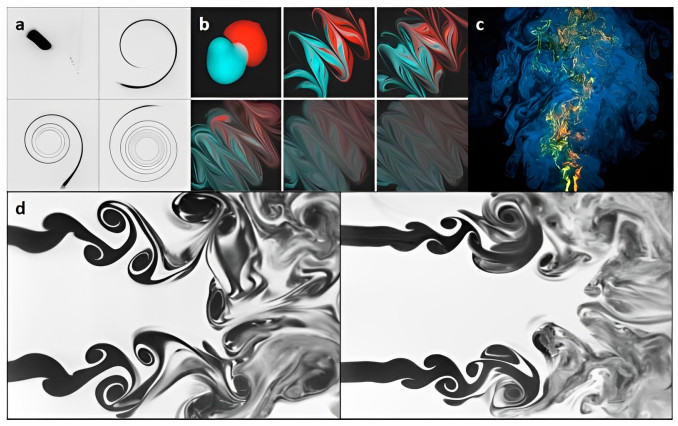
Mixing is the basis of stable and efficient combustion in air-breathing power systems, and it is also an important problem in fluid mechanics, which has been extensively studied from various perspectives. The purpose of this review is to investigate mixing mechanisms based on two commonly-used mixing indicators, namely ci (c refers to concentration, and i is either 1 or 2, indicating first- or second-order statistics), with a focus on passive-scalar (PS) and variable-density (VD) mixing. For PS mixing, the flow is not influenced by the mixing process. By using first-order statistics with concentration as the core, the PS mixing mechanisms on lamella structures can be described as stretching enhancing diffusion and promoting mixing. On the other hand, second-order statistics represented by the scalar dissipation rate can investigate mixing mechanisms on specific type of flow structures described by the invariants of velocity gradient tensors and the rotation of principal strain axis. As such, it has been found that strain-dominated flow structures can promote mixing, while rotation-dominated flow structures hinder it. For VD mixing, it has two distinct characteristics: flow changes due to baroclinic vorticity, and the inherent velocity divergence alters the mixing indicators. Studies using first-order statistics center on the mixing time in different types of VD flows, leading to the discovery of new phenomena. For instance, the second baroclinic vorticity can promote stretching in shock bubble interactions. Studies on second-order statistics for VD mixing have defined several mixing indicators from the component-transport equation, which have been utilized in phenomenological studies on VD mixing. This review aims to provide an overview of mixing phenomena, mixing indicators, and mixing mechanisms, and proposes research directions for understanding the mixing characteristics, flow structures, and their relationship with specific combustion phenomena particularly by second-order statistics.
Citation: Xu Han, Bin Yu, Hong Liu. Mixing mechanisms in the view of mixing indicators: from passive-scalar mixing to variable-density mixing[J]. Metascience in Aerospace, 2024, 1(1): 1-37. doi: 10.3934/mina.2024001
[1] | James H. Page, Lorenzo Dorbolò, Marco Pretto, Alessandro Zanon, Pietro Giannattasio, Michele De Gennaro . Sensitivity analysis of mixed analysis-synthesis flight profile reconstruction. Metascience in Aerospace, 2024, 1(4): 401-415. doi: 10.3934/mina.2024019 |
[2] | Sarker Ashraful Islam, Farhana Kabir Esheta, Md Mahir Shahriar, Dewan Hasan Ahmed . Numerical study of aerodynamic drag reduction of a circular cylinder with an inbuilt nozzle. Metascience in Aerospace, 2024, 1(4): 379-400. doi: 10.3934/mina.2024018 |
[3] | Pasynok Sergey . Cumulative STF coefficients evaluation and validation. Metascience in Aerospace, 2024, 1(4): 371-378. doi: 10.3934/mina.2024017 |
[4] | Hussein Kokash, G. Gilou Agbaglah . A two- to three-dimensional wake transition mechanism induced by the angle of attack of a NACA0012 airfoil. Metascience in Aerospace, 2024, 1(3): 329-345. doi: 10.3934/mina.2024015 |
[5] | Shorob Alam Bhuiyan, Ikram Hossain, Redwan Hossain, Md. Sakib Ibn Mobarak Abir, Dewan Hasan Ahmed . Effect of a bioinspired upstream extended surface profile on flow characteristics and a drag coefficient of a circular cylinder. Metascience in Aerospace, 2024, 1(2): 130-158. doi: 10.3934/mina.2024006 |
[6] | Hui Ying WANG, Némo DECAMPS . Effects of oxygen concentration of oxidizer flow on laminar diffusion flame established over liquid fuel beds at microgravity. Metascience in Aerospace, 2024, 1(2): 159-184. doi: 10.3934/mina.2024007 |
[7] | Weizhen Tang, Jie Dai, Zhousheng Huang . Comprehensive assessment of air pollutant and noise emissions at airports across different altitudes. Metascience in Aerospace, 2024, 1(3): 292-308. doi: 10.3934/mina.2024013 |
[8] | Jiaqing Kou, Tianbai Xiao . Artificial intelligence and machine learning in aerodynamics. Metascience in Aerospace, 2024, 1(2): 190-218. doi: 10.3934/mina.2024009 |
[9] | Victor Cánepa, Pablo Servidia . Design and evaluation of INS/GNSS loose and tight coupling applied to launch vehicle integrated navigation. Metascience in Aerospace, 2024, 1(1): 66-109. doi: 10.3934/mina.2024004 |
[10] | Grégoire Dannet, Olivier Boucher, Nicolas Bellouin . Features and evolution of civil aviation CO$ _2 $ emissions based on ADS-B data for the period between 2019–2024. Metascience in Aerospace, 2024, 1(4): 346-370. doi: 10.3934/mina.2024016 |
Mixing is the basis of stable and efficient combustion in air-breathing power systems, and it is also an important problem in fluid mechanics, which has been extensively studied from various perspectives. The purpose of this review is to investigate mixing mechanisms based on two commonly-used mixing indicators, namely ci (c refers to concentration, and i is either 1 or 2, indicating first- or second-order statistics), with a focus on passive-scalar (PS) and variable-density (VD) mixing. For PS mixing, the flow is not influenced by the mixing process. By using first-order statistics with concentration as the core, the PS mixing mechanisms on lamella structures can be described as stretching enhancing diffusion and promoting mixing. On the other hand, second-order statistics represented by the scalar dissipation rate can investigate mixing mechanisms on specific type of flow structures described by the invariants of velocity gradient tensors and the rotation of principal strain axis. As such, it has been found that strain-dominated flow structures can promote mixing, while rotation-dominated flow structures hinder it. For VD mixing, it has two distinct characteristics: flow changes due to baroclinic vorticity, and the inherent velocity divergence alters the mixing indicators. Studies using first-order statistics center on the mixing time in different types of VD flows, leading to the discovery of new phenomena. For instance, the second baroclinic vorticity can promote stretching in shock bubble interactions. Studies on second-order statistics for VD mixing have defined several mixing indicators from the component-transport equation, which have been utilized in phenomenological studies on VD mixing. This review aims to provide an overview of mixing phenomena, mixing indicators, and mixing mechanisms, and proposes research directions for understanding the mixing characteristics, flow structures, and their relationship with specific combustion phenomena particularly by second-order statistics.
concentration of the passive scalar | |
diffusion coefficient | |
mixing time of PS mixing | |
mixing time of VD mixing | |
circulation of the vortex | |
highest concentration on lamella structure in first-order statistics theory | |
Batchelor scale of PS mixing in first-order statistics theory | |
mixedness proposed by Cetegen [1] | |
mixedness proposed by Verzicco [2] | |
scalar gradient | |
scalar dissipation rate | |
strain rate tensor | |
element line vector | |
vorticity vector | |
the ith principal strain | |
principal strain axis of the ith principal strain | |
the cosine of the angle between the scalar gradient |
|
modulus of the principal strain in two-dimensional flow | |
stretch rate of the element line | |
angular velocity | |
rotation of strain axis | |
effective rotation vector | |
the first dimensionless number of the two-dimensional alignment theory | |
the second dimensionless number of the two-dimensional alignment theory | |
the first invariant of velocity gradient tensor | |
the second invariant of velocity gradient tensor | |
the third invariant of velocity gradient tensor | |
mass fraction of ith component | |
Atwood number | |
mixing enhancement number | |
mixed mass | |
normalized mixed mass | |
mixing length | |
molecular mixing fraction | |
new scalar dissipation rate for mixedness in VD mixing |
Efficient mixing is essential for maintaining stable and efficient combustion in air-breathing power systems. The characteristics of mixing are critical determinants of key combustion factors, such as combustion efficiency, flame extinction, and pollution emissions. Despite significant advances in ramjet and gas turbine engines, achieving mixing enhancement remains a significant challenge in these two advanced representative engines.
In the case of a ramjet engine, the environment is characterized by a high-speed incoming flow (Ma=2∼6+), and achieving stable and efficient combustion in this setting is a critical challenge [3]. While the feasibility of supersonic combustion was theoretically analyzed in 1964 [4], Ferri further noted in 1973 that efficient supersonic combustion is mixing-controlled [5]. However, a unified design methodology for organizing the flow in the ramjet combustion chamber to achieve efficient mixing has not been established [3].
The primary focus with turbo-based engines is to reduce fuel consumption and carbon emissions. Over nearly half a century of exploration, the design of turbo-based combustion chambers has been based on swirling flow [6]. The approach is characterized by using the recirculation region to stabilize the flame and enhance mixing [7]. To achieve significant thrust, a high temperature rise is required, which necessitates a high fuel-air ratio in the combustion chamber. Consequently, additional intake air should be introduced into the primary zone, as discussed by Bahr [8]. In that case, the combustion chamber utilizes a strong swirl flow to mix these additional fuel and intake air, that may result in flame extinction caused by excessive mixing in some lean fuel conditions [9].
The design of these two advanced power systems demonstrates that while ramjet combustion is mixing-controlled, there is currently no established methodology for organizing the flow inside the combustion chamber to enhance mixing. Although the turbo-based engine primarily relies on the use of swirling flow, problems such as flame extinction resulting from excessive mixing still persist. The practical engineering design problems in these two systems can be largely attributed to the need for a better understanding of the mixing mechanisms and enhancement methods in the combustion chamber.
From a more fundamental perspective, mixing is a general and fascinating flow behavior that appears in numerous fields, including ocean flow [10], biological reproduction [11], virus diffusion [12], and natural/forced heat convection [13]. Accordingly, various schools of mixing theory have emerged. An important branch of mixing mechanism can be traced back to 1958 when based on the Kolmogorov turbulent cascade theory [14], Batchelor [15] proposed the existence of a diffusion scale smaller than the Kolmogorov scale at low diffusion coefficients. This type of mixing is called passive-scalar (PS) mixing as it is passively affected by the flow [16]. This theory paved the way for large eddy simulation (LES) and was later verified by subsequent direct numerical simulation (DNS) results [17]. It is commonly accepted that turbulent flow promotes mixing. Therefore, a criterion to predict the transition of turbulent mixing is crucial. It was not until 2000 that Dimotakis proposed a scale separation physical concept [18] and put forward the criteria for the onset of turbulent mixing. In recent years, Villermaux has provided theoretical solutions to the advection-diffusion equation based on simple flows such as single vortex flow [19] and shear flow [20]. Moreover, Villermaux also pointed out that stretching on moving lamellas enhances diffusion, confirming that turbulent flow may exponentially increase the mixing indicator. However, some researchers recently suggested that turbulence may not be the optimal mixing organizer at low diffusion coefficients, i.e., large Péclet numbers (Pe) [21]. In some specific cases, laminar mixing may actually be more efficient than turbulent mixing [22]. Overall, the complexity of mixing mechanisms results from the diversity and intricacy of flows, the analytical difficulty of solving equations, and the disagreement among views. Mixing can only be analytically described in simple flows and is still far from the controllable stage.
Regarding the internal flow of engine combustor, a significant density change occurs. In 2005, Dimotakis categorized mixing into three levels [23]. The first level is passive-scalar (PS) mixing, where the mixing process does not affect the flow. The second level is variable-density (VD) mixing, characterized by mixing in fluid with varying densities. The misalignment of the density gradient and the pressure gradient generates baroclinic vorticity, altering the local flow field. The third level is combustion mixing, characterized by the flame changing quantities such as temperature, density, pressure, viscous coefficient, etc. Although the second and third levels of mixing are the main types that occur in actual combustion chambers, Dimotakis [23] concluded in the Annu. Rev. Fluid Mech. that, "Unfortunately, however, there is no (even empirical) theory to describe the variable density mixing problem." The lack of connection between basic theory and practical problems underlies the urgent need for more theoretical guidance in the design of mixing enhancement devices, which is still mainly based on experience.
