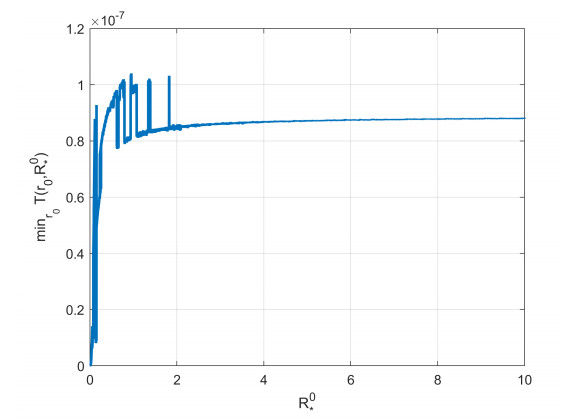
This article investigates the quality of the Romanian population data for the years 1966–2018. The following age heaping measures are being used, each with its recommended population age span: Whipple, Myers, Bachi, UNASA, Kannisto, Coale and Li. In addition, the Kannisto measure of old age overstatement is used. Our analysis corroborates the results of our measures with the demographic characteristic of the Romanian population. We aim to establish whether; (a) the data spanning the time interval 1966–1989 is more accurate than the data collected during the 1990–2018 interval, (b) the Romanian data is at least of comparable quality with the tier 2 HMD data and/or (c) the Romanian data is not grossly inaccurate and therefore suitable for research. We find support for (a) and, cautioning against the live data for the time interval 2002–2018, for (b) and (c). Limitations aside (no through overstatement or cohort analysis), this is the first study to attempt such evaluation and hopefully not the last.
Citation: Iulia Toropoc. Population data quality checks: Romanian adult deaths and lives, an evaluation[J]. National Accounting Review, 2023, 5(3): 282-297. doi: 10.3934/NAR.2023017
[1] | Shihe Xu, Junde Wu . Qualitative analysis of a time-delayed free boundary problem for tumor growth with angiogenesis and Gibbs-Thomson relation. Mathematical Biosciences and Engineering, 2019, 16(6): 7433-7446. doi: 10.3934/mbe.2019372 |
[2] | H. J. Alsakaji, F. A. Rihan, K. Udhayakumar, F. El Ktaibi . Stochastic tumor-immune interaction model with external treatments and time delays: An optimal control problem. Mathematical Biosciences and Engineering, 2023, 20(11): 19270-19299. doi: 10.3934/mbe.2023852 |
[3] | Yuting Ding, Gaoyang Liu, Yong An . Stability and bifurcation analysis of a tumor-immune system with two delays and diffusion. Mathematical Biosciences and Engineering, 2022, 19(2): 1154-1173. doi: 10.3934/mbe.2022053 |
[4] | Alessandro Bertuzzi, Antonio Fasano, Alberto Gandolfi, Carmela Sinisgalli . Interstitial Pressure And Fluid Motion In Tumor Cords. Mathematical Biosciences and Engineering, 2005, 2(3): 445-460. doi: 10.3934/mbe.2005.2.445 |
[5] | Jiaxin Nan, Wanbiao Ma . Stability and persistence analysis of a microorganism flocculation model with infinite delay. Mathematical Biosciences and Engineering, 2023, 20(6): 10815-10827. doi: 10.3934/mbe.2023480 |
[6] | Maria Vittoria Barbarossa, Christina Kuttler, Jonathan Zinsl . Delay equations modeling the effects of phase-specific drugs and immunotherapy on proliferating tumor cells. Mathematical Biosciences and Engineering, 2012, 9(2): 241-257. doi: 10.3934/mbe.2012.9.241 |
[7] | Marek Bodnar, Monika Joanna Piotrowska, Urszula Foryś . Gompertz model with delays and treatment: Mathematical analysis. Mathematical Biosciences and Engineering, 2013, 10(3): 551-563. doi: 10.3934/mbe.2013.10.551 |
[8] | Qiaoling Chen, Fengquan Li, Sanyi Tang, Feng Wang . Free boundary problem for a nonlocal time-periodic diffusive competition model. Mathematical Biosciences and Engineering, 2023, 20(9): 16471-16505. doi: 10.3934/mbe.2023735 |
[9] | Avner Friedman, Harsh Vardhan Jain . A partial differential equation model of metastasized prostatic cancer. Mathematical Biosciences and Engineering, 2013, 10(3): 591-608. doi: 10.3934/mbe.2013.10.591 |
[10] | Hui Cao, Dongxue Yan, Ao Li . Dynamic analysis of the recurrent epidemic model. Mathematical Biosciences and Engineering, 2019, 16(5): 5972-5990. doi: 10.3934/mbe.2019299 |
This article investigates the quality of the Romanian population data for the years 1966–2018. The following age heaping measures are being used, each with its recommended population age span: Whipple, Myers, Bachi, UNASA, Kannisto, Coale and Li. In addition, the Kannisto measure of old age overstatement is used. Our analysis corroborates the results of our measures with the demographic characteristic of the Romanian population. We aim to establish whether; (a) the data spanning the time interval 1966–1989 is more accurate than the data collected during the 1990–2018 interval, (b) the Romanian data is at least of comparable quality with the tier 2 HMD data and/or (c) the Romanian data is not grossly inaccurate and therefore suitable for research. We find support for (a) and, cautioning against the live data for the time interval 2002–2018, for (b) and (c). Limitations aside (no through overstatement or cohort analysis), this is the first study to attempt such evaluation and hopefully not the last.
Over the past few decades, considerable attention has been paid to the rigorous analysis of mathematical models describing tumor growth and great progress has been achieved. Most work in this direction focuses on the sphere-shaped or nearly sphere-shaped tumor models; see [1,2,3,4,5,6,7,8,9] and the references therein. Observing that the work concerning models for tumors having different geometric configurations from spheroids is less frequent, in this paper we are interested in the situation of tumor cord–a kind of tumor that grows cylindrically around the central blood vessel and receives nutrient materials (such as glucose and oxygen) from the blood vessel [10]. The model only describes the evolution of the tumor cord section perpendicular to the length direction of the blood vessel due to the cord's uniformity in that direction. Assume that the radius of the blood vessel is r0 and denote by J and Γ(t) the section of the blood vessel wall and the section of the exterior surface of the tumor cord, respectively, then J={x∈R2;|x|=r0}. We also denote by Ω(t) the region of the section of the tumor cord, so that Ω(t) is an annular-like bounded domain in R2 and ∂Ω(t)=J∪Γ(t). The mathematical formulation of the tumor model under study is as follows:
cσt(x,t)−Δσ(x,t)+σ(x,t)=0,x∈Ω(t),t>0, | (1.1) |
−Δp(x,t)=μ[σ(ξ(t−τ;x,t),t−τ)−˜σ],x∈Ω(t),t>0, | (1.2) |
{dξds=−∇p(ξ,s),t−τ≤s≤t,ξ=x,s=t, | (1.3) |
σ(x,t)=ˉσ,∂→np(x,t)=0,x∈J,t>0, | (1.4) |
∂→νσ(x,t)=0,p(x,t)=γκ(x,t),x∈Γ(t),t>0, | (1.5) |
V(x,t)=−∂→νp(x,t),x∈Γ(t),t>0, | (1.6) |
Γ(t)=Γ0,−τ≤t≤0, | (1.7) |
σ(x,t)=σ0(x),x∈Ω0,−τ≤t≤0, | (1.8) |
p(x,t)=p0(x),x∈Ω0,−τ≤t≤0. | (1.9) |
Here, σ and p denote the nutrient concentration and pressure within the tumor, respectively, which are to be determined together with Ω(t), and c=Tdiffusion /Tgrowth is the ratio of the nutrient diffusion time scale to the tumor growth (e.g., tumor doubling) time scale; thus, it is very small and can sometimes be set to be 0 (quasi-steady state approximation). Assume that the time delay τ is reflected between the time at which a cell commences mitosis and the time at which the daughter cells are produced and ξ(s;x,t) represents the cell location at time s as cells are moving with the velocity field →V, then the function ξ(s;x,t) satisfies
{dξds=→V(ξ,s),t−τ≤s≤t,ξ|s=t=x. | (1.10) |
In other words, ξ tracks the path of the cell currently located at x. (1.3) is further derived from (1.10) under the assumption of a porous medium structure for the tumor, where Darcy's law →V=−∇p holds true. Because of the presence of time delay, the tumor grows at a rate that is related to the nutrient concentration when it starts mitosis and a combination of the conservation of mass and Darcy's law yields (1.2), in which μ represents the growth intensity of the tumor and ˜σ is the nutrient concentration threshold required for tumor cell growth. Additionally, ˉσ is the nutrient concentration in the blood vessel, ˉσ>˜σ, V, κ and →ν denote the normal velocity, the mean curvature and the unit outward normal field of the outer boundary Γ(t), respectively, →n denotes the unit outward normal field of the fixed inner boundary J, and γ is the outer surface tension coefficient. Thus, the boundary condition σ=ˉσ on J indicates that the tumor receives constant nutrient supply from the blood vessel, ∂→νσ=0 on Γ(t) implies that the nutrient cannot pass through Γ(t), ∂→np=0 on J means that tumor cells cannot pass through the blood vessel wall, p=γκ on Γ(t) is due to the cell-to-cell adhesiveness, and V=−∂→νp on Γ(t) is the well-known Stefan condition representing that the normal velocity of the tumor cord outer boundary Γ(t) is the same with that of tumor cells adjacent to Γ(t). Finally, σ0(x), p0(x), Γ0 are given initial data and Ω(t)=Ω0 for −τ≤t≤0.
Before going to our interest, we prefer to recall some relevant works. Models for the growth of the strictly cylindrical tumor cord were studied in [11,12,13]. For the model (1.1)–(1.9) without the time delay, if c=0, Zhou and Cui [14] showed that the unique radially symmetric stationary solution exists and is asymptotically stable for any sufficiently small perturbations. Meanwhile, if c>0, Wu et al. [15] proved that the stationary solution is locally asymptotically stable provided that c is small enough. On the other hand, Zhao and Hu [16] considered the multicell spheroids with time delays. For the case c=0, they analyzed the linear stability of the radially symmetric stationary solution as well as the impact of the time delay.
Motivated by the works [14,15,16], here we aim to discuss the linear stability of stationary solutions to the problem (1.1)–(1.9) with the quasi-steady-state assumption, i.e., c=0, and investigate the effect of time delay on tumor growth. Our first main result is given below.
Theorem 1.1. For small time delay τ, the problem (1.1)–(1.9) admits a unique radially symmetric stationary solution.
