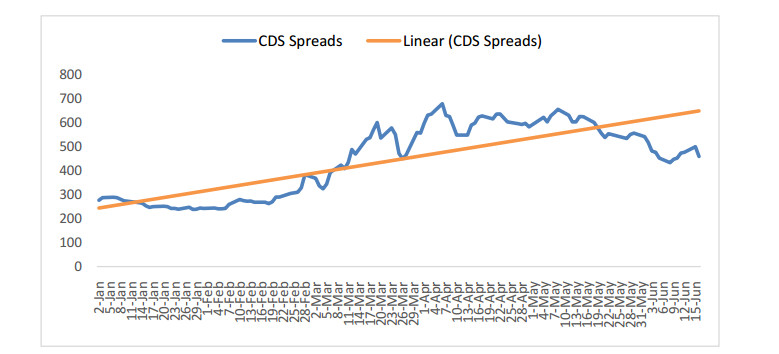
Citation: Mustafa Tevfik Kartal. The behavior of Sovereign Credit Default Swaps (CDS) spread: evidence from Turkey with the effect of Covid-19 pandemic[J]. Quantitative Finance and Economics, 2020, 4(3): 489-502. doi: 10.3934/QFE.2020022
[1] | Mustafa Tevfik Kartal, Özer Depren, Serpil Kılıç Depren . The determinants of main stock exchange index changes in emerging countries: evidence from Turkey in COVID-19 pandemic age. Quantitative Finance and Economics, 2020, 4(4): 526-541. doi: 10.3934/QFE.2020025 |
[2] | Selahattin Kaynak, Aykut Ekinci, Havvanur Feyza Kaya . The effect of COVID-19 pandemic on residential real estate prices: Turkish case. Quantitative Finance and Economics, 2021, 5(4): 623-639. doi: 10.3934/QFE.2021028 |
[3] | Raéf Bahrini, Assaf Filfilan . Impact of the novel coronavirus on stock market returns: evidence from GCC countries. Quantitative Finance and Economics, 2020, 4(4): 640-652. doi: 10.3934/QFE.2020029 |
[4] | Bharti, Ashish Kumar . Asymmetrical herding in cryptocurrency: Impact of COVID 19. Quantitative Finance and Economics, 2022, 6(2): 326-341. doi: 10.3934/QFE.2022014 |
[5] | Saeed Sazzad Jeris, Ridoy Deb Nath . Covid-19, oil price and UK economic policy uncertainty: evidence from the ARDL approach. Quantitative Finance and Economics, 2020, 4(3): 503-514. doi: 10.3934/QFE.2020023 |
[6] | Lorna Katusiime . Time-Frequency connectedness between developing countries in the COVID-19 pandemic: The case of East Africa. Quantitative Finance and Economics, 2022, 6(4): 722-748. doi: 10.3934/QFE.2022032 |
[7] | Nilcan Mert, Mustafa Caner Timur . Bitcoin and money supply relationship: An analysis of selected country economies. Quantitative Finance and Economics, 2023, 7(2): 229-248. doi: 10.3934/QFE.2023012 |
[8] | Hüseyin T. Eğen, Hale Akbulut . The import tariff changes and sectoral production in Turkey: a computable general equilibrium (CGE) modeling approach. Quantitative Finance and Economics, 2019, 3(3): 456-472. doi: 10.3934/QFE.2019.3.456 |
[9] | Chikashi Tsuji . The meaning of structural breaks for risk management: new evidence, mechanisms, and innovative views for the post-COVID-19 era. Quantitative Finance and Economics, 2022, 6(2): 270-302. doi: 10.3934/QFE.2022012 |
[10] | Jian Xu, Muhammad Haris, Muhammad Irfan . Assessing intellectual capital performance of banks during COVID-19: Evidence from China and Pakistan. Quantitative Finance and Economics, 2023, 7(2): 356-370. doi: 10.3934/QFE.2023017 |
There are a variety of indicators in economies like economic growth, foreign exchange rates, inflation, interest rates which are significant in development of countries. Each indicator has an important role in terms of its effects in economies (Orhan et al., 2019). Besides, most of these indicators have effect on price stability, financial stability and macroeconomic stability in turn by affecting and reflecting the riskiness, soundness and vulnerability of countries. In this context, one of the main instrument is CDS spreads.
CDS are derivative instruments which are used for protection against default risks on debts securities by foreign investors (Hibbert & Pavlova, 2017; The Central Bank of the Republic of Turkey (CBRT), 2020a). There are two main types of CDS as either sovereign or corporate CDS (Shahzad et al., 2017), and sovereign CDS are related with countries’ debts securities on which we focus on the study.
By considering that countries have been much more interdependent, multiple factors, which have effect on CDS spreads, should be taken into consideration. It is clear that global factors (fund flows, gold prices, market volatility, oil prices etc.) are not under control of countries itself. However, macro and market variables could be controlled mainly by countries which provides the decreasing contribution on CDS spreads.
CDS spreads can be evaluated as risk, financial stability, and macroeconomic stability indicator. Besides these, CDS spreads are significant for foreign investors in portfolio allocation decisions. This is not only a preference, but it also is a requirement which is resulting from the considering country risk, diversification of risks and finance theory (Dooley & Hutchison, 2009; Yang et al., 2018). For this reason, low-level CDS spreads have become one of the most important indicator for foreign portfolio investments which are very important for emerging countries because of the fact that they need much more (direct and portfolio) investments to be developed.
When examining current literature, it can be seen that, global, macroeconomic, and market variables affect CDS spreads of countries (Galil et al., 2014; Kocsis & Monostori, 2016). In this context, influential indicators must be followed up and directed by regulatory authorities of countries in order to decrease CDS spreads and hence stimulate foreign portfolio investments. Although starting point should be macroeconomic and market indicators, global indicators should also be considered. By this way, emerging countries are able to attract much more foreign investments.
On the other hand, all countries have been faced with Covid-19 which has become pandemic. It has originated from China at the beginning of the year 2020. There are totally 8.1 million disease cases, and 435 thousand deaths as of 06.16.2020 over the world (World Health Organization, 2020). Naturally, Covid-19 spreads to Turkey. The first case has been announced on 03.10.2020 officially. There are totally 181 thousand disease cases, and 4.8 thousand deaths as of 06.16.2020 (Turkey Ministry of Health, 2020). In this environment, all economic and financial indicators have been deteriorating in countries including Turkey. Therefore, this situation began to be called as “new normal”, and new normal should be considered in making any analysis in macroeconomic and financial issues.
In the light of all information above, the study examines the behavior of Turkey’s sovereign CDS spreads in recent times which includes before Covid-19 pandemic times and in Covid-19 times by employing MARS method. Daily data between 12.06.2019 and 06.16.2020 and 6 (3 global, 3 macro) indicators and 6 Covid-19 situations (presence, numbers of new cases, cumulative cases, new deaths, cumulative deaths, increasing rates of new cases and new deaths, presence of measures) are considered for this aim. The study focuses on Turkey because of the fact that Turkey has quite high level of CDS spreads. Another important reason is that Turkey has the highest 6th CDS spreads among the all countries as of 06.16.2020. The study defines that the most influential factors on Turkey’s CDS spreads vary according to the period which Turkey has been in Covid-19 pandemic or not. The results of the analysis shows the importance of number of new deaths and number of new cases from a common disease (Covid-19 pandemic) situation for CDS spreads of Turkey.
