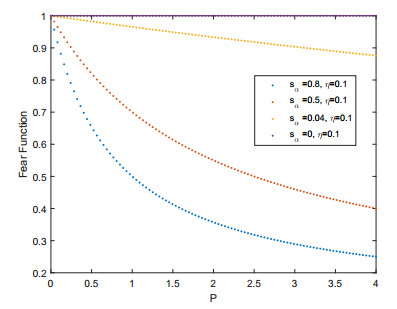
We considered predator-prey models which incorporated both an Allee effect and a new fear factor effect together, and where the predator predated the prey with a Holling type I functional response. We started off with a two-dimensional model where we found possible equilibria and examined their stabilities. By using the predator mortality rate as the bifurcation parameter, the model exhibited Hopf-bifurcation for the coexistence equilibrium. Furthermore, our numerical illustrations demonstrated the effect of fear and the Allee effect on the population densities, and we found that the level of fear had little impact on the long-term prey population level. The population of predators, however, declined as the fear intensity rose, indicating that the fear effect might result in a decline in the predator population. The dynamics of the delayed system were examined and Hopf-bifurcation was discussed. Finally, we looked at an eco-epidemiological model that took into account the same cost of fear and the Allee effect. In this model, the prey was afflicted with a disease. The prey was either susceptible or infected. Numerical simulations were carried out to show that as the Allee threshold rose, the uninfected prey and predator decreased, while the population of infected prey increased. When the Allee threshold hit a certain value, all populations became extinct. As fear intensity increased, the population of uninfected prey decreased, and beyond a certain level of fear, habituation prevented the uninfected prey from changing. After a certain level of fear, the predator population went extinct and, as a result, the only interaction left was between uninfected and infected prey which increased disease transmission, and so the infected prey increased. Hopf-bifurcation was studied by taking the time delay as the bifurcation parameter. We estimated the delay length to preserve stability.
Citation: Kawkab Al Amri, Qamar J. A Khan, David Greenhalgh. Combined impact of fear and Allee effect in predator-prey interaction models on their growth[J]. Mathematical Biosciences and Engineering, 2024, 21(10): 7211-7252. doi: 10.3934/mbe.2024319
[1] | Chunmei Zhang, Suli Liu, Jianhua Huang, Weiming Wang . Stability and Hopf bifurcation in an eco-epidemiological system with the cost of anti-predator behaviors. Mathematical Biosciences and Engineering, 2023, 20(5): 8146-8161. doi: 10.3934/mbe.2023354 |
[2] | Yuhong Huo, Gourav Mandal, Lakshmi Narayan Guin, Santabrata Chakravarty, Renji Han . Allee effect-driven complexity in a spatiotemporal predator-prey system with fear factor. Mathematical Biosciences and Engineering, 2023, 20(10): 18820-18860. doi: 10.3934/mbe.2023834 |
[3] | Fang Liu, Yanfei Du . Spatiotemporal dynamics of a diffusive predator-prey model with delay and Allee effect in predator. Mathematical Biosciences and Engineering, 2023, 20(11): 19372-19400. doi: 10.3934/mbe.2023857 |
[4] | Juan Ye, Yi Wang, Zhan Jin, Chuanjun Dai, Min Zhao . Dynamics of a predator-prey model with strong Allee effect and nonconstant mortality rate. Mathematical Biosciences and Engineering, 2022, 19(4): 3402-3426. doi: 10.3934/mbe.2022157 |
[5] | Moitri Sen, Malay Banerjee, Yasuhiro Takeuchi . Influence of Allee effect in prey populations on the dynamics of two-prey-one-predator model. Mathematical Biosciences and Engineering, 2018, 15(4): 883-904. doi: 10.3934/mbe.2018040 |
[6] | Rongjie Yu, Hengguo Yu, Chuanjun Dai, Zengling Ma, Qi Wang, Min Zhao . Bifurcation analysis of Leslie-Gower predator-prey system with harvesting and fear effect. Mathematical Biosciences and Engineering, 2023, 20(10): 18267-18300. doi: 10.3934/mbe.2023812 |
[7] | Saheb Pal, Nikhil Pal, Sudip Samanta, Joydev Chattopadhyay . Fear effect in prey and hunting cooperation among predators in a Leslie-Gower model. Mathematical Biosciences and Engineering, 2019, 16(5): 5146-5179. doi: 10.3934/mbe.2019258 |
[8] | Yuanfu Shao . Bifurcations of a delayed predator-prey system with fear, refuge for prey and additional food for predator. Mathematical Biosciences and Engineering, 2023, 20(4): 7429-7452. doi: 10.3934/mbe.2023322 |
[9] | Mengyun Xing, Mengxin He, Zhong Li . Dynamics of a modified Leslie-Gower predator-prey model with double Allee effects. Mathematical Biosciences and Engineering, 2024, 21(1): 792-831. doi: 10.3934/mbe.2024034 |
