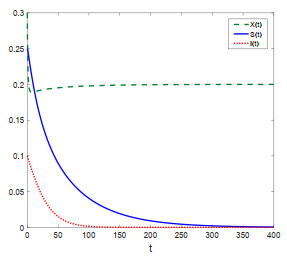
The aim of the research was to study the effect of inoculum type and fermentation time on microbial growth patterns (yeast, fungi and bacteria), β-glucan formation and antioxidant activity during soybean fermentation into tempe. The research was conducted using factorial Completely Randomized Block Design with 3 replications. The first factor was the types of inoculum: commercial inoculum of tempe, Raprima (3%), a single inoculum of S. cerevisiae (3%), a single inoculum of R. oligosporus (3%), and mixed inoculum of 1.5% S. cerevisiae and 1.5% R. oligosporus. The second factor was the length of fermentation which consisted of 0, 8, 16, 24, 32 and 40 hours at room temperature. Regarding the number of fungi, yeasts and bacteria, the observational data were presented descriptively in the form of graphs, while for the data from the analysis of β-glucan and antioxidant activity, the data obtained were analyzed for variance with analysis of variance (ANOVA) and then analyzed further by the Least Significant Difference (LSD) at the 5% significance level. The results showed that the type of inoculum and duration of fermentation had an effect on increasing the growth of fungi, yeasts and bacteria, as well as increasing β-glucan content and the antioxidant activity of tempe. Yeast growth had a more dominant effect on increasing β-glucan content and antioxidant activity compared to fungi and bacteria. Tempe inoculated with a mixed inoculum of 1.5% R. oligosporus + 1.5% S. cerevisiae, resulted in the highest β-glucan content of 0.58% and the highest antioxidant activity at 82.42%. In conclusion, a mixed inoculum of 1.5% R. oligosporus + 1.5% S. cerevisiae with 36−40 hours of fermentation produced tempe with the highest β-glucan content and antioxidant activity. Therefore, the β-glucan content causes tempe to have better potential health benefits than tempe without the addition of S. cerevisiae.
Citation: Samsul Rizal, Maria Erna Kustyawati, Murhadi, Udin Hasanudin, Subeki. The effect of inoculum types on microbial growth, β-glucan formation and antioxidant activity during tempe fermentation[J]. AIMS Agriculture and Food, 2022, 7(2): 370-386. doi: 10.3934/agrfood.2022024
[1] | Kawkab Al Amri, Qamar J. A Khan, David Greenhalgh . Combined impact of fear and Allee effect in predator-prey interaction models on their growth. Mathematical Biosciences and Engineering, 2024, 21(10): 7211-7252. doi: 10.3934/mbe.2024319 |
[2] | Saheb Pal, Nikhil Pal, Sudip Samanta, Joydev Chattopadhyay . Fear effect in prey and hunting cooperation among predators in a Leslie-Gower model. Mathematical Biosciences and Engineering, 2019, 16(5): 5146-5179. doi: 10.3934/mbe.2019258 |
[3] | Dirk Stiefs, Ezio Venturino, Ulrike Feudel . Evidence of chaos in eco-epidemic models. Mathematical Biosciences and Engineering, 2009, 6(4): 855-871. doi: 10.3934/mbe.2009.6.855 |
[4] | Yuhong Huo, Gourav Mandal, Lakshmi Narayan Guin, Santabrata Chakravarty, Renji Han . Allee effect-driven complexity in a spatiotemporal predator-prey system with fear factor. Mathematical Biosciences and Engineering, 2023, 20(10): 18820-18860. doi: 10.3934/mbe.2023834 |
[5] | Yuanfu Shao . Bifurcations of a delayed predator-prey system with fear, refuge for prey and additional food for predator. Mathematical Biosciences and Engineering, 2023, 20(4): 7429-7452. doi: 10.3934/mbe.2023322 |
[6] | Hongqiuxue Wu, Zhong Li, Mengxin He . Dynamic analysis of a Leslie-Gower predator-prey model with the fear effect and nonlinear harvesting. Mathematical Biosciences and Engineering, 2023, 20(10): 18592-18629. doi: 10.3934/mbe.2023825 |
[7] | Ranjit Kumar Upadhyay, Swati Mishra . Population dynamic consequences of fearful prey in a spatiotemporal predator-prey system. Mathematical Biosciences and Engineering, 2019, 16(1): 338-372. doi: 10.3934/mbe.2019017 |
[8] | Shunyi Li . Hopf bifurcation, stability switches and chaos in a prey-predator system with three stage structure and two time delays. Mathematical Biosciences and Engineering, 2019, 16(6): 6934-6961. doi: 10.3934/mbe.2019348 |
[9] | Rongjie Yu, Hengguo Yu, Chuanjun Dai, Zengling Ma, Qi Wang, Min Zhao . Bifurcation analysis of Leslie-Gower predator-prey system with harvesting and fear effect. Mathematical Biosciences and Engineering, 2023, 20(10): 18267-18300. doi: 10.3934/mbe.2023812 |
[10] | Wanxiao Xu, Ping Jiang, Hongying Shu, Shanshan Tong . Modeling the fear effect in the predator-prey dynamics with an age structure in the predators. Mathematical Biosciences and Engineering, 2023, 20(7): 12625-12648. doi: 10.3934/mbe.2023562 |
The aim of the research was to study the effect of inoculum type and fermentation time on microbial growth patterns (yeast, fungi and bacteria), β-glucan formation and antioxidant activity during soybean fermentation into tempe. The research was conducted using factorial Completely Randomized Block Design with 3 replications. The first factor was the types of inoculum: commercial inoculum of tempe, Raprima (3%), a single inoculum of S. cerevisiae (3%), a single inoculum of R. oligosporus (3%), and mixed inoculum of 1.5% S. cerevisiae and 1.5% R. oligosporus. The second factor was the length of fermentation which consisted of 0, 8, 16, 24, 32 and 40 hours at room temperature. Regarding the number of fungi, yeasts and bacteria, the observational data were presented descriptively in the form of graphs, while for the data from the analysis of β-glucan and antioxidant activity, the data obtained were analyzed for variance with analysis of variance (ANOVA) and then analyzed further by the Least Significant Difference (LSD) at the 5% significance level. The results showed that the type of inoculum and duration of fermentation had an effect on increasing the growth of fungi, yeasts and bacteria, as well as increasing β-glucan content and the antioxidant activity of tempe. Yeast growth had a more dominant effect on increasing β-glucan content and antioxidant activity compared to fungi and bacteria. Tempe inoculated with a mixed inoculum of 1.5% R. oligosporus + 1.5% S. cerevisiae, resulted in the highest β-glucan content of 0.58% and the highest antioxidant activity at 82.42%. In conclusion, a mixed inoculum of 1.5% R. oligosporus + 1.5% S. cerevisiae with 36−40 hours of fermentation produced tempe with the highest β-glucan content and antioxidant activity. Therefore, the β-glucan content causes tempe to have better potential health benefits than tempe without the addition of S. cerevisiae.
