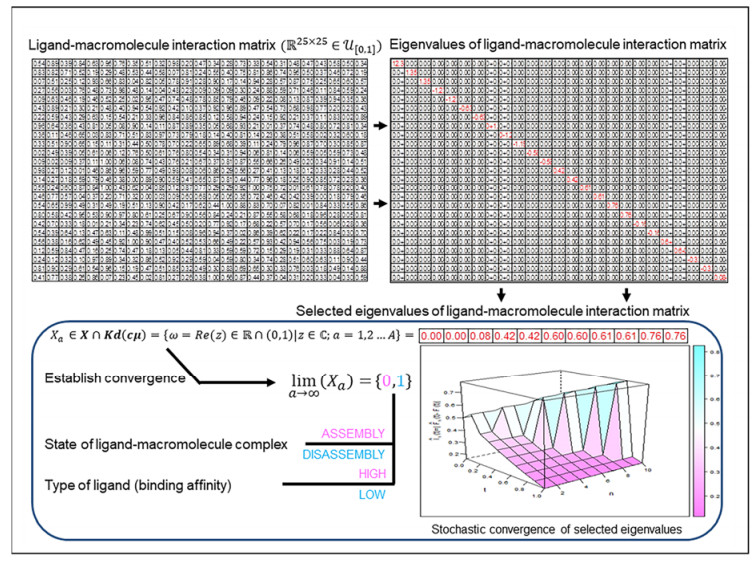
Patchouli is one of the essential oil-producing plants which is commonly found in Indonesia, but the productivity tends to decline. Tissue culture technology is one alternative to increase patchouli plant productivity. Tissue culture requires growth regulators in order for the plants to grow optimally. Moringa plants have the potential as a source of growth regulators for tissue culture because Moringa leaves contain the zeatin hormone. Therefore, a comparison test of various concentrations of the zeatin and the moringa leaf extract is needed to determine whether the Moringa leaf extract can be an alternative to the zeatin hormone. This study aimed to investigate the effect of Moringa leaf extract on the growth of patchouli bud explants. The study employed a complete random design (CRD) with the treatment of concentrations of Moringa leaf extract in MS media. Moringa leaf extract was given at 30 grams/L, 40 grams/L, and 50 grams/L, respectively. Meanwhile, the zeatin hormone was given at a concentration of 1.5 ppm, 2 ppm, and 2.5 ppm. Each treatment was repeated four times. Moringa leaves were extracted by maceration method, and patchouli explants were obtained from the 3rd sub-culture of patchouli shoots. From the research results, it can be concluded that (1) the use of Moringa leaf extract can improve the growth of patchouli explants, and (2) the use of 40 grams/L of Moringa leaf extract can be an alternative to substitute the use of 1.5 ppm zeatin hormone.
Citation: Ellis Nihayati, Merynda Wardatun Najah. Comparative assessment of The Effect of Moringa oleifera Leaf Extract (MLE) and Zeatin on invitro Regeneration Response of Pogostemon cablin Bud Explants[J]. AIMS Agriculture and Food, 2021, 6(1): 308-320. doi: 10.3934/agrfood.2021019
[1] | Christian Winkel, Simon Neumann, Christina Surulescu, Peter Scheurich . A minimal mathematical model for the initial molecular interactions of death receptor signalling. Mathematical Biosciences and Engineering, 2012, 9(3): 663-683. doi: 10.3934/mbe.2012.9.663 |
[2] | Hong Yuan, Jing Huang, Jin Li . Protein-ligand binding affinity prediction model based on graph attention network. Mathematical Biosciences and Engineering, 2021, 18(6): 9148-9162. doi: 10.3934/mbe.2021451 |
[3] | Yuewu Liu, Mengfang Zeng, Shengyong Liu, Chun Li . Dynamics analysis of building block synthesis reactions for virus assembly in vitro. Mathematical Biosciences and Engineering, 2023, 20(2): 4082-4102. doi: 10.3934/mbe.2023191 |
[4] | Ronald Lai, Trachette L. Jackson . A Mathematical Model of Receptor-Mediated Apoptosis: Dying to Know Why FasL is a Trimer. Mathematical Biosciences and Engineering, 2004, 1(2): 325-338. doi: 10.3934/mbe.2004.1.325 |
[5] | Feng Rao, Carlos Castillo-Chavez, Yun Kang . Dynamics of a stochastic delayed Harrison-type predation model: Effects of delay and stochastic components. Mathematical Biosciences and Engineering, 2018, 15(6): 1401-1423. doi: 10.3934/mbe.2018064 |
[6] | Max-Olivier Hongler, Roger Filliger, Olivier Gallay . Local versus nonlocal barycentric interactions in 1D agent dynamics. Mathematical Biosciences and Engineering, 2014, 11(2): 303-315. doi: 10.3934/mbe.2014.11.303 |
[7] | Yutong Man, Guangming Liu, Kuo Yang, Xuezhong Zhou . SNFM: A semi-supervised NMF algorithm for detecting biological functional modules. Mathematical Biosciences and Engineering, 2019, 16(4): 1933-1948. doi: 10.3934/mbe.2019094 |
[8] | O. E. Adebayo, S. Urcun, G. Rolin, S. P. A. Bordas, D. Trucu, R. Eftimie . Mathematical investigation of normal and abnormal wound healing dynamics: local and non-local models. Mathematical Biosciences and Engineering, 2023, 20(9): 17446-17498. doi: 10.3934/mbe.2023776 |
[9] | Zhenzhen Zheng, Ching-Shan Chou, Tau-Mu Yi, Qing Nie . Mathematical analysis of steady-state solutions in compartment and continuum models of cell polarization. Mathematical Biosciences and Engineering, 2011, 8(4): 1135-1168. doi: 10.3934/mbe.2011.8.1135 |
[10] | Linlu Song, Shangbo Ning, Jinxuan Hou, Yunjie Zhao . Performance of protein-ligand docking with CDK4/6 inhibitors: a case study. Mathematical Biosciences and Engineering, 2021, 18(1): 456-470. doi: 10.3934/mbe.2021025 |
Patchouli is one of the essential oil-producing plants which is commonly found in Indonesia, but the productivity tends to decline. Tissue culture technology is one alternative to increase patchouli plant productivity. Tissue culture requires growth regulators in order for the plants to grow optimally. Moringa plants have the potential as a source of growth regulators for tissue culture because Moringa leaves contain the zeatin hormone. Therefore, a comparison test of various concentrations of the zeatin and the moringa leaf extract is needed to determine whether the Moringa leaf extract can be an alternative to the zeatin hormone. This study aimed to investigate the effect of Moringa leaf extract on the growth of patchouli bud explants. The study employed a complete random design (CRD) with the treatment of concentrations of Moringa leaf extract in MS media. Moringa leaf extract was given at 30 grams/L, 40 grams/L, and 50 grams/L, respectively. Meanwhile, the zeatin hormone was given at a concentration of 1.5 ppm, 2 ppm, and 2.5 ppm. Each treatment was repeated four times. Moringa leaves were extracted by maceration method, and patchouli explants were obtained from the 3rd sub-culture of patchouli shoots. From the research results, it can be concluded that (1) the use of Moringa leaf extract can improve the growth of patchouli explants, and (2) the use of 40 grams/L of Moringa leaf extract can be an alternative to substitute the use of 1.5 ppm zeatin hormone.
Ligands, are biochemical modifiers of macromolecular structure and can impact biological function. These can be co-factors (iron, cobalt, copper, zinc), co-enzymes such as Nicotinamide- and Flavin- Adenine Dinucleotides (NAD, FAD) and full-length molecules with short binding sites [1,2]. Ligands, unlike substrates/co-substrates are either reversibly altered or not at all. The biochemical role of ligands, in vivo, is complex and can influence both, enzyme-mediated substrate catalysis and non-enzymatic association and dissociation interactions. Whilst, the interaction with competitive inhibitors, co-factors or co-enzymes involves definitive and direct modifications to the active site residues, the effect of a ligand can be allosteric and indirect [3,4,5]. The latter involves both long-distance conformational changes and non-covalent interactions (hydrogen, Van der Waals, hydrophobic, electrostatic) [6,7,8,9,10,11]. Empirical data suggests that the binding affinity or the strength-of-association of a macromolecule for its ligand is a critical determinant of function [7,8,9,10,11]. For example, 2, 3-Bisphophoglycerate is a potent modifier of Hemoglobin function and does so by shifting the oxygen-dissociation curve to the right. In its absence Hemoglobin retains high affinity for molecular oxygen (left-shift of the oxygen dissociation curve), an undesirable effect on its role as a transporter [10,11]. Similarly, Ascorbic acid maintains iron in its reduced state in the gastrointestinal tract and as part of the active site of non-haem iron (Ⅱ)- and 2-oxoglutarate-dependent dioxygenases [12,13]. Deficiency of Ascorbic acid is implicated in tardy iron absorption in the ileum as well as a range of collagen disorders such as scurvy (Prolyl- and Lysyl-hydroxylases) [14,15]. Conversely, proteins which are modified such as those that may originate from missense or amino acid substitutions and are secondary to genomic variants such as single nucleotide polymorphisms (SNPs) and insertions-deletions (indels), will also result in several clinical outcomes [16,17]. Here, too, enzyme catalysis is directly affected if these are present at the active site or is impacted indirectly (folding, stability, complex formation) when present elsewhere [16,17,18].
There is a large volume of literature which describes macromolecules in terms of either residues (amino acids, nucleotides) interacting or an all atom-based interaction matrix. The proponent of the 2D approach is the Gaussian network model (GNM), while the Anisotropic network model (ANM) is representative of the 3D approach [19,20,21,22]. The fundamental premise of both these approaches is the elastic network model (ENM) [19]. Here, an atom or residue is modeled as an elastic mass and the interaction between a pair of atoms/residues is dependent on the selection of a pre-determined cut-off distance [22]. The force constant, although, not a parameter by definition, has also been studied and shows good correlation with B-factor data [23]. A major application of these studies is normal mode analysis (NMA), which has been used to glean valuable insights into the structural dynamics of the investigated macromolecule and into B-factor distribution [23,24]. Despite this success, there are significant limitations of this approach, including inadequate descriptors for the type of interactions computed by the Hessian matrix and data points that are dependent on a preselected cut-off distance (5–10 Ang, GNM; 10–15 Ang, ANM) [20,22,25]. Parameter-free versions of the ENM (pfENM), GNM (pfGNM) and ANM (pfANM), to resolve the latter, have been described and compared to establish B-factor distribution (isotropic, anisotropic) [25]. Additionally, many of these studies have focused on inferring biophysical characteristics such as cross-correlational fluctuations and mean square displacements. From a functional standpoint, however, it is not clear whether these data can be utilized to derive/study parameters such as the Michaelis-Menten constant (Km) or the association/dissociation (Ka/Kd). Since, these depend on the presence of an organic or inorganic modifier, i.e., ligand/substrate/co-factor/co-substrate, in addition to the modeled macromolecule, its exclusion is another major lacuna of these studies.
Despite the availability of clinical, empirical, analytical and computational data, a mathematically rigorous explanation for the heterogeneity in biochemical function, for a ligand-macromolecular complex, is missing. The work presented models a ligand and macromolecule as a homo- or hetero-dimer and subsumes a finite and equal number of atoms/residues per monomer. The pairwise interactions of the resulting square matrix will be chosen randomly from a standard uniform distribution. The resulting eigenvalues will be analyzed and modeled in accordance with known literature on biochemical reactions to generate biologically viable and usable dissociation constants. The theoretical results will be complemented by numerical studies where applicable. Additionally, and through various theorems, lemmas and corollaries, a schema to partition ligands into high- and low-affinity variants will also be discussed. The suitability of the transition-state dissociation constants as a model for ligand-macromolecular interactions will be inferentially assessed by analyzing the clinical outcomes of amino acid substitutions of selected enzyme homodimers. The relevance of the model to biochemical function will be discussed by examining the ligand-macromolecular complex for known ligands of non-haem iron (Ⅱ)- and 2-oxoglutarate-dependent dioxygenases (Fe2OG) and the major histocompatibility complex (Ⅰ) (MHC1).
The general outline of the manuscript includes an initial section where the system to be modeled, rationale for this study, and formal definitions are introduced (Section 2). The model is analyzed, formulated and presented as theorems, lemmas and corollaries (Section 3). This section also includes a numerical study to demonstrate and validate the theoretical assertions made. The biological relevance of these findings are discussed with an analysis of clinical outcomes of enzyme sequence variants and case studies of enzyme- and non-enzymatic complex formation (Section 4). A brief conclusion that summarizes the presented study, limitations and future directions is included at the end of the manuscript (Section 5). Details of all proofs are included after the conclusions (Section 6).