[10] | Zhenliang Zhu, Yuming Chen, Zhong Li, Fengde Chen . Dynamic behaviors of a Leslie-Gower model with strong Allee effect and fear effect in prey. Mathematical Biosciences and Engineering, 2023, 20(6): 10977-10999. doi: 10.3934/mbe.2023486 |
We considered predator-prey models which incorporated both an Allee effect and a new fear factor effect together, and where the predator predated the prey with a Holling type I functional response. We started off with a two-dimensional model where we found possible equilibria and examined their stabilities. By using the predator mortality rate as the bifurcation parameter, the model exhibited Hopf-bifurcation for the coexistence equilibrium. Furthermore, our numerical illustrations demonstrated the effect of fear and the Allee effect on the population densities, and we found that the level of fear had little impact on the long-term prey population level. The population of predators, however, declined as the fear intensity rose, indicating that the fear effect might result in a decline in the predator population. The dynamics of the delayed system were examined and Hopf-bifurcation was discussed. Finally, we looked at an eco-epidemiological model that took into account the same cost of fear and the Allee effect. In this model, the prey was afflicted with a disease. The prey was either susceptible or infected. Numerical simulations were carried out to show that as the Allee threshold rose, the uninfected prey and predator decreased, while the population of infected prey increased. When the Allee threshold hit a certain value, all populations became extinct. As fear intensity increased, the population of uninfected prey decreased, and beyond a certain level of fear, habituation prevented the uninfected prey from changing. After a certain level of fear, the predator population went extinct and, as a result, the only interaction left was between uninfected and infected prey which increased disease transmission, and so the infected prey increased. Hopf-bifurcation was studied by taking the time delay as the bifurcation parameter. We estimated the delay length to preserve stability.
Ecologists have observed the prey-predator interaction cycle with great interest. In past, they believed that the prey population declined due to direct killing by predators. In recent years, they realized through field studies that the mere presence of predators affects the growth of the prey population. The effect of stress in the reduction of the prey population is more powerful than direct killing. It has been pointed out that environmental stress affects the physiology and behavior of the prey to such an extent that it causes delayed mammal reproduction. Fear of the presence of predators, social life, competition for food, and similar factors are all causes that can lead to a reduction in mammal reproduction [1,2]. For small size prey such as sparrows and bank voles, the anti-predation response influence on the prey population is much higher [2,3,4].
Prey species where the physical prey size is small make coordinated feeding groups and avoid being detected by predators. They are vulnerable to a greater range of predators and cannot defend themselves or outrun predators. On the other hand, prey species where the physical prey size is large tend to form a large feeding group. They can defend themselves against predators and are more likely to depend upon group defense, group alertness, self-defense within a group, and speed to avoid being killed by a predator. All prey of either small or large physical size respond to predation risk and take a variety of anti-predation measures. They change habitat, practice vigilance whilst foraging, change their behavior whilst mating, and also undergo physiological changes [5,6]. It has been observed biologically, based on experimental studies, that mammals suppress breeding in response to strong predation pressure [4,7,8,9]. This has a long-term effect on the population of prey. The nonbreeding individuals have a better chance of avoiding predation than those who are breeding. Predation induced breeding suppression affects the prey-predator dynamics. Individuals who do not breed experience less predation because during pregnancy individuals are less active and can be captured more easily.
