbol{h}}{\boldsymbol{\mathcal{C}}}_{\boldsymbol{z}}\right) |
\left\langle{\boldsymbol{H}\boldsymbol{P}{\bf{4}}\boldsymbol{H}|\boldsymbol{\mu }}\right\rangle , \left\langle{\boldsymbol{C}\boldsymbol{P}{\bf{4}}\boldsymbol{H}|\boldsymbol{\mu }}\right\rangle \boldsymbol{K}\boldsymbol{d}\left(\boldsymbol{H}\boldsymbol{P}{\bf{4}}\boldsymbol{H}|{\boldsymbol{\mu }}_{\boldsymbol{h}\boldsymbol{i}\boldsymbol{g}\boldsymbol{h}}\right)=\boldsymbol{K}\boldsymbol{d}\left({\boldsymbol{\mu }}_{\boldsymbol{h}\boldsymbol{i}\boldsymbol{g}\boldsymbol{h}}\boldsymbol{H}\boldsymbol{P}{\bf{4}}\boldsymbol{H}\right) \boldsymbol{K}\boldsymbol{d}\left(\boldsymbol{C}\boldsymbol{P}{\bf{4}}\boldsymbol{H}|{\boldsymbol{\mu }}_{\boldsymbol{l}\boldsymbol{o}\boldsymbol{w}}\right)=\boldsymbol{K}\boldsymbol{d}\left({\boldsymbol{\mu }}_{\boldsymbol{l}\boldsymbol{o}\boldsymbol{w}}\boldsymbol{C}\boldsymbol{P}{\bf{4}}\boldsymbol{H}\right) |
\left\langle{\boldsymbol{M}{\bf{1}}\boldsymbol{\beta }|\boldsymbol{\mu }}\right\rangle \boldsymbol{K}\boldsymbol{d}\left(\boldsymbol{M}{\bf{1}}\boldsymbol{\beta }|{\boldsymbol{\mu }}_{\boldsymbol{h}\boldsymbol{i}\boldsymbol{g}\boldsymbol{h}}\right)=\boldsymbol{K}\boldsymbol{d}\left({\boldsymbol{\mu }}_{\boldsymbol{h}\boldsymbol{i}\boldsymbol{g}\boldsymbol{h}}\boldsymbol{M}{\bf{1}}\boldsymbol{\beta }\right) \boldsymbol{K}\boldsymbol{d}\left(\boldsymbol{M}{\bf{1}}\boldsymbol{\beta }|{\boldsymbol{\mu }}_{\boldsymbol{l}\boldsymbol{o}\boldsymbol{w}}\right)=\boldsymbol{K}\boldsymbol{d}\left({\boldsymbol{\mu }}_{\boldsymbol{l}\boldsymbol{o}\boldsymbol{w}}\boldsymbol{M}{\bf{1}}\boldsymbol{\beta }\right) |
Higher-order complex \left\langle {} \right.\boldsymbol{c}|\boldsymbol{\mu }\left. {} \right\rangle .{{\mathcal{C}}}^{y} High-affinity variant :=\boldsymbol{K}\boldsymbol{d}\left({\boldsymbol{\mu }}_{\boldsymbol{h}\boldsymbol{i}\boldsymbol{g}\boldsymbol{h}}{\boldsymbol{\mathcal{C}}}_{\boldsymbol{z}}.{{\mathcal{C}}}^{y}\right) Low-affinity variant :=\boldsymbol{K}\boldsymbol{d}\left({\boldsymbol{\mu }}_{\boldsymbol{l}\boldsymbol{o}\boldsymbol{w}}{\boldsymbol{\mathcal{C}}}_{\boldsymbol{z}}.{{\mathcal{C}}}^{y}\right) |
--- --- --- |
\left\langle{\boldsymbol{M}{\bf{1}}\boldsymbol{\beta }|\boldsymbol{\mu }}\right\rangle.PLC \boldsymbol{K}\boldsymbol{d}\left(\boldsymbol{M}{\bf{1}}\boldsymbol{\beta }|{\boldsymbol{\mu }}_{\boldsymbol{h}\boldsymbol{i}\boldsymbol{g}\boldsymbol{h}}.\boldsymbol{P}\boldsymbol{L}\boldsymbol{C}\right)=\boldsymbol{K}\boldsymbol{d}\left({\boldsymbol{\mu }}_{\boldsymbol{h}\boldsymbol{i}\boldsymbol{g}\boldsymbol{h}}\boldsymbol{M}{\bf{1}}\boldsymbol{\beta }.\boldsymbol{P}\boldsymbol{L}\boldsymbol{C}\right) \boldsymbol{K}\boldsymbol{d}\left(\boldsymbol{M}{\bf{1}}\boldsymbol{\beta }|{\boldsymbol{\mu }}_{\boldsymbol{l}\boldsymbol{o}\boldsymbol{w}}.\boldsymbol{P}\boldsymbol{L}\boldsymbol{C}\right)=\boldsymbol{K}\boldsymbol{d}\left({\boldsymbol{\mu }}_{\boldsymbol{l}\boldsymbol{o}\boldsymbol{w}}\boldsymbol{M}{\bf{1}}\boldsymbol{\beta }.\boldsymbol{P}\boldsymbol{L}\boldsymbol{C}\right) |
Functional relevance | {R}_{HP4H}\left(t\right)\gg {R}_{CP4H}\left(t\right) | M1\beta |{\mu }_{high}.PLC\to \alpha M1\beta (Anterograde) {M1\beta |\mu }_{low}.PLC\to rM1\beta (Retrograde) |
Note: {\boldsymbol{\mathcal{C}}}_{\boldsymbol{z}\boldsymbol{\mu }} : All pairwise-atom/residue based square interaction matrix of ligand and macromolecule, \left\langle {} \right.\boldsymbol{c}|\boldsymbol{\mu }\left. {} \right\rangle ; \mathit{\boldsymbol{\mathcal{K}}\boldsymbol{\mathcal{C}}}_{\boldsymbol{z}\boldsymbol{\mu }} : Diagonal matrix of the interactions of a ligand and macromolecule; {z}_{i=j}=z : Set of eigenvalues of \mathit{\boldsymbol{\mathcal{K}}\boldsymbol{\mathcal{C}}}_{\boldsymbol{z}\boldsymbol{\mu }} where z\in diag\left(\mathit{\boldsymbol{\mathcal{K}}\boldsymbol{\mathcal{C}}}_{\boldsymbol{z}\boldsymbol{\mu }}\right)\subset \mathbb{C} ; \boldsymbol{K}\boldsymbol{d}\left(\boldsymbol{c}\boldsymbol{\mu }\right) : Set of eigenvalue-based transition-state dissociation constants for monomer- and multimer-forms of ligand-macromolecular complexes, \left\{\omega \in \boldsymbol{K}\boldsymbol{d}\left(\boldsymbol{c}\boldsymbol{\mu }\right)={\alpha }_{i=j}=Re\left(z\right)\in \boldsymbol{K}\boldsymbol{d}\left({\boldsymbol{\mathcal{C}}}_{\boldsymbol{z}\boldsymbol{\mu }}\right)\cap \left(\mathrm{0, 1}\right)\right\} ; {\mu }_{high}, {\mu }_{low} : High- and low-affinity variants of an arbitrary ligand, \mu =\{{\mu }_{high}, {\mu }_{low}\}\in {\boldsymbol{\mathcal{L}}} ; \boldsymbol{\mu }{\boldsymbol{\mathcal{C}}}_{\boldsymbol{z}}.{{\mathcal{C}}}^{y} : Higher-order complex of ligand and macromolecule; \boldsymbol{K}\boldsymbol{i}\boldsymbol{n}\boldsymbol{f} : Subset of transition-state dissociation constants of ligand-macromolecular interaction; \boldsymbol{K}\boldsymbol{s}\boldsymbol{u}\boldsymbol{p} :Subset of transition-state dissociation constants of ligand-macromolecular interaction; R\left(t\right) : Rate of reaction; HP4H: Hypoxia stimulated- and Collagen Proline 4-Hydroxylase; CP4H: M1\beta : Heterodimer of major histocompatibility complex I (MHC1) with beta-2 microglobulin; PLC: Peptide loading complex. |
In particular, binding of HIF restricts the range of the binding affinity of HP4H for molecular oxygen significantly,
\frac{\Delta {Km}_{HP4H}}{\Delta {Km}_{CP4H}}\times 100\approx 45\% | (44) |
Here, the set of conformers of HP4H once bound to HIF ensures that the catalytic activity of HP4H for HIF is significantly reduced in the presence of hypoxia. This will extend the half-life of HIF and facilitate transcription of HIF-responsive genes [36,37]. In contrast, CP4H exhibits no such differential activity. Furthermore, there is a significant variation in the catalytic activity (turnover number) of these enzymes for their cognate substrate (L-Proline),
\frac{max\left({Kcat}_{HP4H}\right)-min\left({Kcat}_{HP4H}\right)}{max\left({Kcat}_{CP4H}\right)-min\left({Kcat}_{CP4H}\right)} = \frac{\Delta {Kcat}_{HP4H}}{\Delta {Kcat}_{CP4H}}\approx 600 | (45) |
These data suggest that a ligand when bound to a macromolecule can affect the rate at which the resulting complex assembles or disassembles and thereby influence biochemical function. Hence, partitioning the transition-state dissociation constants of the ligand-macromolecular complex into distinct subsets may offer valuable insights into the in vivo function of enzymes in physiological and pathological states (T4, C2, C3).
Case 2: Generic model of MHC1-mediated high-affinity peptide export
The peptide loading complex (PLC) is a higher-order (Z > 2;Tapasin, ERp57, MHC1) complex that assembles at the endoplasmic reticulum (ER)-membrane and functions to transport cytosolic peptides into the ER-lumen en route to the plasma membrane [38,39]. Whilst, regulation of this process, by Tapasin is well studied, the role of peptides and the possible mechanism(s) of action is unclear [40,41,42,43]. A low-affinity peptide-driven (LAPD)-model of the MHC1-mediated export of high-affinity peptides to the plasma membrane of nucleated cells has been proposed and investigated in silico [44]. A major proponent of this study was simulating the differential disassembly of PLC in response to peptides with varying affinities (high, low) for the MHC1- {\beta }_{2} -microglobulin [44]. In fact, data from the simulations suggested that low-affinity peptides may not only actively participate in the transport of high-affinity peptide export, but could also regulate the same [44]. Another interesting observation discussed was the role of low-affinity peptides in priming the MHC1-export apparatus, such that irrespective of the nature of the cellular insult (acute, chronic), export of high-affinity peptides was rapid, continuous and efficient [44].