This review is centered around exposing the mixing mechanisms in PS mixing and VD mixing from the perspective of mixing indicators. The fundamental step in mixing research is defining objective mixing indicators. The two commonly utilized indicators can be represented by ci, where c represents concentration, and i=1 denotes first-order statistics, while i=2 stands for second-order statistics [24]. The following sections are organized as follows. Section 2 focuses on PS mixing by introducing the first-order and second-order statistics used to describe the mixing phenomena and the corresponding mixing mechanisms discovered through related mixing indicators. Section 3 is devoted to VD mixing, discussing new mixing indicators and phenomena and reviewing the unique characteristics of VD mixing compared with PS mixing. Finally, Section 4concludes this review and points out future research directions.
PS mixing, according to the classification proposed by Dimotakis [23], is the first-level mixing characterized by the mixing process not affecting the flow. Research on PS mixing mainly focuses on low-velocity flows, thus, PS mixing can be described by the advection-diffusion equation:
∂c∂t+uj∂c∂xj=D∂2c∂x2j, | (2.1) |
where D is the diffusion coefficient. There is also a restriction condition that the velocity divergence is 0, given by
∂uj∂xj=0. | (2.2) |
Therefore, how does PS mixing occur, and what is the mechanism behind it? To answer this, we can illustrate the mechanism by examining the mixing process in particular flows, as shown in Figure 1.
The mixing processes depicted in Figure 1 are single-vortex mixing [19], random flow mixing [25], jet mixing [26], and coaxial jet mixing [27], respectively. These mixing processes demonstrate that the initially clustered high-concentration region is stretched into various structures, including lamellas and bubbles. During the stretching and elongation of the lamellas, the scalar concentration on these structures decreases until it is conserved with the surrounding fluid. On the bubble structures, the concentration remains virtually unchanged. These examples underscore that the mixing process can be influenced by stretching and that the mixing dynamics are distinct on different kinds of flow structures. Describing these phenomena has always been the primary focus of mixing research. As mentioned earlier, mixing indicators can be categorized into two groups: first-order statistics and second-order statistics. From the following review, one can find that the first-order statistics describe the mixing mechanisms on the lamella structures, and the second-order statistics describe the mixing mechanisms on the flow structures, which is described by the invariants of velocity gradient tensors and the rotation of principal strain axis.
The first type of mixing indicators is the first-order statistics, which typically refers to the scalar concentration c. This theory focuses on the concentration distribution p(c) on the lamella structures and aims to establish the relationship between the evolution of the concentration and lamella stretching. Concentration is a vital physical quantity in various mixing scenarios. For instance, factories need to monitor whether the concentration of pollutants in wastewater exceeds the standard level. In 1989, Vidick observed that the residue of additives in glass or cement could lead to mechanical defects [28]. Poulain discovered that the lifespan of the liquid film, such as bubbles in seawater, is determined by the concentration of impurities [29]. These examples underline the importance of describing the distribution of concentration c over time and space and its probability density distribution p(c).
The theory of first-order statistics was firstly introduced by Marble in 1985 to explore the relationship between product generation and circulation in the single vortex flame [30]. Since Marble's proposal, using this theory to analyze PS mixing has drawn significant attention, and Villermaux further developed this approach, which has now become a popular research paradigm. Villermaux explains this method in detail in his publication [31], and only a brief outline of the framework will be presented here.
In this theoretical framework, a Lagrangian coordinate system is established on a two-dimensional moving lamella, as shown in Figure 2. The x axis points in the direction of the largest scalar gradient, while the y axis is perpendicular to it. The width of the lamella is denoted by s(t), and the length of the lamella is denoted by l(t). The velocity in the x direction is denoted by u1, and the velocity in the y direction is denoted by u2. Given that ∂uj∂xj=0, the velocity (u1,u2) can be associated with the stretching of this lamella using
{u1=(˙s/s)x,u2=−(˙s/s)y, | (2.3) |
where ˙s represents the time derivative of the width s(t).
The advection-diffusion equation on the lamella is given by ∂c∂t+(˙s/s)x∂c∂x=D∂2c∂x2, where a Ranz transform [32,33] is applied to convert it into the Fourier equation for analytical solutions, given as ξ=xs(t),τ=D∫t0dt′s(t′)2. Thus, we have:
∂c∂τ=D∂2c∂ξ2. | (2.4) |
Eq 2.4 describes the corresponding relationship between the concentration c, spatial position ξ, and stretch time τ to obtain the probability density function p(c). The solution of Eq 2.4 is
c(ξ,τ)=12[erf(ξ+1/22√τ)−erf(ξ−1/22√τ)]. | (2.5) |
When considering the interaction between two lamellas, the linearity of the Fourier equation allows for direct addition of the concentration, giving:
c(ξ,τ)=c1(ξ,τ)+c2(ξ,τ). | (2.6) |
As the result the direct addition of the concentration in Eq 2.6, the probability density function can be calculated using convolution as
p(c)=∫c=c1+c2p1(c1)p2(c2)dc1=p1⊗p2. | (2.7) |
This method is described in-depth in the literature [34,35].
In this theoretical framework, two important mixing indicators can be defined: mixing time and Batchelor scale. From the solution of Fourier equation Eq 2.4 as described in Eq 2.5, x=0 is the position with the highest concentration, which can be expressed as
cmax=erf(14√τ). | (2.8) |
When τ≪1, cmax(τ) approaches 1, whereas when τ≫1, cmax(τ)=1τ decreases with time. The distribution of concentration on the lamella is Gaussian [31]:
c(x,t)∼12√πte−x22η2, | (2.9) |
where the spatial scale is given by:
η(t)=s(t)√τ(t). | (2.10) |
If the lamella is stretched exponentially, s(t)=s0e−γt, then η(t) is constant, indicating that the concentration distribution is no longer affected by the flow. Therefore, the time ts satisfying the condition τ(ts)∼O(1) is defined as the mixing time. For instance, the mixing time of PS mixing in a single vortex can be expressed as:
tPSm=R20Γ(3π216)1/3(s0R0)2/3(ΓD)1/3, | (2.11) |
where Γ is the circulation of the vortex, D is the diffusion coefficient, and R0 and s0 are the shape factors [19]. The mixing time signifies that after time ts, mixing transitions from the stretch-dominant stage to the diffusion-dominant stage. The scale η(t) corresponds to the Batchelor scale, which has a similar physical meaning as proposed in Batchelor's turbulent mixing theory [15].
Villermaux et al. have applied this theoretical framework to study three types of problems:
The first type of research involves establishing stretching models in different flows and examining the corresponding concentration c. For example, in 2003, Meunier et al. studied the concentration distribution and mixing time in a single vortex flow [19]. Villermaux et al. investigated mixing in jet flow and obtained its p(c) function [34]. Duplat et al. examined the mixing behavior in random and turbulent flows. Duplat concluded that the concentration follows a gamma distribution in random flows [25,35], and highlighted the self-convolution behavior resulting from the interaction between lamella [36].
The second type of research aims to investigate more accurate ways to describe the stretching indicator. In 2019, Gotzfried et al. examined whether the Finite-time Lyapunov exponent (FTLE) proposed by Haller [37] could be used as the stretching indicator for the concentration distribution of turbulence. FTLE has been used in other areas such as vortex [38], mixing layer [39], and vortex interaction [40]. The concentration distribution predicted by using FTLE as the stretching indicator was consistent with the results of DNS [41]. In 2022, Meunier attempted to describe the stretching behavior of "diffuselet" accurately in three-dimensional flows by using the velocity gradient tensors or the FTLE number [42].
The third type of research is focused on predicting scalar distribution using stretching information of the flow without using computational fluid dynamics (CFD) methods. In 2010, Meunier and Villermaux proposed the Diffusive Strip Method (DSM), which accurately predicted the concentration distribution of a single vortex system or sine flow [43]. In 2018, Martinez-Ruiz et al. extended this method from two to three dimensions, resulting in the three-dimensional Diffusive Sheet Method, which accurately predicted scalar distribution in Taylor-Couette flow [44]. Recently, Sen et al. used DSM to analyze the dynamics of solute mixing and reaction in a mixing-limited reactive flow in both shear flow and Rankine vortex cases [45].
To summarize, the first-order statistics theory provides an analytical solution to the Fourier equation using the Ranz transform, which accurately describes the relationship between scalar distribution and lamella stretching on high Péclet number lamellas. This theory effectively describes the PS mixing mechanisms on lamella structures. Recent research on first-order statistics has focused on two frontiers for studying mixing: 1) This theoretical framework is extended to reactive flows, such as mixing-limited reactive flow in linear shear and Rankline vortex cases [45], porous media flow [46], and reactive flow with different Damköhler numbers [47,48]. 2) The stretching indicator was given by modeling in the research before. Recently, discussions have focused on developing a more fundamental stretching indicator using basic physical quantities in the flow field such as velocity and pressure [42].
Second-order statistics in mixing research typically include mixedness f and scalar dissipation rate χ. Mixedness is a measure of the degree of mixing and is expressed as a second moment of concentration. Depending on the specific research problem, different forms of mixedness may be used. For example, Cetegen proposed the mixedness given by Eq 2.12 in 1993 to study laminar flow single-vortex mixing [1].
f=4c(1−c) | (2.12) |
This definition assumes f=1 at c=0.5. Another commonly used form of mixedness is fs in Eq 2.13,
fs={1A∫Accs(2−ccs)dAforc≤cs1A∫A−c2+2ccs+1−2cs(1−cs)2dA forc≥cs | (2.13) |
which was introduced by Verzicco in 1995 for combustion problems [2]. fs assumes fs=1 when the concentration c is equal to the stoichiometry concentration cs.
Scalar dissipation rate χ is defined as the dot product of the scalar gradient ∇c [49]
χ=∇c⋅∇c. | (2.14) |
If mixedness is defined as Eq 2.12, the relationship between the mixedness and scalar dissipation is
(∂∂t+uj∂∂xj−D∇2)f=−8Dχ. | (2.15) |
Eq 2.15 shows that the scalar dissipation rate is related to the rate of change of mixedness with respect to time, so this quantity is an indicator of the mixing rate.
Mixedness and scalar dissipation rate have significant importance in the field of mixing research.
1) These two quantities objectively evaluate the degree of mixing and mixing rate. By using mixedness and scalar dissipation rate, researchers have discovered new mixing phenomena. For instance, Cetegen found a positive linear correlation between mixedness and time and vortex intensity for single vortex and vortex pairs [1,50]. Basu further developed an empirical relationship between mixedness and temperature, circulation, and interaction time [51].
2) The scalar dissipation rate provides a crucial link between flow and mixing. Researchers have utilized the scalar dissipation rate to gain insights into the relationship between flow and mixing. One representative study is that Flohr [52] investigated mixing cascade affect by a given single vortex by analyzing the evolution of scalar dissipation rate in the spectral space, and this work demonstrated the usefulness of scalar dissipation rate in understanding the relationship between flow and mixing. In this section, the studies on this relationship will be reviewed in detail.
3) Scalar dissipation rate is a fundamental physical quantity in combustion theory that is highly relevant to various combustion factors. In non-premixed combustion, the scalar dissipation rate positively correlates with combustion efficiency [53,54]. In premixed combustion, the scalar dissipation rate affects flame speed and thickness [55,56]. Consequently, the scalar dissipation rate has been the subject of much research in the field of combustion, with numerous studies examining this quantity in the context of practical combustion problems [57,58,59,60,61,62].
In this section, we will review studies that have explored the dynamic properties of scalar dissipation rate and how this physical quantity can be used to establish the relationship between the flow structure and mixing.
As shown in Figure 3, the starting point of relating flow and mixing is the scalar dissipation rate equation. Buch et al. [49] derived the transport equation for scalar dissipation rate for the first time
(∂∂t+uj∂∂xj−D∇2)12(∇c⋅∇c)=−(∇c⋅S⋅∇c)−D∇(∇c):∇(∇c), | (2.16) |
where S is the strain rate tensor:
Sij=∂ui∂xj+∂uj∂xi. | (2.17) |
In Eq 2.16, the first term on the right side is called the "stretching term". This term describes how the fluid stretches the scalar gradient, thus increasing or decreasing it. In turbulent mixing and combustion research, the stretching term is often called "turbulence-scalar interaction" [68,69], which is the core of studying the kinematic properties of the scalar dissipation rate equation. The second term on the right is always negative, indicating that the scalar dissipation rate decreases due to the effects of diffusion.
The stretching term in the scalar dissipation rate equation, (∇c⋅S⋅∇c), bears a resemblance to the stretching term of the element line stretching equation in incompressible, inviscid flow [65], given by
(∂∂t+uj∂∂xj)12(l(t)⋅l(t))=l(t)⋅S⋅l(t), | (2.18) |
where l(t) is the element line vector. When this element line is stretched, its width decreases, leading to an increase in the scalar gradient on this element line. The similarity between the stretching term in the scalar dissipation rate equation and the element line stretching equation is thus evident. The stretching term also occurs in the enstrophy equation [70], given by
(∂∂t+uj∂∂xj)12(ω⋅ω)=ω⋅S⋅ω+νω⋅∇2ω, | (2.19) |
where ω represents the vorticity vector of the flow.