There have been quite a few previous attempts to incorporate the effect of fear of the predator into predator-prey systems. Kumar and Dubey [10] studied a mathematical model to investigate the fear effect and prey refuge in a predator-prey system with gestation time delay. Their analysis demonstrated that the fear effect in the prey population induces Hopf-bifurcation. The combined effects of fear and group defense in a fractional order prey-predator system are investigated by Das and Samanta [11]. Wang et al. [12] examined a predator-prey model by incorporating the cost of fear of the predator on prey. Their analysis found that a rise in fear stabilizes the system and a drop in fear leads to bistability. Wang and Zou [13] incorporated maturity after a delay and adaptive defense in the predator-prey fear model. They found that a higher predator population leads to robust anti-predator defense and higher predation risk implies a weak anti-predatory defense. Mondal et al. [14] studied a predator-prey system with a fear of prey and alternative food available for predators. According to their findings, alternative food to predators lowers the attack on prey and thus helps in the growth of both populations. Zhang et al. [15] and Wang et al. [16] incorporated prey refuge with fear effect. Duan et al. [17] investigated that maturation delay in a fear model leads to Hopf-bifurcation. The fear amongst the prey can stabilize as well as destabilize the population model as investigated by Pal et al. [18]. Pal et al. [19] observed that the fear effect induced by cooperative hunting destabilizes the system. Das and Samanta [20] examined a stochastic model where fear is in a prey population and an alternative food source is available for predators.
A lot of papers build on the previous work of Wang et al. [12] to introduce fear of the predator into the predator-prey system. If N represents the total population size and P the total predator population size, their fear function reduced the growth rate of the prey population by a multiplicative factor
11+kP, |
so in their model the growth rate of the prey population is given by
rN1+kP, |
where r represents the per capita birth rate of the prey population in the absence of the predator. This fear function was used by many authors [21,22,23,24,25]. However, it has the drawback that as P becomes very large (in other words, the predator population gets very large), the growth rate of the prey population tends to zero. This is not ecologically true since the prey will become habituated to coexist with the predator, and as the size of the predator population increases the growth rate will reduce, but not to zero.
Sarkar and Khajanchi [26] corrected this by introducing a fear function
f(α,η,P)=η+α(1−η)α+P, |
where α represents level of fear and η∈[0,1] represents the minimum cost of fear. They incorrectly state that as the level of fear, α, increases the fear function decreases. Although ecologically this should be true, with their fear function it is mathematically not true, as the fear function increases as the level of fear increases. We make a minor redefinition to the model of Sarkar and Khajanchi and define our fear function to be
f(α1,η,P)=η+1−η1+sα1P, |
where α1 is a parameter and sα1 is the level of fear. This fear function decreases as the level of fear increases. The fear function which we are using is more ecologically sound than the fear function of of Wang et al. [12], because after a certain level of fear, the prey becomes habituated to live with the predators. Thus, it reduces its reproduction to a certain extent but does not stop it completely.
The paper is structured as follows. In Section 2 we first outline the full eco-epidemiological model that we will be studying. The model combines an Allee effect and the modified fear function. There is a disease spreading amongst the prey, consequently there are three population classes, susceptible prey, infected prey, and predators. We also introduce the full eco-epidemiological model with a time delay corresponding to the delayed effect of predators consuming prey on predator reproduction. In Section 3 we will focus on and analyze the model with no disease present so that it is a predator-prey model with no disease amongst the prey. There is no time delay in the model discussed in this section. Section 4 analyzes the model with no disease present and a time delay. Section 5 will return to the full eco-epidemiological model with disease amongst the prey but without a time delay, and Section 6 analyzes this model with a time delay. This includes finding the length of the time delay to preserve the stability. In all of these models the reproduction of the prey is reduced due to our modified fear function as well as Allee effects. A final conclusion section summarizes and discusses the paper.
In this section we will outline the full eco-epidemiological model that we will be studying. All prey are born susceptible. The reproduction rate of the prey depends on the combined effects of fear of the predator together with the strong Allee effect. The function g(α1,η,y)=η+α1(1−η)α1+y represents the cost of fear of predators on the reproduction of prey [26]. η∈[0,1] represents the minimum cost of fear and α1 is a parameter for which 1α1=sα1 is the fear factor. In the case of a high predator population, the prey survives under the minimum fear η, and after a certain level of fear, the prey becomes habituated to living with the predators.
In the full eco-epidemiological model, we will study a predator-prey model where the prey is infected with some disease. We divide the total prey population into two classes called susceptible prey and infected prey. Let u1(t1),u2(t1), and v(t1) be the uninfected prey density, infected prey density, and predator density, respectively at time t1. We will formulate the model using the following assumptions:
(a) The uninfected prey population grows logistically when there is no predator.
(b) The susceptible prey population u1(t1) becomes infected when it comes in contact with infected prey u2(t1). This infection follows the nonlinear incidence rate. If we assume that the incidence rate is given by the law of mass action β1u1f(u2), then f(u2), will increase as u2 increases, which is not realistic. Lui et al. [27] investigated many reasons for taking a nonlinear incidence rate rather than a bilinear one.