The main contributions of the study could be summarized as the study (i) uses MARS method which a non-parametric regression is building the functional relationship between variables without requiring any pre-assumption. Also, MARS method is used in the first time to examine CDS spreads of Turkey for making a comparison between before Covid-19 times and in Covid-19 times. Moreover, the study is a pioneer study in finance area in Turkey examining the relationship of Covid-19 with a financial issue, as far as known; (ii) examines the variables of Turkey’s sovereign CDS spreads by using global, market, and Covid-19 related variables; (iii) examines recent times from 12.06.2019 to 06.16.2020 on daily basis which covers Covid-19 times (iv) focuses on solely Turkey which is 6th country on the world which has the highest CDS spreads.
The remaining parts of the study is organized as follows. Part 2 presents the conceptual framework. Part 3 explains the variables, data, and theoretical background of MARS method. Part 4 tells the empirical results and presents a discussion based on the findings of the study with recommending some proposals. Part 5 presents the conclusion.
Because of the fact that emerging countries have mainly low-level savings, they need much more foreign investments both direct and portfolio in order to finance their growth so as to be developed. By considering foreign direct investments depend on a variety of global and macro conditions including geopolitical and political issues, emerging countries try to increase stimulating foreign portfolio investments. In this context, bonds/bills are main investment instruments.
Security investments to foreign countries, however, include country risks. This type of risks is higher especially for emerging countries. That is why because investors are doubtful about whether emerging countries will repay the borrowed funds or not. Therefore, foreign investors seek a protection shield against country risks. In this context, one of the main and important tool is CDS. CDS are used for protection against losses on foreign exchange rate (FER) denominated securities by foreign investors (Hibbert & Pavlova, 2017; CBRT, 2020a).
CDS buyer receives a compensation from CDS seller when issuer of the bonds/bills defaults. Amount of compensation is calculated based on CDS spreads on yearly base. Hence, CDS spreads have a role in reflecting the riskiness of the assets. An example, Turkey’s 5-years sovereign CDS spreads are 640 basis points (implying 6.4% interest rates) as of May 5, 2020 whereas it was 276 basis points (showing 2.76% interest rates) as of January 1, 2020. So, CDS spreads are calculated as USD 640 thousand as of May 5, 2020 nearly multiplying by 2.5 times with regard to it was USD 276 thousand as of January 1, 2020 for each year throughout CDS maturity for USD 10 billion bonds/bills (Hasan et al., 2016). In this context, amount of foreign portfolio investments could be negatively affected from the increasing of CDS spreads which show the increasing of country risks. This is because of the fact that insurance of securities become more expensive.
As one of the most important and leading emerging country, Turkey has quite high CDS spreads. Figure 1 shows the development of Turkey’s sovereign CDS spreads in 2020 including times of Covid-19 pandemic.
There is an increasing, quite volatile and high-level trend in CDS spreads of Turkey. This increase trend has begun to accelerate in February with the spreading of Covid-19 pandemic over the world. Also, CDS spreads peaked in April (677 as of 04.06.2020) in times at when Covid-19 pandemic has been accelerating in terms of new cases and number of deaths resulting from Covid-19 in Turkey. Although CDS spreads has decreased a bit in April, it has again increased in May and reached to the level of 640 points. As general, Turkey has quite volatile, increasing, and high-level CDS spreads which are a threat to provide sustainable foreign portfolio investment inflows.
In addition, Covid-19 pandemic has reached to Turkey. The first case was seen on 03.10.2020, and the first death was seen on 03.17.2020. Figure 2 shows the development of Covid-19 in Turkey.
Figure 2 shows that Covid-19 cases have been seen in Turkey since 10 March. Also, deaths have been seen since 17 March. Numbers of cumulative cases and cumulative deaths resulting from Covid-19 have been still increasing continuously in Turkey. Therefore, second and third waves are expected in Covid-19 pandemic in Turkey.
Negative effects have been seen on Turkey’s economy resulting from Covid-19. To prevent the occurrence or the degree of negative effects, Turkey’s regulatory authorities have announced fiscal, monetary, and administrative measures since beginning of 2020 March. Some of them could be summarized as local lockdowns; quarantines on residential areas; closure of schools, social spaces; ban on flights; decrease on the minimum payment requirements of credit cards; increasing the amount of funding markets by CBRT including buying government bonds; deferred credit payments to banks; VAT, social security premium payments deferred for six months in various sectors; minimum wage subsidies; cash transfers to families in need; state banks to provide cheaper loans to firms promising not to lay off employees; hotel taxes deferred for six months; credit guarantee fund size increased. In addition to these measures, some companies like Akbank, Halkbank, and Vakıfbank have cancelled dividend distribution (Morgan Stanley, 2020).
Although a variety of measures have been taken in order to decrease or prevent the negative effects of Covid-19 pandemic on Turkey’s economy, unfortunately negative developments have been seen on the economy. For example, FER have been increasing, current account deficit and foreign trade deficit have been increasing, and unemployment rate is expected to be increased for Covid-19 pandemic period. In addition, CDS spreads of Turkey have increased in Covid-19 period. While CDS spreads of Turkey was at the level of 407 points on 03.10.2020 which is the first date Covid-19 determined in Turkey, on the other hand, CDS spreads of Turkey have become 654 points on 05.08.2020. This condition suggests that Covid-19 pandemic has been causing an increase in CDS spreads of Turkey. That is why because Covid-19 pandemic has been an important and a main risk indicator for all developed and emerging countries including Turkey.
In this context, defining which factors have effect on Turkey’s CDS spreads would be useful and to test whether Covid-19 pandemic has an effect on Turkey’s CDS spreads or not. Therefore, the study focuses on examining the behavior of Turkey’s sovereign CDS spreads before Covid-19 times and in Covid-19 times. Hence, the study could examine the effects of Covid-19 on Turkey’s CDS spreads.
The present literature includes various studies examining the relationship between sovereign CDS spreads and a variety of variables. These variables could be mainly grouped as under two groups which are global and macro factors. Besides these, Covid-19 pandemic situations are taken into consideration in the study.
In the first group, studies examine the effects of global factors on CDS spreads. There are Morgan Stanley Capital International (MSCI) emerging market index, MSCI Turkey index, and volatility index in this group. In some studies, equity market price is used (Cremers et al., 2008; Zhang et al., 2009). Similarly, Yang et al. (2018) use MSCI all country world index as a determinant. Therefore, MSCI indices are expected to be related with CDS spread. By considering Turkey is an emerging country, MSCI emerging market index and MSCI Turkey index are selected to be used and a negative relationship is expected with CDS spreads. In addition, as an indicator implying market volatility, volatility (VIX) index is quite related with CDS spreads of countries. A strong positive relationship is defined between VIX index and CDS spreads in studies of Galil et al. (2014), Hibbert & Pavlova (2017), Akçelik & Fendoğlu (2019), CBRT (2020a).