By comparing the equations for scalar dissipation rate (Eq 2.16), element line (Eq 2.18), and enstrophy (Eq 2.19), it can be observed that the stretching terms of these equations have a similar mathematical form, except for the opposite sign in the scalar dissipation rate equation. This suggests that stretching is an essential fluid characteristic which occurs not only in the evolution scalar dissipation rate but also in the element line stretching and vortex stretching phenomena. Thus, studying the mechanisms of element line and vortex stretch can contribute to a deeper understanding of the scalar dissipation rate.
The stretching term ∇c⋅S⋅∇c can be expressed in tensor form as ∇ciSij∇cj. This term can also be written in vector form as ∇cTS∇c, where ∇c=[∇c1,∇c2,∇c3]T and S=[Sij]. Since S is a real symmetric matrix, one can perform an orthogonal decomposition such that S=XTDX, where D=diag{si} is a diagonal matrix, X=[ξ1,ξ2,ξ3]T is an orthonormal basis, and ξi indicates the direction of the principal strain axis. Here, si represents the principal strain. Without loss of generality, one can define s1>s2>s3, where s1,s3 are principal strains in the stretching and compression directions, respectively. If ∇⋅U=0, then s1+s2+s3=0. Expressing ∇cTS∇c using the orthogonal decomposition yields,
∇cTS∇c=(X∇c)TDX∇c=|∇c|2∑isiλi2. | (2.20) |
Here λi=∇c|∇c|⋅ξi represents the cosine of the angle between the scalar gradient ∇c and the principal strain axis ξi. The principal strains si and the angle λi determine the stretching term.
Early research on the stretching mechanism focused mainly on the stretching of the element line. However, due to limitations in experimental and numerical methods, the angle λi could not be directly measured, and thus, the first-stage research on the stretching mechanism was based primarily on hypotheses, as shown in Figure 3. One such hypothesis was proposed by Batchelor and Townsend. They suggested that the element line vector l(t) was aligned with the principal strain in the stretching direction, denoted by ξ1, with λ1=1 and λ2=λ3=0.
Townsend first proposed the hypothesis that the element line vector was aligned with the principal strain in the stretching direction in his study of the cooling of heat spots in grid turbulence in 1951 [71]. In 1952, Batchelor studied the element line stretching in isotropic turbulence and found that the element line was exponentially stretched. Assuming this hypothesis [63], Eq 2.18 can be simplified to:
(∂∂t+uj∂∂xj)12¯δL(t)2=s1¯δL(t)2. | (2.21) |
Here, ¯δL(t) represents the modulus of the element line vector l(t), then the exponential stretch rate ζline can be described by
ζline=1¯δL(t)∂¯δL(t)∂t=s1→¯δL(t)=¯δL(t0)es1t | (2.22) |
Cocke provided a strict description of the exponential stretching of the element line over a limited period of time in 1969 [72]. However, after the 1980s, with the widespread use of CFD technology in fluid mechanics research, numerical results showed that the hypothesis proposed by Batchelor and Townsend was not valid, as represented in the second stage of research shown in Figure 3. For instance, as in Figure 4, using DNS simulations, Ashurst observed that the vorticity tends to align more closely with the intermediate principal strain direction, whereas the scalar gradient direction has a high probability of aligning with other directions [73]. Vincent obtained similar conclusions when studying the angle between vorticity and principal strain in isotropic turbulence using DNS in 1991 [74]. Girimaji and Pope et al. simulated isotropic turbulence with ReT=90 to study the element line stretching, and found that the average exponential stretching rate was only one-third of the stretching rate proposed by Batchelor, as given by Eq 2.22 [64]. The contradiction between the hypothesis and these phenomena suggests that the element line vector is not consistent with the principal strain direction:
{⟨ζline⟩≠s1,⟨λ1⟩≠1. | (2.23) |
This discrepancy has prompted researchers to investigate which flow properties affect the alignment dynamics.
In 1991, Dresselhaus derived the alignment equation for three-dimensional element line stretching problems for the first time [65]. The alignment equation is given by:
ddtλ=(s1000s2000s3)λ−ξlineλ+(Ω−Ω′)×λ. | (2.24) |
ξline represents the stretching rate of the element line:
ξline=∑isiλ2i, | (2.25) |
Ω is the angular velocity:
Ωi=12εijk∂uk∂xj, | (2.26) |
where εijk is the alternating tensor, and Ω′ is the rotation of principal strain axis:
Ω′k=ϵijk⟨ξi,˙Sξj⟩si−sj, | (2.27) |
where ˙S represents the time derivative of strain rate tensor.
The first two terms of the alignment equation given by Eq 2.24 attract the element line vector to the principal strain axis at an exponential rate, while the third term rotates the element line around the "effective rotation vector" R=Ω−Ω′.
Dresselhaus emphasized that the "effective rotation" is crucial for the alignment dynamics. However, determining its direction in three-dimensional flows is challenging, adding considerable complexity to the alignment problems. Therefore, simplifying the alignment equation given by Eq 2.24 to obtain the general properties of a particular flow has attracted significant attention, and the two-dimensional stretching theory is an excellent example of simplification and represents the fourth-stage research in Figure 3. The alignment equation Eq 2.24 can be simplified because the direction of R in a 2D flow is always perpendicular to the plane.
Early studies on the two-dimensional stretching problem were represented by Okubo's study on particle aggregation [75], and preliminary conclusions were obtained in Weiss's research on the enstrophy transport represented by Eq 2.19 in two-dimensional turbulence [76]. In incompressible, two-dimensional flows, the principal strains have the relationship s1=−s3,s2=0. Under the assumption that the material derivative of the strain is negligible, i.e.,
(∂∂t+uj∂∂xj)s1≈0, | (2.28) |
they introduced the parameter θ=s21−ω2, similar to the widely used vortex identification method Q criterion [77]. Based on this parameter, they proposed the Okubo-Weiss criterion: in the region of θ>0, the fluid has hyperbolic characteristics, and the vorticity gradient increases exponentially, while in the region of θ<0, the fluid has elliptic properties, and the vorticity gradient oscillates periodically.
Following Weiss's proposal, many researchers verified the validity of the hypothesis in Eq 2.28. Representative studies include Basdevant [78] and Hua [79], who found that Weiss's hypothesis was only valid near the core of the vortex and not at the edge of the vortex. They also discovered that the strain acceleration tensor and pressure Hessian matrix play a crucial role in stretching problems. Similar phenomena were also observed in three-dimensional turbulence [80,81,82].
Subsequently, Klein et al. studied the problem of passive scalar gradient in two-dimensional turbulence and defined dimensionless numbers r [66] and n [67] that determine the two-dimensional stretching properties. They accomplished this by using the geometric model shown in Figure 5 to simplify the alignment equation given by Eq 2.24.
The stretching equation of the scalar gradient without diffusion effect, (∂∂t+uj∂∂xj)12(∇c⋅∇c)=−(∇c⋅S⋅∇c), can be transformed using the geometric model shown in Figure 5. The strain and vorticity are defined as:
{σn=∂xu1−∂yu2,σs=∂xu2+∂yu1,ω=∂xu2−∂yu1. | (2.29) |
The scalar gradient and strain are represented by their modulus and orientation:
{∇c=|∇c|(cosθ,sinθ),(σs,σn)=σ(cos2ϕ,sin2ϕ), | (2.30) |
where ρ is the modulus of the scalar gradient, θ represents the angle between the scalar gradient and the x axis, and the angle between the principal strain and x axis is −ϕ−π4.
Using this geometric model, Eq 2.24 can be simplified,
dζdτ=r−cosζ, | (2.31) |
where 12ζ+14π is the angle between the scalar gradient and the principal strain axis in compression direction.
Klein et al. defined a dimensionless number r to discuss the two-dimensional alignment equation given by Eq 2.31,
r=ω+2(dϕ/dt)σ, | (2.32) |
where the numerator part ω+2(dϕ/dt) represents the sum of vorticity and rotation of the principal strain axis, which is a simplification of the "effective rotation" R=Ω−Ω′ in the two-dimensional case. The denominator part σ is the modulus of the principal strain. Therefore, this dimensionless number measures the ratio of "effective rotation" to the principal strain. If r2<1, Eq. 2.31 has a stable point, and the flow is hyperbolic. The scalar gradient vector will converge to the stable direction. If r2>1, Eq 2.31 is unstable, and the flow is elliptical. The scalar gradient will not converge and rotate around the z axis.
The second-order time-derivative equation d2(∇c⋅∇c)/dt2=−∇c⋅N⋅∇c can also be transformed using the same geometric model. Here, N involves dS/dt and is directly relate to pressure Hessian, the specific expression of which can be found in Klein's publication [67]. Another dimensionless number n can be defined, given by
n=dσ/dtσ2, | (2.33) |
which measures the time change rate of the principal strain. This dimensionless number determines the alignment dynamics in the elliptic region. The derivative of the scalar gradient angle is the smallest when it aligns with the eigenvector N− of matrix N, which N− depends on the dimensionless number r and n. The above conclusions were confirmed by Klein's observations in two-dimensional turbulence [66,67].
Based on the discussion of the alignment dynamics of the element line (Eq. 2.31) and the scalar gradient (Eq. 2.24), one can conclude that the alignment dynamics are influenced by the flow properties, which are determined by the velocity gradient tensors that contain two parts: the strain rate tensor in Eq 2.17 and the vorticity in Eq 2.26 [84], and they are closely related to the "flow structure" [77,85]. One way to study the "flow structure" is through the application of velocity gradient tensor invariants, which define flow topologies [84,86,87]. Different flow topologies can be defined based on the eigenvalue properties of the velocity gradient tensor matrix:
λ3+Pλ2+Qλ+R=0. | (2.34) |
Flow topologies with focus, UF/C (unstable focus/compressing), SF/ST (stable focus/stretching), SF/C (stable focus/compressing), and UF/ST (unstable focus/stretching), are dominated by rotation, while flow topologies with saddle points, UN/S/S (unstable node/saddle/saddle), SN/S/S (stable node/saddle/saddle), SN/SN/SN (stable node/stable node/stable node), and UN/UN/UN (unstable node/unstable node/unstable node), are dominated by stretching/compression, as shown in Figure 6. While scalar dissipation rate has been investigated in different flow topologies in compressible turbulence [88], shock turbulence interaction [89], premixed flame [83,90] and non-premixed flame [91], the alginment dynamics cannot be determined solely by the invariants of velocity gradient tensors from Eq. 2.24. The rotation of the principal strain axis also plays a vital role in influencing the alignment dynamics of the scalar gradient, which is often neglected in flow structure research. To capture the full dynamics of the alignment process, it is necessary to consider both the velocity gradient tensor invariants and the rotation of the principal strain axis simultaneously. One of the frontiers in mixing research is to establish the complete stretching dynamic equation to explore the relationship between mixing and "flow structure" by scalar dissipation rate.
Regarding the second-order statistics, significant progress has been made in studying mixedness and scalar dissipation rate.
This section mainly reviews the studies that aim to establish a deep understanding of flow and mixing through scalar dissipation rate, by examining the dynamic properties of the stretching term in Eq 2.16. These studies mainly focus on the alignment dynamics of the scalar gradient. The research history can be divided into four stages: hypothesis [15], experimental verification [73], theoretical derivation [65], and equation simplification [66]. It has been shown that scalar dissipation rate exhibits different dynamical properties in flows with different flow characteristics. To gain a more thorough understanding of the relationship between "flow structure" and mixing by scalar dissipation rate is a crucial frontier in mixing research.
The PS mixing phenomena are described by two types of mixing indicators: first-order statistics and second-order statistics. These indicators reveal PS mixing mechanisms from different levels.
First-order statistics describe the PS mixing mechanism on the lamella structures. This theory solves the Fourier equation to obtain concentration distribution p(c), and then defines two significant mixing characteristic parameters: the Batchelor scale and mixing time [25,30,31,34,35,36]. At present, the first-order statistics theory has two priorities: 1) applying this theory to combustion flows [45,47,48,92]; 2) defining an objective stretch indicator by basic flow indicators such as velocity and pressure [41,42].
Second-order statistics theory describes the PS mixing mechanism on the general flow structures. This theory focuses on the stretching term of the scalar dissipation rate equation [49]. The angle between the scalar gradient and the principal strain axis determines this term. By discussing the alignment dynamics [65,66,67], it is observed that the stretching mechanism, i.e. the mixing mechanism is different on the flow with different flow characteristics, as defined by velocity gradient tensors [83,88,89,90,91]. At present, one of the frontiers of second-order statistics theory research is to explain the relationship between "flow structure" and mixing thoroughly by scalar dissipation rate.
Theories of single vortex PS mixing have guided the design of mixing enhancement devices such as lobe mixers [93] or strut mixers [94,95] that generate interacting streamwise vortex to enhance mixing [96]. However, as mentioned before, the flow density in the engine combustion chamber is always non-uniform. Therefore, it is necessary to understand the role of density gradient [97] and shock compression [98] in compressible mixing enhancement. The main focus of research on VD flows is on Richtmyer-Meshkov instability (RMI), Rayleigh-Taylor instability (RTI), VD jet/shear layer, and VD isotropic turbulence. As a classical VD flow, RMI/RTI has been reviewed before [99,100,101]. This section focuses on the characteristics of VD mixing compared to PS mixing. According to the mixing classification proposed by Dimotakis [23], VD mixing is the second-level mixing, which should be described using the component-transport equation:
∂(ρYi)∂t+∂(ρYiuj)∂xj=∂∂xj(ρD∂Yi∂xj) | (3.1) |
where Yi is the mass fraction of ith component.