Utilizing the partition schema for the transition-state dissociation constants from the current analysis (Table 2), we can model and rewrite the differential disassembly of the PLC,
\begin{array}{rr}\left\langle{\boldsymbol{M}{\bf{1}}\boldsymbol{\beta }|{\boldsymbol{\mu }}_{\boldsymbol{h}\boldsymbol{i}\boldsymbol{g}\boldsymbol{h}}}\right\rangle.PLC\begin{array}{c}\stackrel{{r}_{f}}{\to }\\ \underset{{r}_{b}}{\leftarrow }\end{array}Tapasin-ERp57+aM1\beta & RXN\left(4\right)\\ \left\langle{\boldsymbol{M}{\bf{1}}\boldsymbol{\beta }|{\boldsymbol{\mu }}_{\boldsymbol{l}\boldsymbol{o}\boldsymbol{w}}}\right\rangle.PLC\begin{array}{c}\stackrel{{r}_{f}}{\to }\\ \underset{{r}_{b}}{\leftarrow }\end{array}Tapasin-ERp57-{\mu }_{low}+rM1\beta & RXN\left(5\right)\end{array} |
\begin{array}{l}{r}_{f}, {r}_{b} : = Rate\;constants\;for\;forward\;and\;backward\;reactions\;at\;steady\;state\\ M1\beta : = Heterodimer\;of\;MHC1\;with\;beta-2\;microglobulin\\ {\mu }_{low} : = Low-affinity\;peptide\\ {\mu }_{high} : = High-affinity\;peptide\\ \left\langle{\boldsymbol{M}{\bf{1}}\boldsymbol{\beta }|{\boldsymbol{\mu }}_{\boldsymbol{l}\boldsymbol{o}\boldsymbol{w}}}\right\rangle : = Set\;of\;peptides\;with\;low\;affinity\;for\;MHC1\;and\;in\;complex\;with\;MHC1\\ \left\langle{\boldsymbol{M}{\bf{1}}\boldsymbol{\beta }|{\boldsymbol{\mu }}_{\boldsymbol{h}\boldsymbol{i}\boldsymbol{g}\boldsymbol{h}}}\right\rangle : = Set\;of\;peptides\;with\;high\;affinity\;for\;MHC1\;and\;incomplex\;with\;MHC1\\ eM1\beta : = Net\;exportable\;complex\;of\;high-affinity\;peptide\;with\;MHC1\\ aM1\beta : = Anterograde-derived\;exportable\;complex\;of\;high-affinity\;peptide\;with\;MHC1\\ rM1\beta : = Retrograde-derived\;exportable\;complex\;of\;high-affinityp\;eptide\;with\;MHC1\\ PLC : = Peptide\;loading\;complex\\ ERp57 : = Endoplasmic\;reticulum\;protein\;disulfide\;isomerase\end{array} |
The appropriate dissociation constants are,
![]() |
(46) |
![]() |
(47) |
Rewriting these equations in terms of the peptide-bound MHC1,
{Kd}_{RXN4} = \frac{\zeta }{{\left[M1\beta |{\mu }_{high}\right]}^{{L}_{M1\beta |{\mu }_{high}}\ge 0}} | (48) |
where,
\zeta = \frac{\left[Tapasin-ERp57\right]\left[aM1\beta \right]}{{\left[PLC\right]}^{{L}_{PLC}\ge 0}} | (48.1) |
{Kd}_{RXN5} = \frac{\zeta }{{\left[M1\beta |{\mu }_{low}\right]}^{{L}_{M1\beta |{\mu }_{low}}\ge 0}} | (49) |
where,
\zeta = \frac{\left[Tapasin-ERp57-{\mu }_{low}\right]\left[rM1\beta \right]}{{\left[PLC\right]}^{{L}_{PLC}\ge 0}} | (49.1) |
Clearly,
{Kd}_{RXN4}\propto \frac{1}{{\left[M1\beta |{\mu }_{high}\right]}^{{L}_{M1\beta |{\mu }_{high}}\ge 0}} | (50) |
{Kd}_{RXN5}\propto \frac{1}{{\left[M1\beta |{\mu }_{low}\right]}^{{L}_{M1\beta |{\mu }_{low}}\ge 0}} | (51) |
These results suggest that,
{Kd}_{RXN4}\simeq 1.0\left(Perfect\;disassociation\right)and\left[aM1\beta \right]\to \infty | (52) |
{Kd}_{RXN5}\simeq 0.0\left(Perfect\;association\right)and\left[rM1\beta \right]\to 0 | (53) |
and is in accordance with existing empirical and simulation data,
\left[{\mu }_{high}\right] < < < \left[{\mu }_{low}\right], \left[aM1\beta \right] > > > \left[rM1\beta \right] | (54) |
Here, the partitioning of transition-state dissociation constants into low- and high-affinity peptides for the MHC1 can provide valuable insights into the underlying molecular biology of MHC1-mediated high-affinity peptide transport under physiological and pathological conditions (T5–T7, P) [42,43,44].
The work presented models ligand-macromolecular interactions as eigenvalue-based transition-state disassociation constants. The interaction matrix is an all-atom/residue pairwise comparison between the ligand and macromolecule and comprises numerical values drawn randomly from a standard uniform distribution. The transition-state dissociation constants are the strictly positive real part of all complex eigenvalues of this ligand-macromolecular interaction matrix, belong to the open interval (0, 1) and form a sequence whose terms are finite, monotonic, non-increasing and convergent. The findings are rigorous, numerically robust and can be extended to higher-order complexes. The study, additionally, suggests a schema by which a ligand may be partitioned into high- and low-affinity variants. This study, although theoretical offers a plausible explanation into the underlying biochemistry (enzyme-mediated substrate catalysis, assembly/disassembly and inhibitor kinetics) of ligand-macromolecular complexes. Future investigations may include assigning weights to each interaction, investigating origins of co-operativity in enzyme catalysis and inhibitory kinetics amongst others.
This section provides formal proofs for the included theorems, corollaries and proposition.
Proof (T1):
From Defs. (4) and (5),
{\text{For every}}\; \omega \in \boldsymbol{K}\boldsymbol{d}\left(\boldsymbol{c}\boldsymbol{\mu }\right)\exists g\left(\omega \right)\in \boldsymbol{K}\boldsymbol{d}\left(\boldsymbol{\mathcal{C}}_{\boldsymbol{z}\boldsymbol{\mu }}\right)|{g}^{-1}\circ g\left(\omega \right) = \omega | (55) |
Let,
{\omega }_{x}, {\omega }_{y}\in \boldsymbol{K}\boldsymbol{d}\left(\boldsymbol{c}\boldsymbol{\mu }\right)|g\left({\omega }_{x}\right), g\left({\omega }_{y}\right)\in \boldsymbol{K}\boldsymbol{d}\left(\boldsymbol{\mathcal{C}}_{\boldsymbol{z}\boldsymbol{\mu }}\right);x\ne y;\left\{x, y\right\}\le A |
If,
g\left({\omega }_{x}\right) = g\left({\omega }_{y}\right) | (56) |
then,
{g}^{-1}\circ g\left({\omega }_{x}\right) = {g}^{-1}\circ g\left({\omega }_{y}\right) | (57) |
\Rightarrow {\omega }_{x} = {\omega }_{y} | (58) |
If,
t = 0|t\in \boldsymbol{K}\boldsymbol{d}\left(\boldsymbol{\mathcal{C}}_{\boldsymbol{z}\boldsymbol{\mu }}\right) | (59) |
From (55),
{g}^{-1}\circ g\left(t\right) = 0\notin \boldsymbol{K}\boldsymbol{d}\left(\boldsymbol{c}\boldsymbol{\mu }\right) | (60) |
Similarly, For,
t\ge 1|t\in \boldsymbol{K}\boldsymbol{d}\left(\boldsymbol{\mathcal{C}}_{\boldsymbol{z}\boldsymbol{\mu }}\right) | (61) |
From (55),
{g}^{-1}\circ g\left(\omega \right)\ge 1\notin \boldsymbol{K}\boldsymbol{d}\left(\boldsymbol{c}\boldsymbol{\mu }\right) | (62) |
From (58), (60) and (62),
Proof (T2): (By induction)
For a = 1 ,
{\omega }_{a} = \mathrm{max}\left(\boldsymbol{K}\boldsymbol{d}\left(\boldsymbol{c}\boldsymbol{\mu }\right)\right) < 1 \ \ \ \ \ \ \ \ \ \ {\rm{(By\ Def.\ (5)), }} | (63) |
Assume a = A-1 ,
{\omega }_{a}\ge {\omega }_{A-1} | (64) |
Then \exists a = A ,
{\omega }_{a}\ge {\omega }_{A-1}\ge {\omega }_{A} | (65) |
For a = 1 ,
{\omega }_{a} = \mathrm{min}\left(\boldsymbol{K}\boldsymbol{d}\left(\boldsymbol{c}\boldsymbol{\mu }\right)\right) > 0 \ \ \ \ \ \ \ \ \ \ ({\rm{By}}\ Def.\ (5)), | (66) |
{\omega }_{a}\le {\omega }_{A-1} | (67) |
{\Rightarrow 0 < \left\{{\omega }_{a}\right\}}_{a\le a+1}\sim \boldsymbol{K}\boldsymbol{d}\left(\boldsymbol{c}\boldsymbol{\mu }\right) < 1 \forall \omega |
Proof (T3):
From (T2),
{\left\{{\omega }_{a}\right\}}_{a\le a+1} < 1 |
Choose \varepsilon \in {\mathbb{R}}_{+}, \varepsilon \to 0 ,
{\varepsilon }^{a > A} < \left|{\omega }_{a > A}-1\right| < \varepsilon |
For every a > A ,
\begin{array}{l} \left|\underset{a\to \infty }{\mathrm{lim}}{\omega }_{a > A}-1\right| < \varepsilon \\ \underset{a\to \infty }{\mathrm{lim}}|{\omega }_{a > A}-1| < \varepsilon\\ \ \ \ {\underset{a\to \infty }{\mathrm{lim}}{\omega }_{a > A}} = 1 \end{array} | (69) |
Similarly,
{\left\{{\omega }_{a}\right\}}_{a\le a+1} > 0 |
Choose \varepsilon \in {\mathbb{R}}_{+}, \varepsilon \to 0 ,
\frac{1}{\varepsilon } > \left|{\omega }_{a > A}-0\right| > \varepsilon |
For every a > A ,
\begin{array}{c} \left|\underset{a\to \infty }{\mathrm{lim}}{\omega }_{a > A}-0\right| > \varepsilon \\ \underset{a\to \infty }{\mathrm{lim}}|{\omega }_{a > A}-0| > \varepsilon \\ \underset{a\to \infty }{\mathrm{lim}}{\omega }_{a > A} = 0 \end{array} | (70) |
From (69) and (70),
\underset{a\to \infty }{\mathrm{lim}}{\left\{{\omega }_{a}\right\}}_{a\le a+1} = \left\{\mathrm{0, 1}\right\} |
Proof (C1):
Assume,
sup{\left\{{\omega }_{a}\right\}}_{a\le a+1} = max{\left\{{\omega }_{a}\right\}}_{a\le a+1} = {\omega }_{a = 1} |
