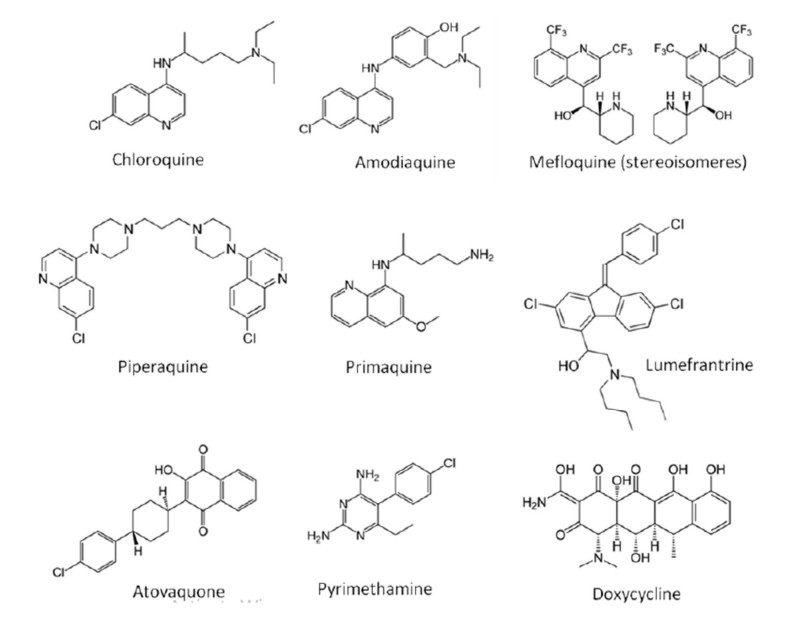
Parkinson's Disease is a neurodegenerative central nervous system (CNS) disease that primarily affects the dopaminergic cells of the Substantia Nigra in the midbrain and causes a diverse array of symptoms, including dystonia, a loss of balance, difficulty initiating movements, akinesia, muscle spasms, and tremors. It has long been known that environmental and commercial compounds are linked to an increased risk of Parkinson's Disease. Of importance, gluten, a complex polysaccharide, has been hypothesized to cause some of the symptoms related to Parkinson's Disease. It is hypothesized that gluten causes a chronic inflammatory state which may lead to plaque formation and neuronal cell death in the substantia nigra, alongside the symptoms of Parkinson's Disease. This literature review hopes to explore the relationship gluten has as an inflammatory molecule and its role in the production and prolongation of the disease processes in Parkinson's Disease.
Citation: Zohaer Muttalib, Dana Aboukhalil, Chisom Nwosu, Dave D. Manguerra, Jimmy Wen, Ubaid Ansari, Meraj Alam, Ihab Abed, Ethan Tabaie, Ahmed Salem, Forshing Lui. Gluten and its relationship with inflammation and Parkinson's Disease: A literature review[J]. AIMS Neuroscience, 2025, 12(1): 28-41. doi: 10.3934/Neuroscience.2025004
[1] | Deepalakshmi Sarkarai, Kalyani Desikan . QSPR/QSAR analysis of some eccentricity based topological descriptors of antiviral drugs used in COVID-19 treatment via Dε- polynomials. Mathematical Biosciences and Engineering, 2023, 20(9): 17272-17295. doi: 10.3934/mbe.2023769 |
[2] | Saylé C. Sigarreta, Saylí M. Sigarreta, Hugo Cruz-Suárez . On degree–based topological indices of random polyomino chains. Mathematical Biosciences and Engineering, 2022, 19(9): 8760-8773. doi: 10.3934/mbe.2022406 |
[3] | Cheng-Peng Li, Cheng Zhonglin, Mobeen Munir, Kalsoom Yasmin, Jia-bao Liu . M-polynomials and topological indices of linear chains of benzene, napthalene and anthracene. Mathematical Biosciences and Engineering, 2020, 17(3): 2384-2398. doi: 10.3934/mbe.2020127 |
[4] | Edil D. Molina, Paul Bosch, José M. Sigarreta, Eva Tourís . On the variable inverse sum deg index. Mathematical Biosciences and Engineering, 2023, 20(5): 8800-8813. doi: 10.3934/mbe.2023387 |
[5] | José M. Sigarreta . Extremal problems on exponential vertex-degree-based topological indices. Mathematical Biosciences and Engineering, 2022, 19(7): 6985-6995. doi: 10.3934/mbe.2022329 |
[6] | Fengwei Li, Qingfang Ye, Juan Rada . Extremal values of VDB topological indices over F-benzenoids with equal number of edges. Mathematical Biosciences and Engineering, 2023, 20(3): 5169-5193. doi: 10.3934/mbe.2023240 |
[7] | Wanlin Zhu, Minglei Fang, Xianya Geng . Enumeration of the Gutman and Schultz indices in the random polygonal chains. Mathematical Biosciences and Engineering, 2022, 19(11): 10826-10845. doi: 10.3934/mbe.2022506 |
[8] | Bo Sun, Ming Wei, Wei Wu, Binbin Jing . A novel group decision making method for airport operational risk management. Mathematical Biosciences and Engineering, 2020, 17(3): 2402-2417. doi: 10.3934/mbe.2020130 |
[9] | Qingqun Huang, Muhammad Labba, Muhammad Azeem, Muhammad Kamran Jamil, Ricai Luo . Tetrahedral sheets of clay minerals and their edge valency-based entropy measures. Mathematical Biosciences and Engineering, 2023, 20(5): 8068-8084. doi: 10.3934/mbe.2023350 |
[10] | Yi Liu, Xuan Zhao, Feng Mao . The synergy degree measurement and transformation path of China's traditional manufacturing industry enabled by digital economy. Mathematical Biosciences and Engineering, 2022, 19(6): 5738-5753. doi: 10.3934/mbe.2022268 |
Parkinson's Disease is a neurodegenerative central nervous system (CNS) disease that primarily affects the dopaminergic cells of the Substantia Nigra in the midbrain and causes a diverse array of symptoms, including dystonia, a loss of balance, difficulty initiating movements, akinesia, muscle spasms, and tremors. It has long been known that environmental and commercial compounds are linked to an increased risk of Parkinson's Disease. Of importance, gluten, a complex polysaccharide, has been hypothesized to cause some of the symptoms related to Parkinson's Disease. It is hypothesized that gluten causes a chronic inflammatory state which may lead to plaque formation and neuronal cell death in the substantia nigra, alongside the symptoms of Parkinson's Disease. This literature review hopes to explore the relationship gluten has as an inflammatory molecule and its role in the production and prolongation of the disease processes in Parkinson's Disease.
One of the life-threatening diseases world-wide is Malaria caused by the bite of female anopheles’ mosquitoes. There are 5 such parasite species that affect humans of which 2 of these viz., P. falciparum and P. vivax are the greatest threats. These two deadliest parasites are prevalent in African continent and Saharan Africa. It is an acute febrile illness which causes rapid onset of fever with headache and chills only after 10 to 15 days after the mosquito bite. If the symptoms are not treated immediately, it will lead to fatal in just one day [1].
In the year 2020, there was a high risk for nearly half the world population of contracting malaria. Some population groups with high risk include infants, and young children, pregnant women, and people with low immunity.
A considerable number of mortality and complications of morbidity is caused by malaria that has become a major health problem globally. As the resistant parasite strains emerge, the therapeutic options are limited which has led to the large spread of malaria. This can be prevented by potential public health emergency in designing new drugs, providing single dose cures and by introducing some novel mechanism of action. New excipients may be included in the medicine that could be effective in fighting the new variants of the strain. Using the available genomic techniques, advancement of the study of biology of the parasites can be developed for the new therapy.
In recent years, various advanced drug interventions have been revealed. This focusses on the discovery of antimalarial agents using the latest scientific and technological advances. There are many antimalarial targets that include proteins such as proteases, plasmodium sugar, farnesyltransferase inhibitor and DNA replication. World malaria report shows an increase of cases from 2019 to 2020 and an increase of almost 69,000 deaths in 2020 compared to 2019 [2].
To test the molecular properties in a laboratory consumes more time and money. This inconvenience can be avoided using quantitative structure activity relationship models to forecast different properties of various chemical compounds using topological descriptors. Topological indices find significant applications in various areas of mathematical chemistry [3,4] such as isomer discrimination, chirality, molecular complexity, drug design, selection of database, QSAR/QSPR/QSTR studies in the recent years [5].
The main objectives of this study are:
● QSPR analysis of the properties of the topological indices using regression models for the nine anti-malaria drugs.
● Computation of topological indices for the considered drugs.
● Comparison of topological indices with correlation coefficients of few physical properties and statistical parameters.
Identifying drug-like from non-drug-like molecules is very much necessary to reduce the cost and time with failed drug development. There are various approaches that screen chemical databases against biological targets in the progress of new potential leads. QSAR modelling is a significant approach in the discovery of drug that correlates the structure of the molecule with biological activities. There are different methods like 2D (topological indices) and 3D (structure-based) of which 2D requires less calculation time and hence used for the preliminary screening of the drug development. Academia, industry, and research institutions across the globe, widely use 2D approach.
The principal step involves the selection of the right descriptor for various reasons. Only few descriptorsgive a better understanding of the results and their interpretability, can reduce the risk of overfitting from redundant descriptors, and provides speedy and cost-effective models.
Topological index represents translations of molecular structures into structural descriptors that are expressed as numerical indices. These topological indices find their applications in drug design where the QSAR/QSPR/QSTR studies are involved. The QSAR/QSPR methods are based on the assumption that the activity or the property, such as a drug binding to DNA or toxic effect, of a certain chemical compound related to its structure through a certain mathematical algorithm.
For the chemical compound under the study, a series of parameters, called chemical descriptors are computed. Then, an algorithm that provides a quite accurate value, similar to theoretical experimental value is found. The final step is to check if the obtained algorithm is capable of predicting the activity/property values.
QSAR/QSPR/QSTR models are used to predict the association between the molecular structure and its activity/property/toxicity. Over the years many algorithms have been proposed and applied in QSAR/QSPR studies. The model framework includes molecular structure (graph) representation, calculation of molecular descriptors (graph invariants) and multiple linear regression method. The model will be validated through statistical parameters (r and r2). The same approach is employed in this work and the statistical parameters have shown significant results.
Recently, Adnan et. al. made a study on the QSPR analysis of anti tuberculosis drugs in which 15 drugs were considered for the regression analysis of 6 physico chemical properties. Eleven topological indices were computed for which the correlation analysis showed a high positive good correlation with all the 6 physico chemical properties. Shanmukha et.al. studied 21 breast cancer drugs for which QSPR analysis were carried out. Eleven topological indices were studied in the study and linear models for each index were carried out. In 2020, Sigarreta [6,7] provided new tools that obtained a unified way inequalities involving many different topological indices. Joseobtained new optimal bounds on the variable Zagreb indices, the variable sum-connectivity index, the variable geometric-arithmetic index and the variable inverse sum indeg index. Recently, Jose computed new lower and upper optimal bounds for general (exponential) indices of a graph. In the same direction, new inequalities involving some well-known topological indices like the generalized atom-bound connectivity index and the generalized second Zagreb index were shown. Some extremal problems for their corresponding exponential indices were computed.
The numerical representation of the arrangement of a molecule of a compound refers to topological index. Wiener index was one of the first topological indices proposed by Harold Wiener in 1947 which proved that his index correlated well with the boiling points of alkanes. At present, there are various indices introduced by various researchers which are divided into three categories, namely, degree-based, neighborhood degree-based and distance based topological indices [8,9,10]. Sixty years ago, QSAR was introduced that included modelling of biological activities of molecules which was extended to modelling of few physicochemical properties of molecules leading to QSPR studies. Initially, it was chemical interpretation of topological indices [11,12] which has now extended to modelling of structural characteristics of molecules with their biological activity leading to complex modelling of compounds in QSAR/QSPR/QSTR studies [13,14,15,16,17,18,19,20,21,22,23,24,25].
This model does not require any lab equipment to perform the analysis. It saves a lot of time and money and the results obtained using the QSPR model are compared with the actual values for further analysis.
A graph G(V, E) with vertex set V(G) and edge set E(G) is connected if there exists an edge between every pair of vertices in G.