Consider the generic interaction between macromolecule (c) and ligand (μ),
cμrf→←rbc+μRXN(1) |
We can represent this interaction/reaction, at a steady state, with the rate equations [26],
Rd(cμ)=rf.[cμ]Lcμ≥0 | (1) |
Ra(cμ)=rb.[c]Lc≥0[μ]Lμ≥0 | (2) |
At steady state,
Rd(cμ)=Ra(cμ) | (3) |
⇒rfrb=[c]Lc≥0.[μ]Lμ≥0[cμ]Lcμ≥0 | (4) |
rfrb=Kd(cμ) | (5) |
Here,
c:=Macromolecule |
μ:=Ligand |
[.]:=Molarconcentrationofreactantinstandardform(M) |
Ra(cμ):=Rateofassociationofcomplex(Ms−1) |
Rd(cμ):=Rateofdissociationofcomplex(Ms−1) |
rf:=Rateconstantsofforwardreaction(s−1) |
rb:=Rateconstantsofreversereaction(M−1s−1) |
L:=Stoichiometryofreactant(s) |
Kd(cμ):=Dissociationconstantforligand−macromolecularcomplex(M) |
It is clear that a ligand-macromolecular complex may exist in one of three distinct states. These include: a) perfect association, b) perfect dissociation and c) an intermediate- or transition-state; and can be represented in terms of the dissociation constant,
Case (1) Perfectassociation Def. (1)
1rb→∞;rf→0 | (6, 7) |
⇒Kd(cμ)≈0 | (8) |
Case (2) Perfectdisassociation Def. (2)
rf>>>rb | (9) |
⇒Kd(cμ)≥1 | (10) |
Case (3) Transient−statedisassociationconstant Def. (3)
rf≶rb | (11) |
⇒Kd(cμ)∈R∩(0,1) | (12) |
Consider an atom/residue-based representation (amino acids/nucleotides) of a generic set of monomeric macromolecules, {\boldsymbol{\mathcal{C}}} = \{protein, DNA, RNA\} , with z = \mathrm{1, 2}, \dots ., Z components each with i -indexed ( i = \mathrm{1, 2}, \dots ., I ) c-atoms/residues,
\begin{array}{cc}{{\bf c}\equiv {{{\mathcal{C}}}}_{z}\in {\boldsymbol{\mathcal{C}}}|{\bf c} = \left[{c}_{1}{c}_{2}\dots .{c}_{i = I}\right]}^{T}, I\in \mathbb{N}& \end{array} | (13) |
The analogous model of a monomer ligand, {\boldsymbol{\mathcal{L}}} = \{small\;molecule, peptide, oligonucleotide\} , with j -indexed (j = \mathrm{1, 2}, \dots , J) \mu -atoms/residues is,
\boldsymbol{\mu }\in {\boldsymbol{\mathcal{L}}}|{\bf{ \pmb{\mathsf{ μ}} }} = \left[{\mu }_{1}{\mu }_{2}\dots .{\mu }_{j = J}\right], J\in \mathbb{N} | (14) |
It is also assumed that the ligand-macromolecular complex is a homo- or hetero-dimer with an equal number of atoms/residues \left(I = J\right) per monomer. The interaction matrix is,
\begin{array}{rr}\left\langle{\boldsymbol{c}|\boldsymbol{\mu }}\right\rangle = {\left[{c}_{1}{c}_{2}..{c}_{i = I}\right]}^{T}\times \left[{\mu }_{1}{\mu }_{2}..{\mu }_{j = J}\right] = {{\boldsymbol{\mathcal{C}}}}_{\boldsymbol{z}\boldsymbol{\mu }} = \left({{c}_{i}\mu }_{j}\right)\subset {\mathbb{R}}^{I\times J}& \end{array} | (15) |
The numerical values of this matrix are chosen randomly from the standard uniform distribution,
\begin{array}{rr}{{\boldsymbol{\mathcal{C}}}}_{z\mu } = \left({{c}_{i}\mu }_{j}\right)\in {\mathcal{U}}_{\left[\mathrm{0, 1}\right]}& \end{array} | (16) |
The rationale for this choice is that each pairwise interaction is subsumed to be a function of an arbitrary number of non-bonded interactions (long- and short-range) and is therefore, unique. Clearly, this implies the existence of \left\{I, J\right\} -linear independent vectors,
\begin{array}{cc}rank\left({{\boldsymbol{\mathcal{C}}}}_{z\mu }\right) = \left\{I, J\right\}& \end{array} | (17) |
Since {{\boldsymbol{\mathcal{C}}}}_{\boldsymbol{z}\boldsymbol{\mu }} is diagonalizable there exists a diagonal matrix, \mathit{\boldsymbol{\mathcal{K}}{\boldsymbol{\mathcal{C}}}}_{z\mu } ,
\mathit{\boldsymbol{\mathcal{K}}{\boldsymbol{\mathcal{C}}}}_{z\mu } = {\boldsymbol{X}}^{-1}{{\boldsymbol{\mathcal{C}}}}_{z\mu }\boldsymbol{X} | (18) |
{z}_{i = j} = z\in diag\left(\mathit{\boldsymbol{\mathcal{K}}{\boldsymbol{\mathcal{C}}}}_{z\mu }\right)\subset \mathbb{C} | (19) |
{{\boldsymbol{\mathcal{C}}}}_{\boldsymbol{z}\boldsymbol{\mu }} , is non-symmetric the computed eigenvalues of the modeled ligand-macromolecular interaction matrix can have positive and negative real parts,
\left\{{\alpha }_{ij} = Re\left(z\right)\in \boldsymbol{K}\boldsymbol{d}\left({{\boldsymbol{\mathcal{C}}}}_{\boldsymbol{z}\boldsymbol{\mu }}\right)\subset \mathbb{R}|i = j, z\in \mathbb{C}\right\} | (20) |
\left\{{\alpha }_{ij} = Re\left(z\right)\in \boldsymbol{K}\boldsymbol{d}\left({{\boldsymbol{\mathcal{C}}}}_{\boldsymbol{z}\boldsymbol{\mu }}\right)\subset \mathbb{R}|i = j, z\in \mathbb{C}\right\} | (20.1) |
A further subdivision can be made in accordance with established literature,
\left\{{\alpha }_{ij} = Re\left(z\right)\in \boldsymbol{K}\boldsymbol{d}\left({{\boldsymbol{\mathcal{C}}}}_{\boldsymbol{z}\boldsymbol{\mu }}\right)\subset \mathbb{R}|i = j, z\in \mathbb{C}\right\} {\omega }_{\infty } = Kd\left(c\mu \right)\ge 1 | (21) |
Perfect\;association\equiv Reverse \;\; {\omega }_{0} = Kd\left(c\mu \right) = 0 | (22) |
This selection generates the set,
\omega = {\alpha }_{ij}\in \boldsymbol{K}\boldsymbol{d}\left({{\boldsymbol{\mathcal{C}}}}_{\boldsymbol{z}\boldsymbol{\mu }}\right)\subset \mathbb{R}\cap \left(\mathrm{0, 1}\right) | (23) |
\omega \in \boldsymbol{K}\boldsymbol{d}\left(\boldsymbol{c}\boldsymbol{\mu }\right)\;\;\;\;\;\;\;\;\;\;\;\;\;\;\;\;\;\;\;\;\;\;\;\;\;\;\;\;\;Def.\; {\mathit{(4)}} |
\#\boldsymbol{K}\boldsymbol{d}\left(\boldsymbol{c}\boldsymbol{\mu }\right) = A | (23.1) |
We can combine these to get a preliminary definition of the transition-state disassociation constants. These are the strictly positive real part of all complex eigenvalues that characterize a ligand-macromolecular complex with an equal number of atoms/residues per monomer and belong to the open interval (0, 1),
\left\{\omega = \mathcal{R}\mathrm{e}\left(\mathrm{z}\right)\in \boldsymbol{K}\boldsymbol{d}\left(\boldsymbol{c}\boldsymbol{\mu }\right)\subset \boldsymbol{K}\boldsymbol{d}\left({{\boldsymbol{\mathcal{C}}}}_{\boldsymbol{z}\boldsymbol{\mu }}\right)\subset \mathbb{R}\cap \left(\mathrm{0, 1}\right)|z\in \mathbb{C}\right\} | (Def. \; {\mathit{(5a)}}) |
Whilst, the states of perfect association and dissociation are key determinants of whether a reaction will occur or not, the transition-state dissociation constants may offer insights into the origins of threshold values, feedback mechanisms and other regulatory checkpoints. However, in order to ascribe biological relevance to these findings we must establish various bounds which can then be utilized to assess and thence assay the function of a ligand-macromolecular complex.
Theorem 1 (T1): The linear map between the transition-state dissociation constants and the eigenvalues that characterize the interactions of the homo- or hetero-dimer form of the modeled ligand-macromolecular complex is the injection,
g:\omega \in \boldsymbol{K}\boldsymbol{d}\left(\boldsymbol{c}\boldsymbol{\mu }\right)\mapsto \boldsymbol{K}\boldsymbol{d}\left({{\boldsymbol{\mathcal{C}}}}_{\boldsymbol{z}\boldsymbol{\mu }}\right) | (24) |
Theorem 2 (T2): The transition-state dissociation constants that characterize the interactions of the homo- or hetero-dimer form of the modeled ligand-macromolecular complex is a monotonic and non-increasing sequence,
\begin{array}{cc}{\left\{{\omega }_{a}\right\}}_{a\le a+1}|& \omega \in \boldsymbol{K}\boldsymbol{d}\left(\boldsymbol{c}\boldsymbol{\mu }\right)\end{array} | (25) |
Theorem 3 (T3): The transition-state dissociation constants that characterize the interactions of the homo- or hetero-dimer form of the modeled ligand-macromolecule complex are monotonic, bounded and therefore, convergent,
\begin{array}{cc}\underset{a\to \infty }{\mathrm{lim}}{\left\{{\omega }_{a}\right\}}_{a\le a+1} = \left\{\mathrm{0, 1}\right\}|& \omega \in \boldsymbol{K}\boldsymbol{d}\left(\boldsymbol{c}\boldsymbol{\mu }\right)\end{array} | (26) |
Corollary 1 (C1): The transition-state dissociation constants that characterize the interactions of the homo- or hetero-dimer form of the modeled ligand-macromolecule complex is a sequence with defined greatest-lower and least-upper -bounds,
inf{\left\{{\omega }_{a}\right\}}_{a\le a+1} < {\left\{{\omega }_{a}\right\}}_{a\le a+1} < sup{\left\{{\omega }_{a}\right\}}_{a\le a+1} | (27) |
Corollary 2 (C2; without proof): The cardinality of the set of transition-state dissociation constants that characterize the interactions of the homo- or hetero-dimer form of the modeled ligand-macromolecule complex is finite,
\begin{array}{c}\#\boldsymbol{K}\boldsymbol{d}\left(\boldsymbol{c}\boldsymbol{\mu }\right) = A < \#\boldsymbol{K}\boldsymbol{d}\left({{\boldsymbol{\mathcal{C}}}}_{\boldsymbol{z}\boldsymbol{\mu }}\right) = \left\{I, J\right\}\end{array} | (28) |
Using T1–T3 and C1, C2 we can refine our definition of the transition-state dissociation constants for the modeled ligand-macromolecular complex,
\begin{array}{cc}{\left\{{\omega }_{a}\right\}}_{a\le a+1}|& \omega \in \boldsymbol{K}\boldsymbol{d}\left(\boldsymbol{c}\boldsymbol{\mu }\right)\end{array};a = \mathrm{1, 2}\dots A | (Def. \; {\mathit{(5b)}}) |
where,
\omega = \mathcal{R}\mathrm{e}\left(\mathrm{z}\right)\in \boldsymbol{K}\boldsymbol{d}\left({{\boldsymbol{\mathcal{C}}}}_{\boldsymbol{z}\boldsymbol{\mu }}\right)\subset \mathbb{R}\cap \left(\mathrm{0, 1}\right)|z\in \mathbb{C} |
It is clear from the above results that the eigenvalue-based transition-state dissociation constants are continuous and can potentially model the multiplicity of intermediate- or transient-states that a ligand-macromolecular complex may adopt. It should therefore, be possible to partition the transition-state dissociation constants into functionally distinct subsets and will be characteristic for a specific ligand-macromolecular complex.
Theorem 4 (T4): The transition-state dissociation constants that characterize the interactions of the homo- or hetero-dimer form of the modeled ligand-macromolecule complex is a proper subset of the complete set of the real part of all complex eigenvalues that comprise the interaction matrix,
\boldsymbol{K}\boldsymbol{d}\left(\boldsymbol{c}\boldsymbol{\mu }\right)\subset \boldsymbol{K}\boldsymbol{d}\left({{\boldsymbol{\mathcal{C}}}}_{\boldsymbol{z}\boldsymbol{\mu }}\right) | (29) |
Corollary 3 (C3): The distribution of the transition-state dissociation constants that characterize the interactions of the homo- or hetero-dimer form of the modeled ligand-macromolecule complex will result in a schema by which we can annotate the ligand as a high \left({\mu }_{high}\right) - or low \left({\mu }_{low}\right) -affinity variant,
\mu = \left\{{\mu }_{high}, {\mu }_{low}\right\} | (30) |
Biologically relevant macromolecular complexes are characterized by heterogeneity and high-order \left(Z\ge 2\right) . This multimer-form of a macromolecule is formed around a primary molecule and its interactions. These may be protein-protein, DNA/RNA-protein or DNA-RNA-protein.
The multimer-form of ligand-macromolecular complex is easily modeled using the mathematical framework defined earlier. Here, the ligand-macromolecular complex is considered as a set of interacting monomers and the binding to a ligand occurs via a single unique monomer,
\left\{{{{\mathcal{C}}}}_{z}\in {\boldsymbol{\mathcal{C}}}|{{{\mathcal{C}}}}_{1} = {{{\mathcal{C}}}}_{2}\dots = {{{\mathcal{C}}}}_{z = Z}|Z\ge 2\right\} | (Def. \; {\mathit{(6a)}}) |
where,
{{{\mathcal{C}}}}_{z}\equiv {{{\mathcal{C}}}}_{z\mu }\equiv \mu {{{\mathcal{C}}}}_{z} | (Def. \; {\mathit{(6b)}}) |
Here,
\mu : = Ligand |
{{{\mathcal{C}}}}_{z}: = Unique\;monomer\;of\;a\;macromolecule\;that\;associates\;with\;a\;ligand |
\mu {{{\mathcal{C}}}}_{z}: = Ligand-macromolecular\;complex |
On the basis of these definitions we can re-index the remaining monomers, i.e., after excluding the unique monomer that binds to the ligand,
\left\{{{{\mathcal{C}}}}_{y}\in \widetilde {{\boldsymbol{\mathcal{C}}}} = {\boldsymbol{\mathcal{C}}}\backslash \left\{{{{\mathcal{C}}}}_{z}\right\}|y = \mathrm{1, 2}\dots Y;Y = Z-1\right\} | (Def. \; {\mathit{(7)}}) |
We now derive an expression for the multimer (higher-order)-form of a ligand-macromolecular complex.
Theorem 5 (T5): The multimer-form of a ligand-macromolecular complex comprising identical monomer units and with an arbitrary unit associating with a ligand is,
\begin{array}{rr}{\prod }_{y = 1}^{y = Y}{{{\mathcal{C}}}}_{z}.{\mathcal{C}}_{y} = {\mathcal{C}}_{z}.{\mathcal{C}}_{Y}^{y}|y = \mathrm{1, 2}\dots Y;Y = Z-1;Z\ge 2;z = \mathrm{1, 2}\dots Z& \end{array} | (31) |
Rewriting, this result in terms of the definition of the multimer form of a ligand-macromolecular complex,
\begin{array}{cc}{\mathcal{C}}_{z}.{\mathcal{C}}^{Y}\equiv \mu {\mathcal{C}}_{z}.{\mathcal{C}}^{Y}& \end{array} | (Def. \; {\mathit{(8)}}) |
Theorem 6 (T6): The linear map between the transition-state dissociation constants that characterize the interactions of the monomer- and multimer-forms of a ligand-macromolecular complex is a bijection,
\begin{array}{cc}{h}^{-1}\circ h:\omega \in \boldsymbol{K}\boldsymbol{d}\left(\boldsymbol{c}\boldsymbol{\mu }\right)\leftrightarrow u\in \boldsymbol{K}\boldsymbol{d}\left(\mu \boldsymbol{\mathcal{C}}_{z}\right)& \end{array} | (32) |
Theorem 7 (T7): The linear map between the transition-state dissociation constants and the eigenvalues that characterize the multimer-form of a ligand-macromolecular complex is a composition and an injection,
\begin{array}{cc}g\circ {h}^{-1}\circ h:u\in \boldsymbol{K}\boldsymbol{d}\left(\mu \boldsymbol{\mathcal{C}}_{z}.{\mathcal{C}}^{Y}\right)\mapsto \boldsymbol{K}\boldsymbol{d}\left(\boldsymbol{\mathcal{C}}_{\boldsymbol{z}\boldsymbol{\mu }}\right)& \end{array} | (33) |
The aforementioned theoretical results establish the mathematical rigor behind the definition and development of the transition-state dissociation constants as a model for ligand-macromolecular interactions (T1–T7, C1–C3). These assertions are complemented and numerically validated in R-4.1.2. Here, the R-packages, "ConvergenceConcepts" and "pracma" are utilized to investigate and analyze the stochastic convergence of the eigenvalues generated by the interaction matrix of a ligand-macromolecular complex (Supplementary Text 1) [27]. The R-scripts to establish convergence along with data processing are developed in-house (Supplementary Text 2). The stepwise algorithm to compute and numerically validate the transition-state dissociation constants is presented (Figure 1).
