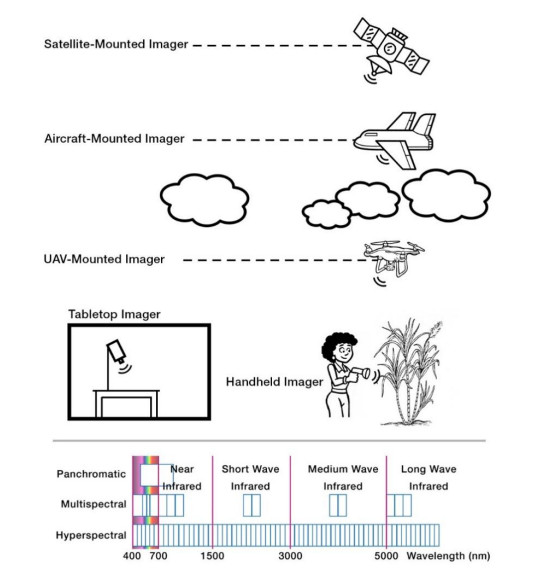
Citation: Fernando Arias, Maytee Zambrano, Kathia Broce, Carlos Medina, Hazel Pacheco, Yerenis Nunez. Hyperspectral imaging for rice cultivation: Applications, methods and challenges[J]. AIMS Agriculture and Food, 2021, 6(1): 273-307. doi: 10.3934/agrfood.2021018
[1] | Raulston Derrick Gillette, Norio Sakai, Godfrid Erasme Ibikoule . Role and impact of contract farming under various pricing standards: A case of Guyana's rice sector. AIMS Agriculture and Food, 2024, 9(1): 336-355. doi: 10.3934/agrfood.2024020 |
[2] | Heni Purwaningsih, Kristamtini, Pamudji Rahardjo, Bangun Prajanto Nusantoro, Erni Apriyati, Siti Dewi Indrasari, Rubiyo . The effect of different storage times and methods on the chemical and organoleptic properties of white cooked rice and black cooked rice. AIMS Agriculture and Food, 2022, 7(1): 184-196. doi: 10.3934/agrfood.2022012 |
[3] | Santi Noviasari, Feri Kusnandar, Agus Setiyono, Slamet Budijanto . Antioxidant activity and inhibition of α-amylase and α-glucosidase in fermented black rice bran-based analog rice. AIMS Agriculture and Food, 2022, 7(1): 61-72. doi: 10.3934/agrfood.2022004 |
[4] | Radhwane Derraz, Farrah Melissa Muharam, Noraini Ahmad Jaafar . Uncertainty sources affecting operational efficiency of ML algorithms in UAV-based precision agriculture: A 2013–2020 systematic review. AIMS Agriculture and Food, 2023, 8(2): 687-719. doi: 10.3934/agrfood.2023038 |
[5] | Ardiansyah, Fauziyyah Ariffa, Rizki Maryam Astuti, Wahyudi David, Dody Dwi Handoko, Slamet Budijanto, Hitoshi Shirakawa . Non-volatile compounds and blood pressure-lowering activity of Inpari 30 and Cempo Ireng fermented and non-fermented rice bran. AIMS Agriculture and Food, 2021, 6(1): 337-359. doi: 10.3934/agrfood.2021021 |
[6] | Thornthan Sawangwan, Chompoonuth Porncharoennop, Harit Nimraksa . Antioxidant compounds from rice bran fermentation by lactic acid bacteria. AIMS Agriculture and Food, 2021, 6(2): 578-587. doi: 10.3934/agrfood.2021034 |
[7] | Rasyid Sukifto, Rosimah Nulit, Yap Chee Kong, Noorhazira Sidek, Siti Nuratiqah Mahadi, Nurfatiha Mustafa, Roslinda A. Razak . Enhancing germination and early seedling growth of Malaysian indica rice (Oryza sativa L.) using hormonal priming with gibberellic acid (GA3). AIMS Agriculture and Food, 2020, 5(4): 649-665. doi: 10.3934/agrfood.2020.4.649 |
[8] | Eric Tzyy Jiann Chong, Lucky Poh Wah Goh, Mariam Abd. Latip, Zaleha Abdul Aziz, Noumie Surugau, Ping-Chin Lee . Genetic diversity of upland traditional rice varieties in Malaysian Borneo based on mitochondrial cytochrome c oxidase 3 gene analysis. AIMS Agriculture and Food, 2021, 6(1): 235-246. doi: 10.3934/agrfood.2021015 |
[9] | Syajariah Sanusi, Huck Ywih Ch’ng, Suhaimi Othman . Effects of incubation period and Christmas Island rock phosphate with different rate of rice straw compost on phosphorus availability in acid soil. AIMS Agriculture and Food, 2018, 3(4): 384-396. doi: 10.3934/agrfood.2018.4.384 |
[10] | Ki A. Sarwono, Rohmatussolihat Rohmatussolihat, Muh Watman, Shanti Ratnakomala, Wulansih D. Astuti, Rusli Fidriyanto, Roni Ridwan, Yantyati Widyastuti . Characteristics of fresh rice straw silage quality prepared with addition of lactic acid bacteria and crude cellulase. AIMS Agriculture and Food, 2022, 7(3): 481-499. doi: 10.3934/agrfood.2022030 |
Rice (Oriza sativa L.) is the main food staple for more than half of the world's population, particularly those living in some of the most populous countries, such as China, India and Japan [1,2,3]. Humans have a long history of cultivating rice crops; Rice was first domesticated approximately 9000 years ago by people living in the region of the Yangtze River valley in China [4]. For the Food and Agriculture Organization (FAO) of the United Nations (UN), world rice production forecasts an overall increase of 10.3 million tons per year to a new maximum of 769.9 million tons in 2018 [5], at normal growing conditions. This growth in production output driven by human consumption, which would decrease other uses, such as industrial and feed uses. Due population growth, global per capita food consumption showed an increase (0.4%) from 53.7 kg in 2017, to 53.9 kg in 2018. In terms of global rice consumption, by 2020 this amounted to a total of 379.035 million tons for the major consuming countries (China, India, Indonesia, Bangladesh, Vietnam, Philippines, Myanmar, Thailand, Japan and Brazil) [6]. Rice cultivation activity currently faces a series of challenges in order to meet current and projected future global consumption rates. Of most importance is the limited opportunity for expansion due to outright decline of available arable land [7,8], the increasingly limited availability of fresh water for irrigation due to climate change, increased industrial activity and urban freshwater usage [7,9,10,11]. Moreover, several additional factors have been identified as key contributors to declining rice crop yields such as the narrow genetic background of rice plants, inadequate soil drainage, which contributes to increased salinity and alkalinity over time, soil nutrient and organic matter deficiencies, and further soil quality decline from fertilizer and pesticide overuse [7,12]. Finally, the prevalent lack of strong research extension-farmer relationships, farmer organizations and public-private partnerships limits the capacity of producers to overcome these challenges [7].
Due to the urgent imminent requirement of supplying the growing world population with a sufficient volume of rice crop, it is important to know the development cycles of this plant, as well as the factors, both climatic and environmental, that limit its development and yield. In addition, it is important to study the influence of environmental factors, such as the presence of heavy metals or other contaminants, in the nutritional profile and potential toxicity of cultivated rice. It is also necessary to consider all the methodologies and technologies available for crop analysis such as the mechanisms of pollutants uptake and translocation in rice, phenology, canopy reflectance spectra, remote sensing, near-surface imaging spectroscopy and, of particular interest to this work, hyperspectral imaging [13].
The tangible and potential improvements introduced by Information and Communications Technologies (ICTs), particularly from the disruptive fields of machine learning and digital signal processing and including widely-deployed acquisition technologies such as the Internet of Things (IoT) [14,15], biosensors [16] and wireless sensor networks [17], have impacted such a wide variety of fields that the UN has stated their confidence that these technologies have changed the modern world, and possess the potential to continue to do so in a manner that makes them key to fulfilling their Sustainable Development Goals [18]. These technologies have introduced tangible benefits in various precision agriculture applications for rice cultivation applications [19,20,21,22]. Additionally, these technologies have enabled improvements in automated crop monitoring [23], efficient production input distribution [24], and crop quality evaluation tasks [25]. Several of these applications have been academically proposed but have faced difficulties in reaching sufficiently large-scale deployments as to shift the established agricultural production paradigms. Precision agriculture implied the use of sensors and advanced imaging technique to obtain data to monitoring the health status of the crops [26]. Hyperspectral imaging systems have gained notable relevance due to the advanced development of remote sensing systems with higher spectral and spatial resolution and have been used as a tool in precision agriculture in studies of morphological and physical stages of different crops however does not have been fully exploited. This spectral sensing technique allows the study of crop biophysical and biochemical properties, soil characteristics and crops classification as well to monitoring large fields of cultivation. Due to the advances in optoelectronics and data acquisitions systems HSI is now more accessible and allows the access to low cost and small size hyperspectral sensors. [27]. Additionally, a plural development of algorithms to work with high amount of data to extract information from the spectral signature of the materials with advanced techniques such as linear regression, nonlinear regression, and combined methods that incorporate machine learning techniques such as Support Vector Machines, neural networks and others [26,28,29,30]. Recent studies use the spectral measurements of various crops to infer important magnitudes such as nitrogen (N) content [31], landcover classification of cultivated areas [32] and individual plants [33], detection of plant diseases [34], detection of pest presence [35] and damage to plants [36] and various others, which creates opportunities for improved yield and process efficiency in rice cultivation through their use in smart remote sensing applications.
The main contribution of this work is to provide the community with an up-to-date survey of the most relevant works regarding the use of hyperspectral imaging sensing technology and hyperspectral image processing algorithms for the estimation and monitoring of various aspects of the rice cultivation process without the requirement of a field-wide deployment of individual sensors. Additionally, we provide a comparison of the signal processing methodologies used in the most relevant studies and present the main challenges for application deployments in the field. The methodologies proposed in these studies can potentially contribute to the cost reduction of measurement systems in precision agriculture operations, and alleviate the human labor requirements for the processes of assessing the health and expected productivity of rice plants in all its stages, from seeding to harvest on the field. Advances made towards alleviating these challenges are of special interest to all involved in the development and deployment of innovative precision agriculture applications. Particularly, funding agencies for rice producers such as banks, insurance companies, private investors, and other entities who would benefit from more accurate return of investment (ROI) estimates. With more informed yield estimates, more accurate risk assessment studies can be conducted by these agencies and more producers can potentially benefit from increased availability of funding sources for their activities. In this manner, it is our hope that this work serves as a starting point for future research efforts.
Rice production, like other types of crops, have been adapting farming methods to deal with problems connected to climate change, the growing demand for food, and the limitation of the amount of land for cultivation. The key challenges for rice cultivation are: sea water intrusion which is a problem of salinity in landscape areas (particularly sea level rise zones in Asia), deforestation, variability in precipitations patterns and water scarcity that requires a dynamic between availability and management of this resource [37]. Those problems require the use of new rice species that are resistant as well as new technologies to support monitoring, predictive analysis and water saving systems which are commonly linked to precision agriculture. Precision agriculture is a set of signal processing techniques and specialized machinery, sensors, actuators and various information systems in order to optimize crop production in agricultural systems [38]. Thus, the main object of study of this field is to further increase the effectiveness of agricultural activity by incorporating additional variables of interest into the decision-making systems from sensor technology developments, improving decision making processes through various algorithms, and new innovations in actuator technology. In this manner, the potential yields of the agricultural process are maximized by continuously ensuring the optimal conditions for adequate plant growth and development. In most ecosystems around the world, Photosynthesis is one of the most important processes for capturing energy from sunlight. The balance of the photosynthetic and respiration processes in plants is crucial for understanding their growth and productivity expectations, as well as the overall carbon cycle of ecosystems [39,40].
Chlorophyll is an important indicator of the photosynthetic capacity and vegetation stress in plants, changing its concentration in response to environmental conditions and solar radiation [41,42]. In previous studies, plant chlorophyll content has been identified to describe a close relationship to the gross food and biomass production capabilities of plants [43,44]. Further, there exists an established correlation between the measured N levels present in plant leaves and its expected productivity levels [45]. Thus, N is considered as a limiting element for plant growth since is the key nutrient parameter determining the photosynthetic functioning and productivity in rice crops [46]. N deficiency in plants can lead to lower chlorophyll content, lower photosynthetic assimilation, less biomass production, and reduced grain yield. On the other hand, a high content of N can improve chlorophyll density, which results in higher levels of photosynthetic activity and, by extension, productivity levels [47]. However, increasing N levels through external production inputs, such as fertilizers, can cause a series of environmental pollution issues and even diminished yields when fertilization become excessive [48]. The excess reactive N, derived from over and/or improper application of N fertilizers, may produce detrimental effects on public health and ecosystems. Excess N deposition in atmosphere considered recognition as a potent contributor to global warming and stratospheric ozone depletion [49].
In addition, the study of the vegetation ecosystem carbon cycle attracts considerable interest to assess carbon sequestration capability [23,50]. In the face of rising global atmospheric CO2 levels that contribute to climate change, elevated carbon assimilation in crop plants has promising effects on both crop yields and sequestration capabilities to aid in lowering the overall carbon levels in the atmosphere [51].
A key factor to consider in precision agriculture is the amount of nutrients present in the soil. Nutrient availability in soils play an important role in agricultural productivity, food security and agroecological sustainable development [52,53]. Parameters such as soil moisture, organic carbon, organic matter, heavy metals and soil nutrient contents are common soil properties and should be consider in prediction models for reducing soil nutrient loss and improving soil fertilization management practices, based on more robust and more sensitive analytical techniques, to complement plant information. To this end, specific wavelength ranges in aerial hyperspectral images have been used to characterize the nutrient content and distribution over regional scales [53].
Due to the rapid development of the industry economy in adjacency to agricultural land, rice paddies have been subjected to heavy metal pollution in some areas [27,54,55]. Some metals as cadmium (Cd), arsenic (As), and lead (Pb) are of great concern. It is known, that in China, for example, nearly 20 million hectares of cultivated land is contaminated with metals, resulting in about 12 million tons of contaminated grains which translate to about 2.4 billion US dollars of economic loss per year. Heavy metal pollution is becoming a worldwide concern to both agriculture and human health. One of the most contaminant elements is Cd, characterized as a toxic heavy metal, because either can contaminate the soil with a high mobility in living organisms, or have toxic effects on rice expose to this metal. As of constants applications of N fertilizers in agriculture, many soils become more acidic allowing Cd being easily absorbed by plants [13]. Cd is transferred from soil to rice and accumulates in rice plants and grains and then is enriched in the human body thought the food chain, thereby threatening human health. The high mobility of Cd causes effect on rice plants and crops exposed to this metal by interfering with the physiological activities of plants, such as photosynthesis, gaseous exchange, and nutrient absorption, to cause reduction in plant growth and dry matter accumulation [56]. In addition to crop health, heavy metal pollution has been demonstrated to negatively impact human health, and to cause effects such as anemia, cancer, heart failure, hypertension, cerebral infarction, proteinuria, severe lung damage, eye cataract formation, osteoporosis, emphysema, and renal insufficiency [13,57,58].
Shifting weather patterns as a result of imminent climate change is a growing concern for government agencies worldwide due to the potential shifting in water availability around the world [59,60]. Failure to adapt proper water management practices can have significant adverse effects on the food security of a given region. For the purposes of properly assessing and managing both the quantity and quality of available water resources, hyperspectral imaging applications have been devised in various regions [61,62,63,64]. On the subject of hyperspectral imaging applications for water management precision agriculture, there is a growing interest in monitoring and managing drought stress to maintain high production levels in intensive crop farming applications [65,66,67,68,69]. Through a combination of drought-tolerant cultivars and precise water resource availability monitoring, irrigation practices can be optimized in order to ensure the continued increase in yields and food security for communities in drought-prone areas.
Hyperspectral images provide valuable, spatially-resolved information for remote sensing applications in hundreds of individual wavelengths beyond the visible spectrum. With the increase of available spectral information about their targets, it is possible to perform accurate classification of objects and/or materials within a spatial scene of interest. This ability has demonstrated to be of use in fields such as studies of the atmosphere, vegetation, agriculture and coastal environments. Hyperspectral imaging has important applications in precision agriculture, where the health status of crops in different growth stages can be assessed from their spectral signatures.
Hyperspectral imaging sensors are spatial measurement devices that capture the spectral behavior of a scene of interest in the form of many simultaneous digital images, each representing a narrow spectral region across a continuous or discontinuous spectrum [70]. When a given material is exposed to a light source of a known spectral bandwidth, it emits, absorbs, and/or reflects specific portions of the electromagnetic spectrum according to its composition. This material-specific response is known as a material's spectral signature [70]. As illustrated in Figure 1, this information is stored in a cubic data structure, where individual spectral bands are "stacked" on top of each other, ordered by their individual wavelengths.
