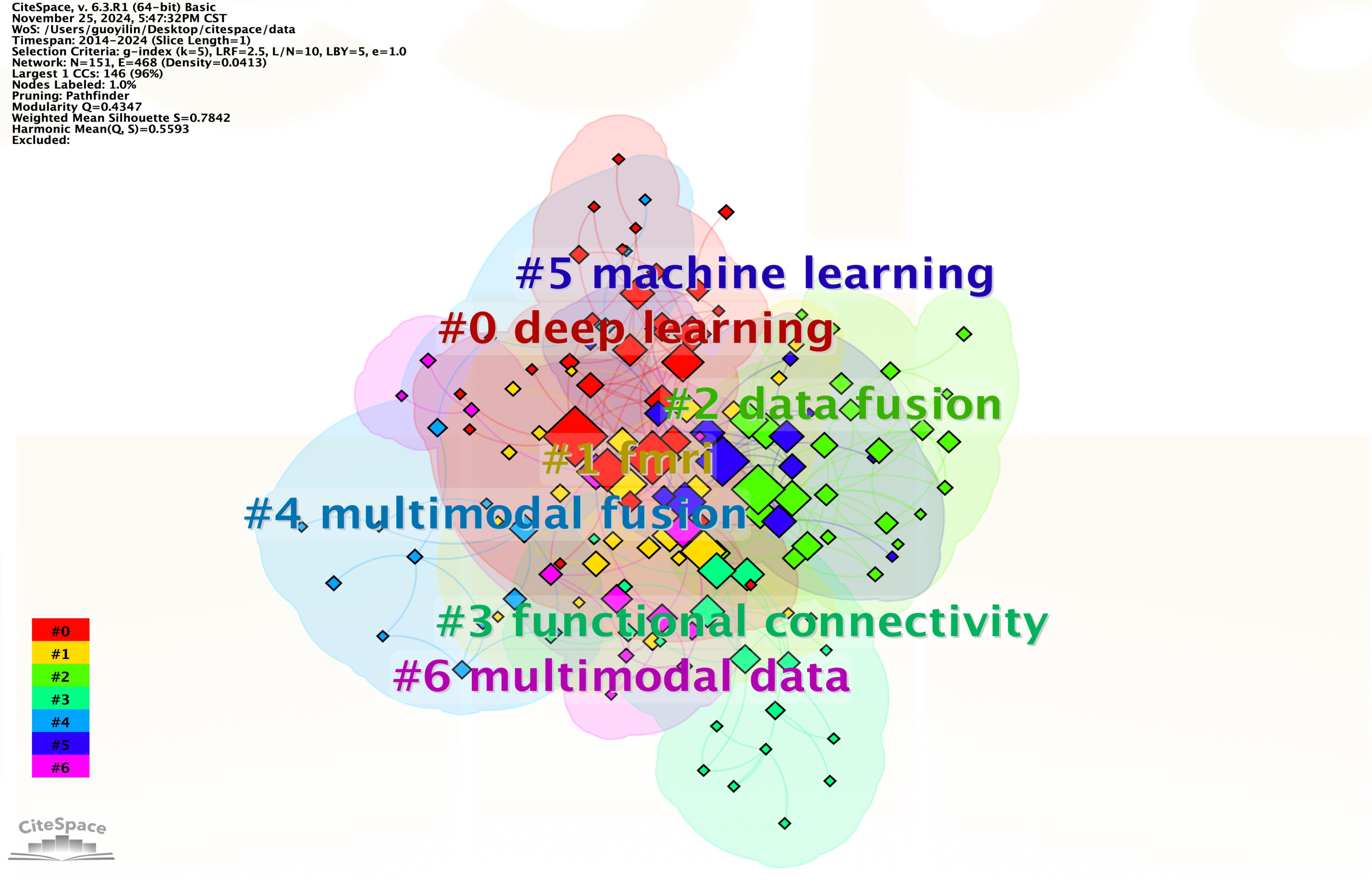
The application of multimodal data is particularly important in accurately assessing behavioral effects and optimizing the decision-making process. This type of data provides more comprehensive and in-depth insights by integrating information from different sources and formats. Comprehensive data support not only enhances the science and accuracy of decision-making but also significantly improves the quality of behavioral effectiveness assessment. This study first describes the practical significance and theoretical value of multimodal data in behavioral effect assessment. Subsequently, the types of multimodal data involved and the construction methods of data sets are introduced. In order to demonstrate the role of multimodal data in behavioral effect assessment, the teaching effect of English classroom presentations at a comprehensive university in China is taken as a case study, and the effect of the target behavior was statistically measured based on multimodal data such as students' classroom behavioral videos, images, questionnaires, interviews, and assessment data. The results of the case study show that AI+ demonstrates significant advantages in behavioral effect assessment, which is more objective and effectively avoids the limitations of subjectivity in traditional assessment methods. At the same time, multimodal data helps optimize behavioral effects. For example, the presentations made at the beginning of the class show significant advantages in teaching effect compared with the presentation made before the end of the class, which provides data support and optimization direction for the implementation of teaching activities.
