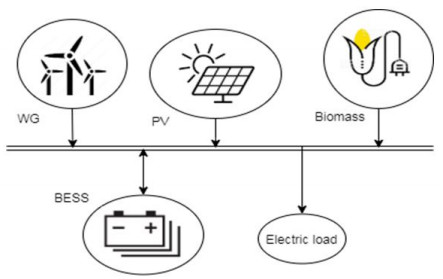
Two handheld near infrared (NIR) spectrometers were used to quantify crude protein (CP) content of mixed forage and feedstuff composed of Sweet Bran, distiller's grains, corn silage, and corn stalk. First was a transportable spectrometer, which measured in the visible and NIR ranges (320–2500 nm) with a spectral interval of 1 nm (H1). Second was a smartphone spectrometer, which measured from 900–1700 nm with a spectral interval of 4 nm (H2). Spectral data of 147 forage and feed samples were collected by both handheld instruments and split into calibration (n = 120) and validation (n = 27) sets. For H1, only absorbances in the NIR region (780–2500 nm) were used in the multivariate analyses, while for H2, absorbances in the second and third overtone regions (940–1660 nm) were used. Principal component analysis (PCA) and partial least squares (PLS) regression models were developed using mean-centered data that had been preprocessed using standard normal variate (SNV) or Savitzky-Golay first derivative (SG1) or second derivative (SG2) algorithm. PCA models showed two major groups—one with Sweet Bran and distillers grains, and the other with corn silage and corn stalk. Using H1 spectra, the PLS regression model that best predicted CP followed SG1 preprocessing. This model had low root mean square error of prediction (RMSEP = 2.22%) and high ratio of prediction to deviation (RPD = 5.24). With H2 spectra, the model best predicting CP was based on SG2 preprocessing, returning RMSEP = 2.05% and RPD = 5.74. These values were not practically different than those of H1, indicating similar performance of the two devices despite having absorbance measurements only in the second and third overtone regions with H2. The result of this study showed that both handheld NIR instruments can accurately measure forage and feed CP during screening, quality, and process control applications.
Citation: Isaac R. Rukundo, Mary-Grace C. Danao, James C. MacDonald, Randy L. Wehling, Curtis L. Weller. Performance of two handheld NIR spectrometers to quantify crude protein of composite animal forage and feedstuff[J]. AIMS Agriculture and Food, 2021, 6(2): 462-477. doi: 10.3934/agrfood.2021027
[1] | S. Vinoth John Prakash, P.K. Dhal . Cost optimization and optimal sizing of standalone biomass/diesel generator/wind turbine/solar microgrid system. AIMS Energy, 2022, 10(4): 665-694. doi: 10.3934/energy.2022032 |
[2] | Aaron St. Leger . Demand response impacts on off-grid hybrid photovoltaic-diesel generator microgrids. AIMS Energy, 2015, 3(3): 360-376. doi: 10.3934/energy.2015.3.360 |
[3] | Mohamed G Moh Almihat . An overview of AC and DC microgrid energy management systems. AIMS Energy, 2023, 11(6): 1031-1069. doi: 10.3934/energy.2023049 |
[4] | Paul K. Olulope, Oyinlolu A. Odetoye, Matthew O. Olanrewaju . A review of emerging design concepts in applied microgrid technology. AIMS Energy, 2022, 10(4): 776-800. doi: 10.3934/energy.2022035 |
[5] | Gustavo Aguilar, Pranjali D. Muley, Charles Henkel, Dorin Boldor . Effects of biomass particle size on yield and composition of pyrolysis bio-oil derived from Chinese tallow tree (Triadica Sebifera L.) and energy cane (Saccharum complex) in an inductively heated reactor. AIMS Energy, 2015, 3(4): 838-850. doi: 10.3934/energy.2015.4.838 |
[6] | W. E. P. Sampath Ediriweera, N. W. A. Lidula, R. Samarasinghe . Ring connected microgrid clusters for improved resiliency in distribution systems with high solar PV penetration. AIMS Energy, 2024, 12(4): 872-904. doi: 10.3934/energy.2024041 |
[7] | Rashid Al Badwawi, Mohammad Abusara, Tapas Mallick . Speed control of synchronous machine by changing duty cycle of DC/DC buck converter. AIMS Energy, 2015, 3(4): 728-739. doi: 10.3934/energy.2015.4.728 |
[8] | Mohamed G Moh Almihat, MTE Kahn . Centralized control system for islanded minigrid. AIMS Energy, 2023, 11(4): 663-682. doi: 10.3934/energy.2023033 |
[9] | Jin H. Jo, Jamie Cross, Zachary Rose, Evan Daebel, Andrew Verderber, David G. Loomis . Financing options and economic impact: distributed generation using solar photovoltaic systems in Normal, Illinois. AIMS Energy, 2016, 4(3): 504-516. doi: 10.3934/energy.2016.3.504 |
[10] | Mohamed Hamdi, Hafez A. El Salmawy, Reda Ragab . Optimum configuration of a dispatchable hybrid renewable energy plant using artificial neural networks: Case study of Ras Ghareb, Egypt. AIMS Energy, 2023, 11(1): 171-196. doi: 10.3934/energy.2023010 |
Two handheld near infrared (NIR) spectrometers were used to quantify crude protein (CP) content of mixed forage and feedstuff composed of Sweet Bran, distiller's grains, corn silage, and corn stalk. First was a transportable spectrometer, which measured in the visible and NIR ranges (320–2500 nm) with a spectral interval of 1 nm (H1). Second was a smartphone spectrometer, which measured from 900–1700 nm with a spectral interval of 4 nm (H2). Spectral data of 147 forage and feed samples were collected by both handheld instruments and split into calibration (n = 120) and validation (n = 27) sets. For H1, only absorbances in the NIR region (780–2500 nm) were used in the multivariate analyses, while for H2, absorbances in the second and third overtone regions (940–1660 nm) were used. Principal component analysis (PCA) and partial least squares (PLS) regression models were developed using mean-centered data that had been preprocessed using standard normal variate (SNV) or Savitzky-Golay first derivative (SG1) or second derivative (SG2) algorithm. PCA models showed two major groups—one with Sweet Bran and distillers grains, and the other with corn silage and corn stalk. Using H1 spectra, the PLS regression model that best predicted CP followed SG1 preprocessing. This model had low root mean square error of prediction (RMSEP = 2.22%) and high ratio of prediction to deviation (RPD = 5.24). With H2 spectra, the model best predicting CP was based on SG2 preprocessing, returning RMSEP = 2.05% and RPD = 5.74. These values were not practically different than those of H1, indicating similar performance of the two devices despite having absorbance measurements only in the second and third overtone regions with H2. The result of this study showed that both handheld NIR instruments can accurately measure forage and feed CP during screening, quality, and process control applications.
In Egypt, many new projects are being performed in various areas, such as those in the new valley of the western desert and those in Egypt's northern coastal borders. These projects will help in the horizontal transferring of people from the cramped Nile valley to other uninhabited different locations to reduce the metropolis's crowdedness as approximately 95% of the country's population live along the sides of the Nile river and inside the Nile Delta [1]. Egypt's electricity consumption grew rapidly in the last few years as a result of economic growth. Still, it remains a challenge for Egypt to provide stable electricity access to rural areas. The population augmentation and the new towns' establishment are presenting an extreme burden on the electrical network. The Egyptian government is trying these days to turn to renewable energy sources to tackle fossil fuel shortage. Egyptian economy balance would be threatened without depending on renewable sources as it will be a great importer of fossil fuels. Integrating renewable energy sources to power systems and microgrids establishment are the best options for handling the electricity crises and decreasing the burden on the grid [2].
Microgrid systems transfer the conventional power system with a single power supply and radial network into a new distribution system with multi-power sources. It can be categorized as isolated or grid-connected, relying on the connection with the public grid [3]. In isolated microgrids, it is essential for each microgrid to self satisfy its load requirements as it is considered a small power system with its own generation, transmission, control and distribution [4]. Renewable energy system is a suitable cheap technological approach for rural electrification. It stimulates the economy and communities to enhance their standards. Small-scale distributed renewable generation systems are considered these days as a vital solution for energy problems [5].
Globally, the total renewable power capacity reached 2195 GW. Renewable energy sector employed around 10.3 million people (directly and indirectly), and approximately $ 280 billion were spent on new investments [6]. Almost 145 countries have implemented policies to promote clean energy technologies over the last few decades. According to the reports of BP Energy (2020), renewable energy (including biofuels) recorded a large increment in energy consumption of (3.2 EJ) in 2019. Egypt has committed to increasing its dependency on sustainable energy technologies. According to the international renewable energy agency's (IRENA) reports, the total combined renewable capacity to the grid was represented by 3.7 gigawatts (GW) in 2018. This amount is composed of 2.8 GW from hydropower and about 0.9 GW from solar and wind power. The Egyptian government aims to have 20% of its electricity from renewable energies by 2022 and 42% in 2035 [7]. Egypt has abundant chances to achieve these goals, as it enjoys plenty of renewable energy resources, including hydropower, wind, solar and biomass. Egypt has a significant potential for investments in solar energy sector because it is one of the Sunbelt countries. Besides, its sunlight period is between 3500 and 4500 hr/year with around 12–30 MJ/m2/day [8]. Egypt is also encouraging wind farms establishment. Its average wind speed is about 10.5 m/s for average 100-meter hub heights.