With this information, an observation of the measured spectral responses allows for the classification of different materials, or the observation of specific compositional qualities of interest in biological subjects. This technology has been applied to water resources and flood management, food quality assessment, medical diagnosis, military applications and various others [29,71,72,73]. Of particular importance to the current work, the advantages introduced by hyperspectral image analysis in precision agriculture are crucial for monitoring the effects of various factors on the spectral response of plant tissues such as fertilization [74], micronutrient content [75], pest damages [36], pollutant uptakes [76] or extreme conditions, such as droughts and flooding in localized regions [77,78]. From the measured variations in spectral responses, further processing of spectral data can determine the effects of these factors on the safety and productivity of crops. In order to provide images with a high spectral resolution, hyperspectral imaging devices' construction requires complex optomechanical components, which limits their deployability due to increased weight, volume and power requirements. As illustrated in Figure 2, these devices can be mounted on various platforms such as satellites [79], aircraft [80], Unmanned Aerial Vehicles (UAV) [81], or handheld devices which can be used in the field or in laboratory settings. Due to the fact that each platform provides different tradeoffs regarding spatial resolution, spectral resolution, measurement noise, coverage and/or deployment costs, the selection of the optimal imaging platform is application specific. Hyperspectral imaging enables researchers and plant breeders to analyze various traits of interest like nutritional values in high throughput [82]. For these authors, it is necessary to obtain an optimal design of a reliable calibration model, linking the measured spectra with the investigated traits. It is important to consider, the inclusion of samples from several time and locations to improve the predictions of the investigated nutrient trait at small calibration set sizes. However, some models obtained have some limitations because they work only in certain environments and are not transferable to others, for this reason they should be upgraded with new calibration data to enable a reliable response of desired traits.
Another important aspect to consider in agriculture in general, and specially in rice cultivation, for setting calibration of remote sensing hyperspectral imaging, is the spatial distribution of soil nutrients which depends mostly on field sampling and laboratory analysis, but in some cases, result inefficient and time consuming [53]. Nevertheless, applying variables to predict soil nutrients is a key means of clarifying their spatial variations; is in our opinion, to select carefully representative sampling field sites followed by the proper laboratory analysis of selected parameters e.g. N, phosphorus, and soil potassium contents of soil due to they are closely related to nutrient cycling for growth and fertilizer application in human activities, to support all the calibration models to get more accurate hyperspectral imaging data.
In the context of precision agriculture, the introduction of hyperspectral imaging presents some important advantages as a noninvasive observation and/or monitoring methodology that does not interfere with crops' growth process. In addition, a single aerial hyperspectral image provides a higher volume of detailed information about the developmental progress and chemical composition of both the plants and soil in a reduced labor-intensive manner compared to laboratory analysis of field samples. With the objective of estimating productivity levels, previous work on the spectral analysis of agriculture hyperspectral images has determined mathematical models that describe the relationships between the spectral behavior of rice, considering both individual plants and canopies and their chlorophyll, N and total carbon content [23,49,83]. Similar studies have been conducted for additional food crops such as wheat [84], corn [85,86], barley [82] and others [87,88,89,90,91].
In addition, hyperspectral images have been used to estimate various agriculturally relevant soil properties such as carbon content [92,93,94], heavy metal content [95,96], salinity [97], pH [98] and overall fertility [99]. Moreover, previous studies have demonstrated the possibility of hyperspectral image-based methodologies for the detection of fungal organisms and toxins in both crop plants and food products, which hinder the productivity of plants [100,101,102,103], contribute to additional levels of food waste [104,105], and have adverse effects on human health when consumed [106,107]. Finally, hyperspectral imaging has been employed for detecting the presence of heavy metal contaminants in the soil [106] and crop plants themselves [108].
In this section, we highlight and summarize the most relevant contributions of hyperspectral imaging rice cultivation applications in different stages of plant growth, for various quantities of interest. After reviewing 168 articles of the most relevant literature, the most significant studies in hyperspectral rice studies includes a total of 26 (49%) studies aimed at Chlorophyll and/or N estimation, 11 (19%) for various seed studies, 11 (19%) for biomass and vegetation quantity estimation and 10 (17%) for detection of pests, diseases and various pollutants. The distribution of studies is illustrated in Figure 4b.
Furthermore, the significant contributions in literature are organized, according to their objectives, in Tables 2 to 5. Table 2 lists the surveyed studies relative to biomass and vegetation estimation in rice crops or landcover classification of rice growing sites; Table 3 summarizes significant work concerning chlorophyll and N content estimation; studies of diseases, pests, heavy metals and other pollutants' effects on rice crops are summarized in Table 4; studies focused in various seed properties, lodging and others variables are presented in Table 5.
Sensor type | Commercial name | Studies |
Sensors that measure radiance or irradiance, as well as transmittance and reflectance. Usually portable devices | ASD QualitySpec Pro 350-2800 nm | [130,131] |
ASD FieldSpec Pro Spectrometer, 350-2500 nm | [36,132,133,134,135,136] | |
ASD FieldSpec 3 Spectroradiometer, 350-2500 nm | [75,96,137] | |
ASD Field Spec 2 | [11,138] | |
ASD FieldSpec-FR (350-2500 nm) | [48,139] | |
GER-2600 Spectroradiometer, 330-2600 nm | [103] | |
GER1500 Spectroradiometer, 300-1100 nm | [23] | |
HR-1024 Spectroradiometer, 350-2500 nm | [140] | |
Portable ground MSR16 Radiometer Cropscan, 450-1750 nm | [76] | |
Hyperspec HEADWALL | [10,11,141] | |
MS-720 Spectroradiometer, 350-1050 nm | [142] | |
LI-1800 Spectroradiometer, 380-1100 nm | [48,49] | |
Sensors based on a spectrograph + CCD system/optic | ImSpector V10E Spectrograph, 390-1050 nm | [35,90,119,136,143,144] |
ImSpector N17E Spectrograph, 874-1734 nm | [58,125,145] | |
SP2500i Spectrograph | [118,119] | |
Multispectral systems with incorporated filters | Tetra CAM: ADC Micro, ADC Lite system, MiniMCA | [11,74,146,147,148] |
Sensors with onboard DSP software for parameter estimation | SPAD-502 Chlorophyll Meter, Measure the leaf transmittance in two wave bands: 400-500 nm, 600-700 nm | [46,126] |
Dualex 4 Fluorescence Sensor | [46] |
Study | Region | Rice species | Inferred magnitude | Acquisition | Spectral region |
[146] | Colombia | Indica IR64, Line 23 | Above Ground Biomass | Unmanned Aerial Vehicle (UAV) | 520-690 nm, 760-900 nm |
[131] | Sanjiang Plain, China | Kongyu | Above Ground Biomass | Manual | 350-1800 nm |
[141] | China | 152 Cultivars | Above Ground Biomass | Manual | 400-1000 nm |
[132] | Jiangsu, China | Yliangyou 1, Wuyunjing 24 | Above Ground Biomass | Manual | 350-2500 nm |
[112] | South Korea/California | Not Specified (N/S) | Paddy Rice Landcover | Satellite | 430-1200 nm |
[114] | West Java, Indonesia | N/S | Paddy Rice Landcover | Satellite | 479-841 nm |
[32] | Hunan, China | N/S | Paddy Rice Landcover | Satellite | 430-1200 nm |
[33] | Rugao City, Juangsu, China | N/S | Plant Presence | UAV | 430-900 nm |
[149] | China | N/S | Plant Species | Manual | 415,561,687,705,735, 1007 nm |
[84] | Jiangsu, China | Wheat (Ningmai 13, Huaimai 33), Rice (Nanjing 9108, Lianjing 10) | Ratio Vegetation Index (RVI) | Manual | 730,815 nm |
[129] | Nanchang, China | N/S | Rice Paddy Presence | Satellite | 400-1200 nm |
Study | region | Rice species | Inferred magnitude | Acquisition | Spectral region |
[144] | China | Yongyou 4949 | Chlorophyll | N/S | 539-910 nm |
[137] | New Delhi, India | 13 Species | Chlorophyll | Manual | 690,700-750 nm, 940-1104 nm |
[153] | Japan | Haenuki and Koshihikari SD | Chlorophyll | UAV | 327-763 nm, 640-1050 nm |
[46] | Jiangsu, China | Oryza sativa L. | Chlorophyll | Automated Ground System | 360-1025 nm |
[31] | Jiangsu, China. | Ningjing-4, Wuyunjing-24 | LNC | Manual | 375,710,850 nm |
[120] | Hubei, China | Yangliangyou 6 | LNC | Manual | 355,460,556 nm |
[118] | Jianghan Plain, China | Yongyou 4949, Yangliangyou 6 | LNC | Manual | 360-800 nm |
[133] | Jiangsu, China | Yliangyou 1, Wuyunjing 24 | LNC | Manual | 350-2500 nm |
[154] | N/S | N/S | LNC | Manual | 450-900 nm |
[74] | Jiangsu, China | Wuyunjing24, Yliangyou1 | LNC/Plant Nitrogen Accumulation (PNA) | UAV | 490-900 nm |
[148] | Juangsu, China | N/S | N Content | UAV | 450-950 nm |
[151] | China | Yongyou4949, Yangliangyou 6 | N Content | Manual | 538-910 nm |
[117] | China | N/S | N Content | Manual | 450-740 nm |
[49] | Taiwan | Tainung 67 | N Content | Manual | 400-1100 nm |
[76] | Nanjing, China | Wuxiangjing 9, Nipponbare, Huajing 2 | N Content | Manual | 610,660,680 nm |
[119] | Hubei, China | Yongyou4949, Yangliangyou 6 | N Content | Manual | 360-800 nm |
[110] | Heilongjiang, China | Longjing 31, Longjing 21 | N Content | Manual | 670,730,780 nm |
[135] | Jiangsu, China | Y Liangyou 1, Wuyunjing24 | N Content | UAV | 490,550,680,720,800 nm |
[48] | China, Japan | Tsugaru Roman | N Content | Manual | 350-2500 nm |
[130] | Suizhou City, China | Japonica, non-glutinous Rice | N Content | Lab | 538-910 nm |
[140] | Southern NSW, Australia | Reiziq, Sherpa | N Uptake | Manual | 350-2500 nm |
[23] | Suwon, Korea | Hwasungbyeo, Dasanbyeo, Juanbyeo, SNU-SG1 | N, Chlorophyll | Manual | 520-600 nm |
[130] | China | Japonica and non-glutinous rice | N, Chlorophyll | Manual | 538-910 nm |
[75] | New Delhi, India | PRH-10 | N, Phosphorus, Sulphur concentration | Manual | 350-2500 nm |
[111] | Rosasco, Gaggiano | 43 Cultivars | PNA | Manual | 450-700 nm |
Study | Region | Rice species | Inferred magnitude | Acquisition | Spectral region |
[126] | Harbin, China | N/S | Disease Presence | Manual | 560,620,670 nm |
[103] | N/S | Nipponbare | Disease Presence | Manual | 900-1700 nm |
[139] | Wufeng, Taiwan | TNG 67, TCS 10 | Disease Presence | Manual | 350-2500 nm |
[155] | Anhui, Jiangxi and Hunan Province, China | N/S | Disease Presence | Manual | N/S |
[34] | Arkansas, USA | TIL 654.13 | Disease Presence | Manual | 480,760,800, 1000 nm |
[36] | Jiangsu, China | Sanyou 63 | Leaf Pest Damage | Manual | 400-1000 nm |
[35] | Hangzhou, China | Y Liangyou689 | Pest Presence | Manual | 380-1030 nm |
[115] | N/S | N/S | Heavy Metal Concentration | Manual | 350-2500 nm |
[76] | Suzhou, China | Oryza sativa | Heavy Metal Concentration | Manual | 420-980 nm |
[90] | China | Xiushi 134, Zhejing88 | Herbicide Toxicity | Manual | 380-1030 nm |
Study | Region | Rice species | Inferred magnitude | Acquisition | Spectral region |
[50] | Zhenjiang, China | N/S | Seed Origin | Manual | 499-950 nm |
[143] | Vietnam | 90 Cultivars | Seed quality: Shape, length, width and colour | Manual | 385-1000 nm |
[145] | China | Zhongzheyou No.1, 5, 8 and 86 | Seed Type | Manual | 1069-1558 nm |
[125] | China | N/S | Seed Vitality | Manual | 992, 1012, 1119, 1167, 1305, 1402, 1629, 1649 nm |
[142] | Laos | Oryza Sativa | Grain Yield | Manual | 710-740 nm, 830 nm |
[134] | Zhejiang, China | Xiushui 110 | Panicle Health | Manual | 350-2500 nm |
[158] | Kyoto, Japan | Kinu-Hikari | Protein Content | Manual | 400-1000 nm |
[47] | South Korea and China | N/S | Rice Geographical Origin | Manual | 400-1000 nm |
[147] | Heilongjiang, China | Longjing 32 | Rice Lodging Classification | UAV | 400-700 nm |
[138] | Taibao City, Taiwan | TaiKeng 2 | Rice Lodging Classification | UAV | VIS |
[109] | South Korea | N/S | Yield | Satellite | 400-890 nm |
For rice cultivation, the studies in Tables 2-5 contemplate different rice species, inferred magnitudes such as total N content, chlorophyll, seed type, viability and/or origin, disease and plague presence, herbicide toxicity, carbon content, and the spectral region in which these properties manifest as a measurable spectral response in plants or cultivated rice. Nevertheless, only 20% of the reviewed literature examine soil variables and their direct relation with rice plant growth and health [31,32,33,49,76,109,110,111,112,113,114,115], especially to set up a single data base which appraise both to establish accurate calibration model for remote sensing imaging predictions. As observed in Tables 2 to 5, the majority of rice studies are executed in Asian locations, particularly in mainland China, as is illustrated in Figure 3. Further, the predominant acquisition methodology in the surveyed literature is manual acquisition, where spectral measurements are captured in the field by handheld spectral measurement devices. It is important to know that, while most handheld devices measure a single spatially averaged spectrum (depending on the instrument's Field of View), the insights obtained from the studies on collected spectra can be applied to spatial HSIs if/when available. The justification for the use of handheld spectrometers is twofold: first, there is a limited number of satellite missions with onboard measurement devices that are relevant to agricultural applications in adequate spatial resolutions with public data accessibility. Second, for the purposes of a single or small amount of studies, there is a significant decrease in cost and planning time requirements when using handheld devices in comparison to mobile acquisition platforms, such as UAVs. Figure 1a illustrates the distribution of employed sensor platforms for hyperspectral imaging of rice, showing a clear majority of handheld measurement devices, followed by UAV-mounted sensors. Additionally, the majority of the studies are performed on a nonstandardized plurality of individual rice cultivars, which have not been spectrally catalogued according to their visible or NIR spectral responses for future reference.
This problem is further compounded by the continuous selective breeding of improved rice cultivars with attractive features for individual regions such as resistance to heat/cold, pests, presence of pollutants or increased productivity for a given amount of fertilizer input. For this reason, signal processing methodologies for precision agriculture of rice crops must be robust enough to accommodate for slight variations in spectral responses from newly introduced rice cultivars, in addition to various factors that are vulnerable to imminent shifting climate conditions, such as drought/flood responses and soil quality [55].
A large part of recent studies is focused on the use of hyperspectral reflectance data. That is, the evaluation of the spectral behavior of an incident light's fraction of reflected photons off an object of study. However, a subset of studies includes the fluorescent spectrum, which are obtained by capturing the spectrum of the light emitted by the target of interest when exposed to an ultraviolet (UV) light source. Due to the low intensity of the emitted light, fluorescence studies tend to be limited to laboratory environments, where the reflectance component of other light sources can introduce additive noise to the fluorescence spectral measurements [116,117,118,119,120].
While there exist various hardware configurations for hyperspectral image acquisition such as Whisk Broom [121], Push Broom [122] and Computer Tomography Imaging Spectrometers [123], the current study is focused on the study of the measured spectral response data. Table 1 summarizes the most commonly used sensors in studies utilizing multispectral and/or hyperspectral imaging devices for rice cultivation applications, according to their operating principles.
For applications where hyperspectral reflectance is measured in the field, the incident light from the sun is considered. This presents an advantage in crop field applications, where the use of the sun as a light source reduces the complexity of the acquisition platform. Conversely, in laboratory measurements, a broadband illumination source is employed [103,124,125,126]. Across the reviewed literature, we found that a total of 37 studies (63%) performed measurements in the field, while 15 (25%) performed lab measurements with an artificial light source. The proportion of these studies, is illustrated in Figure 5, including the 7 studies that make use of publicly available spectral measurements.
Tables 2 to 5 summarize the most relevant work performed on the application of hyperspectral imaging for rice cultivation, highlighting the main objects of study and the extent of the spectral ranges evaluated in each work. These Tables present the acquisition method, spectral region, rice species use in each work and the region of the study, which can be used as a reference of local climate conditions. It is important to highlight that the local soil conditions were only taken into consideration for the proposed methodologies in two individual studies [33,49]. With the exceptions of [127] (which employed a publicly available dataset from the NEON 2013 Imaging Campaign in California [128]), and [32,109,112,113,114,129] (which employed publicly available Landsat and/or MODIS measurements), all the surveyed literature included their individual data collection campaigns as part of their contributions.