Step 1: A ligand and macromolecule with an equal number of atoms/residues ( n = 25 ) is chosen. Whilst, the complex can be modeled as a perfect homodimer, imperfect forms such as alternatively spliced isoenzymes are prevalent and commonly observed. Alternatively, the ligand can be modeled as a different macromolecule altogether.
Step 2: Populate the square interaction matrix with values randomly chosen from a uniform distribution, {\mathcal{U}}_{\left[\mathrm{0, 1}\right]} . These will represent one of three potential states for each interacting pair of atoms/residues of the modeled ligand-macromolecular complex (association, complete disassembly, transition-state).
Step 3: Compute the complex eigenvalues of this matrix and extract the real part of each.
Step 4: Form a sequence of the subset comprising those values that are strictly positive and belong to the open interval \left(\mathrm{0, 1}\right) .
Step 5: Establish the stochastic convergence in distribution and/or probability of the terms of this sequence to the expected upper (tsup)- and lower (tinf)-bounds, i.e., 0 and 1.
Step 5.1: Construct a sequence of random numbers, X, whose elements are uniquely mapped to the eigenvalue-based transition-state dissociation constants and represent intermediate- or transition-states of the modeled ligand-macromolecular complex,
\left\{{X}_{a}\in \boldsymbol{X}\cap \boldsymbol{K}\boldsymbol{d}\left(\boldsymbol{c}\boldsymbol{\mu }\right)\subset \mathbb{R}\cap \left(\mathrm{0, 1}\right)|a = \mathrm{1, 2}\dots A\right\} | (Def. \; {\mathit{(9)}}) |
Here,
A = \#\left(\boldsymbol{X}\cap \boldsymbol{K}\boldsymbol{d}\left(\boldsymbol{c}\boldsymbol{\mu }\right)\right) | (34) |
Step 5.2: Establish convergence of this set of random numbers. Here, weak convergence will suffice (distribution, probability),
\underset{a\to \infty }{\mathrm{lim}}\left({X}_{a}\right)\to \left\{tinf, tsup\right\} = \left\{\mathrm{0, 1}\right\} | (35) |
The parameters to accomplish this numerically are,
\begin{array}{r}nmax: = Number\;of\;values\;to\;analyse\\ M: = Number\;of\;paths\\ \varepsilon : = Threshold\;value\\ tinf: = Lower\;limit\;of\;interval\;to\;establish\;convergence\\ tsup: = Upper\;limit\;of\;interval\;to\;establish\;convergence\end{array} |
The values of these parameters for the numerically studied example are,
nmax = A = 11 | (35.1) |
M = 500 | (35.2) |
\varepsilon = 0.01 | (35.3) |
tinf = 0 | (35.4) |
tsup = 1 | (35.5) |
The eigenvalue-based model of transition-state dissociation constants of a ligand-macromolecular complex asserts that there are several intermediate- or transition-states of a complex and that each of these has the potential to modify the biochemical process that the complex participates in.
Ligand-macromolecular complexes, in vivo, possess a finite and in most cases, an incomparable number of atoms/residues. The theoretical results establish definition(s), bounds and metrics to assess biochemical function for both, monomer (T1–T4, C1–C3)- and multimer (T5–T7)-forms. The numerical data suggests that the set of transition-state dissociation constants can be finite, converge and retain statistical relevance (Figure 1).
Proposition (P): The transition-state dissociation constants for the monomer ( z = Z = 1 )- and multimer ( Z\ge 2 )-forms of a ligand-macromolecular complex with a finite number of atoms/residues of each \left(I, J\right) per monomer,
\left\{u\in \boldsymbol{K}\boldsymbol{d}\left(\boldsymbol{\mu }\boldsymbol{\mathcal{C}}_{\boldsymbol{z}}.\boldsymbol{\mathcal{C}}^{\boldsymbol{Y}}\right)|{\mathcal{C}}_{z}\in \boldsymbol{\mathcal{C}}, \mu \in {\boldsymbol{\mathcal{L}}};z = \mathrm{1, 2}\dots Z;A = \left\{I, J\right\}\right\} | (Def. \; {\mathit{(10)}}) |
is the finite set,
\boldsymbol{K}\boldsymbol{d}\left(\boldsymbol{\mu }\boldsymbol{\mathcal{C}}_{\boldsymbol{z}}.\boldsymbol{\mathcal{C}}^{\boldsymbol{Y}}\right)\subset \boldsymbol{K}\boldsymbol{d}\left(\boldsymbol{\mathcal{C}}_{\boldsymbol{z}\boldsymbol{\mu }}\right) |
Here,
I = \#{\mathcal{C}}_{z}|{\mathcal{C}}_{z}\in \boldsymbol{\mathcal{C}} | (36) |
J = \#\mu |\mu \in {\boldsymbol{\mathcal{L}}} | (37) |
where,
\boldsymbol{K}\boldsymbol{d}\left(.\right): = Set\;of\;constrained\;eigenvalue-based\;transition-state\;dissociation\;constants |
I: = Finite\;number\;of\;atoms\;or\;residues\;of\;macromolecule |
J: = Finite\;number\;of\;atoms\;or\;residues\;of\;ligand |
{\mathcal{C}}_{\mu z}.{\mathcal{C}}^{Y}: = Multimer\;form\;of\;ligand-macromolecular\;complex |
Enzyme-mediated catalysis, or lack thereof, results in metabolic enzyme disorders and may be inherited (inborn errors of metabolism) or acquired [28]. In order to assess the biomedical relevance of modeling ligand-macromolecule interactions as transition-state dissociation constants, the clinical outcomes of amino acid substitutions of selected enzyme homo- or hetero-dimers are examined (Table 1). These outcomes, i.e., benign, likely benign, pathologic, likely pathologic, conflicting, uncertain significance, are defined in accordance with the prevalent nomenclature of the ClinVar database [16]. Here, the data annotated as "uncertain significance" are those sequence variants with a high likelihood ( \approx 90–95\% ) of being "benign" or "pathogenic" [17]. This means they are likely to classified as "true positive", and if ignored will result in a "false negative". On the other hand, an outcome designated as with a "conflicting interpretation" is likely to be due to unresolved contradictory findings in the presence or absence of confounding factors. If we assume perfect contradiction, i.e., 50%, and couple this with the previous result, we get a \approx 70–73\% possibility that the variant of interest is a "true positive". This means, that here too, if missed a "false negative" will result. The metric of choice is the Recall (R) percentage,
R = \frac{TP}{TP+FN}\times 100 | (38) |
R: = Recall |
\begin{array}{l} TP: = Known\;positives(benign, likelybenign,\\ pathogenic, likely\;pathogenic) \end{array} | (Def. \; {\mathit{(12)}}) |
\begin{array}{l} FN: = Likely\;positives(conflicting\\ \;data, uncertain\;significance) \end{array} | (Def. \; {\mathit{(13)}}) |
Enzyme | EC | CV | SNP | M | Co | US | B | LB | P | LP | FN | TP | R (%) | |
1 | Glucokinase | 2.7.1.2 | 778 | 619 | 370 | 49 | 157 | 4 | 4 | 65 | 113 | 206 | 186 | 47.45 |
2 | Pyruvate kinase | 2.7.1.40 | 1239 | 629 | 209 | 14 | 135 | 7 | 7 | 30 | 20 | 149 | 64 | 30.05 |
3 | Cathepsin A | 3.4.16.x | 1776 | 1270 | 501 | 20 | 382 | 26 | 23 | 26 | 34 | 402 | 109 | 21.33 |
4 | Pyruvate dehydrogenase | 1.2.4.1 | 2317 | 1591 | 584 | 25 | 391 | 35 | 46 | 62 | 43 | 416 | 186 | 30.9 |
5 | Phosphofructokinase 1 | 2.7.1.11 | 165 | 32 | 10 | 2 | 4 | 2 | 1 | 1 | 1 | 6 | 5 | 45.45 |
6 | Phosphofructokinase 2 | 2.7.1.105 | 206 | 76 | 23 | 1 | 17 | 1 | 1 | 2 | 1 | 18 | 5 | 21.74 |
7 | Cystathione beta-synthase | 4.2.1.22 | 930 | 744 | 255 | 21 | 172 | 3 | 4 | 46 | 32 | 193 | 85 | 30.58 |
8 | DNA topoisomerase Ⅱ | 5.6.2.2 | 466 | 319 | 152 | 0 | 122 | 11 | 14 | 3 | 1 | 122 | 29 | 19.21 |
9 | Guanylate cyclase 1 | 4.6.1.2 | 1572 | 1117 | 576 | 29 | 417 | 11 | 10 | 34 | 59 | 446 | 114 | 20.36 |
10 | Phenylalanine hydroxylase | 1.14.16.1 | 1314 | 1102 | 631 | 5 | 182 | 0 | 3 | 161 | 254 | 187 | 418 | 69.09 |
Note: EC: Enzyme commission number; CV: Number of clinical variants; SNP: Single nucleotide polymorphisms; M: Missense mutations; Co: Conflicting data; US: Uncertain significance; B: Benign; LB: Likely; P: Pathogenic; LP: Likely pathogenic; FN: False negative (Co + US); TP: True positive (B + LB + P + LP); R: Recall ( \frac{TP}{TP+FN}\times 100 ). |
It is clear from these data that amino acid substitutions (nature, type), either alone or in combination, comprise distinct transition-states and are significant contributors to the biochemical function of each enzyme dimer ( Recall\approx 19–70\% ). Since each of these states will result in a distinct Kd , it is easily inferred that the transition-state dissociation constants, for a complex, may be more representative of biochemical function (T1–T4, C1–C3).
The discussion, vide supra, presents and highlights the biomedical relevance of modeling ligand-macromolecular interactions as transition-state dissociation constants. The results for monomer- and multimer-forms of ligand-macromolecular complexes are mathematically rigorous and have been validated, in silico. The results are now examined in context of biochemical function (enzyme, non-enzyme) for selected cases.
Case 1: Oxygen sensitive variants of non-haem iron (II)- and 2-oxoglutarate-dependent dioxygenases
The non-haem iron (Ⅱ)- and 2-oxoglutarate-dependent dioxygenases \left(EC1.14.x.y\right) , comprise a large superfamily of enzymes, are present in all kingdoms of life and is chemically diverse (variable reaction chemistry, multiple substrates) [12,13,29]. Clinically relevant members include Phytanoyl-CoA dioxygenase (PHYT), Lysine Hydroxylases, and the Proline 4-Hydroxylases (P4H) amongst several others [12,13,14,15,29]. These enzymes have important roles in Phytanic acid metabolism and collagen maturation, with sub-optimal activities contributing to diseases such as Refsum and the Ehlers-Danlos (ED)-syndrome [14,15,30]. Here, too, the outcomes (clinical, non-clinical) associated with substitution mutations for PHYT suggest that modeling ligand-macromolecular interactions as transition-state dissociation constants may be a better index of biochemical function (T1–T4, C1–C3, P) [31].
P4Hs, are classified as being either hypoxia-sensitive \left(H-P4H\equiv HP4H;EC\mathrm{1.14.11.29}\right) or collagen transforming \left(C-P4H\equiv CP4H;EC\mathrm{1.14.11.2}\right) [28]. These reactions may be written,
\begin{array}{rr}HP4H-HIF+{O}_{2}+2OG+Proline\begin{array}{c}\stackrel{{r}_{f}}{\to }\\ \underset{{r}_{b}}{\leftarrow }\end{array}Hydroxyproline+SA+{CO}_{2}& RXN\left(2\right)\\ CP4H+{O}_{2}+2OG+Proline\begin{array}{c}\stackrel{{r}_{f}}{\to }\\ \underset{{r}_{b}}{\leftarrow }\end{array}Hydroxyproline+SA+{CO}_{2}& RXN\left(3\right)\end{array} |
\begin{array}{rrr}{r}_{f}, {r}_{b}& : = & Rate\;constants\;for\;forward\;and\;backward\;reactions\;at\;steady\;state\\ HP4H& : = & Hypoxia\;inducible\;factor-dependent\;Proline\;4-Hydroxylase\\ HIF\equiv {\mu }_{high}& : = & Hypoxia-inducible\;factor(high-affinity\;modifier)\\ 2OG& : = & 2-oxoglutarate\\ SA& : = & Succinic\;acid\\ C{O}_{2}& : = & Carbon\;dioxide\end{array} |
The amino acid identity between HP4H and CP4H notwithstanding, there are significant differences between the molecular biology that they exhibit. This implies that despite the similarity of co-factor \left(iron\left(II\right)\right) , substrate \left(L-Proline\right) and co-substrate \left(2-oxoglutarate\right) , the binding affinities for molecular dioxygen vary considerably [7,32],
{Km}_{HP4H} = 0.1-0.76\;mM | (39) |
{Km}_{CP4H} = 0.03-1.5\;mM | (40) |
The turnover numbers for the cognate substrate, too, differ significantly [7],
{Kcat}_{HP4H} = 0.015-0.733{s}^{-1} | (41) |
{Kcat}_{CP4H} = 0.0188-0.02{s}^{-1} | (42) |
Clearly, a plausible explanation for these disparate empirical observations is the binding of the hypoxia-inducible factors (HIF) to P4H. The hypoxia-inducible factors (HIFs), are a family (n = 3) of transcription factors which sense hypoxia and trigger the upregulation of hypoxia-dependent genes [32,33,34]. Here, although hypoxia-inducible factor, is a full length protein, the actual binding site is the C-terminal end of HP4H [7,35].