Choose \varepsilon \in {\mathbb{R}}_{+}, \varepsilon \to 0
then for any a = \mathrm{1, 2}..A , we can find,
{\varepsilon }^{A}.{\omega }_{a = 1}⋘{\omega }_{a = 1} | (71) |
{\varepsilon }^{A}.{\omega }_{a = 1}\le {\omega }_{a = A} | (72) |
{\omega }_{a = 1}\le {\omega }_{a = A}.\left(\frac{1}{{\varepsilon }^{A}}\right) | (73) |
Let \delta \in {\mathbb{R}}_{+}, \delta \to 0 ,
{\omega }_{a = 1}-\delta < {\omega }_{a = A}.\left(\frac{1}{{\varepsilon }^{A}}\right) | (74) |
Assume,
inf{\left\{{\omega }_{a}\right\}}_{a\le a+1} = min{\left\{{\omega }_{a}\right\}}_{a\le a+1} = {\omega }_{a = A} |
Choose \varepsilon \in {\mathbb{R}}_{+}, \varepsilon \to 0
then for any a = \mathrm{1, 2}..A , we can find,
{\varepsilon }^{A}.{\omega }_{a = A} < {\omega }_{a = A} | (75) |
{\omega }_{a = A}⋘{\omega }_{a = A}.\left(\frac{1}{{\varepsilon }^{A}}\right) | (76) |
{\omega }_{a = 1}\le {\omega }_{a = 1}.\left(\frac{1}{{\varepsilon }^{A}}\right) | (77) |
Let \delta \in {\mathbb{R}}_{+}, \delta \to 0 ,
{\omega }_{a = 1} < {\omega }_{a = 1}.\left(\frac{1}{{\varepsilon }^{A}}\right)+\delta | (78) |
From (74) and (78),
Proof (T4):
From (T2 and T3), (C1 and C2)
Case (1)
If
{\left\{{\omega }_{a}\right\}}_{a > A} = \boldsymbol{K}\boldsymbol{s}\boldsymbol{u}\boldsymbol{p}\subset \boldsymbol{K}\boldsymbol{d}\left({\boldsymbol{\mathcal{C}}}_{\boldsymbol{z}\boldsymbol{\mu }}\right) |
then,
{\left\{{\omega }_{a}\right\}}_{a\le A}\in \boldsymbol{K}\boldsymbol{i}\boldsymbol{n}\boldsymbol{f}\sim \boldsymbol{K}\boldsymbol{d}\left(\boldsymbol{c}\boldsymbol{\mu }\right) | (79) |
Case (2)
If
{\left\{{\omega }_{a}\right\}}_{a > A} = \boldsymbol{K}\boldsymbol{i}\boldsymbol{n}\boldsymbol{f}\subset \boldsymbol{K}\boldsymbol{d}\left({\boldsymbol{\mathcal{C}}}_{\boldsymbol{z}\boldsymbol{\mu }}\right) |
then,
{\left\{{\omega }_{a}\right\}}_{a\le A}\in \boldsymbol{K}\boldsymbol{s}\boldsymbol{u}\boldsymbol{p}\sim \boldsymbol{K}\boldsymbol{d}\left(\boldsymbol{c}\boldsymbol{\mu }\right) | (80) |
From (79) and (80),
\boldsymbol{K}\boldsymbol{i}\boldsymbol{n}\boldsymbol{f}\cap \boldsymbol{K}\boldsymbol{s}\boldsymbol{u}\boldsymbol{p} = \left\{{\varnothing }\right\} | (81) |
Since,
\boldsymbol{K}\boldsymbol{d}\left({\boldsymbol{\mathcal{C}}}_{\boldsymbol{z}\boldsymbol{\mu }}\right)\supset \left(\boldsymbol{K}\boldsymbol{i}\boldsymbol{n}\boldsymbol{f}\cup \boldsymbol{K}\boldsymbol{s}\boldsymbol{u}\boldsymbol{p}\right)\cup \left(\boldsymbol{K}\boldsymbol{i}\boldsymbol{n}\boldsymbol{f}\cap \boldsymbol{K}\boldsymbol{s}\boldsymbol{u}\boldsymbol{p}\right) |
From (79)–(81),
\begin{array}{l} \boldsymbol{K}\boldsymbol{d}\left({\boldsymbol{\mathcal{C}}}_{\boldsymbol{z}\boldsymbol{\mu }}\right)\supset \boldsymbol{K}\boldsymbol{i}\boldsymbol{n}\boldsymbol{f}\cup \boldsymbol{K}\boldsymbol{s}\boldsymbol{u}\boldsymbol{p}\\ \ \ \ \ \ \Rightarrow \boldsymbol{K}\boldsymbol{d}\left(\boldsymbol{c}\boldsymbol{\mu }\right)\subset \boldsymbol{K}\boldsymbol{d}\left({\boldsymbol{\mathcal{C}}}_{\boldsymbol{z}\boldsymbol{\mu }}\right) \end{array} | (82) |
Proof (C3):
If,
\exists \mu \in {\boldsymbol{\mathcal{L}}}|\#\boldsymbol{K}\boldsymbol{s}\boldsymbol{u}\boldsymbol{p} > > > \#\boldsymbol{K}\boldsymbol{i}\boldsymbol{n}\boldsymbol{f}\forall a |
Then,
\mu \equiv {\mu }_{low} | (83) |
Similarly, if,
\exists \mu \in {\boldsymbol{\mathcal{L}}}|\#\boldsymbol{K}\boldsymbol{i}\boldsymbol{n}\boldsymbol{f} > > > \#\boldsymbol{K}\boldsymbol{s}\boldsymbol{u}\boldsymbol{p}\forall a |
Then,
\mu \equiv {\mu }_{high} | (84) |
From (83) and (84),
Proof (T5) (By induction)
Assume z = 1;Z\ge 2 , y = 1;Z = 2 ,
{\mathcal{C}}_{1}.{\prod }_{y = 1}^{y = Y = Z-1}{\mathcal{C}}_{y} = {\mathcal{C}}_{1}.{\prod }_{y = 1}^{y = 1}{\mathcal{C}}_{y} | (85) |
= {\mathcal{C}}_{1}.{\mathcal{C}}_{Y} | (85.1) |
= {\mathcal{C}}_{1}.{\mathcal{C}}^{Y} | (85.2) |
Assume truth for y = Y\gg 1 ,
{\mathcal{C}}_{1}.{\prod }_{y = 1}^{y = Y = Z-1}{\mathcal{C}}_{y} = {\mathcal{C}}_{z}.\left({\mathcal{C}}_{1}{\dots .{\mathcal{C}}}_{Y}\right) | (86) |
= {\mathcal{C}}_{1}.{\mathcal{C}}^{Y} | (86.1) |
For y = Y+1 ,
{\mathcal{C}}_{1}.{\prod }_{y = 1}^{y = Y+1}{\mathcal{C}}_{y} = {\mathcal{C}}_{1}.{\prod }_{y = 1}^{y = Y+1}{\mathcal{C}}_{y} | (87) |
= {\mathcal{C}}_{1}.\left({\prod }_{y = 1}^{y = Y}{\mathcal{C}}_{y}\right).\left({\prod }_{y = 1}^{y = 1}{\mathcal{C}}_{y}\right) | (87.1) |
= {\mathcal{C}}_{1}.{\mathcal{C}}_{Y}^{Y}.{\mathcal{C}}_{1}^{1} | (87.2) |
= {\mathcal{C}}_{1}.{\mathcal{C}}^{Y+1} | (87.3) |
From (85)–(87),
Proof (T6):
From Defs. (6–8),
\mu {\mathcal{C}}_{z}.{\mathcal{C}}^{Y}\equiv \mu {\mathcal{C}}_{z}\equiv ⟨\boldsymbol{c}|\boldsymbol{\mu }⟩ | (88) |
\Rightarrow \boldsymbol{K}\boldsymbol{d}\left(\boldsymbol{\mu }{\boldsymbol{\mathcal{C}}}_{\boldsymbol{z}}.{\boldsymbol{\mathcal{C}}}^{\boldsymbol{Y}}\right)\sim \boldsymbol{K}\boldsymbol{d}\left(\boldsymbol{c}\boldsymbol{\mu }\right) | (89) |
\Rightarrow \boldsymbol{K}\boldsymbol{d}\left(\boldsymbol{\mu }{\boldsymbol{\mathcal{C}}}_{\boldsymbol{z}}.{\boldsymbol{\mathcal{C}}}^{\boldsymbol{Y}}\right)\cap \boldsymbol{K}\boldsymbol{d}\left(\boldsymbol{c}\boldsymbol{\mu }\right) | (90) |
From Def. (5),
{\omega }_{a\in [1, A]}\in \left(\boldsymbol{K}\boldsymbol{d}\left(\boldsymbol{\mu }{\boldsymbol{\mathcal{C}}}_{\boldsymbol{z}}.{\boldsymbol{\mathcal{C}}}^{\boldsymbol{Y}}\right)\cap \boldsymbol{K}\boldsymbol{d}\left(\boldsymbol{c}\boldsymbol{\mu }\right)\right) | (91) |
\left({\omega }_{a\in [1, A]}\in \boldsymbol{K}\boldsymbol{d}\left(\boldsymbol{\mu }{\boldsymbol{\mathcal{C}}}_{\boldsymbol{z}}.{\boldsymbol{\mathcal{C}}}^{\boldsymbol{Y}}\right)\right)\cap \left({\omega }_{a\in [1, A]}\in \boldsymbol{K}\boldsymbol{d}\left(\boldsymbol{c}\boldsymbol{\mu }\right)\right) | (92) |
From (T2–T4), (C1–C3),
\forall a\left({\omega }_{a = \mathrm{1, 2}\dots A}\subseteq \boldsymbol{K}\boldsymbol{d}\left(\boldsymbol{\mu }{\boldsymbol{\mathcal{C}}}_{\boldsymbol{z}}.{\boldsymbol{\mathcal{C}}}^{\boldsymbol{Y}}\right)\right)\cap \left({\omega }_{a = \mathrm{1, 2}\dots A}\subseteq \boldsymbol{K}\boldsymbol{d}\left(\boldsymbol{c}\boldsymbol{\mu }\right)\right) | (93) |
\Rightarrow \left({\left\{{\omega }_{a}\right\}}_{a = \mathrm{1, 2}\dots A}\sim \boldsymbol{K}\boldsymbol{d}\left(\boldsymbol{\mu }{\boldsymbol{\mathcal{C}}}_{\boldsymbol{z}}.{\boldsymbol{\mathcal{C}}}^{\boldsymbol{Y}}\right)\right)\cap \left({\left\{{\omega }_{a}\right\}}_{a = \mathrm{1, 2}\dots A}\sim \boldsymbol{K}\boldsymbol{d}\left(\boldsymbol{c}\boldsymbol{\mu }\right)\right) | (94) |
Conversely, let,
u\in \boldsymbol{K}\boldsymbol{d}\left(\boldsymbol{\mu }{\boldsymbol{\mathcal{C}}}_{\boldsymbol{z}}.{\boldsymbol{\mathcal{C}}}^{\boldsymbol{Y}}\right);\omega \in \boldsymbol{K}\boldsymbol{d}\left(\boldsymbol{c}\boldsymbol{\mu }\right) |
From (92) and (94),
\forall \omega \in \boldsymbol{K}\boldsymbol{d}\left(\boldsymbol{c}\boldsymbol{\mu }\right)\exists u\in \boldsymbol{K}\boldsymbol{d}\left(\boldsymbol{\mu }{\boldsymbol{\mathcal{C}}}_{\boldsymbol{z}}.{\boldsymbol{\mathcal{C}}}^{\boldsymbol{Y}}\right)|u = h\left(\omega \right) | (95) |
\forall u\in \boldsymbol{K}\boldsymbol{d}\left(\boldsymbol{\mu }{\boldsymbol{\mathcal{C}}}_{\boldsymbol{z}}.{\boldsymbol{\mathcal{C}}}^{\boldsymbol{Y}}\right)\exists \omega \in \boldsymbol{K}\boldsymbol{d}\left(\boldsymbol{c}\boldsymbol{\mu }\right)|\omega = {h}^{-1}\left(u\right) = {h}^{-1}\left(h\left(\omega \right)\right) | (96) |
From (95) and (96),
Proof (T7):
From (T6),
{h}^{-1}\circ h:\omega \in \boldsymbol{K}\boldsymbol{d}\left(\boldsymbol{c}\boldsymbol{\mu }\right) |
From (T1), Def. (4)
g:\omega \in \boldsymbol{K}\boldsymbol{d}\left(\boldsymbol{c}\boldsymbol{\mu }\right)\mapsto \boldsymbol{K}\boldsymbol{d}\left(\boldsymbol{c}\boldsymbol{\mu }\right)\cup \mathbb{R} = \boldsymbol{K}\boldsymbol{d}\left({\boldsymbol{\mathcal{C}}}_{\boldsymbol{z}\boldsymbol{\mu }}\right) |
Rewriting,
g:\omega \mapsto \boldsymbol{K}\boldsymbol{d}\left({\boldsymbol{\mathcal{C}}}_{\boldsymbol{z}\boldsymbol{\mu }}\right) | (97) |
g\left({h}^{-1}\left(u\right)\right)\mapsto \boldsymbol{K}\boldsymbol{d}\left({\boldsymbol{\mathcal{C}}}_{\boldsymbol{z}\boldsymbol{\mu }}\right) | (98) |