Citation: Suyan Tan, Yunyi Zhao, Jinjun Wang, Jia Fang. Statistical measurement of behavioral effects based on multimodal data[J]. National Accounting Review, 2024, 6(4): 573-589. doi: 10.3934/NAR.2024027
[1] | Shengyin Ouyang, Ziqing Du, Meiling Cai . Statistical measurement of the impact of monetary policy on price levels. National Accounting Review, 2020, 2(2): 188-203. doi: 10.3934/NAR.2020011 |
[2] | Peter A.G. van Bergeijk . On the inaccuracies of macroeconomic observations. National Accounting Review, 2024, 6(3): 367-383. doi: 10.3934/NAR.2024017 |
[3] | Laura Grassini, Gianni Dugheri . Mobile phone data and tourism statistics: a broken promise?. National Accounting Review, 2021, 3(1): 50-68. doi: 10.3934/NAR.2021002 |
[4] | Tinghui Li, Zimei Huang, Benjamin M Drakeford . Statistical measurement of total factor productivity under resource and environmental constraints. National Accounting Review, 2019, 1(1): 16-27. doi: 10.3934/NAR.2019.1.16 |
[5] | Serena Fatica, Wildmer Daniel Gregori . Income shifting by multinational enterprises as a source of mismeasurement in aggregate statistics. National Accounting Review, 2024, 6(4): 548-563. doi: 10.3934/NAR.2024025 |
[6] | Sylvie Kotíková . Spillover effects: A challenging public interest to measure. National Accounting Review, 2023, 5(4): 373-404. doi: 10.3934/NAR.2023022 |
[7] | Adolfo Maza . Scientific method for assessing "Real Madrid DNA": merely a catchy journalistic term or reality?. National Accounting Review, 2024, 6(2): 291-313. doi: 10.3934/NAR.2024013 |
[8] | Jawad Saleemi . In COVID-19 outbreak, correlating the cost-based market liquidity risk to microblogging sentiment indicators. National Accounting Review, 2020, 2(3): 249-262. doi: 10.3934/NAR.2020014 |
[9] | Iulia Toropoc . The case for life expectancy at age 60 as a prominent health indicator. A comparative analysis. National Accounting Review, 2022, 4(4): 390-411. doi: 10.3934/NAR.2022022 |
[10] | Iulia Toropoc . Population data quality checks: Romanian adult deaths and lives, an evaluation. National Accounting Review, 2023, 5(3): 282-297. doi: 10.3934/NAR.2023017 |
The application of multimodal data is particularly important in accurately assessing behavioral effects and optimizing the decision-making process. This type of data provides more comprehensive and in-depth insights by integrating information from different sources and formats. Comprehensive data support not only enhances the science and accuracy of decision-making but also significantly improves the quality of behavioral effectiveness assessment. This study first describes the practical significance and theoretical value of multimodal data in behavioral effect assessment. Subsequently, the types of multimodal data involved and the construction methods of data sets are introduced. In order to demonstrate the role of multimodal data in behavioral effect assessment, the teaching effect of English classroom presentations at a comprehensive university in China is taken as a case study, and the effect of the target behavior was statistically measured based on multimodal data such as students' classroom behavioral videos, images, questionnaires, interviews, and assessment data. The results of the case study show that AI+ demonstrates significant advantages in behavioral effect assessment, which is more objective and effectively avoids the limitations of subjectivity in traditional assessment methods. At the same time, multimodal data helps optimize behavioral effects. For example, the presentations made at the beginning of the class show significant advantages in teaching effect compared with the presentation made before the end of the class, which provides data support and optimization direction for the implementation of teaching activities.
As technology advances and demand grows, multimodal behavioral data is of great importance for behavioral effect assessment. The wide application of modern sensors, cameras, and smart devices has significantly simplified and facilitated the process of multimodal data collection. Multimodal data, which integrates multiple data forms such as vision, speech, text, and sensor signals, is gradually leading behavioral effect assessment from the traditional simple assessment mode to a new stage of in-depth understanding and personalized optimization. Studies have shown that in the broad field of behavior recognition, multimodal data, with its rich information dimensions, can capture and analyze the complex features of behavior in a more comprehensive and accurate way than traditional methods that rely only on single-mode data, and thus achieve significant improvement in the comprehensiveness and accuracy of behavior description. Taking human behavior research as an example, traditional unimodal data sources (e.g., questionnaires or activity counters) are often limited by the subjectivity of the respondents and the influence of specific environmental factors, which may lead to biased or incomplete data. In contrast, multimodal data can provide a more three-dimensional, continuous, and close-to-reality description of behavior by combining multiple dimensions such as activity tracking, sentiment analysis, and geolocation information. This comprehensive approach to data analysis can not only reveal the deeper motivations and patterns behind behaviors but also provide strong support for the development of personalized behavioral interventions and optimization strategies (Oresti et al., 2016). Therefore, with the continuous progress of technology and the improvement of data fusion capability, multimodal behavioral data will play an increasingly important role in the field of behavioral outcome assessment in the future.
The results of the visualization analysis with the help of the Citespace software show that the seven research hotspots of multimodal data processing are mainly related to medicine, deep learning, and multimodal data fusion (see Figure 1). There are a lot of intersections between research hotspots, indicating that multimodal data-related research mainly focuses on multimodal data fusion technology and its application areas. In these areas, researchers explore how to achieve multimodal data fusion through machine learning and how to maximize the value of multimodal data in the medical field.
In multimodal data research, multimodal fusion methods based on deep learning have become a focus of research in recent years. For example, extracting features by using convolutional neural networks (CNNs) and bi-directional long- and short-term memory networks (Bi-LSTMs) (Smoliński et al., 2024) or constructing a multimodal internet of driver behavioral analysis (MODAL-IoCV) (Aboulola, et al., 2021), which allows real-time monitoring of driver's attention and behavior and a reduction in accident risk. In addition, models with attention mechanisms can optimize the fusion effect of remotely sensed data and socio-perceptual data for urban functional area identification (Xie et al., 2022). Several studies have further explored the potential of data fusion in specific application scenarios. Examples include recognizing gambling sites by integrating visual and semantic features (Wang et al., 2022), combining 3D point cloud data and generative adversarial networks (GANs) to process large-scale automated driving data (Ignatious et al., 2023), and using decision trees and correlation analysis algorithms on behavioral data collected through cameras, microphones, and other sensors for multimodal perception to improve the efficiency of processing complex events (Alkhomsan et al., 2017). While multimodal data fusion shows strong potential, research has also shown that the effects of different fusion methods and modal combinations vary significantly. For example, models using facial expression and eye movement data worked best in the prediction of learning outcomes for online learning instructors, while models combining brainwave data were more effective in the prediction of cognitive and affective engagement (Xiao et al., 2023). In addition, the high dimensionality and heterogeneity of multimodal data are still the challenge of research, and how to realize efficient fusion without losing key information is a future research direction.