In the second group, studies examine the relationship between macroeconomic factors and CDS spreads. Variables related with FER, Borsa İstanbul (BIST), and CBRT present in this group. In the context of FER, USD/TL is considered since this is the most important and used FER in Turkey. According to the studies of Ertuğrul & Öztürk (2013), Fontana & Scheicher (2016), Hassan et al. (2017), a positive relationship is expected between FER and CDS spreads. Also, equity index is used to examine CDS spreads. In this context, main stock exchange of Turkey (i.e. BIST100 index) is considered. Alexander & Kaeck (2008), Galil et al. (2014), Lahiani et al. (2016), Cremers et al. (2008), Zhang et al. (2009) use equity index as a variable and they define a significant effect on CDS. By considering these studies, a negative relationship is expected between BIST100 index and CDS spreads. In addition, CBRT weighted average cost of fund (WACF) is considered as a variables in the study. This is expected to be related with CDS spread. A positive relationship is expected between WACF and CDS spreads.
In the third group, Covid-19 situations are taken into consideration. In this group, there are 6 variables related with the Covid-19 pandemic in Turkey which is seen since 03.10.2020.
As dependent variables, 5-years CDS spreads are used due to the fact that this is the most liquid CDS spread types (CBRT, 2020a). Moreover, Table 1 summarizes the details of independent variables taking place in the analysis.
Variable Group | Variable | Symbol | Description | Expected Effect |
Global | MSCI Emerging Market Index | MSCIEM | MSCI Emerging Market Index | - |
MSCI Turkey Index | MSCITR | MSCI Turkey Index | - | |
VIX Index | VIX | Chicago Board Options Exchange Volatility Index | + | |
Macro | USD FER | USD/TL | USD/TL FER | + |
BIST100 Index | BIST100 | Trading Day Closing Value | - | |
CBRT WACF | WACF | CBRT WACF (%) | + | |
Covid-19 Pandemic | Presence | CVD | 1: If Covid-19 exist; 0: otherwise | + |
New Cases | NCASE | Number of New Cases | + | |
Cumulative Cases | CCASE | Cumulative Number of Cases | + | |
New Deaths | NDEATH | Number of New Deaths | + | |
Cumulative Deaths | CDEATH | Cumulative Number of Deaths | + | |
Measures | MEASURE | 1: If any measures (monetary, fiscal, administrative etc.) are taken; 0: otherwise | - | |
Note: Positive (+) relationship means that CDS increase when independent variable increases. Negative (-) relationship means that CDS decrease when independent variable increases. |
Apart from the variables summarized in above which are selected to be used in the study, it is possible to mention about other variables. In the literature, bond spreads (Fontana & Scheicher, 2016), credit ratings (Galil & Soffer, 2011), economic growth (Benbouzid et al., 2017; CBRT, 2020a), industrial production index (Johnson, 2002; Sagi & Seasholes, 2007; Liu & Zhang, 2008; Galil et al., 2014), inflation (Galil et al., 2014; Benbouzid et al., 2017; Akçelik & Fendoğlu, 2019; CBRT, 2020a), and reserves (Akçelik & Fendoğlu, 2019; CBRT, 2020a). Data for these variables are announced quarterly or monthly basis. However, the study focuses on Covid-19 pandemic times which requires to work on daily base data. Therefore, such variables could not be included in the study.
This study includes the period between 12.06.2019 and 06.16.2020. This period is selected to focus on the very recent period including Covid-19 pandemic times and the nearest times before Covid-19. For this reason, daily data is used in the study. In addition, data includes from 06.12.2019 to 03.09.2020 for the period which is before Covid-19 pandemic whereas data between 03.10.2020 and 06.16.2020 is included in Covid-19 pandemic times.
Data for CDS spreads, BIST100 index, VIX index, MSCI indices are gathered Bloomberg Terminal. Data for CBRT WACF and USD/TL FER are gathered from CBRT (2020b). Data for Covid-19 situations are gathered from Turkey Ministry of Health (2020).
Data for business days is considered because of the fact that data of some variables like FER and BIST100 can be obtained only for business day.
MARS method is introduced by Friedman (1991). MARS is a non-parametric regression modeling of high dimensional data to estimate underlying functional relationships between variables. Although linear model regressions require some pre-assumptions, MARS does not have any pre-assumption. Also, MARS method is flexible, computational speed and accurate predictors of piecewise-defined polynomials from correlated data (Goh et al., 2017).
There is two-steps in MARS method. In first step, the algorithm starts from a constant term that is the mean of the dependent values. In second step, the piecewise linear segments, known as basis functions (BFs), are iteratively added to the model.
BFs are dependent on spline functions that are defined on a given segment and the end points of the segment are called knots. The forward step finds the potential knots to improve the performance and leads to overfitting.
MARS model can be formulated as follows:
Y=f(x)=B0+∑Kk=1anBn(Xt)+ε | (1) |
In which Y is the dependent variable and X represents independent variables. B0 denotes the constant term and Bn(Xt) describes the basis function that is estimated by minimizing the residual sum of squares. an shows the coefficient of nth basis functions (Friedman, 1991). In the backward stepwise stage (pruning stage), it can be eliminated the redundant knots that have the least contribution to the complex model by using Generalized Cross-Validation (GCV).
GCV can be determined as follows:
GCV(K)=1N∑Ni=1[yi−f(xi)]2/[1−K+dKN]2 | (2) |
where d is the penalizing parameter for each basis function and N is the number of observations.
The best model is selected according to the highest coefficient of determination (R2) and the lowest GCV value (Hastie et al., 2009).
The MARS method has not been used in the literature to examine CDS spreads as far as it is known. On the other hand, the method has high prediction capacity. Therefore, the MARS method is performed in this study by considering these facts. It is though that by employing the MARS method to examine CDS spreads make a contribution to the literature. Hence, the study has a characteristic which is a pioneer study in finance area in Turkey examining the relationship of Covid-19 with a financial issue.
MARS creates all results which are obtained with 10-fold cross-validation approach and the best model is chosen based on GCV and R2 statistics. Appendix 1 shows the all models which are produced by MARS method. Totally 22 models (BFs) are produced for both before Covid-19 pandemic times and in Covid-19 pandemic by MARS.
In addition, MARS shows the important thresholds of independent variables which is shown in Appendix 2 and 3. As it can be seen from these appendixes, there are interactions between BIST100 index and MSCI Turkey index; BIST100 index and VIX index; USD/TL FER and VIX; MSCI Turkey index and USD/TL FER; MSCI Turkey index and VIX index in the period which is before Covid-19 times. On the other hand, interactions are seen between MSCI emerging market index and number of deaths; MSCI emerging market index and number of cases; USD/TL FER and number of deaths; VIX index and number of deaths in Covid-19 pandemic times.
As a result of MARS analysis, BF9 is the best model in terms of the value of GCV and R2 for the period which is before Covid-19 pandemic times. On the other hand, BF12 is the best model in Covid-19 pandemic times. 4 variables and 6 variables have a statistically significant effect on Turkey’s CDS spreads in these periods, respectively. According to the determination, which is presented in Table 2, the most significant variable on CDS spreads of Turkey is BIST100 index for the period which is before Covid-19 pandemic. In addition, VIX index, MSCI Turkey index, and USD/TL FER have significance on CDS spreads of Turkey in this period. On the other hand, MSCI emerging market index, number of new deaths, USD/TL FER, WACF, and number of new cases are influential in Covid-19 pandemic times.