Table 2 summarizes the most relevant works in hyperspectral imaging applications for biomass and vegetation estimation. These studies allow for remote detection of individual rice plants [33], common weeds [149] and large-scale landcover identification of the presence of rice paddies [112,114,129]. In addition, several studies introduce the capability of estimating the total Above Ground Biomass (AGB) of individual plants from their spectral measurements [131,132,141,146].
Development of automated plant detection methodologies represent a useful tool for estimating germination rates during early development stages, as well as determining the presence of weeds in rice fields, which can hinder the development of rice plants and reduce yields. To this end, the study [33] employs visible and NIR multispectral measurements, acquired using an UAV, to discriminate rice plants from background soil spectral measurements. Supplementally, an in-depth comparative study of the classification performance of various machine learning algorithms for discriminating between rice plants and common weeds is presented in [149]. [84] study the optimal height to operate a UAV to extract the Leaf area index LAI, and leaf dry weight (LDW) and evaluating the performance of hyperspectral images as a metric of how good their measurements were.
Currently, leaf color charts are distributed to rice farmers in order to estimate the N levels present in the plants. This visual cue is intended as a low-cost diagnostic tool in order to ensure adequate fertilization levels and to maximize yields [150]. However, previous work on the spectral properties of rice plants suggests that the 700-900 nm spectral region describes significantly superior statistical separability between spectral measurements of rice leaves at different fertilization levels [119,120,126,130,151]. Due to the fact that this optimal spectral range is outside the visible spectrum (380-740 nm) [136], hyperspectral imaging has been adopted as an imaging solution that is capable of visually capturing the differences in N levels in the appropriate spectral range. Thus, timely monitoring of N status of rice crops with remote sensing can aid the optimization of N fertilizer management and reduce environmental stresses for improving plant growth.
Spectral measurement sensors (either imaging or non-imaging spectrometers) have the sole function of acquiring the spectral response of the target of interest [70]. That is, these devices are not capable, nor is it their intended function, of directly measuring the physical magnitudes of relevance to rice crop health and productivity assessment efforts. For this reason, several studies have been performed towards building signal processing models to estimate rice plant and soil conditions of interest from their spectral responses. [23,103,117,127,144,148,153].
Table 3 summarizes the most relevant studies that use spectral measurements to estimate the N [31,118,120] or Chlorophyll [137,144,152,153] contents in rice plants using a variety of acquisition methodologies and processing techniques.
Of these physical magnitudes, Leaf Nitrogen Content (LNC) is the most common in the current state of the art, due to its closer relationship to rice crop yields. While the majority of these approaches attempt to directly estimate N levels from hyperspectral measurements, there have been efforts in further refining the accuracy of these models by incorporating additional sources of information, such as remote LIDAR measurements [74,117].
The LNC is an indicator of the N nutrients in the plants which an indirect variable extracted under different techniques from visible, Near infrared (NIR) or Short Wave Infrared (SWIR) data. It is used as indicator of the crops growth status, which it is related with the crop field. An estimation of the N content indicators in rice crop leaves using to different leaf clip meters is presented in [31], where two clippers provide an estimated value of N nutrient related indicators as: Chlorophyll, Flavonoid Content and Nitrogen Nutrient Index (NNI). By linear regression a comparative performance is presented for different fertilization rates and three experiments sets using various types of rice species. In [120], LNC is estimated from random leaf leave samples were collected from a single cultivar where different level of urea fertilizer is applied at seeding, tillering and shooting stages. Fluorescence intensity and ratio where performed at lab at 350 nm, 460 nm and 556 nm and compare the results and provides an insight of better performance of the regions where fluorescence data is used. Similar than [120], in [118] and estimation of the LNC from different samples collected at footing, heading and tillering stages. Additional their work propose a combine spectral index using hyperspectral LiDAR and fluorescence LiDAR. A study using SWIR to improve the accuracy in the estimation of LNC using Continuous Wavelet Analysis (CWA) is developed in [133], where they combine CWA and spectral index method using random data from a 4 set of experiments using rice and wheat cultivar to extract Narea, and Nmass with the leaf mass area (LMA). A difference of [133], in [120] use Back propagation Neural network BPNN combines with principal component analysis to show the spectral response of LNC. A single spectral study in [49] computes a spectral index and relates it to the N levels in canopy rice. In addition, it is of great interest to detect the presence of rice plants in the early season in order to reseed specific areas with convenient timing [33].
Regarding the different stages of plant growth, the state of the art on hyperspectral image analysis of rice plants at the seedling stage is focused on the detection of the presence of pests [35] and diseases [103,139] that can severely impact yields by preventing plants from developing into their productive stages. Conversely, studies on plants past the seedling stage are centered on the maximization of crop yield through the determination of various chemical properties indicative of plant health [127,152,153]. Due to the need to supply the growing world population with this basic crop, it is important to know the development cycles of this plant, as well as the factors, both climatic and environmental, that limit its development and yield, and the impact on human health. It is also necessary to consider all the methodologies and technologies available for crop analysis including hyperspectral images, canopy reflectance spectra, examine the mechanisms of pollutants uptake and translocation in rice [13], Phenology, remote sensing and near-surface imaging spectroscopy, among others. The presence of various contaminants and/or diseases can negatively impact the nutritional content of crops [35,108,103,139,156]. All the studies are summarized in Table 4 where diseases, pest, heavy metal contaminants and Pollutants are presented.
Furthermore, hyperspectral imaging applications have been developed for specific aspects of the rice cultivation industry beyond plant health and growing status, such as identification of specific plant genotypes [137,145,149,157], and seed type and vitality identification [91,125]. These studies address important concerns beyond the growing process and aid in the assessment of various aspects of grain quality, viability and/or geographical origins. These studies are summarized in Table 5.
The mathematical relationship between the spectral measurements of rice plants extracted from hyperspectral images, and the actual physical magnitudes of interest in the plants to be estimated is highly nonlinear, and a nontrivial task to accurately determine [45]. When attempting to establish a mathematical model that describes the relationship between two such magnitudes, it is important to carefully evaluate the available established methodologies for achieving this task. The most relevant considerations in the surveyed literature regarding the specific methodologies used are summarized in Tables 6 and 7.
Study | Processing method | Input variable | Output variable | Performance metric | Performance |
[137] | ANOVA/CART | Spectral Reflectance | Cultivar Species | Accuracy | 0.98 |
[126] | PCA-SVM | Spectral Reflectance | Rice Blast Presence | Accuracy | 0.975 |
[103] | PCA/Linear Discriminant Method | Spectral Reflectance | Rice Blast Presence | Accuracy | 0.92 |
[155] | Convolutional Neural Network | RGB Image | Pest Presence | Accuracy. | 70.0%-87.2% |
[34] | Sequential Forward Selection (SFS) | Spectral Reflectance | Disease Presence | Accuracy | 0.965 |
[165] | PCA + SVM | Spectral Reflectance | Quinclorac Presence | Accuracy | 0.9 |
[112] | Random Forest/SVM | Spatiotemporal Satellite Data | Paddy Rice Map | Accuracy | 98.67%/93.87% |
[114] | EVI Thresholding | Satellite Images | Hazardous Flooding Classification | Overall Accuracy | 0.7596 |
[32] | CNN | Satellite Images | Paddy Rice Map | R2 | 0.9945 |
[35] | SPA/Neural Network Method | Spectral Reflectance | Infestation Degree | Accuracy | 0.95 |
[149] | SPA + Weighted SVM | Spectral Reflectance | Crop Class | Accuracy | 0.97 |
[10] | Partial least square discriminant analysis (PLS-DA) | Spectral Reflectance | Rice Origin Classification | Accuracy | 0.99 |
[147] | Convolutional Neural Network | RGB + Multispectral Image | Rice Lodging Classification | Dice coefficient | 0.9284 |
[33] | Decision Tree | RGB, NIR, MS images | Rice Plant Presence | Overall Accuracy, Kappa Coefficient | 92.56%/0.86 |
[138] | Maximum Likelihood/Decision Tree Classification | Hybrid spatio-spectral image | Rice Lodging Classification | Accuracy | 0.9384 |
[129] | N/S | Spectral Reflectance | Rice Paddy Presence | Kappa Coefficient | 0.89 |
[50] | SVM | Spectral Reflectance | Seed Origin | Accuracy | 0.9167 |
[143] | LDA + RandomForest | Spectral Reflectance | Seed Variety | Precision | 0.9859 |
[145] | Random Forest | Spectral Reflectance | Cultivar Species | Accuracy | 1 |
[125] | PLSDA, LSSVM, ELM | Spectral Reflectance | Seed Viability | Accuracy | 0.9367 |
[113] | Linear Regression | Spatiotemporal Satellite Data | Cultivated Area | R2 | 0.94 |
Study | Processing method | Input variable | Output variable | Performance metric | Performance |
[146] | Multivariate regression | Multispectral NIR Images | Above Ground Biomass | Correlation | 0.76 |
[131] | Correlation Analysis | Spectral Reflectance | Above Ground Biomass | R2 | 0.77 |
[141] | Thresholding/Linear Regression Approach | Spectral Reflectance | Above GroundBiomass | R2 | 0.94 |
[132] | Linear and Nonlinear Regression | Spectral Reflectance | Above Ground Biomass | R2 | 0.82 |
[144] | SVM | Spectral Reflectance | Chlorophyll density | R2 | 0.55 |
[153] | TUI | Spectral Reflectance | Chlorophyll density | Correlation | 0.803 |
[152] | Linear Regression | Spectral Reflectance | Chlorophyll density | R2 | 0.84 (Sunlit Leaves), 0.9 (Shadowed Leaves) |
[139] | Multiple Linear Regression | Spectral Reflectance | Infestation Degree | R2 | 0.889 |
[142] | PLS Regression | Spectral Measurements | Estimated Yield | R2 | 0.873 |
[115] | PLS Regression | Spectral Reflectance | Cd-Pb Stress Level | R2 | 0.90 for Cd and 0.92 for Pb |
[76] | PLS Regression | Spectral Reflectance | Cd-Pb Concentration | R2 | 0.592, 0.013 |
[36] | Linear Regression | Spectral Reflectance | RLF Damage Severity | R2 | 0.987 |
[31] | Linear Regression | Spectral Reflectance | LNC | R2 | 0.84 |
[120] | Fully-Connected NN | Fluorescence Spectra | LNC | R2 | 0.867 |
[118] | Fully-Connected NN | Reflectance + Fluorescence Measurements | LNC | R2 | 0.81 |
[133] | Continuous Wavelet Analysis | Spectral Reflectance | LNC | R2 | 0.77 |
[154] | Multivariate regression | Spectral Reflectance | LNC | R2 | 0.72 |
[74] | Regression | RGB/MS/CIR Data | LNC / PNA | R2 | LNC 0.81. PNA 0.84 |
[148] | Linear Regression | Spectral Reflectance | LNC | R2 | 0.56 |
[151] | SVM | Spectral Reflectance | LNC | R2 | 0.73 |
[117] | SVM, BP-NN, RBF-NN | Spectral Reflectance and Fluorescence | LNC | R2 | 0.979 |
[49] | Correlation | Spectral Reflectance | LNC | R2 | 0.679 |
[24] | Color Visibility Analysis | RGB Image | N Fertilizer Level Needed | R2 | 0.998 |
[152] | Linear and Nonlinear Correlation | Spectral Reflectance | LNC | R2 | 0.78 |
[119] | PCA/Neural Network Method | Spectral Reflectance and Fluorescence | LNC | R2 | 0.912 |
[110] | Linear Regression | Spectral Reflectance | LNC | R2 | Elongation stage:0.68; Heading stage:0.85 |
[135] | Stepwise Multiple Linear Regression (SMLR) | Spectral Reflectance | LNC, PNA | R2 | LNC: 0.20, PNA:0.73 |
[48] | PLS Regression | Spectral Reflectance | Canopy N Content | R2 | 0.917 |
[130] | SVM | Hyperspectral LIDAR Measurements | LNC | R2 | 0.75 |
[140] | PLS Regression | Spectral Reflectance | LNC | R2 | 0.84 |
[23] | Linear Regression | Spectral Reflectance | LNC | R2 | 0.84 |
[130] | SVM, MLP-NN, RBF-NN | Spectral Reflectance | LNC | R2 | 0.75 |
[75] | Correlation Analysis | Spectral Reflectance | N, Phosphorus, Sulphur | R2 | N 0.80, P 0.69, S 0.73 |
[134] | PCA + SVM | Spectral Reflectance | Panicle Health | Accuracy | 0.9914 |
[111] | Linear regression, K-means Clustering | RGB Image | PNA | R2 | 0.95 |
[158] | PLS Regression | Spectral Reflectance | Protein content | R2 | 0.76 |
[84] | Linear Regression | Spectral Reflectance | RVI | R2 | 0.81 |
[109] | GRAMI | Landcover/Spectral Reflectance | Rice Yield | Overall Accuracy | 89.5%-90.2% |
In this regard, a significant subset of the surveyed literature employs regression-based approaches to optimize for maximum correlation between specific spectral regions and the physical magnitudes of interest [23,139,148], where the proportion of the dependent variable's variance that can be explained from the selected independent variables [159] is taken as a performance measure (R2 value). In these approaches, the spectral measurements (or a subset thereof) are approximated to be independent variables, or predictors, in a linear fashion for the specific dependent variables, or measured responses (such as N content, carbon content, etc.), being studied. Additionally, several studies employ correlation analysis between the behavior of a reduced subset of spectral bands and the target magnitudes of interest [49,127,157]. These approaches explore the correlation coefficient r, {r ∈ R|0 ≤ r ≤ 1} between individual spectral intensities x ∈ R, and the measured responses of interest y ∈ R:
(1) |
where cov(x, y) is the covariance of the spectral measurement x and the magnitude of interest y, and sx2, sy2 are the variances of x and y, respectively. Several regression-based approaches perform a comparative statistical study to find a subset of spectral bands with high correlation to the variables of interest [31,132,152]. It is important to note that while this practice introduces an advantageous computational complexity reduction, it discards a significant number of spectral measurements that could be taken into consideration with the use of Principal Component Analysis (PCA) or similar dimensionality reduction techniques [119,134]. For classification-oriented applications, the surveyed literature presents a variety of methodologies including Support Vector Machines (SVM) [50,125,126,149,159], Neural Networks [35] and Random Forest-based approaches [145].
Support Vector Machine (also, later generalized under the name Kernel Machine) classification of HSIs is a binary approach that relies on finding an optimal separating hyperplane by maximizing the perpendicular distance (given by support vectors) from the hyperplane to the closest data points from the two classes on both sides of it. In the case that a linear hyperplane is not able to satisfactorily discriminate between the two features with a significant margin using the dimensionality of the features, it is possible to map the features to be classified to a higher-dimensional space, in order to maximize class separability using a nonlinear kernel function (hence the alternate description of kernel machine) [160,161]. Compared to modern approaches (particularly, deep neural networks), SVM classification approaches consistently produce accurate results in applications with the limitation of reduced availability of training data [162].
Neural Network classification of HSIs is a non-parametric approach that employs the backpropagation algorithm [163] to train a neural network for pixel classification in a "black box" approach. In this case, a neural network is setup to have as many input nodes as spectral bands in a HSI pixel, one or more hidden layers with a variable number of nodes, and an output node consisting of the class assignment or inferred magnitude estimation for the input information. Alternatively, Convolutional Neural Networks (CNN) are Neural Network architectures optimized for operating on spatially distributed data, as is the case with HSIs [147,155], as opposed to Fully-Connected approaches that operate on a pixelwise manner [118,120,164]. While these approaches have recently introduced significant improvements on classification problems, they require vast amounts of training data to produce accurate models, which include nontrivial processing hardware requirements for the network training operation. These methodologies present the advantage of producing accurate statistical models for rice cultivation in reasonable processing times without the need of high-performance specialized hardware. However, the accuracy of the calculated models shows a consistent disadvantage when compared to alternate, more computationally complex, methodologies such as those based on PCA [103,119,126,134,165], Support Vector Machines [50,112,117,125,126,130,144,151] and Artificial Neural Networks [117,119,130]. At the cost of increased accuracy, it is important to know that these methods describe a higher computational complexity, and it is important to take their required dedicated resource allocation and computing time when designing a deployable hyperspectral image analysis-based application.
In classification problems, where the employed methodologies produce discrete class outputs for detection purposes, results are stated using the Accuracy metric, calculated by dividing the number of accurately classified pixels from each respective study's testing set by the total number of pixels for each class [166]. As can be observed in several studies in Table 6 [10,103,126,134,137,143,165], these discrete classification applications tend to produce results with high accuracy. This can be explained by the high statistical separability of the target spectra in these studies, which most commonly related to the presence various types of plant pests and/or diseases, when compared to the spectra of healthy plant tissue and background contents in specific wavelength ranges.
Contrary to classification-based approaches, applications concerned with estimating a nondiscrete magnitude, such as LNC, from spectral measurements tend to describe lower performance values [49,130,140,148,154]. This discrepancy can be explained by the considerably larger continuous solution space of magnitude inference problems, compared to the discrete solution space in classification problems [167].