The application of multimodal data within the medical field is mainly based on multimodal data to enhance decision-making. First, in the prediction of problematic behaviors of children with autism, by analyzing multimodal data including behavioral observations, physiological indicators, and environmental factors through the machine learning framework PreMAC, researchers were able to capture the subtle changes in the behavioral patterns of children with autism with greater precision, which significantly improves prediction accuracy (Zheng et al, 2021). This data-based prediction method not only provides a scientific basis for early intervention for children with autism but also reduces the burden for families and society. Similarly, deep learning models of multimodal data play an important role in the early diagnosis of attention deficit hyperactivity disorder (ADHD). Traditional diagnostic methods often rely on doctors' subjective assessment, which has large errors and uncertainties. Deep learning models based on multimodal data, on the other hand, are able to comprehensively consider information from multiple aspects, such as the patient's behavioral performance, cognitive function, and physiological indicators, among others, so as to more accurately determine whether or not the patient suffers from ADHD (Zhang et al., 2024). In addition, with the continuous progress of artificial intelligence technology, research on multimodal data analysis methods in the field of intelligent medical treatment has become more and more extensive. In medical signal monitoring systems, for multimodal data such as ECG and arterial blood pressure, researchers have developed a convolutional neural network (CNN)-based information fusion (CIF) algorithm to detect heartbeat location. The algorithm is versatile, robust, and efficient, and can accurately identify a patient's cardiac condition and provide timely diagnosis and treatment recommendations for physicians (Chandra et al, 2018). In addition to the above applications, the open-source web application IMPatienT (Integrated digital Multimodal PATIENt daTa) provides a new platform for the application of multimodal data in the medical field. By processing multimodal patient data that combines image data with free text, IMPatienT is able to provide physicians with comprehensive diagnostic information to help them make more accurate decisions (Meyer et al., 2024). This decision-support system based on multimodal data not only improves the efficiency and quality of healthcare services but also brings better treatment experience and prognosis to patients. Finally, the genetic evolutionary randomized neural network clustering (GERNNC) model, which combines genetic evolutionary algorithms and neural networks, also shows great potential in the study of the pathogenesis of Alzheimer's disease (AD). Through multimodal data fusion analysis, the GERNNC model is able to reveal the complex relationship between brain structure, function, and genetic information of AD patients, which provides important clues for a deeper understanding of the pathogenesis of AD and the development of effective treatments (Wang et al., 2023).
The related research on behavioral effects is more about exploring the results or impacts of human behaviors in various fields. Based on the econometric analysis of behavioral effect-related studies in recent years conducted by the Citespace software (see Figure 2), it can be seen that the relevant studies mainly focus on medicine, artificial intelligence, and education, indicating that behavioral effect analysis with the help of artificial intelligence technology has been widely used in the fields of medicine and education.
In the field of medicine, behavioral outcome research has focused not only on the direct effects of drug treatment but also on revealing the potential impact of drugs on patient behavior. For example, a study of Chilean epilepsy patients aged 4–15 years found that family history of psychiatric disorders and prior behavioral disorders were predisposing factors for patients to develop adverse behavioral effects after treatment with levetiracetam (LEV) (Cortes & Manterola, 2020). This study not only enriches our understanding of patient behavioral changes during drug therapy but also suggests the need for clinicians to consider more individualized factors when developing treatment plans. Meanwhile, a behavioral quality assessment of 727 patients using levetiracetam showed that children taking levetiracetam were at risk of experiencing a number of behavioral side effects compared to children not taking the drug (Halma et al., 2014). These studies not only emphasize the importance of behavioral outcome studies in assessing the efficacy and safety of medication but also provide comprehensive information about the efficacy of medication for patients and families.
In the field of education, behavioral effectiveness research has focused more on students' problem behaviors and classroom behaviors, especially focusing on how to use diverse strategies to promote the healthy development of children's emotion and behavior. Numerous literature have shown the effectiveness of emotional education activities in enhancing children's emotional intelligence and reducing behavioral problems. For example, Lim's (2014) study reveals that an emotional education program can substantially increase the level of emotional intelligence of 4-year-old children and effectively reduce their behavioral disturbances. Meanwhile, the family environment, as an important background for children's growth, should not be ignored for its influence on children's behavior. Ho (2021), through an in-depth exploration of the impact of family members' conflicts over children's education on children's behavior, found that reducing conflicts within the family, especially through parental education to enhance communication and conflict resolution among family members, has a positive effect on alleviating young children's behavioral problems. This study emphasizes the importance of family harmony in the development of children's behavior. In terms of classroom behavior, positive behavior support (PBS), as an effective educational strategy, has been widely recognized as having a positive impact on shaping young children's pro-social behaviors. Kim's (2019) study demonstrated that the implementation of PBS significantly enhances young children's social behavioral performance in the classroom and promotes the formation of positive peer relationships. However, the effects of classroom quality on children's behavior are not static and exhibit some complexity. Watts (2021) noted that while improvements in classroom quality have a relatively limited impact on children's behavior in general, access to a high-quality classroom significantly improves academic achievement and behavioral performance for children living in poor environments. This finding highlights the critical role of classroom quality in the development of behavior for specific groups of children. In addition, differences in behavioral effects are also seen across individuals of different ages and levels of education. Chew's (2017) study found that individuals with higher levels of education showed more abnormalities in risk attitudes but fewer abnormalities in time management, revealing a complex relationship between educational attainment and individual behavioral patterns. In terms of character education, Jeynes (2019) pointed out that the impact of character education on the behavior of students of different ages is differentiated, and that high school students have a more significant effect of behavioral change in character education compared to elementary school students, emphasizing the relevance and differentiation of the implementation of character education.
With the continuous development and popularization of artificial intelligence technology, its application in the analysis of behavioral effects has become increasingly widespread. Whether in the medical field or in the field of education, artificial intelligence provides new methods and tools for behavioral effect research with its powerful data processing ability and intelligent decision-making ability.
The marginal contributions of this study are mainly in two points. First, this study uses AI tools to statistically measure behavioral effects based on multimodal data. This approach not only transcends the limitations of traditional single-modal data analysis but can also reveal the complexity and diversity of behavioral effects in greater depth by combining multimodal data, such as images and texts. Second, this study further broadens the application area of multimodal data by taking English classroom teaching as a case study. Traditional teaching assessment methods often rely on single test scores or questionnaires, which are difficult to fully reflect students' real performance in the classroom. Instead, this study evaluates the effectiveness of classroom presentation as a teaching activity by integrating multimodal data from student behavior, student feedback, and teacher evaluation.
The rest of this paper is structured as follows: Chapter 2 presents the multimodal data set for behavioral effectiveness assessment. In this chapter, we will introduce the multimodal data involved in behavioral effectiveness assessment. Chapter 3 is a case study of behavioral effectiveness assessment based on multimodal data. In this chapter, we will discuss the behavioral effect assessment method based on multimodal data using English classroom presentation as a concrete case. First, we will introduce the collection process of multimodal data, including the selection of collection tools, the setting of collection points, and the preliminary organization of data. Then, we will analyze the collected multimodal data to reveal students' behavioral characteristics and effects in classroom presentations. Chapter 4 is a conclusion. In this chapter, we will summarize and discuss the research content of this paper.