Before Covid-19 Pandemic Times | In Covid-19 Pandemic Times | ||||
Variable | Importance Level | -GCV | Variable | Importance Level | -GCV |
BIST100 | 100.00 | 429.177 | MSCIEM | 100.000 | 2, 906.607 |
VIX | 79.567 | 303.918 | NDEATH | 91.866 | 2, 520.902 |
MSCITR | 43.033 | 151.003 | USD/TL | 63.669 | 1, 437.044 |
USD/TL | 35.847 | 131.653 | WACF | 43.247 | 897.408 |
NCASE | 30.813 | 669.828 | |||
VIX | 10.088 | 460.341 |
Daily data includes 66 observations for the both period. Table 3 shows the descriptive statistics of the variables for the periods.
Period | Variable | Observation | Average | Standard Deviation | Minimum | Maximum |
Before Covid-19 | CDS1 | 66 | 283.159 | 37.706 | 237.85 | 422.02 |
MSCITR | 66 | 1, 506, 688.15 | 62, 963.455 | 1, 357, 979 | 1, 615, 513 | |
VIX | 66 | 17.744 | 9.061 | 12.10 | 54.46 | |
USD/TL | 66 | 5.977 | 0.106 | 5.78 | 6.24 | |
BIST100 | 66 | 115, 716.301 | 5, 111.936 | 103, 524.023 | 123, 556.102 | |
In Covid-19 | CDS1 | 66 | 556.608 | 67.291 | 407.11 | 677 |
USD/TL | 66 | 6.742 | 0.241 | 6.12 | 7.15 | |
MSCIEM | 66 | 899.231 | 59.047 | 758.2 | 1, 012.51 | |
NDEATH | 66 | 48.409 | 39.06 | 0 | 126 | |
WACF | 66 | 8.816 | 0.863 | 7.64 | 10.65 | |
NCASE | 66 | 1, 836.439 | 1, 398.946 | 0 | 4, 801 | |
VIX | 66 | 42.151 | 15.046 | 24.52 | 82.69 | |
Note: The dependent variable. |
Table 4 presents the details of best model (BF9).
Basis Functions | Details | Coefficient |
Constant | 223.817 | |
BF1 | max(0, MSCITR-1, 462, 604) | - |
BF2 | max(0, 1, 462, 604-MSCITR) | -0.482987E-03 |
BF3 | max(0, VIX-18.23) | 3.058 |
BF4 | max(0, 18.23-VIX) | - |
BF5 | max(0, BIST100-109, 600.531) * BF2 | -0.787367E-06 |
BF8 | max(0, 118, 341.523-BIST100) * BF4 | -0.582810E-03 |
BF9 | max(0, USD/TL-6.15) * BF3 | 19.274 |
BF14 | max(0, 5.89-USD/TL) | 125.673 |
BF16 | max(0, 122, 320.773-BIST100) | 0.008 |
BF19 | max(0, USD/TL-5.95) * BF1 | 0.003 |
BF26 | max(0, 16.39-VIX) * BF1 | 0.418337E-04 |
F Test: 246.321 (0.000) Adjusted R2: 0.971 |
According to the best model (BF9), totally 4 variables also affect CDS spreads of Turkey in the period which is before Covid-19 pandemic times.
Table 5 presents the details of best model (BF12).
Basis Functions | Details | Coefficient |
Constant | 611.856 | |
BF1 | max(0, NDEATH-59) | 4.504 |
BF2 | max(0, 59-NDEATH) | -3.682 |
BF3 | max(0, MSCIEM-842.540) * BF2 | -0.012 |
BF4 | max(0, 842.54-MSCIEM) * BF2 | 0.023 |
BF5 | max(0, USD/TL-6.5) * BF2 | 10.146 |
BF7 | max(0, NDEATH-98) | 6.976 |
BF9 | max(0, NDEATH-76) | -8.515 |
BF10 | max(0, 76-NDEATH) | - |
BF11 | max(0, NCASE-311) | -0.014 |
BF13 | max(0, MSCIEM-966.32) * BF11 | -0.002 |
BF15 | max(0, USD/TL-6.84) * BF10 | 6.444 |
BF17 | max(0, VIX-45.41) * BF1 | -0.427 |
BF19 | max(0, WACF-8.91) | 68.109 |
F Test: 171.252 (0.000) Adjusted R2: 0.969 |
According to the best model (BF12), totally 6 variables also affect CDS spreads of Turkey in Covid-19 pandemic times.
Adjusted R2 of the best MARS model is 0.971 before Covid-19 pandemic times, and 0.969 in Covid-19 pandemic times. These results imply that the changes in Turkey’s sovereign CDS spreads can be explained with the independent variables used in these models.
As a result of the analysis, the best model for Turkey’s sovereign CDS spreads before Covid-19 pandemic times is estimated as follows:
CDS Spreads =223.817−0.482987E−03∗BF2+3.058∗BF3−0.787367E−06∗BF5−0.582810E−03∗BF8+19.274∗BF9+125.673∗BF14+0.008∗BF16+0.003∗BF19+0.418337E−04∗BF26 | (3) |
model CDS = BF2 BF3 BF5 BF8 BF9 BF14 BF16 BF19 BF26.
In addition, the best estimated for Turkey’s sovereign CDS spreads in Covid-19 pandemic times is determined as follows:
CDS Spreads =611.856+4.504∗ BF1 −3.682∗ BF2 −0.012∗ BF3 +0.023∗ BF4 +10.146∗ BF5 +6.976∗ BF7 −8.515∗ BF9 −0.014∗ BF11 −0.002∗ BF13 +6.444∗ BF15 −0.427∗ BF1 +68.109∗ BF19 |
(4) |
model CDS = BF1 BF2 BF3 BF4 BF5 BF7 BF9 BF11 BF13 BF15 BF17 BF19.
According to the analysis results, the most significant variables are BIST100 index (importance: 100), VIX index (importance: 79.567), MSCI Turkey index (importance: 43.033), and USD/TL FER (importance: 35.847), respectively for the period which is before Covid-19 pandemic times. On the other hand, the most significant variables are MSCI emerging market index (importance: 100), number of new deaths from Covid-19 (importance: 91.866), USD/TL FER (importance: 63.669), WACF (importance: 43.247), number new cases from Covid-19 (importance: 30.813), and VIX index (importance: 10.088), respectively in Covid-19 pandemic times.
The analysis results reveal that Turkey’s sovereign CDS spreads are mainly affected from USD/TL FER, VIX index, and MSCI index (MSCI Turkey index and emerging market index) in the both period. Also, 2 Covid-19 situation (number of new cases and deaths) are determined as influential factors in Covid-19 times. On the other hand, number of cumulative cases, number of cumulative deaths and measures do not have effect on Turkey’s CDS spreads in any period examined which is quite surprising since it was expected that they would have effect.