The graphs used in this work are simple graphs and have no loops and multiple edges. The number of edges incident to vertex v is the degree of the vertex v, denoted by d_v. For graph terminologies and notations refer [26,27].
Some of the topological descriptors which are used in this work are given below.
The earliest set of topological indices are the first and the second version of Zagreb indices. They have been found impressive in finding the total π-electron energy of molecules. Gutman and Polansky [28] introduced these indices in the year 1986 and are defined as follows
M1(G)=∑vw∈E(G)(dv+dw) | (1) |
M2(G)=∑vw∈E(G)(dv×dw) | (2) |
Harmonic index is proposed and defined by Fatjlowicz [29] as
H(G)=∑vw∈E(G)2(dv+dw) | (3) |
Forgotten index was first defined by Furtula and Gutman [30] in 2015. It became popular as its performance in prediction of the index analogues to that of the original Zagreb index and is defined as
F(G)=∑vw∈E(G)(dv)2+(dw)2 | (4) |
A novel graph invariant called the SS index of a graph is proposed by Zhao et al. [31] and is stated as
SS(G)=∑vw∈E(G)√dv×dwdv+dw | (5) |
Ranjini et al. [32] introduced redefined version of the second and third Zagreb indices and are defined as
ReZG2(G)=∑vw∈E(G)dv×dwdv+dw | (6) |
ReZG3(G)=∑vw∈E(G)(dv×dw)(dv+dw) | (7) |
In this study, anti-malaria drugs are modelled by simple graphs. To compute the TIs of the considered drug's structure, the employed methods are vertex partitioning, edge partitioning, neighbourhood vertex partitioning and computational techniques. For depicting the results in terms of graphs and verification of computed results, the work is equipped with SPSS17 version.
In this work, degree-based topological indices are computed for the drugs used in the treatment of malaria. The QSPR analysis of the computed indices are discussed, and it is observed that these indices are highly correlated with the physico chemical properties of the drugs that are used in the treatment of malaria.
The nine drugs viz., Chloroquine, Amodiaquine, Mefloquine, Piperaquine, Primaquine, Lumefrantrine, Atovaquone, Pyrimethamine and Doxycycline used in the malaria treatment are considered for the analysis. The molecular structures of these drugs are shown in Figure 1. It is modelled as a graph where the atoms are referred to as vertices while the bonds by its edges which is represented in Figure 2.
The six physical properties of the drugs viz., boiling point, enthalpy, flash point, molar refraction, molar volume and polarizability for the 9 drugs are studied. A linear regression model used in this work is given below.
P=A+B[TI] | (8) |
where P→ Physical property of the drug, A,B→ constants, TI→ topological descriptor.
Using the linear regression equation discussed, the regression model for the above considered topological indices are defined.
The physical properties of anti-malaria drugs are considered as dependent variables and the topological indices for molecular graphs of 9 drugs are considered as independent variables. A linear regression model is fitted using SPSS software where the constants A and B in the regression equation (8) are calculated by using the training set in Tables 1 and 2.
Drugs | BP (℃ at 760 mmHg) | Enthalpy (kj/mol) | Flash point (℃) | Molar refraction | Molar volume (cm3) | Polarizability |
Chloroquine | 460.6 | 72.1 | 232.23 | 97.4 | 287.9 | 38.6 |
Amodiaquine | 478 | 77 | 242.9 | 105.5 | 282.8 | 41.8 |
Mefloquine | 415.7 | 70.5 | 205.2 | 83 | 273.4 | 32.9 |
Piperoquine | 721.1 | 105.3 | 389.9 | 153.7 | 414.2 | 60.9 |
Primaquine | 451.1 | 71 | 226.6 | 80.5 | 230.3 | 31.9 |
Lumefrantrine | 642.5 | 99.6 | 342.3 | 151 | 422.3 | 59.9 |
Atovaquine | 535 | 85.4 | 277.3 | 99.5 | 271.8 | 39.5 |
Pyrimethamine | 368.4 | 61.5 | 176.6 | 67.1 | 180.2 | 26.6 |
Doxycycline | 762.6 | 116.5 | 415 | 109 | 271.1 | 43.2 |
Drugs | M1(G) | M2(G) | H(G) | F(G) | SS(G) | ReZG2(G) | ReZG3(G) |
Chloroquine | 106 | 120 | 10.3 | 262 | 23.9599 | 25.2333 | 584 |
Amodiaquine | 128 | 149 | 11.7333 | 324 | 28.5664 | 30.5333 | 742 |
Mefloquine | 114 | 137 | 9.5857 | 306 | 24.5829 | 26.631 | 728 |
Piperoquine | 202 | 240 | 17.8 | 510 | 45.1804 | 48.9 | 1200 |
Primaquine | 92 | 105 | 9 | 226 | 20.903 | 22.0833 | 512 |
Lumefrantrine | 182 | 213 | 16.3666 | 466 | 40.3883 | 43.4333 | 1090 |
Atovaquine | 158 | 194 | 12.6047 | 446 | 33.4116 | 36.5619 | 1064 |
Pyrimethamine | 86 | 100 | 7.7667 | 222 | 18.9478 | 20.2167 | 506 |
Doxycycline | 200 | 264 | 14.4164 | 608 | 40.4521 | 45.55 | 1594 |
Using the linear regression equation discussed, the regression model for the above considered topological indices are defined.
Boilingpoint=124.096+2.932[M1(G)] |
Enthalpy=49.292+0.228[M1(G)] |
Flashpoint=28.813+1.773[M1(G)] |
MolarRefraction=28.194+0.546[M1(G)] |
MolarVolume=114.389+1.265[M1(G)] |
Polarizability=11.164+0.217[M1(G)] |
Boilingpoint=161.996+2.219[M2(G)] |
Enthalpy=33.198+0.302[M2(G)] |
Flashpoint=51.729+1.342[M2(G)] |
MolarRefraction=41.872+0.374[M2(G)] |
MolarVolume=150.949+0.838[M2(G)] |
Polarizability=16.589+0.148[M2(G)] |
Boilingpoint=87.905+36.905[H(G)] |
Enthalpy=25.031+4.870[H(G)] |
Flashpoint=6.939+22.319[H(G)] |
MolarRefraction=3.348+8.365[H(G)] |
MolarVolume=44.044+20.421[H(G)] |
Polarizability=1.318+3.317[H(G)] |
Boilingpoint=177.821+0.960[F(G)] |
Enthalpy=34.961+0.132[F(G)] |
Flashpoint=61.301+0.581[F(G)] |
MolarRefraction=48.115+0.152[F(G)] |
MolarVolume=167.744+0.334[F(G)] |
Polarizability=19.061+0.060[F(G)] |
Boilingpoint=111.354+13.867[SS(G)] |
Enthalpy=27.343+1.855[SS(G)] |
Flashpoint=21.110+8.387[SS(G)] |
MolarRefraction=18.858+2.811[SS(G)] |
MolarVolume=87.819+6.670[SS(G)] |
Polarizability=7.465+1.115[SS(G)] |
Boilingpoint=123.738+12.440[RezG2(G)] |
Enthalpy=28.782+1.671[RezG2(G)] |
Flashpoint=28.597+7.524[RezG2(G)] |
MolarRefraction=24.545+2.426[RezG2(G)] |
MolarVolume=103.449+5.693[RezG2(G)] |
Polarizability=9.720+0.962[RezG2(G)] |
Boilingpoint=223.151+0.352[RezG3(G)] |
Enthalpy=40.942+0.049[RezG3(G)] |
Flashpoint=88.713+0.213[RezG3(G)] |
MolarRefraction=59.754+0.051[RezG3(G)] |
MolarVolume=196.894+0.107[RezG3(G)] |
Polarizability=23.678+0.020[RezG3(G)] |
In Table 1, six physical properties of the anti-malaria drugs considered in the study are presented. Various degree-based topological indices mentioned above are computed and tabulated in Table 2. The correlation of the indices in this study against all the six physical properties are tabulated in Table 3. It is observed that the indices and their corresponding physical properties are highly correlated for most of the properties and the correlation coefficient of second Zagreb index with enthalpy being the highest value of correlation (0.978). The correlation coefficients of the discussed topological indices with physical properties and graphically presented in Figure 3. The statistical parameters viz., the sample size (N), constant (A), slope (b), correlation coefficient (r), the percentage of the dependent variable r2, and p-value for the QSPR study for all the above seven topological indices are studied. The null hypothesis is tested for p-value of each term is where the coefficient equals zero while the higher the p-value infers those changes in predictor are not related to changes in response. In this case, all the regression coefficients of a null hypothesis are zero while testing gives rise to F value. In such a case, the model does not have predictive capability. Using this test, one can compare their model with zero predictor variables to decide their coefficients improve the model.
Drugs | BP | Enthalpy | Flash point | Molar refraction | Molar volume | Polarizability |
M1(G) | 0.961 | 0.968 | 0.961 | 0.838 | 0.735 | 0.838 |
M2(G) | 0.962 | 0.978 | 0.963 | 0.76 | 0.645 | 0.76 |
H(G) | 0.908 | 0.894 | 0.908 | 0.963 | 0.891 | 0.964 |
F(G) | 0.947 | 0.969 | 0.947 | 0.704 | 0.584 | 0.704 |
SS(G) | 0.948 | 0.945 | 0.948 | 0.899 | 0.809 | 0.899 |
ReZG2(G) | 0.957 | 0.958 | 0.957 | 0.873 | 0.776 | 0.873 |
ReZG3(G) | 0.934 | 0.962 | 0.934 | 0.632 | 0.505 | 0.633 |
Tables 4–10 represent the statistical parameters such as number of drugs considered, constant, regression coefficient, correlation coefficient, Fisher’s statistic, significant value, and standard error denoted by N, A, b, r, r2, F and p respectively, for all the considered topological indices and physical properties. Table 11 denotes the standard error of estimate for physical properties of drugs. Tables 12–17 denote the comparison of actual and computed values of all physical properties of anti-malaria drugs. Figure 3 depicts the graphical representation of physical properties and the topological indices.