Kd\approx 0.000016-0.023\;mM | (43) |
Some of these observations may be inferred from the partitioning of the transition-state dissociation constants into distinct subsets (Table 2):
Case 1 | Case 2 | |
Ligand \left(\boldsymbol{\mu }\in {\boldsymbol{\mathcal{L}}}\right) | Hypoxia-inducible factor | Peptide |
Macromolecule \left(\boldsymbol{c}\equiv {\boldsymbol{\mathcal{C}}}_{\boldsymbol{z}}\in \boldsymbol{\mathcal{C}}\right) | HP4H, CP4H | M1\beta |
Primary complex \left\langle {} \right.\boldsymbol{c}|\boldsymbol{\mu }\left. {} \right\rangle High-affinity variant :=\boldsymbol{K}\boldsymbol{d}\left({\boldsymbol{\mu }}_{\boldsymbol{h}\boldsymbol{i}\boldsymbol{g}\boldsymbol{h}}{\boldsymbol{\mathcal{C}}}_{\boldsymbol{z}}\right) Low-affinity variant :=\boldsymbol{K}\boldsymbol{d}\left({\boldsymbol{\mu }}_{\boldsymbol{h}\boldsymbol{i}\boldsymbol{g}\boldsymbol{h}}{\boldsymbol{\mathcal{C}}}_{\boldsymbol{z}}\right) |
\left\langle{\boldsymbol{H}\boldsymbol{P}{\bf{4}}\boldsymbol{H}|\boldsymbol{\mu }}\right\rangle , \left\langle{\boldsymbol{C}\boldsymbol{P}{\bf{4}}\boldsymbol{H}|\boldsymbol{\mu }}\right\rangle \boldsymbol{K}\boldsymbol{d}\left(\boldsymbol{H}\boldsymbol{P}{\bf{4}}\boldsymbol{H}|{\boldsymbol{\mu }}_{\boldsymbol{h}\boldsymbol{i}\boldsymbol{g}\boldsymbol{h}}\right)=\boldsymbol{K}\boldsymbol{d}\left({\boldsymbol{\mu }}_{\boldsymbol{h}\boldsymbol{i}\boldsymbol{g}\boldsymbol{h}}\boldsymbol{H}\boldsymbol{P}{\bf{4}}\boldsymbol{H}\right) \boldsymbol{K}\boldsymbol{d}\left(\boldsymbol{C}\boldsymbol{P}{\bf{4}}\boldsymbol{H}|{\boldsymbol{\mu }}_{\boldsymbol{l}\boldsymbol{o}\boldsymbol{w}}\right)=\boldsymbol{K}\boldsymbol{d}\left({\boldsymbol{\mu }}_{\boldsymbol{l}\boldsymbol{o}\boldsymbol{w}}\boldsymbol{C}\boldsymbol{P}{\bf{4}}\boldsymbol{H}\right) |
\left\langle{\boldsymbol{M}{\bf{1}}\boldsymbol{\beta }|\boldsymbol{\mu }}\right\rangle \boldsymbol{K}\boldsymbol{d}\left(\boldsymbol{M}{\bf{1}}\boldsymbol{\beta }|{\boldsymbol{\mu }}_{\boldsymbol{h}\boldsymbol{i}\boldsymbol{g}\boldsymbol{h}}\right)=\boldsymbol{K}\boldsymbol{d}\left({\boldsymbol{\mu }}_{\boldsymbol{h}\boldsymbol{i}\boldsymbol{g}\boldsymbol{h}}\boldsymbol{M}{\bf{1}}\boldsymbol{\beta }\right) \boldsymbol{K}\boldsymbol{d}\left(\boldsymbol{M}{\bf{1}}\boldsymbol{\beta }|{\boldsymbol{\mu }}_{\boldsymbol{l}\boldsymbol{o}\boldsymbol{w}}\right)=\boldsymbol{K}\boldsymbol{d}\left({\boldsymbol{\mu }}_{\boldsymbol{l}\boldsymbol{o}\boldsymbol{w}}\boldsymbol{M}{\bf{1}}\boldsymbol{\beta }\right) |
Higher-order complex \left\langle {} \right.\boldsymbol{c}|\boldsymbol{\mu }\left. {} \right\rangle .{{\mathcal{C}}}^{y} High-affinity variant :=\boldsymbol{K}\boldsymbol{d}\left({\boldsymbol{\mu }}_{\boldsymbol{h}\boldsymbol{i}\boldsymbol{g}\boldsymbol{h}}{\boldsymbol{\mathcal{C}}}_{\boldsymbol{z}}.{{\mathcal{C}}}^{y}\right) Low-affinity variant :=\boldsymbol{K}\boldsymbol{d}\left({\boldsymbol{\mu }}_{\boldsymbol{l}\boldsymbol{o}\boldsymbol{w}}{\boldsymbol{\mathcal{C}}}_{\boldsymbol{z}}.{{\mathcal{C}}}^{y}\right) |
--- --- --- |
\left\langle{\boldsymbol{M}{\bf{1}}\boldsymbol{\beta }|\boldsymbol{\mu }}\right\rangle.PLC \boldsymbol{K}\boldsymbol{d}\left(\boldsymbol{M}{\bf{1}}\boldsymbol{\beta }|{\boldsymbol{\mu }}_{\boldsymbol{h}\boldsymbol{i}\boldsymbol{g}\boldsymbol{h}}.\boldsymbol{P}\boldsymbol{L}\boldsymbol{C}\right)=\boldsymbol{K}\boldsymbol{d}\left({\boldsymbol{\mu }}_{\boldsymbol{h}\boldsymbol{i}\boldsymbol{g}\boldsymbol{h}}\boldsymbol{M}{\bf{1}}\boldsymbol{\beta }.\boldsymbol{P}\boldsymbol{L}\boldsymbol{C}\right) \boldsymbol{K}\boldsymbol{d}\left(\boldsymbol{M}{\bf{1}}\boldsymbol{\beta }|{\boldsymbol{\mu }}_{\boldsymbol{l}\boldsymbol{o}\boldsymbol{w}}.\boldsymbol{P}\boldsymbol{L}\boldsymbol{C}\right)=\boldsymbol{K}\boldsymbol{d}\left({\boldsymbol{\mu }}_{\boldsymbol{l}\boldsymbol{o}\boldsymbol{w}}\boldsymbol{M}{\bf{1}}\boldsymbol{\beta }.\boldsymbol{P}\boldsymbol{L}\boldsymbol{C}\right) |
Functional relevance | {R}_{HP4H}\left(t\right)\gg {R}_{CP4H}\left(t\right) | M1\beta |{\mu }_{high}.PLC\to \alpha M1\beta (Anterograde) {M1\beta |\mu }_{low}.PLC\to rM1\beta (Retrograde) |
Note: {\boldsymbol{\mathcal{C}}}_{\boldsymbol{z}\boldsymbol{\mu }} : All pairwise-atom/residue based square interaction matrix of ligand and macromolecule, \left\langle {} \right.\boldsymbol{c}|\boldsymbol{\mu }\left. {} \right\rangle ; \mathit{\boldsymbol{\mathcal{K}}\boldsymbol{\mathcal{C}}}_{\boldsymbol{z}\boldsymbol{\mu }} : Diagonal matrix of the interactions of a ligand and macromolecule; {z}_{i=j}=z : Set of eigenvalues of \mathit{\boldsymbol{\mathcal{K}}\boldsymbol{\mathcal{C}}}_{\boldsymbol{z}\boldsymbol{\mu }} where z\in diag\left(\mathit{\boldsymbol{\mathcal{K}}\boldsymbol{\mathcal{C}}}_{\boldsymbol{z}\boldsymbol{\mu }}\right)\subset \mathbb{C} ; \boldsymbol{K}\boldsymbol{d}\left(\boldsymbol{c}\boldsymbol{\mu }\right) : Set of eigenvalue-based transition-state dissociation constants for monomer- and multimer-forms of ligand-macromolecular complexes, \left\{\omega \in \boldsymbol{K}\boldsymbol{d}\left(\boldsymbol{c}\boldsymbol{\mu }\right)={\alpha }_{i=j}=Re\left(z\right)\in \boldsymbol{K}\boldsymbol{d}\left({\boldsymbol{\mathcal{C}}}_{\boldsymbol{z}\boldsymbol{\mu }}\right)\cap \left(\mathrm{0, 1}\right)\right\} ; {\mu }_{high}, {\mu }_{low} : High- and low-affinity variants of an arbitrary ligand, \mu =\{{\mu }_{high}, {\mu }_{low}\}\in {\boldsymbol{\mathcal{L}}} ; \boldsymbol{\mu }{\boldsymbol{\mathcal{C}}}_{\boldsymbol{z}}.{{\mathcal{C}}}^{y} : Higher-order complex of ligand and macromolecule; \boldsymbol{K}\boldsymbol{i}\boldsymbol{n}\boldsymbol{f} : Subset of transition-state dissociation constants of ligand-macromolecular interaction; \boldsymbol{K}\boldsymbol{s}\boldsymbol{u}\boldsymbol{p} :Subset of transition-state dissociation constants of ligand-macromolecular interaction; R\left(t\right) : Rate of reaction; HP4H: Hypoxia stimulated- and Collagen Proline 4-Hydroxylase; CP4H: M1\beta : Heterodimer of major histocompatibility complex I (MHC1) with beta-2 microglobulin; PLC: Peptide loading complex. |
In particular, binding of HIF restricts the range of the binding affinity of HP4H for molecular oxygen significantly,
\frac{\Delta {Km}_{HP4H}}{\Delta {Km}_{CP4H}}\times 100\approx 45\% | (44) |
Here, the set of conformers of HP4H once bound to HIF ensures that the catalytic activity of HP4H for HIF is significantly reduced in the presence of hypoxia. This will extend the half-life of HIF and facilitate transcription of HIF-responsive genes [36,37]. In contrast, CP4H exhibits no such differential activity. Furthermore, there is a significant variation in the catalytic activity (turnover number) of these enzymes for their cognate substrate (L-Proline),
\frac{max\left({Kcat}_{HP4H}\right)-min\left({Kcat}_{HP4H}\right)}{max\left({Kcat}_{CP4H}\right)-min\left({Kcat}_{CP4H}\right)} = \frac{\Delta {Kcat}_{HP4H}}{\Delta {Kcat}_{CP4H}}\approx 600 | (45) |
These data suggest that a ligand when bound to a macromolecule can affect the rate at which the resulting complex assembles or disassembles and thereby influence biochemical function. Hence, partitioning the transition-state dissociation constants of the ligand-macromolecular complex into distinct subsets may offer valuable insights into the in vivo function of enzymes in physiological and pathological states (T4, C2, C3).
Case 2: Generic model of MHC1-mediated high-affinity peptide export
The peptide loading complex (PLC) is a higher-order (Z > 2;Tapasin, ERp57, MHC1) complex that assembles at the endoplasmic reticulum (ER)-membrane and functions to transport cytosolic peptides into the ER-lumen en route to the plasma membrane [38,39]. Whilst, regulation of this process, by Tapasin is well studied, the role of peptides and the possible mechanism(s) of action is unclear [40,41,42,43]. A low-affinity peptide-driven (LAPD)-model of the MHC1-mediated export of high-affinity peptides to the plasma membrane of nucleated cells has been proposed and investigated in silico [44]. A major proponent of this study was simulating the differential disassembly of PLC in response to peptides with varying affinities (high, low) for the MHC1- {\beta }_{2} -microglobulin [44]. In fact, data from the simulations suggested that low-affinity peptides may not only actively participate in the transport of high-affinity peptide export, but could also regulate the same [44]. Another interesting observation discussed was the role of low-affinity peptides in priming the MHC1-export apparatus, such that irrespective of the nature of the cellular insult (acute, chronic), export of high-affinity peptides was rapid, continuous and efficient [44].
Utilizing the partition schema for the transition-state dissociation constants from the current analysis (Table 2), we can model and rewrite the differential disassembly of the PLC,
\begin{array}{rr}\left\langle{\boldsymbol{M}{\bf{1}}\boldsymbol{\beta }|{\boldsymbol{\mu }}_{\boldsymbol{h}\boldsymbol{i}\boldsymbol{g}\boldsymbol{h}}}\right\rangle.PLC\begin{array}{c}\stackrel{{r}_{f}}{\to }\\ \underset{{r}_{b}}{\leftarrow }\end{array}Tapasin-ERp57+aM1\beta & RXN\left(4\right)\\ \left\langle{\boldsymbol{M}{\bf{1}}\boldsymbol{\beta }|{\boldsymbol{\mu }}_{\boldsymbol{l}\boldsymbol{o}\boldsymbol{w}}}\right\rangle.PLC\begin{array}{c}\stackrel{{r}_{f}}{\to }\\ \underset{{r}_{b}}{\leftarrow }\end{array}Tapasin-ERp57-{\mu }_{low}+rM1\beta & RXN\left(5\right)\end{array} |
\begin{array}{l}{r}_{f}, {r}_{b} : = Rate\;constants\;for\;forward\;and\;backward\;reactions\;at\;steady\;state\\ M1\beta : = Heterodimer\;of\;MHC1\;with\;beta-2\;microglobulin\\ {\mu }_{low} : = Low-affinity\;peptide\\ {\mu }_{high} : = High-affinity\;peptide\\ \left\langle{\boldsymbol{M}{\bf{1}}\boldsymbol{\beta }|{\boldsymbol{\mu }}_{\boldsymbol{l}\boldsymbol{o}\boldsymbol{w}}}\right\rangle : = Set\;of\;peptides\;with\;low\;affinity\;for\;MHC1\;and\;in\;complex\;with\;MHC1\\ \left\langle{\boldsymbol{M}{\bf{1}}\boldsymbol{\beta }|{\boldsymbol{\mu }}_{\boldsymbol{h}\boldsymbol{i}\boldsymbol{g}\boldsymbol{h}}}\right\rangle : = Set\;of\;peptides\;with\;high\;affinity\;for\;MHC1\;and\;incomplex\;with\;MHC1\\ eM1\beta : = Net\;exportable\;complex\;of\;high-affinity\;peptide\;with\;MHC1\\ aM1\beta : = Anterograde-derived\;exportable\;complex\;of\;high-affinity\;peptide\;with\;MHC1\\ rM1\beta : = Retrograde-derived\;exportable\;complex\;of\;high-affinityp\;eptide\;with\;MHC1\\ PLC : = Peptide\;loading\;complex\\ ERp57 : = Endoplasmic\;reticulum\;protein\;disulfide\;isomerase\end{array} |
The appropriate dissociation constants are,
![]() |
(46) |
![]() |
(47) |
Rewriting these equations in terms of the peptide-bound MHC1,
{Kd}_{RXN4} = \frac{\zeta }{{\left[M1\beta |{\mu }_{high}\right]}^{{L}_{M1\beta |{\mu }_{high}}\ge 0}} | (48) |
where,
\zeta = \frac{\left[Tapasin-ERp57\right]\left[aM1\beta \right]}{{\left[PLC\right]}^{{L}_{PLC}\ge 0}} | (48.1) |
{Kd}_{RXN5} = \frac{\zeta }{{\left[M1\beta |{\mu }_{low}\right]}^{{L}_{M1\beta |{\mu }_{low}}\ge 0}} | (49) |
where,
\zeta = \frac{\left[Tapasin-ERp57-{\mu }_{low}\right]\left[rM1\beta \right]}{{\left[PLC\right]}^{{L}_{PLC}\ge 0}} | (49.1) |
Clearly,
{Kd}_{RXN4}\propto \frac{1}{{\left[M1\beta |{\mu }_{high}\right]}^{{L}_{M1\beta |{\mu }_{high}}\ge 0}} | (50) |
{Kd}_{RXN5}\propto \frac{1}{{\left[M1\beta |{\mu }_{low}\right]}^{{L}_{M1\beta |{\mu }_{low}}\ge 0}} | (51) |
These results suggest that,
{Kd}_{RXN4}\simeq 1.0\left(Perfect\;disassociation\right)and\left[aM1\beta \right]\to \infty | (52) |
{Kd}_{RXN5}\simeq 0.0\left(Perfect\;association\right)and\left[rM1\beta \right]\to 0 | (53) |
and is in accordance with existing empirical and simulation data,
\left[{\mu }_{high}\right] < < < \left[{\mu }_{low}\right], \left[aM1\beta \right] > > > \left[rM1\beta \right] | (54) |
Here, the partitioning of transition-state dissociation constants into low- and high-affinity peptides for the MHC1 can provide valuable insights into the underlying molecular biology of MHC1-mediated high-affinity peptide transport under physiological and pathological conditions (T5–T7, P) [42,43,44].