Next, in order to deal with the linear stability of the radially symmetric stationary solution, denoted by (σ∗,p∗,Ω∗), where Ω∗={x∈R2: r0<r=|x|<R∗}, we assume that the initial conditions are perturbed as follows:
Ω(t)={x∈R2:r0<r<R∗+ερ0(θ)},−τ≤t≤0,σ(r,θ,t)=σ∗(r)+εw0(r,θ),p(r,θ,t)=p∗(r)+εq0(r,θ),−τ≤t≤0. |
The linearized problem of (1.1)–(1.9) at (σ∗,p∗,Ω∗) is then obtained by substituting
Ω(t):r0<r<R∗+ερ(θ,t)+O(ε2), | (1.11) |
σ(r,θ,t)=σ∗(r)+εw(r,θ,t)+O(ε2), | (1.12) |
p(r,θ,t)=p∗(r)+εq(r,θ,t)+O(ε2) | (1.13) |
into (1.1)–(1.9) and collecting the ε-order terms. Now, we can state the second main result of this paper.
Theorem 1.2. For small time delay τ, the radially symmetric stationary solution (σ∗,p∗,Ω∗) of (1.1)–(1.9) with c=0 is linearly stable, i.e.,
max0≤θ≤2π|ρ(θ,t)|≤Ce−δt,t>0 | (1.14) |
for some positive constants C and δ.
Remark 1.1. Compared with results of the problem modeling the growth of tumor cord without time delays in [14], the introduction of the time delay does not affect the stability of the radially symmetric stationary solution even under non-radial perturbations. However, as we shall see in Subsection 3.3, the numerical result shows that adding time delay would result in a larger stationary tumor. Moreover, the stronger the growth intensity of the tumor is, the greater the influence of time delay on the size of the stationary tumor is.
Remark 1.2. Compared with results of the nearly sphere-shaped tumor model with time delays in [16], which state that the radially symmetric stationary solution is linearly stable for small μ in the sense that limt→∞max0≤θ≤2π|ρ(θ,t)−(a1cosθ+b1sinθ)|=0 for some constants a1 and b1, the radially symmetric stationary solution of tumor cord with the time delay is linearly stable for any μ>0 in the normal sense.
This paper is organized as follows. In Section 2, we give the proof of Theorem 1.1 by first transforming the free boundary problem into an equivalent problem with fixed boundary and then applying the contraction mapping principle combined with Lp estimates to this fixed boundary problem. In Section 3, we prove Theorem 1.2 and Remark 1.1 by first introducing the linearization of (1.1)–(1.9) at the radially symmetric stationary solution (σ∗,p∗,Ω∗), and then making a delicate analysis of the expansion in the time delay τ provided that τ is sufficiently small. A brief conclusion in Section 4 completes the paper.
In this section, we study radially symmetric stationary solutions (σ∗,p∗,Ω∗) to the system (1.1)–(1.9), which satisfy
−Δrσ∗(r)+σ∗(r)=0,σ∗(r0)=ˉσ,σ′∗(R∗)=0,r0<r<R∗, | (2.1) |
−Δrp∗(r)=μ[σ∗(ξ(−τ;r,0))−˜σ],p′∗(r0)=0,p∗(R∗)=γR∗,r0<r<R∗, | (2.2) |
{dξds(s;r,0)=−∂p∗∂r(ξ(s;r,0)),−τ≤s≤0,ξ(s;r,0)=r,s=0, | (2.3) |
∫R∗r0[σ∗(ξ(−τ;r,0))−˜σ]rdr=0, | (2.4) |
where Δr is the radial part of the Laplacian in R2.
Before proceeding further, let us recall that the modified Bessel functions Kn(r) and In(r), standard solutions of the equation
r2y′′+ry′−(r2+n2)y=0,r>0, | (2.5) |
have the following properties:
In+1(r)=In−1(r)−2nrIn(r),Kn+1(r)=Kn−1(r)+2nrKn(r),n≥1, | (2.6) |
I′n(r)=12[In−1(r)+In+1(r)],K′n(r)=−12[Kn−1(r)+Kn+1(r)],n≥1, | (2.7) |
I′n(r)=In−1(r)−nrIn(r), K′n(r)=−Kn−1(r)−nrKn(r),n≥1, | (2.8) |
I′n(r)=nrIn(r)+In+1(r), K′n(r)=nrKn(r)−Kn+1(r),n≥0, | (2.9) |
In(r)Kn+1(r)+In+1(r)Kn(r)=1r,n≥0 | (2.10) |
and
I′n(r)>0,K′n(r)<0. |
Proof of Theorem 1.1 In view of (2.5), the solution of (2.1) is clearly given by
σ∗(r)=ˉσI0(r)K1(R∗)+I1(R∗)K0(r)I0(r0)K1(R∗)+I1(R∗)K0(r0). | (2.11) |
Introducing the notations:
ˆr=r−r0R∗−r0,ˆσ(ˆr)=σ∗(r),ˆp(ˆr)=(R∗−r0)p∗(r),ˆξ(s,ˆr,0)=ξ(s,r,0)−r0R∗−r0, |
(2.1)–(2.4) reduces to the following system after dropping the "^" in the above variables:
∂2σ∂r2+R∗−r0r(R∗−r0)+r0∂σ∂r=(R∗−r0)2σ,σ(0)=ˉσ,σ′(1)=0, | (2.12) |
{∂2p∂r2+R∗−r0r(R∗−r0)+r0∂p∂r=−μ(R∗−r0)3[σ(r+1(R∗−r0)3∫0−τ∂p∂r((R∗−r0)ξ(s;r,0)+r0)ds)−˜σ],p′(0)=0,p(1)=γ(R∗−r0)R∗, | (2.13) |
{dξds(s;r,0)=−1(R∗−r0)3∂p∂r((R∗−r0)ξ(s;r,0)+r0),−τ≤s≤0,ξ(s;r,0)=r,s=0, | (2.14) |
∫10[r(R∗−r0)+r0][σ(r+1(R∗−r0)3∫0−τ∂p∂r((R∗−r0)ξ(s;r,0)+r0)ds)−˜σ]dr=0. | (2.15) |
It is clear that (2.12) can be solved explicitly. For convenience, we extend the solution of (2.12) outside [0, 1]:
σ(r;R∗)={ˉσI0(r(R∗−r0)+r0)K1(R∗)+I1(R∗)K0(r(R∗−r0)+r0)I0(r0)K1(R∗)+I1(R∗)K0(r0),0≤r≤1,ˉσI0(R∗)K1(R∗)+I1(R∗)K0(R∗)I0(r0)K1(R∗)+I1(R∗)K0(r0),1<r≤2. | (2.16) |
Assume that Rmin and Rmax are positive constants to be determined later and r0<Rmin<Rmax. For any R∗∈[Rmin,Rmax], we will prove that p is also uniquely determined by applying the contraction mapping principle.
Noticing that 0 is a lower solution of (2.14), but there is no assurance that ξ(s;r,0)≤1 for −τ≤s≤0, we suppose ξ(s;r,0)∈[0,2] and take
X={p∈W2,∞[0,2];‖p‖W2,∞[0,2]≤M}, |
where M>0 is to be determined. For each p∈X, we first solve for ξ from (2.14) and substitute it into (2.13), then the following system
{∂2ˉp∂r2+R∗−r0r(R∗−r0)+r0∂ˉp∂r=−μ(R∗−r0)3[σ(r+1(R∗−r0)3∫0−τ∂p∂r((R∗−r0)ξ(s;r,0)+r0)ds)−˜σ],ˉp(1)=γ(R∗−r0)R∗,∂ˉp∂r(0)=0 | (2.17) |
allows a unique solution ˉp∈W2,∞[0,1]. Applying the strong maximum principle combined with the Hopf lemma to (2.1) shows that σ(r;R∗)≤ˉσ. Thus, integrating (2.17), we obtain
‖1r(R∗−r0)+r0∂ˉp∂r‖L∞[0,1]≤μ2(Rmax−r0)2(ˉσ+˜σ), | (2.18) |
‖ˉp‖L∞[0,1]≤γ(Rmax−r0)Rmin+μ4R2max(Rmax−r0)(ˉσ+˜σ), | (2.19) |
‖∂2ˉp∂r2‖L∞[0,1]≤3μ2(Rmax−r0)3(ˉσ+˜σ). | (2.20) |
Define the mapping
Lp(r)={ˉp(r),0≤r≤1,ˉp(1)+ˉp′(1)(r−1),1<r≤2,p∈X, |
then ‖Lp‖∈W2,∞[0,2] and ‖Lp‖W2,∞[0,2]≤2‖ˉp‖W2,∞[0,1]. Combining (2.18)–(2.20), we find
‖Lp‖W2,∞[0,2]≤2{μ2(Rmax−r0)2(ˉσ+˜σ)+3μ2(Rmax−r0)3(ˉσ+˜σ)+γ(Rmax−r0)Rmin+μ4R2max(Rmax−r0)(ˉσ+˜σ)}≜M1. | (2.21) |
If we choose M≥M1, then Lp∈X by (2.21) and L maps X to itself.