Phy. Pro, | N | A | b | r | r2 | F | p | Indicator |
BP | 9 | 124.096 | 2.932 | 0.961 | 0.923 | 83.956 | 0 | Significant |
EN | 9 | 28.483 | 0.396 | 0.968 | 0.937 | 104.502 | 0 | Significant |
FP | 9 | 29.813 | 1.773 | 0.961 | 0.923 | 83.973 | 0 | Significant |
MR | 9 | 28.194 | 0.546 | 0.838 | 0.702 | 16.501 | 0.05 | Significant |
MV | 9 | 114.389 | 1.265 | 0.735 | 0.541 | 8.244 | 0.02 | Significant |
PO | 9 | 11.164 | 0.217 | 0.838 | 0.702 | 16.529 | 0 | Significant |
Phy. Pro, | N | A | b | r | r2 | F | p | Indicator |
BP | 9 | 161.996 | 2.219 | 0.962 | 0.926 | 88.088 | 0 | Significant |
EN | 9 | 33.198 | 0.302 | 0.978 | 0.956 | 151.468 | 0 | Significant |
FP | 9 | 51.729 | 1.342 | 0.963 | 0.926 | 88.162 | 0 | Significant |
MR | 9 | 41.872 | 0.374 | 0.76 | 0.578 | 9.575 | 0.01 | Significant |
MV | 9 | 150.949 | 0.838 | 0.645 | 0.416 | 4.982 | 0.06 | Significant |
PO | 9 | 16.589 | 0.148 | 0.76 | 0.578 | 9.588 | 0.01 | Significant |
Phy. Pro, | N | A | b | r | r2 | F | p | Indicator |
BP | 9 | 87.905 | 36.905 | 0.908 | 0.825 | 33.003 | 0.01 | Significant |
EN | 9 | 25.031 | 4.87 | 0.894 | 0.798 | 27.731 | 0 | Significant |
FP | 9 | 60939 | 22.319 | 0.908 | 0.825 | 32.986 | 0 | Significant |
MR | 9 | 3.348 | 8.365 | 0.963 | 0.928 | 90.501 | 0 | Significant |
MV | 9 | 44.044 | 20.421 | 0.891 | 0.795 | 27.106 | 0 | Significant |
PO | 9 | 1.318 | 3.317 | 0.964 | 0.928 | 90.704 | 0 | Significant |
Phy. Pro, | N | A | b | r | r2 | F | p | Indicator |
BP | 9 | 177.821 | 0.96 | 0.947 | 0.897 | 60.643 | 0 | Significant |
EN | 9 | 34.961 | 0.132 | 0.969 | 0.94 | 109.495 | 0 | Significant |
FP | 9 | 61.301 | 0.581 | 0.947 | 0.897 | 60.678 | 0 | Significant |
MR | 9 | 48.115 | 0.152 | 0.704 | 0.495 | 6.865 | 0.03 | Significant |
MV | 9 | 167.744 | 0.334 | 0.584 | 0.341 | 3.619 | 0.09 | Significant |
PO | 9 | 19.061 | 0.06 | 0.704 | 0.496 | 6.877 | 0.03 | Significant |
Phy. Pro, | N | A | b | r | r2 | F | p | Indicator |
BP | 9 | 111.354 | 13.867 | 0.948 | 0.898 | 61.748 | 0 | Significant |
EN | 9 | 27.343 | 1.855 | 0.945 | 0.894 | 58.818 | 0 | Significant |
FP | 9 | 21.11 | 8.387 | 0.948 | 0.898 | 61.739 | 0 | Significant |
MR | 9 | 18.858 | 2.811 | 0.899 | 0.808 | 29.522 | 0 | Significant |
MV | 9 | 87.819 | 6.67 | 0.809 | 0.654 | 13.222 | 0 | Significant |
PO | 9 | 7.465 | 1.115 | 0.899 | 0.809 | 29.572 | 0 | Significant |
Phy. Pro, | N | A | b | r | r2 | F | p | Indicator |
BP | 9 | 123.738 | 12.44 | 0.957 | 0.915 | 75.323 | 0 | Significant |
EN | 9 | 28.782 | 1.671 | 0.958 | 0.918 | 77.887 | 0 | Significant |
FP | 9 | 28.597 | 7.524 | 0.957 | 0.915 | 75.331 | 0 | Significant |
MR | 9 | 24.545 | 2.426 | 0.873 | 0.762 | 22.437 | 0 | Significant |
MV | 9 | 103.449 | 5.693 | 0.776 | 0.603 | 10.626 | 0.01 | Significant |
PO | 9 | 9.72 | 0.962 | 0.873 | 0.762 | 22.47 | 0 | Significant |
Phy. Pro, | N | A | b | r | r2 | F | p | Indicator |
BP | 9 | 223.151 | 0.352 | 0.934 | 0.873 | 47.933 | 0 | Significant |
EN | 9 | 40.942 | 0.049 | 0.962 | 0.925 | 86.586 | 0 | Significant |
FP | 9 | 88.713 | 0.213 | 0.934 | 0.873 | 47.97 | 0 | Significant |
MR | 9 | 59.754 | 0.051 | 0.632 | 0.4 | 4.665 | 0.05 | Significant |
MV | 9 | 196.894 | 0.107 | 0.505 | 0.255 | 2.4 | 0.05 | Significant |
PO | 9 | 23.678 | 0.02 | 0.633 | 0.4 | 4.671 | 0.05 | Significant |
Drugs | BP | Enthalpy | Flash point | Molar refraction | Molar volume | Polarizability |
M1(G) | 41.3818 | 5.0133 | 25.0251 | 17.3965 | 56.9863 | 6.8934 |
M2(G) | 40.4727 | 402053 | 24.4681 | 20.7148 | 64.279 | 8.21 |
H(G) | 62.3993 | 8.9827 | 32.985 | 8.5408 | 38.0989 | 3.3828 |
F(G) | 47.9859 | 4.9047 | 29.014 | 22.6488 | 68.2796 | 8.9761 |
SS(G) | 47.5989 | 6.6252 | 28.7891 | 13.955 | 49.478 | 5.5292 |
ReZG2(G) | 43.4975 | 5.7457 | 26.3056 | 15.5439 | 52.9971 | 6.1595 |
ReZG3(G) | 53.2487 | 5.4722 | 32.1935 | 24.6926 | 72.5723 | 9.7875 |
Drug | Boiling point ℃ (at 760 mmHg) | M1(G) | M2(G) | H(G) | F(G)) | SS(G) | ReZG2(G) | ReZG3(G) |
Chloroquine | 460.6 ± 40.0 | 434.88 | 428.27 | 468.02 | 429.341 | 443.60 | 437.64 | 428.71 |
Amodiaquine | 478.0 ± 45.0 | 499.39 | 492.62 | 520.92 | 488.861 | 507.48 | 503.57 | 484.33 |
Mefloquine | 415.7 ± 40.0 | 458.34 | 465.99 | 441.66 | 471.581 | 452.24 | 455.02 | 479.40 |
Piperoquine | 721.1 ± 60.0 | 716.36 | 694.55 | 744.81 | 667.421 | 737.87 | 732.05 | 645.55 |
Primaquine | 451.1 ± 45.0 | 393.84 | 394.99 | 420.05 | 394.781 | 401.21 | 398.45 | 403.37 |
Lumefrantrine | 642.5 ± 55.0 | 657.72 | 634.64 | 691.91 | 625.181 | 671.41 | 664.04 | 606.83 |
Atovaquine | 535.0 ± 50.0 | 587.35 | 592.48 | 553.08 | 605.981 | 574.67 | 578.56 | 597.67 |
Pyrimethamine | 368.4 ± 52.0 | 376.24 | 383.89 | 374.53 | 390.941 | 374.10 | 375.23 | 401.26 |
Doxycycline | 762.6 ± 60.0 | 710.49 | 747.81 | 619.94 | 761.501 | 672.30 | 690.38 | 784.23 |
Drug | Flash point (℃) | M1(G) | M2(G) | H(G) | F(G)) | SS(G) | ReZG2(G) | ReZG3(G) |
Chloroquine | 232.23 ± 27.3 | 216.75 | 212.76 | 236.82 | 213.52 | 222.06 | 218.45 | 213.10 |
Amodiaquine | 242.9 ± 28.7 | 255.75 | 251.68 | 268.81 | 249.54 | 260.69 | 258.32 | 246.75 |
Mefloquine | 205.2 ± 27.3 | 230.93 | 235.58 | 220.88 | 239.08 | 227.28 | 228.96 | 243.77 |
Piperoquine | 389.9 ± 32.9 | 386.95 | 373.80 | 404.21 | 357.61 | 400.03 | 396.52 | 344.31 |
Primaquine | 226.6 ± 28.7 | 191.92 | 192.63 | 207.81 | 192.60 | 196.42 | 194.75 | 197.76 |
Lumefrantrine | 342.3 ± 31.5 | 351.49 | 337.57 | 372.22 | 332.04 | 359.84 | 355.38 | 320.88 |
Atovaquine | 277.3 ± 30.1 | 308.94 | 312.07 | 288.26 | 320.42 | 301.33 | 303.68 | 315.34 |
Pyrimethamine | 176.6 ± 30.7 | 181.29 | 185.92 | 180.28 | 190.28 | 180.02 | 180.70 | 196.49 |
Doxycycline | 415.0 ± 32.9 | 383.41 | 406.01 | 328.69 | 414.54 | 360.38 | 371.31 | 428.23 |
Drug | Enthalpy (kj/mol) | M1(G) | M2(G) | H(G) | F(G)) | SS(G) | ReZG2(G) | ReZG3(G) |
Chloroquine | 72.1 ± 3.0 | 73.46 | 69.438 | 75.19 | 69.545 | 71.78 | 70.94 | 69.55 |
Amodiaquine | 77.0 ± 3.0 | 78.476 | 78.19 | 82.17 | 77.72 | 80.33 | 79.80 | 77.3 |
Mefloquine | 70.5 ± 3.0 | 75.28 | 74.57 | 71.71 | 75.35 | 72.94 | 73.28 | 76.61 |
Piperoquine | 105.3 ± 3.0 | 95.34 | 105.67 | 111.71 | 102.28 | 111.15 | 110.49 | 99.74 |
Primaquine | 71.0 ± 3.0 | 70.268 | 64.90 | 68.86 | 64.79 | 66.11 | 65.68 | 66.03 |
Lumefrantrine | 99.6 ± 3.0 | 90.788 | 97.52 | 104.73 | 96.47 | 102.26 | 101.35 | 94.35 |
Atovaquine | 85.4 ± 3.0 | 85.316 | 91.78 | 86.41 | 93.83 | 89.31 | 89.87 | 93.07 |
Pyrimethamine | 61.5 ± 3.0 | 68.9 | 63.39 | 62.85 | 64.26 | 62.48 | 62.56 | 65.73 |
Doxycycline | 116.5 ± 3.0 | 94.892 | 112.92 | 95.23 | 115.21 | 102.37 | 104.89 | 119.04 |
Drug | Molar refraction (cm3) | M1(G) | M2(G) | H(G) | F(G)) | SS(G) | ReZG2(G) | ReZG3(G) |
Chloroquine | 97.4 ± 0.3 | 86.07 | 86.75 | 89.50 | 87.93 | 86.20 | 85.76 | 89.53 |
Amodiaquine | 105.5 ± 0.3 | 98.08 | 97.59 | 101.49 | 97.36 | 99.15 | 98.61 | 97.59 |
Mefloquine | 83.0 ± 0.3 | 90.43 | 93.11 | 83.53 | 94.62 | 87.96 | 89.15 | 96.88 |
Piperoquine | 153.7 ± 0.3 | 138.48 | 131.63 | 152.24 | 125.63 | 145.86 | 143.17 | 120.95 |
Primaquine | 80.5 ± 0.3 | 78.42 | 81.14 | 78.63 | 82.46 | 77.61 | 78.11 | 85.86 |
Lumefrantrine | 151.0 ± 0.3 | 127.56 | 121.53 | 140.25 | 118.94 | 132.38 | 129.91 | 115.34 |
Atovaquine | 99.5 ± 0.3 | 114.46 | 114.42 | 108.78 | 115.90 | 112.77 | 113.24 | 114.01 |
Pyrimethamine | 67.1 ± 0.5 | 75.15 | 79.27 | 68.31 | 81.85 | 72.12 | 73.59 | 85.56 |
Doxycycline | 109 ± 0.4 | 137.39 | 140.60 | 123.94 | 140.53 | 132.56 | 135.04 | 141.04 |
Drug | Molar volume (cm3) | M1(G) | M2(G) | H(G) | F(G)) | SS(G) | ReZG2(G) | ReZzG3(G) |
Chloroquine | 287.9 ± 3.0 | 248.47 | 251.509 | 254.38 | 255.25 | 247.63 | 247.10 | 259.38 |
Amodiaquine | 282.8 ± 3.0 | 276.30 | 275.811 | 283.64 | 275.96 | 278.35 | 277.27 | 276.28 |
Mefloquine | 273.4 ± 3.0 | 258.59 | 265.755 | 239.79 | 269.94 | 251.78 | 255.05 | 274.79 |
Piperoquine | 414.2 ± 3.0 | 369.91 | 352.069 | 407.53 | 338.08 | 389.17 | 381.83 | 325.29 |
Primaquine | 230.3 ± 3.0 | 230.76 | 238.939 | 227.83 | 243.22 | 227.24 | 229.16 | 251.67 |
Lumefrantrine | 422.3 ± 3.0 | 344.61 | 329.443 | 378.26 | 323.38 | 357.20 | 350.71 | 313.52 |
Atovaquine | 271.8 ± 3.0 | 314.25 | 313.521 | 301.44 | 316.70 | 310.67 | 311.59 | 310.74 |
Pyrimethamine | 180.2 ± 7.0 | 223.17 | 234.749 | 202.64 | 241.89 | 214.20 | 218.54 | 251.03 |
Doxycycline | 271.1 ± 5.0 | 367.38 | 372.181 | 338.44 | 370.81 | 357.63 | 362.76 | 367.45 |
Drug | Polarizability (10-24 cm3) | M1(G) | M2(G) | H(G) | F(G)) | SS(G) | ReZG2(G) | ReZG3(G) |
Chloroquine | 38.6 ± 0.5 | 34.16 | 34.34 | 35.48 | 34.78 | 34.18 | 33.99 | 35.35 |
Amodiaquine | 41.8 ± 0.5 | 38.94 | 38.64 | 40.23 | 38.50 | 39.31 | 39.09 | 38.51 |
Mefloquine | 32.9 ± 0.5 | 35.90 | 36.86 | 33.11 | 37.42 | 34.87 | 35.33 | 38.23 |
Piperoquine | 60.9 ± 0.5 | 54.99 | 52.10 | 60.36 | 49.66 | 57.84 | 56.76 | 47.67 |
Primaquine | 31.9 ± 0.5 | 31.12 | 32.12 | 31.17 | 32.62 | 30.77 | 30.96 | 33.91 |
Lumefrantrine | 59.9 ± 0.5 | 50.65 | 48.11 | 55.60 | 47.02 | 52.49 | 51.50 | 45.47 |
Atovaquine | 39.5 ± 0.5 | 45.45 | 45.30 | 43.12 | 45.82 | 44.71 | 44.89 | 44.95 |
Pyrimethamine | 26.6 ± 0.5 | 29.82 | 31.38 | 27.08 | 32.38 | 28.59 | 29.16 | 33.79 |
Doxycycline | 43.2 ± 0.5 | 54.56 | 55.66 | 49.13 | 55.54 | 52.56 | 53.53 | 55.55 |
In this study, seven degree-based topological indices are computed for nine anti-malaria drugs which is presented in Table 2. The coefficient of correlation for the said indices against six physical properties are computed and tabulated in Table 3. It is observed from Table 3 that every topological index shows good correlation with its corresponding physical properties in which, considering second Zagreb index row wise, the correlation coefficient is high with that of enthalpy for M2(G). The first Zagreb index has a high correlation with enthalpy of 0.963. The Harmonic index shows good correlation with polarizability, Forgotten index with enthalpy having r = 0.969, SS index has highest correlation with boiling point and flash point, both being 0.948, while the redefined Zagreb indices with enthalpy having 0.947, 0.958 and 0.962 respectively for the three indices.