The work presented models ligand-macromolecular interactions as eigenvalue-based transition-state disassociation constants. The interaction matrix is an all-atom/residue pairwise comparison between the ligand and macromolecule and comprises numerical values drawn randomly from a standard uniform distribution. The transition-state dissociation constants are the strictly positive real part of all complex eigenvalues of this ligand-macromolecular interaction matrix, belong to the open interval (0, 1) and form a sequence whose terms are finite, monotonic, non-increasing and convergent. The findings are rigorous, numerically robust and can be extended to higher-order complexes. The study, additionally, suggests a schema by which a ligand may be partitioned into high- and low-affinity variants. This study, although theoretical offers a plausible explanation into the underlying biochemistry (enzyme-mediated substrate catalysis, assembly/disassembly and inhibitor kinetics) of ligand-macromolecular complexes. Future investigations may include assigning weights to each interaction, investigating origins of co-operativity in enzyme catalysis and inhibitory kinetics amongst others.
This section provides formal proofs for the included theorems, corollaries and proposition.
Proof (T1):
From Defs. (4) and (5),
{\text{For every}}\; \omega \in \boldsymbol{K}\boldsymbol{d}\left(\boldsymbol{c}\boldsymbol{\mu }\right)\exists g\left(\omega \right)\in \boldsymbol{K}\boldsymbol{d}\left(\boldsymbol{\mathcal{C}}_{\boldsymbol{z}\boldsymbol{\mu }}\right)|{g}^{-1}\circ g\left(\omega \right) = \omega | (55) |
Let,
{\omega }_{x}, {\omega }_{y}\in \boldsymbol{K}\boldsymbol{d}\left(\boldsymbol{c}\boldsymbol{\mu }\right)|g\left({\omega }_{x}\right), g\left({\omega }_{y}\right)\in \boldsymbol{K}\boldsymbol{d}\left(\boldsymbol{\mathcal{C}}_{\boldsymbol{z}\boldsymbol{\mu }}\right);x\ne y;\left\{x, y\right\}\le A |
If,
g\left({\omega }_{x}\right) = g\left({\omega }_{y}\right) | (56) |
then,
{g}^{-1}\circ g\left({\omega }_{x}\right) = {g}^{-1}\circ g\left({\omega }_{y}\right) | (57) |
\Rightarrow {\omega }_{x} = {\omega }_{y} | (58) |
If,
t = 0|t\in \boldsymbol{K}\boldsymbol{d}\left(\boldsymbol{\mathcal{C}}_{\boldsymbol{z}\boldsymbol{\mu }}\right) | (59) |
From (55),
{g}^{-1}\circ g\left(t\right) = 0\notin \boldsymbol{K}\boldsymbol{d}\left(\boldsymbol{c}\boldsymbol{\mu }\right) | (60) |
Similarly, For,
t\ge 1|t\in \boldsymbol{K}\boldsymbol{d}\left(\boldsymbol{\mathcal{C}}_{\boldsymbol{z}\boldsymbol{\mu }}\right) | (61) |
From (55),
{g}^{-1}\circ g\left(\omega \right)\ge 1\notin \boldsymbol{K}\boldsymbol{d}\left(\boldsymbol{c}\boldsymbol{\mu }\right) | (62) |
From (58), (60) and (62),
Proof (T2): (By induction)
For a = 1 ,
{\omega }_{a} = \mathrm{max}\left(\boldsymbol{K}\boldsymbol{d}\left(\boldsymbol{c}\boldsymbol{\mu }\right)\right) < 1 \ \ \ \ \ \ \ \ \ \ {\rm{(By\ Def.\ (5)), }} | (63) |
Assume a = A-1 ,
{\omega }_{a}\ge {\omega }_{A-1} | (64) |
Then \exists a = A ,
{\omega }_{a}\ge {\omega }_{A-1}\ge {\omega }_{A} | (65) |
For a = 1 ,
{\omega }_{a} = \mathrm{min}\left(\boldsymbol{K}\boldsymbol{d}\left(\boldsymbol{c}\boldsymbol{\mu }\right)\right) > 0 \ \ \ \ \ \ \ \ \ \ ({\rm{By}}\ Def.\ (5)), | (66) |
{\omega }_{a}\le {\omega }_{A-1} | (67) |
{\Rightarrow 0 < \left\{{\omega }_{a}\right\}}_{a\le a+1}\sim \boldsymbol{K}\boldsymbol{d}\left(\boldsymbol{c}\boldsymbol{\mu }\right) < 1 \forall \omega |
Proof (T3):
From (T2),
{\left\{{\omega }_{a}\right\}}_{a\le a+1} < 1 |
Choose \varepsilon \in {\mathbb{R}}_{+}, \varepsilon \to 0 ,
{\varepsilon }^{a > A} < \left|{\omega }_{a > A}-1\right| < \varepsilon |
For every a > A ,
\begin{array}{l} \left|\underset{a\to \infty }{\mathrm{lim}}{\omega }_{a > A}-1\right| < \varepsilon \\ \underset{a\to \infty }{\mathrm{lim}}|{\omega }_{a > A}-1| < \varepsilon\\ \ \ \ {\underset{a\to \infty }{\mathrm{lim}}{\omega }_{a > A}} = 1 \end{array} | (69) |
Similarly,
{\left\{{\omega }_{a}\right\}}_{a\le a+1} > 0 |
Choose \varepsilon \in {\mathbb{R}}_{+}, \varepsilon \to 0 ,
\frac{1}{\varepsilon } > \left|{\omega }_{a > A}-0\right| > \varepsilon |
For every a > A ,
\begin{array}{c} \left|\underset{a\to \infty }{\mathrm{lim}}{\omega }_{a > A}-0\right| > \varepsilon \\ \underset{a\to \infty }{\mathrm{lim}}|{\omega }_{a > A}-0| > \varepsilon \\ \underset{a\to \infty }{\mathrm{lim}}{\omega }_{a > A} = 0 \end{array} | (70) |
From (69) and (70),
\underset{a\to \infty }{\mathrm{lim}}{\left\{{\omega }_{a}\right\}}_{a\le a+1} = \left\{\mathrm{0, 1}\right\} |
Proof (C1):
Assume,
sup{\left\{{\omega }_{a}\right\}}_{a\le a+1} = max{\left\{{\omega }_{a}\right\}}_{a\le a+1} = {\omega }_{a = 1} |
Choose \varepsilon \in {\mathbb{R}}_{+}, \varepsilon \to 0
then for any a = \mathrm{1, 2}..A , we can find,
{\varepsilon }^{A}.{\omega }_{a = 1}⋘{\omega }_{a = 1} | (71) |
{\varepsilon }^{A}.{\omega }_{a = 1}\le {\omega }_{a = A} | (72) |
{\omega }_{a = 1}\le {\omega }_{a = A}.\left(\frac{1}{{\varepsilon }^{A}}\right) | (73) |
Let \delta \in {\mathbb{R}}_{+}, \delta \to 0 ,
{\omega }_{a = 1}-\delta < {\omega }_{a = A}.\left(\frac{1}{{\varepsilon }^{A}}\right) | (74) |
Assume,
inf{\left\{{\omega }_{a}\right\}}_{a\le a+1} = min{\left\{{\omega }_{a}\right\}}_{a\le a+1} = {\omega }_{a = A} |
Choose \varepsilon \in {\mathbb{R}}_{+}, \varepsilon \to 0
then for any a = \mathrm{1, 2}..A , we can find,
{\varepsilon }^{A}.{\omega }_{a = A} < {\omega }_{a = A} | (75) |
{\omega }_{a = A}⋘{\omega }_{a = A}.\left(\frac{1}{{\varepsilon }^{A}}\right) | (76) |
{\omega }_{a = 1}\le {\omega }_{a = 1}.\left(\frac{1}{{\varepsilon }^{A}}\right) | (77) |
Let \delta \in {\mathbb{R}}_{+}, \delta \to 0 ,
{\omega }_{a = 1} < {\omega }_{a = 1}.\left(\frac{1}{{\varepsilon }^{A}}\right)+\delta | (78) |
From (74) and (78),
Proof (T4):
From (T2 and T3), (C1 and C2)
Case (1)
If
{\left\{{\omega }_{a}\right\}}_{a > A} = \boldsymbol{K}\boldsymbol{s}\boldsymbol{u}\boldsymbol{p}\subset \boldsymbol{K}\boldsymbol{d}\left({\boldsymbol{\mathcal{C}}}_{\boldsymbol{z}\boldsymbol{\mu }}\right) |
then,
{\left\{{\omega }_{a}\right\}}_{a\le A}\in \boldsymbol{K}\boldsymbol{i}\boldsymbol{n}\boldsymbol{f}\sim \boldsymbol{K}\boldsymbol{d}\left(\boldsymbol{c}\boldsymbol{\mu }\right) | (79) |
Case (2)
If
{\left\{{\omega }_{a}\right\}}_{a > A} = \boldsymbol{K}\boldsymbol{i}\boldsymbol{n}\boldsymbol{f}\subset \boldsymbol{K}\boldsymbol{d}\left({\boldsymbol{\mathcal{C}}}_{\boldsymbol{z}\boldsymbol{\mu }}\right) |
then,
{\left\{{\omega }_{a}\right\}}_{a\le A}\in \boldsymbol{K}\boldsymbol{s}\boldsymbol{u}\boldsymbol{p}\sim \boldsymbol{K}\boldsymbol{d}\left(\boldsymbol{c}\boldsymbol{\mu }\right) | (80) |
From (79) and (80),
\boldsymbol{K}\boldsymbol{i}\boldsymbol{n}\boldsymbol{f}\cap \boldsymbol{K}\boldsymbol{s}\boldsymbol{u}\boldsymbol{p} = \left\{{\varnothing }\right\} | (81) |
Since,
\boldsymbol{K}\boldsymbol{d}\left({\boldsymbol{\mathcal{C}}}_{\boldsymbol{z}\boldsymbol{\mu }}\right)\supset \left(\boldsymbol{K}\boldsymbol{i}\boldsymbol{n}\boldsymbol{f}\cup \boldsymbol{K}\boldsymbol{s}\boldsymbol{u}\boldsymbol{p}\right)\cup \left(\boldsymbol{K}\boldsymbol{i}\boldsymbol{n}\boldsymbol{f}\cap \boldsymbol{K}\boldsymbol{s}\boldsymbol{u}\boldsymbol{p}\right) |
From (79)–(81),
\begin{array}{l} \boldsymbol{K}\boldsymbol{d}\left({\boldsymbol{\mathcal{C}}}_{\boldsymbol{z}\boldsymbol{\mu }}\right)\supset \boldsymbol{K}\boldsymbol{i}\boldsymbol{n}\boldsymbol{f}\cup \boldsymbol{K}\boldsymbol{s}\boldsymbol{u}\boldsymbol{p}\\ \ \ \ \ \ \Rightarrow \boldsymbol{K}\boldsymbol{d}\left(\boldsymbol{c}\boldsymbol{\mu }\right)\subset \boldsymbol{K}\boldsymbol{d}\left({\boldsymbol{\mathcal{C}}}_{\boldsymbol{z}\boldsymbol{\mu }}\right) \end{array} | (82) |
Proof (C3):
If,
\exists \mu \in {\boldsymbol{\mathcal{L}}}|\#\boldsymbol{K}\boldsymbol{s}\boldsymbol{u}\boldsymbol{p} > > > \#\boldsymbol{K}\boldsymbol{i}\boldsymbol{n}\boldsymbol{f}\forall a |
Then,
\mu \equiv {\mu }_{low} | (83) |
Similarly, if,
\exists \mu \in {\boldsymbol{\mathcal{L}}}|\#\boldsymbol{K}\boldsymbol{i}\boldsymbol{n}\boldsymbol{f} > > > \#\boldsymbol{K}\boldsymbol{s}\boldsymbol{u}\boldsymbol{p}\forall a |
Then,
\mu \equiv {\mu }_{high} | (84) |
From (83) and (84),
Proof (T5) (By induction)
Assume z = 1;Z\ge 2 , y = 1;Z = 2 ,
{\mathcal{C}}_{1}.{\prod }_{y = 1}^{y = Y = Z-1}{\mathcal{C}}_{y} = {\mathcal{C}}_{1}.{\prod }_{y = 1}^{y = 1}{\mathcal{C}}_{y} | (85) |
= {\mathcal{C}}_{1}.{\mathcal{C}}_{Y} | (85.1) |
= {\mathcal{C}}_{1}.{\mathcal{C}}^{Y} | (85.2) |
Assume truth for y = Y\gg 1 ,
{\mathcal{C}}_{1}.{\prod }_{y = 1}^{y = Y = Z-1}{\mathcal{C}}_{y} = {\mathcal{C}}_{z}.\left({\mathcal{C}}_{1}{\dots .{\mathcal{C}}}_{Y}\right) | (86) |
= {\mathcal{C}}_{1}.{\mathcal{C}}^{Y} | (86.1) |
For y = Y+1 ,
{\mathcal{C}}_{1}.{\prod }_{y = 1}^{y = Y+1}{\mathcal{C}}_{y} = {\mathcal{C}}_{1}.{\prod }_{y = 1}^{y = Y+1}{\mathcal{C}}_{y} | (87) |
= {\mathcal{C}}_{1}.\left({\prod }_{y = 1}^{y = Y}{\mathcal{C}}_{y}\right).\left({\prod }_{y = 1}^{y = 1}{\mathcal{C}}_{y}\right) | (87.1) |
= {\mathcal{C}}_{1}.{\mathcal{C}}_{Y}^{Y}.{\mathcal{C}}_{1}^{1} | (87.2) |
= {\mathcal{C}}_{1}.{\mathcal{C}}^{Y+1} | (87.3) |
From (85)–(87),
Proof (T6):
From Defs. (6–8),
\mu {\mathcal{C}}_{z}.{\mathcal{C}}^{Y}\equiv \mu {\mathcal{C}}_{z}\equiv ⟨\boldsymbol{c}|\boldsymbol{\mu }⟩ | (88) |
\Rightarrow \boldsymbol{K}\boldsymbol{d}\left(\boldsymbol{\mu }{\boldsymbol{\mathcal{C}}}_{\boldsymbol{z}}.{\boldsymbol{\mathcal{C}}}^{\boldsymbol{Y}}\right)\sim \boldsymbol{K}\boldsymbol{d}\left(\boldsymbol{c}\boldsymbol{\mu }\right) | (89) |
\Rightarrow \boldsymbol{K}\boldsymbol{d}\left(\boldsymbol{\mu }{\boldsymbol{\mathcal{C}}}_{\boldsymbol{z}}.{\boldsymbol{\mathcal{C}}}^{\boldsymbol{Y}}\right)\cap \boldsymbol{K}\boldsymbol{d}\left(\boldsymbol{c}\boldsymbol{\mu }\right) | (90) |
From Def. (5),
{\omega }_{a\in [1, A]}\in \left(\boldsymbol{K}\boldsymbol{d}\left(\boldsymbol{\mu }{\boldsymbol{\mathcal{C}}}_{\boldsymbol{z}}.{\boldsymbol{\mathcal{C}}}^{\boldsymbol{Y}}\right)\cap \boldsymbol{K}\boldsymbol{d}\left(\boldsymbol{c}\boldsymbol{\mu }\right)\right) | (91) |