Hybrid renewable generation offers more flexibility and lower cost alternatives compared to relying upon one source. Countries with a wealth of sunlight radiation, strong potential of bio-degradable waste and a decent wind energy supply can handle the electricity access for far areas. By considering the sustainable energy sources uncertainties (solar, wind, etc.), battery energy storage systems (BESS) and other reservoirs like biogas energy sources are nominated to be integrated with renewable sources to ensure uninterrupted electricity and power security [9].
Biomass is a renewable energy source that can be easily transformed into electricity through the gasification process. Compared with fossil fuel technologies, biomass technology has many environmental and economic advantages. High-capacity biomass gasification units are the best choice because of their investment efficiency and effectiveness. Small-scale biomass gasification is vital in providing electricity for localized usage where the low electrification level and the unstable transmission network [10]. Rice straw is an attractive biomass solid waste due to its availability in rural areas and its high calorific value. Rice is one of the most common crops in Egypt. Open-field burning is the main environmental issue of rice farming in most countries. Farmers have a limited time to clear their fields after the harvest ends. They burn the rice straw to start planting seeds for the next season. This behaviour has many impacts on carbon dioxide (CO2) emissions, which is the main combustion product that creates dark clouds, and plays a major role in children's exacerbating asthma. Managing the straw after rice harvest is a critical problem these days, and one of the appropriate solutions is using it as a fuel in gasification-electricity procedures [11,12].
Many authors have studied the optimum operation of hybrid microgrids, including renewable energy sources. Reference [13] studied the microgrid's short-term economic and environmental dispatch problem using enhanced quantum particle swarm optimization (QPSO) to improve the units' performance that helps to minimize polluting gas emissions. In reference [14], a detailed comparison of three different DC microgrids has been held (one grid-powered based on conventional fuel generation, and the other two off-grid based on solar energy generation). The comparison aimed to highlight the most notable positive and negative aspects regarding technical efficiency, economic and environmental impacts. In reference [15], a detailed literature review of the current microgrid's sizing methods was presented. The surveyed methods were split into two major groups of cost-based and non-cost-based sizing methods. Reference [16] provides a comprehensive overview of the most widely used tools for designing HRESs. It also explains how social factors such as job development and social acceptance improve such software packages' capabilities. Renewable energy integration to replace diesel power generation is projected to be cost-effective for off-grids and substantially reduces greenhouse gas emissions. Hybrid systems are highly recommended for isolated microgrids to guarantee reliability overcome battery problems [17]. In reference [18], the authors explored the possibility and the benefits of merging renewable energy technologies such as solar and wind with traditional power generation systems like diesel and natural gas sources. In [19], the optimum generation capacity and storage for a hybrid (PV/wind/BESS) system based on instantaneous wind speed variations are introduced to maintain system reliability and reduce the investment cost. In reference [20], optimal sizing of PV/diesel/battery system is researched, including V2G. In reference [21], PV/WG isolated microgrid is sized using the critical month by algorithmic optimization techniques and Homer Pro. In reference [22], Grasshopper Optimization Algorithm (GOA) is used in optimal microgrid sizing to supply energy demand reliably based on the deficiency of power supply probability (DPSP) and the cost of energy (COE). Energy storage systems act as a tool for handling peak load demand as well as boosting the gains from the thermal power plant while enhancing the system stability and reliability [23]. Authors in ref [24] studied the integration of BESS in efficient rural electrification. BESS' optimum sizing guarantees the system reliability and extends battery life which affects the replacement cost. In reference [25] general parallel genetic algorithm is used to maximize the technical benefits for the distribution system by optimally integrating PV and energy storage systems.
Biomass is a valuable resource that supplements other renewable sources if it is used in electricity generation. It has zero emissions if combined with technologies for carbon capture and storage [26]. Biomass gasification is a low-cost tool for providing electricity to off-grid rural communities [27]. For the Sharjah town's optimal electrification, micropower optimization methods are used to design a solar/biomass hybrid renewable energy-based microgrid [28]. Reference [29] used rice husk as the basis of energy for paddy parboiling and drying, also studied the worked gasifier's energy, exergy analysis and the gas-fired boiler equipment. Large-scale biomass to hydrogen technology is one of the brightest future technology in the sustainable energy sector [30]. Gasification technology is viewed as an effective thermochemical process either to substitute fossil fuel in existing diesel engines or to produce electricity using gas engines [31]. This technique enables the implementation of electrification programs in rural areas and improves grid quality. Reference [32] has studied the combustion of rice straw to obtain energy and its related problems in China. Roughly 3.1 million tons/year of rice straw in Egypt are projected to be disposed of by burning directly in the open air, so the recycling is an urgent necessity. Reference [33] investigated rice straw's ability for energy production in Egypt. The simulation also illustrated the rice straw power plant's efficiency to determine the required amount of energy, minimize fossil fuels reliance and greenhouse gas emissions reduction.
On the other hand, RESs have been shown to have short-term fluctuations, and their energy profiles are not entirely predictable. Using information from nearby substations improves prediction accuracy and reduced data imputation errors [34]. Uncertainty modelling outlines renewable sources' fluctuations. Reference [35] showed the impacts of load and wind uncertainties in increasing the cost by 22%. In reference [36], the stochastic energy operation management is studied for grid-connected MG, including WT/PV/Micro-Turbine/Fuel Cell and BESS. Scenario-based strategies convert the stochastic problem to several deterministic problems. Monte-Carlo method is a suitable method for scenarios generation [37]. Genetic algorithm and Monte-Carlo method are used in optimal BESS sizing, taking into consideration the wind power uncertainty due to wind speed fluctuation in reference [38]. Optimal design and operation for isolated microgrids are discussed in reference [39], considering solar energy generation's stochastic behaviour. In reference [40], The point estimate method (PEM) is used to view the uncertainty linked to wind and solar powers. Reference [41] studied the consequences of renewable sources, market price and load uncertainties in optimal scheduling of a grid-connected microgrid over a 24-hour horizon, using the modified bat algorithm (MBA). The probability density functions are used in modelling renewable energy sources uncertainty. In reference [42], a multi-scenario optimization model based on the minimum life cycle cost is applied using the general algebraic modelling system (GAMS) to choose the best siting, sizing, and type of renewable sources in the distribution system.
The power sector is essential to Egypt's socioeconomic growth and lowering poverty goals [43]. Depending on the previous literature, this paper presents a renewable energy study for rural communities electrification without grid connections. A comparison between different combinations of sources is also presented, using PV modules, wind, and biogas generators. The main contribution of the proposed scheme in this manuscript depending on all the above, are as follows:
1. An optimal sizing approach of a real new residential area in Egypt using renewable energy sources is proposed.
2. Uncertainty of renewable energy methodology is considered with a complete study for its effects on the optimal sizing and cost schemes.
3. The bio-gasification generation is implemented to deepen its effects on the environment and power system generation.
4. Environmental effects of rice straw recycling through biomass gasification are introduced.
5. Three different operation mechanisms are introduced according to the availability of power conversion sources.
6. Nine case studies with detailed analysis are presented to confirm their used renewable generation robustness and effectiveness.
7. A comparison of two optimization algorithms is held to ensure the system's feasibility.
The frame of the remaining sections is written as follows; in section 2, the system is described while the objective functions are discussed in section 3. In section 4, the system constraints are introduced. Different optimization techniques are discussed in section 5. The system uncertainties are illustrated in section 6. Also, the simulation and comparison between nine optimized scenarios are shown in section 7. The results are discussed in section 8, while the conclusion and future work are then held in section 9.
The proposed system configuration with the available power generation units is presented in Figure 1. The system's operation is categorized into three different operation mechanisms depending on the used units and the storage system availability. The first category uses renewable energies without using batteries. The load generation mismatch is faced through biomass generation. The second category uses a combination of renewable energy sources, BESS, and biomass generation to ensure full system reliability. In the third category, the system consists of renewable energy sources with batteries and without biomass generation availability. Different scenarios of each category are studied in this paper, taking into consideration the system constraints to reach the optimal cost and reliable configuration of each scenario. Also, a comparison between scenarios is held to show the economic feasibility and the environmental response of each system. Figure 2 shows the mechanisms of each category. The system components are discussed in the following paragraphs.
Photovoltaic (PV) is the simplest energy technology to convert solar energy into electricity. It features easy installation, high reliability and low maintenance cost. PV solar modules' power depends mainly on environmental and meteorological data like solar radiation and the daily sun existence duration. The instantaneous generated output power of a PV system (Ppv(t)) with respect to the incident solar irradiance Id(t) in (Wh/m2) can be calculated by using [44]:
Ppv(t)=ηpv∗Spv∗Id(t)Id,Stc | (2.1) |
Where ηpv is PV's efficiency, Spv and Id,Stc are the rated capacity of the PV in kW and solar radiation at the standard test condition, respectively.