In order to improve the specific performance of the surveyed methodologies, several approaches incorporate an additional preprocessing step in order to maximize the statistical separability of key variables of interest in the acquired hyperspectral data. Depending on the required complexity of each methodology, this preprocessing step can range from a computationally inexpensive subset selection and/or scaling on the values of key spectral bands [130,133,144,148], to a more computationally complex operation, such as Linear Discriminant Analysis PCA [103,119,126,134,165] that takes into account the behavior of the relevant spectral signatures across the available spectrum.
We have observed a series of common challenges across the reviewed literature that represent limitations regarding the viability of deployment of hyperspectral imaging applications in the field. These challenges are summarized in Figure 6.
Due to the difficulty and cost of obtaining hyperspectral images of sites of interest, some of the surveyed studies perform field measurements at the locations of previously performed aerial hyperspectral imaging expeditions. This temporal mismatch between hyperspectral and field-collected measurements introduces a significant error into the final mathematical models due to a myriad of possible condition changes (illumination, change in vegetation composition, erosion, anthropogenic canopy changes, water content, etc.) between the acquisition of the input and target model data.
Because the acquisition and processing of these images into useful results for precision agriculture is an economically, computationally, and time intensive process, research efforts have been focused on finding static relationships between the acquired spectral measurements and the physical magnitudes of interest for a specific geography. Collectively, these approaches do not incorporate the modeling of the productivity influence of additional factors that are at risk of undergoing significant variations in behavior due to imminent climate change, such as shifts in temperature trends, soil and/or water acidity, wind and water current patterns, seasonal duration changes, among others. In addition, the surveyed literature is concerned with the study of the behavior of specific rice cultivars under the variation of specific relevant factors, such as fertilization levels. Thus, an important direction for future research is to begin working towards a unified characterization model for various cultivars and their requirements. As it is, the performance levels obtained in the surveyed literature do not necessarily imply that growing different cultivars under the same conditions would provide similar results. An accurate future productivity model that is able to adequately incorporate these factors would enable a significant increase in estimated yield predictions for any region around the globe.
Further, a study for hyperspectral images mountain forest canopy suggests that there is a nontrivial discrepancy in the measured spectral responses of remotely sensed canopies due to topographical irregularities [168]. While this is an issue that is less prevalent in most current rice fields due to their conventionally flat topographies, it is within the realm of possibility that planting areas will expand to less favorable topographies in the future due to the increasing need for additional farmland to support the growing population and compensate for the nutrient depletion of existing farmland.
Rice cultivation and, by extension, any hyperspectral imaging-based applications for its improvement, is a highly multivariate dynamic problem where a variety of intertwined factors such as soil composition, location, local macro/microclimates, fertilization levels, fertilizer usage, farming practices and a myriad of additional factors can impact the viability of a given method outside of the conditions present in individual research efforts. Given the imminent threat of global climate change which, beyond the effects of changes in climate, can have additional effects on rice cultivation practices, among which can be migration to new agricultural regions with more favorable climates but different soil chemical properties and the emergence of novel rice cultivars better suited to these conditions. In addition, even small supply chain disruptions can have a significant impact on the composition and efficacy of the various production inputs used in precision agriculture.
The surveyed literature consists mostly of studies performed over 1-5 growing seasons in a given region with known conditions. Currently, shifting climate dynamics around the world potentially threaten the long-term usability of the studies performed for any given region. Thus, hyperspectral imaging applications for rice cultivation will be required to take into account several dynamic factors and, most importantly, adequately model their influences on time-resolved recorded spectral measurements over multiple decades, whenever possible. It is our consideration that addressing these considerations will play a critical role in consolidating the long-term deployability and effectiveness of a given contribution in the face of imminent climate change.
Moreover, the lack of a standardized, publicly-available dataset for the development of signal processing methodologies for various aspects of the rice cultivation using hyperspectral images requires individual research groups to plan and execute their data collection expeditions over significant stretches of time, spanning up to various cultivation cycles [23]. Beyond this, it hinders the capability of research groups in different geographies to accurately compare their results to the available literature by isolating the differences in spectral responses between local cultivars and soil compositions. In the surveyed literature presented in Tables 2 to 5, it is apparent that a significant majority of studies relies on manual acquisition of data using specialized spectral measurement equipment, such as spectrometers and/or hyperspectral cameras. These approaches require, in addition to the nontrivial matter of the equipment acquisition, skilled personnel capable of operating the equipment in a manner conductive to estimating the relevant magnitudes for a given application. These concerns present an important limitation on the widespread deployment of laboratory-derived innovation to applications in the field. In contrast, a significant minority of the surveyed literature describes systems capable of automated acquisition using UAVs [33,74,135,138,146,147,148,153]. In order to provide better accessibility of the technological advances being developed, it is our consideration that it is necessary for either public or private actors to further advance the consolidation and deployability of these analytical studies in order to facilitate their availability as a service, either directly to crop farmers in order to maximize the efficiency of their efforts, or towards funding agencies in order to streamline the process of evaluating the development of their investments, and better calibrate their investment return expectations to increase availability of funding sources for crop producers. One major limitation consistent across the majority of surveyed literature is the use of manual measurements to compute the published models. In order to minimize potential ongoing costs for widespread hyperspectral technology deployments to rice growers, it is important to continue producing models based on UAV-acquired hyperspectral data. These approaches enable the acquisition of a significantly higher volume of data, in an easily scalable manner according to plantation size with reduced labor requirements. On the topic of widespread deployability to growers, it is our consideration that additional research effort is required on the development of solutions that provide succinct, actionable insights to end users to maximize the effects of their labor. An example of such applications is the work published in [24], which uses the inferred LNC to provide end users with an indication of the required fertilizer levels to be applied. Beyond the possibility of providing instructions to a human operator, further work on these applications can play a pivotal role in the context of a precision agriculture system that distributes fertilizers through automated actuators with little to no human intervention, further reducing the potential impact on food production due to population migration and worker shortages.
It is important to highlight that most previous studies include the cultivation of rice plants under predefined conditions in a controlled environment in order to isolate the relevant parameters for each study. Thus, in order to successfully perform a large-scale deployment of the findings of the surveyed literature, it is required to dedicate future research efforts in the modeling of the influence of various local conditions on the health and productivity of rice crops outside of the specific regions of study. Success in these efforts would enable efficient deployments based on these findings in a wider variety of regions with different soil compositions and climatological conditions. In addition, in the current atmospheric conditions, it is becoming increasingly necessary to model the influence of atmospheric pollution both on the health of rice plants in existing plantations, and on the viability of soil for the informed allocation of land for new plantations in order to satisfy growing production needs.
The current review synthesizes and highlights the most relevant works regarding the use of hyperspectral imaging sensing technology and hyperspectral image processing algorithms for the estimation and monitoring of various aspects of the rice cultivation process. The significant contributions in literature are summarized according to their objectives and summarized in tables according to the specific constraining factors considered such as biomass and vegetation estimation, landcover classification of rice growing sites; Chlorophyll and N content estimation; Studies of diseases, pests, heavy metals and other pollutants' effects on rice crops; Studies focused in various seed properties, lodging and others variables. Relevant information about the studies performed are presented, such as the acquisition methodology, considered spectral region, rice cultivars used in each work and the region of the study, which can be used as a reference of local climate conditions.
The hyperspectral signal processing methods used in rice production are summarized in terms of whether the objective of the individual studies is to infer a specific magnitude, or to classify the spectral measurements into discrete classes. Further, the specific approach used in each study, where the most relevant methodologies are discussed, such as statistical, linear or multiple spectral transformations and combined advanced methodologies. Additionally, the current review identifies the common challenges present across the proposed hyperspectral systems for rice cultivation that, in our consideration, are highly relevant to the goal of developing widely deployable applications of this technology, with the objective of supporting the efficient agro-industrial production of rice crops. The widespread deployment of the surveyed technological advancements is crucial for achieving global sustainability requirements, which is an important challenge in the current age. Further, these developments enable the advancement of various important global challenges related to uplifting communities from poverty, and to provide them with equal opportunities in a manner that is environmentally sustainable.
Regional or country-scale remote sensing deployments of rice field mapping and yield-estimating technologies can be a valuable planning tool for policymakers and help to reach established Sustainable Development Goals. Successful integration of these methodologies into the decision-making process can significantly reduce potential food waste and economic losses through more accurate import/export rates. For these reasons, it is of great importance for upcoming research to focus on the development of low-cost, user-friendly spectral imaging devices that can be more feasibly deployed in growing operations of varying scales.
Finally, in order to more effectively extract as much information from the necessary higher volume of time-resolved hyperspectral remote sensing images, and adequately model the influence and trends of shifting climate conditions and its effects on rice cultivation in a precision agriculture setting, it is imperative to take advantage of any developments in multidimensional signal processing methodologies for feature extraction applications.
This research was funded by Grant/Award Number: FID 2018-096 of the Research funding programs of the National Secretariat for Science, Technology, and Innovation of Panama (SENACYT). The authors acknowledge also, SENACYT for the support through its Panama National Research System (SNI by his acronym in Spanish).
The authors declare no conflict of interest. The funding entity had no role in the design of the study; in the collection, analyses methodologies, or interpretation of data; in the writing of this manuscript, or the decision to publish the results.
[1] | Chauhan BS, Jabran K, Mahajan G (2017) Rice production worldwide, Springer. |
[2] |
Hu EA, Pan A, Malik V, et al. (2012) White rice consumption and risk of type 2 diabetes: Meta-analysis and systematic review. Bmj 344: e1454. doi: 10.1136/bmj.e1454
![]() |
[3] |
Seck PA, Diagne A, Mohanty S, et al. (2012) Crops that feed the world 7: Rice. Food Secur 4: 7-24. doi: 10.1007/s12571-012-0168-1
![]() |
[4] |
Fuller DQ, Sato YI, Castillo C, et al. (2010) Consilience of genetics and archaeobotany in the entangled history of rice. Archaeol Anthropol Sci 2: 115-131. doi: 10.1007/s12520-010-0035-y
![]() |
[5] | Food and Agriculture Organization of the United Nations (2018) Rice market monitor 2018, Rice Market Monitor XXI. |
[6] | Tan BL, Norhaizan ME (2020) Rice demands: A brief description, Rice by-products: Phytochemicals and food products application, Springer, 7-11. |
[7] |
Peng S, Tang Q, Zou Y (2009) Current status and challenges of rice production in China. Plant Prod Sci 12: 3-8. doi: 10.1626/pps.12.3
![]() |
[8] |
Van Nguyen N, Ferrero A (2006) Meeting the challenges of global rice production. Paddy Water Environ 4: 1-9. doi: 10.1007/s10333-005-0031-5
![]() |
[9] | Barker R, Dawe D, Tuong T, et al. (2020) The outlook for water resources in the year 2020: Challenges for research on water management in rice production. Southeast Asia 1: 1-5. |
[10] | Mo C, Lim J, Kwon SW, et al. (2017) Hyperspectral imaging and partial least square discriminant analysis for geographical origin discrimination of white rice. J Biosyst Eng 42: 293-300. |
[11] |
Tong Q, Swallow B, Zhang L, et al. (2019) The roles of risk aversion and climate-smart agriculture in climate risk management: Evidence from rice production in the Jianghan Plain, China. Clim Risk Manage 26: 100199. doi: 10.1016/j.crm.2019.100199
![]() |
[12] |