The effect of disease on eco-epidemiology system is a significant topic from both mathematical and ecological perspectives. The disease factor usually leads to a more complex and diverting dynamics than those in the disease-free system [1,2]. Within the interactions between predator and prey, the disease could only spread in prey or predator population, also could spread between prey and predator [3,4,5]. Birds (particularly pelicans) infect vibrio and die by preying on vibrio-infected fish (particularly tilapia) at the Salton Sea in the desert of Southern California [3], which is an example of disease spreads amongst the prey. For the disease in predator, taking fox rabies as an example, foxes (Vulpis) infect rabies and transmit to other foxes or their prey rabbits by biting in Europe and North America [6]. More relevant examples could be found in [7]. From the mathematical epidemiology point of view, one needs much more attention in the dynamics of infected predator to observe whether the presence of the prey allows the survival of a part of the predator population [8].
A variety of diseased predator models have been proposed to study the complex interaction between prey and predator with infected diseases [2,9,10] and the reference therein. Most common epidemic model applied in predator-prey interactions is the SI-type, i.e., the predator population Y(t) is divided into two sub-classes, namely susceptible predator S(t) and infected predator I(t), respectively [10,11,12]. The infection term could be mass-action term (bilinear form) βSI or saturation form βSIS+I [4]. The infected predators usually behave differently with susceptible ones, and suffer an additional death rate. In a epidemic model, the global dynamics are usually determined by the basic reproduction number R0, i.e., the disease will dies out in the population when R0≤1, and the disease will persist in the population when R0>1. However, the basic reproduction number is no longer a threshold parameter determining the global dynamics in diseased predator models, on the contrary, the dynamics are relatively comprehensive and unexpected.
Predation is the key force in a prey-predator interaction, which could affect the size of prey population by direct hunting [9,13,14,15], and elicit a variety of anti-predator responses [16,17,18]. Consequently, prey tends to alter behaviors in a certain extent, such as change of habitat, foraging activity, vigilance, physiological changes. This anti-predator behaviors accelerate the extinction, evolution and development of prey population in the long run. Under the risk of predation, prey may reduce its foraging activity in order to stay alert, leading to starvation which impacts on population growth [19,20]. Therefore, an immediately result of anti-predator behaviors is the reduction of prey growth rate, which is the cost for prey in prey defense [19,21,22,23,24,25,26].
Consider a simple birth-death process of the prey X(t) with the cost of anti-predator behaviors [27]:
dXdt=[F(k,Y)a]X−dX, |
where X,Y represent the density of the prey and predator, respectively. a is the birth rate of prey, d is the natural death rate of prey. F(k,Y) accounts for the cost of anti-predator defence due to fear, the parameter k reflects the level of fear which drives anti-predator behaviors of prey. The fear factor F(k,Y) has some specific assumptions under the ecological motions, for details see [20,27].
To derive a simple diseased predator model incorporating the anti-predator defence due to fear, we adopted the following fear effect term F(k,Y):
F(k,Y)=11+kY=11+k(S+I). |
Based on the results in [4,9,11], we can obtain the eco-epidemiological system with cost of anti-predator behaviors as following system of nonlinear differential equations:
{dXdt=rX1+k(S+I)−rX2K−aXS1+bX,dSdt=eaXS1+bX−d1S−βSI,dIdt=βSI−d2I, | (1.1) |
where X,S,I represent the density of prey, susceptible predator and infected predator at time t, respectively. r is the intrinsic growth rate of prey, K is the carrying capacity of the prey, a is the predation coefficient, b is the predators handling time of a prey, e is the biomass conversion constant, β is the transmissibility coefficient. d1 and d2 are the mortality rates of the susceptible predator and infected predator, and naturally d1<d2.
This paper consists of six sections. In the next section, we prove the positivity and boundedness of the solution of system (1.1). In Section 3, we provide the existence conditions of the equilibria of the model. We analyze the stability of equilibria and show the occurrence of Hopf bifurcation in Section 4. In Section 5, the correctness of the theoretical proof is illustrated by numerical simulation. Finally, we summarize our results with ecological interpretations in Section 6.
In view of the ecological significance, we only consider the solutions (X(t),S(t),I(t)) of system (1.1) on
R3+={(X(t),S(t),I(t))∈R3+:X(t)≥0,S(t)≥0,I(t)≥0}. |
Theorem 2.1. Each solution of system (1.1) with initial value (X(0),S(0),I(0))∈R3+ is positive and ultimately bounded.
Proof. Since the right-hand side of system (1.1) is completely continuous and locally Lipschitzian on R3+, the solution (X(t),S(t),I(t)) with initial condition (X(0),S(0),I(0))∈R3+ exists and is unique on R3+.