g\circ {h}^{-1}\left(h\left(\omega u\right)\right)\mapsto \boldsymbol{K}\boldsymbol{d}\left({\boldsymbol{\mathcal{C}}}_{\boldsymbol{z}\boldsymbol{\mu }}\right) | (99) |
g\circ {h}^{-1}\circ h\left(\omega \right)\mapsto \boldsymbol{K}\boldsymbol{d}\left({\boldsymbol{\mathcal{C}}}_{\boldsymbol{z}\boldsymbol{\mu }}\right) | (100) |
Proof (P):
From (T1–T7) and Defs. (4–8, 10),
For z = Z = 1 ,
{\mu {\mathcal{C}}}_{z}.{\mathcal{C}}^{Y} = \mu {\mathcal{C}}_{z} | (101) |
g\circ {h}^{-1}\left(u\in \boldsymbol{K}\boldsymbol{d}\left(\boldsymbol{\mu }{\boldsymbol{\mathcal{C}}}_{\boldsymbol{z}}\right)\right)\mapsto \boldsymbol{K}\boldsymbol{d}\left({\boldsymbol{\mathcal{C}}}_{\boldsymbol{z}\boldsymbol{\mu }}\right)\subset \mathbb{R} | (102) |
g\circ {h}^{-1}\circ h\left(\omega \right)\mapsto \boldsymbol{K}\boldsymbol{d}\left({\boldsymbol{\mathcal{C}}}_{\boldsymbol{z}\boldsymbol{\mu }}\right) | (102.1) |
\Rightarrow \boldsymbol{K}\boldsymbol{d}\left(\boldsymbol{\mu }{\boldsymbol{\mathcal{C}}}_{\boldsymbol{z}}\right)\subset \boldsymbol{K}\boldsymbol{d}\left({\boldsymbol{\mathcal{C}}}_{\boldsymbol{z}\boldsymbol{\mu }}\right) | (103) |
For Z\ge 2 ,
g\circ {h}^{-1}\left(u\in \boldsymbol{K}\boldsymbol{d}\left(\boldsymbol{\mu }{\boldsymbol{\mathcal{C}}}_{\boldsymbol{z}}.{\boldsymbol{\mathcal{C}}}^{\boldsymbol{Y}}\right)\right)\mapsto \boldsymbol{K}\boldsymbol{d}\left({\boldsymbol{\mathcal{C}}}_{\boldsymbol{z}\boldsymbol{\mu }}\right)\subset \mathbb{R} | (104) |
g\circ {h}^{-1}\circ h\left(\omega \right)\mapsto \boldsymbol{K}\boldsymbol{d}\left({\boldsymbol{\mathcal{C}}}_{\boldsymbol{z}\boldsymbol{\mu }}\right) | (104.1) |
\Rightarrow \boldsymbol{K}\boldsymbol{d}\left(\boldsymbol{\mu }{\boldsymbol{\mathcal{C}}}_{\boldsymbol{z}}.{\boldsymbol{\mathcal{C}}}^{\boldsymbol{Y}}\right)\subset \boldsymbol{K}\boldsymbol{d}\left({\boldsymbol{\mathcal{C}}}_{\boldsymbol{z}\boldsymbol{\mu }}\right) | (105) |
From (103) and (105),
This work is funded by an early career intramural grant (Code No. A-766) awarded to SK by the All India Institute of Medical Sciences (AIIMS, New Delhi, INDIA).
The author declare there is no conflict of interest.
[1] |
Jaganathan SK, Mandal M (2009) Honey constituents and their apoptotic effect in colon cancer cells. J Apiproduct Apimedical Sci 1: 29-36. https://doi.org/10.3896/IBRA.4.01.2.02 doi: 10.3896/IBRA.4.01.2.02
![]() |
[2] |
Saxena S, Gautam S, Sharma A (2010) Physical, biochemical and antioxidant properties of some Indian honeys. Food Chem 118: 391-397. https://doi.org/10.1016/j.foodchem.2009.05.001 doi: 10.1016/j.foodchem.2009.05.001
![]() |
[3] |
Kamal MA, Klein P (2011) Determination of sugars in honey by liquid chromatography. Saudi J Biol Sci 18: 17-21. https://doi.org/10.1016/j.sjbs.2010.09.003 doi: 10.1016/j.sjbs.2010.09.003
![]() |
[4] |
Dinca OR, Ionete RE, Popescu R, et al. (2015) Geographical and botanical origin discrimination of Romanian honey using complex stable isotope data and chemometrics. Food Anal Methods 8: 401-412. https://doi.org/10.1007/s12161-014-9903-x doi: 10.1007/s12161-014-9903-x
![]() |
[5] |
Al-Farsi M, Al-Belushi S, Al-Amri A, et al. (2018) Quality evaluation of Omani honey. Food Chem 262: 162-167. https://doi.org/10.1016/j.foodchem.2018.04.104 doi: 10.1016/j.foodchem.2018.04.104
![]() |
[6] | Bogdanov S, Ruoff K, Oddo, LP (2004) Physico-chemical methods for the characterisation of unifloral honeys: A review. Apidologie 35: S4-S17. https://doi.10.1051/apido:2004047 |
[7] |
Gomes S, Dias LG, Moreira LL, et al. (2010) Physicochemical, microbiological and antimicrobial properties of commercial honeys from Portugal. Food Chem Toxicol 48: 544-548. https://doi.org/10.1016/j.fct.2009.11.029 doi: 10.1016/j.fct.2009.11.029
![]() |
[8] |
Da Silva PM, Gauche C, Gonzaga LV, et al. (2016) Honey: Chemical composition, stability and authenticity. Food Chem 196: 309-323. https://doi.org/10.1016/j.foodchem.2015.09.051 doi: 10.1016/j.foodchem.2015.09.051
![]() |
[9] |
Kwakman PH, Velde AAT, de Boer L, et al. (2010) How honey kills bacteria. The FASEB J 24: 2576-2582. https://doi.org/10.1096/fj.09-150789 doi: 10.1096/fj.09-150789
![]() |
[10] |
Nolan VC, Harrison J, Cox JA (2019) Dissecting the antimicrobial composition of honey. Antibiotics 8: 251. https://doi.org/10.3390/antibiotics8040251 doi: 10.3390/antibiotics8040251
![]() |
[11] |
Olaitan PB, Adeleke OE, Ola IO (2007) Honey: A reservoir for microorganisms and an inhibitory agent for microbes. Afr Health Sci 7: 159-165. https://doi.org/10.5555/afhs.2007.7.3.159 doi: 10.5555/afhs.2007.7.3.159
![]() |
[12] |
Snowdon JA, Cliver DO (1996) Microorganisms in honey. Int J Food Microbiol 31: 1-26. https://doi.org/10.1016/0168-1605(96)00970-1 doi: 10.1016/0168-1605(96)00970-1
![]() |
[13] |
Gilliam M (1997) Identification and roles of non-pathogenic microflora associated with honeybees. FEMS Microbiol Letters 155: 1-10. https://doi.org/10.1111/j.1574-6968.1997.tb12678.x doi: 10.1111/j.1574-6968.1997.tb12678.x
![]() |
[14] | Tysset C, Durand C, Taliercio YP (1970) Contribution to the study of the microbial contamination and the hygiene of commercial honey. Rec Med Vet 1970: 1471-1492. |
[15] |
Nakano H, Sakagucki G (1991) An unusually heavy contamination of honey products by Clostridium botulinum type F and Bacillus alvei. FEMS Microbiol Letters 79: 171-178. https://doi.org/10.1111/j.1574-6968.1991.tb04524.x doi: 10.1111/j.1574-6968.1991.tb04524.x
![]() |
[16] |
Veress A, Nagy T, Wilk T, et al. (2020) Abundance of mobile genetic elements in an Acinetobacter lwoffii strain isolated from Transylvanian honey sample. Sci Rep 10: 2969. https://doi.org/10.1038/s41598-020-59938-9 doi: 10.1038/s41598-020-59938-9
![]() |
[17] |
Kostić AŽ, Petrović TS, Krnjaja VS, et al. (2017) Mold/aflatoxin contamination of honeybee collected pollen from different Serbian regions. J Apic Res 56: 13-20. https://doi.org/10.1080/00218839.2016.1259897 doi: 10.1080/00218839.2016.1259897
![]() |
[18] |
Tanzi MG, Gabay MP (2002) Association between honey consumption and infant botulism. Pharmacotherapy: The Journal of Human Pharmacology and Drug Therapy 22: 1479-1483. https://doi.org/10.1592/phco.22.16.1479.33696 doi: 10.1592/phco.22.16.1479.33696
![]() |
[19] |
Brown KL (2000) Control of bacterial spores. Br Med Bull 56: 158-171. https://doi.org/10.1258/0007142001902860 doi: 10.1258/0007142001902860
![]() |
[20] |
Hebbar HU, Nandini KE, Lakshmi MC, et al. (2003) Microwave and infrared heat processing of honey and its quality. Food Sci Technol Res 9: 49-53. https://doi.org/10.3136/fstr.9.49 doi: 10.3136/fstr.9.49
![]() |
[21] |
Tosi EA, Ré E, Lucero H, et al. (2004) Effect of honey high-temperature short-time heating on parameters related to quality, crystallisation phenomena and fungal inhibition. LWT-Food Sci Technol 37: 669-678. https://doi.org/10.1016/j.lwt.2004.02.005 doi: 10.1016/j.lwt.2004.02.005
![]() |
[22] |
Scripcă LA, Amariei S (2021) The use of ultrasound for preventing honey crystallization. Foods 10: 773. https://doi.org/10.3390/foods10040773 doi: 10.3390/foods10040773
![]() |
[23] | Silva EB (2000) The cook, the cooker and the gendering of the kitchen. The Sociol Rev 48: 612-628. https://doi.org/10.1111%2F1467-954X.00235 |
[24] |
Camel V (2001) Recent extraction techniques for solid matrices—supercritical fluid extraction, pressurized fluid extraction and microwave-assisted extraction: Their potential and pitfalls. Analyst 126: 1182-1193. https://doi.org/10.1039/B008243K doi: 10.1039/B008243K
![]() |
[25] | Singh RP, Heldman DR (2001) Introduction to food engineering. Gulf Professional Publishing. |
[26] | Lauf RJ, Bible DW, Johnson AC, et al. (1993) Two to 18 GHz broadband microwave heating systems. Microwave J 36: 24-30. |
[27] |
Chandrasekaran S, Ramanathan S, Basak T (2013) Microwave food processing-A review. Food Res Int 52: 243-261. https://doi.org/10.1016/j.foodres.2013.02.033 doi: 10.1016/j.foodres.2013.02.033
![]() |
[28] |