Balasubramanian V, Sie M, Hijmans R, et al. (2007) Increasing rice production in sub-Saharan Africa: Challenges and opportunities. Adv Agron 94: 55-133. doi: 10.1016/S0065-2113(06)94002-4
![]() |
[13] |
Chen J, Zou W, Meng L, et al. (2019) Advances in the uptake and transport mechanisms and QTLs mapping of cadmium in rice. Int J Mol Sci 20: 3417. doi: 10.3390/ijms20143417
![]() |
[14] |
Jin XB, Yu XH, Wang XY, et al. (2020) Deep learning predictor for sustainable precision agriculture based on internet of things system. Sustainability 12: 1433. doi: 10.3390/su12041433
![]() |
[15] | Salam A, Shah S (2019) Internet of things in smart agriculture: Enabling technologies, 2019 IEEE 5th world forum on internet of things (WF-IoT), 692-695. |
[16] |
Kundu M, Krishnan P, Kotnala R, et al. (2019) Recent developments in biosensors to combat agricultural challenges and their future prospects. Trends Food Sci Technol 88: 157-178. doi: 10.1016/j.tifs.2019.03.024
![]() |
[17] |
Thakur D, Kumar Y, Kumar A, et al. (2019) Applicability of wireless sensor networks in precision agriculture: A review. Wireless Pers Commun 107: 471-512. doi: 10.1007/s11277-019-06285-2
![]() |
[18] | International Telecommunications Union and XPRIZE Foundation (2017) AI for good global summit report, Geneva, Switzerland, United Nations. |
[19] | Bejaoui C, Guitton A, Kachouri A (2017) Efficient data monitoring of rice-fields based on WMSN, 2017 IEEE/ACS 14th international conference on computer systems and applications (AICCSA), 1090-1094. |
[20] |
Chen WL, Lin YB, Ng FL, et al. (2019) RiceTalk: Rice blast detection using internet of things and artificial intelligence technologies. IEEE Internet Things J 7: 1001-1010. doi: 10.1109/JIOT.2019.2947624
![]() |
[21] |
Gao Z, Li W, Zhu Y, et al. (2018) Wireless channel propagation characteristics and modeling research in rice field sensor networks. Sensors 18: 3116. doi: 10.3390/s18093116
![]() |
[22] |
Sabet FS, Hosseini M, Khabbaz H, et al. (2017) FRET-based aptamer biosensor for selective and sensitive detection of aflatoxin B1 in peanut and rice. Food Chem 220: 527-532. doi: 10.1016/j.foodchem.2016.10.004
![]() |
[23] |
Hung T, Byun W (2006) Assessment of rice leaf growth and nitrogen status by hyperspectral canopy reflectance and partial least square regress. Eur J Agron 24: 349-356. doi: 10.1016/j.eja.2006.01.001
![]() |
[24] |
Intaravanne Y, Sumriddetchkajorn S (2015) Android-based rice leaf color analyzer for estimating the needed amount of nitrogen fertilizer. Comput Electron Agric 116: 228-233. doi: 10.1016/j.compag.2015.07.005
![]() |
[25] |
Wang L, Liu D, Pu H, et al. (2015) Use of hyperspectral imaging to discriminate the variety and quality of rice. Food Anal Methods 8: 515-523. doi: 10.1007/s12161-014-9916-5
![]() |
[26] | Caballero D, Calvini R, Amigo JM (2020) Hyperspectral imaging in crop fields: Precision agriculture, Data handling in science and technology, Elsevier, 453-473. |
[27] |
Lu B, Dao PD, Liu J, et al. (2020) Recent advances of hyperspectral imaging technology and applications in agriculture. Remote Sens 12: 2659. doi: 10.3390/rs12162659
![]() |
[28] |
Foster DH, Amano K (2019) Hyperspectral imaging in color vision research: tutorial. JOSA A 36: 606-627. doi: 10.1364/JOSAA.36.000606
![]() |
[29] |
Khan MJ, Khan HS, Yousaf A, et al. (2018) Modern trends in hyperspectral image analysis: A review. IEEE Access 6: 14118-14129. doi: 10.1109/ACCESS.2018.2812999
![]() |
[30] |
Paoletti M, Haut J, Plaza J, et al. (2019) Deep learning classifiers for hyperspectral imaging: A review. ISPRS J Photogramm Remote Sens 158: 279-317. doi: 10.1016/j.isprsjprs.2019.09.006
![]() |
[31] |
Zhang K, Liu X, Ma Y, et al. (2020) A comparative assessment of measures of leaf nitrogen in rice using two leaf-clip meters. Sensors 20: 175. doi: 10.3390/s20010175
![]() |
[32] |
Zhang M, Lin H, Wang G, et al. (2018) Mapping paddy rice using a convolutional neural network (CNN) with Landsat 8 datasets in the Dongting Lake Area, China. Remote Sens 10: 1840. doi: 10.3390/rs10111840
![]() |
[33] |
Zheng H, Zhou X, He J, et al. (2020) Early season detection of rice plants using RGB, NIR-GB and multispectral images from unmanned aerial vehicle (UAV). Comput Electron Agric 169: 105223. doi: 10.1016/j.compag.2020.105223
![]() |
[34] |
Baek I, Kim MS, Cho BK, et al. (2019) Selection of optimal hyperspectral wavebands for detection of discolored, diseased rice seeds. Appl Sci 9: 1027. doi: 10.3390/app9051027
![]() |
[35] |
Fan Y, Wang T, Qiu Z, et al. (2017) Fast detection of striped stem-borer (Chilo suppressalis Walker) infested rice seedling based on visible/near-infrared hyperspectral imaging system. Sensors 17: 2470. doi: 10.3390/s17112470
![]() |
[36] |
Huang J, Liao H, Zhu Y, et al. (2012) Hyperspectral detection of rice damaged by rice leaf folder (Cnaphalocrocis medinalis). Comput Electron Agric 82: 100-107. doi: 10.1016/j.compag.2012.01.002
![]() |
[37] |
Schneider P, Asch F (2020) Rice production and food security in Asian Mega deltas-A review on characteristics, vulnerabilities and agricultural adaptation options to cope with climate change. J Agron Crop Sci 206: 491-503. doi: 10.1111/jac.12415
![]() |
[38] |
Gebbers R, Adamchuk VI (2010) Precision agriculture and food security. Science 327: 828-831. doi: 10.1126/science.1183899
![]() |
[39] |
Ehleringer JR, Cerling TE, Helliker BR (1997) C 4 photosynthesis, atmospheric CO2, and climate. Oecologia 112: 285-299. doi: 10.1007/s004420050311
![]() |
[40] |
Sonnino A (1994) Agricultural biomass production is an energy option for the future. Renewable Energy 5: 857-865. doi: 10.1016/0960-1481(94)90105-8
![]() |
[41] |
Lichtenthaler HK, Rinderle U (1988) The role of chlorophyll fluorescence in the detection of stress conditions in plants. CRC Crit Rev Anal Chem 19: S29-S85. doi: 10.1080/15476510.1988.10401466
![]() |
[42] | Mafakheri A, Siosemardeh A, Bahramnejad B, et al. (2010) Effect of drought stress on yield, proline and chlorophyll contents in three chickpea cultivars. Aust J Crop Sci 4: 580. |
[43] |
Bannister T (1974) Production equations in terms of chlorophyll concentration, quantum yield, and upper limit to production. Limnol Oceanogr 19: 1-12. doi: 10.4319/lo.1974.19.1.0001
![]() |
[44] | Wood C, Reeves D, Himelrick D (1993) Relationships between chlorophyll meter readings and leaf chlorophyll concentration, N status, and crop yield: A review, Proceedings of the agronomy society of New Zealand, 1-9. |
[45] |
Makino A (2011) Photosynthesis, grain yield, and nitrogen utilization in rice and wheat. Plant Physiol 155: 125-129. doi: 10.1104/pp.110.165076
![]() |
[46] |
Zhou K, Cheng T, Zhu Y, et al. (2018) Assessing the impact of spatial resolution on the estimation of leaf nitrogen concentration over the full season of paddy rice using near-surface imaging spectroscopy data. Frontiers in plant science 9: 964. doi: 10.3389/fpls.2018.00964
![]() |
[47] | Matsushima S (1993) Researches on the requirements for achieving high yields in rice. Sci Rice Plant 2: 737-747. |
[48] |
Inoue Y, Sakaiya E, Zhu Y, et al. (2012) Diagnostic mapping of canopy nitrogen content in rice based on hyperspectral measurements. Remote Sens Environ 126: 210-221. doi: 10.1016/j.rse.2012.08.026
![]() |
[49] |
Lee YJ, Yang CM, Chang KW, et al. (2008) A simple spectral index using reflectance of 735 nm to assess nitrogen status of rice canopy. Agron J 100: 205-212. doi: 10.2134/agronj2007.0018
![]() |
[50] |
Sun J, Lu X, Mao H, et al. (2017) A method for rapid identification of rice origin by hyperspectral imaging technology. J Food Process Eng 40: e12297. doi: 10.1111/jfpe.12297
![]() |
[51] |
Raines CA (2011) Increasing photosynthetic carbon assimilation in C3 plants to improve crop yield: Current and future strategies. Plant Physiol 155: 36-42. doi: 10.1104/pp.110.168559
![]() |
[52] |
Nowak B, Nesme T, David C, et al. (2015) Nutrient recycling in organic farming is related to diversity in farm types at the local level. Agric, Ecosyst Environ 204: 17-26. doi: 10.1016/j.agee.2015.02.010
![]() |
[53] |
Song YQ, Zhao X, Su HY, et al. (2018) Predicting spatial variations in soil nutrients with hyperspectral remote sensing at regional scale. Sensors 18: 3086. doi: 10.3390/s18093086
![]() |
[54] |
Luo JS, Huang J, Zeng DL, et al. (2018) A defensin-like protein drives cadmium efflux and allocation in rice. Nat Commun 9: 1-9. doi: 10.1038/s41467-017-02088-w
![]() |
[55] |
Muehe EM, Wang T, Kerl CF, et al. (2019) Rice production threatened by coupled stresses of climate and soil arsenic. Nat Commun 10: 1-10. doi: 10.1038/s41467-019-12946-4
![]() |
[56] | Sharma RK, Agrawal M (2005) Biological effects of heavy metals: An overview. J Environ Biol 26: 301-313. |
[57] |
Xie P, Deng J, Zhang H, et al. (2015) Effects of cadmium on bioaccumulation and biochemical stress response in rice (Oryza sativa L.). Ecotoxicol Environ Saf 122: 392-398. doi: 10.1016/j.ecoenv.2015.09.007
![]() |
[58] |
Xue D, Jiang H, Deng X, et al. (2014) Comparative proteomic analysis provides new insights into cadmium accumulation in rice grain under cadmium stress. J Hazard Mater 280: 269-278. doi: 10.1016/j.jhazmat.2014.08.010
![]() |
[59] |
Hanak E, Lund JR (2012) Adapting California's water management to climate change. Clim Change 111: 17-44. doi: 10.1007/s10584-011-0241-3
![]() |
[60] |
Iglesias A, Garrote L (2015) Adaptation strategies for agricultural water management under climate change in Europe. Agric Water Manage 155: 113-124. doi: 10.1016/j.agwat.2015.03.014
![]() |
[61] | Ahangarha M, Seydi ST, Shahhoseini R (2019) Hyperspectral change detection in wetland and water-body areas based on machine learning. Int Arch Photogramm, Remote Sens Spat Inf Sci XLⅡ-4/W18: 1-19. |
[62] | León CD, Kosow H, Zahumensky Y, et al. (2019) Solutions and planning tools for water supply and wastewater management in prosperous regions tackling water scarcity, Mid-term Conference-Frankfurt am main, Germany 20-21 February 2019, 28. |
[63] |
Liew S, Choo C, Lau J, et al. (2019) Monitoring water quality in Singapore reservoirs with hyperspectral remote sensing technology. Water Pract Technol 14: 118-125. doi: 10.2166/wpt.2018.119
![]() |
[64] |
Prošek J, Gdulová K, Barták V, et al. (2020) Integration of hyperspectral and LiDAR data for mapping small water bodies. Int J Appl Earth Obs Geoinformation 92: 102181. doi: 10.1016/j.jag.2020.102181
![]() |
[65] |
Ge X, Wang J, Ding J, et al. (2019) Combining UAV-based hyperspectral imagery and machine learning algorithms for soil moisture content monitoring. Peer J 7: e6926. doi: 10.7717/peerj.6926
![]() |
[66] |
Huo H, Li Z-L, Xing Z (2019) Temperature/emissivity separation using hyperspectral thermal infrared imagery and its potential for detecting the water content of plants. Int J Remote Sens 40: 1672-1692. doi: 10.1080/01431161.2018.1513668
![]() |
[67] | Hurley SP, Horney M, Drake A (2019) Using hyperspectral imagery to detect water stress in vineyards, Autonomous air and ground sensing systems for agricultural optimization and phenotyping IV, 1100807. |
[68] |
Krishna G, Sahoo RN, Singh P, et al. (2019) Comparison of various modelling approaches for water deficit stress monitoring in rice crop through hyperspectral remote sensing. Agric Water Manage 213: 231-244. doi: 10.1016/j.agwat.2018.08.029
![]() |
[69] |
Zhang Z, Lou Y, Li R, et al. (2019) Estimation of relative water content in rice panicle based on hyperspectral vegetation indexes under water saving irrigation. Spectrosc Lett 52: 150-158. doi: 10.1080/00387010.2019.1594309
![]() |
[70] | Manolakis DG, Lockwood RB, Cooley TW (2016) Hyperspectral imaging remote sensing: Physics, sensors, and algorithms, Cambridge University Press. |
[71] |
Ahmed MR, Yasmin J, Mo C, et al. (2016) Outdoor applications of hyperspectral imaging technology for monitoring agricultural crops: A review. J Biosyst Eng 41: 396-407. doi: 10.5307/JBE.2016.41.4.396
![]() |
[72] | Fei B (2020) Hyperspectral imaging in medical applications, Data handling in science and technology, Elsevier, 523-565. |
[73] |
Ma J, Sun DW, Pu H, et al. (2019) Advanced techniques for hyperspectral imaging in the food industry: principles and recent applications. Annu Rev Food Sci Technol 10: 197-220. doi: 10.1146/annurev-food-032818-121155
![]() |
[74] |
Zheng H, Cheng T, Li D, et al. (2018) Evaluation of RGB, color-infrared and multispectral images acquired from unmanned aerial systems for the estimation of nitrogen accumulation in rice. Remote Sens 10: 824. doi: 10.3390/rs10060824
![]() |
[75] |
Mahajan G, Pandey R, Sahoo R, et al. (2017) Monitoring nitrogen, phosphorus and sulphur in hybrid rice (Oryza sativa L.) using hyperspectral remote sensing. Precis Agric 18: 736-761. doi: 10.1007/s11119-016-9485-2
![]() |
[76] |
Zhu Y, Tian Y, Yao X, et al. (2007) Analysis of common canopy reflectance spectra for indicating leaf nitrogen concentrations in wheat and rice. Plant Prod Sci 10: 400-411. doi: 10.1626/pps.10.400
![]() |
[77] |
Behmann J, Steinrücken J, Plümer L (2014) Detection of early plant stress responses in hyperspectral images. ISPRS J Photogramm Remote Sens 93: 98-111. doi: 10.1016/j.isprsjprs.2014.03.016
![]() |
[78] |
Römer C, Wahabzada M, Ballvora A, et al. (2012) Early drought stress detection in cereals: Simplex volume maximisation for hyperspectral image analysis. Funct Plant Biol 39: 878-890. doi: 10.1071/FP12060
![]() |
[79] |
Pearlman JS, Barry PS, Segal CC, et al. (2003) Hyperion, a space-based imaging spectrometer. IEEE Trans Geosci Remote Sens 41: 1160-1173. doi: 10.1109/TGRS.2003.815018
![]() |
[80] |
Green RO, Eastwood ML, Sarture CM, et al. (1998) Imaging spectroscopy and the airborne visible/infrared imaging spectrometer (AVIRIS). Remote Sens Environ 65: 227-248. doi: 10.1016/S0034-4257(98)00064-9
![]() |
[81] |
Banerjee BP, Raval S, Cullen P (2020) UAV-hyperspectral imaging of spectrally complex environments. Int J Remote Sens 41: 4136-4159. doi: 10.1080/01431161.2020.1714771
![]() |
[82] |
Wiegmann M, Backhaus A, Seiffert U, et al. (2019) Optimizing the procedure of grain nutrient predictions in barley via hyperspectral imaging. PloS One 14: e0224491. doi: 10.1371/journal.pone.0224491
![]() |
[83] |
Smith ML, Ollinger SV, Martin ME, et al. (2002) Direct estimation of aboveground forest productivity through hyperspectral remote sensing of canopy nitrogen. Ecol Appl 12: 1286-1302. doi: 10.1890/1051-0761(2002)012[1286:DEOAFP]2.0.CO;2
![]() |
[84] |
Yao L, Wang Q, Yang J, et al. (2019) UAV-Borne dual-band sensor method for monitoring physiological crop status. Sensors 19: 816. doi: 10.3390/s19040816
![]() |
[85] |
Fan L, Zhao J, Xu X, et al. (2019) Hyperspectral-based estimation of leaf nitrogen content in corn using optimal selection of multiple spectral variables. Sensors 19: 2898. doi: 10.3390/s19132898
![]() |
[86] |
Hruska Z, Yao H, Kincaid R, et al. (2020) Spectral-based screening approach evaluating two specific maize lines with divergent resistance to invasion by aflatoxigenic fungi. Front Microbiol 10: 3152. doi: 10.3389/fmicb.2019.03152
![]() |
[87] |
Biswas DK, Coulman B, Biligetu B, et al. (2019) Advancing bromegrass breeding through imaging phenotyping and genomic selection: A review. Front Plant Sci 10: 1673. doi: 10.3389/fpls.2019.01673
![]() |
[88] |