Data collection is an indispensable core component of behavioral effectiveness evaluation. Before collecting data, we first need to define the goals of the assessment. This requires the evaluator to clarify the following questions: What behavioral effects are being evaluated? What is the purpose of the assessment? What specific information or conclusions do you hope to obtain through the assessment? Based on the assessment objectives, we need to further define the type of data to be collected. According to the data collection method, there are five main types of data: numerical data, text data, image data, audio data, and sensor data.
Numerical data is one of the most direct and objective data types in behavioral effectiveness assessment. It is usually obtained by measuring, counting, or calculating, such as the respondent's age, height, weight, income, and other basic information, as well as quantitative indicators such as the number of times the behavior occurs, frequency, duration, and so on. Numerical data can provide us with a precise and quantifiable basis for assessment and help us understand the behavioral characteristics and trends of respondents more intuitively.
Text data, as the most basic and common type of data in behavioral effect assessment, is mainly obtained through interview records, questionnaire survey results, social media comments, online evaluations, and other methods. This type of data can directly reflect the views, attitudes, and feelings of respondents, providing rich qualitative information for the evaluation.
Image data also plays an important role in behavioral effect assessment. By capturing key information such as respondents' behavioral processes, expression changes, and environmental layout, image data can visually display behavioral effects and help us understand respondents' behavioral patterns and emotional responses more intuitively.
Audio data is also a data type that cannot be ignored in the evaluation of behavioral effects. It is acquired through voice recording and voice analysis. Voice information is converted into text information with the help of speech recognition and speech transcription technology, which facilitates subsequent analysis and processing. Audio data can reveal the respondent's voice characteristics such as intonation, speech speed, volume, etc., thus reflecting their emotional state, self-confidence level, and other psychological characteristics.
Sensor data, on the other hand, is an emerging and important data type in behavioral outcome assessment. It is mainly obtained through biometric sensors, motion sensors, environmental monitoring sensors, and other devices, which can monitor the physiological indicators, physical activity, environmental conditions, and other information of the respondents in real time. This type of data provides objective and quantitative data support for the assessment, making the results more accurate and reliable. When collecting data, we make sure to focus on the assessment objectives to ensure the accuracy, completeness, and representativeness of the data.
After completing data collection, the next step is the construction of data sets. The core purpose of data set construction is to organize the collected raw data into a collection of data that is clearly structured and easy to compare and analyze. Such a data set can more intuitively show the relationship and trends among the data. Data cleansing is the first and crucial step in data set construction. Since the data collection process may produce invalid, duplicate, erroneous, or abnormal data, if not cleaned, these data will be seriously misleading for subsequent data analysis. Therefore, it is important to ensure the accuracy and completeness of the data before performing comparative analysis. In data set construction, data are usually divided into two data sets, the experimental group and the control group. The experimental group is a collection of data that received a specific behavior or intervention, while the control group is a collection of data that did not receive any specific behavior or intervention. By comparing the data from the experimental and control groups, the effectiveness of the specific behavior or intervention can be assessed. After completing the data set construction, the next process usually involves further processing and analysis of the data as well as interpretation and application of the results. At this stage, we will use statistical methods and data mining techniques to analyze the data in depth and reveal the patterns and trends behind the data. At the same time, we also need to interpret and apply the analysis results to develop corresponding strategies, plans, or improvement measures to guide subsequent behavioral practices.
Enhancing the effect of classroom presentations not only stimulates students' learning interest and expression ability but also promotes teamwork and knowledge application. It is an important way to cultivate innovative and critical thinking, and several scholars have analyzed how to improve the quality of presentations. Related studies cover content optimization (e.g., AceTalk system, PPT, and manuscript suggestions), structure improvement (e.g., slide structure modification system), and presentation skill enhancement (e.g., Pecha Kucha fast-paced presentations, presentation tools in PBL courses) (Stapa et al., 2014; Trinh et al., 2016; Park, 2016; Lee, 2018; Zhao & Fei, 2022; Takahashi et al., 2024). In addition, the effects of factors such as collocation ability, group cooperation and feedback mechanism, and teaching mode on presentations have also been explored (Park, 2012; Choi, 2018; Hartono, 2023; Han, 2024). However, most of these studies rely on questionnaires and interviews and lack the application of multi-tools such as computer visualization techniques; few studies have focused on the effects of different time slots on presentation effect. Is there any difference in students' performance when presenting at different periods of a lesson? Is there any difference in classroom behavior when listening to presentations in different time slots? Can the above questions be answered with the help of artificial intelligence and supplemented with multimodal data generated from evaluations, questionnaires, interviews, etc., to automatically identify classroom behaviors?
The case samples selected for this study were 319 second-year students from a comprehensive university in China, including 89 male and 230 female students. Students attended seven different classes and were freely organized into 84 presentation groups, with one group from each class presenting in class every week. Thirty groups presented 10 minutes before the end of class, and all other groups presented 10 minutes at the beginning of class. The data involved in this case were presentation scores, students' self-performance assessments, and students' classroom behavior. Presentation scores were collected and presented through numerical scales; students' self-performance assessment data were obtained through questionnaires and interviews; and students' classroom behavior was obtained through artificial intelligence technology.
1. Presentation score
After the students finished the presentation, the students who listened to the presentation made an oral peer evaluation of the presentation group's performance. After, the teacher made further comments on content, structure, oral expression, stage manner, etc. Finally, the students and the teacher rated the presentation group separately, with the students' rating accounting for 30% and the teacher's rating accounting for 70% of the total score of 20 points.
2. Questionnaire survey
After all groups finished the presentation, the teacher distributed questionnaires to find out the students' behavioral engagement. The questionnaire was developed based on Ren Qingmei's Classroom Learning Engagement Measure (Ren, 2023). The questionnaire completed by students who made presentations in the first 10 minutes of class contained four questions:
● I listen more attentively to presentations that begin as soon as class starts than to presentations before class ends.
● I responded more actively to the questions posed by groups presenting at the beginning of class than I did to the presentation delivered near the end of class.
● I was more likely to ask questions regarding the content of presentations that start at the beginning of class, compared to the presentations made before class ended.
● I was more willing to evaluate the presentations that began as soon as class began, compared to the presentations that were made before class ended.