Compared with PS mixing, VD mixing has two characteristics that significantly affect the mixing mechanism:
1) The misalignment between density gradient and pressure gradient can generate baroclinic vorticity 1ρ2(∇ρ×∇p)i=1ρ2(εijk∂ρ∂xj∂p∂xk). This vorticity can change the flow field [102]. As Dimotakis proved in Annu. Rev. Fluid Mech. [23]: "An important characteristic that distinguishes such flows from Level-1 mixing is the generation of baroclinic vorticity that derives from misalignments between pressure and density gradients, or, equivalently, temperature and entropy gradients in the flow."
DωiDt=ωj∂ui∂xj−ωi∂uj∂xj+1ρ2(εijk∂ρ∂xj∂p∂xk)+ν∂2∂x2jωi | (3.2) |
2) The mixing governing function changes from the advection-diffusion equation to the component-transport equation in VD mixing. This change leads to two significant features that distinguish VD mixing from PS mixing.
The first feature is the velocity divergence ∂uj∂xj is not 0 due to density. Livescu [103] provided a detailed review of the expressions of the velocity divergence in different VD flows. One of the expressions is given by Eq 3.3:
∂Uj∂xj=−∂∂xj(Dρ∂ρ∂xj). | (3.3) |
The second feature is that when the component-transport equation is written in the form of the advection-diffusion equation, a non-zero term appears on the right side of Eq 3.4, leading to the loss of hyperbolic persistence of the advection-diffusion equation [104].
(∂∂t+uj∂∂xj−D∇2)Y=−Dη′−11+(η′−1)Y∇Y⋅∇Y, | (3.4) |
where η′=ρ1/ρ2 is the density ratio. These two features brought by the change of mixing governing equation can lead to the difference of mixing indicators in VD mixing compared with PS mixing.
The VD mixing mechanism, similar to PS mixing, is influenced by fluid stretching. However, the misalignment between the density gradient and pressure gradient induces baroclinic vorticity, which causes alterations in the flow field, leading to changes in the evolution of mixing indicators. Consequently, research on VD mixing can be categorized into two parts shown in Figure 7: 1) The first part is dedicated to understanding the flow characteristics introduced by the density effect in VD mixing through linear stability theory and vortex dynamics. 2) The second part examines the evolution of VD mixing indicators, which includes first-order statistics focused on concentration, and second-order statistics focused on mixedness and scalar dissipation rate.
VD mixing, for example RMI/RTI etc., classified as a second-level mixing process, is distinguished by its ability to alter the flow field. Richtmyer initially discovered the instability of shock interface interaction through experiments conducted in 1960 [109], with Meshkov later corroborating this phenomenon through numerical simulation [110]. Subsequently, this instability was named after these two researchers. The RMI phenomenon involves the amplification of a perturbed density discontinuity under shock waves causing the interface to develop unsteadily. Two key controlling parameters in RMI are the shock Mach number Ma, and the Atwood number At=(ρ2−ρ1)/(ρ2+ρ1), which represents the density gradient at the interface.
The generation of baroclinic vorticity at the disrupted interface triggers the formation of complex structures, such as bubbles, spikes, and large-scale vortex structures. These structures then develop into more intricate turbulence. The RMI phenomenon occurs in different scenarios, including supernova explosion [111,112], inertial confinement fusion [113], detached-to-detonation transition [114], supersonic mixing [115,116] and supersonic combustion [117,118].
To study the flow characteristics of RMI, two primary methods are employed. The first method is to understand the disturbance growth law through linear stability theory [105]. The second approach is to describe the VD flow utilizing vortex dynamics and attempt to model and analyze the vortex [106,119,120].
The earliest investigations of RMI were conducted utilizing linear stability theory. Richtmyer's assumption of a discontinuous shock wave revealed that the disturbance growth rate is influenced by the shock wave and initial disturbance [110]. Mikaelian went on to propose a nonlinear perturbation growth rate [105]. More recently, Luo et al. developed a nonlinear perturbation growth theory on column shock wave and interface interaction problems [121]. Similarly, the growth of disturbance in RTI garnered significant attention as with RMI. The Cook et al. study pointed out that the growth rate of RTI disturbance is related to t2 [122,123]. Clark, in the same year, gave a comparable belief of t2 for the RTI disturbance growth rate based on self-similarity theory [124].
In many RMI, VD jet, and shear layer problems, the VD flow characteristics are also described from the perspective of vortex dynamics. RMI, especially single-mode RMI, has a clear vortex structure, with several circulation prediction models proposed for this reason [125]. In comparison to constant density flow, Zabusky et al. discovered for the first time that secondary baroclinic vortex structure is a critical flow characteristic influencing the evolution of VD flows [102]. This structure significantly affects the middle and late-period flow evolution, and the phenomenon is also present in variable-density jet and shear layers. Marugan et al. modeled the impact of secondary baroclinic vortices on circulation and vortex structure by introducing the Fr number [126]. Soteriou and Ghoniem further suggested that this structure would reduce the entrainment from heavy to light fluids [127]. This phenomenon is called asymmetric entrainment by Livescu, which means in RMI mixing layer, the light-density fluids tend to occupy a larger proportion than heavy-density fluids [103,128]. Much work has been done on the circulation and vorticity distribution in different types of RMI by Luo et al. [129,130,131,132].
In PS mixing theories, what affects the mixing indicators are the local flow characteristics. In first-order statistics theory, scalar concentration is the primary focus, and the stretching of the lamellas influences mixing. Second-order statistics theory, which emphasizes scalar dissipation rate, is affected by the velocity gradient tensors represented by vorticity and strain. Whilst current linear stability theory and vortex dynamics theory can describe global VD flow characteristics, it remains challenging to link these flow characteristics and mixing indicators. As such, it is crucial to describe VD flow characteristics from the perspective of local characteristics.
In the framework of first-order statistics mixing theory, predicting mixing time using the stretching model is the primary focus of VD flow studies. This type of research primarily concentrates on shock bubble interaction (SBI). Though SBI's configuration is straightforward, it can be seen as a single vortical mixing once the shock interaction stage concludes. Therefore, SBI mixing is a distinct and particular branch of VD mixing problems.
When not considering the diffusion process, the bubble shape determines the mixing time of SBI. In general, the bubble shape transition can be observed by the stretching rate of the bubble boundary contour, as provided by Eq 2.22 [115,133], to obtain the mixing time. The stretchomg rate is a global stretching indicator comparable to the first-order statistics theory in PS mixing. However, this method is only effective when the boundary of the mass fraction contour can be identified, ensuring the corresponding stretching rate obtained is suitable to model the mixing time [107]. It is worth noting that other studies in RMI also focus on mixing time. Vorobieff et al. [134] found changes in the mixing characteristics during shock-gas film interaction at dimensionless time u′1t/λ≈6.6 (u′1t is the gas velocity after the shock wave, λ is the perturbation wavelength of the gas film). Niederhaus et al. [135] observed the mixing process of light and heavy gases under various shock Mach numbers and discovered that dissimilar working conditions can be better normalized under dimensionless time u′1t/R. Recently, based on Richtmyer's model [109] and the numerical simulation outcomes of Lombardini, Pullin, and Meiron [136], Oggian et al. [137] observed a self-similar growth in multi-mode RMI at the characteristic time At+Δut/λmin≈250. Table 1 summarizes the mixing time in the current RMI/SBI studies.
References | Cases | Dimensionaless mixing time | Mixing status |
Marble et al. [30] | Air−He(Ma=1.1) | c0t/D(Ma2−1)<2.86 | well-mixed |
Vorobieff et al. [134] | Air−SF6(Ma=1.2) | u′1t/λ≈6.6 | well-mixed |
Niederhaus et al. [135] | Air−He(Ma=1.22−3) | u′1t/R=10 | well-mixed |
N2−Ar(Ma=1.33−3.38) | u′1t/R=15 | partially-mixed | |
Air−SF6(Ma=1.2−3) | u′1t/R=12 | well-mixed | |
Oggian et al.[137] | At = 0.5(Ma=1.84) | At+Δut/λmin≈250 | well-mixed |
Liu et al. [138] | Air−He(Ma=1.22−4) | τ(tVDs)∼O(1) | well-mixed |
The mixing time can be modeled based on the first-order statistics theory, as in PS mixing. SBI is a single-vortex mixing problem, and the stretching indicator can be obtained using the single-vortex model, after which the mixing time can be defined. Marble [30] and Meunier [34] developed the mixing time model for the single-vortex system in PS mixing given by Eq 2.11. Though the mixing characteristics, including stretching and diffusion effects, are considered, normalizing the mixing time of SBI is challenging because of the unconsidered secondary baroclinic vorticity characteristics of VD flow. In contrast to the mixing time model shown in Eq 2.11 proposed by Meunier et al. [19], Liu et al. [138] proposed a mixing time model predicting the mixing time accurately in VD SBI by considering additional stretching from second baroclinic vorticity:
tVDm=η0R20Γ(3π216)1/3(s0R0)2/3(1+32|At+|)−2/3(ΓD)1/3, | (3.5) |
where Γ is the circulation of the vortex, D is the diffusion coefficient, R0 and s0 are the shape factors, η0 is the compression rate [139], and At+ is the post shock Atwood number. Compared with Marble's mixing time model [30], Liu et al.'s model proposed in Eq 3.5 accurately predicts mixing time in a large Ma interval. Moreover, the ratio between the VD mixing time in Eq 3.5 and PS mixing time in Eq 2.11 can be defined as the mixing enhancement number:
Msbv=tVDmtPSm=(1+32|At+|)−2/3. | (3.6) |
When investigating VD mixing using second-order statistics, the governing equation of mixing changes from the advection-diffusion equation (Eq 2.1) to the component-transport equation (Eq 3.1). As a result, the form of the mixing indicators also changes.
The first kind of VD mixing indicators redefines the concept of mixedness. Zhou [108] introduced an indicator similar to mixedness used in PS mixing when studying VD mixing of RMI and RTI and named it mixed mass. The physical meaning of mixed mass is the mass of the mixture produced by the mixing process, and it is defined as:
M=∫4ρY1Y2dV | (3.7) |
Mixed mass can also be related to an indicator similar to the scalar dissipation rate used in PS mixing:
ddt∫ρY1Y2dV=2∫ρD∂Y1∂xj∂Y2∂xjdV | (3.8) |
This equation shares similarities with the mixedness equation for PS mixing 2.15. Therefore, it provides an analogy that helps explain the relationship between mixedness and scalar dissipation rate for VD mixing.
To normalized the mixed mass indicator, Zhou [108] used the following equation:
ψ=∫ρY1Y2dV∫⟨ρ⟩⟨Y1⟩⟨Y2⟩dV. | (3.9) |
The denominator represents the mixture mass generated when all the fluids in the area are mixed. Together with two other mixing indicators, mixing length and molecular mixing fraction, these three indicators were used to study the asymptotic phenomenon in RMI and RTI. The mixing length indicator is defined as:
Ξ=∫∞−∞⟨(min(X1,X2))⟩dzh | (3.10) |
And, the molecular mixing fraction is given by:
Θ=∫X1X2dV∫⟨X1⟩⟨X2⟩dV | (3.11) |
These three indicators were found to have limits in RMI and RTI and tended to be around 0.8 shown in Figure 8. This asymptotic phenomenon was also observed in the numerical simulations by Thornber et al. [140].
Tian et al. also used mixed mole fraction and mixed mass to study RMI and RTI mixing, and observed similar asymptotic phenomenon [141,142]. They established a prediction model for the asymptotic limit [143], which is given by:
Ψ(A)=1−β∫1−¯Y1(1−R)[¯Y1(1−R)+R]2(∂¯Y1∂X)2dX∫¯Y1(1−¯Y1)¯Y1(1−R)+RdX. | (3.12) |
The second type of VD mixing indicators retains the original mixedness definition but proposed a new definition of scalar dissipation rate. Yu et al. [144] studied this problem in SBI and proposed a new definition of scalar dissipation rate for VD mixing based on the mixedness definition proposed by Cetegen [1].
ddt⟨f⟩=⟨χ∗⟩ | (3.13) |
The new scalar dissipation rate for mixedness in VD mixing is denoted as χ∗ and can be expressed as
χ∗=4PeK1,f(σ,Y)∇Y⋅∇Y+4PeK2,f(σ,Y)∇2Y, | (3.14) |
along with the coefficient of density gradient accelerated dissipation term K1,f(σ,Y) and the redistributed diffusion term K2,f(σ,Y). It was found that this new scalar dissipation rate has a weak correlation with Pe and Re numbers in their research. Using this new defination of scalar dissipation rate, the optimal Atwood number interval for different SBI can be obtained as in Figure 9 [145].