We now show that L is a contraction. Given p(1),p(2)∈X, one can first get ξ(1),ξ(2) from the following two systems:
{dξ(1)ds(s;r,0)=−1(R∗−r0)3∂p(1)∂r((R∗−r0)ξ(1)(s;r,0)+r0),−τ≤s≤0,ξ(1)(s;r,0)=r,s=0, | (2.22) |
{dξ(2)ds(s;r,0)=−1(R∗−r0)3∂p(2)∂r((R∗−r0)ξ(2)(s;r,0)+r0),−τ≤s≤0,ξ(2)(s;r,0)=r,s=0. | (2.23) |
Integrating (2.22) and (2.23) with regard to s over the interval [−τ,0] and making a subtraction yield
|ξ(1)−ξ(2)|≤τ(R∗−r0)3max−τ≤s≤00≤r≤1[|∂p(1)∂r((R∗−r0)ξ(1)+r0)−∂p(2)∂r((R∗−r0)ξ(1)+r0)|+|∂p(2)∂r((R∗−r0)ξ(1)+r0)−∂p(2)∂r((R∗−r0)ξ(2)+r0)|]≤τ(R∗−r0)3‖p(1)−p(2)‖W2,∞[0,2]+τM(R∗−r0)2max−τ≤s≤00≤r≤1|ξ(1)−ξ(2)| |
for all −τ≤s≤0 and 0≤r≤1. Consequently,
max−τ≤s≤00≤r≤1|ξ(1)−ξ(2)|≤τ(R∗−r0)3−τM(R∗−r0)‖p(1)−p(2)‖W2,∞[0,2]. | (2.24) |
Next, we substitute ξ(1),ξ(2) into (2.17) and solve for ˉp(1) and ˉp(2), respectively, then it follows from (2.17) that (ˉp(1)−ˉp(2))(1)=0, ∂∂r(ˉp(1)−ˉp(2))(0)=0 and
−∂2∂r2(ˉp(1)−ˉp(2))−R∗−r0r(R∗−r0)+r0∂∂r(ˉp(1)−ˉp(2))=μ(R∗−r0)3[σ(r+1(R∗−r0)3∫0−τ∂p(1)∂r((R∗−r0)ξ(1)(s;r,0)+r0)ds)−σ(r+1(R∗−r0)3∫0−τ∂p(2)∂r((R∗−r0)ξ(2)(s;r,0)+r0)ds)]. |
Using (2.24), we derive
‖1r(R∗−r0)+r0∂∂r(ˉp(1)−ˉp(2))‖L∞[0,1]≤μ2(R∗−r0)2‖σ(r+1(R∗−r0)3∫0−τ∂p(1)∂r((R∗−r0)ξ(1)+r0)ds)−σ(r+1(R∗−r0)3∫0−τ∂p(2)∂r((R∗−r0)ξ(2)+r0)ds)‖L∞[0,1]≤μ2(R∗−r0)‖∂σ∂r‖L∞[0,2]∫0−τ(∂p(1)∂r((R∗−r0)ξ(1)+r0)−∂p(2)∂r((R∗−r0)ξ(2)+r0))ds≤μτ2(R∗−r0)‖∂σ∂r‖L∞[0,2](‖p(1)−p(2)‖W2,∞[0,2]+(R∗−r0)‖p(2)‖W2,∞[0,2]max−τ≤s≤00≤r≤1|ξ(1)−ξ(2)|)≤M2τ‖p(1)−p(2)‖W2,∞[0,2] |
and similarly,
‖ˉp(1)−ˉp(2)‖L∞[0,1]≤M3τ‖p(1)−p(2)‖W2,∞[0,2],‖∂2∂r2(ˉp(1)−ˉp(2))‖L∞[0,1]≤M4τ‖p(1)−p(2)‖W2,∞[0,2], |
where
M2=μˉσRmax2r0(Rmax−r0)3(Rmin−r0)2−Mτ,M3=μˉσR3max4r0(Rmax−r0)2(Rmin−r0)2−Mτ,M4=3μˉσRmax2r0(Rmax−r0)4(Rmin−r0)2−Mτ. |
Here, we employed the fact that
‖∂σ∂r‖L∞[0,2]=‖∂σ∂r‖L∞[0,1]≤ˉσRmaxr0(Rmax−r0)2 |
by (2.1) and σ≤ˉσ. Let M5=M2+M3+M4, then M5 is independent of τ and
‖ˉp(1)−ˉp(2)‖W2,∞[0,1]≤M5τ‖p(1)−p(2)‖W2,∞[0,2], |
which together with Lp(1)(1)=Lp(2)(1)=γ(R∗−r0)R∗ and (Lp(1))′(0)=(Lp(2))′(0)=0 implies that
‖Lp(1)−Lp(2)‖W2,∞[0,2]≤2M5τ‖p(1)−p(2)‖W2,∞[0,2]. |
Hence, if τ is sufficiently small such that 2M5τ<1, then we derive a contracting mapping L. The existence and uniqueness of p are therefore obtained.
It suffices to prove that there exists a unique R∗∈[Rmin,Rmax] satisfying (2.15). Substituting (2.16) into (2.15), we find that it is equivalent to solving the following equation for R:
G(R,τ)=∫10r(R−r0)+r0R+r0[σ∗(r+1(R−r0)3∫0−τ∂p∗∂r((R−r0)ξ(s;r,0)+r0)ds)−˜σ]dr=0. |
Clearly,
G(R,0)=∫10(σ∗(r;R)−˜σ)r(R−r0)+r0R+r0dr=∫10σ∗(r;R)r(R−r0)+r0R+r0dr−˜σ2. |
Using Lemma 3.1 and Theorem 3.2 in [14] and the condition ˉσ>˜σ, we know that
limR→r0G(R,0)=ˉσ−˜σ2>0,limR→∞G(R,0)=−˜σ2<0,∂G(R,0)∂R<0, |
which implies that the equation G(R,0)=0 has a unique solution, denoted by RS, and
G(12(RS+r0),0)>0,G(32RS,0)<0. |
Since
∂G(R,τ)∂R=∂G(R,0)∂R+∂2G(R,η)∂R∂ττ+O(τ2),0≤η≤τ |
when τ is sufficiently small, ∂G(R,τ)∂R and ∂G(R,0)∂R have the same sign. Thus, G(R,τ) is monotone decreasing in R. Using the fact that G(R,τ) is continuous in τ, we further have
G(12(RS+r0),τ)>0,G(32RS,τ)<0. |
Hence, when τ is sufficiently small, the equation G(R,τ)=0 has a unique solution R∗. Taking Rmin=12(RS+r0) and Rmax=32RS, we complete the proof of the theorem.
This section is devoted to the linear stability of the radially symmetric stationary solution (σ∗,p∗,Ω∗) of the problem (1.1)–(1.9) and the effect of time delay on the stability and the size of the stationary tumor. Let (σ,p,Ω(t)), given by (1.11)–(1.13), be solutions to (1.1)–(1.9), and denote by →er, →eθ the unit normal vectors in r, θ directions, respectively. Written in the rectangular coordinates in R2,
→er=(cosθ,sinθ)T,→eθ=(−sinθ,cosθ)T. |
Using the notation ξ1(s;r,θ,t), ξ2(s;r,θ,t) for the polar radius and angle of ξ(s;r,θ,t), respectively, we have
ξ(s;r,θ,t)=ξ1(s;r,θ,t)→er(ξ)=ξ1(s;r,θ,t)(cosξ2(s;r,θ,t),sinξ2(s;r,θ,t))T. |
Expand ξ1, ξ2 in ε as
{ξ1=ξ10+εξ11+O(ε2),ξ2=ξ20+εξ21+O(ε2), | (3.1) |
then we derive from (1.3) and (1.13) that
{dξ10ds=−∂p∗∂r(ξ10),t−τ≤s≤t,ξ10|s=t=r; | (3.2) |
{dξ11ds=−∂2p∗∂r2(ξ10)ξ11−∂q∂r(ξ10,ξ20,s),t−τ≤s≤t,ξ11|s=t=0; | (3.3) |
{dξ20ds=0,t−τ≤s≤t,ξ20|s=t=θ; | (3.4) |
{dξ21ds=−1ξ210∂q∂θ(ξ10,ξ20,s),t−τ≤s≤t,ξ21|s=t=0. | (3.5) |
It is evident that ξ20≡θ. Noticing that the equation for ξ10 is the same as that for ξ∗ in the radially symmetric case, ξ10 is independent of θ.
Substituting (1.11)–(1.13) and (3.1)–(3.5) into (1.1), (1.2), (1.4)–(1.6), using the mean-curvature formula in the 2-dimensional case for the curve r=ρ(θ):
κ=ρ2+2ρ2θ−ρ⋅ρθθ(ρ2+(ρθ)2)3/2 |
and collecting the ε-order terms, we obtain the linearized system in BR∗×{t>0}:
Δω(r,θ,t)=ω(r,θ,t),ω(r0,θ,t)=0,∂ω∂r(R∗,θ,t)+σ∗(R∗)ρ(θ,t)=0, | (3.6) |
{Δq(r,θ,t)=−μ∂σ∗∂r(ξ10(t−τ;r,t))ξ11(t−τ;r,θ,t)−μw(ξ10(t−τ;r,t),θ,t−τ),∂q∂r(r0,θ,t)=0,q(R∗,θ,t)+γR2∗(ρ(θ,t)+∂2ρ∂θ2(θ,t))=0, | (3.7) |
∂ρ(θ,t)∂t=−∂q∂r(R∗,θ,t)−∂2p∗∂r2(R∗,θ,t)ρ(θ,t). | (3.8) |
Due to the presence of the time delay, the linearization problem (3.6)–(3.8) cannot be solved explicitly. Assume that ω,q, ρ and ξ11 have the following Fourier expansions:
{ω(r,θ,t)=A0(r,t)+∑∞n=1[An(r,t)cosnθ+Bn(r,t)sinnθ],q(r,θ,t)=E0(r,t)+∑∞n=1[En(r,t)cosnθ+Fn(r,t)sinnθ],ρ(θ,t)=a0(t)+∑∞n=1[an(t)cosnθ+bn(t)sinnθ],ξ11(s;r,θ,t)=e0(s;r,t)+∑∞n=1[en(s;r,t)cosnθ+fn(s;r,t)sinnθ]. | (3.9) |
Substituting (3.9) into (3.6)–(3.8) yields the following system in BR∗×{t>0}:
{∂2An∂r2(r,t)+1r∂An∂r(r,t)−n2r2An(r,t)=An(r,t),An(r0,t)=0,∂An∂r(R∗,t)+σ∗(R∗)an(t)=0, | (3.10) |
{∂2Bn∂r2(r,t)+1r∂Bn∂r(r,t)−n2r2Bn(r,t)=Bn(r,t),Bn(r0,t)=0,∂Bn∂r(R∗,t)+σ∗(R∗)bn(t)=0, | (3.11) |
{∂2En∂r2(r,t)+1r∂En∂r(r,t)−n2r2En(r,t)=−μ∂σ∗∂r(ξ10(t−τ;r,t))en(t−τ;r,t)−μAn(ξ10(t−τ;r,t),t−τ),∂En∂r(r0,t)=0,En(R∗,t)+γ(1−n2)R2∗an(t)=0, | (3.12) |
{∂2Fn∂r2(r,t)+1r∂Fn∂r(r,t)−n2r2Fn(r,t)=−μ∂σ∗∂r(ξ10(t−τ;r,t))fn(t−τ;r,t)−μBn(ξ10(t−τ;r,t),t−τ),∂Fn∂r(r0,t)=0,Fn(R∗,t)+γ(1−n2)R2∗bn(t)=0, | (3.13) |
{∂en∂s(s;r,t)=−∂2p∗∂r2(ξ10)en(s;r,t)−∂En∂r(ξ10,s),t−τ≤s≤t,en∣s=t=0, | (3.14) |
{∂fn∂s(s;r,t)=−∂2p∗∂r2(ξ10)fn(s;r,t)−∂Fn∂r(ξ10,s),t−τ≤s≤t,fn∣s=t=0, | (3.15) |
dan(t)dt=−∂2p∗∂r2(R∗)an(t)−∂En∂r(R∗,t), | (3.16) |
dbn(t)dt=−∂2p∗∂r2(R∗)bn(t)−∂Fn∂r(R∗,t). | (3.17) |
Since it is impossible to solve the systems (2.1)–(2.4) and (3.10)–(3.17) explicitly and the time delay τ is actually very small, in what follows, we analyze the expansion in τ for (2.1)–(2.4) and (3.10)–(3.17).