(c) The infected prey population will remain infected and will not recover.
(d) Infected prey will not be able to reproduce because it has the disease. Only uninfected prey species have the capability to reproduce.
(e) The predator consumes infected prey more than susceptible prey because infected prey is easily catchable. For example, wolves attack moose more successfully when they are heavily infected by Echinococcus granulosus[28]. Hence, we assumed that the consumption rate p1 of infected prey is more than the consumption rate b1 of uninfected prey.
(f) The conversion rates b1 and p1 represent the reproduction of predators for capturing each uninfected and infected prey species, respectively.
(g) All the parameters used in the model are positive and u1(0)>0,u2(0)>0, and v(0)>0.
We will investigate the following ecological issues through our model:
(1) The effect of predator fear on the stability of the system.
(2) The level of infected prey.
Using the above assumptions, we will formulate a mathematical model as below:
du1dt1=ru1(1−u1+u2k)(u1−θ1)(η+1−η1+sα1v)−β1u1u2c1+δu2−a1u1v,du2dt1=β1u1u2c1+δu2−g1u2v−μ1u2,dvdt1=b1u1v+p1u2v−m1v, | (2.1) |
where u1 represents the susceptible prey population, u2 represents the infected prey population, v represents the infected predator population, k is the carrying capacity in the absence of infected prey, θ1 is the Allee threshold, η represents the minimum cost of fear, α1 is a parameter for which 1α1=sα1 is the fear factor, β1 represents the the disease transmission coefficient, c1 is the half saturation constant, δ is the predator preference rate of u2, and a1 represents the predation rate between susceptible prey and predator. g1 represents the predation rate between infected prey and predator, μ1 is the total per capita death rate of infected prey which includes both natural death and disease induced additional mortality, b1 is the consumption rate of uninfected prey, p1 represents the consumption rate of infected prey, and m1 represents the predator per capita natural mortality rate.
We additionally assume that
(h) u1(0)+u2(0)<k.
Note that if there are no infected prey, then u1(t), the total size of the susceptible prey population, will always be less than the carrying capacity. So, as normally the disease will be introduced by only a few infected prey, it makes sense to assume that u1(0)+u2(0)<k, which ensures that u1(t)+u2(t)<k for all time.
We consider in our study the following fear function to measure the cost of fear:
g(α,η,P)=(η+α(1−η)α+P)=(η+1−η1+1αP)=(η+1−η1+sαP), |
where 1α=sα is the nondimensional form of the fear factor. Figure 1 demonstrates that the fear function decreases as the level of fear sα increases. Also, as the predator population increases, the fear function declines. Because the fear factor multiplies with prey growth rate in model (3.1), the growth of the prey population will be low when the value of the fear function is low, that is, when the predator population is high.
To make the model simple, we non-dimensionalize model (2.1) by taking x=u1k, y=u2k, z=vk, t=t1kr, θ=θ1k, β=β1kr, c=c1k, a=a1r, g=g1r, μ=μ1kr, b=b1r, p=p1r, m=m1kr, and α=α1k as the parameter for which 1α=sα is the fear factor. So, model (2.1) in nondimensional form becomes:
dxdt=x(1−x−y)(x−θ)(η+1−η1+sαz)−βxyc+δy−axz,dydt=βxyc+δy−gyz−μy,dzdt=bxz+pyz−mz. | (2.2) |
In Eq (2.2), axz is the total rate at which susceptible prey are consumed by predators, and bxz is the total rate at which predators increase due to the consumption of susceptible prey. So a is the per capita rate at which a single predator attacks and consumes each susceptible prey, and b is the additional per capita rate at which each predator reproduces due to this consumption. As predators are typically much larger than prey, one predator consuming one prey is unlikely to give the predator sufficient energy to reproduce an entire predator. Therefore, b≤a, and similarly p≤g. Another way to see this is that the conversion rate of prey into predators will always be less than the rate at which predators attack prey because some of the intake energy gained by eating prey will go to waste since it will not all be converted into predator growth. Similar assumptions were made in [29] and [30].