By integrating, it follows from system (1.1) that
X(t)=X(0)exp{t∫0(r1+k(S(τ)+I(τ))−rX(τ)K−aS(τ)1+bX(τ))dτ}≥0,S(t)=S(0)exp{t∫0(eaX(τ)1+bX(τ)−d1−βI(τ))dτ}≥0,I(t)=I(0)exp{t∫0(βS(τ)−d2)dτ}≥0. |
Hence, the solution (X(t),S(t),I(t)) of system (1.1) with the initial condition (X(0),S(0),I(0))∈R3+ remains positive.
From the first equation of (1.1), we can obtain
dXdt=rX1+k(S+I)−rX2K−aXS1+bX≤rX−rX2K=rX(1−XK), |
then
lim supt→∞X(t)≤K. |
Let N(t)=eX(t)+S(t)+I(t), we can get
dNdt=erX1+k(S+I)−erX2K−d1S−d2I≤erX−erX2K−d1S−d2I≤erX(1−XK)+ed1X−d1N≤eK(r+d1)24r−d1N, |
then
lim supt→∞N(t)≤eK(r+d1)24rd1. |
This ends the proof.
Remark 2.2. From Theorem 2.1, we know that all positive solutions of system (1.1) with initial conditions (X(0),S(0),I(0))∈R3+ are defined in the following positive bounded invariant:
Γ:={(X(t),S(t),I(t))∈R3+:0≤X(t)≤K,0≤eX(t)+S(t)+I(t)≤eK(r+d1)24rd1}. |
System (1.1) possesses at most three boundary equilibria:
(i) Trivial equilibrium: E0=(0,0,0);
(ii) Axial equilibrium: E1=(K,0,0);
(iii) Planar equilibrium: E2=(X2,S2,0) exists if ea−bd1>0 and K>d1ea−bd1, where
X2=d1ea−bd1,S2=−[K(ea−bd1)2+rd1ke]+√[K(ea−bd1)2−rd1ke]2+4K2kre(ea−bd1)32Kk(ea−bd1)2. | (3.1) |
For epidemic models, the most critical problem is the threshold property for the extinction and persistence of the disease, which is generally governed by the basic reproduction number R0. The basic reproduction number can be interpreted as the expected number of secondary cases produced, in a completely susceptible population, by a typical infected individual during its entire period of infectiousness. Following [28], we define the basic reproduction number for the predator population in the system (1.1) by
R0:=βS2d2, |
where S2 is given by (3.1).
Next, we mainly focus on the existence of positive equilibrium E3=(X3,S3,I3) of system (1.1). The coordinates X3,S3,I3 are positive solutions to the following system of equilibrium equations:
{r1+k(S3+I3)−rX3K−aS31+bX3=0,eaX31+bX3−d1−βI3=0,βS3−d2=0. |
Thus,
S3=d2β,I3=X3(ea−bd1)−d1(bX3+1)β, |
and X3 is the positive root of (3.2) in (X2,+∞):
Q(X)=m3X3+m2X2+m1X+m0=0, | (3.2) |
where
m3:=−bβr(k(ea−bd1)+b(kd2+β)),m2:=βr(Kb2β−k(ea−bd1)−2b(kd2+β)+bkd1),m1:=(−kd2a(ea−bd1)−b(akd22+aβd2−2β2r))K−βr(−kd1+kd2+β),m0:=−K(−ad2(kd1−kd2−β)−β2r). |
If ea−bd1>0 and r>ad2(β+k(d2−d1))β2, we have
m3<0,m0>0. |
By Descartes' rule of signs, system (1.1) has at least one positive equilibrium E3.
Hence, we have the following results on the existence of the positive equilibrium. It is worthy to note that the positive equilibrium is not unique due to the impact of fear effect k.
Theorem 3.1. If ea−bd1>0 and r>ad2(β+k(d2−d1))β2, then system (1.1) has at least one positive equilibrium E3=(X3,S3,I3), where S3=S2R0, I3=X3(ea−bd1)−d1(bX3+1)β and X3 is the positive root of (3.2) in (X2,+∞).
Regarding the local stability of trivial equilibrium E0 and axial equilibrium E1, we have the following results. The proof is standard, so we omit it here.
Theorem 4.1. For system (1.1),
(i) The trivial equilibrium E0=(0,0,0) is unstable;
(ii) If one of the following inequalities holds:
(ii-1) ea−bd1<0;
(ii-2) ea−bd1>0 and K<d1ea−bd1,
then the axial equilibrium E1=(K,0,0) is stable; while E1=(K,0,0) is unstable if ea−bd1>0 and K>d1ea−bd1.
Secondly, we will show the local stability of the planar equilibrium E2 of system (1.1). For convenience, set
r1:=d2(ea−bd1)βe,r2:=d2(ea−bd1)(ea+bd1)aβe2,K1:=ea+bd1b(ea−bd1),K2:=βerd1(ea−bd1)(βer−d2(ea−bd1)),k1:=Kb(ea−bd1)2(Kb(ea−bd1)−(ea+bd1))ae2r(ea+bd1),k2:=−β((ea−bd1)(d2(ea−bd1)−βer)K+βerd1)(Kd2(ea−bd1)2+βerd1)d2. | (4.1) |
Theorem 4.2. For system (1.1), assume that ea−bd1>0. If one of the following inequalities holds:
(Ⅰ) r≤r1 and one of the following inequalities holds:
(Ⅰ-1) d1ea−bd1<K≤K1;
(Ⅰ-2) K>K1 and k>k1;
(Ⅱ) r1<r<r2 and one of the following inequalities holds:
(Ⅱ-1) d1ea−bd1<K≤K1;
(Ⅱ-2) K1<K and k>max{k1,k2};
(Ⅲ) r>r2 and one of the following inequalities holds:
(Ⅲ-1) d1ea−bd1<K≤K2;
(Ⅲ-2) K2<K and k>max{k1,k2},
then equilibrium E2 is stable; otherwise, it is unstable.