The instantaneous wind generator's (WG) output power depends on the wind speed at a specified hub height. The output power of a WG can be described by using the function described in Eq (2.2):
{Swt∗w3−w3cinw3r−w3cot(wcin≤w≤wr)Swt(wr≤w≤wcot)0(w<wcinorw>wr)} | (2.2) |
Where Swt is the rated power capacity of the WG. wcin, wr and wcot are the cut-in, rated and cut-out wind speed, respectively [45].
Biomass gasification is the method of transforming solid bio-waste into an ignitable gas mixture composed mainly of carbon monoxide, oxygen, methane, hydrogen, etc. It can be used for thermal purposes or as a fuel for mechanical or electric power generation in internal combustion engines.
The power of biomass depends mainly on the calorific value and the available biomass amount [46]:
Pbg=Totalbiomassavailable(Ton/yr)∗1000∗CVbm∗ηbm365∗860∗Operatinghours/day | (2.3) |
The biogas operating and maintenance cost includes two parts (fixed and variable cost), depending on the proposed power and the used fuel amount:
OMbg,npv=θ1bg∗Pbg∗N∑j=1(1+μbg1+ir)j+θ2bg∗PWyrbg∗N∑j=1(1+μbg1+ir)j | (2.4) |
The amount of biogas fuel cost is described in Eq (2.5):
Fbg,npv=θ3bg∗BFyrr∗N∑j=1(1+μbg1+ir)j | (2.5) |
The capital and salvage values of biomass unit are described in Eqs (2.6) and (2.7):
Cbg=γbg∗Pbg | (2.6) |
SVbg,npv=λbg∗Pbg∗(1+δ1+ir)N | (2.7) |
Where CVbm is the biomass calorific valve, ηbm is the overall conversion efficiency, θ1bg is the annual fixed operation and maintenance cost ($/kW/year), Pbg is the power produce by biogas generator, ir is the interest rate, μbg is the escalation rate, θ2bg is the variable operation and maintenance cost ($/kWh), PWyrbg is the annual working power of biogas generator (kWh/year), θ3bg is the biomass fuel cost ($/ton), BFyrr is the annual required biomass fuel (ton/year), γbg is the initial cost of biogas system ($/kW), λbg is the resale price of the system ($/kW), and δ is the inflation rate [47].
Battery energy storage systems are used to match the generated energy with the consumed energy and enhance the system's energy balance by storing the energy from different sources such as solar and/or wind generators. For example, excess energy due to high levels of wind speeds or solar radiation will be stored in batteries. This stored energy will be released for smoothing the injected power into the system, especially in low renewable energy capabilities [48].
The charging equation:
SOC(t)=SOC(t−1)∗(1−σ)+(Pg(t)−Pl(t)ηinv)∗ηbc | (2.8) |
The discharging equation:
SOC(t)=SOC(t−1)∗(1−σ)+(Pl(t)ηinv−Pg(t))/ηbd | (2.9) |
Eqs (2.10) and (2.11) describe the capital and replacement cost values of BESS:
CBat=NBat∗αBat | (2.10) |
Rnpv,bat=NBat∗αbat∗∑j=5,10,15(1+μbat1+ir)j | (2.11) |
Where SOC is the state of charge, σ is the hourly rate of self-discharge, Pl(t) is the load demand, Pg(t) is the generated power, NBat is the number of battery units, ηbc is the charging efficiency, ηbd is the discharging efficiency, μbat is the escalation rate, αbat is the cost of battery set in $ and ηinv is the inverter efficiency [49].
The optimum system sizing's objective functions are the minimization of load-generation mismatch (loss of power supply LPS) and the total system cost minimization. The first objective function is described in the following equation:
F1=min:(LPS(t))=min:(|Pl(t)−(Pg(t))|) | (3.1) |
If the system uses BESS, the first objective function will be expressed as :
F1=min:(LPS(t))=min:(|Pl(t)−(Pg(t)+SOC(t−1)−SOC(t))|) | (3.2) |
Where Pg(t) and Pl(t) are the summation of the generated power and load power, respectively.
The total cost minimization is the second objective function:
F2=min:(cost)=min:∑Ck+OMk+Rk−SVk∀k∈PV,W,Bat,Bio | (3.3) |
Where Ck is the capital cost of component k, OMk is the operating and maintenance cost of component k, Rk is the replacement cost, and SVk is the salvage cost of each component.
For optimal sizing and achieving all objective functions, the system is subjected to some restrictions.
The limits constraints of maximum and minimum power values of each component are represented in Eqs (4.1)–(4.4):
0≤PPV≤PPv,max | (4.1) |
0≤PW≤PW,max | (4.2) |
0≤Pbio≤Pbio,max | (4.3) |
0≤NBat≤NBat,max | (4.4) |
Battery's SOC defines the remaining power and reflects battery effectiveness. At any time, the value of SOC has to be within the specified limits (20% and 80% for most control strategies) to prevent batteries' damage, secure battery, avoid over-discharging, and increase battery life-time [50,51]. The state of charge is controlled within limits for also dealing with the sudden fluctuations and changes in renewable energies, especially for deterministic scenarios [52].
SOCmin≤SOC(t)≤SOCmax | (4.5) |
where
SOCmin=0.2∗NBat∗Cb | (4.6) |
SOCmax=0.8∗NBat∗Cb | (4.7) |
The total generated power by the available generating units of each scenario, such as WG, PV, biogas generator and ESS should always meet the load demand requirements in all considered scenarios. Eq (4.8) shows the sum of available PV and WG powers at each time, depending on their availability in each scenario configuration. Eqs (4.9)–(4.11) show the power balance constraint of each category.
Pgr(t)=Pw(t)+PPV(t) | (4.8) |
For category (A):
Pgr(t)+Pbio(t)=PL(t) | (4.9) |
For category (B):
Pgr(t)+Pbio(t)+PdisBESS(t)−PchBESS(t)=PL(t) | (4.10) |
For category (C):
Pgr(t)+PdisBESS(t)−PchBESS(t)=PL(t) | (4.11) |
Multi-objective optimization methods are used in this paper to consider all potential trade-offs between different objective functions, which are normally conflicting. Two optimization algorithms are discussed in this paper to find the optimum solution. Each algorithm uses the available input data (load demand profile, generating components, available resources and weather data) to achieve all the system's objectives. Optimal system configuration determines optimal system size, net present cost, total capital cost and the corresponding CO2 reduction. The architecture of the optimization tool is illustrated in Figure 3.
Multi-objective genetic algorithm is a meta-heuristic mechanism motivated by the natural selection technique, which is a part of larger classes of evolutionary algorithms. Genetic algorithms are widely used and biologically inspired by developers to produce high-quality optimization and search prospects, like mutation, crossover and selection.
MOGA utilizes a weighted sum of various objective functions in the selection stage and merges them into a scalar fitness function. The design characteristic of the various objective functions weights are not specified and randomly changed through each selection. Thus the search orientation in this algorithm is not fixed.
At each generation over the process of MOGA, an empirical series of pareto optimal solutions are stored and updated. Also, a certain number of solutions are picked at random from the series. Those solutions are considered elite individuals. The elite mechanism has the benefit of preserving the diversity of each population [53].
The block diagram of the proposed MOGA algorithm is shown in Figure 4, and it is described below:
● Stage 1 (Initialization): generate an initial population.
● Stage 2 (Evaluation): calculate the values of the objective functions for the created population.
● Stage 3 (Selection): use random weights to determine each population's fitness value, then pick a pair of strings from the existing population.
● Stage 4 (Crossover and Mutation): a crossover strategy is implemented for each chosen pair to produce a new population via the crossover process. After that, the mutation process is carried out.
● Stage 5 (Elitist): delete some strings of created strings haphazardly and substitute them with elite strings picked at random from temporary optimal pareto solutions.
● Stage 6 (Termination): if the stopping requirement is not satisfied, go to Stage 2.
● Stage 7 (Optimal Solution): the MOGA suggests the preferable options.
The ε−multi-objective genetic algorithm depends on the vision of ε−dominance to test the optimisation's stored results in the A(t) archive. ε−pareto set (x∗) is produced, and it seeks to reach the optimal pareto front F(x∗) with bounded memory sources. Furthermore, It continuously changes the pareto front boundaries and avoids any loss of the solutions belonging to the front ends. The objective space is sectioned to a particular number of boxes Nbox which can be calculated from:
Nbox=Fmaxi−Fminiε,Fmaxi=maxF(x)i,Fmini=minF(x)i,∀x∈X∗ | (5.1) |
where ε is the box's width and i∈ [1, 2....., N].