Let
R∗=R0∗+τR1∗+O(τ2),σ∗=σ0∗+τσ1∗+O(τ2),p∗=p0∗+τp1∗+O(τ2),An=A0n+τA1n+O(τ2),Bn=B0n+τB1n+O(τ2),En=E0n+τE1n+O(τ2),Fn=F0n+τF1n+O(τ2),an=a0n+τa1n+O(τ2),bn=b0n+τb1n+O(τ2). |
Substitute these expansions into (2.1)–(2.4) and (3.10)–(3.17). Since an(t) and bn(t) have the same asymptotic behavior at ∞, we will only make an analysis of an(t). For this, we discuss the expansions of R∗, σ∗, p∗, An, En and an. Since the equations for the expansions of σ∗, p∗, An, En and an are the same as those in [16], here we only compute the expansions of the boundary conditions of σ∗, p∗, An and En.
● Expansions of the boundary conditions of σ∗:
It follows from (2.10) and (2.11) that
σ∗(r)=ˉσK1(R∗)I0(r)+I1(R∗)K0(r)I0(r0)K1(R∗)+I1(R∗)K0(r0)=ˉσK1(R0∗)I0(r)+I1(R0∗)K0(r)I0(r0)K1(R0∗)+I1(R0∗)K0(r0)+τˉσR1∗R0∗I0(r0)K0(r)−K0(r0)I0(r)[I0(r0)K1(R0∗)+I1(R0∗)K0(r0)]2+O(τ2), |
which implies
σ0∗(r)=ˉσK1(R0∗)I0(r)+I1(R0∗)K0(r)I0(r0)K1(R0∗)+I1(R0∗)K0(r0), | (3.18) |
σ1∗(r)=ˉσR1∗R0∗I0(r0)K0(r)−K0(r0)I0(r)[I0(r0)K1(R0∗)+I1(R0∗)K0(r0)]2. | (3.19) |
By the boundary conditions in (2.1), we find
σ0∗(r0)+τσ1∗(r0)+O(τ2)=ˉσ,∂σ0∗∂r(R0∗)+τ∂2σ0∗∂r2(R0∗)R1∗+τ∂σ1∗∂r(R0∗)+O(τ2)=0. |
● Expansions of the boundary conditions of p∗:
One obtains from the boundary conditions in (2.2) that
∂p0∗∂r(r0)+τ∂p1∗∂r(r0)+O(τ2)=0,p0∗(R0∗)+τ∂p0∗∂r(R0∗)R1∗+τp1∗(R0∗)+O(τ2)=γR0∗−τγR1∗(R0∗)2+O(τ2). |
● Expansion of (2.4):
In view of (4.31) in [16], there holds
0=∫R∗r0[σ∗(ξ(−τ;r,0))−˜σ]rdr=∫R∗r0[σ0∗(r)−˜σ]rdr+τ∫R0∗r0(∂σ0∗∂r(r)∂p0∗∂r(r)+σ1∗(r))rdr+O(τ2). | (3.20) |
Using (3.18), we compute
∫R∗r0[σ0∗(r)−˜σ]rdr=∫R∗r0(ˉσK1(R0∗)I0(r)+I1(R0∗)K0(r)I0(r0)K1(R0∗)+I1(R0∗)K0(r0)−˜σ)rdr=ˉσr0I1(R0∗)K1(r0)−I1(r0)K1(R0∗)I0(r0)K1(R0∗)+I1(R0∗)K0(r0)+˜σ2[r20−(R0∗)2]+τR1∗(ˉσI0(r0)K1(R0∗)+I1(R0∗)K0(r0)−˜σR0∗)+O(τ2). | (3.21) |
A combination of (3.20) and (3.21) gives
τ[ˉσR1∗I0(r0)K1(R0∗)+I1(R0∗)K0(r0)−˜σR0∗R1∗+∫R0∗r0(∂σ0∗∂r(r)∂p0∗∂r(r)+σ1∗(r))rdr]+ˉσr0I1(R0∗)K1(r0)−I1(r0)K1(R0∗)I0(r0)K1(R0∗)+I1(R0∗)K0(r0)+˜σ2[r20−(R0∗)2]+O(τ2)=0. | (3.22) |
● Expansions of the boundary conditions of An:
We derive from the boundary conditions in (3.10) that
A0n(r0,t)+τA1n(r0,t)+O(τ2)=0,0=∂A0n∂r(R0∗+τR1∗,t)+τ∂A1n∂r(R0∗,t)+[σ0∗(R0∗+τR1∗)+τσ1∗(R0∗)][a0n(t)+τa1n(t)]+O(τ2)=∂A0n∂r(R0∗,t)+σ0∗(R0∗)a0n(t)+τ(∂2A0n∂r2(R0∗,t)R1∗+∂A1n∂r(R0∗,t)+∂σ0∗∂r(R0∗,t)R1∗a0n(t)+σ0∗(R0∗)a1n(t)+σ1∗(R0∗)a0n(t))+O(τ2). |
● Expansions of the boundary conditions of En:
Substituting the expansion of En into the boundary conditions in (3.12) yields
∂E0n∂r(r0,t)+τ∂E1n∂r(r0,t)+O(τ2)=0,0=E0n(R0∗+τR1∗,t)+τE1n(R0∗,t)+γ1−n2(R0∗+τR1∗)2[a0n(t)+τa1n(t)]+O(τ2)=E0n(R0∗,t)+γ1−n2(R0∗)2a0n(t)+τ(∂E0n∂r(R0∗,t)R1∗+E1n(R0∗,t)−2γ1−n2(R0∗)3R1∗a0n(t)+γ1−n2(R0∗)2a1n(t))+O(τ2). |
Collecting all zeroth-order terms in τ leads to the following system for r0<r<R0∗:
−∂2σ0∗∂r2−1r∂σ0∗∂r=−σ0∗,σ0∗(r0)=ˉσ,∂σ0∗∂r(R0∗)=0, | (3.23) |
−∂2p0∗∂r2−1r∂p0∗∂r=μ(σ0∗−˜σ),∂p0∗∂r(r0)=0,p0∗(R0∗)=γR0∗, | (3.24) |
ˉσr0I1(R0∗)K1(r0)−I1(r0)K1(R0∗)I0(r0)K1(R0∗)+I1(R0∗)K0(r0)+˜σ2[r20−(R0∗)2]=0, | (3.25) |
{−∂2A0n∂r2−1r∂A0n∂r+(n2r2+1)A0n=0,A0n(r0,t)=0,∂A0n∂r(R0∗,t)+σ0∗(R0∗)a0n(t)=0, | (3.26) |
{−∂2B0n∂r2−1r∂B0n∂r+(n2r2+1)B0n=0,B0n(r0,t)=0,∂B0n∂r(R0∗,t)+σ0∗(R0∗)b0n(t)=0, | (3.27) |
{−∂2E0n∂r2−1r∂E0n∂r+n2r2E0n=μA0n,∂E0n∂r(r0,t)=0,E0n(R0∗,t)=γn2−1(R0∗)2a0n(t), | (3.28) |
{−∂2F0n∂r2−1r∂F0n∂r+n2r2F0n=μB0n,∂F0n∂r(r0,t)=0,F0n(R0∗,t)=γn2−1(R0∗)2b0n(t), | (3.29) |
\begin{align} &\frac{\mathrm{d}a^0_n(t)}{\mathrm{d}t} = -\frac{\partial^2 p^0_\ast}{\partial r^2}(R^0_\ast)a^0_n(t)-\frac{\partial E^0_n}{\partial r}(R^0_\ast,t), \end{align} | (3.30) |
\begin{align} &\frac{\mathrm{d}b^0_n(t)}{\mathrm{d}t} = -\frac{\partial^2 p^0_\ast}{\partial r^2}(R^0_\ast)b^0_n(t)-\frac{\partial F^0_n}{\partial r}(R^0_\ast,t). \end{align} | (3.31) |
A direct calculation gives
\begin{align} &\frac{\partial p^0_\ast}{\partial r}(r) = \frac{\mu\bar{\sigma}}{I_0(r_0)K_1(R^0_\ast)+I_1(R^0_\ast)K_0(r_0)}\bigg\{K_1(R^0_\ast)\left(\frac{r_0}{r}I_1(r_0)-I_1(r)\right) \\ &\; \; \; \; \; \; \; \; \; \; \; \; \; \; \; \; \; \; \; \; \; \; \; \; \; \; \; \qquad\qquad+I_1(R^0_\ast)\left(K_1(r)-\frac{r_0}{r}K_1(r_0)\right)\bigg\}+\frac{\mu\tilde{\sigma}r}{2}-\frac{\mu\tilde{\sigma}r^2_0}{2r}, \end{align} | (3.32) |
\begin{align} &\frac{\partial^2 p^0_\ast}{\partial r^2}(R^0_\ast) = \frac{\mu\bar{\sigma}}{R^0_\ast[(R^0_\ast)^2-r^2_0]}\frac{2r_0R^0_\ast[I_1(R^0_\ast)K_1(r_0)-I_1(r_0)K_1(R^0_\ast)]-(R^0_\ast)^2+r^2_0}{I_0(r_0)K_1(R^0_\ast)+I_1(R^0_\ast)K_0(r_0)}, \end{align} | (3.33) |
\begin{align} &A^0_n(r,t) = \frac{\bar{\sigma}a^0_n(t)h_n(r_0,R^0_\ast)}{R^0_\ast }\frac{K_n(r_0)I_n(r)-I_n(r_0)K_n(r)}{I_0(r_0)K_1(R^0_\ast)+I_1(R^0_\ast)K_0(r_0)}, \end{align} | (3.34) |
\begin{align} &\frac{\partial A^0_n}{\partial r}(r,t) = \frac{-\bar{\sigma}a^0_n(t)h_n(r_0,R^0_\ast)}{R^0_\ast [I_0(r_0)K_1(R^0_\ast)+I_1(R^0_\ast)K_0(r_0)]}\frac1{h_n(r_0,r)}, \end{align} | (3.35) |
\begin{align} &\frac{\partial^2 A^0_n}{\partial r^2}(R^0_\ast,t) = \frac{-\bar{\sigma}a^0_n(t)h_n(r_0,R^0_\ast)g_n(r_0,R^0_\ast)}{R^0_\ast [I_0(r_0)K_1(R^0_\ast)+I_1(R^0_\ast)K_0(r_0)]}, \end{align} | (3.36) |
where