Based on the analysis results, it can be mainly recommended that the critical barriers in each variable should be exceed. For example, 109.6 thousand is critical barrier for BIST100, TL 6.15 is important for USD/TL FER before Covid-19 pandemic. On the other hand, TL 6.5 is important for USD/TL FER, 311 is important for number of new cases, and 59is significant number of new deaths on each day in Covid-19 pandemic times. By considering that Turkey has been in Covid-19 pandemic times, and Turkey does not have the capacity to lower the negative effects of global factors, Turkey should focus on the national variables which are USD/TL FER, BIST100 index, WACF, and number of new cases and deaths resulting from Covid-19 pandemic. Especially, USD/TL FER is so important for Turkey’s CDS by taking into consideration that there are quite high amount of foreign exchange denominated debts. Turkey could prevent the negative contagion effect of FER through keeping USD/TL FER under control.
Moreover, other polices, besides the points mentioned above, could be applied by regulatory authorities in Turkey. An important point is that there are interactions between variables and this condition should be considered in developing polies. For this reason, possible outcomes of the precautions on CDS spreads must be foreseen before implementation.
The study examines the behavior of Turkey’s sovereign CDS spreads before and in time of Covid-19 pandemic. Because, emerging countries need foreign financing, foreign portfolio investments are significant for emerging countries, CDS spreads are important credit default indicator, and there is Covid-19 pandemic over the world including Turkey. In this context, 5-years CDS spread is used as dependent variable; 6 (3 global, 6 macroeconomic) indicators are selected by benefitting from the studies present in the literature; 6 Covid-19 situations are added to the analysis; and daily data between 12.06.2019 and 06.16.2020 is analyzed with using MARS method, which provides the high predictive accuracy of the results.
As a result of MARS analysis, it is defined that the most influential factors vary according to period whether Turkey has been in Covid-19 time or not. The results imply that Covid-19 pandemic causes the increasing effect on Turkey’s CDS spreads. In addition, USD/TL FER, VIX index and some MSCI indices are determined as influential in both period. On the other hand, some variables like number of cumulative cases, number of cumulative deaths, and measures do not have effect on Turkey’s sovereign CDS spreads which were expected to have effect. Based on the results, some recommendations are presented in the study.
In the study, Turkey is examined because Turkey is 6th country which has the highest CDS spreads on the world. Also, Turkey’s CDS spreads have increased in time of Covid-19 rapidly. Taking measures by considering the results of the study, which emphasize the importance of new cases and deaths number from Covid-19 pandemic on Turkey’s CDS spreads, is quite significant. Hence, Turkey could increase foreign portfolio investment inflows to Turkey. Moreover, additional analysis for Turkey at the end of Covid-19 pandemic times could provide additional contributions to the literature, and new policy proposals could be recommended.
Including of a different bundle of countries and including more emerging countries like Venezuela, Argentina, Pakistan, Egypt, Ukraine, South Africa, Brazil, Italy, Greece, and Mexico which have highest CDS spread on the world as of 05.10.2020 or emerging country groups like BRICS-T, Fragile Five, MINT, and E7 could be beneficial in terms of extending current literature. Moreover, new variables could be included in analysis and new statistical and econometric methods could be applied in the forthcoming studies.
There is no conflict of interest to be disclosed.
[1] | Akçelik F, Fendoğlu S (2019) Country Risk Premium and Domestic Macroeconomic Fundamentals When Global Risk Appetite Slides. CBRT Research and Monetary Policy Department, No. 2019-04. |
[2] |
Alexander C, Kaeck A (2008) Regime Dependent Determinants of Credit Default Swap Spreads. J Bank Financ 32: 1008-1021. doi: 10.1016/j.jbankfin.2007.08.002
![]() |
[3] |
Benbouzid N, Mallick SK, Sousa RM (2017) An International Forensic Perspective of the Determinants of Bank CDS Spreads. J Financ Stability 33: 60-70. doi: 10.1016/j.jfs.2017.10.004
![]() |
[4] | CBRT (2020a) Inflation Report 2020-I. Available from: https://www.tcmb.gov.tr/wps/wcm/connect/EN/TCMB+EN/Main+Menu/Publications/Reports/Inflation+Report. |
[5] | CBRT (2020b) Electronic Data Distribution System (EVDS). Available from: https://evds2.tcmb.gov.tr/index.php?/evds/serieMarket. |
[6] |
Cremers KM, Driessen J, Maenhout P (2008) Explaining the Level of Credit Spreads: Option-Implied Jump Risk Premia in a Firm Value Model. Rev Financ Stud 21: 2209-2242. doi: 10.1093/rfs/hhn071
![]() |
[7] |
Dooley M, Hutchison M (2009) Transmission of the US Subprime Crisis to Emerging Markets: Evidence on the Decoupling-Recoupling Hypothesis. J Int Money Financ 28: 1331-1349. doi: 10.1016/j.jimonfin.2009.08.004
![]() |
[8] |
Ertuğrul HM, Öztürk H (2013) The Drivers of Credit Swap Prices: Evidence from Selected Emerging Market Countries. Emerging Markets Financ Trade 49: 228-249. doi: 10.2753/REE1540-496X4905S514
![]() |
[9] |
Fontana A, Scheicher M (2016) An Analysis of Euro Area Sovereign CDS and Their Relation with Government Bonds. J Bank Financ 62: 126-140. doi: 10.1016/j.jbankfin.2015.10.010
![]() |
[10] |