Each box can be filled with only one solution, which helps to preserve the divergence of F(x∗). For a specific solution, the boxi(x) is defined by:
boxi(x)=Fi(x)−FminiFmaxi−Fmini∗Nbox | (5.2) |
A solution with x1ε-dominates x2 where x1<εx2, when and only if
(box(x1)<box(x2)∨(box(x1)=box(x2)andx1<x2) | (5.3) |
Thus, a set x∗∈x is ε-pareto when and only if
∀x1,x2∈x∗,x1≠x2,box(x1)≠box(x2)∧box(x1)>εbox(x2) | (5.4) |
A(t) is always updated by ε-dominant solutions which do not share the same box. The remaining solution always occupies the center of the box to accomplish the prevention of solutions belonging to neighboring boxes and increase the solution variety [54,55]. ε's goal is to reach X∗ with as many solutions as possible to adequately characterize the pareto front, which is bounded by:
Nsmax=ΠNi=1Nboxi+1Nmaxbox+1,Nmaxbox=max[Nboxi] | (5.5) |
Three populations are generated To ensure solution variance. P(t) is the main population, Q(t) is the auxiliary population, and A(t) represents the archive of the best solutions. The proposed algorithm's principal steps are as follows:
● Step 1: Begin with creating empty A(t).
● Step 2: Initializing the base population at random P(0).
● Step 3: Calculate the corresponding function value P(t).
● Step 4: Test the applicable values to be included in A(t).
● Step 5: Generate Q(t) by using two random individuals from P(t) and A(t).
● Step 6: Calculate the corresponding function value for each individual in Q(t).
● Step 7: According to the objective space location, test which individual can be added to A(t).
● Step 8: Update P(t) with individuals from G(t) by randomly comparing each value of G(t) with selected individuals of P(t).
● Step 9: Eventually, the pareto front's smart characterisation is formed by individuals from A(t).
Because of weather effects on renewable energy, it became increasingly difficult to guarantee the system's reliability. Therefore, it is necessary to solve this issue by introducing PV and wind sources' uncertainty that primarily depend on weather conditions. Monte-Carlo Simulation (MCS) or Monte-Carlo approach provides an appropriate solution to quantitative issues by carrying out statistical sampling procedures.
A variety of random configurations may be used in this case to produce data and samples to reflect the solar radiation and wind speed variation. Each input is independently sampled to construct a random sequence {xi}Ni=1 from Ud(0, 1) with estimated mean of [56]:
¯x=1NN∑i=1xi | (6.1) |
The general approach of solving stochastic formulations is to transform stochastic models into deterministic problems. Monte Carlo approach succeeds in representing uncertainties by inputs sampling and defining them through the probabilities distribution functions [57,58].
MCS is conducted to generate a finite number of possible scenarios based on the probability distributions for all stochastic parameters [59], as shown in Figure 5. The microgrid sizing procedures with MCS used the same parameters of the deterministic problem formulation and can be described as follows [38]:
● Step 1: Initialize algorithm parameters.
● Step 2: Apply Monte-Carlo simulation techniques to produce plentiful different scenarios with the same probability.
● Step 3: In each scenario, the optimum size is calculated by operating strategy.
● Step 4: Test the constraint satisfaction to create the next generation and jump to step 5.
● Step 5: Repeat step 2 to 4 till the specific iteration step is completed, or other termination conditions are met.
Figure 6 shows fifty uncertain-based scenarios for wind speed and solar radiation.
Two optimization techniques are applied for nine scenarios of the microgrid to ensure load satisfactions. The comparison between these techniques is shown in Table 1. For comparison, the same systems are also studied in deterministic and stochastic cases to show the uncertainties effects in selecting the appropriate capacities of generating units, storage systems, and proper biomass generation implementation. Table 2 shows the stochastic results. The economic and technical specifications of the proposed system's components are listed in Table 3. In this work, the optimization and Monte Carlo simulation have been coded in MATLAB software.
Cost | PV | WG | No of | Pbiomass | ||
($) | (KW) | (KW) | Batteries | (max) KW | ||
Category A | ||||||
Scenario 1 | MOGA | 1.787∗108 | 100.072 | 0 | 0 | 321.810 |
(PV+Biomass) | ϵ−MOGA | 1.789∗108 | 99.883 | 0 | 0 | 328.254 |
Scenario 2 | MOGA | 1.539∗108 | 0 | 705.943 | 0 | 277.906 |
(WG+Biomass) | ϵ−MOGA | 1.540∗108 | 0 | 705.770 | 0 | 278.426 |
Scenario 3 | MOGA | 1.480∗108 | 21.118 | 678.794 | 0 | 279.669 |
(PV+WG+Biomass) | ϵ−MOGA | 1.479∗108 | 20.052 | 684.906 | 0 | 280.355 |
Category B | ||||||
Scenario 4 | MOGA | 3.455∗107 | 462.114 | 0 | 23 | 94.150 |
(PV+Biomass+BESS) | ϵ−MOGA | 2.647∗107 | 488.195 | 0 | 22 | 328.917 |
Scenario 5 | MOGA | 6.649∗107 | 0 | 1883.090 | 14 | 95.797 |
(WG+Biomass+BESS) | ϵ−MOGA | 2.953∗107 | 0 | 2297.645 | 14 | 303.548 |
Scenario 6 | MOGA | 6.030∗107 | 413.147 | 292.798 | 24 | 88.689 |
(PV+WG+Biomass+BESS) | ϵ− MOGA | 3.236∗107 | 0 | 2500 | 17 | 330 |
Category C | ||||||
Scenario 7 | MOGA | 4.040∗106 | 535.060 | 0 | 22 | 0 |
(PV+BESS) | ϵ−MOGA | 3.359∗106 | 474.495 | 0 | 18 | 0 |
Scenario 8 | MOGA | 9.397∗106 | 0 | 2469.141 | 13 | 0 |
(WG+BESS) | ϵ−MOGA | 9.397∗106 | 0 | 2469.182 | 13 | 0 |
Scenario 9 | MOGA | 5.599∗106 | 562.579 | 272.012 | 27 | 0 |
(PV+WG+BESS) | ϵ−MOGA | 5.204∗106 | 634.020 | 0 | 29 | 0 |
Configuration | Cost | PV | WG | No of | Pbiomass | |
($) | (KW) | (KW) | Batteries | (max) KW | ||
Category A | ||||||
Scenario 1 | PV+Biomass | 1.911∗108 | 77.534 | 0 | 0 | 322.528 |
Scenario 2 | WG+Biomass | 1.933∗108 | 0 | 317.317 | 0 | 329.821 |
Scenario 3 | PV+WG+Biomass | 1.890∗108 | 42.377 | 182.208 | 0 | 320.965 |
Category B | ||||||
Scenario 4 | PV+Biomass+BESS | 8.979∗107 | 501.204 | 0 | 33 | 191.922 |
Scenario 5 | WG+Biomass+BESS | 1.246∗108 | 0 | 1698.970 | 30 | 155.320 |
Scenario 6 | PV+WG+Biomass+BESS | 7.533∗107 | 458.217 | 701.070 | 42 | 103.754 |
Category C | ||||||
Scenario 7 | PV+BESS | 4.327∗106 | 504.755 | 0 | 25 | 0 |
Scenario 8 | WG+BESS | 1.007∗107 | 0 | 2166.951 | 25 | 0 |
Scenario 9 | PV+WG+BESS | 7.912∗106 | 773.901 | 9.108 | 47 | 0 |
Component and Economic Specification | ||
Discount rate (r) | 5% | |
Escalation rate | 7% | |
PV Module | ||
Investment cost | 1690 | $/KW |
Maintenance | 26 | $/KW/yr |
(PV) reduction factor | 84% | |
lifetime | 25 | years |
Wind Generator | ||
Investment cost | 2030 | $/KW |
Operating and Maintenance | 75 | $/KW/yr |
lifetime | 25 | years |
Cut-in wind speed | 4 | m/s |
Cut-out wind speed | 25 | m/s |
Rated wind speed | 14.5 | m/s |
Shear coefficient | 0.14 | |
Turbine hub height | 50 | m |
BESS | ||
Investment cost | 200 | $/KWh |
Replacement cost | 200 | $/KWh |
Self discharge rate | 0.01% | |
Efficiency | 90% | |
Life time | 5 | years |
Biomass generator | ||
Investment cost(γbg) | 6200 | $/KWh |
Annual fixed operation and maintenance cost of biomass system(θ1bg) | 0.05*γbg | $/Kw/year |
Variable operation and maintenance cost of biogas system(θ2bg) | 0.0042 | $/Kw/year |
Biomass fuel cost of biogas system(θ2bg) | 35 | ($/t/year) |
Biomass system resale price(λbg) | 0.3*γbg | ($/Kw) |
Electrical conversion efficiency(ηbm) | 0.21 |
In this category, there is no storage system. All available generated power is used directly to fulfil load requirements. Three combinations of generating units are studied in this category. Scenario (1) consists of PV with biomass, while scenario (2) consists of WG with biomass, and scenario (3) consists of a combination of PV and WG with biomass generator.
Figures 7–9 show the generation load mismatch and the energy share of each generating unit for each scenario. The total cost of each scenario is plotted in Figure 10 to show the comparison between scenarios and show the percentages of capital cost with running cost.
Category (B) represents batteries and biomass generation's participation in the same configuration with other renewable sources. Scenario (4) consists of PV with biomass and BESS units. Scenario (5) integrates batteries with WG and biomass generators. Scenario (6) represents the share of all available system components (PV, WG, BESS and biomass).