\begin{align} &h_n(r_0,x) = \frac1{I_n(r_0)\left(\frac{n}{x}K_n(x)-K_{n+1}(x)\right) -K_n(r_0)\left(\frac{n}{x}I_n(x)+I_{n+1}(x)\right)}, \\ &g_n(r_0,x) = I_n(r_0)\left[\left(1+\frac{n(n-1)}{x^2}\right)K_n(x)+\frac{1}{x}K_{n+1}(x)\right] \\ &\qquad\qquad\qquad\qquad-K_n(r_0)\bigg[\left(1+\frac{n(n-1)}{x^2}\right)I_n(x)-\frac{1}{x}I_{n+1}(x)\bigg]. \end{align} | (3.37) |
Let \eta^0_n = E^0_n+\mu A^0_n , then we find from (3.26) and (3.28) that \eta^0_n satisfies
\begin{align*} -\frac{\partial^2 \eta^0_n}{\partial r^2}-\frac{1}{r}\frac{\partial \eta^0_n}{\partial r}+\frac{n^2}{r^2}\eta^0_n = 0, \end{align*} |
whose solution is
\begin{align*} \eta^0_n(r,t) = C_1(t)r^n+C_2(t)r^{-n} \end{align*} |
and thus,
\begin{equation} E^0_n(r,t) = \eta^0_n(r,t)-\mu A^0_n(r,t) = C_1(t)r^n+C_2(t)r^{-n}-\mu A^0_n(r,t), \end{equation} | (3.38) |
where C_1(t) and C_2(t) are to be determined by the boundary conditions in (3.28). By (2.10), (3.34), (3.35) and (3.37), we get
\begin{align} C_1(t) = &\frac{\mu\bar{\sigma} a^0_n(t)h_n(r_0,R^0_\ast)}{nR^0_\ast[(R^0_\ast)^{2n}+r_0^{2n}]}\frac{n(R^0_\ast)^n[I_n(R^0_\ast)K_n(r_0)-I_n(r_0)K_n(R^0_\ast)]+r^n_0}{I_0(r_0)K_1(R^0_\ast)+I_1(R^0_\ast)K_0(r_0)} \\ &+\frac{(n^2-1)\gamma a^0_n(t)}{(R^0_\ast)^{2n}+r_0^{2n}}(R^0_\ast)^{n-2}, \end{align} | (3.39) |
\begin{align} C_2(t) = &\frac{\mu\bar{\sigma} a^0_n(t)h_n(r_0,R^0_\ast)r_0^n(R^0_\ast)^{n}}{nR^0_\ast[(R^0_\ast)^{2n}+r_0^{2n}]}\frac{nr_0^n[I_n(R^0_\ast)K_n(r_0)-I_n(r_0)K_n(R^0_\ast)]-(R^0_\ast)^n}{I_0(r_0)K_1(R^0_\ast)+I_1(R^0_\ast)K_0(r_0)} \\ &+\frac{(n^2-1)\gamma a^0_n(t)}{(R^0_\ast)^{2n}+r_0^{2n}}r_0^{2n}(R^0_\ast)^{n-2}. \end{align} | (3.40) |
Using (3.35) and (3.38)–(3.40), we further derive
\begin{align} \frac{\partial E^0_n}{\partial r}(r,t) = &\frac{\mu\bar{\sigma} a^0_n(t)h_n(r_0,R^0_\ast)}{R^0_\ast [I_0(r_0)K_1(R^0_\ast)+I_1(R^0_\ast)K_0(r_0)]}\bigg\{r_0^{n}\frac{r^{n-1}+(R^0_\ast)^{2n}r^{-n-1}}{(R^0_\ast)^{2n}+r_0^{2n}} \\ &\; \; \; \; \; \; \; \; \; \; +n(R^0_\ast)^n\frac{r^{n-1}-r_0^{2n}r^{-n-1}}{(R^0_\ast)^{2n}+r_0^{2n}}[I_n(R^0_\ast)K_n(r_0)-I_n(r_0)K_n(R^0_\ast)] \\ &\; \; \; \; \; \; \; \; \; \; +\frac1{h_n(r_0,r)}\bigg\}+n(n^2-1)\gamma a^0_n(t)(R^0_\ast)^{n-2}\frac{r^{n-1}-r_0^{2n}r^{-n-1}}{(R^0_\ast)^{2n}+r_0^{2n}}. \end{align} | (3.41) |
Substituting (3.33) and (3.41) into (3.30) yields
\begin{equation} \frac{\mathrm{d}a^0_n(t)}{\mathrm{d}t} = U_n(r_0,R^0_\ast)a^0_n(t), \end{equation} | (3.42) |
whose solution is explicitly given by
\begin{equation} a^0_n(t) = a^0_n(0)\exp\{U_n(r_0,R^0_\ast)t\}. \end{equation} | (3.43) |
Here,
\begin{align} U_n(r_0,R^0_\ast) = &\frac{\mu\bar{\sigma}h_n(r_0,R^0_\ast)} {I_0(r_0)K_1(R^0_\ast)+I_1(R^0_\ast)K_0(r_0)} \bigg[\frac{2r_0(I_1(r_0)K_1(R^0_\ast)-I_1(R^0_\ast)K_1(r_0))} {h_n(r_0,R^0_\ast)[(R^0_\ast)^2-r_0^2]} \\ &+\frac{n}{(R^0_\ast)^2}\frac{(R^0_\ast)^{2n}-r_0^{2n}}{(R^0_\ast)^{2n}+r_0^{2n}} (I_n(r_0)K_n(R^0_\ast)-I_n(R^0_\ast)K_n(r_0)) -\frac{2r_0^n(R^0_\ast)^{n-2}}{(R^0_\ast)^{2n}+r_0^{2n}}\bigg] \\ &-\frac{\gamma n(n^2-1) }{(R^0_\ast)^3}\frac{(R^0_\ast)^{2n}-r_0^{2n}}{(R^0_\ast)^{2n}+r_0^{2n}}. \end{align} | (3.44) |
It was proven in Lemma 4.4 of [14] that U_n(r_0, R^0_\ast) < 0 for any n\geq 0 . Thus, we have the following:
Lemma 3.1. For any n\geq 0 , there exists \delta > 0 such that |a_0^n(t)|\leq|a_0^n(0)|e^{-\delta t} for all t > 0 .
Lemma 3.1 shows that a_n^0(t) decays to 0 exponentially at +\infty ; hence, when \tau = 0 , the radially symmetric stationary solution is asymptotically stable for all \mu > 0 .
Recalling that R_\ast = R^0_\ast + \tau R^1_\ast + O(\tau^2) , in order to see the effect of the time delay \tau on the size of the stationary tumor, in this subsection we discuss the sign of R^1_\ast by a theoretical analysis combined with numerical simulations.
We obtain from (3.22) that
\begin{equation} \frac{\bar{\sigma}R^1_\ast}{I_0(r_0)K_1(R^0_\ast)+I_1(R^0_\ast)K_0(r_0)}-\tilde{\sigma}R^0_\ast R^1_\ast+\int^{R^0_\ast}_{r_0}\left(\frac{\partial \sigma^0_\ast}{\partial r}(r)\frac{\partial p^0_\ast}{\partial r}(r)+\sigma^1_\ast(r)\right)rdr = 0, \end{equation} | (3.45) |
then by using (2.6)–(2.10), (3.18), (3.19), (3.25) and (3.32), one can solve (3.45) to obtain
\begin{equation} R^1_\ast = -\frac{\mu\bar{\sigma}}{2R^0_\ast}\frac{T(r_0,R^0_\ast)}{S(r_0,R^0_\ast)}, \end{equation} | (3.46) |
where
\begin{align*} T(r_0,R^0_\ast)& = 1-r_0^2[I_0(r_0)K_1(R^0_\ast)+I_1(R^0_\ast)K_0(r_0)]^2 +r_0^2\frac{(R^0_\ast)^2-r_0^2-4}{(R^0_\ast)^2-r_0^2} [I_1(R^0_\ast)K_1(r_0)-I_1(r_0)K_1(R^0_\ast)]^2, \\ S(r_0,R^0_\ast)& = \frac{2r_0}{(R^0_\ast)^2-r_0^2}[I_1(r_0)K_1(R^0_\ast)-I_1(R^0_\ast)K_1(r_0)] [I_0(r_0)K_1(R^0_\ast) +I_1(R^0_\ast)K_0(r_0)]+\frac{1}{(R^0_\ast)^2}. \end{align*} |
Since
U_0(r_0, R^0_\ast) = \frac{\mu\bar{\sigma}}{[I_0(r_0)K_1(R^0_\ast)+I_1(R^0_\ast)K_0(r_0)]^2}S(r_0,R^0_\ast) |
by (3.44), we know S(r_0, R^0_\ast) < 0 . Additionally, we numerically compute the function T(r_0, R^0_\ast) and find it is positive (see Figure 1). Hence, it follows from (3.46) that R^1_\ast > 0 and R^1_\ast is monotone increasing in \mu .
Remark 3.1. The discussion above indicates that the presence of the time delay leads to a larger stationary tumor. Furthermore, the bigger the tumor aggressive parameter \mu is, the greater the effect of time delay on the size of the stationary tumor is.