Proof. The Jacobian matrix of system (1.1) at E2 is given by
J2=(a11a12a13a210−βS200βS2−d2), |
where
a11:=X2(−rK+abS2(1+bX2)2),a12:=−krX2(1+kS2)2−aX21+bX2,a13:=−krX2(1+kS2)2,a21:=eaS2(1+bX2)2. |
Hence, the characteristic equation of J2 is given as
f(λ)(λ−βS2+d2)=0, | (4.2) |
where
f(λ):=λ2−a11λ−a12a21. |
Clearly, one can see that J2 has three eigenvalues λ1, λ2 and λ3=βS2−d2. Since a12<0,a21>0, then −a12a21>0.
From (3.1), we can obtain
a11=X2(−rK+abS2(1+bX2)2)=X2Φ2Kka2e2, |
where
Φ:=ab√(K(ea−bd1)2−rd1ke)2+4K2kre(ea−bd1)3−ab(K(ea−bd1)2+rd1ke)−2rka2e2. |
Note that the sign of Φ depends on
˜Φ:=a2b2((K(ea−bd1)2−rd1ke)2+4K2kre(ea−bd1)3)−(ab(K(ae−bd1)2+rd1ke)+2rka2e2)2=4a2kerP(k), |
where
P(k):=−ae2r(ea+bd1)k+Kb(ea−bd1)2(Kb(ea−bd1)−(ea+bd1)). |
One can obtain that P(k) is decreasing with respect to k. If K≤ea+bd1b(ea−bd1) holds, we have P(0)≤0, which means that P(k)<0 for all k>0; if K>ea+bd1b(ea−bd1) and k>k1 hold, we can get P(k)<0. Therefore, when one of the following inequalities holds:
(i) K≤ea+bd1b(ea−bd1);
(ii) K>ea+bd1b(ea−bd1) and k>k1,
we can obtain a11<0, which implies that the real parts of λ1 and λ2 are all negative.
It follows from system (3.1) that
βS2−d2=−β[K(ea−bd1)2+rd1ke]+β√[K(ea−bd1)2−rd1ke]2+4K2kre(ea−bd1)32Kk(ea−bd1)2−d2=Θ2Kk(ea−bd1)2, |
where
Θ:=−K(ea−bd1)2(2kd2+β)−βekrd1+β√(K(ea−bd1)2−rd1ke)2+4K2kre(ea−bd1)3. |
Note that the sign of Θ depends on
˜Θ:=β2(K(ea−bd1)2−rd1ke)2+4β2K2kre(ea−bd1)3−(K(ea−bd1)2(2kd2+β)+βekrd1)2=−4Kk(ae−bd1)2[(Kd2(ea−bd1)2+βerd1)d2k+β((ea−bd1)(d2(ea−bd1)−βer)K+βerd1)]. |
Then if one of the following inequalities holds:
(Ⅰ) ea−bd1>0 and r≤d2(ea−bd1)βe;
(Ⅱ) ea−bd1>0, r>d2(ea−bd1)βe and one of the following inequalities:
(Ⅱ-1) K≤βerd1(ea−bd1)(βer−d2(ea−bd1));
(Ⅱ-2) K>βerd1(ea−bd1)(βer−d2(ea−bd1)) and k>k2:=−β((ea−bd1)(d2(ea−bd1)−βer)K+βerd1)(Kd2(ea−bd1)2+βerd1)d2,
we have λ3=βS2−d2<0.
Thus, we can arrive at the conclusion.
It should be pointed out that another way to state Theorem 4.2 is as follows.
Remark 4.3. For system (1.1), assume that ea−bd1>0 and R0<1. If one of the following inequalities:
(Ⅰ) d1ea−bd1<K≤K1;
(Ⅱ) K>K1 and k>k1
holds, then the planar equilibrium E2 is stable; otherwise, it is unstable.
Next, we will show the local stability of the positive equilibrium E3 of system (1.1).
The Jacobian matrix of system (1.1) at E3 is given by
J3=(b11b12b13b210−d20βI30), |
where
b11=X3(−rK+abS3(1+bX3)2),b12=−krX3(1+k(S3+I3))2−aX31+bX3<0,b13=−krX3(1+k(S3+I3))2<0,b21=eaS3(1+bX3)2>0. | (4.3) |
The characteristic equation of J3 is given as
λ3+A1λ2+A2λ+A3=0, | (4.4) |
where
A1=−b11,A2=βd2I3−b12b21,A3=−b11βd2I3−b13b21βI3. | (4.5) |
Note that if A1>0 holds, then b11<0, which means that A3>0. According to Routh-Hurwitz criterion, the positive equilibrium E3 is locally asymptotically stable when A1>0 and A1A2−A3>0.
Therefore, we can establish the following statement.
Theorem 4.4. Assume that ea−bd1>0 and r>ad2(β+k(d2−d1))β2 hold. The positive equilibrium E3 of system (1.1) is locally asymptotically stable if A1>0 and A1A2−A3>0, where Ai,i=1,2,3 is defined as in (4.5). Otherwise, it is unstable.
Remark 4.5. Theorem 4.4 gives a sufficient condition about the stability of the positive equilibrium E3 for system (1.1). However, the complexity of model (1.1) leads to the failure to theoretically demonstrate how the fear factor affects the stability of the positive equilibrium. This will be discussed later through numerical simulations.
In this subsection, we take k as the bifurcation parameter. The characteristic equation of system (1.1) at E3 is (4.4), and Ai(k),i=1,2,3 are defined as (4.5).