Figures 11–13 show the generation load mismatch and each generating unit's energy share for scenarios 4–6, respectively. Figure 14 shows the total system cost for each of category B' scenarios.
This category is considered the most simple and economical category, but it has the lowest environmental concern of CO2 emissions and rice straw problems. In this kind of systems, there is no existence of biomass technology. The power generation depends mainly on a combination of PV and WG. Figures 15–17 show the generation load mismatch and each generating unit's energy share for scenarios 7–9, respectively. The capital cost and operating with maintenance cost of this category are shown in Figure 18.
In all scenarios, the load is met with optimal system size using two multi-optimization techniques. It is found that the results of the two optimization techniques have some similarities in some cases and differences in other scenarios. Thus, the simulation results for the nine scenarios confirm the following features:
1. Scenario 7 is the cheapest scenario between all discussed scenarios by a total system cost of $ 4.040 million with a PV capacity of 535.060 kWh and a total number of 22 battery sets. In contrast, PV without BESS scenario is not recommended because it is the highest cost scenario. It does not use the available capabilities of solar energy due to the lack of energy storage systems. However, it would be applicable in case of applying load demand control strategies.
2. ε-MOGA gives almost nearly equal results as MOGA for category A and C, but it gives different results for category B. It uses biomass generators with high capacity in category B, but it is used for a limited no of hours during the day, which causes the low cost of fuel and low corresponding total cost. Although the results of ε-MOGA for category B seems to be better than MOGA, it is not accepted as biomass generator is planned to be used for a limited number of hours during the day. It can not be used as a backup generator because biomass units take time to be inserted to the system.
3. Without using BESS as in category A, the amount of biomass share is very high. It reaches 81% in scenario 1 as the PV is the only generating system with biomass. Besides, PV does not work for more than thirteen hours of the all-day hours, as seen in Figure 7a. Introducing wind generators in category (A) is noticed to be more dependable as it exists during all-day hours. Although wind speed is not high at all times, its existence is better than solar existence. Biomass share is reduced to around 69% in scenario 2 and 3, which reduces the cost and increases the share of other generating units like WG in scenario (2) and both PV and WG in Scenario 3.
4. Category (B) is considered a mix between category (A) and (C). Both BESS and biomass are considered in this category. It is the most reliable category as BESS enhances the system performance in fluctuated weather conditions. In addition, bio-gas can be considered a backup generation in case of load increase or other generating unit outages. In this category, the percentage of biomass's share is decreased, and the percentage share of other renewable sources is increased. Wind generation share is about 75% and 50% in scenarios 5 and 6, respectively. While PV energy share is about 86% and 35% in Scenarios 4 and 5. Biomass energy share in scenario 4 is the same as scenario 6 by a value of 14%. This percentage increases in the case of PV absence, as in scenario 5.
5. From deterministic results, PV and wind power capacities increased by the integration of BESS. By comparing category A with category B, PV increased by (3.6%) from scenario 1 to scenario 4. Also, WG capacity increased by (1.6%) from scenario 2 to scenario 4. In scenario 3, BESS integration increases PV capacity by (392.029 kWh) and decreases WG capacity by (386.004 kWh) as noticed from scenario 6's results.
6. From shown results in table 1, if BESS replaces biomass units in category A as in Category C, the cost of scenarios 1–3 will be decreased by ($ 174.66 million), ($ 144.503 million) and ($ 142.401 million) respectively. Removing biomass generators from category B reduces the total system cost and increases other renewable sources' share. By comparing scenario 4 with 7 and 5 with 8, PV power increased by (72.9 kWh), and wind power increased by (586.051 kWh). Also, by comparing scenario 6 with scenario 9, PV power is increased, and WG power is decreased like what happened in comparing scenarios 3 and 6.
7. PV energy share represents 100% in scenario 7, and WG represents the same amount in scenario 8. It is found that category c is the most economically effective category as it recorded the least cost between other categories with about total cost of ($ 4.040 million), ($ 9.397 million) and ($ 5.599 million) for scenarios 7–9, respectively.
8. In scenario 9, the power share of PV is nine times the power share of wind. In the case of no storage, it is not economical to use a high PV amount.
9. When comparing scenario 7 with scenario 8, PV introduction is better than WG, with a cost reduction of about (57%). In comparison, (PV+WG+BESS) scenario is noticed to have ($ 1.559 million) over cost compared to scenario 7.
10. Biomass generation cost is high due to the high operating cost, including fuel, operating and maintenance costs. The high cost of Categories A and B illustrates the high use of biomass generation, as shown in Figures 10 and 14. Differently, the capital cost in category C's scenarios represents a significant percentage of the total system's cost, including the installation and establishment of the system, as seen in Figure 18.
11. (PV + BESS) is the best economical choice for sizing, but it is still unreliable if the weather changes as there is only a single generating source. In contrast, applying (PV with Biomass) is the highest cost scenario due to the storage system absence, so all power is fed at night from the biomass system, which is costly.
12. Biomass is an expensive choice for electricity generation, but it is an environmentally friendly choice that increases system reliability, especially in critical situations. It can be used as a backup in case of generation shortage. It is not economically recommended to use biomass generators alone without other generation sources. Hybrid renewable energy is the best energy choice for planning isolated microgrid as it leans on different types of sources. Moreover, renewable sources with storage systems are considered the best reliable option.
13. Using biomass for electrical generation is not effective only as a power source. It is considered a recycling process to get rid of unwanted waste. Rice straw using as biomass fuel helps in different environmental trends like clean electricity production, recycling process and CO2 emission reduction instead of burning after harvest. The amount of rice straw satisfies the CO2 emissions reduction.
14. Category (A) has the highest amount of biomass share, so it has the highest value of CO2 reduction. Still, it is not economically to be applied, although it is the most eco-friendly category to reduce rice straw burning's threats. This category does not enhance or make the best benefits of renewable energy sources (PV and WG) due to the lack of storage systems.
15. Scenario 1 represents the highest dependence on rice straw of about (3.1∗105 tons). Scenario 2's amount is near to scenario 3's amount with around (2.6∗105 tons). The amount of rice straw for category (B) is less than category (A). Scenario 4 is the least dependant scenario on the biomass of category B's scenarios.
16. Category (C) is the cheapest microgrid sizing strategy, especially using (PV only with BESS). Due to the absence of biomass in this strategy, there is no CO2 emission reduction from agricultural waste recycling.
17. The rating of biomass gasifiers is increased in all scenarios when comparing deterministic and stochastic results except scenario (1), which is almost the same as this scenario depends mainly on biomass generation. In addition, the number of battery sets increased in all scenarios by ratios between (0.13%) and (1.14%). It helps to overcome the uncertainties of renewable energies through the use of reserved power during unexpected bad weather periods.
18. Besides the increase in the energy storage or biomass capacities in most scenarios, PV and WG capacities are decreased as the sources uncertainties affect their productivity. Systems depend mainly on using extra power during good weather conditions or low load periods for charging batteries to enable its reuse during unexpected periods of low PV or WG generation capability. Furthermore, biomass generation guarantees more system reliability by having backup or controllable power sources in the worst conditions.
19. All scenarios cost increased by applying Monte-Carlo simulation to all scenarios to consider renewable energy uncertainties in the sizing process. The minimum cost increase is in scenario 7 by ($ 0.287 million). Whereas Scenario 5 has a maximum increase of about ($ 58.11 million) due to the increase of batteries and biomass share.
20. By taking into consideration renewable energy uncertainty, the cost increased by ($ 12.4 million), ($ 39.4 million) and ($ 41 million) for scenarios 1–3 respectively. Moreover, the solar and wind power capacities decreased by (22.538 kWh), (388.626 kWh) and (475.324 kWh). In contrast, the biomass share increased to handle the shortage of power. WG's use in scenario 9 is highly decreased, and the scenario depends mainly on PV power and storing it for low radiation periods. Furthermore, scenario 8's cost increased by ($ 0.673 million), the number of batteries is almost doubled, and the wind generation rating decreased by 302.19 kWh.
This paper proposed a 100 % clean microgrid from renewable resources. Multi-objective optimization techniques are utilized for sizing the system with minimizing load-generation mismatch and total system cost minimization as objective functions. Nine scenarios classified into three categories are presented to meet the load requirements. The first category is considered the basic case study with biomass generation implementation and without storage systems. BESS is presented in category B. Category C is considered the cheapest category without biomass generation integration and without consideration to the environmental impacts of biomass use.
Renewable energy is the prospective key for reducing the dependence on harmful fossil fuels in power sectors. Also, biomass is considered the future of recycling and energy production. The on-site generation will be cost-effective to reduce the operating and transportation cost of biomass fuel, so it is preferred for small-scale ranges in isolated microgrids. Biomass generation is costly compared to other generation units. Still, it has two fundamental preferences: it provides reliable generation, especially in considering solar and wind uncertainties. It also presents a recycling option for solving the threatening environmental problem of rice straw disposal in agricultural communities instead of open-air burning. Simulation results confirm that solar energy with a storage system is the best-preferred economical option. Moreover, the hybrid system of scenario 6 gives the reasonable ecological configuration of (PV/WG/BESS/Biomass), especially for sources uncertainties consideration. Applying demand response programs with a detailed feasibility study of its effect on enhancing the energy use and optimal generation sizing is the planned future work.