Now, we tackle the system consisting of all the first-order terms in \tau for r_0 < r < R^0_\ast :
\begin{align} &-\frac{\partial^2 \sigma^1_\ast}{\partial r^2}-\frac{1}{r}\frac{\partial \sigma^1_\ast}{\partial r} = -\sigma^1_\ast, \quad \sigma^1_\ast(r_0) = 0, \quad \frac{\partial \sigma^1_\ast}{\partial r}(R^0_\ast)+\frac{\partial^2 \sigma^0_\ast}{\partial r^2}(R^0_\ast)R^1_\ast = 0, \end{align} | (3.47) |
\begin{align} &\left\{\begin{array}{l} -\frac{\partial^2 p^1_\ast}{\partial r^2}-\frac{1}{r}\frac{\partial p^1_\ast}{\partial r} = \mu\frac{\partial \sigma^0_\ast}{\partial r}\frac{\partial p^0_\ast}{\partial r}+\mu\sigma^1_\ast, \\ \frac{\partial p^1_\ast}{\partial r}(r_0) = 0,\quad p_\ast^1(R_\ast^0) = -\frac{\gamma R_\ast^1}{(R_\ast^0)^2}-\frac{\partial p_\ast^0}{\partial r}(R_\ast^0)R_\ast^1, \end{array}\right. \end{align} | (3.48) |
\begin{align} &\frac{\bar{\sigma}R^1_\ast}{I_0(r_0)K_1(R^0_\ast)+I_1(R^0_\ast)K_0(r_0)}-\tilde{\sigma}R^0_\ast R^1_\ast+\int^{R^0_\ast}_{r_0}\left(\frac{\partial \sigma^0_\ast}{\partial r}(r)\frac{\partial p^0_\ast}{\partial r}(r)+\sigma^1_\ast(r)\right)rdr = 0, \end{align} | (3.49) |
\begin{align} &\left\{\begin{array}{l} -\frac{\partial^2 A^1_n}{\partial r^2}-\frac{1}{r}\frac{\partial A^1_n}{\partial r}+\left(\frac{n^2}{r^2}+1\right)A^1_n = 0, \\ A^1_n(r_0,t) = 0, \quad\frac{\partial^2 A^0_n}{\partial r^2}(R^0_\ast,t)R^1_\ast+\frac{\partial A^1_n}{\partial r}(R^0_\ast,t)+\sigma^0_\ast(R^0_\ast)a^1_n(t)+\sigma^1_\ast(R^0_\ast)a^0_n(t) = 0, \end{array}\right. \end{align} | (3.50) |
\begin{align} &\left\{\begin{array}{l} -\frac{\partial^2 B^1_n}{\partial r^2}-\frac{1}{r}\frac{\partial B^1_n}{\partial r}+\left(\frac{n^2}{r^2}+1\right)B^1_n = 0, \\ B^1_n(r_0,t) = 0, \quad\frac{\partial^2 B^0_n}{\partial r^2}(R^0_\ast,t)R^1_\ast+\frac{\partial B^1_n}{\partial r}(R^0_\ast,t)+\sigma^0_\ast(R^0_\ast)b^1_n(t)+\sigma^1_\ast(R^0_\ast)b^0_n(t) = 0, \end{array}\right. \end{align} | (3.51) |
\begin{align} &\left\{\begin{array}{l} -\frac{\partial^2 E^1_n}{\partial r^2}-\frac{1}{r}\frac{\partial E^1_n}{\partial r}+\frac{n^2}{r^2}E^1_n = \mu\frac{\partial \sigma^0_\ast}{\partial r}\frac{\partial E^0_n}{\partial r}+\mu\frac{\partial A_n^0}{\partial r}\frac{\partial p^0_\ast}{\partial r}-\mu\frac{\partial A_n^0}{\partial t}+\mu A_n^1, \\ \frac{\partial E^1_n}{\partial r}(r_0,t) = 0,\; E^1_n(R_\ast^0,t) = \gamma\frac{n^2-1}{(R_\ast^0)^2}a^1_n(t)-\frac{\partial E^0_n}{\partial r}(R_\ast^0,t)R_\ast^1-2\gamma\frac{n^2-1}{(R_\ast^0)^3}R_\ast^1a^0_n(t), \end{array}\right. \end{align} | (3.52) |
\begin{align} &\left\{\begin{array}{l} -\frac{\partial^2 F^1_n}{\partial r^2}-\frac{1}{r}\frac{\partial F^1_n}{\partial r}+\frac{n^2}{r^2}F^1_n = \mu\frac{\partial \sigma^0_\ast}{\partial r}\frac{\partial F^0_n}{\partial r}+\mu\frac{\partial B_n^0}{\partial r}\frac{\partial p^0_\ast}{\partial r}-\mu\frac{\partial B_n^0}{\partial t}+\mu B_n^1, \\ \frac{\partial F^1_n}{\partial r}(r_0,t) = 0,\; F^1_n(R_\ast^0,t) = \gamma\frac{n^2-1}{(R_\ast^0)^2}b^1_n(t)-\frac{\partial F^0_n}{\partial r}(R_\ast^0,t)R_\ast^1-2\gamma\frac{n^2-1}{(R_\ast^0)^3}R_\ast^1b^0_n(t), \end{array}\right. \end{align} | (3.53) |
\begin{align} &\frac{\mathrm{d}a_n^1(t)}{\mathrm{d}t} = -\frac{\partial^2 p_\ast^0}{\partial r^2}(R_\ast^0) a_n^1(t)-\frac{\partial^3 p_\ast^0}{\partial r^3}(R_\ast^0)R_\ast^1a_n^0(t) \\ &\qquad\qquad-\frac{\partial^2 p_\ast^1}{\partial r^2}(R_\ast^0) a_n^0(t)-\frac{\partial^2 E_n^0}{\partial r^2}(R_\ast^0,t)R_\ast^1-\frac{\partial E_n^1}{\partial r}(R_\ast^0,t), \end{align} | (3.54) |
\begin{align} &\frac{\mathrm{d}b_n^1(t)}{\mathrm{d}t} = -\frac{\partial^2 p_\ast^0}{\partial r^2}(R_\ast^0) b_n^1(t)-\frac{\partial^3 p_\ast^0}{\partial r^3}(R_\ast^0)R_\ast^1b_n^0(t) \\ &\qquad\qquad-\frac{\partial^2 p_\ast^1}{\partial r^2}(R_\ast^0) b_n^0(t)-\frac{\partial^2 F_n^0}{\partial r^2}(R_\ast^0,t)R_\ast^1-\frac{\partial F_n^1}{\partial r}(R_\ast^0,t). \end{align} | (3.55) |
To obtain the asymptotic behavior of a_n^1(t) as \infty , by (3.54) and the boundedness of the modified Bessel functions I_n(r) and K_n(r) on [r_0, R^0_\ast] , it suffices to analyze \frac{\partial E^1_n}{\partial r}(R^0_\ast, t) . For this purpose, in view of (3.52), we first compute A^1_n(r, t) . Solving (3.50) yields
\begin{align} A_n^1(r,t) = \frac{\bar{\sigma}h_n(r_0,R^0_\ast)}{R^0_\ast} \frac{I_n(r_0)K_n(r)-K_n(r_0)I_n(r)}{I_0(r_0)K_1(R^0_\ast)+I_1(R^0_\ast)K_0(r_0)} V_n(r_0,R^0_\ast,R^1_\ast,a^0_n(t),a^1_n(t)) \end{align} | (3.56) |
with
\begin{align*} V_n(r_0,R^0_\ast,R^1_\ast,a^0_n(t),a^1_n(t)) = &a_n^0(t)R^1_\ast h_n(r_0,R^0_\ast)g_n(r_0,R^0_\ast)-a_n^1(t) \\ &+a_n^0(t)R^1_\ast\frac{I_0(R^0_\ast)K_0(r_0)-I_0(r_0)K_0(R^0_\ast)} {I_0(r_0)K_1(R^0_\ast)+I_1(R^0_\ast)K_0(r_0)}, \end{align*} |
where we have employed (2.8)–(2.10), (3.19), (3.36) and (3.37). Furthermore,
\begin{align} \frac{\partial A_n^1}{\partial r}(r,t) = \frac{\bar{\sigma}h_n(r_0,R^0_\ast)V_n(r_0,R^0_\ast,R^1_\ast,a^0_n(t),a^1_n(t))} {R^0_\ast[I_0(r_0)K_1(R^0_\ast)+I_1(R^0_\ast)K_0(r_0)]} \frac1{h_n(r_0,r)}. \end{align} | (3.57) |
Next, being similar to the computation of E^0_n , we set \eta^1_n = E^1_n+\mu A^1_n , then we derive from (3.50), (3.52) and (3.56) that
\begin{align} &\left\{\begin{array}{l} -\frac{\partial^2 \eta^1_n}{\partial r^2}-\frac{1}{r}\frac{\partial \eta^1_n}{\partial r}+\frac{n^2}{r^2}\eta^1_n = \mu\frac{\partial \sigma^0_\ast}{\partial r}\frac{\partial E^0_n}{\partial r}+\mu\frac{\partial A^0_n}{\partial r}\frac{\partial p^0_\ast}{\partial r}-\mu\frac{\partial A^0_n}{\partial t}, \\ \frac{\partial \eta^1_n}{\partial r}(r_0,t) = \mu\frac{\partial A^1_n}{\partial r}(r_0,t),\quad \eta^1_n(R^0_\ast,t) = E^1_n(R^0_\ast,t)+\mu A^1_n(R^0_\ast,t). \end{array}\right. \end{align} | (3.58) |
For brevity, we introduce the differential operator L_n = -\partial_{rr}-\frac1r\partial_r+\frac{n^2}{r^2} and write \eta^1_n = u^{(1)}_n+u^{(2)}_n+u^{(3)}_n+u^{(4)}_n , where u^{(1)}_n , u^{(2)}_n , u^{(3)}_n and u^{(4)}_n solve the following problems, respectively:
\begin{align} &\left\{\begin{array}{l} L_nu^{(1)}_n = \mu\frac{\partial\sigma^0_\ast}{\partial r}\frac{\partial E^0_n}{\partial r}, \\ \frac{\partial u^{(1)}_n}{\partial r}(r_0,t) = 0, \quad u^{(1)}_n(R^0_\ast,t) = 0; \end{array}\right. \end{align} | (3.59) |
\begin{align} &\left\{\begin{array}{l} L_nu^{(2)}_n = \mu\frac{\partial A^0_n}{\partial r}\frac{\partial p^0_\ast}{\partial r}, \\ \frac{\partial u^{(2)}_n}{\partial r}(r_0,t) = 0, \quad u^{(2)}_n(R^0_\ast,t) = 0; \end{array}\right. \end{align} | (3.60) |
\begin{align} &\left\{\begin{array}{l} L_nu^{(3)}_n = -\mu\frac{\partial A^0_n}{\partial t}, \\ \frac{\partial u^{(3)}_n}{\partial r}(r_0,t) = 0, \quad u^{(3)}_n(R^0_\ast,t) = 0; \end{array}\right. \end{align} | (3.61) |
\begin{align} &\left\{\begin{array}{l} L_nu^{(4)}_n = 0, \\ \frac{\partial u^{(4)}_n}{\partial r}(r_0,t) = \mu\frac{\partial A_n^1}{\partial r}(r_0,t), \quad u^{(4)}_n(R^0_\ast,t) = E^1_n(R^0_\ast,t)+\mu A^1_n(R^0_\ast,t). \end{array}\right. \end{align} | (3.62) |
Let us first estimate u^{(1)}_n . By (3.18), (3.41) and (3.59), we have
\begin{align} L_nu^{(1)}_n = &\mu\bar{\sigma}a_n^0(t)\frac{K_1(R^0_\ast)I_1(r)-I_1(R^0_\ast)K_1(r)}{I_0(r_0)K_1(R^0_\ast)+I_1(R^0_\ast)K_0(r_0)}\bigg\{n(n^2-1)\gamma(R^0_\ast)^{n-2}\frac{r^{n-1}-r_0^{2n}r^{-n-1}}{(R^0_\ast)^{2n}+r_0^{2n}} \\ &\; \; \; \; \; \; \; \; \; \; \; +\frac{\mu\bar{\sigma}h_n(r_0,R^0_\ast)}{R^0_\ast[I_0(r_0)K_1(R^0_\ast)+I_1(R^0_\ast)K_0(r_0)]} \bigg[\frac1{h_n(r_0,r)}+r_0^n\frac{r^{n-1}+(R^0_\ast)^{2n}r^{-n-1}}{(R^0_\ast)^{2n}+r_0^{2n}} \\ &\; \; \; \; \; \; \; \; \; \; \; +n(R^0_\ast)^n\frac{r^{n-1}-r_0^{2n}r^{-n-1}}{(R^0_\ast)^{2n}+r_0^{2n}}(I_n(R^0_\ast)K_n(r_0)-I_n(r_0)K_n(R^0_\ast))\bigg]\bigg\}. \end{align} | (3.63) |
Based on the properties of the modified Bessel functions I_n(r) and K_n(r) , the righthand side of (3.63) is less than Q(n)a^0_n(t) when r_0 \leq r < R^0_\ast . Here, Q(n) denotes a polynomial function of n . Similar estimates can be established for u^{(2)}_n and u^{(3)}_n by (3.60) and (3.61).