We also introduce a time delay to make the model (2.1) more realistic. There is a time delay τ between predators consuming susceptible or infected prey and the susceptible or infected prey which has been consumed being converted into predators through reproduction. The population of predators will grow with interactions with uninfected prey and infected prey which take place at time t−τ. Hence, the population dynamics for the interaction of uninfected prey, infected prey, and predators can be described by the following system of differential equations:
du1dt=ru1(1−u1+u2k)(u1−θ1)(η+1−η1+sαv)−βu1u2c+δu2−au1v,du2dt=βu1u2a+δu2−gu2v−μu2,dvdt=bu1(t−τ)v(t−τ)+pu2(t−τ)v(t−τ)−mv. | (2.3) |
All the variables and parameters have the same meaning as given in (2.1). After non-dimensionalizing model (2.3) as we did in model (2.1), we get:
dxdt=x(1−x−y)(x−θ)(η+1−η1+sαz)−βxyc+δy−axz,dydt=βxyc+δy−gyz−μy,dzdt=bx(t−τ)z(t−τ)+py(t−τ)z(t−τ)−mz. | (2.4) |
We now proceed to analyze this model in four cases: the model with no disease present and no time delay, then the model with no disease present and a time delay, next the full eco-epidemiological model but with no time delay, and finally the full eco-epidemiological model with a time delay.
In this section we focus on the model with no disease present and no time delay. Putting y=0 in Eq (2.2), we obtain
dxdt=x(1−x)(x−θ)(η+1−η1+sαz)−axz,dzdt=bxz−mz. |
For the model with no disease present, we make the further transformations to simplify the model N=x, P=az, α′=αa, T=tba, r0=ba, and d=mab. Then, these equations become
dNdT=1r0[N(1−N)(N−θ)(η+α′(1−η)α′+P)−NP],dPdT=NP−dP. | (3.1) |
these are the equations which we shall work with in the remainder of this section. For notational simplicity, we write α for α′ and t for T.
Theorem 1. All the solutions (N(t),P(t)) of the system (3.1) with initial conditions N(0)≥0, P(0)≥0 are bounded for all t>0.
Proof. We define w=N+1r0P. The derivative of w along with (3.1) is
dwdt=dNdt+1r0dPdt,=1r0[N(1−N)(N−θ)(η+1−η1+sαP)−dP]. |
For any positive constant s, we have
dwdt+sw=1r0[N(1−N)(N−θ)(η+1−η1+sαP)−dP]+sN+sr0P,≤1r0[N(1−N)(N−θ)(η+(1−η)−dP]+sN+sr0P,≤θ+1r0N2−(θr0−s)N−(dr0−sr0)P. |
Here, N<1, and if s=min(θr0,d), then
dwdt+sw≤θ+1r0. |
Let θ+1r0=L. Then, the above differential inequality can be expressed in the form
dwdt+sw≤L. |
Using the theory of differential inequality for w(t), we obtain
0<w(N,P)≤Ls(1−e−st)+w0e−st, |
where w0=w(N0,P0). We can deduce that for t→∞, 0<w≤Ls.
For any ϵ>0, define
Δ={(P,N)∈R2+;N+1r0P≤Ls+ϵ}. |
This shows that the solution of the system represented by (3.1) is bounded, i.e., for every trajectory of (3.1), there is a time t0 such that (N(t),P(t))∈Δ for all t>t0. Hence, the theorem is proved.
It can be verified that model (3.1) has four possible equilibria:
(1) The extinction equilibrium E0=(0,0).
(2) The axial equilibrium Eθ=(θ,0).
(3) The axial equilibrium E1=(1,0).
(4) The coexistence equilibrium E∗=(N∗,P∗), where N∗=d and P∗ is a nonnegative root of the quadratic equation: P∗2+σ1P∗+σ2=0. Define σ1=α−η(1−d)(d−θ) and σ2=−α(1−d)(d−θ). By Descartes' rule of signs, the quadratic equation has a unique nonnegative root if θ<d<1, as shown in Figure 2.
The stability matrix (J) for system (3.1) is
(S1S2PN−d) |
where S1=1r0(η+1−η1+sαP)[(1−2N)(N−θ)+N(1−N)]−Pr0 and S2=−1r0[N(1−N)(N−θ)α(1−η)(α+P)2+N].
(1)E0 is locally asymptotically stable because the characteristic roots are –θr0<0 and −d<0.
(2) The characteristic roots for Eθ are θ(1−θ)r0 and θ−d, θ(1−θ)r0>0 because 0<θ<1 from system (3.1). Thus, Eθ is unstable.
(3)E1 is stable if d>1 because the characteristic roots are –(1−θ)r0<0 and 1−d.