Feng X, Zhan Y, Wang Q, et al. (2020) Hyperspectral imaging combined with machine learning as a tool to obtain high-throughput plant salt-stress phenotyping. Plant J 101: 1448-1461. doi: 10.1111/tpj.14597
![]() |
[89] |
Liu B, Li R, Li H, et al. (2019) Crop/Weed discrimination using a field imaging spectrometer system. Sensors 19: 5154. doi: 10.3390/s19235154
![]() |
[90] |
Wang D, Vinson R, Holmes M, et al. (2019) Early Detection of tomato spotted wilt virus by hyperspectral imaging and outlier removal auxiliary classifier generative adversarial nets (OR-AC-GAN). Sci Rep 9: 1-14. doi: 10.1038/s41598-018-37186-2
![]() |
[91] |
Nie P, Zhang J, Feng X, et al. (2019) Classification of hybrid seeds using near-infrared hyperspectral imaging technology combined with deep learning. Sens Actuators B: Chem 296: 126630. doi: 10.1016/j.snb.2019.126630
![]() |
[92] |
Bangelesa F, Adam E, Knight J, et al. (2020) Predicting soil organic carbon content using hyperspectral remote sensing in a degraded mountain landscape in lesotho. Appl Environ Soil Sci 2020: 2158573. doi: 10.1155/2020/2158573
![]() |
[93] |
Chen Y, Wang J, Liu G, et al. (2019) Hyperspectral estimation model of forest soil organic matter in northwest Yunnan Province, China. Forests 10: 217. doi: 10.3390/f10030217
![]() |
[94] |
Guo L, Zhang H, Shi T, et al. (2019) Prediction of soil organic carbon stock by laboratory spectral data and airborne hyperspectral images. Geoderma 337: 32-41. doi: 10.1016/j.geoderma.2018.09.003
![]() |
[95] |
Shen Q, Xia K, Zhang S, et al. (2019) Hyperspectral indirect inversion of heavy-metal copper in reclaimed soil of iron ore area. Spectrochim Acta Part A 222: 117191. doi: 10.1016/j.saa.2019.117191
![]() |
[96] |
Zhang S, Shen Q, Nie C, et al. (2019) Hyperspectral inversion of heavy metal content in reclaimed soil from a mining wasteland based on different spectral transformation and modeling methods. Spectrochim Acta Part A 211: 393-400. doi: 10.1016/j.saa.2018.12.032
![]() |
[97] |
Hu J, Peng J, Zhou Y, et al. (2019) Quantitative estimation of soil salinity using UAV-borne hyperspectral and satellite multispectral images. Remote Sens 11: 736. doi: 10.3390/rs11070736
![]() |
[98] |
Grafton M, Kaul T, Palmer A, et al. (2019) Regression analysis of proximal hyperspectral data to predict soil pH and Olsen P. Agriculture 9: 55. doi: 10.3390/agriculture9030055
![]() |
[99] | Patel AK, Ghosh JK (2019) Soil fertility status assessment using hyperspectral remote sensing, Remote sensing for agriculture, Ecosystems, and Hydrology XXI, 111490E. |
[100] |
Gold KM, Townsend PA, Chlus A, et al. (2020) Hyperspectral measurements enable pre-symptomatic detection and differentiation of contrasting physiological effects of late blight and early blight in potato. Remote Sens 12: 286. doi: 10.3390/rs12020286
![]() |
[101] | Gorretta N, Nouri M, Herrero A, et al. (2019) Early detection of the fungal disease "apple scab" using SWIR hyperspectral imaging, 2019 10th workshop on hyperspectral imaging and signal processing: Evolution in remote sensing (WHISPERS), 1-4. |
[102] |
Mahlein AK, Alisaac E, Al Masri A, et al. (2019) Comparison and combination of thermal, fluorescence, and hyperspectral imaging for monitoring Fusarium head blight of wheat on spikelet scale. Sensors 19: 2281. doi: 10.3390/s19102281
![]() |
[103] | Yan Y, Yu W (2019) Early detection of rice blast (pyricularia) at seedling stage based on near-infrared hyper-spectral image, Proceedings of the 2019 8th international conference on bioinformatics and biomedical science, 64-68. |
[104] | Gustavsson J, Cederberg C, Sonesson U, et al. (2011) Global food losses and food waste. |
[105] |
Parfitt J, Barthel M, Macnaughton S (2010) Food waste within food supply chains: Quantification and potential for change to 2050. Philos Trans R Soc, B 365: 3065-3081. doi: 10.1098/rstb.2010.0126
![]() |
[106] |
Liu S, Liu X, Liu M, et al. (2017) Extraction of rice phenological differences under heavy metal stress using EVI time-series from HJ-1A/B Data. Sensors 17: 1243. doi: 10.3390/s17061243
![]() |
[107] |
Xing F, Yao H, Liu Y, et al. (2019) Recent developments and applications of hyperspectral imaging for rapid detection of mycotoxins and mycotoxigenic fungi in food products. Crit Rev Food Sci Nutr 59: 173-180. doi: 10.1080/10408398.2017.1363709
![]() |
[108] |
Liu T, Liu X, Liu M, et al. (2018) Classification of rice heavy metal stress levels based on phenological characteristics using remote sensing time-series images and data mining algorithms. Sensors 18: 4425. doi: 10.3390/s18124425
![]() |
[109] |
Jeong S, Ko J, Yeom J-M (2018) Nationwide projection of rice yield using a crop model integrated with geostationary satellite imagery: a case study in South Korea. Remote Sens 10: 1665. doi: 10.3390/rs10101665
![]() |
[110] |
Lu J, Miao Y, Shi W, et al. (2020) Developing a proximal active canopy sensor-based precision nitrogen management strategy for high-yielding rice. Remote Sens 12: 1440. doi: 10.3390/rs12091440
![]() |
[111] |
Paleari L, Movedi E, Vesely FM, et al. (2019) Estimating crop nutritional status using smart apps to support nitrogen fertilization. A case study on paddy rice. Sensors 19: 981. doi: 10.3390/s19040981
![]() |
[112] |
Park S, Im J, Park S, et al. (2018) Classification and mapping of paddy rice by combining Landsat and SAR time series data. Remote Sens 10: 447. doi: 10.3390/rs10030447
![]() |
[113] |
Ranghetti L, Cardarelli E, Boschetti M, et al. (2018) Assessment of water management changes in the Italian rice paddies from 2000 to 2016 using satellite data: A contribution to agro-ecological studies. Remote Sens 10: 416. doi: 10.3390/rs10030416
![]() |
[114] |
Sianturi R, Jetten VG, Ettema J, et al. (2018) Distinguishing between hazardous flooding and non-hazardous agronomic inundation in irrigated rice fields: A case study from west java. Remote Sens 10: 1003. doi: 10.3390/rs10071003
![]() |
[115] |
Zhang S, Li J, Wang S, et al. (2020) Repaid identification and prediction of Cadmium-Lead cross-stress of different stress levels in rice canopy based on visible and near-infrared spectroscopy. Remote Sens 12: 469. doi: 10.3390/rs12030469
![]() |
[116] |
Barnes PW, Searles PS, Ballaré CL, et al. (2000) Non-invasive measurements of leaf epidermal transmittance of UV radiation using chlorophyll fluorescence: field and laboratory studies. Physiol Plant 109: 274-283. doi: 10.1034/j.1399-3054.2000.100308.x
![]() |
[117] |
Du L, Shi S, Yang J, et al. (2016) Using different regression methods to estimate leaf nitrogen content in rice by fusing hyperspectral LiDAR data and laser-induced chlorophyll fluorescence data. Remote Sens 8: 526. doi: 10.3390/rs8060526
![]() |
[118] |
Du L, Yang J, Chen B, et al. (2020) Novel combined spectral indices derived from hyperspectral and laser-induced fluorescence LiDAR spectra for leaf nitrogen contents estimation of rice. Remote Sens 12: 185. doi: 10.3390/rs12010185
![]() |
[119] | Yang J, Du L, Sun J, et al. (2016) Estimating the leaf nitrogen content of paddy rice by using the combined reflectance and laser-induced fluorescence spectra. Opt Express 24: 19354-19365. |
[120] |
Yang J, Song S, Du L, et al. (2018) Analyzing the effect of fluorescence characteristics on leaf nitrogen concentration estimation. Remote Sens 10: 1402. doi: 10.3390/rs10091402
![]() |
[121] | Fowler JE (2014) Compressive pushbroom and whiskbroom sensing for hyperspectral remote-sensing imaging, 2014 IEEE international conference on image processing (ICIP), 684-688. |
[122] | Kerekes JP, Schott JR (2007) Hyperspectral imaging systems. Hyperspectral data exploitation: Theory and applications, 19-45. |
[123] | Iturrino C, Arias FX, Sierra H, et al. (2019) Single-shot multispectral image acquisition for low-altitude remote sensing using light diffraction techniques, 2019 10th workshop on hyperspectral imaging and signal processing: Evolution in remote sensing (WHISPERS), 1-5. |
[124] |
Blasinski H, Farrell J, Wandell B (2020) Simultaneous surface reflectance and fluorescence spectra estimation. IEEE Trans Image Process 29: 8791-8804. doi: 10.1109/TIP.2020.2973810
![]() |
[125] |
He X, Feng X, Sun D, et al. (2019) Rapid and nondestructive measurement of rice seed vitality of different years using near-infrared hyperspectral imaging. Molecules 24: 2227. doi: 10.3390/molecules24122227
![]() |
[126] | Yuan J, Su Z, Jia Y, et al. (2016) Identification of rice leaf blast and nitrogen deficiency in cold region using hyperspectral imaging. Trans Chin Soc Agric Eng 32: 155-160. |
[127] | Gewali UB, Monteiro ST (2016) A novel covariance function for predicting vegetation biochemistry from hyperspectral imagery with Gaussian processes, 2016 IEEE international conference on image processing (ICIP), 2216-2220. |
[128] | Kampe T, Leisso N, Musinsky J, et al. (2013) The NEON 2013 airborne campaign at domain 17 terrestrial and aquatic sites in California. NEON Technical Memorandum Series, TM-005. |
[129] |
Ding M, Guan Q, Li L, et al. (2020) Phenology-based rice paddy mapping using multi-source satellite imagery and a fusion algorithm applied to the poyang lake plain, Southern China. Remote Sens 12: 1022. doi: 10.3390/rs12061022
![]() |
[130] |
Du L, Gong W, Shi S, et al. (2016) Estimation of rice leaf nitrogen contents based on hyperspectral LIDAR. Int J Appl Earth Obs Geoinformation 44: 136-143. doi: 10.1016/j.jag.2015.08.008
![]() |
[131] |
Gnyp ML, Miao Y, Yuan F, et al. (2014) Hyperspectral canopy sensing of paddy rice aboveground biomass at different growth stages. Field Crops Res 155: 42-55. doi: 10.1016/j.fcr.2013.09.023
![]() |
[132] |
Cheng T, Song R, Li D, et al. (2017) Spectroscopic estimation of biomass in canopy components of paddy rice using dry matter and chlorophyll indices. Remote Sens 9: 319. doi: 10.3390/rs9040319
![]() |
[133] |
Li D, Wang X, Zheng H, et al. (2018) Estimation of area-and mass-based leaf nitrogen contents of wheat and rice crops from water-removed spectra using continuous wavelet analysis. Plant Methods 14: 76. doi: 10.1186/s13007-018-0344-1
![]() |
[134] |
Liu Z, Shi J, Zhang L, et al. (2010) Discrimination of rice panicles by hyperspectral reflectance data based on principal component analysis and support vector classification. J Zhejiang Univ Sci B 11: 71-78. doi: 10.1631/jzus.B0900193
![]() |
[135] |
Zheng H, Ma J, Zhou M, et al. (2020) Enhancing the nitrogen signals of rice canopies across critical growth stages through the integration of textural and spectral information from unmanned aerial vehicle (UAV) multispectral imagery. Remote Sens 12: 957. doi: 10.3390/rs12060957
![]() |
[136] | Starr C, Evers C, Starr L (2010) Biology: Concepts and applications, Cengage Learning. |
[137] |
Das B, Sahoo RN, Biswas A, et al. (2020) Discrimination of rice genotypes using field spectroradiometry. Geocarto Int 35: 64-77. doi: 10.1080/10106049.2018.1506507
![]() |
[138] |
Yang M-D, Huang K-S, Kuo Y-H, et al. (2017) Spatial and spectral hybrid image classification for rice lodging assessment through UAV imagery. Remote Sens 9: 583. doi: 10.3390/rs9060583
![]() |
[139] |
Yang C-M (2010) Assessment of the severity of bacterial leaf blight in rice using canopy hyperspectral reflectance. Precis Agric 11: 61-81. doi: 10.1007/s11119-009-9122-4
![]() |
[140] |
Dunn BW, Dehaan R, Schmidtke L, et al. (2016) Using field-derived hyperspectral reflectance measurement to identify the essential wavelengths for predicting nitrogen uptake of rice at panicle initiation. J Near Infrared Spectrosc 24: 473-483. doi: 10.1255/jnirs.1246
![]() |
[141] |
Feng H, Jiang N, Huang C, et al. (2013) A hyperspectral imaging system for an accurate prediction of the above-ground biomass of individual rice plants. Rev Sci Instrum 84: 095107. doi: 10.1063/1.4818918
![]() |
[142] |
Kawamura K, Ikeura H, Phongchanmaixay S, et al. (2018) Canopy hyperspectral sensing of paddy fields at the booting stage and PLS regression can assess grain yield. Remote Sens 10: 1249. doi: 10.3390/rs10081249
![]() |
[143] |
Fabiyi SD, Vu H, Tachtatzis C, et al. (2020) Varietal classification of rice seeds using RGB and hyperspectral images. IEEE Access 8: 22493-22505. doi: 10.1109/ACCESS.2020.2969847
![]() |
[144] |
Sun J, Shi S, Yang J, et al. (2018) Estimating leaf chlorophyll status using hyperspectral lidar measurements by PROSPECT model inversion. Remote Sens Environ 212: 1-7. doi: 10.1016/j.rse.2018.04.024
![]() |
[145] |
Kong W, Zhang C, Liu F, et al. (2013) Rice seed cultivar identification using near-infrared hyperspectral imaging and multivariate data analysis. Sensors 13: 8916-8927. doi: 10.3390/s130708916
![]() |
[146] |
Devia CA, Rojas JP, Petro E, et al. (2019) High-throughput biomass estimation in rice crops using UAV multispectral imagery. J Intell Rob Syst 96: 573-589. doi: 10.1007/s10846-019-01001-5
![]() |
[147] |
Zhao X, Yuan Y, Song M, et al. (2019) Use of unmanned aerial vehicle imagery and deep learning unet to extract rice lodging. Sensors 19: 3859. doi: 10.3390/s19183859
![]() |
[148] | Zheng H, Zhou X, Cheng T, et al. (2016) Evaluation of a UAV-based hyperspectral frame camera for monitoring the leaf nitrogen concentration in rice, 2016 IEEE international geoscience and remote sensing symposium (IGARSS), 7350-7353. |
[149] |
Zhang Y, Gao J, Cen H, et al. (2019) Automated spectral feature extraction from hyperspectral images to differentiate weedy rice and barnyard grass from a rice crop. Comput Electron Agric 159: 42-49. doi: 10.1016/j.compag.2019.02.018
![]() |
[150] | Witt C, Pasuquin J, Mutters R, et al. (2005) New leaf color chart for effective nitrogen management in rice. Better Crops 89: 36-39. |
[151] |
Sun J, Shi S, Gong W, et al. (2017) Evaluation of hyperspectral LiDAR for monitoring rice leaf nitrogen by comparison with multispectral LiDAR and passive spectrometer. Sci Rep 7: 40362. doi: 10.1038/srep40362
![]() |
[152] |
Zhou W, Zhang J, Zou M, et al. (2019) Feasibility of using rice leaves hyperspectral data to estimate CaCl 2-extractable concentrations of heavy metals in agricultural soil. Sci Rep 9: 1-9. doi: 10.1038/s41598-018-37186-2
![]() |
[153] |
Uto K, Seki H, Saito G, et al. (2013) Characterization of rice paddies by a UAV-mounted miniature hyperspectral sensor system. IEEE J Sel Top Appl Earth Obs Remote Sens 6: 851-860. doi: 10.1109/JSTARS.2013.2250921
![]() |
[154] |
Inoue Y, Penuelas J (2001) An AOTF-based hyperspectral imaging system for field use in ecophysiological and agricultural applications. Int J Remote Sens 22: 3883-3888. doi: 10.1080/01431160110069863
![]() |
[155] |
Li D, Wang R, Xie C, et al. (2020) A recognition method for rice plant diseases and pests video detection based on deep convolutional neural network. Sensors 20: 578. doi: 10.3390/s20030578
![]() |
[156] |
Liu Z, Zhang Q, Han T, et al. (2016) Heavy metal pollution in a soil-rice system in the Yangtze river region of China. Int J Environ Res Pub Health 13: 63. doi: 10.3390/ijerph13010063
![]() |
[157] | Shwetank JK, Bhatia K (2010) Review of rice crop identification and classification using hyper-spectral image processing system. Int J Comput Sci Commun 1: 253-258. |
[158] |
Onoyama H, Ryu C, Suguri M, et al. (2018) Estimation of rice protein content before harvest using ground-based hyperspectral imaging and region of interest analysis. Precis Agric 19: 721-734. doi: 10.1007/s11119-017-9552-3