Lemma 3.2. Consider the elliptic problem
\begin{align} &-\Delta\omega(x,t)+\frac{n^2}{|x|^2}\omega(x,t) = b(x,t), \qquad x\in\Omega_R, \end{align} | (3.64) |
\begin{align} &\partial_{\vec n}\omega\big|_{|x| = r_0} = 0, \quad \omega\big|_{|x| = R} = 0, \end{align} | (3.65) |
where \Omega_R = \big\{x\in \mathbb{R}^2: r_0 < |x| < R\big\} . If b(x, t) = b(|x|, t) and b(\cdot, t)\in L^2(\Omega_R) , then the problem (3.64) and (3.65) admits a unique solution \omega in H^2(\Omega_R) with estimates
\begin{align} \|\omega(\cdot,t)\|_{H^2(\Omega_R)}\leq C\bigg(\int^R_{r_0}|b(r,t)|^2rdr\bigg)^{1/2}; \end{align} | (3.66) |
\begin{align} \big\|\partial_{\vec\nu}\omega(\cdot,t)\big\|_{L^\infty(\partial B_R)}\leq C\bigg(\int^R_{r_0}|b(r,t)|^2rdr\bigg)^{1/2}, \end{align} | (3.67) |
where the constant C in (3.66) and (3.67) is independent of n .
The lemma can be proven by combining the proofs of [16, Lemma 4.6] and [17, Lemma 3.2]. The details are omitted here.
Lemma 3.2 ensures the existence and uniqueness of u^{(k)}_n in H^2(\Omega{_\ast}) for k = 1, 2, 3 . Furthermore, there holds
\begin{equation} \bigg|\frac{\partial u^{(1)}_n}{\partial r}(R^0_\ast,t)\bigg|+\bigg|\frac{\partial u^{(2)}_n}{\partial r}(R^0_\ast,t)\bigg|+\bigg|\frac{\partial u^{(3)}_n}{\partial r}(R^0_\ast,t)\bigg|\leq Ce^{-\delta t}. \end{equation} | (3.68) |
Obviously, the solution u^{(4)}_n to the problem (3.62) has the form:
\begin{equation} u^{(4)}_n(r,t) = C_5(t)r^n+C_6(t)r^{-n}, \end{equation} | (3.69) |
where C_5(t) and C_6(t) are determined by the boundary conditions in (3.62). Using (2.10), (3.41), (3.56), (3.57) and the boundary conditions in (3.52), we get
\begin{align} C_5(t) = &\frac{a_n^1(t)}{(R^0_\ast)^{2n}+r_0^{2n}}\bigg\{\frac{\mu\bar{\sigma}h_n(r_0,R^0_\ast)}{R^0_\ast[I_0(r_0)K_1(R^0_\ast)+I_1(R^0_\ast)K_0(r_0)]}\bigg[\frac{r_0^n}{n}+(R^0_\ast)^n(K_n(r_0)I_n(R^0_\ast) \\ &\; \; \; \; \; \; \; \; \; \; \; \; \; \; \; \; \; \; -I_n(r_0)K_n(R^0_\ast))\bigg]+\gamma (n^2-1)(R^0_\ast)^{n-2}\bigg\}+H_1(r_0,R^0_\ast,R^1_\ast)a_n^0(t), \end{align} | (3.70) |
\begin{align} C_6(t) = &\frac{a_n^1(t)r_0^n(R^0_\ast)^n}{(R^0_\ast)^{2n}+r_0^{2n}}\bigg\{\frac{\mu\bar{\sigma}h_n(r_0,R^0_\ast)}{R^0_\ast[I_0(r_0)K_1(R^0_\ast)+I_1(R^0_\ast)K_0(r_0)]}\bigg[-\frac{(R^0_\ast)^n}{n}+r_0^n(K_n(r_0)I_n(R^0_\ast) \\ &\; \; \; \; \; \; \; \; \; \; \; \; \; \; \; \; \; \; -I_n(r_0)K_n(R^0_\ast))\bigg]+\frac{\gamma (n^2-1)r_0^n}{(R^0_\ast)^2}\bigg\}+H_2(r_0,R^0_\ast,R^1_\ast)a_n^0(t), \end{align} | (3.71) |
where H_1 , H_2 are functions of r_0 , R^0_\ast and R^1_\ast .
Now, since
\begin{align*} E^1_n(r,t) = \eta^1_n-\mu A^1_n = u^{(1)}_n+u^{(2)}_n+u^{(3)}_n+u^{(4)}_n-\mu A^1_n, \end{align*} |
we derive
\begin{align} &\frac{\partial E^1_n}{\partial r}(R^0_\ast,t) = \frac{\partial u^{(1)}_n}{\partial r}(R^0_\ast,t)+\frac{\partial u^{(2)}_n}{\partial r}(R^0_\ast,t)+\frac{\partial u^{(3)}_n}{\partial r}(R^0_\ast,t)+\frac{\partial u^{(4)}_n}{\partial r}(R^0_\ast,t)-\mu\frac{\partial A^1_n}{\partial r}(R^0_\ast,t). \end{align} | (3.72) |
By (3.33), (3.57) and (3.69)–(3.72), we obtain from (3.54) that
\begin{align*} \frac{\mathrm{d}a^1_n(t)}{\mathrm{d}t} = &-\frac{\partial^2 p^0_\ast}{\partial r^2}(R^0_\ast)a^1_n(t)-\frac{\partial^3 p^0_\ast}{\partial r^3}(R^0_\ast)R^1_\ast a^0_n(t)-\frac{\partial^2 p^1_\ast}{\partial r^2}(R^0_\ast)a^0_n(t)-\frac{\partial^2 E^0_n}{\partial r^2}(R^0_\ast,t)R^1_\ast-\frac{\partial E^1_n}{\partial r}(R^0_\ast,t) \\ = &a_n^1(t)\bigg\{\frac{\mu\bar{\sigma}h_n(r_0,R^0_\ast)} {I_0(r_0)K_1(R^0_\ast)+I_1(R^0_\ast)K_0(r_0)} \bigg[\frac{n}{(R^0_\ast)^2}\frac{(R^0_\ast)^{2n}-r_0^{2n}}{(R^0_\ast)^{2n} +r_0^{2n}}(I_n(r_0)K_n(R^0_\ast)-I_n(R^0_\ast)K_n(r_0)) \\ &\; \; \; \; \; \; \; \; \; \; \; +\frac{2 r_0(I_1(r_0)K_1(R^0_\ast)-I_1(R^0_\ast)K_1(r_0))} {(R^0_\ast)^2-r_0^2}\frac1{h_n(r_0,R^0_\ast)}-\frac{2r_0^n(R^0_\ast)^{n-2}}{(R^0_\ast)^{2n}+r_0^{2n}}\bigg] \\ &\; \; \; \; \; \; \; \; \; \; \; -\frac{\gamma n(n^2-1)}{(R^0_\ast)^3}\frac{(R^0_\ast)^{2n}-r_0^{2n}}{(R^0_\ast)^{2n}+r_0^{2n}}\bigg\}+\tilde{H}(n,r_0,R^0_\ast,R^1_\ast)a_n^0(t) \\ &-\frac{\partial u^{(1)}_n}{\partial r}(R^0_\ast,t)-\frac{\partial u^{(2)}_n}{\partial r}(R^0_\ast,t)-\frac{\partial u^{(3)}_n}{\partial r}(R^0_\ast,t) \\ = &a_n^1(t)U_n(r_0,R^0_\ast)-\frac{\partial u^{(1)}_n}{\partial r}(R^0_\ast,t)-\frac{\partial u^{(2)}_n}{\partial r}(R^0_\ast,t)-\frac{\partial u^{(3)}_n}{\partial r}(R^0_\ast,t) +\tilde{H}(n,r_0,R^0_\ast,R^1_\ast)a_n^0(t), \end{align*} |
where \tilde{H} is a known function of n , r_0 , R^0_\ast , R^1_\ast and satisfies
\begin{equation} |\tilde{H}(n,r_0,R^0_\ast,R^1_\ast)|\leq C. \end{equation} | (3.73) |
Thus, using Lemma 3.1, (3.68) and (3.73) gives
\begin{align} \bigg|&\frac{\mathrm{d}a_n^1(t)}{\mathrm{d}t}-a_n^1(t)U_n(r_0,R^0_\ast)\bigg| \\ \leq& |\tilde{H}(n,r_0,R^0_\ast,R^1_\ast)a_n^0(t)|+\bigg|\frac{\partial u^{(1)}_n}{\partial r}(R^0_\ast,t)\bigg|+\bigg|\frac{\partial u^{(2)}_n}{\partial r}(R^0_\ast,t)\bigg|+\bigg|\frac{\partial u^{(3)}_n}{\partial r}(R^0_\ast,t)\bigg| \\ \leq& Ce^{-\delta t}. \end{align} | (3.74) |
In addition, for n\geq0 , Lemma 3.1 implies
\begin{equation*} -U_n(r_0,R^0_\ast) > \delta > 0. \end{equation*} |
Therefore, applying [16, Lemma 4.7] to (3.74) yields
\begin{align} |a^1_n(t)|\leq Ce^{-\delta t}, \qquad \quad t > 0, \end{align} | (3.75) |
i.e., a^1_n(t) decays exponentially as t\to\infty . Noticing that b_n(t) and a_n(t) have the same asymptotic behavior, we also have
\begin{align} |b^0_n(t)|+|b^1_n(t)|\leq Ce^{-\delta t},\qquad t > 0. \end{align} | (3.76) |
Proof of Theorem 1.2 The desired result (1.14) follows from Lemma 3.1, (3.75) and (3.76). The proof is complete.