This work focusses on the drugs used for the treatment of malaria which is caused by mosquitoes. The analysis done in this paper may help the chemists and pharmaceutical industry researchers to design novel drugs using various excipients by the values of indices computed here. They may constitute different drugs for various ailments based on the available topological indices in the article. The correlation coefficients of various drugs help the chemists to choose the right composition based on the high correlation value to form a new drug for novel ailments. New tools to obtain a unified way of involving inequalities of various topological indices may be carried out. The extremal values for the considered indices may be computed for exponential version of the indices.
The author declare that they have no conflict of interest.
[1] | Tanner CM, Kamel F, Ross GW, et al. (2011) Rotenone, paraquat, and Parkinson's disease. Environ Health Perspect 119: 866-872. https://doi.org/10.1289/ehp.1002839 |
[2] | Nonnekes J, Post B, Tetrud JW, et al. (2018) MPTP-induced parkinsonism: an historical case series. Lancet Neurol 17: 300-301. https://doi.org/10.1016/S1474-4422(18)30072-3 |
[3] | Dorsey ER, Zafar M, Lettenberger SE, et al. (2023) Trichloroethylene: An Invisible Cause of Parkinson's Disease?. J Parkinsons Dis 13: 203-218. https://doi.org/10.3233/JPD-225047 |
[4] | Pyatha S, Kim H, Lee D, et al. (2022) Association between Heavy Metal Exposure and Parkinson's Disease: A Review of the Mechanisms Related to Oxidative Stress. Antioxidants (Basel) 11: 2467. https://doi.org/10.3390/antiox11122467 |
[5] | Molina-Infante J, Santolaria S, Montoro M, et al. (2014) Sensibilidad al gluten no celiaca: una revisión crítica de la evidencia actual [Non-celiac gluten sensitivity: a critical review of current evidence]. Gastroenterol Hepatol 37: 362-371. https://doi.org/10.1016/j.gastrohep.2014.01.005 |
[6] | Hadjivassiliou M, Sanders DS, Woodroofe N, et al. (2008) Gluten ataxia. Cerebellum 7: 494-498. https://doi.org/10.1007/s12311-008-0052-x |
[7] | Obrenovich MEM (2018) Leaky Gut, Leaky Brain?. Microorganisms 6: 107. https://doi.org/10.3390/microorganisms6040107 |
[8] | Pennisi M, Bramanti A, Cantone M, et al. (2017) Neurophysiology of the “Celiac Brain”: Disentangling Gut-Brain Connections. Front Neurosci 11: 498. https://doi.org/10.3389/fnins.2017.00498 |
[9] | Philip A, White ND (2022) Gluten, Inflammation, and Neurodegeneration. Am J Lifestyle Med 16: 32-35. https://doi.org/10.1177/15598276211049345 |
[10] | Bella R, Lanza G, Cantone M, et al. (2015) Effect of a Gluten-Free Diet on Cortical Excitability in Adults with Celiac Disease. PLoS One 10: e0129218. https://doi.org/10.1371/journal.pone.0129218 |
[11] | Shalev H, Serlin Y, Friedman A (2009) Breaching the blood-brain barrier as a gate to psychiatric disorder. Cardiovasc Psychiatry Neurol 2009: 278531. https://doi.org/10.1155/2009/278531 |
[12] | Kordower JH, Olanow CW, Dodiya HB, et al. (2013) Disease duration and the integrity of the nigrostriatal system in Parkinson's disease. Brain 136: 2419-2431. https://doi.org/10.1093/brain/awt192 |
[13] | Uemura N, Yagi H, Uemura MT, et al. (2018) Inoculation of α-synuclein preformed fibrils into the mouse gastrointestinal tract induces Lewy body-like aggregates in the brainstem via the vagus nerve. Mol Neurodegener 13: 21. https://doi.org/10.1186/s13024-018-0257-5 |
[14] | Borghammer P (2018) How does parkinson's disease begin? Perspectives on neuroanatomical pathways, prions, and histology. Mov Disord 33: 48-57. https://doi.org/10.1002/mds.27138 |
[15] | Böttner M, Fricke T, Müller M, et al. (2015) Alpha-synuclein is associated with the synaptic vesicle apparatus in the human and rat enteric nervous system. Brain Res 1614: 51-59. https://doi.org/10.1016/j.brainres.2015.04.015 |
[16] | Schrag A, Horsfall L, Walters K, et al. (2015) Prediagnostic presentations of Parkinson's disease in primary care: a case-control study. Lancet Neurol 14: 57-64. https://doi.org/10.1016/S1474-4422(14)70287-X |
[17] | Williams-Gray CH, Worth PF (2016) Parkinson's disease. Medicine 44: 542-546. |
[18] | Balakireva AV, Zamyatnin AA (2016) Properties of Gluten Intolerance: Gluten Structure, Evolution, Pathogenicity and Detoxification Capabilities. Nutrients 8: 644. https://doi.org/10.3390/nu8100644 |
[19] | Nasserinejad M, Shojaee S, Ghobakhlou M, et al. (2019) The effects of IL-8, IL-6, and IL-1 on the risk of celiac disease: a Bayesian regression analysis. Gastroenterol Hepatol Bed Bench 12: S117-S122. |
[20] | Caio G, Volta U, Sapone A, et al. (2019) Celiac disease: a comprehensive current review. BMC Med 17: 142. https://doi.org/10.1186/s12916-019-1380-z |
[21] | Alaedini A, Okamoto H, Briani C, et al. (2007) Immune cross-reactivity in celiac disease: anti-gliadin antibodies bind to neuronal synapsin I. J Immunol 178: 6590-6595. https://doi.org/10.4049/jimmunol.178.10.6590 |
[22] | Vojdani A, Lerner A, Vojdani E (2021) Cross-Reactivity and Sequence Homology Between Alpha-Synuclein and Food Products: A Step Further for Parkinson's Disease Synucleinopathy. Cells 10: 1111. https://doi.org/10.3390/cells10051111 |
[23] | Lerner A, Benzvi C (2021) “Let Food Be Thy Medicine”: Gluten and Potential Role in Neurodegeneration. Cells 10: 756. https://doi.org/10.3390/cells10040756 |
[24] | Mohan M, Okeoma CM, Sestak K (2020) Dietary Gluten and Neurodegeneration: A Case for Preclinical Studies. Int J Mol Sci 21: 5407. https://doi.org/10.3390/ijms21155407 |
[25] | Chao YX, Gulam MY, Chia NSJ, et al. (2020) Gut-Brain Axis: Potential Factors Involved in the Pathogenesis of Parkinson's Disease. Front Neurol 11: 849. https://doi.org/10.3389/fneur.2020.00849 |
[26] | Millán Solano MV, Salinas Lara C, Sánchez-Garibay C, et al. (2023) Effect of Systemic Inflammation in the CNS: A Silent History of Neuronal Damage. Int J Mol Sci 24: 11902. https://doi.org/10.3390/ijms241511902 |
[27] | Dohan FC, Harper EH, Clark MH, et al. (1984) Is schizophrenia rare if grain is rare?. Biol Psychiatry 19: 385-399. |
[28] | Kelly DL, Demyanovich HK, Rodriguez KM, et al. (2019) Randomized controlled trial of a gluten-free diet in patients with schizophrenia positive for antigliadin antibodies (AGA IgG): a pilot feasibility study. J Psychiatry Neurosci 44: 269-276. https://doi.org/10.1503/jpn.180174 |
[29] | Levinta A, Mukovozov I, Tsoutsoulas C (2018) Use of a Gluten-Free Diet in Schizophrenia: A Systematic Review. Adv Nutr 9: 824-832. https://doi.org/10.1093/advances/nmy056 |
[30] | Genuis SJ, Lobo RA (2014) Gluten sensitivity presenting as a neuropsychiatric disorder. Gastroenterol Res Pract 2014: 293206. https://doi.org/10.1155/2014/293206 |
[31] | Carta MG, Conti A, Lecca F, et al. (2015) The Burden of Depressive and Bipolar Disorders in Celiac Disease. Clin Pract Epidemiol Ment Health 11: 180-185. https://doi.org/10.2174/1745017901511010180 |
[32] | Taraghikhah N, Ashtari S, Asri N, et al. (2020) An updated overview of spectrum of gluten-related disorders: clinical and diagnostic aspects. BMC Gastroenterol 20: 258. https://doi.org/10.1186/s12876-020-01390-0 |
[33] | Sapone A, Bai JC, Ciacci C, et al. (2012) Spectrum of gluten-related disorders: consensus on new nomenclature and classification. BMC Med 10: 13. https://doi.org/10.1186/1741-7015-10-13 |
[34] | Zhang S, Sicherer S, Berin MC, et al. (2021) Pathophysiology of Non-IgE-Mediated Food Allergy. Immunotargets Ther 10: 431-446. https://doi.org/10.2147/ITT.S284821 |
[35] | Di Lazzaro V, Capone F, Cammarota G, et al. (2014) Dramatic improvement of parkinsonian symptoms after gluten-free diet introduction in a patient with silent celiac disease. J Neurol 261: 443-445. https://doi.org/10.1007/s00415-014-7245-7 |
[36] | Appleton J (2018) The Gut-Brain Axis: Influence of Microbiota on Mood and Mental Health. Integr Med (Encinitas) 17: 28-32. |
[37] | Lynch CMK, Cowan CSM, Bastiaanssen TFS, et al. (2023) Critical windows of early-life microbiota disruption on behaviour, neuroimmune function, and neurodevelopment. Brain Behav Immun 108: 309-327. https://doi.org/10.1016/j.bbi.2022.12.008 |