Venkatesh MS, Raghavan GSV (2004) An overview of microwave processing and dielectric properties of agri-food materials. Biosyst Eng 88: 1-18. https://doi.org/10.1016/j.biosystemseng.2004.01.007 doi: 10.1016/j.biosystemseng.2004.01.007
![]() |
[29] |
Kozempel MF, Annous BA, Cook RD, et al. (1998) Inactivation of microorganisms with microwaves at reduced temperaturas. J Food Prot 61: 582-585. https://doi.org/10.4315/0362-028X-61.5.582 doi: 10.4315/0362-028X-61.5.582
![]() |
[30] |
Kuchma T (1998) Synergistic effect of microwave heating and Hydrogen peroxide on inactivation of microorganisms. J Microwave Power Electromagn Energy 33: 77-87. https://doi.org/10.1080/08327823.1998.11688363 doi: 10.1080/08327823.1998.11688363
![]() |
[31] |
Woo IS, Rhee IK, Park HD (2000) Differential damage in bacterial cells by microwave radiation on the basis of cell wall structure. Appl Environ Microbiol 66: 2243-2247. https://doi.org/10.1128/AEM.66.5.2243-2247.2000 doi: 10.1128/AEM.66.5.2243-2247.2000
![]() |
[32] |
Papadopoulou C, Demetriou D, Panagiou A, et al. (1995) Survival of enterobacteria in liquid cultures during microwave radiation and conventional heating. Microbiol Res 150: 305-309. https://doi.org/10.1016/S0944-5013(11)80010-8 doi: 10.1016/S0944-5013(11)80010-8
![]() |
[33] |
Fung DY, Cunningham FE (1980) Effect of microwaves on microorganisms in foods. J Food Prot 43: 641-650. https://doi.org/10.4315/0362-028X-43.8.641 doi: 10.4315/0362-028X-43.8.641
![]() |
[34] |
Liu W, Lanier TC (2016) Rapid (microwave) heating rate effects on texture, fat/water holding, and microstructure of cooked comminuted meat batters. Food Res Int 81: 108-113. https://doi.org/10.1016/j.foodres.2016.01.005 doi: 10.1016/j.foodres.2016.01.005
![]() |
[35] |
Guo W, Zhu X, Liu Y, et al. (2010) Sugar and water contents of honey with dielectric property sensing. J Food Eng 97: 275-281. https://doi.org/10.1016/j.jfoodeng.2009.10.024 doi: 10.1016/j.jfoodeng.2009.10.024
![]() |
[36] |
Vadivambal R, Jayas DS (2010) Non-uniform temperature distribution during microwave heating of food materials—A review. Food Bioprocess Technol 3: 161-171. https://doi.org/10.1007/s11947-008-0136-0 doi: 10.1007/s11947-008-0136-0
![]() |
[37] |
Bhandari BR, Howes T (1999) Implication of glass transition for the drying and stability of dried foods. J Food Eng 40: 71-79. https://doi.org/10.1016/S0260-8774(99)00039-4 doi: 10.1016/S0260-8774(99)00039-4
![]() |
[38] | Santos-Buelga C, González-Paramás AM (2017) Chemical composition of honey. In: Alvarez-Suarez J (Eds.), Bee Products-Chemical and Biological Properties, Springer, Cham, 43-82. https://doi.org/10.1007/978-3-319-59689-1_3 |
[39] |
Yener E, Ungan S, Özilgen M (1987) Drying behavior of honey‐starch mixtures. J Food Sci 52: 1054-1058. https://doi.org/10.1111/j.1365-2621.1987.tb14274.x doi: 10.1111/j.1365-2621.1987.tb14274.x
![]() |
[40] |
BartáKoVá K, DračKoVá M, BorKoVCoVá I, et al. (2011) Impact of microwave heating on hydroxymethylfurfural content in Czech honeys. Czech J Food Sci 29: 328-336. http://dx.doi.org/10.17221/110/2009-CJFS doi: 10.17221/110/2009-CJFS
![]() |
[41] |
Turhan I, Tetik N, Karhan M, et al. (2008) Quality of honeys influenced by thermal treatment. LWT-Food Sci Technol 41: 1396-1399. https://doi.org/10.1016/j.lwt.2007.09.008 doi: 10.1016/j.lwt.2007.09.008
![]() |
[42] |
Tosi E, Ciappini M, Re E, et al. (2002) Honey thermal treatment effects on hydroxymethylfurfural content. Food Chem 77: 71-74. https://doi.org/10.1016/S0308-8146(01)00325-9 doi: 10.1016/S0308-8146(01)00325-9
![]() |
[43] | Kowalski S, Lukasiewicz M, Bednarz S, et al. (2012) Diastase number changes during thermaland microwave processing of honey. Czech J Food Sci 30: 21-26. |
[44] |
Ajandouz EH, Tchiakpe LS, Ore FD, et al. (2001) Effects of pH on caramelization and Maillard reaction kinetics in fructose‐lysine model systems. J Food Sci 66: 926-931. https://doi.org/10.1111/j.1365-2621.2001.tb08213.x doi: 10.1111/j.1365-2621.2001.tb08213.x
![]() |
[45] |
Kowalski S (2013) Changes of antioxidant activity and formation of 5-hydroxymethylfurfural in honey during thermal and microwave processing. Food Chem 141: 1378-1382. https://doi.org/10.1016/j.foodchem.2013.04.025 doi: 10.1016/j.foodchem.2013.04.025
![]() |
[46] |
Matsui KN, Granado LM, De Oliveira, et al. (2007) Peroxidase and polyphenol oxidase thermal inactivation by microwaves in green coconut water simulated solutions. LWT-Food Sci Technol 40: 852-859. https://doi.org/10.1016/j.lwt.2006.03.019 doi: 10.1016/j.lwt.2006.03.019
![]() |
[47] |
Zamora MC, Chirife J (2006) Determination of water activity change due to crystallization in honeys from Argentina. Food Control 17: 59-64. https://doi.org/10.1016/j.foodcont.2004.09.003 doi: 10.1016/j.foodcont.2004.09.003
![]() |
[48] |
Bucekova M, Juricova V, Monton E, et al. (2018) Microwave processing of honey negatively affects honey antibacterial activity by inactivation of bee-derived glucose oxidase and defensin-1. Food Chem 240: 1131-1136. https://doi.org/10.1016/j.foodchem.2017.08.054 doi: 10.1016/j.foodchem.2017.08.054
![]() |
[49] |
Breslin AK, Meyer BM, Dria WS, et al. (2011) Enumeration and identification of bacterial contaminants in commercial and locally produced honey. Bios 82: 103-111. https://doi.org/10.1893/011.082.0401 doi: 10.1893/011.082.0401
![]() |
[50] | Wright MH, Adelskov J, Greene AC (2017) Bacterial DNA extraction using individual enzymes and phenol/chloroform separation. J Microbiol Biol Educ 18: 18-2. https://doi.org/10.1128/jmbe.v18i2.1348 |
[51] | Miyoshi T, Iwatsuki T, Naganuma T (2005) Phylogenetic characterization of 16S rRNA gene clones from deep-groundwater microorganisms that pass through 0.2-micrometer-pore-size filters. Appl Environ Microbiol 71: 1084-1088. https://doi.org/10.1128/AEM.71.2.1084-1088.2005 |
[52] |
Turton JF, Woodford N, Glover J, et al. (2006) Identification of Acinetobacter baumannii by detection of the bla OXA-51-like carbapenemase gene intrinsic to this species. J Clin Microbiol 44: 2974-2976. https://doi.org/10.1128/JCM.01021-06 doi: 10.1128/JCM.01021-06
![]() |
[53] |
Mama M, Teshome T, Detamo J (2019) Antibacterial activity of honey against methicillin-resistant staphylococcus aureus: A laboratory-based experimental study. Int J Microbiol 2019: 7686130. https://doi.org/10.1155/2019/7686130 doi: 10.1155/2019/7686130
![]() |
[54] |
Wiegand I, Hilpert K, Hancock RE (2008) Agar and broth dilution methods to determine the minimal inhibitory concentration (MIC) of antimicrobial substances. Nat Protoc 3: 163. https://doi.org/10.1038/nprot.2007.521 doi: 10.1038/nprot.2007.521
![]() |
[55] |
Iurlina MO, Fritz R (2005) Characterization of microorganisms in Argentinean honeys from different sources. Int J Food Microbiol 105: 297-304. https://doi.org/10.1016/j.ijfoodmicro.2005.03.017 doi: 10.1016/j.ijfoodmicro.2005.03.017
![]() |
[56] | Tysset C, Rousseau M (1981) Problem of microbes and hygiene of commercial honey. Revue de medecine veterinaire 132: 591. |
[57] |
Klein S, Cabirol A, Devaud JM, et al. (2017) Why bees are so vulnerable to environmental stressors. Trends Ecol Evol 32: 268-278. https://doi.org/10.1016/j.tree.2016.12.009 doi: 10.1016/j.tree.2016.12.009
![]() |
[58] | Porrini C, Sabatini AG, Girotti S, et al. (2003) Honeybees and bee products as monitors of the environmental contamination. Apiacta 38: 63-70. |
[59] | Celli G, Maccagnani B (2003) Honeybees as bioindicators of environmental pollution. Bull Insectology 56:137-139. |
[60] |
Khan KA, Al-Ghamdi AA, Ghramh HA, et al. (2020) Structural diversity and functional variability of gut microbial communities associated with honeybees. Microb Pathog 138: 103793. https://doi.org/10.1016/j.micpath.2019.103793 doi: 10.1016/j.micpath.2019.103793
![]() |
[61] | Malika N, Mohamed F, Chakib E (2005) Microbiological and Physico-chemical properties of Moroccan honey. Int J Agric Biol 7: 773-776. https://doi.org/1560-8530/2005/07-5-773-776 |
[62] |