The questionnaire completed by the students who made presentations 10 minutes before the end of class contained the above four questions and also the additional question "I would have performed better if I had made presentations as soon as class began." A five-point Likert Scale was used for each question: 1 = Almost Never, 2 = Rarely, 3 = Sometimes, 4 = Often, and 5 = Always. The questionnaire was filled out anonymously to ensure that data were authentic and reliable.
3. Interviews
Presentation groups were categorized into high subgroups (≥18 points), medium subgroups (15–17 points), and low subgroups (≤14 points) based on presentation scores. The teacher selected one student from each of the high, medium, and low subgroups in each of the two periods for one-on-one interviews. The interviews were usually conducted after class in the form of small talk in the classroom the students were attending in order to keep students from feeling nervous. There were four questions for the interviews:
● Does the time period in which the presentation takes place have an impact on your performance?
● Does the time of presentation have an effect on your listening attentiveness?
● Does the time slot of the presentation have an impact on your participation in interactions (questions, answers, peer evaluations)?
● If you had a choice, which time slot would you prefer to conduct the presentation?
The interviews were audio-recorded, and the recordings were converted to text and analyzed at the end of the interviews.
4. Artificial intelligence monitoring
A high-definition camera with a resolution of 1920 × 1080 was installed above the blackboard in the classroom. There are five camera shooting modes available to choose from: automatic capture, teacher panoramic, student panoramic, teacher close-up, and student close-up. During students' presentations, the teacher can easily activate the camera equipment with a single click and select the "student panoramic mode" to record the entire process of students' classroom learning behaviors while they are listening. The deployment of the classroom camera and the recorded classroom situation are shown in Figure 3.
From each video, one keyframe was selected from every 30 frames and saved. Later, the original image was input into the data annotation software tool for labeling the target detection boxes. In this study, seven common classroom behaviors were labeled: listening to the lecture, reading a book, playing with a mobile phone, talking, sleeping, standing, and raising hands (see Figure 4).
With the continuous advancement of artificial intelligence technology, a variety of algorithms and models have been proposed to automatically recognize classroom behaviors, e.g., moving target detection algorithms, standing behavior recognition algorithms based on region of interest and face tracking, and hand raising behavior recognition algorithms based on skin color detection (Wu et al., 2016). In addition, the combination of IoT technology and convolutional neural network (CNN) also provides new solutions for classroom behavior recognition, such as VGG16, 3D-CNN, CNN-10, and other models, which are excellent in both accuracy and robustness (Lin et al., 2021; Albert et al., 2022; Zhou et al., 2022). Meanwhile, the YOLO series of deep learning algorithms have also been widely studied and applied in the field of classroom behavior recognition. For example, the improved YOLO-v4 network, ET-YOLOv5s, and YOLOv8n_BT algorithms can effectively recognize a wide range of classroom behaviors (Chen & Guan, 2022; Li et al., 2022; Liu et al., 2024).
Based on the above background, in this paper, labeled images were imported into the CA-YOLOv9 network for intelligent recognition of classroom behavior. This network is based on the YOLOv9 network, with the addition of the CA module. The network's graphics processing unit (GPU) used an NVIDIA GeForce RTX 3090, and the deep learning framework employed Pytorch 2.0 with CUDA 11.7. Training parameters were set as follows: batch - size = 8; number of iterations 300. The evaluation metrics for the network mainly included precision, recall, and mean average precision (mAP). mAP adopted the mAP0.5 metric, indicating the mAP when IoU (the intersection over union) is 0.5.
In summary, this paper collected and obtained the scores of 84 group presentations, 303 questionnaires (with a valid questionnaire recovery rate of 94.98%), 24 group presentation videos (12 groups in each time slot), and interview recordings with a length of 31 minutes and 49 seconds (a total of 5,368 Chinese characters).
We categorized the scores of the groups that did presentations in two different time periods; the average, highest, and lowest scores of each time period are shown in Table 1.
Items | Presentations at the beginning of class | Presentations before the end of class |
Average score | 17.25 | 16.5 |
Highest score | 20 | 19 |
Lowest score | 14 | 12 |
As can be seen from Table 1, the presentation made at the beginning of the class led to better performance than the group who presented before the end of the class. The data shows that the average score of presentations at the beginning of class was 17.25, while the average score of presentations before the end of class was 16.5. The highest score at the beginning of the class was 20 out of 20, while the highest score before the end of the class was only 19 out of 20, although the performance was also good. Meanwhile, comparing the lowest scores, the lowest score of 14 at the beginning of the class was also higher than the lowest score of 12 before the end of the class. This phenomenon may be attributed to the fact that students were more focused and energized at the beginning of class and, therefore, better able to demonstrate their abilities and readiness. In contrast, by the end of the class, students may feel tired or distracted due to the long period of study and activities they experienced, which affected their presentation performance to some extent. In addition, presentation before the end of class may also be affected by time constraints, resulting in inadequate preparation or inconsistent performance.
We conducted a questionnaire survey on students' behavioral engagement while listening to the presentation. The first four questions were answered by all students, and the fifth question was limited to those who made the presentation 10 minutes before the end of the class. The results are shown in Table 2.
Items | Average scores | |
Presentation at the beginning of class | Presentation before the end of class | |
1. I listen more attentively to presentations that begin as soon as class starts than to presentations made before class ends. | 3.79 | 3.24 |
2. I responded more actively to the questions posed by groups presenting at the beginning of class than I did in the presentation delivered near the end of class. | 3.63 | 3.41 |
3. I was more likely to ask questions regarding the content of presentations that start at the beginning of class, compared to the presentations made before class ended. | 3.37 | 3.23 |
4. I was more willing to evaluate the presentations that began as soon as class began, compared to the presentations that were made before class ended. | 3.53 | 3.16 |
5. I would have performed better if I had made the presentation as soon as class began. | / | 3.24 |
From the self-assessment of the students who took the questionnaire, they generally believed that their presentations at the beginning of the class were superior to those before the end of the class. Specifically, according to the comparison of the means of each item, the mean of presentation at the beginning of class was significantly higher than that before the end of class in all aspects. This result clearly shows that students demonstrated higher behavioral engagement in the presentation at the beginning of class. Not only did they listen more attentively to the presentation, but they were also more active in answering and asking questions, as well as more willing to evaluate the presentation.
An interesting result was obtained in the interviews addressing the effect of presentation slots on performance. First, the six students interviewed did not believe in general that the time slot in which the presentation was conducted would have a direct impact on their performance. However, when asked which time slot they would prefer to present in if they had a choice, five students expressed a preference to present within the first 10 minutes of class. This shows that even though the students did not believe that the time slot had a direct impact on their performance, they still preferred to present at the beginning of the course when it came to actual choices.