The authors declare no conflicts of interest.
[1] | Roberts CA, Stuth J, Flinn P (2004) Analysis of forages and feedstuffs, In: Roberts CA, Jr. JW, Ⅲ JBR (Eds.), Near‐Infrared Spectroscopy in Agriculture, American Society of Agronomy, Inc., Crop Science Society of America, Inc., Soil Science Society of America, Inc., 229-267. |
[2] |
Shenk JS, Westerhaus MO (1985) Accuracy of NIRS instruments to analyze forage and grain. Crop Sci 25: 1120-1122. doi: 10.2135/cropsci1985.0011183X002500060054x
![]() |
[3] | Barton FE, Windham WR (1988) Determination of acid-detergent fiber and crude protein in forages by near-infrared reflectance spectroscopy: collaborative study. J Assoc Off Anal Chem 71: 1162-1167. |
[4] |
Smith KF, Flinn PC (1991) Monitoring the performance of a broad-based calibration for measuring the nutritive value of two independent populations of pasture using near infrared reflectance (Nir) spectroscopy. Aust J Exp Agric 31: 205-210. doi: 10.1071/EA9910205
![]() |
[5] | Crocombe RA, Flanders DC, Atia W (2004) Micro-optical instrumentation for process spectroscopy, In: Smith LA, Sobek D (Eds.), Lab-on-a-Chip: Platforms, Devices, and Applications, International Society for Optics and Photonics, 11. |
[6] |
Capitán-Vallvey LF, Palma AJ (2011) Recent developments in handheld and portable optosensing-A review. Anal Chim Acta 696: 27-46. doi: 10.1016/j.aca.2011.04.005
![]() |
[7] |
Crocombe RA (2018) Portable spectroscopy. Appl Spectrosc 72: 1701-1751. doi: 10.1177/0003702818809719
![]() |
[8] |
Stark E, Luchter K (2005) NIR instrumentation technology. NIR news 16: 13-16. doi: 10.1255/nirn.855
![]() |
[9] |
Beć KB, Grabska J, Siesler HW, et al. (2020) Handheld near-infrared spectrometers: Where are we heading? NIR news 31: 28-35. doi: 10.1177/0960336020916815
![]() |
[10] |
Blanco M, Villarroya I (2002) NIR spectroscopy: A rapid-response analytical tool. TrAC-Trends Anal Chem 21: 240-250. doi: 10.1016/S0165-9936(02)00404-1
![]() |
[11] |
Shenk JS, Landa I, Hoover MR, et al. (1981) Description and evaluation of a near infrared reflectance spectro‐computer for forage and grain analysis. Crop Sci 21: 355-358. doi: 10.2135/cropsci1981.0011183X002100030001x
![]() |
[12] | Williams PC (1975) Application of near infrared reflectance spectroscopy to analysis of cereal grains and oilseeds. Cereal Chem 57: 561-576. |
[13] |
Valdes EV, Young LG, Leeson S, et al. (1985) Application of near infrared reflectance spectroscopy to analyses of poultry feeds. Poult Sci 64: 2136-2142. doi: 10.3382/ps.0642136
![]() |
[14] | Paz CC da, Maciel e Silva AG, Rêgo AC do (2019) Use of near infrared spectroscopy for the evaluation of forage for ruminants. Rev Ciências Agrárias 62. |
[15] |
Landau S, Glasser T, Dvash L (2006) Monitoring nutrition in small ruminants with the aid of near infrared reflectance spectroscopy (NIRS) technology: A review. Small Rumin Res 61: 1-11. doi: 10.1016/j.smallrumres.2004.12.012
![]() |
[16] |
Reeves JB (1994) Near-versus mid-infrared diffuse reflectance spectroscopy for the quantitative determination of the composition of forages and by-products. J Near Infrared Spectrosc 2: 49-57. doi: 10.1255/jnirs.31
![]() |
[17] |
Monrroy M, Gutiérrez D, Miranda M, et al. (2017) Determination of Brachiaria spp. forage quality by near-infrared spectroscopy and partial least squares regression. J Chil Chem Soc 62: 3472-3477. doi: 10.4067/S0717-97072017000200010
![]() |
[18] | Valdes E V., Young LG, Mcmillan I, et al. (1985) Analysis of hay, haylage and corn silage samples by near infrared reflectance spectroscopy. Can J Anim Sci 65: 753-760. |
[19] |
de Boever JL, Cottyn BG, Vanacker JM, et al. (1995) The use of NIRS to predict the chemical composition and the energy value of compound feeds for cattle. Anim Feed Sci Technol 51: 243-253. doi: 10.1016/0377-8401(94)00695-6
![]() |
[20] |
De Boever JL, Cottyn BG, De Brabander DL, et al. (1997) Prediction of the feeding value of maize silages by chemical parameters, in vitro digestibility and NIRS. Anim Feed Sci Technol 66: 211-222. doi: 10.1016/S0377-8401(96)01101-7
![]() |
[21] |
Albanell E, Plaixats J, Ferret A, et al. (1995) Evaluation of near-infrared reflectance spectroscopy for predicting stover quality trait in semi-exotic populations of maize. J Sci Food Agric 69: 269-273. doi: 10.1002/jsfa.2740690302
![]() |
[22] |
Volkers KC, Wachendorf M, Loges R, et al. (2003) Prediction of the quality of forage maize by near-infrared reflectance spectroscopy. Anim Feed Sci Technol 109: 183-194. doi: 10.1016/S0377-8401(03)00173-1
![]() |
[23] |
Choi SW, Park CH, Lee CS, et al. (2013) Prediction of chemical composition in distillers dried grain with solubles and corn using real-time near-infrared reflectance spectroscopy. J Korean Soc Grassl Forage Sci 33: 177-184. doi: 10.5333/KGFS.2013.33.3.177
![]() |
[24] |
Danieli PP, Carlini P, Bernabucci U, et al. (2004) Quality evaluation of regional forage resources by means of near infrared reflectance spectroscopy. Ital J Anim Sci 3: 363-376. doi: 10.4081/ijas.2004.363
![]() |
[25] |
González-Martín I, Á lvarez-García N, Hernández-Andaluz JL (2006) Instantaneous determination of crude proteins, fat and fibre in animal feeds using near infrared reflectance spectroscopy technology and a remote reflectance fibre-optic probe. Anim Feed Sci Technol 128: 165-171. doi: 10.1016/j.anifeedsci.2005.11.007
![]() |
[26] | AOAC (1965) Official Methods of Analysis. AOAC Off Methods Anal. |
[27] | AOAC (1990) Official Method 990.03. AOAC Off Methods Anal. |
[28] | R. Rukundo I, C. Danao M-G, B. Mitchell R, et al. (2020) Effect of scanning samples through polypropylene film on predicting nitrogen content of forage using handheld NIR. AIMS Agric Food 5: 835-849. |
[29] |
Martens H, Martens M (2000) Modified Jack-knife estimation of parameter uncertainty in bilinear modelling by partial least squares regression (PLSR). Food Qual Prefer 11: 5-16. doi: 10.1016/S0950-3293(99)00039-7
![]() |
[30] |
Verboven S, Hubert M, Goos P (2012) Robust preprocessing and model selection for spectral data. J Chemom 26: 282-289. doi: 10.1002/cem.2446
![]() |
[31] | Williams P (2001) Implementation of near-infrared technology, In: Williams PC, Norris K (Eds.), Near-Infrared Technology in the Agricultural and Food Industries, St. Paul, American Association of Cereal Chemists, 145-169. |
[32] | Williams P (2014) The RPD Statistic: A Tutorial Note. NIR news 25: 22-26. |
[33] |
Weinberg ZG, Szakacs G, Ashbell G, et al. (2001) The effect of temperature on the ensiling process of corn and wheat. J Appl Microbiol 90: 561-566. doi: 10.1046/j.1365-2672.2001.01276.x
![]() |
[34] |
Yitbarek MB, Tamir B (2014) Silage additives: review. Open J Appl Sci 04: 258-274. doi: 10.4236/ojapps.2014.45026
![]() |
[35] | Chen H (2015) Integrated industrial lignocellulose biorefinery chains, In: Chen H (Ed.), Lignocellulose Biorefinery Engineering, Elsevier, 219-245. |