Pomastowski P, Złoch M, Rodzik A, et al. (2019) Analysis of bacteria associated with honeys of different geographical and botanical origin using two different identification approaches: MALDI-TOF MS and 16S rDNA PCR technique. PloS One 14: e0217078. https://doi.org/10.1371/journal.pone.0217078 doi: 10.1371/journal.pone.0217078
![]() |
[63] |
Tsadila C, Nikolaidis M, Dimitriou TG, et al. (2021) Antibacterial activity and characterization of bacteria isolated from diverse types of Greek honey against nosocomial and foodborne pathogens. Appl Sci 11: 5801. https://doi.org/10.3390/app11135801 doi: 10.3390/app11135801
![]() |
[64] |
Alkassab AT, Beims H, Janke M, et al. (2022) Determination, distribution, and environmental fate of Bacillus thuringiensis spores in various honeybee matrices after field application as plant protection product. Environ Sci Pollut Res 29: 25995-26001. https://doi.org/10.1007/s11356-022-19414-5 doi: 10.1007/s11356-022-19414-5
![]() |
[65] | Alvarez-Perez S, Baker LJ, Morris MM, et al. (2021) Acinetobacter pollinis sp. nov., Acinetobacter baretiae sp. nov. and Acinetobacter rathckeae sp. nov., isolated from floral nectar and honeybees. Int J Syst Evol Microbiol 71: 004783. https://doi.org/10.1099/ijsem.0.004783 |
[66] | Alvarez-Perez S, Lievens B, Jacquemyn H, et al. (2013) Acinetobacter nectaris sp. nov. and Acinetobacter boissieri sp. nov., isolated from floral nectar of wild Mediterranean insect-pollinated plants. Int J Syst Evol Microbiol 63: 1532-1539. https://doi.org/10.1099/ijs.0.043489-0 |
[67] | Kim PS, Shin NR, Kim JY, et al. (2014) Acinetobacter apis sp. nov., isolated from the intestinal tract of a honeybee, Apis mellifera. J Microbiol 52: 639-645. https://doi.org/10.1007/s12275-014-4078-0 |
[68] |
Wang FH, Qiao M, Lv ZE, et al. (2014) Impact of reclaimed water irrigation on antibiotic resistance in public parks, Beijing, China. Environ Poll 184: 247-253. https://doi.org/10.1016/j.envpol.2013.08.038 doi: 10.1016/j.envpol.2013.08.038
![]() |
[69] |
Dekic S, Hrenovic J, Durn G, et al. (2020) Survival of extensively-and pandrug-resistant isolates of Acinetobacter baumannii in soils. Appl Soil Ecol 147: 103396. https://doi.org/10.1016/j.apsoil.2019.103396 doi: 10.1016/j.apsoil.2019.103396
![]() |
[70] |
Adewoyin MA, Okoh AI (2018) The natural environment as a reservoir of pathogenic and non-pathogenic Acinetobacter species. Rev Environ Health 33: 265-272. https://doi.org/10.1515/reveh-2017-0034 doi: 10.1515/reveh-2017-0034
![]() |
[71] |
Vázquez-Quiñones CR, Moreno-Terrazas R, Natividad-Bonifacio I, et al. (2018) Microbiological assessment of honey in México. Revista Argentina de Microbiologia 50: 75-80. https://doi.org/10.1016/j.ram.2017.04.005 doi: 10.1016/j.ram.2017.04.005
![]() |
[72] | Elzeini HM, Ali ARAA, Nasr NF, et al. (2021) Probiotic capability of novel lactic acid bacteria isolated from worker honeybees gut microbiota. FEMS Microbiol Lett 368: fnab030. https://doi.org/10.1093/femsle/fnab030 |
[73] |
Benlloch-Tinoco M, Pina-Pérez MC, Martínez-Navarrete N, et al. (2014) Listeria monocytogenes inactivation kinetics under microwave and conventional thermal processing in a kiwifruit puree. Innovative Food Sci Emerging Technol 22: 131-136. https://doi.org/10.1016/j.ifset.2014.01.005 doi: 10.1016/j.ifset.2014.01.005
![]() |
[74] |
Vasudev H, Singh G, Bansal A, et al. (2019) Microwave heating and its applications in surface engineering: A review. Mater Res Express 6: 102001. https://doi.org/10.1088/2053-1591/ab3674 doi: 10.1088/2053-1591/ab3674
![]() |
[75] | Rosenberg U, Bogl W (1987) Microwave pasteurization, sterilization, blanching, and pest control in the food industry. Food Technol 41: 92-99. |
[76] |
De la Paz Moliné M, Fernández NJ, Medici SK, et al. (2015) Effect of microwave treatment on microbial contamination of honeys and on their physicochemical and thermal properties. Pol J Food Nutr Sci 65: 119-126. http://dx.doi.org/10.1515/pjfns-2015-0031 doi: 10.1515/pjfns-2015-0031
![]() |
[77] | Molan PC (1992) The antibacterial nature of honey: 1. The nature of the antibacterial activity. Bee World 73: 5-28. https://doi.org/10.1080/0005772X.1992.11099109 |
[78] |
Molan PC (1992) The antibacterial activity of honey: 2 Variation in the potency of the antibacterial activity. Bee World 73: 59-76. https://doi.org/10.1080/0005772X.1992.11099118 doi: 10.1080/0005772X.1992.11099118
![]() |
[79] |
Xue SJ, Chen L, Jiang H, et al. (2019) High pullulan biosynthesis from high concentration of glucose by a hyperosmotic resistant, yeast-like fungal strain isolated from a natural comb-honey. Food Chem 286: 123-128. https://doi.org/10.1016/j.foodchem.2019.01.206 doi: 10.1016/j.foodchem.2019.01.206
![]() |
[80] |
Brudzynski K, Abubaker K, Castle A (2011) Re-examining the role of hydrogen peroxide in bacteriostatic and bactericidal activities of honey. Front Microbiol 2: 213. https://doi.org/10.3389/fmicb.2011.00213 doi: 10.3389/fmicb.2011.00213
![]() |
[81] |
Pimentel RBDQ, da Costa CA, Albuquerque PM, et al. (2013) Antimicrobial activity and rutin identification of honey produced by the stingless bee Melipona compressipes manaosensis and commercial honey. BMC Complementary Altern Med 13: 151. https://doi.org/10.1186/1472-6882-13-151 doi: 10.1186/1472-6882-13-151
![]() |
[82] |
Anand S, Deighton M, Livanos G, et al. (2019) Agastache honey has superior antifungal activity in comparison with important commercial honeys. Sci Rep 9: 18197. https://doi.org/10.1038/s41598-019-54679-w doi: 10.1038/s41598-019-54679-w
![]() |
[83] |
Nader RA, Mackieh R, Wehbe R, et al. (2021) Beehive products as antibacterial agents: A review. Antibiotics 10: 717. https://doi.org/10.3390/antibiotics10060717 doi: 10.3390/antibiotics10060717
![]() |
[84] |
Kilty SJ, Duval M, Chan FT, et al. (2011) Methylglyoxal: (active agent of manuka honey) in vitro activity against bacterial biofilms. Int Forum Allergy Rhinol 1: 348-350. https://doi.org/10.1002/alr.20073 doi: 10.1002/alr.20073
![]() |
[85] |
Maddocks SE, Jenkins RE (2013) Honey: A sweet solution to the growing problem of antimicrobial resistance?. Future Microbiol 8: 1419-1429. https://doi.org/10.2217/fmb.13.105 doi: 10.2217/fmb.13.105
![]() |
[86] |
Bulman SE, Tronci G, Goswami P, et al. (2017) Antibacterial properties of nonwoven wound dressings coated with manuka honey or methylglyoxal. Materials 10: 954. https://doi.org/10.3390/ma10080954 doi: 10.3390/ma10080954
![]() |
[87] |
Gobin I, Crnković G, Magdalenić M, et al. (2018) Antibacterial potential of Croatian honey against antibiotic resistant pathogenic bacteria. Medicinski Glasnik 15: 139-144. https://doi.org/10.17392/951-18 doi: 10.17392/951-18
![]() |
[88] |
Shamala TR, Shri Jyothi Y, Saibaba P (2000) Stimulatory effect of honey on multiplication of lactic acid bacteria under in vitro and in vivo conditions. Lett Appl Microbiol 30: 453-455. https://doi.org/10.1046/j.1472-765x.2000.00746.x doi: 10.1046/j.1472-765x.2000.00746.x
![]() |
[89] |
Olofsson TC, Butler È, Markowicz P, et al. (2014) Lactic acid bacterial symbionts in honeybees-an unknown key to honey's antimicrobial and therapeutic activities. Int Wound J 13: 668-679. https://doi.org/10.1111/iwj.12345 doi: 10.1111/iwj.12345
![]() |
[90] |
Olofsson TC, Butler E, Lindholm C, et al. (2016) Fighting off wound pathogens in horses with honeybee lactic acid bacteria. Curr Microbiol 73: 463-473. https://doi.org/10.1007/s00284-016-1080-2 doi: 10.1007/s00284-016-1080-2
![]() |
[91] |
Vásquez A, Forsgren E, Fries I, et al. (2012) Symbionts as major modulators of insect health: Lactic acid bacteria and honeybees. PloS One 7: e33188. https://doi.org/10.1371/journal.pone.0033188 doi: 10.1371/journal.pone.0033188
![]() |
[92] |
Von Büren RS, Oehen B, Kuhn NJ, et al. (2019) High-resolution maps of Swiss apiaries and their applicability to study spatial distribution of bacterial honeybee brood diseases. Peer J 7: e6393. https://doi.org/10.7717/peerj.6393 doi: 10.7717/peerj.6393
![]() |
[93] |
Ibarguren C, Raya RR, Apella MC, et al. (2010) Enterococcus faecium isolated from honey synthesized bacteriocin-like substances active against different Listeria monocytogenes strains. J Microbiol 48: 44-52. https://doi.org/10.1007/s12275-009-0177-8 doi: 10.1007/s12275-009-0177-8