![]() |
[159] |
Nagelkerke NJ, others (1991) A note on a general definition of the coefficient of determination. Biometrika 78: 691-692. doi: 10.1093/biomet/78.3.691
![]() |
[160] |
Samat A, Gamba P, Abuduwaili J, et al. (2016) Geodesic flow kernel support vector machine for hyperspectral image classification by unsupervised subspace feature transfer. Remote Sens 8: 234. doi: 10.3390/rs8030234
![]() |
[161] |
Tehrany MS, Pradhan B, Mansor S, et al. (2015) Flood susceptibility assessment using GIS-based support vector machine model with different kernel types. Catena 125: 91-101. doi: 10.1016/j.catena.2014.10.017
![]() |
[162] |
Liu P, Choo K-KR, Wang L, et al. (2017) SVM or deep learning? A comparative study on remote sensing image classification. Soft Comput 21: 7053-7065. doi: 10.1007/s00500-016-2247-2
![]() |
[163] | Hecht-Nielsen R (1992) Theory of the backpropagation neural network, Neural networks for perception, Elsevier, 65-93. |
[164] |
Chen Y, Jiang H, Li C, et al. (2016) Deep feature extraction and classification of hyperspectral images based on convolutional neural networks. IEEE Trans Geosci Remote Sens 54: 6232-6251. doi: 10.1109/TGRS.2016.2584107
![]() |
[165] |
Wang J, Zhang C, Shi Y, et al. (2020) Evaluation of quinclorac toxicity and alleviation by salicylic acid in rice seedlings using ground-based visible/near-infrared hyperspectral imaging. Plant Methods 16: 1-16. doi: 10.1186/s13007-019-0534-5
![]() |
[166] |
Thenkabail PS, Enclona EA, Ashton MS, et al. (2004) Accuracy assessments of hyperspectral waveband performance for vegetation analysis applications. Remote Sens Environ 91: 354-376. doi: 10.1016/j.rse.2004.03.013
![]() |
[167] | Chang YL, Chang L, Xu M-X, et al. (2017) Impurity function band prioritization based on particle swarm optimization and gravitational search algorithm for hyperspectral images, 2017 IEEE international geoscience and remote sensing symposium (IGARSS), 1788-1791. |
[168] |
Knyazikhin Y, Schull MA, Stenberg P, et al. (2013) Hyperspectral remote sensing of foliar nitrogen content. Proc the Natl Acad Sci 110: E185-E192. doi: 10.1073/pnas.1210196109
![]() |
1. | Nik Norasma Che’Ya, Nur Adibah Mohidem, Nor Athirah Roslin, Mohammadmehdi Saberioon, Mohammad Zakri Tarmidi, Jasmin Arif Shah, Wan Fazilah Fazlil Ilahi, Norsida Man, Mobile Computing for Pest and Disease Management Using Spectral Signature Analysis: A Review, 2022, 12, 2073-4395, 967, 10.3390/agronomy12040967 | |
2. | Prabira Kumar Sethy, Chanki Pandey, Yogesh Kumar Sahu, Santi Kumari Behera, Hyperspectral imagery applications for precision agriculture - a systemic survey, 2022, 81, 1380-7501, 3005, 10.1007/s11042-021-11729-8 | |
3. | Amin Taheri-Garavand, Amin Nasiri, Dimitrios Fanourakis, Soodabeh Fatahi, Mahmoud Omid, Nikolaos Nikoloudakis, Automated In Situ Seed Variety Identification via Deep Learning: A Case Study in Chickpea, 2021, 10, 2223-7747, 1406, 10.3390/plants10071406 | |
4. | Ana M. Cavaco, Andrei B. Utkin, Jorge Marques da Silva, Rui Guerra, Making Sense of Light: The Use of Optical Spectroscopy Techniques in Plant Sciences and Agriculture, 2022, 12, 2076-3417, 997, 10.3390/app12030997 | |
5. | Javier E. Sánchez-Galán, Fatima Rangel Barranco, Jorge Serrano Reyes, Evelyn I. Quirós-McIntire, José Ulises Jiménez, José R. Fábrega, Using Supervised Classification Methods for the Analysis of Multi-spectral Signatures of Rice Varieties in Panama, 2021, 6, 24156698, 552, 10.25046/aj060262 | |
6. | To-Chia Ting, Augusto C. M. Souza, Rachel K. Imel, Carmela R. Guadagno, Chris Hoagland, Yang Yang, Diane R. Wang, Quantifying physiological trait variation with automated hyperspectral imaging in rice, 2023, 14, 1664-462X, 10.3389/fpls.2023.1229161 | |
7. | Mohan Singh, Manoj Joshi, Kapil D. Tyagi, Vaibhav B. Tyagi, 2024, 9781394216116, 181, 10.1002/9781394216147.ch10 | |
8. | Rohini Maram, Sathishkumar Samiappan, Seung-Chul Yoon, 2024, Detection and Classification of Plastic Contaminants on Chicken Meat using Color and Hyperspectral Imaging, 979-8-3503-6032-5, 9396, 10.1109/IGARSS53475.2024.10641396 | |
9. | Rodrigo Louzada Campos, Seung-Chul Yoon, Soo Chung, Suchendra M. Bhandarkar, Semisupervised Deep Learning for the Detection of Foreign Materials on Poultry Meat with Near-Infrared Hyperspectral Imaging, 2023, 23, 1424-8220, 7014, 10.3390/s23167014 | |
10. | Perez Mukasa, Dennis Semyalo, Mohammad Akbar Faqeerzada, Hangi Kim, Hyun Jung Min, Moon S. Kim, Insuck Baek, Isa Kabenge, Byoung-Kwan Cho, Deep learning application for real-time gravity-assisted seed conveying system for watermelon seeds purity sorting, 2024, 221, 01681699, 108974, 10.1016/j.compag.2024.108974 | |
11. | Árpád Székely, Tímea Szalóki, Mihály Jancsó, János Pauk, Csaba Lantos, Temporal Changes of Leaf Spectral Properties and Rapid Chlorophyll—A Fluorescence under Natural Cold Stress in Rice Seedlings, 2023, 12, 2223-7747, 2415, 10.3390/plants12132415 | |
12. | Girish Gopinath, U. Surendran, J. Vishak, Nimmi Sasidharan, Muhamed Fasil CT, Hyperspectral data and vegetative indices for paddy: A case study in Kerala, India, 2024, 33, 23529385, 101109, 10.1016/j.rsase.2023.101109 | |
13. | Shiyao Duan, Jiaojiao Li, Rui Song, Yunsong Li, Qian Du, Unmixing-Guided Convolutional Transformer for Spectral Reconstruction, 2023, 15, 2072-4292, 2619, 10.3390/rs15102619 | |
14. | Jing Hu, Tingting Li, Minghua Zhao, Fei Wang, Jiawei Ning, A Gated Content-Oriented Residual Dense Network for Hyperspectral Image Super-Resolution, 2023, 15, 2072-4292, 3378, 10.3390/rs15133378 | |
15. | Muhamad Noor Hazwan Abd Manaf, Abdul Shukor Juraimi, Mst. Motmainna, Nik Norasma Che’Ya, Ahmad Suhaizi Mat Su, Muhammad Huzaifah Mohd Roslim, Anuar Ahmad, Nisfariza Mohd Noor, Detection of Sedge Weeds Infestation in Wetland Rice Cultivation Using Hyperspectral Images and Artificial Intelligence: A Review, 2024, 32, 2231-8526, 1317, 10.47836/pjst.32.3.18 | |
16. | Riki Ridwana, Muhammad Kamal, Sanjiwana Arjasakusuma, Muh Fiqri Abdi Rabbi, M.H. Ibrahim, Evaluation of Machine Learning Models for Mapping Food Crops using Sentinel-2A Imagery in West Java, Indonesia, 2024, 600, 2267-1242, 03007, 10.1051/e3sconf/202460003007 | |
17. | Maja Matoša Kočar, Aleksandra Sudarić, Tomislav Duvnjak, Maja Mazur, Soybean Genotype-Specific Cold Stress and Priming Responses: Chlorophyll a Fluorescence and Pigment-Related Spectral Reflectance Indices as Tools for Breeding, 2025, 15, 2073-4395, 390, 10.3390/agronomy15020390 | |
18. | Fernando Arias, Maytee Zambrano, Edson Galagarza, Kathia Broce, Mapping Harmful Algae Blooms: The Potential of Hyperspectral Imaging Technologies, 2025, 17, 2072-4292, 608, 10.3390/rs17040608 |
Sensor type | Commercial name | Studies |
Sensors that measure radiance or irradiance, as well as transmittance and reflectance. Usually portable devices | ASD QualitySpec Pro 350-2800 nm | [130,131] |
ASD FieldSpec Pro Spectrometer, 350-2500 nm | [36,132,133,134,135,136] | |
ASD FieldSpec 3 Spectroradiometer, 350-2500 nm | [75,96,137] | |
ASD Field Spec 2 | [11,138] | |
ASD FieldSpec-FR (350-2500 nm) | [48,139] | |
GER-2600 Spectroradiometer, 330-2600 nm | [103] | |
GER1500 Spectroradiometer, 300-1100 nm | [23] | |
HR-1024 Spectroradiometer, 350-2500 nm | [140] | |
Portable ground MSR16 Radiometer Cropscan, 450-1750 nm | [76] | |
Hyperspec HEADWALL | [10,11,141] | |
MS-720 Spectroradiometer, 350-1050 nm | [142] | |
LI-1800 Spectroradiometer, 380-1100 nm | [48,49] | |
Sensors based on a spectrograph + CCD system/optic | ImSpector V10E Spectrograph, 390-1050 nm | [35,90,119,136,143,144] |
ImSpector N17E Spectrograph, 874-1734 nm | [58,125,145] | |
SP2500i Spectrograph | [118,119] | |
Multispectral systems with incorporated filters | Tetra CAM: ADC Micro, ADC Lite system, MiniMCA | [11,74,146,147,148] |
Sensors with onboard DSP software for parameter estimation | SPAD-502 Chlorophyll Meter, Measure the leaf transmittance in two wave bands: 400-500 nm, 600-700 nm | [46,126] |
Dualex 4 Fluorescence Sensor | [46] |
Study | Region | Rice species | Inferred magnitude | Acquisition | Spectral region |
[146] | Colombia | Indica IR64, Line 23 | Above Ground Biomass | Unmanned Aerial Vehicle (UAV) | 520-690 nm, 760-900 nm |
[131] | Sanjiang Plain, China | Kongyu | Above Ground Biomass | Manual | 350-1800 nm |
[141] | China | 152 Cultivars | Above Ground Biomass | Manual | 400-1000 nm |
[132] | Jiangsu, China | Yliangyou 1, Wuyunjing 24 | Above Ground Biomass | Manual | 350-2500 nm |
[112] | South Korea/California | Not Specified (N/S) | Paddy Rice Landcover | Satellite | 430-1200 nm |
[114] | West Java, Indonesia | N/S | Paddy Rice Landcover | Satellite | 479-841 nm |
[32] | Hunan, China | N/S | Paddy Rice Landcover | Satellite | 430-1200 nm |
[33] | Rugao City, Juangsu, China | N/S | Plant Presence | UAV | 430-900 nm |
[149] | China | N/S | Plant Species | Manual | 415,561,687,705,735, 1007 nm |
[84] | Jiangsu, China | Wheat (Ningmai 13, Huaimai 33), Rice (Nanjing 9108, Lianjing 10) | Ratio Vegetation Index (RVI) | Manual | 730,815 nm |
[129] | Nanchang, China | N/S | Rice Paddy Presence | Satellite | 400-1200 nm |
Study | region | Rice species | Inferred magnitude | Acquisition | Spectral region |
[144] | China | Yongyou 4949 | Chlorophyll | N/S | 539-910 nm |
[137] | New Delhi, India | 13 Species | Chlorophyll | Manual | 690,700-750 nm, 940-1104 nm |
[153] | Japan | Haenuki and Koshihikari SD | Chlorophyll | UAV | 327-763 nm, 640-1050 nm |
[46] | Jiangsu, China | Oryza sativa L. | Chlorophyll | Automated Ground System | 360-1025 nm |
[31] | Jiangsu, China. | Ningjing-4, Wuyunjing-24 | LNC | Manual | 375,710,850 nm |
[120] | Hubei, China | Yangliangyou 6 | LNC | Manual | 355,460,556 nm |
[118] | Jianghan Plain, China | Yongyou 4949, Yangliangyou 6 | LNC | Manual | 360-800 nm |
[133] | Jiangsu, China | Yliangyou 1, Wuyunjing 24 | LNC | Manual | 350-2500 nm |
[154] | N/S | N/S | LNC | Manual | 450-900 nm |
[74] | Jiangsu, China | Wuyunjing24, Yliangyou1 | LNC/Plant Nitrogen Accumulation (PNA) | UAV | 490-900 nm |
[148] | Juangsu, China | N/S | N Content | UAV | 450-950 nm |
[151] | China | Yongyou4949, Yangliangyou 6 | N Content | Manual | 538-910 nm |
[117] | China | N/S | N Content | Manual | 450-740 nm |
[49] | Taiwan | Tainung 67 | N Content | Manual | 400-1100 nm |
[76] | Nanjing, China | Wuxiangjing 9, Nipponbare, Huajing 2 | N Content | Manual | 610,660,680 nm |
[119] | Hubei, China | Yongyou4949, Yangliangyou 6 | N Content | Manual | 360-800 nm |
[110] | Heilongjiang, China | Longjing 31, Longjing 21 | N Content | Manual | 670,730,780 nm |
[135] | Jiangsu, China | Y Liangyou 1, Wuyunjing24 | N Content | UAV | 490,550,680,720,800 nm |
[48] | China, Japan | Tsugaru Roman | N Content | Manual | 350-2500 nm |
[130] | Suizhou City, China | Japonica, non-glutinous Rice | N Content | Lab | 538-910 nm |
[140] | Southern NSW, Australia | Reiziq, Sherpa | N Uptake | Manual | 350-2500 nm |
[23] | Suwon, Korea | Hwasungbyeo, Dasanbyeo, Juanbyeo, SNU-SG1 | N, Chlorophyll | Manual | 520-600 nm |
[130] | China | Japonica and non-glutinous rice | N, Chlorophyll | Manual | 538-910 nm |
[75] | New Delhi, India | PRH-10 | N, Phosphorus, Sulphur concentration | Manual | 350-2500 nm |
[111] | Rosasco, Gaggiano | 43 Cultivars | PNA | Manual | 450-700 nm |
Study | Region | Rice species | Inferred magnitude | Acquisition | Spectral region |
[126] | Harbin, China | N/S | Disease Presence | Manual | 560,620,670 nm |
[103] | N/S | Nipponbare | Disease Presence | Manual | 900-1700 nm |
[139] | Wufeng, Taiwan | TNG 67, TCS 10 | Disease Presence | Manual | 350-2500 nm |
[155] | Anhui, Jiangxi and Hunan Province, China | N/S | Disease Presence | Manual | N/S |
[34] | Arkansas, USA | TIL 654.13 | Disease Presence | Manual | 480,760,800, 1000 nm |
[36] | Jiangsu, China | Sanyou 63 | Leaf Pest Damage | Manual | 400-1000 nm |
[35] | Hangzhou, China | Y Liangyou689 | Pest Presence | Manual | 380-1030 nm |
[115] | N/S | N/S | Heavy Metal Concentration | Manual | 350-2500 nm |
[76] | Suzhou, China | Oryza sativa | Heavy Metal Concentration | Manual | 420-980 nm |
[90] | China | Xiushi 134, Zhejing88 | Herbicide Toxicity | Manual | 380-1030 nm |
Study | Region | Rice species | Inferred magnitude | Acquisition | Spectral region |
[50] | Zhenjiang, China | N/S | Seed Origin | Manual | 499-950 nm |
[143] | Vietnam | 90 Cultivars | Seed quality: Shape, length, width and colour | Manual | 385-1000 nm |
[145] | China | Zhongzheyou No.1, 5, 8 and 86 | Seed Type | Manual | 1069-1558 nm |
[125] | China | N/S | Seed Vitality | Manual | 992, 1012, 1119, 1167, 1305, 1402, 1629, 1649 nm |
[142] | Laos | Oryza Sativa | Grain Yield | Manual | 710-740 nm, 830 nm |
[134] | Zhejiang, China | Xiushui 110 | Panicle Health | Manual | 350-2500 nm |
[158] | Kyoto, Japan | Kinu-Hikari | Protein Content | Manual | 400-1000 nm |
[47] | South Korea and China | N/S | Rice Geographical Origin | Manual | 400-1000 nm |
[147] | Heilongjiang, China | Longjing 32 | Rice Lodging Classification | UAV | 400-700 nm |
[138] | Taibao City, Taiwan | TaiKeng 2 | Rice Lodging Classification | UAV | VIS |
[109] | South Korea | N/S | Yield | Satellite | 400-890 nm |
Study | Processing method | Input variable | Output variable | Performance metric | Performance |
[137] | ANOVA/CART | Spectral Reflectance | Cultivar Species | Accuracy | 0.98 |
[126] | PCA-SVM | Spectral Reflectance | Rice Blast Presence | Accuracy | 0.975 |
[103] | PCA/Linear Discriminant Method | Spectral Reflectance | Rice Blast Presence | Accuracy | 0.92 |
[155] | Convolutional Neural Network | RGB Image | Pest Presence | Accuracy. | 70.0%-87.2% |
[34] | Sequential Forward Selection (SFS) | Spectral Reflectance | Disease Presence | Accuracy | 0.965 |
[165] | PCA + SVM | Spectral Reflectance | Quinclorac Presence | Accuracy | 0.9 |
[112] | Random Forest/SVM | Spatiotemporal Satellite Data | Paddy Rice Map | Accuracy | 98.67%/93.87% |
[114] | EVI Thresholding | Satellite Images | Hazardous Flooding Classification | Overall Accuracy | 0.7596 |
[32] | CNN | Satellite Images | Paddy Rice Map | R2 | 0.9945 |
[35] | SPA/Neural Network Method | Spectral Reflectance | Infestation Degree | Accuracy | 0.95 |
[149] | SPA + Weighted SVM | Spectral Reflectance | Crop Class | Accuracy | 0.97 |
[10] | Partial least square discriminant analysis (PLS-DA) | Spectral Reflectance | Rice Origin Classification | Accuracy | 0.99 |
[147] | Convolutional Neural Network | RGB + Multispectral Image | Rice Lodging Classification | Dice coefficient | 0.9284 |
[33] | Decision Tree | RGB, NIR, MS images | Rice Plant Presence | Overall Accuracy, Kappa Coefficient | 92.56%/0.86 |
[138] | Maximum Likelihood/Decision Tree Classification | Hybrid spatio-spectral image | Rice Lodging Classification | Accuracy | 0.9384 |
[129] | N/S | Spectral Reflectance | Rice Paddy Presence | Kappa Coefficient | 0.89 |
[50] | SVM | Spectral Reflectance | Seed Origin | Accuracy | 0.9167 |
[143] | LDA + RandomForest | Spectral Reflectance | Seed Variety | Precision | 0.9859 |
[145] | Random Forest | Spectral Reflectance | Cultivar Species | Accuracy | 1 |
[125] | PLSDA, LSSVM, ELM | Spectral Reflectance | Seed Viability | Accuracy | 0.9367 |