Furthermore, as the scalar dissipation rate characterizes the mixing rate, the original definition of scalar dissipation rate provided by Eq 2.14 was employed to express the mixing rate in RMI/SBI experiments (Figure 10).
The stretching mechanisms involved in VD mixing have been investigated by several researchers. Tian et al. [146] explored VD mixing in shock-turbulence interaction using the Favre-averaged scalar variance ~ϕ″2, another second-order statistic. Wong et al. [147] described the evolution of mixedness
Θ=1−4∫¯X′2SF6dxW | (3.15) |
and scalar variance ¯X′2SF6 in RMI with reshock (Figure 11). Livescu et al. [148] studied the vortex stretch in shock-turbulence interaction. Aslangil et al. [149] investigated the joint distribution and evolution of the second and third invariants of velocity gradients, denoted as Q and R, respectively, which is closely related to stretching mechanism, in VD buoyancy-driven turbulence. Tian et al. [150] also examined the joint probability density function (PDF) P(Q,R) in the post-shock turbulence, and discovered that joint PDF is almost completely symmetrical in the heavy-fluid regions, while the joint PDF in light-fluid regions has a similar distribution to that of isotropic turbulence.
Figure 7 summarizes the important areas for VD mixing research. In summary, compared to the well-established understanding of PS mixing, current understanding of VD mixing is still at an early stage. VD mixing has two main characteristics: VD flow characteristics can be influenced by baroclinic vorticity (Eq 3.2), and the mixing indicators change due to the change of the mixing governing equation. VD flow characteristics have been described through linear stability theory [105,109,110,121,122,123] and vortex dynamics theory [102,106,125,126,129,130,131,132]. However, VD mixing indicators are affected by local flow indicators such as the velocity gradient tensors represented by vorticity and strain [49], which brought the challenge to relate mixing indicators and the flow characteristics described by traditional theory. In terms of mixing indicators, research on first-order statistics has mainly focused on the dimensionless mixing time in SBI and RMI problems [134,135,137,138,153]. Furthermore, research has been conducted on second-order statistics to define new VD mixing indicators and study VD mixing phenomena by these new VD indicators [108,141,144]. The evolution of second-order statistics in VD mixing problem have also been preliminarily discussed [138,146,147]. Therefore, coupling the velocity gradient tensor equations and the scalar dissipation rate equation, describing the flow characteristics of VD mixing from the perspective of velocity gradient tensor, and exploring the relationship between scalar dissipation rate and VD flow characteristics, are essential future works for VD mixing research.
Mixing is a fundamental problem in fluid mechanics that has been studied from many different perspectives. PS mixing has been studied in terms of chaotic mixing by Ottino [154], turbulent mixing by Warhaft [16], Dimotakis [23] and Screenivasan [155], and mixing versus stirring by Villermaux [31]. Meanwhile, VD mixing has been analyzed from the perspective of stability theory by Brouillette [99], vortex dynamics by Zabusky [106] and Ranjan [156], VD turbulence by Livescu [103] and Zhou [100,101], and turbulent stratified flow by Caulfield [10]. Despite much progress already made, the relationship between flow and mixing remains the central problem of mixing research and requires further investigation. Understanding this relationship offers the potential for more effective mixing enhancement in applications such as combustor design. Two types of mixing indicators, first-order statistics and second-order statistics, can reflect this relationship. This paper systematically reviews these mixing indicators and their dynamics in PS and VD mixing, as illustrated in Figure 12. Based on the relevant studies reviewed in this paper, the following conclusions can be drawn:
Regarding PS mixing:
1) The first-order statistics theory focuses on the concentration c and reveals the mixing mechanisms on the lamella structures, as highlighted in Figure 12. The advection-diffusion equation (Eq. 2.1) governing the concentration on the moving lamellas can be simplified to the Fourier equation (Eq. 2.4) through the Ranz transform for analytical solution. Within this theoretical framework, key mixing indicators such as the probability distribution of concentration p(c), mixing time ts, and the Batchelor scale η can be defined.
2) The second-order statistics theory focuses on the mixedness f and scalar dissipation rate χ and reveals the mixing mechanisms on the general flow structures. An in-depth understanding of flow and mixing through scalar dissipation rate can be established by examining the stretching term ∇c⋅S⋅∇c in the scalar dissipation rate equation (Eq 2.16). As highlighted by Figure 12, this stretching term is primarily determined by the alignment dynamics of scalar gradient, and by investigating these alignment dynamics, it is shown that scalar dissipation rate exhibits different dynamical properties in flows with different characteristics, which are determined by velocity gradient tensors. In two-dimensional flow, the stretching of the scalar dissipation rate is governed by dimensionless numbers r (Eq. 2.32) and n (Eq. 2.33), while in three-dimensional flow, it is determined by the invariants of the velocity gradient tensor (Eq. 2.34) and the rotation of the strain axis (Eq. 2.27).
Regarding VD mixing:
1) In comparison to PS mixing, baroclinic vorticity generated by the misalignment of the density gradient and pressure gradient can alter the flow in VD mixing. This VD flow characteristic is described from two perspectives: linear stability theory and vortex dynamics. These two methods describe the global characteristic of VD flow, which can hardly relate to molecular mixing.
2) Research on VD mixing under the framework of first-order statistics theory primarily focuses on the dimensionless mixing time in SBI and RMI. As highlighted in Figure 12, mixing time is primarily determined by the stretching rate of the bubble boundary contour given by Eq 2.22. During the modeling of mixing time, new phenomena were discovered, including the second baroclinic vorticity leading to additional stretching and accelerating the mixing process of SBI as in Eq 3.5.
3) Owing to change of the mixing governing equation, second-order indicators for VD mixing are re-defined, such as the mixed mass M in Eq 3.7 and new scalar dissipation rate χ∗ in Eq 3.14. These mixing indicators have been applied to study VD mixing phenomena. Furthermore, the quantities related to the stretching mechanism, such as velocity gradient tensors invariants, have also been primarily investigated in VD mixing problems.
Based on these conclusions, future research in mixing has the following trends:
Regarding PS mixing:
1) Scalar dissipation rate will be key in mixing research and needs to be explored in some extreme conditions, such as turbulence with high Re and Schmidt numbers Sc.
2) The relation between the scalar dissipation rate and "flow structures" needs further clarity.
3) An engineering method for designing flow to achieve optimal mixing is necessary.
Regarding VD mixing:
1) The flow characteristics caused by baroclinic vorticity should be described using local quantities such as velocity gradient tensors.
2) The new second-order statistics should also be explored in limiting conditions in VD turbulence with extremely high Re and Sc numbers.
3) The relationship between VD flow characteristics and VD mixing indicators deserves further study.
This review mainly focuses on non-reacting flow. As mentioned above, scalar dissipation rate is also crucial for studying combustion phenomena and should be used to improve our understanding of combustion and the design of combustion related devices.
This work was supported by the Natural Science Foundation of China (nos. 91941301).
The authors declare they have not used Artificial Intelligence (AI) tools in the creation of this article.
There is no conflict of interest.
[1] |
Cetegen BM, Mohamad N (1993) Experiments on liquid mixing and reaction in a vortex. J Fluid Mech 249: 391–414. https://doi.org/10.1017/S0022112093001223 doi: 10.1017/S0022112093001223
![]() |
[2] |
Verzicco R, Orlandi P (1995) Mixedness in the formation of a vortex ring. Phys Fluids 7: 1513–1515. https://doi.org/10.1063/1.868538 doi: 10.1063/1.868538
![]() |
[3] |
Urzay J (2018) Supersonic combustion in air-breathing propulsion systems for hypersonic flight. Annu Rev Fluid Mech 50: 593–627. https://doi.org/10.1146/annurev-fluid-122316-045217 doi: 10.1146/annurev-fluid-122316-045217
![]() |
[4] | Ferri A, Libby PA, Zakkay V (1964) Theoretical and experimental investigation of supersonic combustion, in High Temperatures in Aeronautics, Elsevier, 55–118. https://doi.org/10.1016/B978-0-08-010558-1.50011-6 |
[5] |
Ferri A (1973) Mixing-controlled supersonic combustion. Annu Rev Fluid Mech 5: 301–338. https://doi.org/10.1146/annurev.fl.05.010173.001505 doi: 10.1146/annurev.fl.05.010173.001505
![]() |
[6] | Gupta AK, Lilley DG, Syred N (1984) Swirl flows. Tunbridge Wells. |
[7] |
Candel S, Durox D, Schuller T, et al. (2014) Dynamics of swirling flames. Annu Rev Fluid Mech 46: 147–173. https://doi.org/10.1146/annurev-fluid-010313-141300 doi: 10.1146/annurev-fluid-010313-141300
![]() |
[8] | Bahr D (1987) Technology for the design of high temperature rise combustors. J Propuls Power 3: 179–186. |
[9] |
An Q, Steinberg AM (2019) The role of strain rate, local extinction, and hydrodynamic instability on transition between attached and lifted swirl flames. Combusti Flame 199: 267–278. https://doi.org/10.1016/j.combustflame.2018.10.029 doi: 10.1016/j.combustflame.2018.10.029
![]() |
[10] |
Caulfield C (2021) Layering, instabilities, and mixing in turbulent stratified flows. Annu Rev Fluid Mech 53: 113–145. https://doi.org/10.1146/annurev-fluid-042320-100458 doi: 10.1146/annurev-fluid-042320-100458
![]() |
[11] |
Crimaldi JP, Zimmer RK (2014) The physics of broadcast spawning in benthic invertebrates. Annu Rev Mar Sci 6: 1. https://doi.org/10.1146/annurev-marine-010213-135119 doi: 10.1146/annurev-marine-010213-135119
![]() |
[12] |
Mingotti N, Wood R, Noakes C, et al. (2020) The mixing of airborne contaminants by the repeated passage of people along a corridor. J Fluid Mech 903: A52. https://doi.org/10.1017/jfm.2020.671 doi: 10.1017/jfm.2020.671
![]() |
[13] |
Lohse D, Xia KQ (2010) Small-scale properties of turbulent rayleigh-bénard convection. Annu Rev Fluid Mech 42: 335–364. https://doi.org/10.1146/annurev-fluid-10908.165152 doi: 10.1146/annurev-fluid-10908.165152
![]() |
[14] |
Kolmogorov AN (1941) The local structure of turbulence in incompressible viscous fluid for very large reynolds numbers. Cr Acad Sci URSS 30: 301–305. https://doi.org/10.1007/978-94-011-3030-1_45 doi: 10.1007/978-94-011-3030-1_45