![]() |
[94] |
Lin D, Long X, Xiao L, et al. (2020) Study on the functional properties and structural characteristics of soybean soluble polysaccharides by mixed bacteria fermentation and microwave treatment. Int J Biol Macromol 157: 561-568. https://doi.org/10.1016/j.ijbiomac.2020.04.133 doi: 10.1016/j.ijbiomac.2020.04.133
![]() |
[95] | Dimins F, Mikelsone V, Kuka P, et al. (2014) Effect of different types of heat treatment on invertase activity in honey. In: 9th Baltic Conference on Food Science and Technology "Food for Consumer Well-Being", 284. |
[96] |
Jenkins R, Roberts A, Brown HL (2015) On the antibacterial effects of manuka honey: Mechanistic insights. Res Rep Biol 6: 215-224. http://dx.doi.org/10.2147/RRB.S75754 doi: 10.2147/RRB.S75754
![]() |
[97] |
Al-Habsi NA, Niranjan K (2012) Effect of high hydrostatic pressure on antimicrobial activity and quality of Manuka honey. Food Chem 135: 1448-1454. https://doi.org/10.1016/j.foodchem.2012.06.012 doi: 10.1016/j.foodchem.2012.06.012
![]() |
1. | Siddhartha Kundu, ReDirection: an R-package to compute the probable dissociation constant for every reaction of a user-defined biochemical network, 2023, 10, 2296-889X, 10.3389/fmolb.2023.1206502 | |
2. | Siddhartha Kundu, A mathematically rigorous algorithm to define, compute and assess relevance of the probable dissociation constants in characterizing a biochemical network, 2024, 14, 2045-2322, 10.1038/s41598-024-53231-9 |
Enzyme | EC | CV | SNP | M | Co | US | B | LB | P | LP | FN | TP | R (%) | |
1 | Glucokinase | 2.7.1.2 | 778 | 619 | 370 | 49 | 157 | 4 | 4 | 65 | 113 | 206 | 186 | 47.45 |
2 | Pyruvate kinase | 2.7.1.40 | 1239 | 629 | 209 | 14 | 135 | 7 | 7 | 30 | 20 | 149 | 64 | 30.05 |
3 | Cathepsin A | 3.4.16.x | 1776 | 1270 | 501 | 20 | 382 | 26 | 23 | 26 | 34 | 402 | 109 | 21.33 |
4 | Pyruvate dehydrogenase | 1.2.4.1 | 2317 | 1591 | 584 | 25 | 391 | 35 | 46 | 62 | 43 | 416 | 186 | 30.9 |
5 | Phosphofructokinase 1 | 2.7.1.11 | 165 | 32 | 10 | 2 | 4 | 2 | 1 | 1 | 1 | 6 | 5 | 45.45 |
6 | Phosphofructokinase 2 | 2.7.1.105 | 206 | 76 | 23 | 1 | 17 | 1 | 1 | 2 | 1 | 18 | 5 | 21.74 |
7 | Cystathione beta-synthase | 4.2.1.22 | 930 | 744 | 255 | 21 | 172 | 3 | 4 | 46 | 32 | 193 | 85 | 30.58 |
8 | DNA topoisomerase Ⅱ | 5.6.2.2 | 466 | 319 | 152 | 0 | 122 | 11 | 14 | 3 | 1 | 122 | 29 | 19.21 |
9 | Guanylate cyclase 1 | 4.6.1.2 | 1572 | 1117 | 576 | 29 | 417 | 11 | 10 | 34 | 59 | 446 | 114 | 20.36 |
10 | Phenylalanine hydroxylase | 1.14.16.1 | 1314 | 1102 | 631 | 5 | 182 | 0 | 3 | 161 | 254 | 187 | 418 | 69.09 |
Note: EC: Enzyme commission number; CV: Number of clinical variants; SNP: Single nucleotide polymorphisms; M: Missense mutations; Co: Conflicting data; US: Uncertain significance; B: Benign; LB: Likely; P: Pathogenic; LP: Likely pathogenic; FN: False negative (Co + US); TP: True positive (B + LB + P + LP); R: Recall ( \frac{TP}{TP+FN}\times 100 ). |
Case 1 | Case 2 | |
Ligand \left(\boldsymbol{\mu }\in {\boldsymbol{\mathcal{L}}}\right) | Hypoxia-inducible factor | Peptide |
Macromolecule \left(\boldsymbol{c}\equiv {\boldsymbol{\mathcal{C}}}_{\boldsymbol{z}}\in \boldsymbol{\mathcal{C}}\right) | HP4H, CP4H | M1\beta |
Primary complex \left\langle {} \right.\boldsymbol{c}|\boldsymbol{\mu }\left. {} \right\rangle High-affinity variant :=\boldsymbol{K}\boldsymbol{d}\left({\boldsymbol{\mu }}_{\boldsymbol{h}\boldsymbol{i}\boldsymbol{g}\boldsymbol{h}}{\boldsymbol{\mathcal{C}}}_{\boldsymbol{z}}\right) Low-affinity variant :=\boldsymbol{K}\boldsymbol{d}\left({\boldsymbol{\mu }}_{\boldsymbol{h}\boldsymbol{i}\boldsymbol{g}\boldsymbol{h}}{\boldsymbol{\mathcal{C}}}_{\boldsymbol{z}}\right) |
\left\langle{\boldsymbol{H}\boldsymbol{P}{\bf{4}}\boldsymbol{H}|\boldsymbol{\mu }}\right\rangle , \left\langle{\boldsymbol{C}\boldsymbol{P}{\bf{4}}\boldsymbol{H}|\boldsymbol{\mu }}\right\rangle \boldsymbol{K}\boldsymbol{d}\left(\boldsymbol{H}\boldsymbol{P}{\bf{4}}\boldsymbol{H}|{\boldsymbol{\mu }}_{\boldsymbol{h}\boldsymbol{i}\boldsymbol{g}\boldsymbol{h}}\right)=\boldsymbol{K}\boldsymbol{d}\left({\boldsymbol{\mu }}_{\boldsymbol{h}\boldsymbol{i}\boldsymbol{g}\boldsymbol{h}}\boldsymbol{H}\boldsymbol{P}{\bf{4}}\boldsymbol{H}\right) \boldsymbol{K}\boldsymbol{d}\left(\boldsymbol{C}\boldsymbol{P}{\bf{4}}\boldsymbol{H}|{\boldsymbol{\mu }}_{\boldsymbol{l}\boldsymbol{o}\boldsymbol{w}}\right)=\boldsymbol{K}\boldsymbol{d}\left({\boldsymbol{\mu }}_{\boldsymbol{l}\boldsymbol{o}\boldsymbol{w}}\boldsymbol{C}\boldsymbol{P}{\bf{4}}\boldsymbol{H}\right) |
\left\langle{\boldsymbol{M}{\bf{1}}\boldsymbol{\beta }|\boldsymbol{\mu }}\right\rangle \boldsymbol{K}\boldsymbol{d}\left(\boldsymbol{M}{\bf{1}}\boldsymbol{\beta }|{\boldsymbol{\mu }}_{\boldsymbol{h}\boldsymbol{i}\boldsymbol{g}\boldsymbol{h}}\right)=\boldsymbol{K}\boldsymbol{d}\left({\boldsymbol{\mu }}_{\boldsymbol{h}\boldsymbol{i}\boldsymbol{g}\boldsymbol{h}}\boldsymbol{M}{\bf{1}}\boldsymbol{\beta }\right) \boldsymbol{K}\boldsymbol{d}\left(\boldsymbol{M}{\bf{1}}\boldsymbol{\beta }|{\boldsymbol{\mu }}_{\boldsymbol{l}\boldsymbol{o}\boldsymbol{w}}\right)=\boldsymbol{K}\boldsymbol{d}\left({\boldsymbol{\mu }}_{\boldsymbol{l}\boldsymbol{o}\boldsymbol{w}}\boldsymbol{M}{\bf{1}}\boldsymbol{\beta }\right) |
Higher-order complex \left\langle {} \right.\boldsymbol{c}|\boldsymbol{\mu }\left. {} \right\rangle .{{\mathcal{C}}}^{y} High-affinity variant :=\boldsymbol{K}\boldsymbol{d}\left({\boldsymbol{\mu }}_{\boldsymbol{h}\boldsymbol{i}\boldsymbol{g}\boldsymbol{h}}{\boldsymbol{\mathcal{C}}}_{\boldsymbol{z}}.{{\mathcal{C}}}^{y}\right) Low-affinity variant :=\boldsymbol{K}\boldsymbol{d}\left({\boldsymbol{\mu }}_{\boldsymbol{l}\boldsymbol{o}\boldsymbol{w}}{\boldsymbol{\mathcal{C}}}_{\boldsymbol{z}}.{{\mathcal{C}}}^{y}\right) |
--- --- --- |
\left\langle{\boldsymbol{M}{\bf{1}}\boldsymbol{\beta }|\boldsymbol{\mu }}\right\rangle.PLC \boldsymbol{K}\boldsymbol{d}\left(\boldsymbol{M}{\bf{1}}\boldsymbol{\beta }|{\boldsymbol{\mu }}_{\boldsymbol{h}\boldsymbol{i}\boldsymbol{g}\boldsymbol{h}}.\boldsymbol{P}\boldsymbol{L}\boldsymbol{C}\right)=\boldsymbol{K}\boldsymbol{d}\left({\boldsymbol{\mu }}_{\boldsymbol{h}\boldsymbol{i}\boldsymbol{g}\boldsymbol{h}}\boldsymbol{M}{\bf{1}}\boldsymbol{\beta }.\boldsymbol{P}\boldsymbol{L}\boldsymbol{C}\right) \boldsymbol{K}\boldsymbol{d}\left(\boldsymbol{M}{\bf{1}}\boldsymbol{\beta }|{\boldsymbol{\mu }}_{\boldsymbol{l}\boldsymbol{o}\boldsymbol{w}}.\boldsymbol{P}\boldsymbol{L}\boldsymbol{C}\right)=\boldsymbol{K}\boldsymbol{d}\left({\boldsymbol{\mu }}_{\boldsymbol{l}\boldsymbol{o}\boldsymbol{w}}\boldsymbol{M}{\bf{1}}\boldsymbol{\beta }.\boldsymbol{P}\boldsymbol{L}\boldsymbol{C}\right) |
Functional relevance | {R}_{HP4H}\left(t\right)\gg {R}_{CP4H}\left(t\right) | M1\beta |{\mu }_{high}.PLC\to \alpha M1\beta (Anterograde) {M1\beta |\mu }_{low}.PLC\to rM1\beta (Retrograde) |
Note: {\boldsymbol{\mathcal{C}}}_{\boldsymbol{z}\boldsymbol{\mu }} : All pairwise-atom/residue based square interaction matrix of ligand and macromolecule, \left\langle {} \right.\boldsymbol{c}|\boldsymbol{\mu }\left. {} \right\rangle ; \mathit{\boldsymbol{\mathcal{K}}\boldsymbol{\mathcal{C}}}_{\boldsymbol{z}\boldsymbol{\mu }} : Diagonal matrix of the interactions of a ligand and macromolecule; {z}_{i=j}=z : Set of eigenvalues of \mathit{\boldsymbol{\mathcal{K}}\boldsymbol{\mathcal{C}}}_{\boldsymbol{z}\boldsymbol{\mu }} where z\in diag\left(\mathit{\boldsymbol{\mathcal{K}}\boldsymbol{\mathcal{C}}}_{\boldsymbol{z}\boldsymbol{\mu }}\right)\subset \mathbb{C} ; \boldsymbol{K}\boldsymbol{d}\left(\boldsymbol{c}\boldsymbol{\mu }\right) : Set of eigenvalue-based transition-state dissociation constants for monomer- and multimer-forms of ligand-macromolecular complexes, \left\{\omega \in \boldsymbol{K}\boldsymbol{d}\left(\boldsymbol{c}\boldsymbol{\mu }\right)={\alpha }_{i=j}=Re\left(z\right)\in \boldsymbol{K}\boldsymbol{d}\left({\boldsymbol{\mathcal{C}}}_{\boldsymbol{z}\boldsymbol{\mu }}\right)\cap \left(\mathrm{0, 1}\right)\right\} ; {\mu }_{high}, {\mu }_{low} : High- and low-affinity variants of an arbitrary ligand, \mu =\{{\mu }_{high}, {\mu }_{low}\}\in {\boldsymbol{\mathcal{L}}} ; \boldsymbol{\mu }{\boldsymbol{\mathcal{C}}}_{\boldsymbol{z}}.{{\mathcal{C}}}^{y} : Higher-order complex of ligand and macromolecule; \boldsymbol{K}\boldsymbol{i}\boldsymbol{n}\boldsymbol{f} : Subset of transition-state dissociation constants of ligand-macromolecular interaction; \boldsymbol{K}\boldsymbol{s}\boldsymbol{u}\boldsymbol{p} :Subset of transition-state dissociation constants of ligand-macromolecular interaction; R\left(t\right) : Rate of reaction; HP4H: Hypoxia stimulated- and Collagen Proline 4-Hydroxylase; CP4H: M1\beta : Heterodimer of major histocompatibility complex I (MHC1) with beta-2 microglobulin; PLC: Peptide loading complex. |