Remark 3.2. The results on tumor cord without time delays in [14] show that the radially symmetric stationary solution is asymptotically stable under nonradially symmetric perturbations. Here, our Theorem 1.2 says that such asymptotic stability does not be affected by small time delay.
In this paper, we have investigated the effects of a time delay in cell proliferation on the growth of tumor cords, where the domain is a bounded subset in \mathbb{R}^2 and its boundary consists of two disjoint closed curves, one fixed and the other moving and a priori unknown. The existence, uniqueness and linear stability of the radially symmetric stationary solution were studied.
Here are some interesting findings. 1) Adding the time delay would not change the stability of the radially symmetric stationary solution when compared with the same system without delay [14], but adding the time delay would result in a larger stationary tumor. The bigger the tumor growth intensity \mu is, the greater impact that time delay has on the size of the stationary tumor. 2) By the result of [16], we know that for tumor spheroids with the same time delay, there exists a threshold \mu_\ast > 0 for the tumor aggressiveness constant \mu such that only for \mu < \mu_\ast , the radially symmetric stationary solution is linearly stable under non-radial perturbations. For tumor cords, however, from Theorem 1.2 we saw that the radially symmetric stationary solution is always linearly stable, regardless of the value of \mu . It showed that there is an essential difference between tumor cords and tumor spheroids with the same time delay.
We think that the linear stability analysis for the full system without quasi-steady state simplification, i.e., c > 0 , may be very challenging, which we expect to solve in future work.
The authors declare they have not used Artificial Intelligence (AI) tools in the creation of this article.
The authors would like to thank the reviewers for their valuable suggestions. This work was partly supported by the National Natural Science Foundation of China (No. 12161045 and No. 12261047), Jiangxi Provincial Natural Science Foundation (No. 20224BCD41001 and No. 20232BAB201010), the Science and Technology Planning Project from Educational Commission of Jiangxi Province, China (No. GJJ2200319), the Scientific Research Project of Hunan Provincial Education Department, China (No. 22B0725, No. 23B0670 and No. 23C0234), and the Research Initiation Project of Hengyang Normal University, China (No. 2022QD01).
The authors declare there is no conflict of interest.
[1] |
Abbott A (2006) Reconceptualizing knowledge accumulation in sociology. Am Soc 37: 57–66. https://doi.org/10.1007/s12108-006-1005-9 doi: 10.1007/s12108-006-1005-9
![]() |
[2] |
Abbott A (2018) Varieties of normative inquiry: Moral alternatives to politicization in sociology. Am Soc 49: 158–180. https://doi.org/10.1007/s12108-017-9367-8 doi: 10.1007/s12108-017-9367-8
![]() |
[3] |
A'Hearn B, Baten J, Crayen D (2009) Quantifying quantitative literacy: Age heaping and the history of human capital. J Eco Hist 69: 783–808. https://doi.org/10.1017/S0022050709001120 doi: 10.1017/S0022050709001120
![]() |
[4] |
A'Hearn, B, Delfino A, Nuvolari A (2021) Rethinking age heaping: a cautionary tale from nineteenth-century Italy. Eco Hist Rev 75: 111–137. https://doi.org/10.1111/ehr.13087 doi: 10.1111/ehr.13087
![]() |
[5] | Bainame K, Letamo G (2015) Evaluation of Data Quality of the Botswana 2011 Population and Housing Census. Botswana Notes and Records 46. |
[6] |
Bairagi R, Aziz KMA, Chowdhury MK, et al. (1982) Age misstatement for young children in rural Bangladesh. Demography 19: 447–458. https://doi.org/10.2307/2061012 doi: 10.2307/2061012
![]() |
[7] | Bucur B (2016) Population Health in Interwar Romania Reflected in the Sociological School of Bucharest's Research and Publications, In: Marinescu, V., Mitu, B., Valentina Marinescu and Bianca Mitu Health and the Media: Essays on the Effects of Mass Communication, Jefferson [NC, USA]: McFarland & Co, 215–240. |
[8] |
Byerle D, Terera G (1981) Factors affecting reliability in age estimation in rural West Africa: A statistical analysis. Popul Stud 35: 455–465. https://doi.org/10.1080/00324728.1981.11878517 doi: 10.1080/00324728.1981.11878517
![]() |
[9] |
Caldwell JC (1966) Study of age misstatement among young children in Ghana. Demography 3: 477–490. https://doi.org/10.2307/2060173 doi: 10.2307/2060173
![]() |
[10] |
Caldwell JC, Igun AA (1971) An experiment with census-type age enumeration in Nigeria. Popul Stud 25: 287–302. https://doi.org/10.1080/00324728.1971.10405804 doi: 10.1080/00324728.1971.10405804
![]() |
[11] |
Coale AJ, Kisker EE (1986) Mortality crossovers: Reality or bad data? Popu stud 40: 389–401. https://doi.org/10.1080/0032472031000142316 doi: 10.1080/0032472031000142316
![]() |
[12] |
Coale AJ, Li S (1991) The effect of age misreporting in China on the calculation of mortality rates at very high ages. Demography 28: 293–301. https://doi.org/10.2307/2061281 doi: 10.2307/2061281
![]() |
[13] |
Chemhaka GB, Odimegwu C, Zwane EN (2016) Is Swaziland census data suitable for fertility measurement? Genus 72. https://doi.org/10.1186/s41118-016-0010-2 doi: 10.1186/s41118-016-0010-2
![]() |
[14] | Dechter AR, Preston SH (1991) Age misreporting and its effects on adult mortality estimates in Latin America. Popul Bull UN, 1–16. |
[15] | Fajardo-González J, Attanasio L, Trang Ha J (2014) An Assessment of the Age Reporting in the IPUMS-I Microdata. Available from: https://paa2014.populationassociation.org/papers/140099. |
[16] | Golopentia A, Grigorescu DC (1948) Populatia Republicii Populare Romane la 25 Ianuarie 1948. Rezultatele provizorii ale recensamantului. Extras din Probleme economice, nr 2. Institutul National de Statistica. |
[17] | Jdanov DA, Jasilionis D, Soroko EL, et al. (2008) Beyond the Kannisto-Thatcher database on old age mortality: An assessment of data quality at advanced ages. Available from: https://www.demogr.mpg.de/papers/working/wp-2008-013.pdf. |
[18] | Kannisto V (1999) Assessing the Information on Age at Death of Old Persons in National Vital Statistics, In: Validation of Exceptional Longevity, Odense: Odense University Press, 6: 240–249. |
[19] |
Lee MM, Zhang N (2017) Legibility and the informational foundations of state capacity. J Polit 79: 118–32. https://doi.org/10.1086/688053 doi: 10.1086/688053
![]() |
[20] | Mihaescu C, Dumitrescu I, Mirica A (2018) Romania: un secol de istorie. Date statistice. Andrei Tudorel (coord). Bucuresti: Editura Institutului National de Statistica, 2018. Populatia, 12–48. |
[21] |
Mokyr J, Gráda CÓ (1982) Emigration and poverty in prefamine Ireland. Explor Econ Hist 19: 360–384. https://doi.org/10.1016/0014-4983(82)90008-0 doi: 10.1016/0014-4983(82)90008-0
![]() |
[22] | Penina O, Jdanov D, Grigoriev P (2015) Producing reliable mortality estimates in the context of distorted population statistics: the case of Moldova. Available from: https://www.demogr.mpg.de/papers/working/wp-2015-011.pdf. |
[23] | Pisica S, Murgescu B, Sora FA, et al. (2018) Romania: un secol de istorie. Date statistice. Andrei Tudorel (coord). Bucuresti: Editura Institutului National de Statistica, 2018. Forta de munca, 49–65. |
[24] |
Preston SH, Elo IT, PRESTON SH (1999) Effects of age misreporting on mortality estimates at older ages. Popul Stud 53: 165–177. https://doi.org/10.1080/00324720308075 doi: 10.1080/00324720308075
![]() |
[25] |
Pripoaie R, Cretu CM, Turtureanu AG, et al. (2022) A Statistical Analysis of the Migration Process: A Case Study—Romania. Sustainability 14. https://doi.org/10.3390/su14052784 doi: 10.3390/su14052784
![]() |
[26] | Sandu D (2018) Migratia temporara in strainatate (1990–2016), In: Ghețău, V., Demografia Romaniei, Editura Academiei Române, 245–278. |
[27] | Shryock HS, Siegel JS (1975) The methods and materials of demography. |
[28] |
Simandan D (2019) Revisiting positionality and the thesis of situated knowledge. Dialogues Hum Geogr 9: 129–149. https://doi.org/10.1177/20438206198500 doi: 10.1177/20438206198500
![]() |
[29] |
Simandan D (2010) Roads to perdition in the knowledge economy. Environ Plan 42: 1519–1520. https://doi.org/10.1068/a4324 doi: 10.1068/a4324
![]() |
[30] | Yi Z, Gu D (2008) Reliability of age reporting among the Chinese oldest-old in the CLHLS datasets. In: Healthy longevity in China, 61–78, Springer, Dordrecht. |
[31] | United Nations (1955) Manual Ⅱ: Methods of appraisal of quality of basic data for population estimates (United Nations Publications, Sales No. 56.ⅩⅢ.2). Chapter 3: The Accuracy of Age and Sex Statistics. |
[32] | United Nations, Department of Economic and Social Affairs Population Division. World Prospects 2022. Romania Demographic Profiles, Line Charts, 2022. Available from: https://population.un.org/wpp/Graphs/DemographicProfiles/Line/642. |
![]() |
![]() |