(4) The stability matrix for the coexistence equilibrium E∗=(N∗,P∗)=(d,P∗) is:
J∗=(1r0(η+1−η1+sαP∗)[−2d2+(θ+1)d]−1r0[d(1−d)(d−θ)(α(1−η)(α+P∗)2)+d]P∗0). |
The corresponding characteristic equation is λ2+Aλ+B=0, where A=−1r0(η+1−η1+sαP∗)[−2d2+(θ+1)d] and B=P∗r0[d(1−d)(d−θ)(α(1−η)(α+P∗)2)+d]. The Routh-Hurwitz criteria for the second order system is given by: A>0 and B>0.
A=−1r0(η+1−η1+sαP∗)[−2d2+(θ+1)d]>0 if d>θ+12 and because (N∗,P∗) exists for θ<d<1, B=P∗r0[d(1−d)(d−θ)(α(1−η)(α+P∗)2)+d]>0. Therefore, (N∗,P∗) is locally asymptotically stable if d>θ+12, i.e., twice the death rate of predator is one unit higher than the Allee threshold value as shown in Figures 3 and 4.
We observed a transcritical bifurcation and a Hopf-bifurcation that occurs around the equilibrium points when the parameter passes through some critical values.
According to Sotomayor's theorem [31], we investigate the transcritical bifurcation for the system (3.1) taking d=d0 as the bifurcation parameter. If the following conditions are satisfied:
1)wTfd(E1,d0)=0,
2)wT(Dfd(E1,d0).υ)≠0,
3)wTD2f(E1,d0)(υ,υ)≠0,
then the system (3.1) experiences a transcritical bifurcation at the equilibrium point E1=(1,0) as the parameter d passes through the bifurcation value d=d0=1, where fd denotes the vector of partial derivatives of the components of f with respect to the scalar d and Df represents the matrix of partial derivatives of the components of f with respect to the components of X=(N,P). Here,
Df(X)=(∂f1∂N∂f1∂P∂f2∂N∂f2∂P), D2f(X)=(∂2f1∂N∂N∂2f1∂N∂P∂2f1∂P∂N∂2f1∂P∂P∂2f2∂N∂N∂2f2∂N∂P∂2f2∂P∂N∂2f2∂P∂P).
υ is an eigenvector of A=Df(¯E1,d0) corresponding to the eigenvalue λ2=0 and w is an eigenvector of AT corresponding to the eigenvalue λ2=0.
Theorem 2. The system (3.1) undergoes a transcritical bifurcation at the positive equilibrium E1=(1,0), as the parameter d passes through the bifurcation value d=d0=1.
Proof. The linearized system around the equilibrium point E1 is:
J(E1)=(−(1−θ)r0−1r001−d).SoJ(E1,d0)=(−(1−θ)r0−1r000). |
Let us define υ=(υ1,υ2)T and w=(w1,w2)T to be the right and left eigenvectors of λ2=0. Now solving J(E1,d0)υ=0 implies that υ=(−υ21−θ,υ2), and by solving JT(E1,d0)w=0, we get w=(0,w2) where υ2,w2 are any nonzero real numbers. Now, system (3.1) can be rewritten as in the following vector form:
˙X=f(X),where X=(N,P) and f(X)=(1r0[N(1−N)(N−θ)(η+1−η1+sαP)−NP]NP−dP). |
Taking the derivative of f(X) with respect to d, we get fd(X)=(0−P), then f(E1,d0)(X)=(00). Hence wTf(E1,d0)(X)=0. Next, taking the derivative of fd(X) with respect to X=(N,P)T, we get
Dfd(X)=(000−1) so that Df(E1,d0)(X)=(000−1). |
We have wT(Df(E1,d0)(X).υ)=−w2υ2≠0. Furthermore,
D2f(X)=(Q1Q2Q3Q40110) |
whereQ1=1r0(η+1−η1+sαP)[−2(N−θ)+2(1−N)−2N],Q2=1r0(−α(1−η)(α+P)2)[(1−N)(N−θ)−N(N−θ)+N(1−N)]−1r0,Q3=−1r0(α(1−η)(α+P)2)[(1−N)(N−θ)−N(N−θ)+N(1−N)]−1r0,Q4=2r0(α(1−η)(α+P)3)[N(1−N)(N−θ)]. |
Hence,
D2f(E1,d0)(X)=(1r0(−2(1−θ)−2)1−ηr0α(1−θ)−1r0−1−ηr0α(−(1−θ))−1r000110), |
D2f(E1,d0)(X)(υ,υ)=(−2r0(2−θ)υ1υ1+(1−ηr0α(1−θ)−1r0)υ1υ2−[1−ηr0α(−(1−θ))−1r0]υ2υ1υ1υ2+υ2υ1), |
where (υ,υ) is a tensor product of (υ1,υ2)T, so wTD2fE1,d0(X)(υ,υ)=(υ1υ2+υ2υ1)w2=2υ1υ2w2≠0. Therefore, according to Sotomayor's theorem [31] for local bifurcation, system (3.1) has a transcritical bifurcation at steady-state E1 when the parameter d passes through the bifurcation value d0=1.