\left({\omega }_{a\in [1, A]}\in \boldsymbol{K}\boldsymbol{d}\left(\boldsymbol{\mu }{\boldsymbol{\mathcal{C}}}_{\boldsymbol{z}}.{\boldsymbol{\mathcal{C}}}^{\boldsymbol{Y}}\right)\right)\cap \left({\omega }_{a\in [1, A]}\in \boldsymbol{K}\boldsymbol{d}\left(\boldsymbol{c}\boldsymbol{\mu }\right)\right) | (92) |
From (T2–T4), (C1–C3),
\forall a\left({\omega }_{a = \mathrm{1, 2}\dots A}\subseteq \boldsymbol{K}\boldsymbol{d}\left(\boldsymbol{\mu }{\boldsymbol{\mathcal{C}}}_{\boldsymbol{z}}.{\boldsymbol{\mathcal{C}}}^{\boldsymbol{Y}}\right)\right)\cap \left({\omega }_{a = \mathrm{1, 2}\dots A}\subseteq \boldsymbol{K}\boldsymbol{d}\left(\boldsymbol{c}\boldsymbol{\mu }\right)\right) | (93) |
\Rightarrow \left({\left\{{\omega }_{a}\right\}}_{a = \mathrm{1, 2}\dots A}\sim \boldsymbol{K}\boldsymbol{d}\left(\boldsymbol{\mu }{\boldsymbol{\mathcal{C}}}_{\boldsymbol{z}}.{\boldsymbol{\mathcal{C}}}^{\boldsymbol{Y}}\right)\right)\cap \left({\left\{{\omega }_{a}\right\}}_{a = \mathrm{1, 2}\dots A}\sim \boldsymbol{K}\boldsymbol{d}\left(\boldsymbol{c}\boldsymbol{\mu }\right)\right) | (94) |
Conversely, let,
u\in \boldsymbol{K}\boldsymbol{d}\left(\boldsymbol{\mu }{\boldsymbol{\mathcal{C}}}_{\boldsymbol{z}}.{\boldsymbol{\mathcal{C}}}^{\boldsymbol{Y}}\right);\omega \in \boldsymbol{K}\boldsymbol{d}\left(\boldsymbol{c}\boldsymbol{\mu }\right) |
From (92) and (94),
\forall \omega \in \boldsymbol{K}\boldsymbol{d}\left(\boldsymbol{c}\boldsymbol{\mu }\right)\exists u\in \boldsymbol{K}\boldsymbol{d}\left(\boldsymbol{\mu }{\boldsymbol{\mathcal{C}}}_{\boldsymbol{z}}.{\boldsymbol{\mathcal{C}}}^{\boldsymbol{Y}}\right)|u = h\left(\omega \right) | (95) |
\forall u\in \boldsymbol{K}\boldsymbol{d}\left(\boldsymbol{\mu }{\boldsymbol{\mathcal{C}}}_{\boldsymbol{z}}.{\boldsymbol{\mathcal{C}}}^{\boldsymbol{Y}}\right)\exists \omega \in \boldsymbol{K}\boldsymbol{d}\left(\boldsymbol{c}\boldsymbol{\mu }\right)|\omega = {h}^{-1}\left(u\right) = {h}^{-1}\left(h\left(\omega \right)\right) | (96) |
From (95) and (96),
Proof (T7):
From (T6),
{h}^{-1}\circ h:\omega \in \boldsymbol{K}\boldsymbol{d}\left(\boldsymbol{c}\boldsymbol{\mu }\right) |
From (T1), Def. (4)
g:\omega \in \boldsymbol{K}\boldsymbol{d}\left(\boldsymbol{c}\boldsymbol{\mu }\right)\mapsto \boldsymbol{K}\boldsymbol{d}\left(\boldsymbol{c}\boldsymbol{\mu }\right)\cup \mathbb{R} = \boldsymbol{K}\boldsymbol{d}\left({\boldsymbol{\mathcal{C}}}_{\boldsymbol{z}\boldsymbol{\mu }}\right) |
Rewriting,
g:\omega \mapsto \boldsymbol{K}\boldsymbol{d}\left({\boldsymbol{\mathcal{C}}}_{\boldsymbol{z}\boldsymbol{\mu }}\right) | (97) |
g\left({h}^{-1}\left(u\right)\right)\mapsto \boldsymbol{K}\boldsymbol{d}\left({\boldsymbol{\mathcal{C}}}_{\boldsymbol{z}\boldsymbol{\mu }}\right) | (98) |
g\circ {h}^{-1}\left(h\left(\omega u\right)\right)\mapsto \boldsymbol{K}\boldsymbol{d}\left({\boldsymbol{\mathcal{C}}}_{\boldsymbol{z}\boldsymbol{\mu }}\right) | (99) |
g\circ {h}^{-1}\circ h\left(\omega \right)\mapsto \boldsymbol{K}\boldsymbol{d}\left({\boldsymbol{\mathcal{C}}}_{\boldsymbol{z}\boldsymbol{\mu }}\right) | (100) |
Proof (P):
From (T1–T7) and Defs. (4–8, 10),
For z = Z = 1 ,
{\mu {\mathcal{C}}}_{z}.{\mathcal{C}}^{Y} = \mu {\mathcal{C}}_{z} | (101) |
g\circ {h}^{-1}\left(u\in \boldsymbol{K}\boldsymbol{d}\left(\boldsymbol{\mu }{\boldsymbol{\mathcal{C}}}_{\boldsymbol{z}}\right)\right)\mapsto \boldsymbol{K}\boldsymbol{d}\left({\boldsymbol{\mathcal{C}}}_{\boldsymbol{z}\boldsymbol{\mu }}\right)\subset \mathbb{R} | (102) |
g\circ {h}^{-1}\circ h\left(\omega \right)\mapsto \boldsymbol{K}\boldsymbol{d}\left({\boldsymbol{\mathcal{C}}}_{\boldsymbol{z}\boldsymbol{\mu }}\right) | (102.1) |
\Rightarrow \boldsymbol{K}\boldsymbol{d}\left(\boldsymbol{\mu }{\boldsymbol{\mathcal{C}}}_{\boldsymbol{z}}\right)\subset \boldsymbol{K}\boldsymbol{d}\left({\boldsymbol{\mathcal{C}}}_{\boldsymbol{z}\boldsymbol{\mu }}\right) | (103) |
For Z\ge 2 ,
g\circ {h}^{-1}\left(u\in \boldsymbol{K}\boldsymbol{d}\left(\boldsymbol{\mu }{\boldsymbol{\mathcal{C}}}_{\boldsymbol{z}}.{\boldsymbol{\mathcal{C}}}^{\boldsymbol{Y}}\right)\right)\mapsto \boldsymbol{K}\boldsymbol{d}\left({\boldsymbol{\mathcal{C}}}_{\boldsymbol{z}\boldsymbol{\mu }}\right)\subset \mathbb{R} | (104) |
g\circ {h}^{-1}\circ h\left(\omega \right)\mapsto \boldsymbol{K}\boldsymbol{d}\left({\boldsymbol{\mathcal{C}}}_{\boldsymbol{z}\boldsymbol{\mu }}\right) | (104.1) |
\Rightarrow \boldsymbol{K}\boldsymbol{d}\left(\boldsymbol{\mu }{\boldsymbol{\mathcal{C}}}_{\boldsymbol{z}}.{\boldsymbol{\mathcal{C}}}^{\boldsymbol{Y}}\right)\subset \boldsymbol{K}\boldsymbol{d}\left({\boldsymbol{\mathcal{C}}}_{\boldsymbol{z}\boldsymbol{\mu }}\right) | (105) |
From (103) and (105),
This work is funded by an early career intramural grant (Code No. A-766) awarded to SK by the All India Institute of Medical Sciences (AIIMS, New Delhi, INDIA).
The author declare there is no conflict of interest.
[1] | Nuryani Y, Emmyzar W (2006) Budidaya tanaman nilam. Balai Penelit Tanam Rempah Dan Aromat Pus Penelit Dan Pengemb Perkeb Badan Penelit Dan Pengemb Pertan. Available from: http://nad.litbang.pertanian.go.id/ind/images/dokumen/modul/19-Budidaya%20tanaman%20Nilam1.pdf. |
[2] | Setiawan RR (2013) Produktivitas nilam nasional semakin menurun (45% total areal pertanaman nilam di Indonesia produksinya < 150 kg/ha). Warta Penelitian Pengembangan Tanaman Industri 19: 8-11. Available from: http://perkebunan.litbang.pertanian.go.id/id/produktivitas-nilam-nasional-semakin-menurun/. |
[3] |
Singh RP, Manchanda G, Maurya IK, et al. (2019) Streptomyces from rotten wheat straw endowed the high plant growth potential traits and agro-active compounds. Biocatal Agric Biotechnol 17: 507-513. doi: 10.1016/j.bcab.2019.01.014
![]() |
[4] |
Prajakta BM, Suvarna PP, Raghvendra SP, et al. (2019) Potential biocontrol and superlative plant growth promoting activity of indigenous Bacillus mojavensis PB-35 (R11) of soybean (Glycine max) rhizosphere. SN Appl Sci 1: 1143. doi: 10.1007/s42452-019-1149-1
![]() |
[5] |
Schä;fer M, Brütting C, Meza-Canales ID, et al. (2015) The role of cis-zeatin-type cytokinins in plant growth regulation and mediating responses to environmental interactions. J Exp Bot 66: 4873-4884. doi: 10.1093/jxb/erv214
![]() |
[6] | Sulis S, Pangesti R (2015) Pengaruh pemberian air tauge dan air kelapa terhadap pertumbuhan tunas nilam (Pogestemon cablin Benth) secara in vitro. STIGMA J Mat Dan Ilmu Pengetah Alam Unipa 8. |
[7] |
Ružić DV, Vujović T (2008) The effects of cytokinin types and their concentration on in vitro multiplication of sweet cherry cv. Lapins (Prunus avium L.). Hortic Sci 35: 12-21. doi: 10.17221/646-HORTSCI
![]() |
[8] | Saburu DV, Polii B, Pinaria A, et al. (2016) Pengaruh zeatin terhadap multiplikasi tunas eksplan nodus pada tanaman krisan varietas kulo dan puspita nusantara. COCOS 7: 1-8. |
[9] |
Peixe A, Raposo A, Lourenço R, et al. (2007) Coconut water and BAP successfully replaced zeatin in olive (Olea europaea L.) micropropagation. Sci Hortic 113: 1-7. doi: 10.1016/j.scienta.2007.01.011
![]() |
[10] |
Ali EF, Hassan FAS, Elgimabi M (2018) Improving the growth, yield and volatile oil content of Pelargonium graveolens L. Herit by foliar application with moringa leaf extract through motivating physiological and biochemical parameters. South Afr J Bot 119: 383-389. doi: 10.1016/j.sajb.2018.10.003
![]() |
[11] |
Vongsak B, Sithisarn P, Mangmool S, et al. (2013) Maximizing total phenolics, total flavonoids contents and antioxidant activity of Moringa oleifera leaf extract by the appropriate extraction method. Ind Crops Prod 44: 566-571. doi: 10.1016/j.indcrop.2012.09.021
![]() |
[12] | Krisnadi A (2015) Kelor Super Nutrisi Edisi Revisi. Available from: https://kelorina.com/ebook.pdf. |
[13] |
Bholah K, Ramful‐Baboolall D, Neergheen‐Bhujun VS (2015) Antioxidant Activity of Polyphenolic Rich M oringa oleifera L am. Extracts in Food Systems. J Food Biochem 39: 733-741. doi: 10.1111/jfbc.12181
![]() |
[14] | Anwar F, Latif S, Ashraf M, et al. (2007) Moringa oleifera: a food plant with multiple medicinal uses. Phytother Res: Int J Devoted Pharmacol Toxicol Eval Nat Prod Deriv 21: 17-25. |
[15] | Ashfaq M, Basra SM, Ashfaq U (2012) Moringa: a miracle plant for agro-forestry. J Agric Soc Sci 8. |
[16] | Suminar E, Anjarsari IRD, Nuraini A, et al. (2015) Pertumbuhan dan perkembangan tunas nilam var. Lhoukseumawe dari jenis eksplan dengan sitokinin yang berbeda secara in vitro. Kultivasi 14. |
[17] |
Susilowati A, Listyawati S (2001) Keanekaragaman jenis Mikroorganisme sumber kontaminasi kultur In Vitro di Sub Lab Biologi laboratorium MIPA Pusat UNS. Biodiversitas 2: 110-114. doi: 10.13057/biodiv/d020105
![]() |
[18] |
Coppin JP, Xu Y, Chen H, et al. (2013) Determination of flavonoids by LC/MS and anti-inflammatory activity in Moringa oleifera. J Funct Foods 5: 1892-1899. doi: 10.1016/j.jff.2013.09.010
![]() |
[19] |
Singh RG, Negi PS, Radha C (2013) Phenolic composition, antioxidant and antimicrobial activities of free and bound phenolic extracts of Moringa oleifera seed flour. J Funct Foods 5: 1883-1891. doi: 10.1016/j.jff.2013.09.009
![]() |
[20] |
Rodríguez-Pérez C, Quirantes-Piné R, Fernández-Gutiérrez A, et al. (2015) Optimization of extraction method to obtain a phenolic compounds-rich extract from Moringa oleifera Lam leaves. Ind Crops Prod 66: 246-254. doi: 10.1016/j.indcrop.2015.01.002
![]() |
[21] |
Singh AK, Rana HK, Tshabalala T, et al. (2020) Phytochemical, nutraceutical and pharmacological attributes of a functional crop Moringa oleifera Lam: An overview. South Afr J Bot 129: 209-220. doi: 10.1016/j.sajb.2019.06.017
![]() |
[22] | Munggarani M, Suminar E, Nuraini A, et al. (2018) Multiplikasi Tunas Meriklon Kentang Pada Berbagai Jenis dan Konsentrasi Sitokinin. Agrologia 7. |
[23] | Phiri C, Mbewe DN (2010) Influence of Moringa oleifera leaf extracts on germination and seedling survival of three common legumes. Int J Agric Biol 12: 315-317. |
[24] | Nouman W, Siddiqui MT, Basra SMA (2012) Moringa oleifera leaf extract: An innovative priming tool for rangeland grasses. Turk J Agric For 36: 65-75. |