[38] | Li Q, Li L, Li Q, et al. (2022) Influence of Natural Polysaccharides on Intestinal Microbiota in Inflammatory Bowel Diseases: An Overview. Foods 11: 1084. https://doi.org/10.3390/foods11081084 |
[39] | Liang J, Tan Y (2023) Highly efficient CRISPR-mediated base editing for the gut Bacteroides spp. with pnCasBS-CBE. Biotechnol J 18: e2200504. https://doi.org/10.1002/biot.202200504 |
[40] | Yuan XY, Chen YS, Liu Z (2024) Relationship among Parkinson's disease, constipation, microbes, and microbiological therapy. World J Gastroenterol 30: 225-237. https://doi.org/10.3748/wjg.v30.i3.225 |
[41] | Burke RE, Dauer WT, Vonsattel JP (2008) A critical evaluation of the Braak staging scheme for Parkinson's disease. Ann Neurol 64: 485-491. https://doi.org/10.1002/ana.21541 |
[42] | Zhang Y, Tan W, Sultonova RD, et al. (2023) Synergistic cancer immunotherapy utilizing programmed Salmonella typhimurium secreting heterologous flagellin B conjugated to interleukin-15 proteins. Biomaterials 298: 122135. https://doi.org/10.1016/j.biomaterials.2023.122135 |
[43] | Bascuñán KA, Araya M, Roncoroni L, et al. (2020) Dietary Gluten as a Conditioning Factor of the Gut Microbiota in Celiac Disease. Adv Nutr 11: 160-174. https://doi.org/10.1093/advances/nmz080 |
[44] | Qiao SW, Ráki M, Gunnarsen KS, et al. (2011) Posttranslational modification of gluten shapes TCR usage in celiac disease. J Immunol 187: 3064-3071. https://doi.org/10.4049/jimmunol.1101526 |
[45] | Caio G, Lungaro L, Segata N, et al. (2020) Effect of Gluten-Free Diet on Gut Microbiota Composition in Patients with Celiac Disease and Non-Celiac Gluten/Wheat Sensitivity. Nutrients 12: 1832. https://doi.org/10.3390/nu12061832 |
[46] | Terenzi D, Muth AK, Park SQ (2022) Nutrition and Gut-Brain Pathways Impacting the Onset of Parkinson's Disease. Nutrients 14: 2781. https://doi.org/10.3390/nu14142781 |
[47] | Scheperjans F, Aho V, Pereira PA, et al. (2015) Gut microbiota are related to Parkinson's disease and clinical phenotype. Mov Disord 30: 350-358. https://doi.org/10.1002/mds.26069 |
[48] | Alfonsetti M, Castelli V, d'Angelo M (2022) Are We What We Eat? Impact of Diet on the Gut-Brain Axis in Parkinson's Disease. Nutrients 14: 380. https://doi.org/10.3390/nu14020380 |
[49] | Daneman R (2012) The blood-brain barrier in health and disease. Ann Neurol 72: 648-672. https://doi.org/10.1002/ana.23648 |
[50] | Carabotti M, Scirocco A, Maselli MA, et al. (2015) The gut-brain axis: interactions between enteric microbiota, central and enteric nervous systems. Ann Gastroenterol 28: 203-209. |
[51] | Pisani V, Stefani A, Pierantozzi M, et al. (2012) Increased blood-cerebrospinal fluid transfer of albumin in advanced Parkinson's disease. J Neuroinflammation 9: 188. https://doi.org/10.1186/1742-2094-9-188 |
[52] | Klann EM, Dissanayake U, Gurrala A, et al. (2022) The Gut-Brain Axis and Its Relation to Parkinson's Disease: A Review. Front Aging Neurosci 13: 782082. https://doi.org/10.3389/fnagi.2021.782082 |
[53] | Di Sabatino A, Ciccocioppo R, Luinetti O, et al. (2003) Increased enterocyte apoptosis in inflamed areas of Crohn's disease. Dis Colon Rectum 46: 1498-1507. https://doi.org/10.1007/s10350-004-6802-z |
[54] | Giuffrè M, Moretti R (2023) The Gut-Liver-Brain Axis: From the Head to the Feet. Int J Mol Sci 24: 15662. https://doi.org/10.3390/ijms242115662 |
1. | Rongbing Huang, Abid Mahboob, Muhammad Waheed Rasheed, Sajid Mahboob Alam, Muhammad Kamran Siddiqui, On molecular modeling and QSPR analysis of lyme disease medicines via topological indices, 2023, 138, 2190-5444, 10.1140/epjp/s13360-023-03867-9 | |
2. | Muhammad Waheed Rasheed, Abid Mahboob, Iqra Hanif, An Estimation of the Physicochemical Properties of Heart Attack Treatment Medicines by Using Molecular Descriptors, 2023, 10269185, 10.1016/j.sajce.2023.04.003 | |
3. | Xiujun Zhang, Muhammad Jawwad Saif, Nazeran Idrees, Salma Kanwal, Saima Parveen, Fatima Saeed, QSPR Analysis of Drugs for Treatment of Schizophrenia Using Topological Indices, 2023, 8, 2470-1343, 41417, 10.1021/acsomega.3c05000 | |
4. | Dongming Zhao, Muhammad Farhan Hanif, Hasan Mahmood, Muhammad Kamran Siddiqui, Mazhar Hussain, Nazir Hussain, Topological analysis of entropy measure using regression models for silver iodide, 2023, 138, 2190-5444, 10.1140/epjp/s13360-023-04432-0 | |
5. | Mazhar Hussain, Muhammad Kamran Siddiqui, Muhammad Farhan Hanif, Hasan Mahmood, Zohaib Saddique, Samuel Asefa Fufa, On K-Banhatti indices and entropy measure for rhodium (III) chloride via linear regression models, 2023, 9, 24058440, e20935, 10.1016/j.heliyon.2023.e20935 | |
6. | Ali N. A. Koam, Muhammad Faisal Nadeem, Ali Ahmad, Hassan A. Eshaq, Weighted Asymmetry Index: A New Graph-Theoretic Measure for Network Analysis and Optimization, 2024, 12, 2227-7390, 3397, 10.3390/math12213397 | |
7. | Muhammad Waheed Rasheed, Abid Mahboob, Iqra Hanif, Uses of degree-based topological indices in QSPR analysis of alkaloids with poisonous and healthful nature, 2024, 12, 2296-424X, 10.3389/fphy.2024.1381887 | |
8. | Micheal Arockiaraj, J. Celin Fiona, Arul Jeya Shalini, Comparative Study of Entropies in Silicate and Oxide Frameworks, 2024, 16, 1876-990X, 3205, 10.1007/s12633-024-02892-2 | |
9. | Asad Ullah, Safina Jabeen, Shahid Zaman, Anila Hamraz, Summeira Meherban, Predictive potential of K‐Banhatti and Zagreb type molecular descriptors in structure–property relationship analysis of some novel drug molecules, 2024, 71, 0009-4536, 250, 10.1002/jccs.202300450 | |
10. | Tahreem Ashraf, Nazeran Idrees, Topological indices based VIKOR assisted multi-criteria decision technique for lung disorders, 2024, 12, 2296-2646, 10.3389/fchem.2024.1407911 | |
11. | Abid Mahboob, Muhammad Waheed Rasheed, Iqra Hanif, Laiba Amin, Abdu Alameri, Role of molecular descriptors in quantitative structure‐property relationship analysis of kidney cancer therapeutics, 2024, 124, 0020-7608, 10.1002/qua.27241 | |
12. | B. Vijayakumar, B. Karthikeyan, A. Nelson, K. Arputha Christy, Synthesis, characterisation and topological indices approach of hydrazone derivatives of disubstituted-2,6-diaryl piperidin-4-one, catalysed by CdO-SnO2 nanocatalyst, 2024, 1306, 00222860, 137843, 10.1016/j.molstruc.2024.137843 | |
13. | Abdul Hakeem, Nek Muhammad Katbar, Fazal Muhammad, Nisar Ahmed, On the molecular structure modelling of gamma graphyne and armchair graphyne nanoribbon via reverse degree-based topological indices, 2024, 122, 0026-8976, 10.1080/00268976.2023.2259510 | |
14. | Deepa Balasubramaniyan, Natarajan Chidambaram, Vignesh Ravi, Muhammad Kamran Siddiqui, QSPR analysis of anti‐asthmatic drugs using some new distance‐based topological indices: A comparative study, 2024, 124, 0020-7608, 10.1002/qua.27372 | |
15. | Ali Raza, Muhammad Mobeen Munir, Exploring spectrum-based descriptors in pharmacological traits through quantitative structure property (QSPR) analysis, 2024, 12, 2296-424X, 10.3389/fphy.2024.1348407 | |
16. | Muhammad Waheed Rasheed, Abid Mahboob, Iqra Hanif, On QSAR modeling with novel degree-based indices and thermodynamics properties of eye infection therapeutics, 2024, 12, 2296-2646, 10.3389/fchem.2024.1383206 | |
17. | Asma Jabeen, Shahzad Ahmad, Shahid Zaman, The study of regression model based on CoM-polynomial in blood cancer drug properties, 2024, 9, 26668181, 100648, 10.1016/j.padiff.2024.100648 | |
18. | Deepa Balasubramaniyan, Natarajan Chidambaram, On some neighbourhood degree-based topological indices with QSPR analysis of asthma drugs, 2023, 138, 2190-5444, 10.1140/epjp/s13360-023-04439-7 | |
19. | Abid Mahboob, Muhammad Waheed Rasheed, Aya Mohammed Dhiaa, Iqra Hanif, Laiba Amin, On quantitative structure-property relationship (QSPR) analysis of physicochemical properties and anti-hepatitis prescription drugs using a linear regression model, 2024, 10, 24058440, e25908, 10.1016/j.heliyon.2024.e25908 | |
20. | Deepalakshmi Sarkarai, Vignesh Ravi, Kalyani Desikan, 2024, 3180, 0094-243X, 020014, 10.1063/5.0224422 | |