![]() |
[15] |
Batchelor GK (1959) Small-scale variation of convected quantities like temperature in turbulent fluid Part 1. general discussion and the case of small conductivity. J Fluid Mech 5: 113–133. https://doi.org/10.1017/S002211205900009X doi: 10.1017/S002211205900009X
![]() |
[16] |
Warhaft Z (2000) Passive scalars in turbulent flows. Annu Rev Fluid Mech 32: 203–240. https://doi.org/10.1146/annurev.fluid.32.1.203 doi: 10.1146/annurev.fluid.32.1.203
![]() |
[17] |
Schwertfirm F, Manhart M (2007) Dns of passive scalar transport in turbulent channel flow at high schmidt numbers. Int J Heat Fluid Fl 28: 1204–1214. https://doi.org/10.1016/j.ijheatfluidflow.2007.05.012 doi: 10.1016/j.ijheatfluidflow.2007.05.012
![]() |
[18] |
Dimotakis PE (2000) The mixing transition in turbulent flows. J Fluid Mech 409: 69–98. https://doi.org/10.1017/S0022112099007946 doi: 10.1017/S0022112099007946
![]() |
[19] |
Meunier P, Villermaux E (2003) How vortices mix. J Fluid Mech 476: 213–222. https://doi.org/10.1017/S0022112002003166 doi: 10.1017/S0022112002003166
![]() |
[20] |
Souzy M, Zaier I, Lhuissier H, et al. (2018) Mixing lamellae in a shear flow. J Fluid Mech 838: R3. https://doi.org/10.1017/jfm.2017.916 doi: 10.1017/jfm.2017.916
![]() |
[21] |
Buaria D, Clay MP, Sreenivasan KR, et al. (2021) Turbulence is an ineffective mixer when schmidt numbers are large. Phys Rev Lett 126: 074501. https://doi.org/10.1103/PhysRevLett.126.074501 doi: 10.1103/PhysRevLett.126.074501
![]() |
[22] |
Raynal F, Gence JN (1997) Energy saving in chaotic laminar mixing. Int J Heat Mass Trans 40: 3267–3273. https://doi.org/10.1016/S0017-9310(96)00383-3 doi: 10.1016/S0017-9310(96)00383-3
![]() |
[23] |
Dimotakis PE (2005) Turbulent mixing. Annu Rev Fluid Mech 37: 329–356. https://doi.org/10.1146/annurev.fluid.36.050802.122015 doi: 10.1146/annurev.fluid.36.050802.122015
![]() |
[24] |
Thiffeault JL (2012) Using multiscale norms to quantify mixing and transport. Nonlinearity 25: R1. https://doi.org/10.1088/0951-7715/25/2/R1 doi: 10.1088/0951-7715/25/2/R1
![]() |
[25] |
Duplat J, Jouary A, Villermaux E (2010) Entanglement rules for random mixtures. Phys Rev Lett 105: 034504. https://doi.org/10.1103/PhysRevLett.105.034504 doi: 10.1103/PhysRevLett.105.034504
![]() |
[26] |
Kree M, Duplat J, Villermaux E (2013) The mixing of distant sources. Phys Fluids 25: 091103. https://doi.org/10.1063/1.4820015 doi: 10.1063/1.4820015
![]() |
[27] |
Villermaux E, Rehab H (2000) Mixing in coaxial jets. J Fluid Mech 425: 161–185. https://doi.org/10.1017/S002211200000210X doi: 10.1017/S002211200000210X
![]() |
[28] | Vidick B (1989) Critical mixing parameters for good control of cement slurry quality. in SPE Production Operations Symposium. OnePetro. https://doi.org/10.2118/18895-PA |
[29] |
Poulain S, Villermaux E, Bourouiba L (2018) Ageing and burst of surface bubbles. J Fluid Mech 851: 636–671. https://doi.org/10.1017/jfm.2018.471 doi: 10.1017/jfm.2018.471
![]() |
[30] | Marble F (1985) Growth of a diffusion flame in the field of a vortex, in Recent advances in the aerospace sciences, Springer, 395–413. https://doi.org/10.1007/978-1-4684-4298-4_19 |
[31] |
Villermaux E (2019) Mixing versus stirring. Annu Rev Fluid Mech 51: 245–273. https://doi.org/10.1146/annurev-fluid-010518-040306 doi: 10.1146/annurev-fluid-010518-040306
![]() |
[32] |
Ranz WE (1979) Applications of a stretch model to mixing, diffusion, and reaction in laminar and turbulent flows. AIChE J 25: 41–47. https://doi.org/10.1002/aic.690250105 doi: 10.1002/aic.690250105
![]() |
[33] | Marble FE, Broadwell JE (1977) The coherent flame model for turbulent chemical reactions. Purdue Univ Lafayette in project squid head quaters. Tech Rep. Available from: https://api.semanticscholar.org/CorpusID:45411925. |
[34] |
Villermaux E, Duplat J (2003) Mixing is an aggregation process. Comptes Rendus Mécanique 331: 515–523. https://doi.org/10.1016/S1631-0721(03)00110-4 doi: 10.1016/S1631-0721(03)00110-4
![]() |
[35] |
Duplat J, Villermaux E (2008) Mixing by random stirring in confined mixtures. J Fluid Mech 617: 51–86. https://doi.org/10.1017/S0022112008003789 doi: 10.1017/S0022112008003789
![]() |
[36] |
Duplat J, Innocenti C, Villermaux E (2010) A nonsequential turbulent mixing process. Phys Fluids 22: 035104. https://doi.org/10.1063/1.3319821 doi: 10.1063/1.3319821
![]() |
[37] |
Haller G (2015) Lagrangian coherent structures. Annu Rev Fluid Mech 47: 137–162. https://doi.org/10.1146/annurev-fluid-010313-141322 doi: 10.1146/annurev-fluid-010313-141322
![]() |
[38] |
Hang H, Yu B, Xiang Y, et al. (2020) An objective-adaptive refinement criterion based on modified ridge extraction method for finite-time lyapunov exponent (ftle) calculation. J Visual 23: 81–95. https://doi.org/10.1007/S12650-019-00605-1 doi: 10.1007/S12650-019-00605-1
![]() |
[39] |
Liang G, Yu B, Zhang B, et al. (2019) Hidden flow structures in compressible mixing layer and a quantitative analysis of entrainment based on lagrangian method. J Hydrodyn 31: 256–265. https://doi.org/10.1017/jfm.2020.295 doi: 10.1017/jfm.2020.295
![]() |
[40] |
Zheng Z, Fan Z, Wang Z, et al. (2021) Lagrangian visualization of mixing enhancement induced by finite-time stretching in compressible vortex interaction. J Visual 24: 19–28. https://doi.org/10.1007/s12650-020-00698-z doi: 10.1007/s12650-020-00698-z
![]() |
[41] |
Götzfried P, Emran MS, Villermaux E, et al. (2019) Comparison of lagrangian and eulerian frames of passive scalar turbulent mixing. Phys Rev Fluids 4: 044607. https://doi.org/10.1103/PhysRevFluids.4.044607 doi: 10.1103/PhysRevFluids.4.044607
![]() |
[42] |
Meunier P, Villermaux E (2022) The diffuselet concept for scalar mixing. J Fluid Mech 951: A33. https://doi.org/10.1017/jfm.2022.771 doi: 10.1017/jfm.2022.771
![]() |
[43] |
Meunier P, Villermaux E (2010) The diffusive strip method for scalar mixing in two dimensions. J Fluid Mechanics 662: 134–172. https://doi.org/10.1017/S0022112010003162 doi: 10.1017/S0022112010003162
![]() |
[44] |
Martínez-Ruiz D, Meunier P, Favier B, et al.(2018) The diffusive sheet method for scalar mixing. J Fluid Mech 837: 230–257. https://doi.org/10.1017/S0022112010003162 doi: 10.1017/S0022112010003162
![]() |
[45] |
Sen S, Singh P, Heyman J, et al. (2020) The impact of stretching-enhanced mixing and coalescence on reactivity in mixing-limited reactive flows. Phys Fluids 32: 106602, 2020. https://doi.org/10.1063/5.0022798 doi: 10.1063/5.0022798
![]() |
[46] |
Heyman J, Lester DR, Turuban R, et al. (2020) Stretching and folding sustain microscale chemical gradients in porous media. P Natl Acad Sci 117: 13 359–13 365. https://doi.org/10.1073/pnas.2002858117 doi: 10.1073/pnas.2002858117
![]() |
[47] |
Guilbert E, Almarcha C, Villermaux E (2021) Chemical reaction for mixing studies. Phys Rev Fluids 6: 114501. https://doi.org/10.1103/PhysRevFluids.6.114501 doi: 10.1103/PhysRevFluids.6.114501
![]() |
[48] |
Guilbert E, Metzger B, Villermaux E (2022) Chemical production on a deforming substrate. J Fluid Mech 934: R1. https://doi.org/10.1017/jfm.2021.1122 doi: 10.1017/jfm.2021.1122
![]() |
[49] |
Buch KA, Dahm WJ (1996) Experimental study of the fine-scale structure of conserved scalar mixing in turbulent shear flows. part 1. sc [dbl greater-than sign] 1. J Fluid Mech 317: 21–71. https://doi.org/10.1017/s0022112096000651 doi: 10.1017/s0022112096000651
![]() |
[50] |
Cetegen B, Aguirre J (1990) Analysis of molecular mixing and chemical reaction in a vortex pair. Phys Fluids 2: 2211–2216. https://doi.org/10.1063/1.857807 doi: 10.1063/1.857807
![]() |
[51] |
Basu S, Barber T, Cetegen B (2007) Computational study of scalar mixing in the field of a gaseous laminar line vortex. Phys Fluids 19: 053601. https://doi.org/10.1063/1.2732454 doi: 10.1063/1.2732454
![]() |
[52] |
Flohr P, Vassilicos J (1997) Accelerated scalar dissipation in a vortex. J Fluid Mech 348: 295–317. https://doi.org/10.1017/S0022112097006927 doi: 10.1017/S0022112097006927
![]() |
[53] |
Bilger R (1976) The structure of diffusion flames. Combust Sci Technol 13: 155–170. https://doi.org/10.1080/00102207608946733 doi: 10.1080/00102207608946733
![]() |
[54] |
Bilger RW (1989) Turbulent diffusion flames. Annu Rev Fluid Mech 21: 101–135. https://doi.org/10.1146/annurev.fl.21.010189.000533 doi: 10.1146/annurev.fl.21.010189.000533
![]() |
[55] |
Yeung P, Girimaji S, Pope S (1990) Straining and scalar dissipation on material surfaces in turbulence: implications for flamelets. Combust Flame 79: 340–365. https://doi.org/10.1016/0010-2180(90)90145-H doi: 10.1016/0010-2180(90)90145-H
![]() |
[56] |
Kim SH, Pitsch H (2007) Scalar gradient and small-scale structure in turbulent premixed combustion. Phys Fluids 19: 115104. https://doi.org/10.1063/1.2784943 doi: 10.1063/1.2784943
![]() |
[57] |
Boratav O, Elghobashi S, Zhong R (1996) On the alignment of the alpha-strain and vorticity in turbulent nonpremixed flames. Phys Fluids 8: 2251–2253. https://doi.org/10.1063/1.869000 doi: 10.1063/1.869000
![]() |
[58] |
Boratav O, Elghobashi S, Zhong R (1998) On the alignment of strain, vorticity and scalar gradient in turbulent, buoyant, nonpremixed flames. Phys Fluids 10: 2260–2267. https://doi.org/10.1063/1.869747 doi: 10.1063/1.869747
![]() |
[59] |
Attili A, Bisetti F (2019) Statistics of scalar dissipation and strain/vorticity/scalar gradient alignment in turbulent nonpremixed jet flames. Flow Turbul Combust 103: 625–642. https://doi.org/10.1007/s10494-019-00044-w doi: 10.1007/s10494-019-00044-w
![]() |
[60] |
McManus TA, Sutton JA (2022) Conditional analysis of temperature and strain rate effects on dissipation structure in turbulent non-premixed jet flames. P Combust Inst. https://doi.org/10.1016/j.proci.2022.07.052 doi: 10.1016/j.proci.2022.07.052
![]() |
[61] |
Chakraborty N, Swaminathan N (2007) Influence of the damköhler number on turbulence-scalar interaction in premixed flames. i. physical insight. Phys Fluids 19: 045103. https://doi.org/10.1063/1.2714070 doi: 10.1063/1.2714070
![]() |
[62] |
Zhao S, Er-Raiy A, Bouali Z, et al. (2018) Dynamics and kinematics of the reactive scalar gradient in weakly turbulent premixed flames. Combust Flame 198: 436–454. https://doi.org/10.1016/j.combustflame.2018.10.002 doi: 10.1016/j.combustflame.2018.10.002
![]() |
[63] |
Batchelor GK (1952) The effect of homogeneous turbulence on material lines and surfaces. Proc Math Phys Sci 213: 349–366. https://doi.org/10.1098/rspa.1952.0130 doi: 10.1098/rspa.1952.0130
![]() |
[64] |
Girimaji S, Pope S (1990) 'Material-element deformation in isotropic turbulence. J Fluid Mech 220: 427–458. https://doi.org/10.1017/S0022112090003330 doi: 10.1017/S0022112090003330
![]() |
[65] |
Dresselhaus E, Tabor M (1992) The kinematics of stretching and alignment of material elements in general flow fields. J Fluid Mech 236: 415–444. https://doi.org/10.1017/S0022112092001460 doi: 10.1017/S0022112092001460
![]() |
[66] |