Enzyme | EC | CV | SNP | M | Co | US | B | LB | P | LP | FN | TP | R (%) | |
1 | Glucokinase | 2.7.1.2 | 778 | 619 | 370 | 49 | 157 | 4 | 4 | 65 | 113 | 206 | 186 | 47.45 |
2 | Pyruvate kinase | 2.7.1.40 | 1239 | 629 | 209 | 14 | 135 | 7 | 7 | 30 | 20 | 149 | 64 | 30.05 |
3 | Cathepsin A | 3.4.16.x | 1776 | 1270 | 501 | 20 | 382 | 26 | 23 | 26 | 34 | 402 | 109 | 21.33 |
4 | Pyruvate dehydrogenase | 1.2.4.1 | 2317 | 1591 | 584 | 25 | 391 | 35 | 46 | 62 | 43 | 416 | 186 | 30.9 |
5 | Phosphofructokinase 1 | 2.7.1.11 | 165 | 32 | 10 | 2 | 4 | 2 | 1 | 1 | 1 | 6 | 5 | 45.45 |
6 | Phosphofructokinase 2 | 2.7.1.105 | 206 | 76 | 23 | 1 | 17 | 1 | 1 | 2 | 1 | 18 | 5 | 21.74 |
7 | Cystathione beta-synthase | 4.2.1.22 | 930 | 744 | 255 | 21 | 172 | 3 | 4 | 46 | 32 | 193 | 85 | 30.58 |
8 | DNA topoisomerase Ⅱ | 5.6.2.2 | 466 | 319 | 152 | 0 | 122 | 11 | 14 | 3 | 1 | 122 | 29 | 19.21 |
9 | Guanylate cyclase 1 | 4.6.1.2 | 1572 | 1117 | 576 | 29 | 417 | 11 | 10 | 34 | 59 | 446 | 114 | 20.36 |
10 | Phenylalanine hydroxylase | 1.14.16.1 | 1314 | 1102 | 631 | 5 | 182 | 0 | 3 | 161 | 254 | 187 | 418 | 69.09 |
Note: EC: Enzyme commission number; CV: Number of clinical variants; SNP: Single nucleotide polymorphisms; M: Missense mutations; Co: Conflicting data; US: Uncertain significance; B: Benign; LB: Likely; P: Pathogenic; LP: Likely pathogenic; FN: False negative (Co + US); TP: True positive (B + LB + P + LP); R: Recall ( \frac{TP}{TP+FN}\times 100 ). |
Case 1 | Case 2 | |
Ligand \left(\boldsymbol{\mu }\in {\boldsymbol{\mathcal{L}}}\right) | Hypoxia-inducible factor | Peptide |
Macromolecule \left(\boldsymbol{c}\equiv {\boldsymbol{\mathcal{C}}}_{\boldsymbol{z}}\in \boldsymbol{\mathcal{C}}\right) | HP4H, CP4H | M1\beta |
Primary complex \left\langle {} \right.\boldsymbol{c}|\boldsymbol{\mu }\left. {} \right\rangle High-affinity variant :=\boldsymbol{K}\boldsymbol{d}\left({\boldsymbol{\mu }}_{\boldsymbol{h}\boldsymbol{i}\boldsymbol{g}\boldsymbol{h}}{\boldsymbol{\mathcal{C}}}_{\boldsymbol{z}}\right) Low-affinity variant :=\boldsymbol{K}\boldsymbol{d}\left({\boldsymbol{\mu }}_{\boldsymbol{h}\boldsymbol{i}\boldsymbol{g}\boldsymbol{h}}{\boldsymbol{\mathcal{C}}}_{\boldsymbol{z}}\right) |
\left\langle{\boldsymbol{H}\boldsymbol{P}{\bf{4}}\boldsymbol{H}|\boldsymbol{\mu }}\right\rangle , \left\langle{\boldsymbol{C}\boldsymbol{P}{\bf{4}}\boldsymbol{H}|\boldsymbol{\mu }}\right\rangle \boldsymbol{K}\boldsymbol{d}\left(\boldsymbol{H}\boldsymbol{P}{\bf{4}}\boldsymbol{H}|{\boldsymbol{\mu }}_{\boldsymbol{h}\boldsymbol{i}\boldsymbol{g}\boldsymbol{h}}\right)=\boldsymbol{K}\boldsymbol{d}\left({\boldsymbol{\mu }}_{\boldsymbol{h}\boldsymbol{i}\boldsymbol{g}\boldsymbol{h}}\boldsymbol{H}\boldsymbol{P}{\bf{4}}\boldsymbol{H}\right) \boldsymbol{K}\boldsymbol{d}\left(\boldsymbol{C}\boldsymbol{P}{\bf{4}}\boldsymbol{H}|{\boldsymbol{\mu }}_{\boldsymbol{l}\boldsymbol{o}\boldsymbol{w}}\right)=\boldsymbol{K}\boldsymbol{d}\left({\boldsymbol{\mu }}_{\boldsymbol{l}\boldsymbol{o}\boldsymbol{w}}\boldsymbol{C}\boldsymbol{P}{\bf{4}}\boldsymbol{H}\right) |
\left\langle{\boldsymbol{M}{\bf{1}}\boldsymbol{\beta }|\boldsymbol{\mu }}\right\rangle \boldsymbol{K}\boldsymbol{d}\left(\boldsymbol{M}{\bf{1}}\boldsymbol{\beta }|{\boldsymbol{\mu }}_{\boldsymbol{h}\boldsymbol{i}\boldsymbol{g}\boldsymbol{h}}\right)=\boldsymbol{K}\boldsymbol{d}\left({\boldsymbol{\mu }}_{\boldsymbol{h}\boldsymbol{i}\boldsymbol{g}\boldsymbol{h}}\boldsymbol{M}{\bf{1}}\boldsymbol{\beta }\right) \boldsymbol{K}\boldsymbol{d}\left(\boldsymbol{M}{\bf{1}}\boldsymbol{\beta }|{\boldsymbol{\mu }}_{\boldsymbol{l}\boldsymbol{o}\boldsymbol{w}}\right)=\boldsymbol{K}\boldsymbol{d}\left({\boldsymbol{\mu }}_{\boldsymbol{l}\boldsymbol{o}\boldsymbol{w}}\boldsymbol{M}{\bf{1}}\boldsymbol{\beta }\right) |
Higher-order complex \left\langle {} \right.\boldsymbol{c}|\boldsymbol{\mu }\left. {} \right\rangle .{{\mathcal{C}}}^{y} High-affinity variant :=\boldsymbol{K}\boldsymbol{d}\left({\boldsymbol{\mu }}_{\boldsymbol{h}\boldsymbol{i}\boldsymbol{g}\boldsymbol{h}}{\boldsymbol{\mathcal{C}}}_{\boldsymbol{z}}.{{\mathcal{C}}}^{y}\right) Low-affinity variant :=\boldsymbol{K}\boldsymbol{d}\left({\boldsymbol{\mu }}_{\boldsymbol{l}\boldsymbol{o}\boldsymbol{w}}{\boldsymbol{\mathcal{C}}}_{\boldsymbol{z}}.{{\mathcal{C}}}^{y}\right) |
--- --- --- |
\left\langle{\boldsymbol{M}{\bf{1}}\boldsymbol{\beta }|\boldsymbol{\mu }}\right\rangle.PLC \boldsymbol{K}\boldsymbol{d}\left(\boldsymbol{M}{\bf{1}}\boldsymbol{\beta }|{\boldsymbol{\mu }}_{\boldsymbol{h}\boldsymbol{i}\boldsymbol{g}\boldsymbol{h}}.\boldsymbol{P}\boldsymbol{L}\boldsymbol{C}\right)=\boldsymbol{K}\boldsymbol{d}\left({\boldsymbol{\mu }}_{\boldsymbol{h}\boldsymbol{i}\boldsymbol{g}\boldsymbol{h}}\boldsymbol{M}{\bf{1}}\boldsymbol{\beta }.\boldsymbol{P}\boldsymbol{L}\boldsymbol{C}\right) \boldsymbol{K}\boldsymbol{d}\left(\boldsymbol{M}{\bf{1}}\boldsymbol{\beta }|{\boldsymbol{\mu }}_{\boldsymbol{l}\boldsymbol{o}\boldsymbol{w}}.\boldsymbol{P}\boldsymbol{L}\boldsymbol{C}\right)=\boldsymbol{K}\boldsymbol{d}\left({\boldsymbol{\mu }}_{\boldsymbol{l}\boldsymbol{o}\boldsymbol{w}}\boldsymbol{M}{\bf{1}}\boldsymbol{\beta }.\boldsymbol{P}\boldsymbol{L}\boldsymbol{C}\right) |
Functional relevance | {R}_{HP4H}\left(t\right)\gg {R}_{CP4H}\left(t\right) | M1\beta |{\mu }_{high}.PLC\to \alpha M1\beta (Anterograde) {M1\beta |\mu }_{low}.PLC\to rM1\beta (Retrograde) |
Note: {\boldsymbol{\mathcal{C}}}_{\boldsymbol{z}\boldsymbol{\mu }} : All pairwise-atom/residue based square interaction matrix of ligand and macromolecule, \left\langle {} \right.\boldsymbol{c}|\boldsymbol{\mu }\left. {} \right\rangle ; \mathit{\boldsymbol{\mathcal{K}}\boldsymbol{\mathcal{C}}}_{\boldsymbol{z}\boldsymbol{\mu }} : Diagonal matrix of the interactions of a ligand and macromolecule; {z}_{i=j}=z : Set of eigenvalues of \mathit{\boldsymbol{\mathcal{K}}\boldsymbol{\mathcal{C}}}_{\boldsymbol{z}\boldsymbol{\mu }} where z\in diag\left(\mathit{\boldsymbol{\mathcal{K}}\boldsymbol{\mathcal{C}}}_{\boldsymbol{z}\boldsymbol{\mu }}\right)\subset \mathbb{C} ; \boldsymbol{K}\boldsymbol{d}\left(\boldsymbol{c}\boldsymbol{\mu }\right) : Set of eigenvalue-based transition-state dissociation constants for monomer- and multimer-forms of ligand-macromolecular complexes, \left\{\omega \in \boldsymbol{K}\boldsymbol{d}\left(\boldsymbol{c}\boldsymbol{\mu }\right)={\alpha }_{i=j}=Re\left(z\right)\in \boldsymbol{K}\boldsymbol{d}\left({\boldsymbol{\mathcal{C}}}_{\boldsymbol{z}\boldsymbol{\mu }}\right)\cap \left(\mathrm{0, 1}\right)\right\} ; {\mu }_{high}, {\mu }_{low} : High- and low-affinity variants of an arbitrary ligand, \mu =\{{\mu }_{high}, {\mu }_{low}\}\in {\boldsymbol{\mathcal{L}}} ; \boldsymbol{\mu }{\boldsymbol{\mathcal{C}}}_{\boldsymbol{z}}.{{\mathcal{C}}}^{y} : Higher-order complex of ligand and macromolecule; \boldsymbol{K}\boldsymbol{i}\boldsymbol{n}\boldsymbol{f} : Subset of transition-state dissociation constants of ligand-macromolecular interaction; \boldsymbol{K}\boldsymbol{s}\boldsymbol{u}\boldsymbol{p} :Subset of transition-state dissociation constants of ligand-macromolecular interaction; R\left(t\right) : Rate of reaction; HP4H: Hypoxia stimulated- and Collagen Proline 4-Hydroxylase; CP4H: M1\beta : Heterodimer of major histocompatibility complex I (MHC1) with beta-2 microglobulin; PLC: Peptide loading complex. |