21. | Esra Öztürk Sözen, Elif Eryaşar, Şükriye Çakmak, Szeged-like topological descriptors and COM -polynomials for graphs of some Alzheimer’s agents , 2024, 122, 0026-8976, 10.1080/00268976.2024.2305853 | |
22. | Xiujun Zhang, Shamaila Yousaf, Anisa Naeem, Ferdous M. Tawfiq, Adnan Aslam, Analyzing topological descriptors of guar gum and its derivatives for predicting physical properties in carbohydrates, 2024, 253, 01697439, 105203, 10.1016/j.chemolab.2024.105203 | |
23. | Gayathri K. B, S. Roy, Quantitative structure property relationship study of postpartum depression medications using topological indices and regression models, 2024, 20904479, 103194, 10.1016/j.asej.2024.103194 | |
24. | Fatima Saeed, Nazeran Idrees, Integrating Structural Modeling and Decision-Making for Anti-psychotic Drugs through Topological Indices, 2025, 15, 2191-1630, 10.1007/s12668-024-01732-2 | |
25. | Deepa Balasubramaniyan, Natarajan Chidambaram, Vignesh Ravi, 2024, Chapter 29, 978-3-031-69145-4, 380, 10.1007/978-3-031-69146-1_29 | |
26. | W. Tamilarasi, B. J. Balamurugan, New reverse sum Revan indices for physicochemical and pharmacokinetic properties of anti-filovirus drugs, 2024, 12, 2296-2646, 10.3389/fchem.2024.1486933 | |
27. | Muneeba Mansha, Sarfraz Ahmad, Zahid Raza, Eccentric indices based QSPR evaluation of drugs for schizophrenia treatment, 2025, 11, 24058440, e42222, 10.1016/j.heliyon.2025.e42222 | |
28. | Micheal Arockiaraj, J. J. Jeni Godlin, S. Radha, Comparative study of degree and neighborhood degree sum-based topological indices for predicting physicochemical properties of skin cancer drug structures, 2025, 0217-9849, 10.1142/S0217984925501064 | |
29. | Adnan Asghar, QSPR analysis of anti-hepatitis prescription drugs using degree based topological indices through M-polynomial and NM-polynomial, 2025, 12, 2411-1414, 10.15826/chimtech.2025.12.2.06 | |
30. | S. Gayathri, K. Dhanalakshmi, Ebenezer Bonyah, QSPR analysis of vitiligo treatment of some drugs using reverse degree-based topological indices, 2025, 24682276, e02636, 10.1016/j.sciaf.2025.e02636 |
Drugs | BP (℃ at 760 mmHg) | Enthalpy (kj/mol) | Flash point (℃) | Molar refraction | Molar volume (cm3) | Polarizability |
Chloroquine | 460.6 | 72.1 | 232.23 | 97.4 | 287.9 | 38.6 |
Amodiaquine | 478 | 77 | 242.9 | 105.5 | 282.8 | 41.8 |
Mefloquine | 415.7 | 70.5 | 205.2 | 83 | 273.4 | 32.9 |
Piperoquine | 721.1 | 105.3 | 389.9 | 153.7 | 414.2 | 60.9 |
Primaquine | 451.1 | 71 | 226.6 | 80.5 | 230.3 | 31.9 |
Lumefrantrine | 642.5 | 99.6 | 342.3 | 151 | 422.3 | 59.9 |
Atovaquine | 535 | 85.4 | 277.3 | 99.5 | 271.8 | 39.5 |
Pyrimethamine | 368.4 | 61.5 | 176.6 | 67.1 | 180.2 | 26.6 |
Doxycycline | 762.6 | 116.5 | 415 | 109 | 271.1 | 43.2 |
Drugs | M1(G) | M2(G) | H(G) | F(G) | SS(G) | ReZG2(G) | ReZG3(G) |
Chloroquine | 106 | 120 | 10.3 | 262 | 23.9599 | 25.2333 | 584 |
Amodiaquine | 128 | 149 | 11.7333 | 324 | 28.5664 | 30.5333 | 742 |
Mefloquine | 114 | 137 | 9.5857 | 306 | 24.5829 | 26.631 | 728 |
Piperoquine | 202 | 240 | 17.8 | 510 | 45.1804 | 48.9 | 1200 |
Primaquine | 92 | 105 | 9 | 226 | 20.903 | 22.0833 | 512 |
Lumefrantrine | 182 | 213 | 16.3666 | 466 | 40.3883 | 43.4333 | 1090 |
Atovaquine | 158 | 194 | 12.6047 | 446 | 33.4116 | 36.5619 | 1064 |
Pyrimethamine | 86 | 100 | 7.7667 | 222 | 18.9478 | 20.2167 | 506 |
Doxycycline | 200 | 264 | 14.4164 | 608 | 40.4521 | 45.55 | 1594 |
Drugs | BP | Enthalpy | Flash point | Molar refraction | Molar volume | Polarizability |
M1(G) | 0.961 | 0.968 | 0.961 | 0.838 | 0.735 | 0.838 |
M2(G) | 0.962 | 0.978 | 0.963 | 0.76 | 0.645 | 0.76 |
H(G) | 0.908 | 0.894 | 0.908 | 0.963 | 0.891 | 0.964 |
F(G) | 0.947 | 0.969 | 0.947 | 0.704 | 0.584 | 0.704 |
SS(G) | 0.948 | 0.945 | 0.948 | 0.899 | 0.809 | 0.899 |
ReZG2(G) | 0.957 | 0.958 | 0.957 | 0.873 | 0.776 | 0.873 |
ReZG3(G) | 0.934 | 0.962 | 0.934 | 0.632 | 0.505 | 0.633 |
Phy. Pro, | N | A | b | r | r2 | F | p | Indicator |
BP | 9 | 124.096 | 2.932 | 0.961 | 0.923 | 83.956 | 0 | Significant |
EN | 9 | 28.483 | 0.396 | 0.968 | 0.937 | 104.502 | 0 | Significant |
FP | 9 | 29.813 | 1.773 | 0.961 | 0.923 | 83.973 | 0 | Significant |
MR | 9 | 28.194 | 0.546 | 0.838 | 0.702 | 16.501 | 0.05 | Significant |
MV | 9 | 114.389 | 1.265 | 0.735 | 0.541 | 8.244 | 0.02 | Significant |
PO | 9 | 11.164 | 0.217 | 0.838 | 0.702 | 16.529 | 0 | Significant |
Phy. Pro, | N | A | b | r | r2 | F | p | Indicator |
BP | 9 | 161.996 | 2.219 | 0.962 | 0.926 | 88.088 | 0 | Significant |
EN | 9 | 33.198 | 0.302 | 0.978 | 0.956 | 151.468 | 0 | Significant |
FP | 9 | 51.729 | 1.342 | 0.963 | 0.926 | 88.162 | 0 | Significant |
MR | 9 | 41.872 | 0.374 | 0.76 | 0.578 | 9.575 | 0.01 | Significant |
MV | 9 | 150.949 | 0.838 | 0.645 | 0.416 | 4.982 | 0.06 | Significant |
PO | 9 | 16.589 | 0.148 | 0.76 | 0.578 | 9.588 | 0.01 | Significant |
Phy. Pro, | N | A | b | r | r2 | F | p | Indicator |
BP | 9 | 87.905 | 36.905 | 0.908 | 0.825 | 33.003 | 0.01 | Significant |
EN | 9 | 25.031 | 4.87 | 0.894 | 0.798 | 27.731 | 0 | Significant |
FP | 9 | 60939 | 22.319 | 0.908 | 0.825 | 32.986 | 0 | Significant |
MR | 9 | 3.348 | 8.365 | 0.963 | 0.928 | 90.501 | 0 | Significant |
MV | 9 | 44.044 | 20.421 | 0.891 | 0.795 | 27.106 | 0 | Significant |
PO | 9 | 1.318 | 3.317 | 0.964 | 0.928 | 90.704 | 0 | Significant |
Phy. Pro, | N | A | b | r | r2 | F | p | Indicator |
BP | 9 | 177.821 | 0.96 | 0.947 | 0.897 | 60.643 | 0 | Significant |
EN | 9 | 34.961 | 0.132 | 0.969 | 0.94 | 109.495 | 0 | Significant |
FP | 9 | 61.301 | 0.581 | 0.947 | 0.897 | 60.678 | 0 | Significant |
MR | 9 | 48.115 | 0.152 | 0.704 | 0.495 | 6.865 | 0.03 | Significant |
MV | 9 | 167.744 | 0.334 | 0.584 | 0.341 | 3.619 | 0.09 | Significant |
PO | 9 | 19.061 | 0.06 | 0.704 | 0.496 | 6.877 | 0.03 | Significant |
Phy. Pro, | N | A | b | r | r2 | F | p | Indicator |
BP | 9 | 111.354 | 13.867 | 0.948 | 0.898 | 61.748 | 0 | Significant |
EN | 9 | 27.343 | 1.855 | 0.945 | 0.894 | 58.818 | 0 | Significant |
FP | 9 | 21.11 | 8.387 | 0.948 | 0.898 | 61.739 | 0 | Significant |
MR | 9 | 18.858 | 2.811 | 0.899 | 0.808 | 29.522 | 0 | Significant |
MV | 9 | 87.819 | 6.67 | 0.809 | 0.654 | 13.222 | 0 | Significant |
PO | 9 | 7.465 | 1.115 | 0.899 | 0.809 | 29.572 | 0 | Significant |
Phy. Pro, | N | A | b | r | r2 | F | p | Indicator |
BP | 9 | 123.738 | 12.44 | 0.957 | 0.915 | 75.323 | 0 | Significant |
EN | 9 | 28.782 | 1.671 | 0.958 | 0.918 | 77.887 | 0 | Significant |
FP | 9 | 28.597 | 7.524 | 0.957 | 0.915 | 75.331 | 0 | Significant |
MR | 9 | 24.545 | 2.426 | 0.873 | 0.762 | 22.437 | 0 | Significant |
MV | 9 | 103.449 | 5.693 | 0.776 | 0.603 | 10.626 | 0.01 | Significant |
PO | 9 | 9.72 | 0.962 | 0.873 | 0.762 | 22.47 | 0 | Significant |
Phy. Pro, | N | A | b | r | r2 | F | p | Indicator |
BP | 9 | 223.151 | 0.352 | 0.934 | 0.873 | 47.933 | 0 | Significant |
EN | 9 | 40.942 | 0.049 | 0.962 | 0.925 | 86.586 | 0 | Significant |
FP | 9 | 88.713 | 0.213 | 0.934 | 0.873 | 47.97 | 0 | Significant |
MR | 9 | 59.754 | 0.051 | 0.632 | 0.4 | 4.665 | 0.05 | Significant |
MV | 9 | 196.894 | 0.107 | 0.505 | 0.255 | 2.4 | 0.05 | Significant |
PO | 9 | 23.678 | 0.02 | 0.633 | 0.4 | 4.671 | 0.05 | Significant |
Drugs | BP | Enthalpy | Flash point | Molar refraction | Molar volume | Polarizability |
M1(G) | 41.3818 | 5.0133 | 25.0251 | 17.3965 | 56.9863 | 6.8934 |
M2(G) | 40.4727 | 402053 | 24.4681 | 20.7148 | 64.279 | 8.21 |
H(G) | 62.3993 | 8.9827 | 32.985 | 8.5408 | 38.0989 | 3.3828 |
F(G) | 47.9859 | 4.9047 | 29.014 | 22.6488 | 68.2796 | 8.9761 |
SS(G) | 47.5989 | 6.6252 | 28.7891 | 13.955 | 49.478 | 5.5292 |
ReZG2(G) | 43.4975 | 5.7457 | 26.3056 | 15.5439 | 52.9971 | 6.1595 |
ReZG3(G) | 53.2487 | 5.4722 | 32.1935 | 24.6926 | 72.5723 | 9.7875 |
Drug | Boiling point ℃ (at 760 mmHg) | M1(G) | M2(G) | H(G) | F(G)) | SS(G) | ReZG2(G) | ReZG3(G) |
Chloroquine | 460.6 ± 40.0 | 434.88 | 428.27 | 468.02 | 429.341 | 443.60 | 437.64 | 428.71 |
Amodiaquine | 478.0 ± 45.0 | 499.39 | 492.62 | 520.92 | 488.861 | 507.48 | 503.57 | 484.33 |