Lapeyre G, Klein P, Hua B (1999) Does the tracer gradient vector align with the strain eigenvectors in 2d turbulence? Phys Fluids 11: 3729–3737. https://doi.org/10.1063/1.870234 doi: 10.1063/1.870234
![]() |
[67] |
Klein P, Hua BL, Lapeyre G (2000) Alignment of tracer gradient vectors in 2d turbulence. Physica D 146: 246–260. https://doi.org/10.1016/S0167-2789(00)00119-6 doi: 10.1016/S0167-2789(00)00119-6
![]() |
[68] |
Swaminathan N, Grout R (2006) Interaction of turbulence and scalar fields in premixed flames. Phys Fluids 18: 045102. https://doi.org/10.1063/1.2186590 doi: 10.1063/1.2186590
![]() |
[69] |
Minamoto Y, Jigjid K, Igari R, et al. (2022) Effect of flame–flame interaction on scalar pdf in turbulent premixed flames. Combust Flame 239: 111660. https://doi.org/10.1016/j.combustflame.2021.111660 doi: 10.1016/j.combustflame.2021.111660
![]() |
[70] |
Holzner M, Lüthi B (2011) Laminar superlayer at the turbulence boundary. Phys Rev Lett 106, : 134503. https://doi.org/10.1103/PhysRevLett.106.134503 doi: 10.1103/PhysRevLett.106.134503
![]() |
[71] |
Townsend AA (1951) The diffusion of heat spots in isotropic turbulence. Proc Math Phys Sci 209: 418–430. https://doi.org/10.1098/rspa.1951.0216 doi: 10.1098/rspa.1951.0216
![]() |
[72] |
Cocke W (1969) Turbulent hydrodynamic line stretching: consequences of isotropy. Phys Fluids 12: 2488–2492. https://doi.org/10.1063/1.1692385 doi: 10.1063/1.1692385
![]() |
[73] |
Ashurst WT, Kerstein A, Kerr R, et al. (1987) Alignment of vorticity and scalar gradient with strain rate in simulated navier–stokes turbulence. Phys Fluids 30: 2343–2353. https://doi.org/10.1063/1.866513 doi: 10.1063/1.866513
![]() |
[74] |
Vincent A, Meneguzzi M (1991) The spatial structure and statistical properties of homogeneous turbulence. J Fluid Mech 225: 1–20. https://doi.org/10.1017/S0022112091001957 doi: 10.1017/S0022112091001957
![]() |
[75] |
Carter HH, Okubo A (1972) Longitudinal dispersion in non-uniform flow. Water Resour Res 8: 648–660. https://doi.org/10.1029/WR008i003p00648 doi: 10.1029/WR008i003p00648
![]() |
[76] |
Weiss J (1991) The dynamics of enstrophy transfer in two-dimensional hydrodynamics. Physica D 48: 273–294. https://doi.org/10.1016/0167-2789(91)90088-Q doi: 10.1016/0167-2789(91)90088-Q
![]() |
[77] | Hunt JC, Wray AA, Moin P (1988) Eddies, streams, and convergence zones in turbulent flows. Studying turbulence using numerical simulation databases, 2. Proceedings of the 1988 summer program. Available from: https://ntrs.nasa.gov/citations/19890015184 |
[78] |
Basdevant C, Philipovitch T (1994) On the validity of the "weiss criterion" in two-dimensional turbulence. Physica D 73: 17–30. https://doi.org/10.1016/0167-2789(94)90222-4 doi: 10.1016/0167-2789(94)90222-4
![]() |
[79] |
Hua B, Klein P (1998) An exact criterion for the stirring properties of nearly two-dimensional turbulence. Physica D 113: 98–110. https://doi.org/10.1016/S0167-2789(97)00143-7 doi: 10.1016/S0167-2789(97)00143-7
![]() |
[80] |
Galanti B, Gibbon J, Heritage M (1997) Vorticity alignment results for the three-dimensional euler and navier-stokes equations. Nonlinearity 10: 1675. https://doi.org/10.1088/0951-7715/10/6/013 doi: 10.1088/0951-7715/10/6/013
![]() |
[81] |
Ohkitani K, Kishiba S (1995) Nonlocal nature of vortex stretching in an inviscid fluid. Phys Fluids 7: 411–421. https://doi.org/10.1063/1.868638 doi: 10.1063/1.868638
![]() |
[82] |
Tsinober A, Ortenberg M, Shtilman L (1999) On depression of nonlinearity in turbulence. Phys Fluids 11: 2291–2297. https://doi.org/10.1063/1.870091 doi: 10.1063/1.870091
![]() |
[83] |
Wacks DH, Chakraborty N, Klein M, et al. (2016) Flow topologies in different regimes of premixed turbulent combustion: A direct numerical simulation analysis. Phys Rev Fluids 1: 083401. https://doi.org/10.1103/PhysRevFluids.1.083401 doi: 10.1103/PhysRevFluids.1.083401
![]() |
[84] |
Meneveau C (2011) Lagrangian dynamics and models of the velocity gradient tensor in turbulent flows. Annu Rev Fluid Mech 43: 219–245. https://doi.org/10.1146/annurev-fluid-122109-160708 doi: 10.1146/annurev-fluid-122109-160708
![]() |
[85] |
Jeong J, Hussain F (1995) On the identification of a vortex. J Fluid Mech 285: 69–94. https://doi.org/10.1017/S0022112095000462 doi: 10.1017/S0022112095000462
![]() |
[86] |
Chong MS, Perry AE, Cantwell BJ (1990) A general classification of three-dimensional flow fields. Phys Fluids A 2: 765–777. https://doi.org/10.1063/1.857730 doi: 10.1063/1.857730
![]() |
[87] |
Perry AE, Chong MS (1987) A description of eddying motions and flow patterns using critical-point concepts. Annu Rev Fluid Mech 19: 125–155. https://doi.org/10.1146/annurev.fl.19.010187.001013 doi: 10.1146/annurev.fl.19.010187.001013
![]() |
[88] |
Danish M, Suman S, Girimaji SS (2016) Influence of flow topology and dilatation on scalar mixing in compressible turbulence. J Fluid Mech 793: 633–655. https://doi.org/10.1017/jfm.2016.145 doi: 10.1017/jfm.2016.145
![]() |
[89] |
Gao X, Bermejo-Moreno I, Larsson J (2020) Parametric numerical study of passive scalar mixing in shock turbulence interaction. J Fluid Mech 895: A21. https://doi.org/10.1017/jfm.2020.292 doi: 10.1017/jfm.2020.292
![]() |
[90] |
Cifuentes L, Dopazo C, Martin J, et al. (2014) Local flow topologies and scalar structures in a turbulent premixed flame. Phys Fluids 26: 065108. https://doi.org/10.1063/1.4884555 doi: 10.1063/1.4884555
![]() |
[91] |
Han W, Scholtissek A, Dietzsch F, et al. (2019) Influence of flow topology and scalar structure on flame-tangential diffusion in turbulent non-premixed combustion. Combust Flame 206: 21–36. https://doi.org/10.1016/j.combustflame.2019.04.038 doi: 10.1016/j.combustflame.2019.04.038
![]() |
[92] |
Heyman J, Lester D, Le Borgne T (2021) Scalar signatures of chaotic mixing in porous media. Phys Rev Lett 126: 034505. https://doi.org/10.1103/PhysRevLett.126.034505 doi: 10.1103/PhysRevLett.126.034505
![]() |
[93] |
Waitz I, Qiu Y, Manning T, et al. (1997) Enhanced mixing with streamwise vorticity. Prog Aerosp Sci 33: 323–351. https://doi.org/10.1016/S0376-0421(96)00008-5 doi: 10.1016/S0376-0421(96)00008-5
![]() |
[94] |
Vergine F, Ground C, Maddalena L (2016) Turbulent kinetic energy decay in supersonic streamwise interacting vortices. J Fluid Mech 807: 353–385. https://doi.org/10.1017/jfm.2016.611 doi: 10.1017/jfm.2016.611
![]() |
[95] |
Wu Z, He M, Yu B, et al. (2022) A circulation prediction model for ramp and vortex generator in supersonic flow: A numerical study. Aerosp Sci Technol 127: 107688. https://doi.org/10.1016/j.ast.2022.107688 doi: 10.1016/j.ast.2022.107688
![]() |
[96] |
Wang Z, Yu B, Zhang B, et al. (2021) Kinematic and mixing characteristics of vortex interaction induced by a vortex generator model: a numerical study. Appl Math Mech 42: 387–404. https://doi.org/10.1007/s10483-021-2711-5 doi: 10.1007/s10483-021-2711-5
![]() |
[97] |
Schetz JA, Maddalena L, Burger SK (2010) Molecular weight and shock-wave effects on transverse injection in supersonic flow. J Propuls Power 26: 1102–1113. https://doi.org/10.2514/1.49355 doi: 10.2514/1.49355
![]() |
[98] |
Tew DE, Hermanson JC, Waitz IA (2004) Impact of compressibility on mixing downstream of lobed mixers. AIAA J 42: 2393–2396. https://doi.org/10.2514/1.11004 doi: 10.2514/1.11004
![]() |
[99] |
Brouillette M (2002) The richtmyer-meshkov instability. Annu Rev Fluid Mech 34: 445–468. https://doi.org/10.1146/annurev.fluid.34.090101.162238 doi: 10.1146/annurev.fluid.34.090101.162238
![]() |
[100] |
Zhou Y (2017) Rayleigh–taylor and richtmyer–meshkov instability induced flow, turbulence, and mixing. ii. Phys Rep 723: 1–160. https://doi.org/10.1016/j.physrep.2017.07.008 doi: 10.1016/j.physrep.2017.07.008
![]() |
[101] |
Zhou Y (2017) Rayleigh–taylor and richtmyer–meshkov instability induced flow, turbulence, and mixing. i. Phys Rep 720-722: 1–136. https://doi.org/10.1016/j.physrep.2017.07.005 doi: 10.1016/j.physrep.2017.07.005
![]() |
[102] |
Peng G, Zabusky NJ, Zhang S (2003) Vortex-accelerated secondary baroclinic vorticity deposition and late-intermediate time dynamics of a two-dimensional richtmyer–meshkov interface. Phys Fluids 15: 3730–3744. https://doi.org/10.1063/1.1621628 doi: 10.1063/1.1621628
![]() |
[103] |
Livescu D (2020) Turbulence with large thermal and compositional density variations. Annu Rev Fluid Mech 52: 309-341. https://doi.org/10.1146/annurev-fluid-010719-060114 doi: 10.1146/annurev-fluid-010719-060114
![]() |
[104] |
Weber C, Haehn N, Oakley J, et al. (2012) Turbulent mixing measurements in the richtmyer-meshkov instability. Phys Fluids 24: 074105. https://doi.org/10.1063/1.4733447 doi: 10.1063/1.4733447
![]() |
[105] |
Mikaelian KO (1998) Analytic approach to nonlinear rayleigh-taylor and richtmyer-meshkov instabilities. Phys Rev Lett 80: 508–511. https://doi.org/10.1103/PhysRevLett.80.508 doi: 10.1103/PhysRevLett.80.508
![]() |
[106] |
Zabusky NJ (1999) Vortex paradigm for accelerated inhomogeneous flows: Visiometrics for the rayleigh-taylor and richtmyer-meshkov environments. Annu Rev Fluid Mech 31: 495–536. https://doi.org/10.1146/annurev.fluid.31.1.495 doi: 10.1146/annurev.fluid.31.1.495
![]() |
[107] |
Kumar S, Orlicz G, Tomkins C, et al. (2005) Stretching of material lines in shock-accelerated gaseous flows. Phys Fluids 17: 082107. https://doi.org/10.1063/1.2031347 doi: 10.1063/1.2031347
![]() |
[108] |
Zhou Y, Cabot WH, Thornber B (2016) Asymptotic behavior of the mixed mass in rayleigh–taylor and richtmyer–meshkov instability induced flows. Phys Plasmas 23: 052712. https://doi.org/10.1063/1.4951018 doi: 10.1063/1.4951018
![]() |
[109] |
Richtmyer RD (1960) Taylor instability in shock acceleration of compressible fluids. Commun Pure Appl Math 13: 297–319. https://doi.org/10.1002/cpa.3160130207 doi: 10.1002/cpa.3160130207
![]() |
[110] |
Meshkov E (1969) Instability of the interface of two gases accelerated by a shock wave. Fluid Dyn 4: 101–104. https://doi.org/10.1007/BF01015969 doi: 10.1007/BF01015969
![]() |
[111] |
Klein RI, McKee CF, Colella P (1994) On the hydrodynamic interaction of shock waves with interstellar clouds. 1: Nonradiative shocks in small clouds. Astrophys J 420: 213–236. https://doi.org/10.1086/173554 doi: 10.1086/173554
![]() |
[112] |
Cabot WH, Cook AW (2006) Reynolds number effects on rayleigh–taylor instability with possible implications for type ia supernovae. Nat Phys 2: 562–568. https://doi.org/10.1038/nphys361 doi: 10.1038/nphys361
![]() |
[113] |
Lindl JD, McCrory RL, Campbell EM (1992) Progress toward ignition and burn propagation in inertial confinement fusion. Phys Today 45: 32–40. https://doi.org/10.1063/1.881318 doi: 10.1063/1.881318
![]() |
[114] |
EOran ES, Gamezo VN (2007) Origins of the deflagration-to-detonation transition in gas-phase combustion. Combust Flame 148: 4–47. https://doi.org/10.1016/j.combustflame.2006.07.010 doi: 10.1016/j.combustflame.2006.07.010
![]() |
[115] |