Friedman J (1991) Multivariate Adaptive Regression Splines. Annals Stat 19: 1-141. doi: 10.1214/aos/1176347963
![]() |
[11] |
Galil K, Shapir OM, Amiram D, et al. (2014) The Determinants of CDS Spreads. J Bank Financ 41: 271-282. doi: 10.1016/j.jbankfin.2013.12.005
![]() |
[12] |
Galil K, Soffer G (2011) Good News, Bad News and Rating Announcements: An Empirical Investigation. J Bank Financ 35: 3101-3119. doi: 10.1016/j.jbankfin.2011.04.010
![]() |
[13] |
Goh ATC, Zhang Y, Zhang R, et al. (2017) Evaluating Stability of Underground Entry-Type Excavations Using Multivariate Adaptive Regression Splines and Logistic Regression. Tunnelling Underground Space Tcehnology 70: 148-154. doi: 10.1016/j.tust.2017.07.013
![]() |
[14] |
Hasan I, Liu L, Zhang G (2016) The Determinants of Global Bank Credit-Default-Swap Spreads. J Financ Serv Res 50: 275-309. doi: 10.1007/s10693-015-0232-z
![]() |
[15] | Hassan MK, Kayhan S, Bayat T (2017). Does Credit Default Swap Spread Affect the Value of the Turkish Lira Against the US Dollar? Borsa İstanbul Rev 17: 1-9. |
[16] | Hastie T, Tibshirani R, Friedman J (2009) The Elements of Statistical Learning: Data Mining, Inference and Prediction. 2nd Edition. New York: Springer. |
[17] |
Hibbert AM, Pavlova I (2017) The Drivers of Sovereign CDS Spread Changes: Local versus Global Factors. Financ Rev 52: 435-457. doi: 10.1111/fire.12140
![]() |
[18] |
Johnson TC (2002) Rational Momentum Effects. J Financ 57: 585-608. doi: 10.1111/1540-6261.00435
![]() |
[19] |
Kocsis Z, Monostori Z (2016) The Role of Country-Specific Fundamentals in Sovereign CDS Spreads: Eastern European Experiences. Emerging Markets Rev 27: 140-168. doi: 10.1016/j.ememar.2016.05.003
![]() |
[20] |
Lahiani A, Hammoudeh S, Gupta R (2016) Linkages between Financial Sector CDS Spreads and Macroeconomic Influence in a Nonlinear Setting. Int Rev Econ Financ 43: 443-456. doi: 10.1016/j.iref.2016.01.007
![]() |
[21] |
Liu LX, Zhang L (2008) Momentum Profits, Factor Pricing, and Macroeconomic Risk. Rev Financ Stud 21: 2417-2448. doi: 10.1093/rfs/hhn090
![]() |
[22] | Morgan Stanley (2020) EEMEA Covid-19 Impact & Response, Research Report. |
[23] |
Orhan A, Kırıkkaleli D, Ayhan F (2019) Analysis of Wavelet Coherence: Service Sector Index and Economic Growth in an Emerging Market. Sustainability 11: 6684. doi: 10.3390/su11236684
![]() |
[24] |
Sagi JS, Seasholes MS (2007) Firm-Specific Attributes and the Cross-Section of Momentum. J Financ Econ 84: 389-434. doi: 10.1016/j.jfineco.2006.02.002
![]() |
[25] |
Shahzad SJH, Nor SM, Ferrer R, et al. (2017) Asymmetric Determinants of CDS Spreads: US Industry-Level Evidence through the NARDL Approach. Econ Model 60: 211-230. doi: 10.1016/j.econmod.2016.09.003
![]() |
[26] | Turkey Ministry of Health (2020) Covid-19 Numbers. Available from: https://covid19.saglik.gov.tr. |
[27] | World Health Organization (2020) Covid-19 Numbers. Available from: https://covid19.who.int. |
[28] |
Yang L, Yang L, Hamori S (2018) Determinants of Dependence Structures of Sovereign Credit Default Swap Spreads between G7 and BRICS Countries. Int Rev Financ Anal 59: 19-34. doi: 10.1016/j.irfa.2018.06.001
![]() |
[29] |
Zhang BY, Zhou H, Zhu H (2009) Explaining Credit Default Swap Spreads with the Equity Volatility and Jump Risks of Individual Firms. Rev Financ Stud 22: 5099-5131. doi: 10.1093/rfs/hhp004
![]() |
![]() |
![]() |
1. | Mustafa Tevfik Kartal, Özer Depren, Serpil Kılıç Depren, The determinants of main stock exchange index changes in emerging countries: evidence from Turkey in COVID-19 pandemic age, 2020, 4, 2573-0134, 526, 10.3934/QFE.2020025 | |
2. | Mustafa Tevfik Kartal, Serpil Kiliç Depren, Özer Depren, How Main Stock Exchange Indices React to Covid-19 Pandemic: Daily Evidence from East Asian Countries, 2021, 50, 1226-508X, 54, 10.1080/1226508X.2020.1869055 | |
3. | Yufei Xia, Lingyun He, Yinguo Li, Yating Fu, Yixin Xu, A DYNAMIC CREDIT SCORING MODEL BASED ON SURVIVAL GRADIENT BOOSTING DECISION TREE APPROACH, 2020, 27, 2029-4913, 96, 10.3846/tede.2020.13997 | |
4. | N.serap VURUR, BİST 100 ENDEKSİ CDS PRİMLERİ ARASINDAKİ İLİŞKİDE COVİD 19 ETKİSİ, 2021, 1307-9832, 10.18092/ulikidince.823358 | |
5. | Mustafa Tevfik KARTAL, Özer DEPREN, Serpil KILIÇ DEPREN, The relationship between mobility and COVID-19 pandemic: Daily evidence from an emerging country by causality analysis, 2021, 10, 25901982, 100366, 10.1016/j.trip.2021.100366 | |
6. | Serpil KILIÇ DEPREN, Türkiye’de Cari Açığı Etkileyen Faktörlerin Belirlenmesine Yönelik İstatistiksel Bir Araştırma, 2021, 8, 2148-4287, 426, 10.17336/igusbd.533434 | |
7. | Jinyu Chen, Yilin Wang, Xiaohang Ren, Asymmetric effects of non-ferrous metal price shocks on clean energy stocks: Evidence from a quantile-on-quantile method, 2022, 78, 03014207, 102796, 10.1016/j.resourpol.2022.102796 | |
8. | Mustafa Tevfik KARTAL, Özer DEPREN, Serpil KILIÇ DEPREN, Do Monetary Policy Measures Affect Foreign Exchange Rates during the COVID-19 Pandemic? Evidence from Turkey, 2021, 1307-5705, 175, 10.46520/bddkdergisi.987416 | |
9. | Fatih Ayhan, Mustafa Tevfik Kartal, Tomiwa Sunday Adebayo, Derviş Kirikkaleli, Nexus between economic risk and political risk in the United Kingdom: Evidence from wavelet coherence and quantile‐on‐quantile approaches, 2022, 0307-3378, 10.1111/boer.12371 | |
10. | Mustafa Tevfik Kartal, Özer Depren, Asymmetric relationship between global and national factors and domestic food prices: evidence from Turkey with novel nonlinear approaches, 2023, 9, 2199-4730, 10.1186/s40854-022-00407-9 | |