[36] | Zentek J, Knorr F, Mader A (2013) Reducing waste in fresh produce processing and households through use of waste as animal feed, Global Safety of Fresh Produce: A Handbook of Best Practice, Innovative Commercial Solutions and Case Studies, Elsevier Ltd., 140-152. |
[37] | Rausch KD, Hummel D, Johnson LA, et al. (2018) Wet milling: The basis for corn biorefineries, Corn: Chemistry and Technology, 3rd Edition, Elsevier, 501-535. |
[38] |
Yu P, Nuez-Ortín WG (2010) Relationship of protein molecular structure to metabolisable proteins in different types of dried distillers grains with solubles: A novel approach. Br J Nutr 104: 1429-1437. doi: 10.1017/S0007114510002539
![]() |
[39] |
Chang C-W, Laird DA, Mausbach MJ, et al. (2001) Near-infrared reflectance spectroscopy-principal components regression analyses of soil properties. Soil Sci Soc Am J 65: 480-490. doi: 10.2136/sssaj2001.652480x
![]() |
[40] |
Andueza D, Picard F, Jestin M, et al. (2011) NIRS prediction of the feed value of temperate forages: Efficacy of four calibration strategies. Animal 5: 1002-1013. doi: 10.1017/S1751731110002697
![]() |
[41] |
Jones GM, Wade NS, Baker JP, et al. (1987) Use of near infrared reflectance spectroscopy in forage testing. J Dairy Sci 70: 1086-1091. doi: 10.3168/jds.S0022-0302(87)80115-7
![]() |
[42] | Yu-meng L, Zhong-ping Y (2009) Prediction of Fermentation Qualities of Baled Corn Stalk Silage with Near Infrared Reflectance Spectroscopy.pdf. Asian Agric Res 1: 32.34. |
[43] | Marten GC, Shenk JS, Barton FE (1989) Near infrared reflectance spectroscopy (NIRS): analysis of forage quality, U.S. Dept. of Agriculture, Agricultural Research Service. |
[44] | Stuth J, Jama A, Tolleson D (2003) Direct and indirect means of predicting forage quality through near infrared reflectance spectroscopy, Field Crops Research, Elsevier, 45-56. |
[45] | Shenk JS, Westerhaus MO (1994) The Application of near Infrared Reflectance Spectroscopy (NIRS) to Forage Analysis, In: Fahey GC (Ed.), Forage Quality, Evaluation, and Utilization, American Society of Agronomy, Inc. Crop Science Society of America, Inc. Soil Science Society of America, Inc, 406-449. |
[46] | Shenk JS, Westerhaus MO, Fahey GC, et al. (1994) The application of near infrared reflectance spectroscopy (NIRS) to forage analysis, In: Fahey GC (Ed.), Forage Quality, Evaluation, and Utilization, American Society of Agronomy, Crop Science Society of America, Soil Science Society of America, 406-449. |
[47] | Aenugu HPR, Sathis Kumar D, Srisudharson, et al. (2011) Near infrared spectroscopy- an overview. Int J ChemTech Res 3: 825-836. |
[48] |
Modroño S, Soldado A, Martínez-Fernández A, et al. (2017) Handheld NIRS sensors for routine compound feed quality control: Real time analysis and field monitoring. Talanta 162: 597-603. doi: 10.1016/j.talanta.2016.10.075
![]() |
1. | Morsy Nour, Gaber Magdy, Jose Pablo Chaves-Avila, Alvaro Sanchez-Miralles, Eduard Petlenkov, Automatic Generation Control of a Future Multisource Power System Considering High Renewables Penetration and Electric Vehicles: Egyptian Power System in 2035, 2022, 10, 2169-3536, 51662, 10.1109/ACCESS.2022.3174080 | |
2. | S. Vinoth John Prakash, P.K. Dhal, Cost optimization and optimal sizing of standalone biomass/diesel generator/wind turbine/solar microgrid system, 2022, 10, 2333-8334, 665, 10.3934/energy.2022032 | |
3. | Shiva Amini, Salah Bahramara, Hêmin Golpîra, Bruno Francois, João Soares, Techno-Economic Analysis of Renewable-Energy-Based Micro-Grids Considering Incentive Policies, 2022, 15, 1996-1073, 8285, 10.3390/en15218285 | |
4. | Gurkan Soykan, Gulfem Er, Ethem Canakoglu, Optimal sizing of an isolated microgrid with electric vehicles using stochastic programming, 2022, 32, 23524677, 100850, 10.1016/j.segan.2022.100850 | |
5. | Santi Agatino Rizzo, Editorial to the 'Special Issue—Distribution network reliability in Smart Grids and Microgrids' of AIMS Energy, 2022, 10, 2333-8334, 533, 10.3934/energy.2022026 | |
6. | Mahmoud M. Gamil, Tomonobu Senjyu, Hasan Masrur, Hiroshi Takahashi, Mohammed Elsayed Lotfy, Controlled V2Gs and battery integration into residential microgrids: Economic and environmental impacts, 2022, 253, 01968904, 115171, 10.1016/j.enconman.2021.115171 | |
7. | Hoda Abd El-Sattar, Salah Kamel, Mohamed H. Hassan, Francisco Jurado, Optimal sizing of an off-grid hybrid photovoltaic/biomass gasifier/battery system using a quantum model of Runge Kutta algorithm, 2022, 258, 01968904, 115539, 10.1016/j.enconman.2022.115539 | |
8. | Zhongzhen Yan, Hui Zhou, Xianglong Wang, Hossein Lotfi, Optimal management of microgrid, considering various renewable and storage units of electrical-thermal generations and demand response program, 2023, 09596526, 137133, 10.1016/j.jclepro.2023.137133 | |
9. | Tarek Ibrahim, Farouk Hachem, Mohamad Ramadan, Jalal Faraj, Georges El Achkar, Mahmoud Khaled, Cooling PV panels by free and forced convections: Experiments and comparative study, 2023, 11, 2333-8334, 774, 10.3934/energy.2023038 | |
10. | Taiyeb Hasan Sakib, Ashik Ahmed, Md. Arif Hossain, Quazi Nafees-Ul-Islam, Optimal sizing of an HRES with probabilistic modeling of uncertainties − a framework for techno-economic analysis, 2024, 318, 01968904, 118899, 10.1016/j.enconman.2024.118899 | |
11. | Ardjouna. Chebabhi, Ilyes. Tegani, Abdelouahab Djoubair. Benhamadouche, Okba. Kraa, Optimal design and sizing of renewable energies in microgrids based on financial considerations a case study of Biskra, Algeria, 2023, 291, 01968904, 117270, 10.1016/j.enconman.2023.117270 | |
12. | Alvin Henao, Luceny Guzman, Exploration of Alternatives to Reduce the Gap in Access to Electricity in Rural Communities—Las Nubes Village Case (Barranquilla, Colombia), 2024, 17, 1996-1073, 256, 10.3390/en17010256 | |
13. | Nishant Thakkar, Priyanka Paliwal, Data driven MCDM models for reliability-economic-environmental analysis of energy storage based autonomous micro-grid, 2024, 81, 2352152X, 110408, 10.1016/j.est.2023.110408 | |
14. | Mohamed H. Hassan, Salah Kamel, Murodbek Safaraliev, Sergey Kokin, Improved techno-economic optimization of hybrid solar/wind/fuel cell/diesel systems with hydrogen energy storage, 2024, 68, 03603199, 998, 10.1016/j.ijhydene.2024.04.124 | |
15. | M.L. Lu, Y.J. Sun, G. Kokogiannakis, Z.J. Ma, Design of flexible energy systems for nearly/net zero energy buildings under uncertainty characteristics: A review, 2024, 205, 13640321, 114828, 10.1016/j.rser.2024.114828 | |
16. | S. Ida Evangeline, K. Baskaran, S. Darwin, Minimizing voltage fluctuation in stand-alone microgrid system using a Kriging-based multi-objective stochastic optimization algorithm, 2024, 0948-7921, 10.1007/s00202-024-02497-3 | |