[25] | Yasmeen A, Basra SMA, Wahid A, et al. (2013) Exploring the potential of Moringa oleifera leaf extract (MLE) as a seed priming agent in improving wheat performance. Turk J Bot 37: 512-520. |
[26] |
Yasmeen A, Nouman W, Basra SMA, et al. (2014) Morphological and physiological response of tomato (Solanumlycopersicum L.) to natural and synthetic cytokinin sources: a comparative study. Acta Physiol Plant 36: 3147-3155. doi: 10.1007/s11738-014-1662-1
![]() |
[27] | Kasutjianingati RP, Khumaida N, Efendi D (2010) Kemampuan pecah tunas dan kemampuan berbiak mother plant pisang Rajabulu (AAB) dan pisang Tanduk (AAB) dalam medium inisiasi in vitro. Agriplus 20: 39-46. |
[28] |
Ulvskov P, Nielsen TH, Seiden P, et al. (1992) Cytokinins and leaf development in sweet pepper (Capsicum annuum L.). Planta 188: 70-77. doi: 10.1007/BF01160714
![]() |
[29] |
Chen JT, Chang C, Chang WC (1999) Direct somatic embryogenesis on leaf explants of Oncidium Gower Ramsey and subsequent plant regeneration. Plant Cell Rep 19: 143-149. doi: 10.1007/s002990050724
![]() |
[30] |
Chen JT, Chang WC (2001) Effects of auxins and cytokinins on direct somatic embryogenesison leaf explants of Oncidium'Gower Ramsey'. Plant Growth Regul 34: 229-232. doi: 10.1023/A:1013304101647
![]() |
[31] |
Gajdošová S, Spíchal L, Kamínek M, et al. (2011) Distribution, biological activities, metabolism, and the conceivable function of cis-zeatin-type cytokinins in plants. J Exp Bot 62: 2827-2840. doi: 10.1093/jxb/erq457
![]() |
[32] |
Rosniawaty S, Anjarsari IRD, Sudirja R (2019) Aplikasi sitokinin untuk meningkatkan pertumbuhan tanaman teh di dataran rendah. Jurnal Tanaman Industri dan Penyegar 5: 31. doi: 10.21082/jtidp.v5n1.2018.p31-38
![]() |
[33] | Kieber JJ, Schaller GE (2014) Cytokinins. Arab Book: American Soc Plant Biol 12. |
[34] |
Hwang I, Sheen J, Müller B (2012) Cytokinin signaling networks. Annu Rev Plant Biol 63: 353-380. doi: 10.1146/annurev-arplant-042811-105503
![]() |
[35] | George EF, Hall MA, De Klerk GJ (2008) Plant growth regulators Ⅱ: cytokinins, their analogues and antagonists. Plant propagation by tissue culture, Springer, 205-226. |
[36] | Sholikhah EN, Wijayanti MA (2006) In vitro antiplasmodial activity and cytotoxicity of new n-benzyl 1, 10-phenanthroline derivatives. Southeast Asian J Trop Med Public Health 37: 1072-1077. |
[37] |
Seswita D (2020) Penggunaan air kelapa sebagai zat pengatur tumbuh pada multiplikasi tunas temulawak (Curcuma xanthorrhiza Roxb.) in vitro. Jurnal Penelitian Tanaman Industri 16: 135-140. doi: 10.21082/jlittri.v16n4.2010.135-140
![]() |
[38] |
Kuraishi S, Muir RM (1964) The relationship of gibberellin and auxin in plant growth. Plant Cell Physiol 5: 61-69. doi: 10.1093/oxfordjournals.pcp.a079024
![]() |
[39] |
Ockerse R, Galston AW (1967) Gibberellin-auxin interaction in pea stem elongation. Plant Physiol 42: 47-54. doi: 10.1104/pp.42.1.47
![]() |
[40] | Dalessandro G (1973) Interaction of auxin, cytokinin, and gibberellin on cell division and xylem differentiation in cultured explants of Jerusalem artichoke. Plant Cell Physiol 14: 1167-1176. |
[41] | Kazama H, Katsumi M (1973) Auxin-gibberellin relationships in their effects on hypocotyl elongation of light-grown cucumber seedlings. Responses of sections to auxin, gibberellin and sucrose. Plant Cell Physiol 14: 449-458. |
[42] | Ross JJ, O'neill DP, Wolbang CM, et al. (2001) Auxin-gibberellin interactions and their role in plant growth. J Plant Growth Regul 20: 336-353. |
[43] |
Ross JJ, O'Neill DP, Rathbone DA (2003) Auxin-gibberellin interactions in pea: integrating the old with the new. J Plant Growth Regul 22: 99-108. doi: 10.1007/s00344-003-0021-z
![]() |
[44] |
DeMason DA (2005) Auxin-cytokinin and auxin-gibberellin interactions during morphogenesis of the compound leaves of pea (Pisum sativum). Planta 222: 151-166. doi: 10.1007/s00425-005-1508-6
![]() |
[45] | Gbadamosi IT, Sulaiman MO (2012) The Influence of Growth Hormones and Cocos nucifera Water on the In Vitro Propagation of Irvingia gabonensis (Aubry-Lecomte ex O'Rorke) Baill. Nat Sci 10: 53-58. |
[46] |
de Souza RAV, Braga FT, Setotaw TA, et al. (2013) Effect of coconut water on growth of olive embryos cultured in vitro. Ciênc Rural 43: 290-296. doi: 10.1590/S0103-84782013000200016
![]() |
[47] |
Muhammad K, Gul Z, Jamal Z, et al. (2015) Effect of coconut water from different fruit maturity stages, as natural substitute for synthetic PGR in in vitro potato micropropagation. Int J Biosci IJB 6: 84-92. doi: 10.12692/ijb/6.2.84-92
![]() |
[48] |
Demason DA, Chawla R (2006) Auxin/gibberellin interactions in pea leaf morphogenesis. Bot J Linn Soc 150: 45-59. doi: 10.1111/j.1095-8339.2006.00491.x
![]() |
[49] |
Ferreira PMP, Farias DF, Oliveira JT de A, et al. (2008) Moringa oleifera: bioactive compounds and nutritional potential. Rev Nutr 21: 431-437. doi: 10.1590/S1415-52732008000400007
![]() |
[50] |
Moyo B, Masika PJ, Hugo A, et al. (2011) Nutritional characterization of Moringa (Moringa oleifera Lam.) leaves. Afr J Biotechnol 10: 12925-12933. doi: 10.5897/AJB10.1599
![]() |
[51] | Madukwe EU, Ezeugwu JO, Eme PE (2013) Nutrient composition and sensory evaluation of dry Moringa oleifera aqueous extract. Corpus ID: 10868940. |
1. | Siddhartha Kundu, ReDirection: an R-package to compute the probable dissociation constant for every reaction of a user-defined biochemical network, 2023, 10, 2296-889X, 10.3389/fmolb.2023.1206502 | |
2. | Siddhartha Kundu, A mathematically rigorous algorithm to define, compute and assess relevance of the probable dissociation constants in characterizing a biochemical network, 2024, 14, 2045-2322, 10.1038/s41598-024-53231-9 |
Enzyme | EC | CV | SNP | M | Co | US | B | LB | P | LP | FN | TP | R (%) | |
1 | Glucokinase | 2.7.1.2 | 778 | 619 | 370 | 49 | 157 | 4 | 4 | 65 | 113 | 206 | 186 | 47.45 |
2 | Pyruvate kinase | 2.7.1.40 | 1239 | 629 | 209 | 14 | 135 | 7 | 7 | 30 | 20 | 149 | 64 | 30.05 |
3 | Cathepsin A | 3.4.16.x | 1776 | 1270 | 501 | 20 | 382 | 26 | 23 | 26 | 34 | 402 | 109 | 21.33 |
4 | Pyruvate dehydrogenase | 1.2.4.1 | 2317 | 1591 | 584 | 25 | 391 | 35 | 46 | 62 | 43 | 416 | 186 | 30.9 |
5 | Phosphofructokinase 1 | 2.7.1.11 | 165 | 32 | 10 | 2 | 4 | 2 | 1 | 1 | 1 | 6 | 5 | 45.45 |
6 | Phosphofructokinase 2 | 2.7.1.105 | 206 | 76 | 23 | 1 | 17 | 1 | 1 | 2 | 1 | 18 | 5 | 21.74 |
7 | Cystathione beta-synthase | 4.2.1.22 | 930 | 744 | 255 | 21 | 172 | 3 | 4 | 46 | 32 | 193 | 85 | 30.58 |
8 | DNA topoisomerase Ⅱ | 5.6.2.2 | 466 | 319 | 152 | 0 | 122 | 11 | 14 | 3 | 1 | 122 | 29 | 19.21 |
9 | Guanylate cyclase 1 | 4.6.1.2 | 1572 | 1117 | 576 | 29 | 417 | 11 | 10 | 34 | 59 | 446 | 114 | 20.36 |
10 | Phenylalanine hydroxylase | 1.14.16.1 | 1314 | 1102 | 631 | 5 | 182 | 0 | 3 | 161 | 254 | 187 | 418 | 69.09 |
Note: EC: Enzyme commission number; CV: Number of clinical variants; SNP: Single nucleotide polymorphisms; M: Missense mutations; Co: Conflicting data; US: Uncertain significance; B: Benign; LB: Likely; P: Pathogenic; LP: Likely pathogenic; FN: False negative (Co + US); TP: True positive (B + LB + P + LP); R: Recall ( \frac{TP}{TP+FN}\times 100 ). |
Case 1 | Case 2 | |
Ligand \left(\boldsymbol{\mu }\in {\boldsymbol{\mathcal{L}}}\right) | Hypoxia-inducible factor | Peptide |
Macromolecule \left(\boldsymbol{c}\equiv {\boldsymbol{\mathcal{C}}}_{\boldsymbol{z}}\in \boldsymbol{\mathcal{C}}\right) | HP4H, CP4H | M1\beta |
Primary complex \left\langle {} \right.\boldsymbol{c}|\boldsymbol{\mu }\left. {} \right\rangle High-affinity variant :=\boldsymbol{K}\boldsymbol{d}\left({\boldsymbol{\mu }}_{\boldsymbol{h}\boldsymbol{i}\boldsymbol{g}\boldsymbol{h}}{\boldsymbol{\mathcal{C}}}_{\boldsymbol{z}}\right) Low-affinity variant :=\boldsymbol{K}\boldsymbol{d}\left({\boldsymbol{\mu }}_{\boldsymbol{h}\boldsymbol{i}\boldsymbol{g}\boldsymbol{h}}{\boldsymbol{\mathcal{C}}}_{\boldsymbol{z}}\right) |
\left\langle{\boldsymbol{H}\boldsymbol{P}{\bf{4}}\boldsymbol{H}|\boldsymbol{\mu }}\right\rangle , \left\langle{\boldsymbol{C}\boldsymbol{P}{\bf{4}}\boldsymbol{H}|\boldsymbol{\mu }}\right\rangle \boldsymbol{K}\boldsymbol{d}\left(\boldsymbol{H}\boldsymbol{P}{\bf{4}}\boldsymbol{H}|{\boldsymbol{\mu }}_{\boldsymbol{h}\boldsymbol{i}\boldsymbol{g}\boldsymbol{h}}\right)=\boldsymbol{K}\boldsymbol{d}\left({\boldsymbol{\mu }}_{\boldsymbol{h}\boldsymbol{i}\boldsymbol{g}\boldsymbol{h}}\boldsymbol{H}\boldsymbol{P}{\bf{4}}\boldsymbol{H}\right) \boldsymbol{K}\boldsymbol{d}\left(\boldsymbol{C}\boldsymbol{P}{\bf{4}}\boldsymbol{H}|{\boldsymbol{\mu }}_{\boldsymbol{l}\boldsymbol{o}\boldsymbol{w}}\right)=\boldsymbol{K}\boldsymbol{d}\left({\boldsymbol{\mu }}_{\boldsymbol{l}\boldsymbol{o}\boldsymbol{w}}\boldsymbol{C}\boldsymbol{P}{\bf{4}}\boldsymbol{H}\right) |
\left\langle{\boldsymbol{M}{\bf{1}}\boldsymbol{\beta }|\boldsymbol{\mu }}\right\rangle \boldsymbol{K}\boldsymbol{d}\left(\boldsymbol{M}{\bf{1}}\boldsymbol{\beta }|{\boldsymbol{\mu }}_{\boldsymbol{h}\boldsymbol{i}\boldsymbol{g}\boldsymbol{h}}\right)=\boldsymbol{K}\boldsymbol{d}\left({\boldsymbol{\mu }}_{\boldsymbol{h}\boldsymbol{i}\boldsymbol{g}\boldsymbol{h}}\boldsymbol{M}{\bf{1}}\boldsymbol{\beta }\right) \boldsymbol{K}\boldsymbol{d}\left(\boldsymbol{M}{\bf{1}}\boldsymbol{\beta }|{\boldsymbol{\mu }}_{\boldsymbol{l}\boldsymbol{o}\boldsymbol{w}}\right)=\boldsymbol{K}\boldsymbol{d}\left({\boldsymbol{\mu }}_{\boldsymbol{l}\boldsymbol{o}\boldsymbol{w}}\boldsymbol{M}{\bf{1}}\boldsymbol{\beta }\right) |
Higher-order complex \left\langle {} \right.\boldsymbol{c}|\boldsymbol{\mu }\left. {} \right\rangle .{{\mathcal{C}}}^{y} High-affinity variant :=\boldsymbol{K}\boldsymbol{d}\left({\boldsymbol{\mu }}_{\boldsymbol{h}\boldsymbol{i}\boldsymbol{g}\boldsymbol{h}}{\boldsymbol{\mathcal{C}}}_{\boldsymbol{z}}.{{\mathcal{C}}}^{y}\right) Low-affinity variant :=\boldsymbol{K}\boldsymbol{d}\left({\boldsymbol{\mu }}_{\boldsymbol{l}\boldsymbol{o}\boldsymbol{w}}{\boldsymbol{\mathcal{C}}}_{\boldsymbol{z}}.{{\mathcal{C}}}^{y}\right) |
--- --- --- |