Mefloquine | 415.7 ± 40.0 | 458.34 | 465.99 | 441.66 | 471.581 | 452.24 | 455.02 | 479.40 |
Piperoquine | 721.1 ± 60.0 | 716.36 | 694.55 | 744.81 | 667.421 | 737.87 | 732.05 | 645.55 |
Primaquine | 451.1 ± 45.0 | 393.84 | 394.99 | 420.05 | 394.781 | 401.21 | 398.45 | 403.37 |
Lumefrantrine | 642.5 ± 55.0 | 657.72 | 634.64 | 691.91 | 625.181 | 671.41 | 664.04 | 606.83 |
Atovaquine | 535.0 ± 50.0 | 587.35 | 592.48 | 553.08 | 605.981 | 574.67 | 578.56 | 597.67 |
Pyrimethamine | 368.4 ± 52.0 | 376.24 | 383.89 | 374.53 | 390.941 | 374.10 | 375.23 | 401.26 |
Doxycycline | 762.6 ± 60.0 | 710.49 | 747.81 | 619.94 | 761.501 | 672.30 | 690.38 | 784.23 |
Drug | Flash point (℃) | M1(G) | M2(G) | H(G) | F(G)) | SS(G) | ReZG2(G) | ReZG3(G) |
Chloroquine | 232.23 ± 27.3 | 216.75 | 212.76 | 236.82 | 213.52 | 222.06 | 218.45 | 213.10 |
Amodiaquine | 242.9 ± 28.7 | 255.75 | 251.68 | 268.81 | 249.54 | 260.69 | 258.32 | 246.75 |
Mefloquine | 205.2 ± 27.3 | 230.93 | 235.58 | 220.88 | 239.08 | 227.28 | 228.96 | 243.77 |
Piperoquine | 389.9 ± 32.9 | 386.95 | 373.80 | 404.21 | 357.61 | 400.03 | 396.52 | 344.31 |
Primaquine | 226.6 ± 28.7 | 191.92 | 192.63 | 207.81 | 192.60 | 196.42 | 194.75 | 197.76 |
Lumefrantrine | 342.3 ± 31.5 | 351.49 | 337.57 | 372.22 | 332.04 | 359.84 | 355.38 | 320.88 |
Atovaquine | 277.3 ± 30.1 | 308.94 | 312.07 | 288.26 | 320.42 | 301.33 | 303.68 | 315.34 |
Pyrimethamine | 176.6 ± 30.7 | 181.29 | 185.92 | 180.28 | 190.28 | 180.02 | 180.70 | 196.49 |
Doxycycline | 415.0 ± 32.9 | 383.41 | 406.01 | 328.69 | 414.54 | 360.38 | 371.31 | 428.23 |
Drug | Enthalpy (kj/mol) | M1(G) | M2(G) | H(G) | F(G)) | SS(G) | ReZG2(G) | ReZG3(G) |
Chloroquine | 72.1 ± 3.0 | 73.46 | 69.438 | 75.19 | 69.545 | 71.78 | 70.94 | 69.55 |
Amodiaquine | 77.0 ± 3.0 | 78.476 | 78.19 | 82.17 | 77.72 | 80.33 | 79.80 | 77.3 |
Mefloquine | 70.5 ± 3.0 | 75.28 | 74.57 | 71.71 | 75.35 | 72.94 | 73.28 | 76.61 |
Piperoquine | 105.3 ± 3.0 | 95.34 | 105.67 | 111.71 | 102.28 | 111.15 | 110.49 | 99.74 |
Primaquine | 71.0 ± 3.0 | 70.268 | 64.90 | 68.86 | 64.79 | 66.11 | 65.68 | 66.03 |
Lumefrantrine | 99.6 ± 3.0 | 90.788 | 97.52 | 104.73 | 96.47 | 102.26 | 101.35 | 94.35 |
Atovaquine | 85.4 ± 3.0 | 85.316 | 91.78 | 86.41 | 93.83 | 89.31 | 89.87 | 93.07 |
Pyrimethamine | 61.5 ± 3.0 | 68.9 | 63.39 | 62.85 | 64.26 | 62.48 | 62.56 | 65.73 |
Doxycycline | 116.5 ± 3.0 | 94.892 | 112.92 | 95.23 | 115.21 | 102.37 | 104.89 | 119.04 |
Drug | Molar refraction (cm3) | M1(G) | M2(G) | H(G) | F(G)) | SS(G) | ReZG2(G) | ReZG3(G) |
Chloroquine | 97.4 ± 0.3 | 86.07 | 86.75 | 89.50 | 87.93 | 86.20 | 85.76 | 89.53 |
Amodiaquine | 105.5 ± 0.3 | 98.08 | 97.59 | 101.49 | 97.36 | 99.15 | 98.61 | 97.59 |
Mefloquine | 83.0 ± 0.3 | 90.43 | 93.11 | 83.53 | 94.62 | 87.96 | 89.15 | 96.88 |
Piperoquine | 153.7 ± 0.3 | 138.48 | 131.63 | 152.24 | 125.63 | 145.86 | 143.17 | 120.95 |
Primaquine | 80.5 ± 0.3 | 78.42 | 81.14 | 78.63 | 82.46 | 77.61 | 78.11 | 85.86 |
Lumefrantrine | 151.0 ± 0.3 | 127.56 | 121.53 | 140.25 | 118.94 | 132.38 | 129.91 | 115.34 |
Atovaquine | 99.5 ± 0.3 | 114.46 | 114.42 | 108.78 | 115.90 | 112.77 | 113.24 | 114.01 |
Pyrimethamine | 67.1 ± 0.5 | 75.15 | 79.27 | 68.31 | 81.85 | 72.12 | 73.59 | 85.56 |
Doxycycline | 109 ± 0.4 | 137.39 | 140.60 | 123.94 | 140.53 | 132.56 | 135.04 | 141.04 |
Drug | Molar volume (cm3) | M1(G) | M2(G) | H(G) | F(G)) | SS(G) | ReZG2(G) | ReZzG3(G) |
Chloroquine | 287.9 ± 3.0 | 248.47 | 251.509 | 254.38 | 255.25 | 247.63 | 247.10 | 259.38 |
Amodiaquine | 282.8 ± 3.0 | 276.30 | 275.811 | 283.64 | 275.96 | 278.35 | 277.27 | 276.28 |
Mefloquine | 273.4 ± 3.0 | 258.59 | 265.755 | 239.79 | 269.94 | 251.78 | 255.05 | 274.79 |
Piperoquine | 414.2 ± 3.0 | 369.91 | 352.069 | 407.53 | 338.08 | 389.17 | 381.83 | 325.29 |
Primaquine | 230.3 ± 3.0 | 230.76 | 238.939 | 227.83 | 243.22 | 227.24 | 229.16 | 251.67 |
Lumefrantrine | 422.3 ± 3.0 | 344.61 | 329.443 | 378.26 | 323.38 | 357.20 | 350.71 | 313.52 |
Atovaquine | 271.8 ± 3.0 | 314.25 | 313.521 | 301.44 | 316.70 | 310.67 | 311.59 | 310.74 |
Pyrimethamine | 180.2 ± 7.0 | 223.17 | 234.749 | 202.64 | 241.89 | 214.20 | 218.54 | 251.03 |
Doxycycline | 271.1 ± 5.0 | 367.38 | 372.181 | 338.44 | 370.81 | 357.63 | 362.76 | 367.45 |
Drug | Polarizability (10-24 cm3) | M1(G) | M2(G) | H(G) | F(G)) | SS(G) | ReZG2(G) | ReZG3(G) |
Chloroquine | 38.6 ± 0.5 | 34.16 | 34.34 | 35.48 | 34.78 | 34.18 | 33.99 | 35.35 |
Amodiaquine | 41.8 ± 0.5 | 38.94 | 38.64 | 40.23 | 38.50 | 39.31 | 39.09 | 38.51 |
Mefloquine | 32.9 ± 0.5 | 35.90 | 36.86 | 33.11 | 37.42 | 34.87 | 35.33 | 38.23 |
Piperoquine | 60.9 ± 0.5 | 54.99 | 52.10 | 60.36 | 49.66 | 57.84 | 56.76 | 47.67 |
Primaquine | 31.9 ± 0.5 | 31.12 | 32.12 | 31.17 | 32.62 | 30.77 | 30.96 | 33.91 |
Lumefrantrine | 59.9 ± 0.5 | 50.65 | 48.11 | 55.60 | 47.02 | 52.49 | 51.50 | 45.47 |
Atovaquine | 39.5 ± 0.5 | 45.45 | 45.30 | 43.12 | 45.82 | 44.71 | 44.89 | 44.95 |
Pyrimethamine | 26.6 ± 0.5 | 29.82 | 31.38 | 27.08 | 32.38 | 28.59 | 29.16 | 33.79 |
Doxycycline | 43.2 ± 0.5 | 54.56 | 55.66 | 49.13 | 55.54 | 52.56 | 53.53 | 55.55 |
Drugs | BP (℃ at 760 mmHg) | Enthalpy (kj/mol) | Flash point (℃) | Molar refraction | Molar volume (cm3) | Polarizability |
Chloroquine | 460.6 | 72.1 | 232.23 | 97.4 | 287.9 | 38.6 |
Amodiaquine | 478 | 77 | 242.9 | 105.5 | 282.8 | 41.8 |
Mefloquine | 415.7 | 70.5 | 205.2 | 83 | 273.4 | 32.9 |
Piperoquine | 721.1 | 105.3 | 389.9 | 153.7 | 414.2 | 60.9 |
Primaquine | 451.1 | 71 | 226.6 | 80.5 | 230.3 | 31.9 |
Lumefrantrine | 642.5 | 99.6 | 342.3 | 151 | 422.3 | 59.9 |
Atovaquine | 535 | 85.4 | 277.3 | 99.5 | 271.8 | 39.5 |
Pyrimethamine | 368.4 | 61.5 | 176.6 | 67.1 | 180.2 | 26.6 |
Doxycycline | 762.6 | 116.5 | 415 | 109 | 271.1 | 43.2 |
Drugs | M1(G) | M2(G) | H(G) | F(G) | SS(G) | ReZG2(G) | ReZG3(G) |
Chloroquine | 106 | 120 | 10.3 | 262 | 23.9599 | 25.2333 | 584 |
Amodiaquine | 128 | 149 | 11.7333 | 324 | 28.5664 | 30.5333 | 742 |
Mefloquine | 114 | 137 | 9.5857 | 306 | 24.5829 | 26.631 | 728 |
Piperoquine | 202 | 240 | 17.8 | 510 | 45.1804 | 48.9 | 1200 |
Primaquine | 92 | 105 | 9 | 226 | 20.903 | 22.0833 | 512 |
Lumefrantrine | 182 | 213 | 16.3666 | 466 | 40.3883 | 43.4333 | 1090 |
Atovaquine | 158 | 194 | 12.6047 | 446 | 33.4116 | 36.5619 | 1064 |
Pyrimethamine | 86 | 100 | 7.7667 | 222 | 18.9478 | 20.2167 | 506 |
Doxycycline | 200 | 264 | 14.4164 | 608 | 40.4521 | 45.55 | 1594 |
Drugs | BP | Enthalpy | Flash point | Molar refraction | Molar volume | Polarizability |
M1(G) | 0.961 | 0.968 | 0.961 | 0.838 | 0.735 | 0.838 |
M2(G) | 0.962 | 0.978 | 0.963 | 0.76 | 0.645 | 0.76 |
H(G) | 0.908 | 0.894 | 0.908 | 0.963 | 0.891 | 0.964 |
F(G) | 0.947 | 0.969 | 0.947 | 0.704 | 0.584 | 0.704 |
SS(G) | 0.948 | 0.945 | 0.948 | 0.899 | 0.809 | 0.899 |
ReZG2(G) | 0.957 | 0.958 | 0.957 | 0.873 | 0.776 | 0.873 |
ReZG3(G) | 0.934 | 0.962 | 0.934 | 0.632 | 0.505 | 0.633 |
Phy. Pro, | N | A | b | r | r2 | F | p | Indicator |
BP | 9 | 124.096 | 2.932 | 0.961 | 0.923 | 83.956 | 0 | Significant |
EN | 9 | 28.483 | 0.396 | 0.968 | 0.937 | 104.502 | 0 | Significant |
FP | 9 | 29.813 | 1.773 | 0.961 | 0.923 | 83.973 | 0 | Significant |
MR | 9 | 28.194 | 0.546 | 0.838 | 0.702 | 16.501 | 0.05 | Significant |
MV | 9 | 114.389 | 1.265 | 0.735 | 0.541 | 8.244 | 0.02 | Significant |
PO | 9 | 11.164 | 0.217 | 0.838 | 0.702 | 16.529 | 0 | Significant |
Phy. Pro, | N | A | b | r | r2 | F | p | Indicator |
BP | 9 | 161.996 | 2.219 | 0.962 | 0.926 | 88.088 | 0 | Significant |
EN | 9 | 33.198 | 0.302 | 0.978 | 0.956 | 151.468 | 0 | Significant |
FP | 9 | 51.729 | 1.342 | 0.963 | 0.926 | 88.162 | 0 | Significant |
MR | 9 | 41.872 | 0.374 | 0.76 | 0.578 | 9.575 | 0.01 | Significant |
MV | 9 | 150.949 | 0.838 | 0.645 | 0.416 | 4.982 | 0.06 | Significant |
PO | 9 | 16.589 | 0.148 | 0.76 | 0.578 | 9.588 | 0.01 | Significant |
Phy. Pro, | N | A | b | r | r2 | F | p | Indicator |
BP | 9 | 87.905 | 36.905 | 0.908 | 0.825 | 33.003 | 0.01 | Significant |
EN | 9 | 25.031 | 4.87 | 0.894 | 0.798 | 27.731 | 0 | Significant |