Theorem 4.6. Hopf bifurcation near the positive equilibrium E3 for system (1.1) occurs whenever the critical parameter k attains the value k=kh in the domain:
Ω={kh∈R+:Δ(kh):=[A1(k)A2(k)−A3(k)]|k=kh=0withA2(kh)>0,[dΔ(k)dk]|k=kh≠0}. |
Proof. If k=kh, the characteristic Eq (4.4) equals
λ3+A1(kh)λ2+A2(kh)λ+A3(kh)=0, | (4.6) |
then (4.6) can be factorized as
(λ2+A2(kh))(λ+A1(kh))=0. | (4.7) |
Clearly, (4.7) has three roots: λ1=i√A2(kh), λ2=−i√A2(kh) and λ3=−A1(kh). The roots are of the form λ1=p1(k)+ip2(k), λ2=p1(k)−ip2(k) and λ3=−p3(k), where pi(k)(i=1,2,3) are real numbers.
From the characteristic Eq (4.4), we can get
dλdk=−λ2A′1+λA′2+A′33λ2+2A1λ+A2, | (4.8) |
where ′=ddk. Substituting λ=i√A2 into (4.8), we obtain that
A′3−A2A′1+iA′2√A22(A2−iA1√A2)=−dΔ(k)dk2(A21+A2)+i[√A2A′22A2−A1√A2dΔ(k)dk2A2(A21+A2)], |
which implies that
[dRe(λ)dk]|k=kh=−dΔ(k)dk2(A21+A2)|k=kh. |
By using monotonicity condition in the real part of the complex root dRe(λ)dk|k=kh≠0, the transversality condition dΔ(k)dk|k=kh≠0 can be obtained to ensure the existence of Hopf bifurcation.
Results from numerical simulations are provided in this section to demonstrate our theoretical results. As we will show, the observations shed lights on the impact of fear factor. We choose the parameters of system (1.1) as follows:
r=0.8,a=0.2,b=0.1,e=0.9,d1=0.05,β=0.1,d2=0.053. | (5.1) |
Then we have
ea−bd1=0.175>0,d1ea−bd1=0.286,r1=d2(ea−bd1)βe=0.103,r2=d2(ea−bd1)(ea+bd1)aβe2=0.106,K1=ea+bd1b(ea−bd1)=10.571,K2=βerd1(ea−bd1)(βer−d2(ea−bd1))=0.328. |
Example 5.1 (The stability of E1).
We adopt K=0.2,k=0.01, then system (1.1) has trivial equilibrium E0=(0,0,0) and axial equilibrium E1=(0.2,0,0). In this case, one can know that the conditions of Theorem 4.1 are satisfied, which means that E1 is locally asymptotically stable. The numerical results are shown in Figure 1.
Example 5.2 (The impacts of K and k on the stability of E2).
In this example, we will choose three values of carrying capacity K for numerical experiments. We conclude that the carrying capacity and fear effect are other key factors related to the extinction of infected predators, in addition to the basic reproduction number R0.
Firstly, we take K=0.3<K2, then we have k=0.1 which yields that R0=0.263<1. In this case, system (1.1) has trivial equilibrium E0=(0,0,0), axial equilibrium E1=(0.3,0,0), and planar equilibrium E2=(0.286,0.139,0). By Theorem 4.5, E2 is locally asymptotically stable, see Figure 2(a). Thus, when the carrying capacity of the prey K is small, no matter what the level of fear k is, the small size of prey population will lead to the extinction of infected predators.
Secondly, for comparison, we take K2<K=15, then
k1=Kb(ea−bd1)2(Kb(ea−bd1)−(ea+bd1))ae2r(ea+bd1)=0.149,k2=−β((ea−bd1)(d2(ea−bd1)−βer)K+βerd1)(Kd2(ea−bd1)2+βerd1)d2=10.873. |
Choosing k=0.1<max{k1,k2} which yields R0=5.792>1, then we have
A1=0.25791>0,A1A2−A3=0.00523>0. |
In this case, system (1.1) has trivial equilibrium E0=(0,0,0), axial equilibrium E1=(15,0,0), planar equilibrium E2=(0.286,3.070,0), and positive equilibrium E3=(7.351,0.530,7.125). By Theorem 4.5, E2=(0.286,3.070,0) is unstable. On the contrary, E3=(7.351,0.530,7.125) is locally asymptotically stable. The numerical simulation is shown in Figure 2(b).
Finally, we take K2<K=60, then we have
k1=Kb(ea−bd1)2(Kb(ea−bd1)−(ea+bd1))ae2r(ea+bd1)=6.629,k2=−β((ea−bd1)(d2(ea−bd1)−βer)K+βerd1)(Kd2(ea−bd1)2+βerd1)d2=12.238. |
Choosing k=30>max{k1,k2} which yields that R0=0.649<1, system (1.1) has trivial equilibrium E0=(0,0,0), axial equilibrium E1=(60,0,0), and planar equilibrium E2=(0.286,0.344,0). By Theorem 4.5, E2 is locally asymptotically stable, see Figure 2(c). Thus, when the carrying capacity of the prey K is relatively large, a high level of fear k will lead to the extinction of infected predators.
Example 5.3 (The impact of k on the stability of E3). We adopt K=60, then we have kh=0.26. In the next, we will choose three values of k, corresponding to the local stability of E3, Hopf bifurcation, and instability of E3, to illustrate the impact of fear factor on the population dynamics.
Firstly, we take k=0.1<kh which yields that R0=5.881>1, then system (1.1) has trivial equilibrium E0=(0,0,0), axial equilibrium E1=(60,0,0), planar equilibrium E2=(0.286,3.117,0) and a unique positive equilibrium E3=(24.047,0.530,12.213). In this case, we obtain that
A1=0.29863>0,A1A2−A3=0.00065>0, |
which means that E3 is local asymptotically stable. The numerical results are shown in Figure 3.
Secondly, we take k=0.26=kh which yields that R0=4.682>1, then system (1.1) has trivial equilibrium E0=(0,0,0), axial equilibrium E1=(60,0,0), planar equilibrium E2=(0.286,2.481,0) and a unique positive equilibrium E3=(12.975,0.530,9.665). In this case, we obtain that
A1=0.14694>0,A1A2−A3=0, |
which means that system (1.1) undergoes a Hopf bifurcation and there is a limit cycle around E3. The numerical results and the bifurcation diagrams of system (1.1) with respect to the parameter k are shown in Figures 4 and 5, respectively. Comparing Figures 3 and 4(a), one can see that there are two different implications induced by the fear factor k: the first is that the stability of E3 converts from stable into unstable, and the second is the decrease of values of X3 and I3 of E3.