\left\langle{\boldsymbol{M}{\bf{1}}\boldsymbol{\beta }|\boldsymbol{\mu }}\right\rangle.PLC \boldsymbol{K}\boldsymbol{d}\left(\boldsymbol{M}{\bf{1}}\boldsymbol{\beta }|{\boldsymbol{\mu }}_{\boldsymbol{h}\boldsymbol{i}\boldsymbol{g}\boldsymbol{h}}.\boldsymbol{P}\boldsymbol{L}\boldsymbol{C}\right)=\boldsymbol{K}\boldsymbol{d}\left({\boldsymbol{\mu }}_{\boldsymbol{h}\boldsymbol{i}\boldsymbol{g}\boldsymbol{h}}\boldsymbol{M}{\bf{1}}\boldsymbol{\beta }.\boldsymbol{P}\boldsymbol{L}\boldsymbol{C}\right) \boldsymbol{K}\boldsymbol{d}\left(\boldsymbol{M}{\bf{1}}\boldsymbol{\beta }|{\boldsymbol{\mu }}_{\boldsymbol{l}\boldsymbol{o}\boldsymbol{w}}.\boldsymbol{P}\boldsymbol{L}\boldsymbol{C}\right)=\boldsymbol{K}\boldsymbol{d}\left({\boldsymbol{\mu }}_{\boldsymbol{l}\boldsymbol{o}\boldsymbol{w}}\boldsymbol{M}{\bf{1}}\boldsymbol{\beta }.\boldsymbol{P}\boldsymbol{L}\boldsymbol{C}\right) |
Functional relevance | {R}_{HP4H}\left(t\right)\gg {R}_{CP4H}\left(t\right) | M1\beta |{\mu }_{high}.PLC\to \alpha M1\beta (Anterograde) {M1\beta |\mu }_{low}.PLC\to rM1\beta (Retrograde) |
Note: {\boldsymbol{\mathcal{C}}}_{\boldsymbol{z}\boldsymbol{\mu }} : All pairwise-atom/residue based square interaction matrix of ligand and macromolecule, \left\langle {} \right.\boldsymbol{c}|\boldsymbol{\mu }\left. {} \right\rangle ; \mathit{\boldsymbol{\mathcal{K}}\boldsymbol{\mathcal{C}}}_{\boldsymbol{z}\boldsymbol{\mu }} : Diagonal matrix of the interactions of a ligand and macromolecule; {z}_{i=j}=z : Set of eigenvalues of \mathit{\boldsymbol{\mathcal{K}}\boldsymbol{\mathcal{C}}}_{\boldsymbol{z}\boldsymbol{\mu }} where z\in diag\left(\mathit{\boldsymbol{\mathcal{K}}\boldsymbol{\mathcal{C}}}_{\boldsymbol{z}\boldsymbol{\mu }}\right)\subset \mathbb{C} ; \boldsymbol{K}\boldsymbol{d}\left(\boldsymbol{c}\boldsymbol{\mu }\right) : Set of eigenvalue-based transition-state dissociation constants for monomer- and multimer-forms of ligand-macromolecular complexes, \left\{\omega \in \boldsymbol{K}\boldsymbol{d}\left(\boldsymbol{c}\boldsymbol{\mu }\right)={\alpha }_{i=j}=Re\left(z\right)\in \boldsymbol{K}\boldsymbol{d}\left({\boldsymbol{\mathcal{C}}}_{\boldsymbol{z}\boldsymbol{\mu }}\right)\cap \left(\mathrm{0, 1}\right)\right\} ; {\mu }_{high}, {\mu }_{low} : High- and low-affinity variants of an arbitrary ligand, \mu =\{{\mu }_{high}, {\mu }_{low}\}\in {\boldsymbol{\mathcal{L}}} ; \boldsymbol{\mu }{\boldsymbol{\mathcal{C}}}_{\boldsymbol{z}}.{{\mathcal{C}}}^{y} : Higher-order complex of ligand and macromolecule; \boldsymbol{K}\boldsymbol{i}\boldsymbol{n}\boldsymbol{f} : Subset of transition-state dissociation constants of ligand-macromolecular interaction; \boldsymbol{K}\boldsymbol{s}\boldsymbol{u}\boldsymbol{p} :Subset of transition-state dissociation constants of ligand-macromolecular interaction; R\left(t\right) : Rate of reaction; HP4H: Hypoxia stimulated- and Collagen Proline 4-Hydroxylase; CP4H: M1\beta : Heterodimer of major histocompatibility complex I (MHC1) with beta-2 microglobulin; PLC: Peptide loading complex. |
Enzyme | EC | CV | SNP | M | Co | US | B | LB | P | LP | FN | TP | R (%) | |
1 | Glucokinase | 2.7.1.2 | 778 | 619 | 370 | 49 | 157 | 4 | 4 | 65 | 113 | 206 | 186 | 47.45 |
2 | Pyruvate kinase | 2.7.1.40 | 1239 | 629 | 209 | 14 | 135 | 7 | 7 | 30 | 20 | 149 | 64 | 30.05 |
3 | Cathepsin A | 3.4.16.x | 1776 | 1270 | 501 | 20 | 382 | 26 | 23 | 26 | 34 | 402 | 109 | 21.33 |
4 | Pyruvate dehydrogenase | 1.2.4.1 | 2317 | 1591 | 584 | 25 | 391 | 35 | 46 | 62 | 43 | 416 | 186 | 30.9 |
5 | Phosphofructokinase 1 | 2.7.1.11 | 165 | 32 | 10 | 2 | 4 | 2 | 1 | 1 | 1 | 6 | 5 | 45.45 |
6 | Phosphofructokinase 2 | 2.7.1.105 | 206 | 76 | 23 | 1 | 17 | 1 | 1 | 2 | 1 | 18 | 5 | 21.74 |
7 | Cystathione beta-synthase | 4.2.1.22 | 930 | 744 | 255 | 21 | 172 | 3 | 4 | 46 | 32 | 193 | 85 | 30.58 |
8 | DNA topoisomerase Ⅱ | 5.6.2.2 | 466 | 319 | 152 | 0 | 122 | 11 | 14 | 3 | 1 | 122 | 29 | 19.21 |
9 | Guanylate cyclase 1 | 4.6.1.2 | 1572 | 1117 | 576 | 29 | 417 | 11 | 10 | 34 | 59 | 446 | 114 | 20.36 |
10 | Phenylalanine hydroxylase | 1.14.16.1 | 1314 | 1102 | 631 | 5 | 182 | 0 | 3 | 161 | 254 | 187 | 418 | 69.09 |
Note: EC: Enzyme commission number; CV: Number of clinical variants; SNP: Single nucleotide polymorphisms; M: Missense mutations; Co: Conflicting data; US: Uncertain significance; B: Benign; LB: Likely; P: Pathogenic; LP: Likely pathogenic; FN: False negative (Co + US); TP: True positive (B + LB + P + LP); R: Recall ( \frac{TP}{TP+FN}\times 100 ). |
Case 1 | Case 2 | |
Ligand \left(\boldsymbol{\mu }\in {\boldsymbol{\mathcal{L}}}\right) | Hypoxia-inducible factor | Peptide |
Macromolecule \left(\boldsymbol{c}\equiv {\boldsymbol{\mathcal{C}}}_{\boldsymbol{z}}\in \boldsymbol{\mathcal{C}}\right) | HP4H, CP4H | M1\beta |
Primary complex \left\langle {} \right.\boldsymbol{c}|\boldsymbol{\mu }\left. {} \right\rangle High-affinity variant :=\boldsymbol{K}\boldsymbol{d}\left({\boldsymbol{\mu }}_{\boldsymbol{h}\boldsymbol{i}\boldsymbol{g}\boldsymbol{h}}{\boldsymbol{\mathcal{C}}}_{\boldsymbol{z}}\right) Low-affinity variant :=\boldsymbol{K}\boldsymbol{d}\left({\boldsymbol{\mu }}_{\boldsymbol{h}\boldsymbol{i}\boldsymbol{g}\boldsymbol{h}}{\boldsymbol{\mathcal{C}}}_{\boldsymbol{z}}\right) |
\left\langle{\boldsymbol{H}\boldsymbol{P}{\bf{4}}\boldsymbol{H}|\boldsymbol{\mu }}\right\rangle , \left\langle{\boldsymbol{C}\boldsymbol{P}{\bf{4}}\boldsymbol{H}|\boldsymbol{\mu }}\right\rangle \boldsymbol{K}\boldsymbol{d}\left(\boldsymbol{H}\boldsymbol{P}{\bf{4}}\boldsymbol{H}|{\boldsymbol{\mu }}_{\boldsymbol{h}\boldsymbol{i}\boldsymbol{g}\boldsymbol{h}}\right)=\boldsymbol{K}\boldsymbol{d}\left({\boldsymbol{\mu }}_{\boldsymbol{h}\boldsymbol{i}\boldsymbol{g}\boldsymbol{h}}\boldsymbol{H}\boldsymbol{P}{\bf{4}}\boldsymbol{H}\right) \boldsymbol{K}\boldsymbol{d}\left(\boldsymbol{C}\boldsymbol{P}{\bf{4}}\boldsymbol{H}|{\boldsymbol{\mu }}_{\boldsymbol{l}\boldsymbol{o}\boldsymbol{w}}\right)=\boldsymbol{K}\boldsymbol{d}\left({\boldsymbol{\mu }}_{\boldsymbol{l}\boldsymbol{o}\boldsymbol{w}}\boldsymbol{C}\boldsymbol{P}{\bf{4}}\boldsymbol{H}\right) |
\left\langle{\boldsymbol{M}{\bf{1}}\boldsymbol{\beta }|\boldsymbol{\mu }}\right\rangle \boldsymbol{K}\boldsymbol{d}\left(\boldsymbol{M}{\bf{1}}\boldsymbol{\beta }|{\boldsymbol{\mu }}_{\boldsymbol{h}\boldsymbol{i}\boldsymbol{g}\boldsymbol{h}}\right)=\boldsymbol{K}\boldsymbol{d}\left({\boldsymbol{\mu }}_{\boldsymbol{h}\boldsymbol{i}\boldsymbol{g}\boldsymbol{h}}\boldsymbol{M}{\bf{1}}\boldsymbol{\beta }\right) \boldsymbol{K}\boldsymbol{d}\left(\boldsymbol{M}{\bf{1}}\boldsymbol{\beta }|{\boldsymbol{\mu }}_{\boldsymbol{l}\boldsymbol{o}\boldsymbol{w}}\right)=\boldsymbol{K}\boldsymbol{d}\left({\boldsymbol{\mu }}_{\boldsymbol{l}\boldsymbol{o}\boldsymbol{w}}\boldsymbol{M}{\bf{1}}\boldsymbol{\beta }\right) |
Higher-order complex \left\langle {} \right.\boldsymbol{c}|\boldsymbol{\mu }\left. {} \right\rangle .{{\mathcal{C}}}^{y} High-affinity variant :=\boldsymbol{K}\boldsymbol{d}\left({\boldsymbol{\mu }}_{\boldsymbol{h}\boldsymbol{i}\boldsymbol{g}\boldsymbol{h}}{\boldsymbol{\mathcal{C}}}_{\boldsymbol{z}}.{{\mathcal{C}}}^{y}\right) Low-affinity variant :=\boldsymbol{K}\boldsymbol{d}\left({\boldsymbol{\mu }}_{\boldsymbol{l}\boldsymbol{o}\boldsymbol{w}}{\boldsymbol{\mathcal{C}}}_{\boldsymbol{z}}.{{\mathcal{C}}}^{y}\right) |
--- --- --- |
\left\langle{\boldsymbol{M}{\bf{1}}\boldsymbol{\beta }|\boldsymbol{\mu }}\right\rangle.PLC \boldsymbol{K}\boldsymbol{d}\left(\boldsymbol{M}{\bf{1}}\boldsymbol{\beta }|{\boldsymbol{\mu }}_{\boldsymbol{h}\boldsymbol{i}\boldsymbol{g}\boldsymbol{h}}.\boldsymbol{P}\boldsymbol{L}\boldsymbol{C}\right)=\boldsymbol{K}\boldsymbol{d}\left({\boldsymbol{\mu }}_{\boldsymbol{h}\boldsymbol{i}\boldsymbol{g}\boldsymbol{h}}\boldsymbol{M}{\bf{1}}\boldsymbol{\beta }.\boldsymbol{P}\boldsymbol{L}\boldsymbol{C}\right) \boldsymbol{K}\boldsymbol{d}\left(\boldsymbol{M}{\bf{1}}\boldsymbol{\beta }|{\boldsymbol{\mu }}_{\boldsymbol{l}\boldsymbol{o}\boldsymbol{w}}.\boldsymbol{P}\boldsymbol{L}\boldsymbol{C}\right)=\boldsymbol{K}\boldsymbol{d}\left({\boldsymbol{\mu }}_{\boldsymbol{l}\boldsymbol{o}\boldsymbol{w}}\boldsymbol{M}{\bf{1}}\boldsymbol{\beta }.\boldsymbol{P}\boldsymbol{L}\boldsymbol{C}\right) |
Functional relevance | {R}_{HP4H}\left(t\right)\gg {R}_{CP4H}\left(t\right) | M1\beta |{\mu }_{high}.PLC\to \alpha M1\beta (Anterograde) {M1\beta |\mu }_{low}.PLC\to rM1\beta (Retrograde) |
Note: {\boldsymbol{\mathcal{C}}}_{\boldsymbol{z}\boldsymbol{\mu }} : All pairwise-atom/residue based square interaction matrix of ligand and macromolecule, \left\langle {} \right.\boldsymbol{c}|\boldsymbol{\mu }\left. {} \right\rangle ; \mathit{\boldsymbol{\mathcal{K}}\boldsymbol{\mathcal{C}}}_{\boldsymbol{z}\boldsymbol{\mu }} : Diagonal matrix of the interactions of a ligand and macromolecule; {z}_{i=j}=z : Set of eigenvalues of \mathit{\boldsymbol{\mathcal{K}}\boldsymbol{\mathcal{C}}}_{\boldsymbol{z}\boldsymbol{\mu }} where z\in diag\left(\mathit{\boldsymbol{\mathcal{K}}\boldsymbol{\mathcal{C}}}_{\boldsymbol{z}\boldsymbol{\mu }}\right)\subset \mathbb{C} ; \boldsymbol{K}\boldsymbol{d}\left(\boldsymbol{c}\boldsymbol{\mu }\right) : Set of eigenvalue-based transition-state dissociation constants for monomer- and multimer-forms of ligand-macromolecular complexes, \left\{\omega \in \boldsymbol{K}\boldsymbol{d}\left(\boldsymbol{c}\boldsymbol{\mu }\right)={\alpha }_{i=j}=Re\left(z\right)\in \boldsymbol{K}\boldsymbol{d}\left({\boldsymbol{\mathcal{C}}}_{\boldsymbol{z}\boldsymbol{\mu }}\right)\cap \left(\mathrm{0, 1}\right)\right\} ; {\mu }_{high}, {\mu }_{low} : High- and low-affinity variants of an arbitrary ligand, \mu =\{{\mu }_{high}, {\mu }_{low}\}\in {\boldsymbol{\mathcal{L}}} ; \boldsymbol{\mu }{\boldsymbol{\mathcal{C}}}_{\boldsymbol{z}}.{{\mathcal{C}}}^{y} : Higher-order complex of ligand and macromolecule; \boldsymbol{K}\boldsymbol{i}\boldsymbol{n}\boldsymbol{f} : Subset of transition-state dissociation constants of ligand-macromolecular interaction; \boldsymbol{K}\boldsymbol{s}\boldsymbol{u}\boldsymbol{p} :Subset of transition-state dissociation constants of ligand-macromolecular interaction; R\left(t\right) : Rate of reaction; HP4H: Hypoxia stimulated- and Collagen Proline 4-Hydroxylase; CP4H: M1\beta : Heterodimer of major histocompatibility complex I (MHC1) with beta-2 microglobulin; PLC: Peptide loading complex. |