Theorem 3. Any system of the form
˙N=f(N,P;d),˙P=g(N,P;d), | (3.2) |
that has an equilibrium (N∗,P∗) for the parameter d whose linearization has eigenvalues λ1,2=γ(d)±iβ(d) such that γ(¯d)=0, β(¯d)=¯β>0 and satisfying the following condition:
d(γ)d(d)|d=¯d≠0, |
experiences Andronov-Hopf bifurcation.
Proof. From the previous analysis, we know that the two equilibrium points (0,0) and (1,0) have no complex eigenvalues. Therefore, we use the coexistence fixed point (N∗,P∗) to check if the conditions stated in the above theorem apply to the model.
The possibility of the Hopf-bifurcation at E∗=(N∗,P∗) has been analyzed by taking d as a bifurcation parameter and keeping the rest of the parameters as constants. To begin, we investigate the linearization condition, which is
J∗=(1r0(η+1−η1+sαP∗)[−2d2+(θ+1)d]−1r0[d(1−d)(d−θ)(α(1−η)(α+P∗)2)+d]P∗0). | (3.3) |
The eigenvalues of (3.3) in terms of the trace and determinant are given by
λ1,2=trJ±√tr2J−4detJ2,trJ=1r0(η+1−η1+sαP∗)[−2d2+(θ+1)d]. |
Let γ(d)=trJ2. For the condition of the theorem to be satisfied, we try to find a value d=¯d such that γ(¯d)=0.
γ(¯d)=12r0(η+1−η1+sαP∗)[−2¯d2+(θ+1)¯d]=0, which implies that ¯d=θ+12. |
Thus, there exists a value d=¯d at which γ(¯d)=0, or similarly the real part of the eigenvalues is 0.
Next, we consider dγ(d)d(d)|d=¯d. We have γ(d)=12r0(η+1−η1+sαP∗)[−2d2+(θ+1)d].
dγ(d)d(d)=12r0(η+1−η1+sαP∗)(−4d+(θ+1))+12r0[−2d2+(θ+1)d]α(1−η)(α+P)2dPdd,dγ(d)d(d)|d=¯d=12r0(η+1−η1+sαP∗)(−(θ+1))≠0. | (3.4) |
Therefore, the condition dγ(d)d(d)|d=¯d≠0 is satisfied.
Now we show that the complex part of the eigenvalues exist at d=¯d and they are not zero. Let iβ(d)=±i√4detJ−tr2J2. We know that at ¯d, trJ=γ(¯d)=0, which implies that tr2J=0. Therefore
iβ(¯d)=±i√detJ(¯d). |
Next, we find detJ(d):
detJ(d)=P∗r0[d(1−d)(d−θ)(α(1−η)(α+P∗)2)+d]>0 as E∗ only exists if θ<d<1.detJ(¯d)=P∗r0[¯d(1−¯d)(¯d−θ)(α(1−η)(α+P∗)2)+¯d]>0.Therefore at d=¯d,β(d)>0. |
Hence, the conditions for the existence of the Hopf-bifurcation are satisfied.
We run the bifurcation diagram using XPPAUT software and using the parameter d as the bifurcation parameter. Figure 5 shows the Hopf-bifurcation at the coexistence equilibrium E∗ when d=1+θ2 and the transcritical bifurcation at the equilibrium E1 when d=1.
It is clear from Figure 6 that as the level of fear sα increases, the initial short-term decrease of the prey population becomes larger. However, after a long period of time, the level of fear has no effect on the long-term level of the prey population as the prey become habituated to the presence of the predator. However, as the level of fear increases, the long-term level of the predator population decreases.