Notably, the only student in the lower subgroup who presented 10 minutes before the end of class stated that he was not particular about the time slot, but that he would be more attentive when listening to class presentations that began at the beginning of class. This view was echoed by two students from the middle and low subgroups who made presentations at the beginning of class and who also felt that presentations at the beginning of class captured their attention more. However, the other three respondents indicated that they would remain attentive listeners regardless of the time slot of the presentation and that the time slot had no effect on their concentration.
When it comes to the impact of presentation slots on participation in classroom interactions, the students' views were equally diverse. Two introverted students indicated that they were less likely to take the initiative to speak in class, and therefore the impact of slots on their participation in interactions was not significant. In contrast, three students explicitly stated that they were more willing to answer questions posed by the classroom presentation group that began as soon as the class started, as well as discuss and evaluate the content of the presentations. This may be related to the fact that they were more focused and engaged at the beginning of the class. Finally, another student offered a more rational viewpoint, arguing that the influence of time slots on the interaction was not direct but rather depended on whether one was able to answer the questions of the presentation group or whether there were issues that one did not understand or agree with that needed to be discussed with the presentation group, and whether the presentation group had something special to comment on. This idea emphasizes the initiative and selectivity of individuals in their interactions, rather than simply being influenced by the time slot.
In summary, although the students did not believe that presentation slots directly affected their performance or interaction participation in general, they still tended to present at the beginning of the class and showed higher levels of concentration and participation in their actual choices. At the same time, individual initiative and selectivity in interaction are also important factors that influence the effectiveness of interaction.
By inputting the labeled raw images into the CA-YOLOv9 network for classroom behavior recognition, we obtained the percentage of seven common classroom behaviors (see Table 3).
Listeners' classroom behavior | Presentations at the beginning of class | Presentations before the end of class | |||
Positive classroom behaviors | listening | 55.71% | 80.73% | 52.43% | 79.44% |
reading | 25.02 % | 27.01% | |||
Negative classroom behaviors | playing | 11.74% | 12.82% | 13.97% | 16.95% |
talking | 1.07% | 2.63% | |||
sleeping | 0.01% | 0.35% | |||
Interactive classroom behaviors | hands-up | 0.49% | 6.45% | 0.06 % | 3.61% |
In terms of students' classroom behavior, students showed high concentration at the beginning of the class but then showed a gradual decline. According to the data in Table 3, it can be seen that when listening to the presentation at the beginning of the class, the percentage of positive classroom behaviors such as listening attentively and reading the relevant materials was 80.73%, slightly higher than the 79.44% listening to the presentation before the end of the class. The percentage of negative classroom behaviors such as playing with cell phones, talking, or sleeping was 12.82%, lower than 16.95% in the presentation before the end of the class. Also, the percentage of raising hands to participate in the interaction and standing up to answer/raise questions in the presentation at the beginning of the class was higher than before the end of the class.
This paper first describes the role of multimodal data in behavioral effect assessment, states its practical significance, and then puts forward its theoretical significance by combining the related research of multimodal data and behavioral effect. Subsequently, this paper introduces the multimodal data involved and the construction of data sets in the process of behavioral effect assessment. On this basis, the paper takes the teaching effect of English classroom presentation as a case study and statistically measures the behavioral effect based on the multimodal data involved. In the process, the study draws two core conclusions. First, regarding the comparison of assessment methods, we find that AI+ technology shows significant advantages in the assessment of behavioral effects. Compared with traditional assessment methods such as interviews and questionnaires, AI+ not only monitors results that are highly consistent with the results obtained by these traditional methods but also has stronger objectivity, effectively avoiding the subjective limitations that may exist in traditional methods. Second, multimodal data helps optimize behavioral effects. Through the case study of English teaching, it can be seen that presentations at the beginning of a class show significant advantages in teaching effectiveness compared with presentations before the end of the class, which is reflected in the students' presentation scores, their self-assessment, and their behavioral performance in the classroom. These provide data to support and optimize instructional activities.
These findings not only provide new perspectives and methods for behavioral effectiveness assessment but also some important insights. First, interdisciplinary cooperation and knowledge integration are the keys to promoting the innovative development of behavioral effectiveness assessment. By integrating knowledge and methods from different disciplines, we can jointly explore more efficient and accurate assessment tools to provide a scientific basis for decision-making in various fields. Second, continuous optimization based on assessment results is an important way to maximize the target effect. By continuously collecting and analyzing multimodal data, we can identify problems and adjust strategies in a timely manner so as to continuously improve the target effect.
The authors declare they have not used Artificial Intelligence (AI) tools in the creation of this article.
This research was funded by the National Social Science Fund Project "Research on Cognitive Load in User Experience of College English Cloud Platform" (22BYY094), the Guangdong Postgraduate Education Innovation Project "Research on Innovative Mode of Postgraduate Moral Education Empowered by Information Technology" (2023JGXM_098), the Project of Philosophy, Social Sciences Planning of Guangdong Province (GD23WZXC01-13), and 2023 Changzhou "Open Competition Mechanism to Select the Best Candidates" for Higher Education Institution(s) VS Vocational Education Partnership Project: "Practice of cultivating business English talents for digital trade"(20240025).
The authors declare no conflict of interest in this paper.