11. | Mustafa Tevfik Kartal, Hasan Murat Ertuğrul, Talat Ulussever, The impacts of foreign portfolio flows and monetary policy responses on stock markets by considering COVID-19 pandemic: Evidence from Turkey, 2022, 22, 22148450, 12, 10.1016/j.bir.2021.06.003 | |
12. | Mustafa Tevfik Kartal, Fatih Ayhan, Derviş Kirikkaleli, Regime-switching effect of COVID-19 pandemic on stock market index: evidence from Turkey as an emerging market example, 2022, 1752-0843, 1, 10.1080/17520843.2022.2091825 | |
13. | Mustafa Tevfik Kartal, Serpil Kılıç Depren, Özer Depren, Housing prices in emerging countries during COVID-19: evidence from Turkey, 2021, 1753-8270, 10.1108/IJHMA-07-2021-0083 | |
14. | Mustafa Tevfik KARTAL, Fatih AYHAN, Murat ERTUĞRUL, DETERMINANTS OF SOVEREIGN CREDIT DEFAULT SWAPS (CDS) SPREADS IN EMERGING COUNTRIES: EVIDENCE FROM TURKEY, 2022, 1301-8752, 10.17065/huniibf.1054042 | |
15. | Hussien Mohsen Ahmed, Sherif Ismail El-Halaby, Hebatallah Ahmed Soliman, The consequence of the credit risk on the financial performance in light of COVID-19: Evidence from Islamic versus conventional banks across MEA region, 2022, 8, 2314-7210, 10.1186/s43093-022-00122-y | |
16. | Qingqing Hu, Xiaobing Wang, Mark Xu, Are there heterogeneous impacts of social support on subjective well-being?, 2021, 3, 2689-3010, 360, 10.3934/NAR.2021019 | |
17. | Takayasu Ito, Contagion of credit risk: Comparative analysis before and after the pandemic of COVID‐19, 2022, 33, 1044-8136, 52, 10.1002/jcaf.22562 | |
18. | Mustafa Tevfik KARTAL, Serpil KILIÇ DEPREN, Özer DEPREN, Sovereign Credit Default Swap (CDS) Spreads Changes in Various Economic Conjunctures: Evidence from Turkey By Machine Learning Algorithms, 2022, 2148-029X, 10.11611/yead.1076897 | |
19. | Özer Depren, Mustafa Tevfik Kartal, Serpil Kılıç Depren, Changes of gold prices in COVID-19 pandemic: Daily evidence from Turkey's monetary policy measures with selected determinants, 2021, 170, 00401625, 120884, 10.1016/j.techfore.2021.120884 | |
20. | Özer Depren, Mustafa Tevfik Kartal, Serpil Kılıç Depren, Recent innovation in benchmark rates (BMR): evidence from influential factors on Turkish Lira Overnight Reference Interest Rate with machine learning algorithms, 2021, 7, 2199-4730, 10.1186/s40854-021-00245-1 | |
21. | Mustafa Tevfik Kartal, Özer Depren, Serpil Kilic Depren, Do Monetary Policy Measures Affect Foreign Exchange Rates during the COVID-19 Pandemic? Evidence from Turkey, 2021, 1556-5068, 10.2139/ssrn.3915756 | |
22. | Tomiwa Sunday Adebayo, Mustafa Tevfik Kartal, Effect of green bonds, oil prices, and COVID-19 on industrial CO2 emissions in the USA: Evidence from novel wavelet local multiple correlation approach, 2023, 0958-305X, 0958305X2311674, 10.1177/0958305X231167463 | |
23. | Ömer Faruk TAN, The Impact of News about Pandemic on Borsa Istanbul during the COVID-19 Financial Turmoil, 2021, 2630-6220, 109, 10.17829/turcom.859299 | |
24. | Arshad Khan, Naveed Raza, Macroeconomic Determinants of the Term Structure of Sovereign Credit Default Swap (CDS) Spread: Insights from MIDAS Regression, 2023, 9, 2410-8162, 10.61506/02.00111 | |
25. | Huthaifa Alqaralleh, The sovereign Credit Default Swap Spreads and Chinese Sectors Stock Market: A Causality in Quantile and Dependence Analysis, 2024, 31, 1387-2834, 845, 10.1007/s10690-023-09433-8 | |
26. | Albert Acheampong, Ngozi Ibeji, Albert Danso, COVID‐19 and credit risk variation across banks: International insights, 2024, 45, 0143-6570, 4415, 10.1002/mde.4271 | |
27. | Muhammet Atlas DOĞAN, Derese Kebede TEKLİE, Türkiye Stock Market in the Shadow of COVID-19 Pandemic: A QARDL Approach, 2024, 8, 2564-7504, 362, 10.25295/fsecon.1317679 | |
28. | Mustafa Tevfik Kartal, Talat Ulussever, Ugur Korkut Pata, Serpil Kılıç Depren, Dynamic link between central bank reserves, credit default swap spreads, and foreign exchange rates: Evidence from Turkey by time series econometrics, 2023, 9, 24058440, e16392, 10.1016/j.heliyon.2023.e16392 | |
29. | Letife Özdemir, Simon Grima, Ercan Özen, Ramona Rupeika-Apoga, Inna Romanova, Sovereign Credit Default Swap Market Volatility in BRICS Countries Before and During the COVID-19 Pandemic, 2024, 71, 2501-3165, 21, 10.47743/saeb-2024-0005 | |
30. | Bilgehan Tekin, Do economic uncertainty and political risk steer CDS dynamics? An analysis of the Türkiye CDS, 2024, 1753-8394, 10.1108/IMEFM-05-2024-0215 |
Variable Group | Variable | Symbol | Description | Expected Effect |
Global | MSCI Emerging Market Index | MSCIEM | MSCI Emerging Market Index | - |
MSCI Turkey Index | MSCITR | MSCI Turkey Index | - | |
VIX Index | VIX | Chicago Board Options Exchange Volatility Index | + | |
Macro | USD FER | USD/TL | USD/TL FER | + |
BIST100 Index | BIST100 | Trading Day Closing Value | - | |
CBRT WACF | WACF | CBRT WACF (%) | + | |
Covid-19 Pandemic | Presence | CVD | 1: If Covid-19 exist; 0: otherwise | + |
New Cases | NCASE | Number of New Cases | + | |
Cumulative Cases | CCASE | Cumulative Number of Cases | + | |
New Deaths | NDEATH | Number of New Deaths | + | |
Cumulative Deaths | CDEATH | Cumulative Number of Deaths | + | |
Measures | MEASURE | 1: If any measures (monetary, fiscal, administrative etc.) are taken; 0: otherwise | - | |
Note: Positive (+) relationship means that CDS increase when independent variable increases. Negative (-) relationship means that CDS decrease when independent variable increases. |
Before Covid-19 Pandemic Times | In Covid-19 Pandemic Times | ||||
Variable | Importance Level | -GCV | Variable | Importance Level | -GCV |
BIST100 | 100.00 | 429.177 | MSCIEM | 100.000 | 2, 906.607 |
VIX | 79.567 | 303.918 | NDEATH | 91.866 | 2, 520.902 |