Yang J, Kubota T, Zukoski EE (1994) A model for characterization of a vortex pair formed by shock passage over a light-gas inhomogeneity. J Fluid Mech 258: 217–244. https://doi.org/10.1017/S0022112094003307 doi: 10.1017/S0022112094003307
![]() |
[116] |
Yu B, He M, Zhang B, et al. (2020) Two-stage growth mode for lift-off mechanism in oblique shock-wave/jet interaction. Phys Fluids 32: 116105. https://doi.org/10.1063/5.0022449 doi: 10.1063/5.0022449
![]() |
[117] |
Zhang B, Liu H, Yu B, et al. (2022) Numerical investigation on combustion-enhancement strategy in shock–fuel jet interaction. AIAA J 60: 393–410. https://doi.org/10.2514/1.J060168 doi: 10.2514/1.J060168
![]() |
[118] |
Zhang B, Chen H, Yu B, et al. (2019) Molecular simulation on viscous effects for microscale combustion in reactive shock-bubble interaction. Combust Flame 208: 351–363. https://doi.org/10.1016/j.combustflame.2019.07.001 doi: 10.1016/j.combustflame.2019.07.001
![]() |
[119] |
Liu H, Yu B, Chen H, et al. (2020) Contribution of viscosity to the circulation deposition in the richtmyer–meshkov instability. J Fluid Mech 895: A10. https://doi.org/10.1017/jfm.2020.295 doi: 10.1017/jfm.2020.295
![]() |
[120] |
Wang Z, Yu B, Chen H, et al. (2018) Scaling vortex breakdown mechanism based on viscous effect in shock cylindrical bubble interaction. Phys Fluids 30: 126103. https://doi.org/10.1063/1.5051463 doi: 10.1063/1.5051463
![]() |
[121] |
Ding J, Si T, Yang J, et al. (2017) Measurement of a richtmyer-meshkov instability at an air-sf 6 interface in a semiannular shock tube. Phys Rev Lett 119: 014501. https://doi.org/10.1103/PhysRevLett.119.014501 doi: 10.1103/PhysRevLett.119.014501
![]() |
[122] |
Cook AW, Dimotakis PE (2001) Transition stages of rayleigh–taylor instability between miscible fluids. J Fluid Mech 443: 69–99. https://doi.org/10.1017/S0022112002007802 doi: 10.1017/S0022112002007802
![]() |
[123] |
Cook AW, Cabot W, Miller PL (2004) The mixing transition in rayleigh–taylor instability. J Fluid Mech 511: 333–362. https://doi.org/10.1017/S0022112004009681 doi: 10.1017/S0022112004009681
![]() |
[124] |
Ristorcelli J, Clark T (2004) Rayleigh–taylor turbulence: self-similar analysis and direct numerical simulations. J Fluid Mech 507: 213–253. https://doi.org/10.1017/S0022112004008286 doi: 10.1017/S0022112004008286
![]() |
[125] |
Samtaney R, Zabusky NJ (1993) On shock polar analysis and analytical expressions for vorticity deposition in shock-accelerated density-stratified interfaces. Phys Fluids A: Fluid Dyn 5: 1285–1287. https://doi.org/10.1063/1.858618 doi: 10.1063/1.858618
![]() |
[126] |
Marugan-Cruz C, Rodriguez-Rodriguez J, Martinez-Bazan C (2013) Formation regimes of vortex rings in negatively buoyant starting jets. J Fluid Mech 716: 470–486. https://doi.org/10.1017/jfm.2012.554 doi: 10.1017/jfm.2012.554
![]() |
[127] |
Soteriou MC, Ghoniem AF (1995) Effects of the free stream density ratio on free and forced spatially developing shear layers. Phys Fluids 7: 2036–2051. https://doi.org/10.1063/1.868451 doi: 10.1063/1.868451
![]() |
[128] |
Livescu D, Ristorcelli J, Petersen M, et al. (2010) New phenomena in variable-density rayleigh–taylor turbulence. Phys Scripta 2010: 014015. https://doi.org/10.1088/0031-8949/2010/T142/014015 doi: 10.1088/0031-8949/2010/T142/014015
![]() |
[129] |
Zhai Z, Si T, Luo X, et al. (2011) On the evolution of spherical gas interfaces accelerated by a planar shock wave. Phys Fluids 23: 084104. https://doi.org/10.1063/1.3623272 doi: 10.1063/1.3623272
![]() |
[130] |
Si T, Long T, Zhai Z, et al. (2015) Experimental investigation of cylindrical converging shock waves interacting with a polygonal heavy gas cylinder. J Fluid Mech 784: 225–251. https://doi.org/10.1017/jfm.2015.581 doi: 10.1017/jfm.2015.581
![]() |
[131] |
Luo X, Wang M, Si T, et al. (2015) On the interaction of a planar shock with an polygon. J Fluid Mech 773: 366–394. https://doi.org/10.1017/jfm.2015.257 doi: 10.1017/jfm.2015.257
![]() |
[132] |
Ding J, Si T, Chen M, et al. (2017) On the interaction of a planar shock with a three-dimensional light gas cylinder. J Fluid Mech 828: 289–317. https://doi.org/10.1017/jfm.2017.528 doi: 10.1017/jfm.2017.528
![]() |
[133] |
Li D, Guan B, Wang G (2022) Effects of interface diffusion and shock strength on shock-accelerated sf6 cylinder. Phys Fluids 34: 076109. https://doi.org/10.1063/5.0099353 doi: 10.1063/5.0099353
![]() |
[134] |
Vorobieff P, Rightley PM, Benjamin RF (1998) Power-law spectra of incipient gas-curtain turbulence. Phys Rev Lett 81: 2240. https://doi.org/10.1103/PhysRevLett.81.2240 doi: 10.1103/PhysRevLett.81.2240
![]() |
[135] |
Niederhaus JH, Greenough J, Oakley J, et al. (2008) A computational parameter study for the three-dimensional shock–bubble interaction. J Fluid Mech 594: 85–124. https://doi.org/10.1017/S0022112007008749 doi: 10.1017/S0022112007008749
![]() |
[136] |
Lombardini M, Pullin D, Meiron D (2012) Transition to turbulence in shock-driven mixing: a mach number study. J Fluid Mech 690: 203–226. https://doi.org/10.1017/jfm.2011.425 doi: 10.1017/jfm.2011.425
![]() |
[137] |
Oggian T, Drikakis D, Youngs D, et al. (2015) Computing multi-mode shock-induced compressible turbulent mixing at late times. J Fluid Mech 779: 411–431. https://doi.org/10.1017/jfm.2015.392 doi: 10.1017/jfm.2015.392
![]() |
[138] |
Liu H, Yu B, Zhang B, et al. (2020) On mixing enhancement by secondary baroclinic vorticity in shock bubble interaction. J Fluid Mech 931: A17. https://doi.org/10.1017/jfm.2021.923 doi: 10.1017/jfm.2021.923
![]() |
[139] |
Li Y, Wang Z, Yu B, et al. (2019) Gaussian models for late-time evolution of two-dimensional shock–light cylindrical bubble interaction. Shock Waves 1–16. https://doi.org/10.1007/s00193-019-00928-w doi: 10.1007/s00193-019-00928-w
![]() |
[140] |
Thornber B, Griffond J, Poujade O, et al. (2017) Late-time growth rate, mixing, and anisotropy in the multimode narrowband richtmyer–meshkov instability: The θ-group collaboration. Phys Fluids 29: 105107. https://doi.org/10.1063/1.4993464 doi: 10.1063/1.4993464
![]() |
[141] |
Li H, He Z, Zhang Y, et al. (2019) On the role of rarefaction/compression waves in richtmyer-meshkov instability with reshock. Phys Fluids 31: 054102. https://doi.org/10.1063/1.5083796 doi: 10.1063/1.5083796
![]() |
[142] |
Bin Y, Xiao M, Shi Y, et al. (2021) A new idea to predict reshocked richtmyer–meshkov mixing: constrained large-eddy simulation. J Fluid Mech 918: R1. https://doi.org/10.1017/jfm.2021.332 doi: 10.1017/jfm.2021.332
![]() |
[143] |
Ruan YC, Zhang YS, Tian BL, et al. (2020) Density-ratio-invariant mean-species profile of classical rayleigh-taylor mixing. Phys Rev Fluids 5: 054501. https://doi.org/10.1103/PhysRevFluids.5.054501 doi: 10.1103/PhysRevFluids.5.054501
![]() |
[144] |
Yu B, Liu H, Liu H (2021) Scaling behavior of density gradient accelerated mixing rate in shock bubble interaction. Phys Rev Fluids 6: 064502. https://doi.org/10.1103/PhysRevFluids.6.064502 doi: 10.1103/PhysRevFluids.6.064502
![]() |
[145] |
Yu B, Li L, Xu H, et al. (2022) Effects of reynolds number and schmidt number on variable density mixing in shock bubble interaction. Acta Mech Sinica 38: 121256. https://doi.org/10.1007/s10409-022-09011-9 doi: 10.1007/s10409-022-09011-9
![]() |
[146] |
Tian Y, Jaberi FA, Li Z, et al. (2017) Numerical study of variable density turbulence interaction with a normal shock wave. J Fluid Mech 829: 551–588. https://doi.org/10.1017/jfm.2017.542 doi: 10.1017/jfm.2017.542
![]() |
[147] |
Wong ML, Livescu D, Lele SK (2019) High-resolution navier-stokes simulations of richtmyer-meshkov instability with reshock. Phys Rev Fluids 4: 104609. https://doi.org/10.1103/PhysRevFluids.4.104609 doi: 10.1103/PhysRevFluids.4.104609
![]() |
[148] |
Livescu D, Ryu J (2016) Vorticity dynamics after the shock–turbulence interaction. Shock Waves 26: 241–251. https://doi.org/10.1007/s00193-015-0580-5 doi: 10.1007/s00193-015-0580-5
![]() |
[149] |
Aslangil D, Livescu D, Banerjee A (2020) Variable-density buoyancy-driven turbulence with asymmetric initial density distribution. Physica D 406: 132444. https://doi.org/10.1016/j.physd.2020.132444 doi: 10.1016/j.physd.2020.132444
![]() |
[150] |
Tian Y, Jaberi FA, Livescu D (2019) Density effects on post-shock turbulence structure and dynamics. J Fluid Mech 880: 935–968. https://doi.org/10.1017/jfm.2019.707 doi: 10.1017/jfm.2019.707
![]() |
[151] |
Orlicz G, Balasubramanian S, Prestridge K (2013)Incident shock mach number effects on richtmyer-meshkov mixing in a heavy gas layer. Phys Fluids 25: 114101. https://doi.org/10.1063/1.4827435 doi: 10.1063/1.4827435
![]() |
[152] |
Tomkins C, Kumar S, Orlicz G, et al. (2008) An experimental investigation of mixing mechanisms in shock-accelerated flow. J Fluid Mech 611: 131–150. https://doi.org/10.1017/S0022112008002723 doi: 10.1017/S0022112008002723
![]() |
[153] |
Marble FE, Zukoski EE, Jacobs JW, et al. (1990) Shock enhancement and control of hypersonic mixing and combustion. AIAA Pap 1981: 1990. https://doi.org/10.2514/6.1990-1981 doi: 10.2514/6.1990-1981
![]() |
[154] |
Ottino JM (1990) Mixing, chaotic advection, and turbulence. Annu Rev Fluid Mech 22: 207–254. https://doi.org/10.1146/annurev.fl.22.010190.001231 doi: 10.1146/annurev.fl.22.010190.001231
![]() |
[155] |
Sreenivasan KR (2019) Turbulent mixing: A perspective. P Natl Acad Sci 116: 18 175–18 183. https://doi.org/10.1073/pnas.1800463115 doi: 10.1073/pnas.1800463115
![]() |
[156] |
Ranjan D, Oakley J, Bonazza R (2011) Shock-bubble interactions. Annu Rev Fluid Mech 43: 117–140. https://doi.org/10.1146/annurev-fluid-122109-160744 doi: 10.1146/annurev-fluid-122109-160744
![]() |
[157] |
Mulla IA, Hardalupas Y (2022) Measurement of instantaneous fully 3d scalar dissipation rate in a turbulent swirling flow. Exp Fluids 63: 173. https://doi.org/10.1007/s00348-022-03518-2 doi: 10.1007/s00348-022-03518-2
![]() |
1. | HAN Xu, YU Bin, LIU Hong, Effect of initial diffusion on mixing enhancement in shock bubble interaction, 2024, 54, 1674-7275, 104707, 10.1360/SSPMA-2024-0124 |
References | Cases | Dimensionaless mixing time | Mixing status |
Marble et al. [30] | Air−He(Ma=1.1) | c0t/D(Ma2−1)<2.86 | well-mixed |
Vorobieff et al. [134] | Air−SF6(Ma=1.2) | u′1t/λ≈6.6 | well-mixed |
Niederhaus et al. [135] | Air−He(Ma=1.22−3) | u′1t/R=10 | well-mixed |
N2−Ar(Ma=1.33−3.38) | u′1t/R=15 | partially-mixed | |
Air−SF6(Ma=1.2−3) | u′1t/R=12 | well-mixed | |
Oggian et al.[137] | At = 0.5(Ma=1.84) | At+Δut/λmin≈250 | well-mixed |
Liu et al. [138] | Air−He(Ma=1.22−4) | τ(tVDs)∼O(1) | well-mixed |
References | Cases | Dimensionaless mixing time | Mixing status |
Marble et al. [30] | Air−He(Ma=1.1) | c0t/D(Ma2−1)<2.86 | well-mixed |
Vorobieff et al. [134] | Air−SF6(Ma=1.2) | u′1t/λ≈6.6 | well-mixed |
Niederhaus et al. [135] | Air−He(Ma=1.22−3) | u′1t/R=10 | well-mixed |
N2−Ar(Ma=1.33−3.38) | u′1t/R=15 | partially-mixed | |
Air−SF6(Ma=1.2−3) | u′1t/R=12 | well-mixed | |
Oggian et al.[137] | At = 0.5(Ma=1.84) | At+Δut/λmin≈250 | well-mixed |
Liu et al. [138] | Air−He(Ma=1.22−4) | τ(tVDs)∼O(1) | well-mixed |