[1] |
Aboulola O, Khayyat M, Al-Harbi B, et al. (2021) Multimodal feature-assisted continuous driver behavior analysis and solving for edge-enabled Internet of connected vehicles using deep learning. Appl Sci 11: 10462. https://doi.org/10.3390/app112110462 doi: 10.3390/app112110462
![]() |
[2] |
Albert CCY, Sun Y, Guang L, et al. (2022) Identifying and monitoring students' classroom learning behavior based on multisource information. Mob Inf Sys 8: 9903342. https://doi.org/10.1155/2022/9903342 doi: 10.1155/2022/9903342
![]() |
[3] |
Alkhomsan MN, Hossain MA, Rahman SKMM, et al. (2017) Situation awareness in ambient assisted living for smart healthcare. IEEE Access 5: 20716–20725. https://doi.org/10.1109/ACCESS.2017.2731363 doi: 10.1109/ACCESS.2017.2731363
![]() |
[4] |
Chandra BS, Sastry CS, Jana S (2018) Robust heartbeat detection from multimodal data via CNN-based generalizable information fusion. IEEE Trans Biomed Eng 66: 710–717. https://doi.org/10.1109/TBME.2018.2854899 doi: 10.1109/TBME.2018.2854899
![]() |
[5] |
Chen H, Guan J (2022) Teacher-student behavior recognition in classroom teaching based on improved YOLO-v4 and Internet of Things technology. Electronics 11: 3998. https://doi.org/10.3390/electronics11233998 doi: 10.3390/electronics11233998
![]() |
[6] |
Chew SH, Yi JJ, Zhang JS, et al. (2017) Education and anomalies in decision making: Experimental evidence from Chinese adult twins. J Risk Uncertain 53: 163–200. https://doi.org/10.1007/s11166-016-9246-7 doi: 10.1007/s11166-016-9246-7
![]() |
[7] | Choi J (2018) Effects of collocational competence and small group cooperative learning on Korean university students' English presentations. Linguist Assoc Korea J 26: 267–285. |
[8] |
Cortes C, Manterola C (2020) Behavioral alterations associated with levetiracetam in pediatric epilepsy. Epilepsy Behav 112: 107472. https://doi.org/10.1016/j.yebeh.2020.107472 doi: 10.1016/j.yebeh.2020.107472
![]() |
[9] | Trinh H, Edge D, Ring L, et al. (2016) Thinking Outside the Box: Co-planning Scientific Presentations with Virtual Agents. In: Traum, D., Swartout, W., Khooshabeh, P., Kopp, S., Scherer, S., Leuski, A. (eds) Intelligent Virtual Agents. IVA 2016. Lecture Notes in Computer Science, 10011: 306–316, Springer, Cham. https://doi.org/10.1007/978-3-319-47665-0_27 |
[10] | Han MH (2024) The effect of peer feedback on Korean college students' English presentation skills. Linguist Assoc Korea J 5: 73–92. |
[11] |
Halma E, de Louw AJA, Halma E (2014) Behavioral side-effects of levetiracetam in children with epilepsy: A systematic review. Seizure-Eur J Epilep 23: 685–691. https://doi.org/10.1016/j.seizure.2014.06.004 doi: 10.1016/j.seizure.2014.06.004
![]() |
[12] |
Hartono H, Mujiyanto J, Fitriati SW, et al. (2023) English presentation self-efficacy development of Indonesian ESP students: the effects of individual versus group presentation tasks. Int J Lang Educ 7: 361–376. https://doi.org/10.26858/ijole.v7i3.34442 doi: 10.26858/ijole.v7i3.34442
![]() |
[13] | Ho YG, Kim HS, Lee SH (2021) The effect of children's private education experiences, parental perception of the necessity of private education, and conflict between family members on children's behavioral problems in China. The Journal of Korea Open Association for Early Childhood Education 26: 271–294. |
[14] |
Ignatious HA, El-Sayed H, Khan MA, et al. (2023) A generic framework for enhancing autonomous driving accuracy through multimodal data fusion. Appl Sci 13: 10749. https://doi.org/10.3390/app131910749 doi: 10.3390/app131910749
![]() |
[15] |
Jeynes WH (2019) A Meta-Analysis on the Relationship Between Character Education and Student Achievement and Behavioral Outcomes. Educ Urban Soc 51: 33–71. https://doi.org/10.1177/0013124517747681 doi: 10.1177/0013124517747681
![]() |
[16] |
Kim SH, Lee BI (2019) A Study on Effects of Universal Positive Behavior Support in Inclusive Classrooms on the Prosocial Behaviors of General Young Children. J Behav Anal Support 6: 49–80. https://doi.org/10.22874/kaba.2019.6.2.49 doi: 10.22874/kaba.2019.6.2.49
![]() |
[17] | Lee JY (2018) An analysis of the presentation materials for effective presentation education of foreign undergraduate students. Urimalgeul: The Korean Language and Literature 79: 57–86. |
[18] |
Li L, Liu M, Sun L, et al. (2022) ET-YOLOv5s: Toward Deep Identification of Students' in-Class Behaviors. IEEE Access 5: 44200–44211. https://doi.org/10.1109/ACCESS.2022.3169586 doi: 10.1109/ACCESS.2022.3169586
![]() |
[19] | Lim SH (2014) The Effects of Emotional Education Activities on 4-year-old Children's Emotional Intelligence and Their Behavioral Problems: Focusing on Use of Picture Books. Journal of Children's Literature and Education 15: 335–355. |
[20] |
Lin J, Li J, Chen J (2021) An analysis of English classroom behavior by intelligent image recognition in IoT. Int J Syst Assur Eng Manag 13: 1063–1071. https://doi.org/10.1007/s13198-021-01327-0 doi: 10.1007/s13198-021-01327-0
![]() |
[21] |
Liu Q et al. (2024) YOLOv8n_BT: research on classroom learning behavior recognition algorithm based on improved YOLOv8n. IEEE Access 12: 36391–36403. https://doi.org/10.1109/ACCESS.2024.3373536 doi: 10.1109/ACCESS.2024.3373536
![]() |
[22] |
Meyer C, Romero NB, Evangelista T, et al. (2024) IMPatienT: An integrated web application to digitize, process and explore multimodal patient data. J Neuromuscul Dis 11: 855–870. https://doi.org/10.3233/JND-230085 doi: 10.3233/JND-230085
![]() |
[23] |
Oresti B, Claudia B, Jaehun B, et al. (2016) Human behavior analysis by means of multimodal context mining. Sensors 16: 1264. https://doi.org/10.3390/s16081264 doi: 10.3390/s16081264
![]() |
[24] |
Ren QM (2023) The Formulation and Verification of the Scale of Multidimensional Evaluation for Student Engagement in College English Classroom. Shandong Foreign Language Teaching 43: 58–66. https://doi.org/10.16482/j.sdwy37-1026.2022-04-006 doi: 10.16482/j.sdwy37-1026.2022-04-006
![]() |
[25] |
Smoliński A, Forczmański P, Nowosielski A (2024) Processing and integration of multimodal image data supporting the detection of behaviors related to reduced concentration level of motor vehicle users. Electronics 13: 2457. https://doi.org/10.3390/electronics13132457 doi: 10.3390/electronics13132457