FP | 9 | 60939 | 22.319 | 0.908 | 0.825 | 32.986 | 0 | Significant |
MR | 9 | 3.348 | 8.365 | 0.963 | 0.928 | 90.501 | 0 | Significant |
MV | 9 | 44.044 | 20.421 | 0.891 | 0.795 | 27.106 | 0 | Significant |
PO | 9 | 1.318 | 3.317 | 0.964 | 0.928 | 90.704 | 0 | Significant |
Phy. Pro, | N | A | b | r | r2 | F | p | Indicator |
BP | 9 | 177.821 | 0.96 | 0.947 | 0.897 | 60.643 | 0 | Significant |
EN | 9 | 34.961 | 0.132 | 0.969 | 0.94 | 109.495 | 0 | Significant |
FP | 9 | 61.301 | 0.581 | 0.947 | 0.897 | 60.678 | 0 | Significant |
MR | 9 | 48.115 | 0.152 | 0.704 | 0.495 | 6.865 | 0.03 | Significant |
MV | 9 | 167.744 | 0.334 | 0.584 | 0.341 | 3.619 | 0.09 | Significant |
PO | 9 | 19.061 | 0.06 | 0.704 | 0.496 | 6.877 | 0.03 | Significant |
Phy. Pro, | N | A | b | r | r2 | F | p | Indicator |
BP | 9 | 111.354 | 13.867 | 0.948 | 0.898 | 61.748 | 0 | Significant |
EN | 9 | 27.343 | 1.855 | 0.945 | 0.894 | 58.818 | 0 | Significant |
FP | 9 | 21.11 | 8.387 | 0.948 | 0.898 | 61.739 | 0 | Significant |
MR | 9 | 18.858 | 2.811 | 0.899 | 0.808 | 29.522 | 0 | Significant |
MV | 9 | 87.819 | 6.67 | 0.809 | 0.654 | 13.222 | 0 | Significant |
PO | 9 | 7.465 | 1.115 | 0.899 | 0.809 | 29.572 | 0 | Significant |
Phy. Pro, | N | A | b | r | r2 | F | p | Indicator |
BP | 9 | 123.738 | 12.44 | 0.957 | 0.915 | 75.323 | 0 | Significant |
EN | 9 | 28.782 | 1.671 | 0.958 | 0.918 | 77.887 | 0 | Significant |
FP | 9 | 28.597 | 7.524 | 0.957 | 0.915 | 75.331 | 0 | Significant |
MR | 9 | 24.545 | 2.426 | 0.873 | 0.762 | 22.437 | 0 | Significant |
MV | 9 | 103.449 | 5.693 | 0.776 | 0.603 | 10.626 | 0.01 | Significant |
PO | 9 | 9.72 | 0.962 | 0.873 | 0.762 | 22.47 | 0 | Significant |
Phy. Pro, | N | A | b | r | r2 | F | p | Indicator |
BP | 9 | 223.151 | 0.352 | 0.934 | 0.873 | 47.933 | 0 | Significant |
EN | 9 | 40.942 | 0.049 | 0.962 | 0.925 | 86.586 | 0 | Significant |
FP | 9 | 88.713 | 0.213 | 0.934 | 0.873 | 47.97 | 0 | Significant |
MR | 9 | 59.754 | 0.051 | 0.632 | 0.4 | 4.665 | 0.05 | Significant |
MV | 9 | 196.894 | 0.107 | 0.505 | 0.255 | 2.4 | 0.05 | Significant |
PO | 9 | 23.678 | 0.02 | 0.633 | 0.4 | 4.671 | 0.05 | Significant |
Drugs | BP | Enthalpy | Flash point | Molar refraction | Molar volume | Polarizability |
M1(G) | 41.3818 | 5.0133 | 25.0251 | 17.3965 | 56.9863 | 6.8934 |
M2(G) | 40.4727 | 402053 | 24.4681 | 20.7148 | 64.279 | 8.21 |
H(G) | 62.3993 | 8.9827 | 32.985 | 8.5408 | 38.0989 | 3.3828 |
F(G) | 47.9859 | 4.9047 | 29.014 | 22.6488 | 68.2796 | 8.9761 |
SS(G) | 47.5989 | 6.6252 | 28.7891 | 13.955 | 49.478 | 5.5292 |
ReZG2(G) | 43.4975 | 5.7457 | 26.3056 | 15.5439 | 52.9971 | 6.1595 |
ReZG3(G) | 53.2487 | 5.4722 | 32.1935 | 24.6926 | 72.5723 | 9.7875 |
Drug | Boiling point ℃ (at 760 mmHg) | M1(G) | M2(G) | H(G) | F(G)) | SS(G) | ReZG2(G) | ReZG3(G) |
Chloroquine | 460.6 ± 40.0 | 434.88 | 428.27 | 468.02 | 429.341 | 443.60 | 437.64 | 428.71 |
Amodiaquine | 478.0 ± 45.0 | 499.39 | 492.62 | 520.92 | 488.861 | 507.48 | 503.57 | 484.33 |
Mefloquine | 415.7 ± 40.0 | 458.34 | 465.99 | 441.66 | 471.581 | 452.24 | 455.02 | 479.40 |
Piperoquine | 721.1 ± 60.0 | 716.36 | 694.55 | 744.81 | 667.421 | 737.87 | 732.05 | 645.55 |
Primaquine | 451.1 ± 45.0 | 393.84 | 394.99 | 420.05 | 394.781 | 401.21 | 398.45 | 403.37 |
Lumefrantrine | 642.5 ± 55.0 | 657.72 | 634.64 | 691.91 | 625.181 | 671.41 | 664.04 | 606.83 |
Atovaquine | 535.0 ± 50.0 | 587.35 | 592.48 | 553.08 | 605.981 | 574.67 | 578.56 | 597.67 |
Pyrimethamine | 368.4 ± 52.0 | 376.24 | 383.89 | 374.53 | 390.941 | 374.10 | 375.23 | 401.26 |
Doxycycline | 762.6 ± 60.0 | 710.49 | 747.81 | 619.94 | 761.501 | 672.30 | 690.38 | 784.23 |
Drug | Flash point (℃) | M1(G) | M2(G) | H(G) | F(G)) | SS(G) | ReZG2(G) | ReZG3(G) |
Chloroquine | 232.23 ± 27.3 | 216.75 | 212.76 | 236.82 | 213.52 | 222.06 | 218.45 | 213.10 |
Amodiaquine | 242.9 ± 28.7 | 255.75 | 251.68 | 268.81 | 249.54 | 260.69 | 258.32 | 246.75 |
Mefloquine | 205.2 ± 27.3 | 230.93 | 235.58 | 220.88 | 239.08 | 227.28 | 228.96 | 243.77 |
Piperoquine | 389.9 ± 32.9 | 386.95 | 373.80 | 404.21 | 357.61 | 400.03 | 396.52 | 344.31 |
Primaquine | 226.6 ± 28.7 | 191.92 | 192.63 | 207.81 | 192.60 | 196.42 | 194.75 | 197.76 |
Lumefrantrine | 342.3 ± 31.5 | 351.49 | 337.57 | 372.22 | 332.04 | 359.84 | 355.38 | 320.88 |
Atovaquine | 277.3 ± 30.1 | 308.94 | 312.07 | 288.26 | 320.42 | 301.33 | 303.68 | 315.34 |
Pyrimethamine | 176.6 ± 30.7 | 181.29 | 185.92 | 180.28 | 190.28 | 180.02 | 180.70 | 196.49 |
Doxycycline | 415.0 ± 32.9 | 383.41 | 406.01 | 328.69 | 414.54 | 360.38 | 371.31 | 428.23 |
Drug | Enthalpy (kj/mol) | M1(G) | M2(G) | H(G) | F(G)) | SS(G) | ReZG2(G) | ReZG3(G) |
Chloroquine | 72.1 ± 3.0 | 73.46 | 69.438 | 75.19 | 69.545 | 71.78 | 70.94 | 69.55 |
Amodiaquine | 77.0 ± 3.0 | 78.476 | 78.19 | 82.17 | 77.72 | 80.33 | 79.80 | 77.3 |
Mefloquine | 70.5 ± 3.0 | 75.28 | 74.57 | 71.71 | 75.35 | 72.94 | 73.28 | 76.61 |
Piperoquine | 105.3 ± 3.0 | 95.34 | 105.67 | 111.71 | 102.28 | 111.15 | 110.49 | 99.74 |
Primaquine | 71.0 ± 3.0 | 70.268 | 64.90 | 68.86 | 64.79 | 66.11 | 65.68 | 66.03 |
Lumefrantrine | 99.6 ± 3.0 | 90.788 | 97.52 | 104.73 | 96.47 | 102.26 | 101.35 | 94.35 |
Atovaquine | 85.4 ± 3.0 | 85.316 | 91.78 | 86.41 | 93.83 | 89.31 | 89.87 | 93.07 |
Pyrimethamine | 61.5 ± 3.0 | 68.9 | 63.39 | 62.85 | 64.26 | 62.48 | 62.56 | 65.73 |
Doxycycline | 116.5 ± 3.0 | 94.892 | 112.92 | 95.23 | 115.21 | 102.37 | 104.89 | 119.04 |
Drug | Molar refraction (cm3) | M1(G) | M2(G) | H(G) | F(G)) | SS(G) | ReZG2(G) | ReZG3(G) |
Chloroquine | 97.4 ± 0.3 | 86.07 | 86.75 | 89.50 | 87.93 | 86.20 | 85.76 | 89.53 |
Amodiaquine | 105.5 ± 0.3 | 98.08 | 97.59 | 101.49 | 97.36 | 99.15 | 98.61 | 97.59 |
Mefloquine | 83.0 ± 0.3 | 90.43 | 93.11 | 83.53 | 94.62 | 87.96 | 89.15 | 96.88 |
Piperoquine | 153.7 ± 0.3 | 138.48 | 131.63 | 152.24 | 125.63 | 145.86 | 143.17 | 120.95 |
Primaquine | 80.5 ± 0.3 | 78.42 | 81.14 | 78.63 | 82.46 | 77.61 | 78.11 | 85.86 |
Lumefrantrine | 151.0 ± 0.3 | 127.56 | 121.53 | 140.25 | 118.94 | 132.38 | 129.91 | 115.34 |
Atovaquine | 99.5 ± 0.3 | 114.46 | 114.42 | 108.78 | 115.90 | 112.77 | 113.24 | 114.01 |
Pyrimethamine | 67.1 ± 0.5 | 75.15 | 79.27 | 68.31 | 81.85 | 72.12 | 73.59 | 85.56 |
Doxycycline | 109 ± 0.4 | 137.39 | 140.60 | 123.94 | 140.53 | 132.56 | 135.04 | 141.04 |
Drug | Molar volume (cm3) | M1(G) | M2(G) | H(G) | F(G)) | SS(G) | ReZG2(G) | ReZzG3(G) |
Chloroquine | 287.9 ± 3.0 | 248.47 | 251.509 | 254.38 | 255.25 | 247.63 | 247.10 | 259.38 |
Amodiaquine | 282.8 ± 3.0 | 276.30 | 275.811 | 283.64 | 275.96 | 278.35 | 277.27 | 276.28 |
Mefloquine | 273.4 ± 3.0 | 258.59 | 265.755 | 239.79 | 269.94 | 251.78 | 255.05 | 274.79 |
Piperoquine | 414.2 ± 3.0 | 369.91 | 352.069 | 407.53 | 338.08 | 389.17 | 381.83 | 325.29 |
Primaquine | 230.3 ± 3.0 | 230.76 | 238.939 | 227.83 | 243.22 | 227.24 | 229.16 | 251.67 |
Lumefrantrine | 422.3 ± 3.0 | 344.61 | 329.443 | 378.26 | 323.38 | 357.20 | 350.71 | 313.52 |
Atovaquine | 271.8 ± 3.0 | 314.25 | 313.521 | 301.44 | 316.70 | 310.67 | 311.59 | 310.74 |
Pyrimethamine | 180.2 ± 7.0 | 223.17 | 234.749 | 202.64 | 241.89 | 214.20 | 218.54 | 251.03 |
Doxycycline | 271.1 ± 5.0 | 367.38 | 372.181 | 338.44 | 370.81 | 357.63 | 362.76 | 367.45 |
Drug | Polarizability (10-24 cm3) | M1(G) | M2(G) | H(G) | F(G)) | SS(G) | ReZG2(G) | ReZG3(G) |
Chloroquine | 38.6 ± 0.5 | 34.16 | 34.34 | 35.48 | 34.78 | 34.18 | 33.99 | 35.35 |
Amodiaquine | 41.8 ± 0.5 | 38.94 | 38.64 | 40.23 | 38.50 | 39.31 | 39.09 | 38.51 |
Mefloquine | 32.9 ± 0.5 | 35.90 | 36.86 | 33.11 | 37.42 | 34.87 | 35.33 | 38.23 |
Piperoquine | 60.9 ± 0.5 | 54.99 | 52.10 | 60.36 | 49.66 | 57.84 | 56.76 | 47.67 |
Primaquine | 31.9 ± 0.5 | 31.12 | 32.12 | 31.17 | 32.62 | 30.77 | 30.96 | 33.91 |
Lumefrantrine | 59.9 ± 0.5 | 50.65 | 48.11 | 55.60 | 47.02 | 52.49 | 51.50 | 45.47 |
Atovaquine | 39.5 ± 0.5 | 45.45 | 45.30 | 43.12 | 45.82 | 44.71 | 44.89 | 44.95 |
Pyrimethamine | 26.6 ± 0.5 | 29.82 | 31.38 | 27.08 | 32.38 | 28.59 | 29.16 | 33.79 |
Doxycycline | 43.2 ± 0.5 | 54.56 | 55.66 | 49.13 | 55.54 | 52.56 | 53.53 | 55.55 |