Finally, we take k=0.5>kh which yields that R0=3.821>1, then system (1.1) has trivial equilibrium E0=(0,0,0), axial equilibrium E1=(60,0,0), planar equilibrium E2=(0.286,2.025,0) and a unique positive equilibrium E3=(7.685,0.530,7.322). In this case, we can obtain that
A1=0.07642>0,A1A2−A3=−0.00051<0, |
which means that E3 is unstable. The numerical results are shown in Figure 6. One can find that the difference between Figures 4 and 6 is the decrease of values of E3 from (12.975,0.530,9.665) to (7.685,0.530,7.322), which is induced by the impact of the feat factor.
In this paper, we explored a predator-prey model that incorporates infectious disease in predator population and the cost of anti-predator behaviors. The cost of anti-predator behaviors is measured by a fear effect k leading to an reduction of prey's birth rate. We fulfill a complete stability analysis of equilibria for system (1.1) and show that the system (1.1) exhibits the Hopf bifurcation. Biologically, we focus on the impact of fear effect on the population dynamics. As we will see later, the cost of a high level of fear effect is disastrously. The main findings are summarized in the following.
1) Small size of prey population leads to the extinction of infected predators.
If the carrying capacity K is relatively small, the planar equilibrium E2 is stable, see Figure 2(a). Thus, no matter what the level of fear effect k is, a small size of prey population will lead to the extinction of infected predators.
2) Low level of the fear effect doesn't impact on the population dynamics.
If the level of fear effect k<kh, the positive (coexistence) equilibrium E3 is stable, see Figure 3. Hence, we conclude that a small fear effect k is not the key disturbance and does not change the coexistence dynamics of system (1.1). However, the densities of the prey and infected predator gradually decrease as k increasing.
3) Certain medium level of the fear effect lead to periodic oscillation.
If k=kh, the fear effect can destabilize the stability of E3 and will benefit the occurrence of periodic oscillation. In other words, system (1.1)undergoes a limit cycle, see Figures 4 and 5.
4) High level of the fear effect leads to complex dynamics and the infected predator can go to extinction.
If k>kh, E3 is unstable, see Figure 6. Therefore, a large fear effect k persistently and dramatically influence the population dynamics of prey and predator. Furthermore, if the level of the fear factor k is extremely high, the planar equilibrium E2 is stable, see Figure 2(c). The prey will respond to perceived predation risk and show a variety of anti-predator responses, dramatically decreasing the recruitment of susceptible predator, which will lead to an extinction of infected predator.
The authors would like to thank the editor and the referees for their helpful comments. This research was supported by the National Natural Science Foundation of China (Grant No. 12171192, 12031020 and 12071173), the Science and Technology Research Projects of the Education Office of Jilin Province, China (JJKH20211033KJ), the Technology Development Program of Jilin Province, China (20210508024RQ) and Huaian Key Laboratory for Infectious Diseases Control and Prevention, China (HAP201704).
The authors declare that there are no conflicts of interest regarding the publication of this paper.
[1] |
Bintari SH, Anisa DP, Veronika EJ, et al. (2008) Effect inoculation of Micrococcus luteus to growth of mold and content isoflavone at tempe processing. Biosaintifika: J Bio Bio Edu 1: 1–8. https://doi.org/10.15294/biosaintifika.v1i1.43 doi: 10.15294/biosaintifika.v1i1.43
![]() |
[2] | Roubous HPJ, Nout MJR (2011) Anti-diarrhoeal aspect of fermented soya beans, In: El-Shemy H. (Eds), Soybean and Health, London: Intech Open, 383–406. |
[3] |
Kustyawati ME (2009) Study on the role of yeast in tempe production. Agritech 29: 64–70. https://doi.org/10.22146/agritech.9765 doi: 10.22146/agritech.9765
![]() |
[4] | Nurdini AL, Nuraida L, Suwanto A, et al. (2015) Microbial growth dynamics during tempe fermentation in two different home industries. Food Res Int 22: 1668–1674. |
[5] | Astawan M, Wresdiyati T, Widowati S, et al. (2013) Phsyco-chemical characteristics and functional properties of tempeh made from different soybeans varieties. Pangan 22: 241–252. |
[6] |
Mambang DEP, Rosidah, Suryanto D (2014) Antibacterial activity of tempe extracts on Bacillus subtilis and Staphylococcus aureus. J Teknol dan Industri Pangan 25: 115–118. https://doi.org/10.6066/jtip.2014.25.1.115 doi: 10.6066/jtip.2014.25.1.115
![]() |
[7] |
Virgianti DP (2015) Uji antagonis jamur tempe (Rhizopus sp.) terhadap bakteri patogen enterik. Majalah Ilmiah Biologi Biosfera: A Sci J 32: 162–168. https://doi.org/10.20884/1.mib.2015.32.3.339 doi: 10.20884/1.mib.2015.32.3.339
![]() |
[8] |