![]() |
[26] |
Stapa M, Murad NA, Ahmad N (2014) Engineering technical oral presentation: voices of the stakeholder. Procedia Soc Behav Sci 118: 463–467. https://doi.org/10.1016/j.sbspro.2014.02.063 doi: 10.1016/j.sbspro.2014.02.063
![]() |
[27] | Park B (2012) The effect of peer feedback on improving English presentation skills. Studies in British and American Language and Literature 105: 193–216. |
[28] | Park ED (2016) A study on the effect of the usage of mobile presentation authoring tool on presentation skill in PBL course. J Humanit Soc Sci 7: 605–624. |
[29] |
Takahashi K, Gu W, Ota K, et al. (2024) An academic presentation support system utilizing structural elements. IEICE Trans Inf Syst 6: 486–494. https://doi.org/10.1587/transinf.2023IHP0006 doi: 10.1587/transinf.2023IHP0006
![]() |
[30] |
Wang C, Zhang M, Shi F, et al. (2022) A hybrid multimodal data-fusion-based method for identifying gambling websites. Electronics 11: 2489. https://doi.org/10.3390/electronics11162489 doi: 10.3390/electronics11162489
![]() |
[31] |
Wang S, Zheng K, Kong W, et al. (2023) Multimodal data fusion based on IGERNNC algorithm for detecting pathogenic brain regions and genes in Alzheimer's disease. Brief Bioinform 24: 1–14. https://doi.org/10.1093/bib/bbac515 doi: 10.1093/bib/bbac515
![]() |
[32] |
Watts TW, Nguyen T, Carr RC, et al. (2021). Examining the Effects of Changes in Classroom Quality on Within-Child Changes in Achievement and Behavioral Outcomes. Child Dev 92: E439–E456. https://doi.org/10.1111/cdev.13552 doi: 10.1111/cdev.13552
![]() |
[33] | Wu L (2016) A study of college students' classroom learning behavior. Educational Teaching Forum 11: 50–51. |
[34] |
Xiao J, Jiang Z, Wang L, et al. (2023) What can multimodal data tell us about online synchronous training: Learning outcomes and engagement of in-service teachers. Front Psychol 13: 1092848. https://doi.org/10.3389/fpsyg.2022.1092848 doi: 10.3389/fpsyg.2022.1092848
![]() |
[35] |
Xie L, Feng X, Zhang C, et al. (2022) Identification of urban functional areas based on the multimodal deep learning fusion of high-resolution remote sensing images and social perception data. Buildings 12: 556. https://doi.org/10.3390/buildings12050556 doi: 10.3390/buildings12050556
![]() |
[36] |
Zhang KF, Yeh SC, Wu EHK, et al. (2024) Fusion of multi-task neurophysiological data to enhance the detection of attention-deficit/hyperactivity disorder. IEEE J Transl Eng Health Med 12: 668–674. https://doi.org/10.1109/JTEHM.2024.3435553 doi: 10.1109/JTEHM.2024.3435553
![]() |
[37] |
Zhao X, Fei F (2022) Investigation on the design of anthropomorphic oral presentation assistant training system. Mobile Information Systems 2022: 3719010. https://doi.org/10.1155/2022/3719010 doi: 10.1155/2022/3719010
![]() |
[38] |
Zheng ZK, Staubitz JE, Weitlauf AS, et al. (2021) Predictive multimodal framework to alert caregivers of problem behaviors for children with ASD (PreMAC). Sensors 21: 370. https://doi.org/10.3390/s21020370 doi: 10.3390/s21020370
![]() |
[39] |
Zhou J, Ran F, Li G, et al. (2022) Classroom learning status assessment based on deep learning. Math Probl Eng 4: 7049458. https://doi.org/10.1155/2022/7049458 doi: 10.1155/2022/7049458
![]() |
Items | Presentations at the beginning of class | Presentations before the end of class |
Average score | 17.25 | 16.5 |
Highest score | 20 | 19 |
Lowest score | 14 | 12 |
Items | Average scores | |
Presentation at the beginning of class | Presentation before the end of class | |
1. I listen more attentively to presentations that begin as soon as class starts than to presentations made before class ends. | 3.79 | 3.24 |
2. I responded more actively to the questions posed by groups presenting at the beginning of class than I did in the presentation delivered near the end of class. | 3.63 | 3.41 |
3. I was more likely to ask questions regarding the content of presentations that start at the beginning of class, compared to the presentations made before class ended. | 3.37 | 3.23 |
4. I was more willing to evaluate the presentations that began as soon as class began, compared to the presentations that were made before class ended. | 3.53 | 3.16 |
5. I would have performed better if I had made the presentation as soon as class began. | / | 3.24 |
Listeners' classroom behavior | Presentations at the beginning of class | Presentations before the end of class | |||
Positive classroom behaviors | listening | 55.71% | 80.73% | 52.43% | 79.44% |
reading | 25.02 % | 27.01% | |||
Negative classroom behaviors | playing | 11.74% | 12.82% | 13.97% | 16.95% |
talking | 1.07% | 2.63% | |||
sleeping | 0.01% | 0.35% | |||
Interactive classroom behaviors | hands-up | 0.49% | 6.45% | 0.06 % | 3.61% |
Items | Presentations at the beginning of class | Presentations before the end of class |
Average score | 17.25 | 16.5 |
Highest score | 20 | 19 |
Lowest score | 14 | 12 |
Items | Average scores | |
Presentation at the beginning of class | Presentation before the end of class | |
1. I listen more attentively to presentations that begin as soon as class starts than to presentations made before class ends. | 3.79 | 3.24 |
2. I responded more actively to the questions posed by groups presenting at the beginning of class than I did in the presentation delivered near the end of class. | 3.63 | 3.41 |
3. I was more likely to ask questions regarding the content of presentations that start at the beginning of class, compared to the presentations made before class ended. | 3.37 | 3.23 |
4. I was more willing to evaluate the presentations that began as soon as class began, compared to the presentations that were made before class ended. | 3.53 | 3.16 |
5. I would have performed better if I had made the presentation as soon as class began. | / | 3.24 |
Listeners' classroom behavior | Presentations at the beginning of class | Presentations before the end of class | |||
Positive classroom behaviors | listening | 55.71% | 80.73% | 52.43% | 79.44% |
reading | 25.02 % | 27.01% | |||
Negative classroom behaviors | playing | 11.74% | 12.82% | 13.97% | 16.95% |
talking | 1.07% | 2.63% | |||
sleeping | 0.01% | 0.35% | |||
Interactive classroom behaviors | hands-up | 0.49% | 6.45% | 0.06 % | 3.61% |