17. | Soraphon Kigsirisin, 2023, Chapter 1, 978-981-99-6289-1, 3, 10.1007/978-981-99-6290-7_1 | |
18. | Marcos Tostado-Véliz, Antonio Escámez, Roque Aguado, Daniel Sánchez-Lozano, Francisco Jurado, David Vera, Optimal sizing of hybrid PV–diesel–biomass gasification plants for electrification of off-grid communities: An efficient approach based on Benders’ decomposition, 2024, 458, 09596526, 142360, 10.1016/j.jclepro.2024.142360 | |
19. | Gulfem Er, Gurkan Soykan, Ethem Canakoglu, Stochastic optimal design of a rural microgrid with hybrid storage system including hydrogen and electric cars using vehicle-to-grid technology, 2024, 75, 2352152X, 109747, 10.1016/j.est.2023.109747 |
Cost | PV | WG | No of | Pbiomass | ||
($) | (KW) | (KW) | Batteries | (max) KW | ||
Category A | ||||||
Scenario 1 | MOGA | 1.787∗108 | 100.072 | 0 | 0 | 321.810 |
(PV+Biomass) | ϵ−MOGA | 1.789∗108 | 99.883 | 0 | 0 | 328.254 |
Scenario 2 | MOGA | 1.539∗108 | 0 | 705.943 | 0 | 277.906 |
(WG+Biomass) | ϵ−MOGA | 1.540∗108 | 0 | 705.770 | 0 | 278.426 |
Scenario 3 | MOGA | 1.480∗108 | 21.118 | 678.794 | 0 | 279.669 |
(PV+WG+Biomass) | ϵ−MOGA | 1.479∗108 | 20.052 | 684.906 | 0 | 280.355 |
Category B | ||||||
Scenario 4 | MOGA | 3.455∗107 | 462.114 | 0 | 23 | 94.150 |
(PV+Biomass+BESS) | ϵ−MOGA | 2.647∗107 | 488.195 | 0 | 22 | 328.917 |
Scenario 5 | MOGA | 6.649∗107 | 0 | 1883.090 | 14 | 95.797 |
(WG+Biomass+BESS) | ϵ−MOGA | 2.953∗107 | 0 | 2297.645 | 14 | 303.548 |
Scenario 6 | MOGA | 6.030∗107 | 413.147 | 292.798 | 24 | 88.689 |
(PV+WG+Biomass+BESS) | ϵ− MOGA | 3.236∗107 | 0 | 2500 | 17 | 330 |
Category C | ||||||
Scenario 7 | MOGA | 4.040∗106 | 535.060 | 0 | 22 | 0 |
(PV+BESS) | ϵ−MOGA | 3.359∗106 | 474.495 | 0 | 18 | 0 |
Scenario 8 | MOGA | 9.397∗106 | 0 | 2469.141 | 13 | 0 |
(WG+BESS) | ϵ−MOGA | 9.397∗106 | 0 | 2469.182 | 13 | 0 |
Scenario 9 | MOGA | 5.599∗106 | 562.579 | 272.012 | 27 | 0 |
(PV+WG+BESS) | ϵ−MOGA | 5.204∗106 | 634.020 | 0 | 29 | 0 |
Configuration | Cost | PV | WG | No of | Pbiomass | |
($) | (KW) | (KW) | Batteries | (max) KW | ||
Category A | ||||||
Scenario 1 | PV+Biomass | 1.911∗108 | 77.534 | 0 | 0 | 322.528 |
Scenario 2 | WG+Biomass | 1.933∗108 | 0 | 317.317 | 0 | 329.821 |
Scenario 3 | PV+WG+Biomass | 1.890∗108 | 42.377 | 182.208 | 0 | 320.965 |
Category B | ||||||
Scenario 4 | PV+Biomass+BESS | 8.979∗107 | 501.204 | 0 | 33 | 191.922 |
Scenario 5 | WG+Biomass+BESS | 1.246∗108 | 0 | 1698.970 | 30 | 155.320 |
Scenario 6 | PV+WG+Biomass+BESS | 7.533∗107 | 458.217 | 701.070 | 42 | 103.754 |
Category C | ||||||
Scenario 7 | PV+BESS | 4.327∗106 | 504.755 | 0 | 25 | 0 |
Scenario 8 | WG+BESS | 1.007∗107 | 0 | 2166.951 | 25 | 0 |
Scenario 9 | PV+WG+BESS | 7.912∗106 | 773.901 | 9.108 | 47 | 0 |
Component and Economic Specification | ||
Discount rate (r) | 5% | |
Escalation rate | 7% | |
PV Module | ||
Investment cost | 1690 | $/KW |
Maintenance | 26 | $/KW/yr |
(PV) reduction factor | 84% | |
lifetime | 25 | years |
Wind Generator | ||
Investment cost | 2030 | $/KW |
Operating and Maintenance | 75 | $/KW/yr |
lifetime | 25 | years |
Cut-in wind speed | 4 | m/s |
Cut-out wind speed | 25 | m/s |
Rated wind speed | 14.5 | m/s |
Shear coefficient | 0.14 | |
Turbine hub height | 50 | m |
BESS | ||
Investment cost | 200 | $/KWh |
Replacement cost | 200 | $/KWh |
Self discharge rate | 0.01% | |
Efficiency | 90% | |
Life time | 5 | years |
Biomass generator | ||
Investment cost(γbg) | 6200 | $/KWh |
Annual fixed operation and maintenance cost of biomass system(θ1bg) | 0.05*γbg | $/Kw/year |
Variable operation and maintenance cost of biogas system(θ2bg) | 0.0042 | $/Kw/year |
Biomass fuel cost of biogas system(θ2bg) | 35 | ($/t/year) |
Biomass system resale price(λbg) | 0.3*γbg | ($/Kw) |
Electrical conversion efficiency(ηbm) | 0.21 |
Cost | PV | WG | No of | Pbiomass | ||
($) | (KW) | (KW) | Batteries | (max) KW | ||
Category A | ||||||
Scenario 1 | MOGA | 1.787∗108 | 100.072 | 0 | 0 | 321.810 |
(PV+Biomass) | ϵ−MOGA | 1.789∗108 | 99.883 | 0 | 0 | 328.254 |
Scenario 2 | MOGA | 1.539∗108 | 0 | 705.943 | 0 | 277.906 |
(WG+Biomass) | ϵ−MOGA | 1.540∗108 | 0 | 705.770 | 0 | 278.426 |
Scenario 3 | MOGA | 1.480∗108 | 21.118 | 678.794 | 0 | 279.669 |
(PV+WG+Biomass) | ϵ−MOGA | 1.479∗108 | 20.052 | 684.906 | 0 | 280.355 |
Category B | ||||||
Scenario 4 | MOGA | 3.455∗107 | 462.114 | 0 | 23 | 94.150 |
(PV+Biomass+BESS) | ϵ−MOGA | 2.647∗107 | 488.195 | 0 | 22 | 328.917 |
Scenario 5 | MOGA | 6.649∗107 | 0 | 1883.090 | 14 | 95.797 |
(WG+Biomass+BESS) | ϵ−MOGA | 2.953∗107 | 0 | 2297.645 | 14 | 303.548 |
Scenario 6 | MOGA | 6.030∗107 | 413.147 | 292.798 | 24 | 88.689 |
(PV+WG+Biomass+BESS) | ϵ− MOGA | 3.236∗107 | 0 | 2500 | 17 | 330 |
Category C | ||||||
Scenario 7 | MOGA | 4.040∗106 | 535.060 | 0 | 22 | 0 |
(PV+BESS) | ϵ−MOGA | 3.359∗106 | 474.495 | 0 | 18 | 0 |
Scenario 8 | MOGA | 9.397∗106 | 0 | 2469.141 | 13 | 0 |
(WG+BESS) | ϵ−MOGA | 9.397∗106 | 0 | 2469.182 | 13 | 0 |
Scenario 9 | MOGA | 5.599∗106 | 562.579 | 272.012 | 27 | 0 |
(PV+WG+BESS) | ϵ−MOGA | 5.204∗106 | 634.020 | 0 | 29 | 0 |
Configuration | Cost | PV | WG | No of | Pbiomass | |
($) | (KW) | (KW) | Batteries | (max) KW | ||
Category A | ||||||
Scenario 1 | PV+Biomass | 1.911∗108 | 77.534 | 0 | 0 | 322.528 |
Scenario 2 | WG+Biomass | 1.933∗108 | 0 | 317.317 | 0 | 329.821 |
Scenario 3 | PV+WG+Biomass | 1.890∗108 | 42.377 | 182.208 | 0 | 320.965 |
Category B | ||||||
Scenario 4 | PV+Biomass+BESS | 8.979∗107 | 501.204 | 0 | 33 | 191.922 |
Scenario 5 | WG+Biomass+BESS | 1.246∗108 | 0 | 1698.970 | 30 | 155.320 |
Scenario 6 | PV+WG+Biomass+BESS | 7.533∗107 | 458.217 | 701.070 | 42 | 103.754 |
Category C | ||||||
Scenario 7 | PV+BESS | 4.327∗106 | 504.755 | 0 | 25 | 0 |
Scenario 8 | WG+BESS | 1.007∗107 | 0 | 2166.951 | 25 | 0 |
Scenario 9 | PV+WG+BESS | 7.912∗106 | 773.901 | 9.108 | 47 | 0 |
Component and Economic Specification | ||
Discount rate (r) | 5% | |
Escalation rate | 7% | |
PV Module | ||
Investment cost | 1690 | $/KW |
Maintenance | 26 | $/KW/yr |
(PV) reduction factor | 84% | |
lifetime | 25 | years |
Wind Generator | ||
Investment cost | 2030 | $/KW |
Operating and Maintenance | 75 | $/KW/yr |
lifetime | 25 | years |
Cut-in wind speed | 4 | m/s |
Cut-out wind speed | 25 | m/s |
Rated wind speed | 14.5 | m/s |
Shear coefficient | 0.14 | |
Turbine hub height | 50 | m |
BESS | ||
Investment cost | 200 | $/KWh |
Replacement cost | 200 | $/KWh |
Self discharge rate | 0.01% | |
Efficiency | 90% | |
Life time | 5 | years |
Biomass generator | ||
Investment cost(γbg) | 6200 | $/KWh |
Annual fixed operation and maintenance cost of biomass system(θ1bg) | 0.05*γbg | $/Kw/year |
Variable operation and maintenance cost of biogas system(θ2bg) | 0.0042 | $/Kw/year |
Biomass fuel cost of biogas system(θ2bg) | 35 | ($/t/year) |
Biomass system resale price(λbg) | 0.3*γbg | ($/Kw) |
Electrical conversion efficiency(ηbm) | 0.21 |