Kuligowski M, Kuligowska IJ, Nowak J (2013) Evaluation of bean and soy tempeh influence on intestinal bacteria and estimation of antibacterial properties of bean tempeh. Pol J Microbiol 62: 189–194. https://doi.org/10.33073/pjm-2013-024 doi: 10.33073/pjm-2013-024
![]() |
[9] |
Pengkumsri N, Sivamaruthi BS, Sirilun S, et al. (2017) Extraction of β-glukan from Saccharomyces cerevisiae: Comparison of different extraction methods and in vivo assessment of immunomodulatory effect in mice. Food Sci Tech 37: 124–130. https://doi.org/10.1590/1678-457X.10716 doi: 10.1590/1678-457X.10716
![]() |
[10] | Hetland G, Johnson E, Eide DM, et al. (2013) Antimicrobial effects of β-glucans and pectin and of the Agaricus blazei-based mushroom extract, AndoSanTM. Examples of mouse models for pneumococcal, fecal bacterial, and mycobacterial infections. Microb Pathog Strategies Combating Them: Sci, Technol Edu 1: 889–898. |
[11] |
Rizal S, Kustyawati ME (2019) Characteristics of sensory and Beta-glucan content of soybean tempe with addition of Saccharomyces cerevisiae. J Teknol Pertanian 20: 127–138. https://doi.org/10.21776/ub.jtp.2019.020.02.6 doi: 10.21776/ub.jtp.2019.020.02.6
![]() |
[12] |
Rizal S, Kustyawati ME, Murhadi, et al. (2020) Growth optimization of Saccharomyces cerevisiae and Rhizopus oligosporus during fermentation to produce tempe with high β-glucan content. Biodiversitas J Biol Diversity 21: 2667–2673. https://doi.org/10.13057/biodiv/d210639 doi: 10.13057/biodiv/d210639
![]() |
[13] |
Rizal S, Kustyawati ME, Murhadi, et al. (2021) The growth of yeast and fungi, the formation of β-glucan, and the antibacterial activities during soybean fermentation in producing tempe. Int J Food Sci. 6: 1–8. https://doi.org/10.1155/2021/6676042 doi: 10.1155/2021/6676042
![]() |
[14] |
Rizal S, Kustyawati ME, Suharyono, et al. (2022) Changes of nutritional composition of tempeh during fermentation with the addition of Saccharomyces cerevisiae. Biodiversitas J Biol Diversity 23: 1553–1559. https://doi.org/10.13057/biodiv/d230345 doi: 10.13057/biodiv/d230345
![]() |
[15] | Rizal S, Kustyawati ME, Murhadi, et al. (2018) Pengaruh konsentrasi Saccharomyces cerevisiae pada kandungan abu, protein, lemak dan β-glukan tempe. Prosiding Seminar Nasional Fakultas Pertanian UNS 2: 96–103. |
[16] |
Kustyawati ME, Subeki, Murhadi, et al. (2020) Vitamin B12 production in soybean fermentation for tempeh. AIMS Agric Food 5: 262–271. https://doi.org/10.3934/agrfood.2020.2.262 doi: 10.3934/agrfood.2020.2.262
![]() |
[17] |
Bavia ACF, Silva CE, Ferreira MP, et al. (2012) Chemical composition of tempeh from soybean cultivars specially developed for human consumption. Food Sci Tech 32: 613–620. https://doi.org/10.1590/S0101-20612012005000085 doi: 10.1590/S0101-20612012005000085
![]() |
[18] |
Kusmiati, Tamat SR, Jusuf E, et al. (2007) Beta-glucan production from two strains of Agrobacterium sp. in medium containing of molases and uracil combine. Biodiversivitas 8: 253–256. https://doi.org/10.13057/biodiv/d080210 doi: 10.13057/biodiv/d080210
![]() |
[19] |
Ismail J, Runtuwene MRJ, Fatimah F (2012) Total phenolic compounds and antioxidant activity of the seed and skin of pinang yaki (areca vestiaria giseke) fruits (Areca vetiaria Giseke). J Ilmiah Sains 12: 84–85. https://doi.org/10.35799/jis.12.2.2012.557 doi: 10.35799/jis.12.2.2012.557
![]() |
[20] |
Moreno MRF, Leisner JJ, Tee LK, et al. (2002) Microbial analysis of Malaysian tempeh, and characterization of two bacteriocins produced by isolates of Enterococcus faecium. J Appl Microbiol 92: 147–157. https://doi.org/10.1046/j.1365-2672.2002.01509.x doi: 10.1046/j.1365-2672.2002.01509.x
![]() |
[21] | Andayani P, Wardani AK, Murtini ES (2008) Isolation and identification of microorganism in sorghum tempeh (sorghum bicolor) and its potency for degrading starch and protein. J Teknol Pertanian 9: 95–105. |
[22] |
Feng XM, Larsen TO, Schnürer J (2007) Production of volatile compounds by Rhizopus oligosporus during soybean and barley tempeh fermentation. Int J Food Microbiol 113: 133–141. https://doi.org/10.1016/j.ijfoodmicro.2006.06.025 doi: 10.1016/j.ijfoodmicro.2006.06.025
![]() |
[23] |
Handoyo T, Morita N (2006) Structural and functional properties of fermented soybean (tempe) by using Rhizopus oligosporus. Int J Food Prop 9: 347–355. https://doi.org/10.1080/10942910500224746 doi: 10.1080/10942910500224746
![]() |
[24] |
Barus T, Suwanto A, Wahyudi A., et al. (2008) Role of bacteria in tempe bitter taste formation: Microbiological and molecular biological analysis based on 16S rRNA gene. Microbiol Indones 2: 17–21. https://doi.org/10.5454/mi.2.1.4 doi: 10.5454/mi.2.1.4
![]() |
[25] |
Keuth S, Bisping B (1994) Vitamin B12 production by Citrobacter freundiior and Klebsiella pneumoniae during tempe fermentation and proof of enterotoxin absence by PCR. Appl Environ Microb 60: 1495–1499. https://doi.org/10.1128/aem.60.5.1495-1499.1994 doi: 10.1128/aem.60.5.1495-1499.1994
![]() |
[26] |