[113] | Linear Regression | Spatiotemporal Satellite Data | Cultivated Area | R2 | 0.94 |
Study | Processing method | Input variable | Output variable | Performance metric | Performance |
[146] | Multivariate regression | Multispectral NIR Images | Above Ground Biomass | Correlation | 0.76 |
[131] | Correlation Analysis | Spectral Reflectance | Above Ground Biomass | R2 | 0.77 |
[141] | Thresholding/Linear Regression Approach | Spectral Reflectance | Above GroundBiomass | R2 | 0.94 |
[132] | Linear and Nonlinear Regression | Spectral Reflectance | Above Ground Biomass | R2 | 0.82 |
[144] | SVM | Spectral Reflectance | Chlorophyll density | R2 | 0.55 |
[153] | TUI | Spectral Reflectance | Chlorophyll density | Correlation | 0.803 |
[152] | Linear Regression | Spectral Reflectance | Chlorophyll density | R2 | 0.84 (Sunlit Leaves), 0.9 (Shadowed Leaves) |
[139] | Multiple Linear Regression | Spectral Reflectance | Infestation Degree | R2 | 0.889 |
[142] | PLS Regression | Spectral Measurements | Estimated Yield | R2 | 0.873 |
[115] | PLS Regression | Spectral Reflectance | Cd-Pb Stress Level | R2 | 0.90 for Cd and 0.92 for Pb |
[76] | PLS Regression | Spectral Reflectance | Cd-Pb Concentration | R2 | 0.592, 0.013 |
[36] | Linear Regression | Spectral Reflectance | RLF Damage Severity | R2 | 0.987 |
[31] | Linear Regression | Spectral Reflectance | LNC | R2 | 0.84 |
[120] | Fully-Connected NN | Fluorescence Spectra | LNC | R2 | 0.867 |
[118] | Fully-Connected NN | Reflectance + Fluorescence Measurements | LNC | R2 | 0.81 |
[133] | Continuous Wavelet Analysis | Spectral Reflectance | LNC | R2 | 0.77 |
[154] | Multivariate regression | Spectral Reflectance | LNC | R2 | 0.72 |
[74] | Regression | RGB/MS/CIR Data | LNC / PNA | R2 | LNC 0.81. PNA 0.84 |
[148] | Linear Regression | Spectral Reflectance | LNC | R2 | 0.56 |
[151] | SVM | Spectral Reflectance | LNC | R2 | 0.73 |
[117] | SVM, BP-NN, RBF-NN | Spectral Reflectance and Fluorescence | LNC | R2 | 0.979 |
[49] | Correlation | Spectral Reflectance | LNC | R2 | 0.679 |
[24] | Color Visibility Analysis | RGB Image | N Fertilizer Level Needed | R2 | 0.998 |
[152] | Linear and Nonlinear Correlation | Spectral Reflectance | LNC | R2 | 0.78 |
[119] | PCA/Neural Network Method | Spectral Reflectance and Fluorescence | LNC | R2 | 0.912 |
[110] | Linear Regression | Spectral Reflectance | LNC | R2 | Elongation stage:0.68; Heading stage:0.85 |
[135] | Stepwise Multiple Linear Regression (SMLR) | Spectral Reflectance | LNC, PNA | R2 | LNC: 0.20, PNA:0.73 |
[48] | PLS Regression | Spectral Reflectance | Canopy N Content | R2 | 0.917 |
[130] | SVM | Hyperspectral LIDAR Measurements | LNC | R2 | 0.75 |
[140] | PLS Regression | Spectral Reflectance | LNC | R2 | 0.84 |
[23] | Linear Regression | Spectral Reflectance | LNC | R2 | 0.84 |
[130] | SVM, MLP-NN, RBF-NN | Spectral Reflectance | LNC | R2 | 0.75 |
[75] | Correlation Analysis | Spectral Reflectance | N, Phosphorus, Sulphur | R2 | N 0.80, P 0.69, S 0.73 |
[134] | PCA + SVM | Spectral Reflectance | Panicle Health | Accuracy | 0.9914 |
[111] | Linear regression, K-means Clustering | RGB Image | PNA | R2 | 0.95 |
[158] | PLS Regression | Spectral Reflectance | Protein content | R2 | 0.76 |
[84] | Linear Regression | Spectral Reflectance | RVI | R2 | 0.81 |
[109] | GRAMI | Landcover/Spectral Reflectance | Rice Yield | Overall Accuracy | 89.5%-90.2% |
Sensor type | Commercial name | Studies |
Sensors that measure radiance or irradiance, as well as transmittance and reflectance. Usually portable devices | ASD QualitySpec Pro 350-2800 nm | [130,131] |
ASD FieldSpec Pro Spectrometer, 350-2500 nm | [36,132,133,134,135,136] | |
ASD FieldSpec 3 Spectroradiometer, 350-2500 nm | [75,96,137] | |
ASD Field Spec 2 | [11,138] | |
ASD FieldSpec-FR (350-2500 nm) | [48,139] | |
GER-2600 Spectroradiometer, 330-2600 nm | [103] | |
GER1500 Spectroradiometer, 300-1100 nm | [23] | |
HR-1024 Spectroradiometer, 350-2500 nm | [140] | |
Portable ground MSR16 Radiometer Cropscan, 450-1750 nm | [76] | |
Hyperspec HEADWALL | [10,11,141] | |
MS-720 Spectroradiometer, 350-1050 nm | [142] | |
LI-1800 Spectroradiometer, 380-1100 nm | [48,49] | |
Sensors based on a spectrograph + CCD system/optic | ImSpector V10E Spectrograph, 390-1050 nm | [35,90,119,136,143,144] |
ImSpector N17E Spectrograph, 874-1734 nm | [58,125,145] | |
SP2500i Spectrograph | [118,119] | |
Multispectral systems with incorporated filters | Tetra CAM: ADC Micro, ADC Lite system, MiniMCA | [11,74,146,147,148] |
Sensors with onboard DSP software for parameter estimation | SPAD-502 Chlorophyll Meter, Measure the leaf transmittance in two wave bands: 400-500 nm, 600-700 nm | [46,126] |
Dualex 4 Fluorescence Sensor | [46] |
Study | Region | Rice species | Inferred magnitude | Acquisition | Spectral region |
[146] | Colombia | Indica IR64, Line 23 | Above Ground Biomass | Unmanned Aerial Vehicle (UAV) | 520-690 nm, 760-900 nm |
[131] | Sanjiang Plain, China | Kongyu | Above Ground Biomass | Manual | 350-1800 nm |
[141] | China | 152 Cultivars | Above Ground Biomass | Manual | 400-1000 nm |
[132] | Jiangsu, China | Yliangyou 1, Wuyunjing 24 | Above Ground Biomass | Manual | 350-2500 nm |
[112] | South Korea/California | Not Specified (N/S) | Paddy Rice Landcover | Satellite | 430-1200 nm |
[114] | West Java, Indonesia | N/S | Paddy Rice Landcover | Satellite | 479-841 nm |
[32] | Hunan, China | N/S | Paddy Rice Landcover | Satellite | 430-1200 nm |
[33] | Rugao City, Juangsu, China | N/S | Plant Presence | UAV | 430-900 nm |
[149] | China | N/S | Plant Species | Manual | 415,561,687,705,735, 1007 nm |
[84] | Jiangsu, China | Wheat (Ningmai 13, Huaimai 33), Rice (Nanjing 9108, Lianjing 10) | Ratio Vegetation Index (RVI) | Manual | 730,815 nm |
[129] | Nanchang, China | N/S | Rice Paddy Presence | Satellite | 400-1200 nm |
Study | region | Rice species | Inferred magnitude | Acquisition | Spectral region |
[144] | China | Yongyou 4949 | Chlorophyll | N/S | 539-910 nm |
[137] | New Delhi, India | 13 Species | Chlorophyll | Manual | 690,700-750 nm, 940-1104 nm |
[153] | Japan | Haenuki and Koshihikari SD | Chlorophyll | UAV | 327-763 nm, 640-1050 nm |
[46] | Jiangsu, China | Oryza sativa L. | Chlorophyll | Automated Ground System | 360-1025 nm |
[31] | Jiangsu, China. | Ningjing-4, Wuyunjing-24 | LNC | Manual | 375,710,850 nm |
[120] | Hubei, China | Yangliangyou 6 | LNC | Manual | 355,460,556 nm |
[118] | Jianghan Plain, China | Yongyou 4949, Yangliangyou 6 | LNC | Manual | 360-800 nm |
[133] | Jiangsu, China | Yliangyou 1, Wuyunjing 24 | LNC | Manual | 350-2500 nm |
[154] | N/S | N/S | LNC | Manual | 450-900 nm |
[74] | Jiangsu, China | Wuyunjing24, Yliangyou1 | LNC/Plant Nitrogen Accumulation (PNA) | UAV | 490-900 nm |
[148] | Juangsu, China | N/S | N Content | UAV | 450-950 nm |
[151] | China | Yongyou4949, Yangliangyou 6 | N Content | Manual | 538-910 nm |
[117] | China | N/S | N Content | Manual | 450-740 nm |
[49] | Taiwan | Tainung 67 | N Content | Manual | 400-1100 nm |
[76] | Nanjing, China | Wuxiangjing 9, Nipponbare, Huajing 2 | N Content | Manual | 610,660,680 nm |
[119] | Hubei, China | Yongyou4949, Yangliangyou 6 | N Content | Manual | 360-800 nm |
[110] | Heilongjiang, China | Longjing 31, Longjing 21 | N Content | Manual | 670,730,780 nm |
[135] | Jiangsu, China | Y Liangyou 1, Wuyunjing24 | N Content | UAV | 490,550,680,720,800 nm |
[48] | China, Japan | Tsugaru Roman | N Content | Manual | 350-2500 nm |
[130] | Suizhou City, China | Japonica, non-glutinous Rice | N Content | Lab | 538-910 nm |
[140] | Southern NSW, Australia | Reiziq, Sherpa | N Uptake | Manual | 350-2500 nm |
[23] | Suwon, Korea | Hwasungbyeo, Dasanbyeo, Juanbyeo, SNU-SG1 | N, Chlorophyll | Manual | 520-600 nm |
[130] | China | Japonica and non-glutinous rice | N, Chlorophyll | Manual | 538-910 nm |
[75] | New Delhi, India | PRH-10 | N, Phosphorus, Sulphur concentration | Manual | 350-2500 nm |
[111] | Rosasco, Gaggiano | 43 Cultivars | PNA | Manual | 450-700 nm |
Study | Region | Rice species | Inferred magnitude | Acquisition | Spectral region |
[126] | Harbin, China | N/S | Disease Presence | Manual | 560,620,670 nm |
[103] | N/S | Nipponbare | Disease Presence | Manual | 900-1700 nm |
[139] | Wufeng, Taiwan | TNG 67, TCS 10 | Disease Presence | Manual | 350-2500 nm |
[155] | Anhui, Jiangxi and Hunan Province, China | N/S | Disease Presence | Manual | N/S |
[34] | Arkansas, USA | TIL 654.13 | Disease Presence | Manual | 480,760,800, 1000 nm |
[36] | Jiangsu, China | Sanyou 63 | Leaf Pest Damage | Manual | 400-1000 nm |
[35] | Hangzhou, China | Y Liangyou689 | Pest Presence | Manual | 380-1030 nm |
[115] | N/S | N/S | Heavy Metal Concentration | Manual | 350-2500 nm |
[76] | Suzhou, China | Oryza sativa | Heavy Metal Concentration | Manual | 420-980 nm |
[90] | China | Xiushi 134, Zhejing88 | Herbicide Toxicity | Manual | 380-1030 nm |
Study | Region | Rice species | Inferred magnitude | Acquisition | Spectral region |
[50] | Zhenjiang, China | N/S | Seed Origin | Manual | 499-950 nm |
[143] | Vietnam | 90 Cultivars | Seed quality: Shape, length, width and colour | Manual | 385-1000 nm |
[145] | China | Zhongzheyou No.1, 5, 8 and 86 | Seed Type | Manual | 1069-1558 nm |
[125] | China | N/S | Seed Vitality | Manual | 992, 1012, 1119, 1167, 1305, 1402, 1629, 1649 nm |
[142] | Laos | Oryza Sativa | Grain Yield | Manual | 710-740 nm, 830 nm |
[134] | Zhejiang, China | Xiushui 110 | Panicle Health | Manual | 350-2500 nm |
[158] | Kyoto, Japan | Kinu-Hikari | Protein Content | Manual | 400-1000 nm |
[47] | South Korea and China | N/S | Rice Geographical Origin | Manual | 400-1000 nm |
[147] | Heilongjiang, China | Longjing 32 | Rice Lodging Classification | UAV | 400-700 nm |
[138] | Taibao City, Taiwan | TaiKeng 2 | Rice Lodging Classification | UAV | VIS |
[109] | South Korea | N/S | Yield | Satellite | 400-890 nm |
Study | Processing method | Input variable | Output variable | Performance metric | Performance |
[137] | ANOVA/CART | Spectral Reflectance | Cultivar Species | Accuracy | 0.98 |
[126] | PCA-SVM | Spectral Reflectance | Rice Blast Presence | Accuracy | 0.975 |
[103] | PCA/Linear Discriminant Method | Spectral Reflectance | Rice Blast Presence | Accuracy | 0.92 |
[155] | Convolutional Neural Network | RGB Image | Pest Presence | Accuracy. | 70.0%-87.2% |
[34] | Sequential Forward Selection (SFS) | Spectral Reflectance | Disease Presence | Accuracy | 0.965 |
[165] | PCA + SVM | Spectral Reflectance | Quinclorac Presence | Accuracy | 0.9 |
[112] | Random Forest/SVM | Spatiotemporal Satellite Data | Paddy Rice Map | Accuracy | 98.67%/93.87% |
[114] | EVI Thresholding | Satellite Images | Hazardous Flooding Classification | Overall Accuracy | 0.7596 |
[32] | CNN | Satellite Images | Paddy Rice Map | R2 | 0.9945 |
[35] | SPA/Neural Network Method | Spectral Reflectance | Infestation Degree | Accuracy | 0.95 |
[149] | SPA + Weighted SVM | Spectral Reflectance | Crop Class | Accuracy | 0.97 |
[10] | Partial least square discriminant analysis (PLS-DA) | Spectral Reflectance | Rice Origin Classification | Accuracy | 0.99 |
[147] | Convolutional Neural Network | RGB + Multispectral Image | Rice Lodging Classification | Dice coefficient | 0.9284 |
[33] | Decision Tree | RGB, NIR, MS images | Rice Plant Presence | Overall Accuracy, Kappa Coefficient | 92.56%/0.86 |
[138] | Maximum Likelihood/Decision Tree Classification | Hybrid spatio-spectral image | Rice Lodging Classification | Accuracy | 0.9384 |
[129] | N/S | Spectral Reflectance | Rice Paddy Presence | Kappa Coefficient | 0.89 |
[50] | SVM | Spectral Reflectance | Seed Origin | Accuracy | 0.9167 |
[143] | LDA + RandomForest | Spectral Reflectance | Seed Variety | Precision | 0.9859 |
[145] | Random Forest | Spectral Reflectance | Cultivar Species | Accuracy | 1 |
[125] | PLSDA, LSSVM, ELM | Spectral Reflectance | Seed Viability | Accuracy | 0.9367 |
[113] | Linear Regression | Spatiotemporal Satellite Data | Cultivated Area | R2 | 0.94 |
Study | Processing method | Input variable | Output variable | Performance metric | Performance |
[146] | Multivariate regression | Multispectral NIR Images | Above Ground Biomass | Correlation | 0.76 |
[131] | Correlation Analysis | Spectral Reflectance | Above Ground Biomass | R2 | 0.77 |
[141] | Thresholding/Linear Regression Approach | Spectral Reflectance | Above GroundBiomass | R2 | 0.94 |
[132] | Linear and Nonlinear Regression | Spectral Reflectance | Above Ground Biomass | R2 | 0.82 |
[144] | SVM | Spectral Reflectance | Chlorophyll density | R2 | 0.55 |
[153] | TUI | Spectral Reflectance | Chlorophyll density | Correlation | 0.803 |
[152] | Linear Regression | Spectral Reflectance | Chlorophyll density | R2 | 0.84 (Sunlit Leaves), 0.9 (Shadowed Leaves) |
[139] | Multiple Linear Regression | Spectral Reflectance | Infestation Degree | R2 | 0.889 |
[142] | PLS Regression | Spectral Measurements | Estimated Yield | R2 | 0.873 |
[115] | PLS Regression | Spectral Reflectance | Cd-Pb Stress Level | R2 | 0.90 for Cd and 0.92 for Pb |
[76] | PLS Regression | Spectral Reflectance | Cd-Pb Concentration | R2 | 0.592, 0.013 |
[36] | Linear Regression | Spectral Reflectance | RLF Damage Severity | R2 | 0.987 |
[31] | Linear Regression | Spectral Reflectance | LNC | R2 | 0.84 |
[120] | Fully-Connected NN | Fluorescence Spectra | LNC | R2 | 0.867 |
[118] | Fully-Connected NN | Reflectance + Fluorescence Measurements | LNC | R2 | 0.81 |
[133] | Continuous Wavelet Analysis | Spectral Reflectance | LNC | R2 | 0.77 |
[154] | Multivariate regression | Spectral Reflectance | LNC | R2 | 0.72 |
[74] | Regression | RGB/MS/CIR Data | LNC / PNA | R2 | LNC 0.81. PNA 0.84 |
[148] | Linear Regression | Spectral Reflectance | LNC | R2 | 0.56 |
[151] | SVM | Spectral Reflectance | LNC | R2 | 0.73 |
[117] | SVM, BP-NN, RBF-NN | Spectral Reflectance and Fluorescence | LNC | R2 | 0.979 |
[49] | Correlation | Spectral Reflectance | LNC | R2 | 0.679 |
[24] | Color Visibility Analysis | RGB Image | N Fertilizer Level Needed | R2 | 0.998 |
[152] | Linear and Nonlinear Correlation | Spectral Reflectance | LNC | R2 | 0.78 |
[119] | PCA/Neural Network Method | Spectral Reflectance and Fluorescence | LNC | R2 | 0.912 |
[110] | Linear Regression | Spectral Reflectance | LNC | R2 | Elongation stage:0.68; Heading stage:0.85 |
[135] | Stepwise Multiple Linear Regression (SMLR) | Spectral Reflectance | LNC, PNA | R2 | LNC: 0.20, PNA:0.73 |
[48] | PLS Regression | Spectral Reflectance | Canopy N Content | R2 | 0.917 |
[130] | SVM | Hyperspectral LIDAR Measurements | LNC | R2 | 0.75 |
[140] | PLS Regression | Spectral Reflectance | LNC | R2 | 0.84 |
[23] | Linear Regression | Spectral Reflectance | LNC | R2 | 0.84 |
[130] | SVM, MLP-NN, RBF-NN | Spectral Reflectance | LNC | R2 | 0.75 |
[75] | Correlation Analysis | Spectral Reflectance | N, Phosphorus, Sulphur | R2 | N 0.80, P 0.69, S 0.73 |
[134] | PCA + SVM | Spectral Reflectance | Panicle Health | Accuracy | 0.9914 |
[111] | Linear regression, K-means Clustering | RGB Image | PNA | R2 | 0.95 |
[158] | PLS Regression | Spectral Reflectance | Protein content | R2 | 0.76 |
[84] | Linear Regression | Spectral Reflectance | RVI | R2 | 0.81 |
[109] | GRAMI | Landcover/Spectral Reflectance | Rice Yield | Overall Accuracy | 89.5%-90.2% |