MSCITR | 43.033 | 151.003 | USD/TL | 63.669 | 1, 437.044 |
USD/TL | 35.847 | 131.653 | WACF | 43.247 | 897.408 |
NCASE | 30.813 | 669.828 | |||
VIX | 10.088 | 460.341 |
Period | Variable | Observation | Average | Standard Deviation | Minimum | Maximum |
Before Covid-19 | CDS1 | 66 | 283.159 | 37.706 | 237.85 | 422.02 |
MSCITR | 66 | 1, 506, 688.15 | 62, 963.455 | 1, 357, 979 | 1, 615, 513 | |
VIX | 66 | 17.744 | 9.061 | 12.10 | 54.46 | |
USD/TL | 66 | 5.977 | 0.106 | 5.78 | 6.24 | |
BIST100 | 66 | 115, 716.301 | 5, 111.936 | 103, 524.023 | 123, 556.102 | |
In Covid-19 | CDS1 | 66 | 556.608 | 67.291 | 407.11 | 677 |
USD/TL | 66 | 6.742 | 0.241 | 6.12 | 7.15 | |
MSCIEM | 66 | 899.231 | 59.047 | 758.2 | 1, 012.51 | |
NDEATH | 66 | 48.409 | 39.06 | 0 | 126 | |
WACF | 66 | 8.816 | 0.863 | 7.64 | 10.65 | |
NCASE | 66 | 1, 836.439 | 1, 398.946 | 0 | 4, 801 | |
VIX | 66 | 42.151 | 15.046 | 24.52 | 82.69 | |
Note: The dependent variable. |
Basis Functions | Details | Coefficient |
Constant | 223.817 | |
BF1 | max(0, MSCITR-1, 462, 604) | - |
BF2 | max(0, 1, 462, 604-MSCITR) | -0.482987E-03 |
BF3 | max(0, VIX-18.23) | 3.058 |
BF4 | max(0, 18.23-VIX) | - |
BF5 | max(0, BIST100-109, 600.531) * BF2 | -0.787367E-06 |
BF8 | max(0, 118, 341.523-BIST100) * BF4 | -0.582810E-03 |
BF9 | max(0, USD/TL-6.15) * BF3 | 19.274 |
BF14 | max(0, 5.89-USD/TL) | 125.673 |
BF16 | max(0, 122, 320.773-BIST100) | 0.008 |
BF19 | max(0, USD/TL-5.95) * BF1 | 0.003 |
BF26 | max(0, 16.39-VIX) * BF1 | 0.418337E-04 |
F Test: 246.321 (0.000) Adjusted R2: 0.971 |
Basis Functions | Details | Coefficient |
Constant | 611.856 | |
BF1 | max(0, NDEATH-59) | 4.504 |
BF2 | max(0, 59-NDEATH) | -3.682 |
BF3 | max(0, MSCIEM-842.540) * BF2 | -0.012 |
BF4 | max(0, 842.54-MSCIEM) * BF2 | 0.023 |
BF5 | max(0, USD/TL-6.5) * BF2 | 10.146 |
BF7 | max(0, NDEATH-98) | 6.976 |
BF9 | max(0, NDEATH-76) | -8.515 |
BF10 | max(0, 76-NDEATH) | - |
BF11 | max(0, NCASE-311) | -0.014 |
BF13 | max(0, MSCIEM-966.32) * BF11 | -0.002 |
BF15 | max(0, USD/TL-6.84) * BF10 | 6.444 |
BF17 | max(0, VIX-45.41) * BF1 | -0.427 |
BF19 | max(0, WACF-8.91) | 68.109 |
F Test: 171.252 (0.000) Adjusted R2: 0.969 |
Variable Group | Variable | Symbol | Description | Expected Effect |
Global | MSCI Emerging Market Index | MSCIEM | MSCI Emerging Market Index | - |
MSCI Turkey Index | MSCITR | MSCI Turkey Index | - | |
VIX Index | VIX | Chicago Board Options Exchange Volatility Index | + | |
Macro | USD FER | USD/TL | USD/TL FER | + |
BIST100 Index | BIST100 | Trading Day Closing Value | - | |
CBRT WACF | WACF | CBRT WACF (%) | + | |
Covid-19 Pandemic | Presence | CVD | 1: If Covid-19 exist; 0: otherwise | + |
New Cases | NCASE | Number of New Cases | + | |
Cumulative Cases | CCASE | Cumulative Number of Cases | + | |
New Deaths | NDEATH | Number of New Deaths | + | |
Cumulative Deaths | CDEATH | Cumulative Number of Deaths | + | |
Measures | MEASURE | 1: If any measures (monetary, fiscal, administrative etc.) are taken; 0: otherwise | - | |
Note: Positive (+) relationship means that CDS increase when independent variable increases. Negative (-) relationship means that CDS decrease when independent variable increases. |
Before Covid-19 Pandemic Times | In Covid-19 Pandemic Times | ||||
Variable | Importance Level | -GCV | Variable | Importance Level | -GCV |
BIST100 | 100.00 | 429.177 | MSCIEM | 100.000 | 2, 906.607 |
VIX | 79.567 | 303.918 | NDEATH | 91.866 | 2, 520.902 |
MSCITR | 43.033 | 151.003 | USD/TL | 63.669 | 1, 437.044 |
USD/TL | 35.847 | 131.653 | WACF | 43.247 | 897.408 |
NCASE | 30.813 | 669.828 | |||
VIX | 10.088 | 460.341 |
Period | Variable | Observation | Average | Standard Deviation | Minimum | Maximum |
Before Covid-19 | CDS1 | 66 | 283.159 | 37.706 | 237.85 | 422.02 |
MSCITR | 66 | 1, 506, 688.15 | 62, 963.455 | 1, 357, 979 | 1, 615, 513 | |
VIX | 66 | 17.744 | 9.061 | 12.10 | 54.46 | |
USD/TL | 66 | 5.977 | 0.106 | 5.78 | 6.24 | |
BIST100 | 66 | 115, 716.301 | 5, 111.936 | 103, 524.023 | 123, 556.102 | |
In Covid-19 | CDS1 | 66 | 556.608 | 67.291 | 407.11 | 677 |
USD/TL | 66 | 6.742 | 0.241 | 6.12 | 7.15 | |
MSCIEM | 66 | 899.231 | 59.047 | 758.2 | 1, 012.51 | |
NDEATH | 66 | 48.409 | 39.06 | 0 | 126 | |
WACF | 66 | 8.816 | 0.863 | 7.64 | 10.65 | |
NCASE | 66 | 1, 836.439 | 1, 398.946 | 0 | 4, 801 | |
VIX | 66 | 42.151 | 15.046 | 24.52 | 82.69 | |
Note: The dependent variable. |
Basis Functions | Details | Coefficient |
Constant | 223.817 | |
BF1 | max(0, MSCITR-1, 462, 604) | - |
BF2 | max(0, 1, 462, 604-MSCITR) | -0.482987E-03 |
BF3 | max(0, VIX-18.23) | 3.058 |
BF4 | max(0, 18.23-VIX) | - |
BF5 | max(0, BIST100-109, 600.531) * BF2 | -0.787367E-06 |
BF8 | max(0, 118, 341.523-BIST100) * BF4 | -0.582810E-03 |
BF9 | max(0, USD/TL-6.15) * BF3 | 19.274 |
BF14 | max(0, 5.89-USD/TL) | 125.673 |
BF16 | max(0, 122, 320.773-BIST100) | 0.008 |
BF19 | max(0, USD/TL-5.95) * BF1 | 0.003 |
BF26 | max(0, 16.39-VIX) * BF1 | 0.418337E-04 |
F Test: 246.321 (0.000) Adjusted R2: 0.971 |
Basis Functions | Details | Coefficient |
Constant | 611.856 | |
BF1 | max(0, NDEATH-59) | 4.504 |
BF2 | max(0, 59-NDEATH) | -3.682 |
BF3 | max(0, MSCIEM-842.540) * BF2 | -0.012 |
BF4 | max(0, 842.54-MSCIEM) * BF2 | 0.023 |
BF5 | max(0, USD/TL-6.5) * BF2 | 10.146 |
BF7 | max(0, NDEATH-98) | 6.976 |
BF9 | max(0, NDEATH-76) | -8.515 |
BF10 | max(0, 76-NDEATH) | - |
BF11 | max(0, NCASE-311) | -0.014 |
BF13 | max(0, MSCIEM-966.32) * BF11 | -0.002 |
BF15 | max(0, USD/TL-6.84) * BF10 | 6.444 |
BF17 | max(0, VIX-45.41) * BF1 | -0.427 |
BF19 | max(0, WACF-8.91) | 68.109 |
F Test: 171.252 (0.000) Adjusted R2: 0.969 |