Klus K, Borger-Papendorf G, Barz W (1993) Formation of 6, 7, 4'-trihydroxyisoflavone (factor 2) from soybean seed isoflavones by bacteria isolated from tempe. Phytochemistry 34: 979–981. https://doi.org/10.1016/s0031-9422(00)90697-6 doi: 10.1016/s0031-9422(00)90697-6
![]() |
[27] |
Sudiana ADG, Kuswendi H, Dewi VYK, et al. (2021) The potential of β-glucan from Saccharomyces cerevisiae cell wall as anticholesterol. J Anim Health Prod 9: 72–77. http://dx.doi.org/10.17582/journal.jahp/2021/9.1.72.77 doi: 10.17582/journal.jahp/2021/9.1.72.77
![]() |
[28] |
Siwicki AK, Patrycja S, Stanisl£ aw R, et al. (2015) Influence of β-glukan Leiber®Βeta-S on selected innate immunity parameters of European eel (Anguilla anguilla) in an intensive farming system. Cen Eur J Immunol 40: 5–10. https://doi.org/10.5114/ceji.2015.50826 doi: 10.5114/ceji.2015.50826
![]() |
[29] |
Febriyanti AE, Sari CN (2016) Effectiveness of growth media commercial yeast (Saccharomyces cerevisiae) for bioethanol fermentation from water Hyacinth (Eicchornia crassipes). Bioma 12: 43–48. https://doi.org/10.21009/Bioma12(2).6 doi: 10.21009/Bioma12(2).6
![]() |
[30] |
Zeković DB, Kwiatkowski S, Vrvić MM, et al. (2005) Natural and modified (1→3)-β-D glucans in health promotion and disease alleviation. Crit Rev Biotechnol 25: 205–230. https://doi.org/10.1080/07388550500376166 doi: 10.1080/07388550500376166
![]() |
[31] |
Kapteyn JC, Van Den Ende H, Klis FM (1999) The contribution of the O-glycosylated protein Pir2p/Hsp150 to the construction of the yeast cell wall in wild-type cells and β1, 6-glucan-deficient mutants. Mol Microbiol 31: 1835–1844. https://doi.org/10.1046/j.1365-2958.1999.01320.x doi: 10.1046/j.1365-2958.1999.01320.x
![]() |
[32] |
Appeldoorn NG, Sergeeva L, Vreugdenhil D, et al. (2002) In situ analysis of enzymes involved in sucrose to hexose-phosphate conversion during stolon-to-tuber transition of potato. Physiol Plantarum 115: 303–310. https://doi.org/10.1034/j.1399-3054.2002.1150218.x doi: 10.1034/j.1399-3054.2002.1150218.x
![]() |
[33] | Bacic A, Fincher GB, Stone BA (2009) Chemistry, biochemistry, and biology of 1-3 beta glucans and related polysaccharides, Burlington: Academic Press. |
[34] | Andriani Y (2007) Uji aktivitas antioksidan ekstrak β-glukan dari Saccharomyces cerevisiae. J Gradien 3: 226–230 |
[35] |
Nicolasi R (1999) Plasmalipid changes after supplementation with β-glucan fiber from yeast. Am J Clin Nutr 70: 208–212. https://doi.org/10.1093/ajcn.70.2.208 doi: 10.1093/ajcn.70.2.208
![]() |
[36] |
Ferdiansyah MK (2018) Lipid effect of dietary fiber of barley grains on lipid metabolism. J Ilmu Pangan dan Hasil Pertanian 2: 72–81. https://doi.org/10.26877/jiphp.v2i1.2441 doi: 10.26877/jiphp.v2i1.2441
![]() |
[37] |
Banobe CO, Kusumawati IGAW, Wiradnyani NK (2019) Value of macro nutrients and antioxidant activities soybean (glycine max l.) combination of winged bean (Psophocarpus Tetragonolobus). Pro Food 5: 486–495. https://doi.org/10.29303/profood.v5i2.111 doi: 10.29303/profood.v5i2.111
![]() |
[38] |
Widoyo S, Handajani S, Nandariyah (2015) The effect of fermentation time to crude fiber contents and antioxidant activities in several soybean varieties of tempeh. Biofarmasi 13: 59–65. https://doi.org/10.13057/biofar/f130203 doi: 10.13057/biofar/f130203
![]() |
[39] |
Ningsih TE, Siswanto, Winarsa R (2018) Activity of antoxidants edamame soybeans fermented result of mixed culture by Rhizopus oligosporus and Bacillus subtilis. Berkala Sainstek 6: 17–21. https://doi.org/10.19184/bst.v6i1.7556 doi: 10.19184/bst.v6i1.7556
![]() |
[40] |
Maryati Y, Susilowati A, Artanti N, et al. (2020) Effect of fermentation on antioxidant activities and betacyanin content of functional beverages from dragon fruit and beetroot. JBBI 7: 48–58. https://doi.org/10.29122/jbbi.v7i1.3732 doi: 10.29122/jbbi.v7i1.3732
![]() |
[41] |
Mojsov K (2010) Effects of different carbon sources on the synthesis of pectinase by Aspergillus niger in submerged and solid state fermentations. Appl Microbiol Biotechnol 39: 36–41. DOI:10.1007/BF00166845 doi: 10.1007/BF00166845
![]() |
1. | Chunmei Zhang, The effect of the fear factor on the dynamics of an eco-epidemiological system with standard incidence rate, 2024, 9, 24680427, 128, 10.1016/j.idm.2023.12.002 | |
2. | Hongqiuxue Wu, Zhong Li, Mengxin He, Dynamic analysis of a Leslie-Gower predator-prey model with the fear effect and nonlinear harvesting, 2023, 20, 1551-0018, 18592, 10.3934/mbe.2023825 | |
3. | Zhuoying Zhao, Xinhong Zhang, Unraveling the transmission mechanism of animal disease: Insight from a stochastic eco-epidemiological model driven by Lévy jumps, 2025, 191, 09600779, 115859, 10.1016/j.chaos.2024.115859 | |
4. | Huazhou Mo, Yuanfu Shao, Stability and bifurcation analysis of a delayed stage-structured predator–prey model with fear, additional food, and cooperative behavior in both species, 2025, 2025, 2731-4235, 10.1186/s13662-025-03879-y |