The existence of the coexistence equilibrium point E∗=(N∗,P∗) implies that θ<d<1. If the Allee threshold θ is small, the coexistence equilibrium is stable when it exists and the Allee effect has no impact on the long-term prey density, as shown in Figure 7. Moreover, for small values of the Allee threshold, the long-term level of the predator population decreases. However, as the Allee threshold increases further, both the long-term predator and long-term prey populations go extinct.
Next, we consider the model with no disease present and a time delay. Putting y=0 in Eq (2.4) and transforming as in Eq (3.1), we get:
dNdt=1r0[N(1−N)(N−θ)(η+α(1−η)α+P)−NP],dPdt=N(t−τ)P(t−τ)−dP. | (4.1) |
At equilibrium ¯N=d. Let N=¯N+u and P=¯P+v where u and v are very small perturbations. Then substituting into the second equation of (4.1), we deduce that
dvdt=[¯N+u(t−τ)][¯P+v(t−τ)]−d[¯P+v], =(¯N¯P−d¯P)−dv+¯Nv(t−τ)+¯Pu(t−τ). |
Let v=Beλt and u=Aeλt. Substituting into the equations and dividing by eλt,
A[¯Pe−λτ]+B[−d+¯Ne−λτ−λ]=0. |
Now,f(N,P)=1r0(η+1−η1+sαP)[N2−N3−Nθ+N2θ]−NPr0,∂f∂N=1r0(η+1−η1+sαP)[2N−3N2−θ+2Nθ]−Pr0=L, say,∂f∂P=−1r0[N(1−N)(N−θ)α(1−η)(α+P)2+N]=M, say. |
The stability matrix is (L−λM¯Pe−λτ−d+¯Nde−λτ−λ), where ¯N=d. The corresponding characteristic equation is:
λ2+a1λ−a2−de−λτ(λ+b1)=0,where a1=d−L,a2=Ld and b1=¯PMd−L. | (4.2) |
Let λ=σ+iρ. Substituting into the characteristic equation and expanding,
σ2−ρ2+2iσρ+a1σ+ia1ρ−a2=de−τσ[cos(τρ)−isin(τρ)][(σ+b1)+iρ]. |
Equating real and imaginary parts,
σ2−ρ2+a1σ−a2=de−τσ[(σ+b1)cos(τρ)+ρsin(τρ)],2σρ+a1ρ=de−τσ[−(σ+b1)sin(τρ)+ρcos(τρ)]. | (4.3) |
At τ=τ∗1, σ(τ∗1)=0,
ρ∗21+a2=−d[b1cos(τ∗1ρ∗1)+ρ∗1sin(τ∗1ρ∗1)], a1ρ∗1=d[−b1sin(τ∗1ρ∗1)+ρ∗1cos(τ∗1ρ∗1)]. | (4.4) |
Squaring and adding the two parts of (4.4), we obtain
ρ∗41+ρ∗21(a21+2a2−d2)+a22−d2b21=0. | (4.5) |
Let ξ=ρ∗21, then (4.5) reduces to
φ(ξ)=ξ2+ξ(a21+2a2−d2)+a22−d2b21=0. | (4.6) |
If ρ∗21=ξ is the last positive single root of (4.6), then
dφdξ|ξ=ρ∗21=2ρ∗21+a21+2a2−d2>0. | (4.7) |
Using (4.4), after removing sin(τ∗1ρ∗1) terms, we get
τ∗1=1ρ∗1cos−1[ρ∗21(b1−a1)+b1a2−d(b2+ρ∗21)]. | (4.8) |
Using the analytic version of the implicit function theorem [32] to establish Hopf-bifurcation at τ=τ∗1, we need to show that
dσdτ|τ=τ∗1≠0. | (4.9) |
Differentiate (4.3) with respect to τ for ρ(τ∗1)=ρ∗1 and σ(τ∗1)=0, and we get
dσdτ[a1+dτ(b1cos(τ∗1ρ∗1)+ρ∗1sin(τ∗1ρ∗1))−dcos(τ∗1ρ∗1)]+dρdτ[−2ρ∗1+db1τ∗1sin(τ∗1ρ∗1)−dsin(τ∗1ρ∗1)−dτ∗1ρ∗1cos(τ∗1ρ∗1)]=ρ∗1[db1sin(τ∗1ρ∗1)+dρ∗1cos(τ∗1ρ∗1)],=ρ∗1(a1ρ∗1)=a1ρ∗21,dσdτ[2ρ∗1−dτ∗1(b1sin(τ∗1ρ∗1)−ρ∗1cos(τ∗1ρ∗1))+dsin(τ∗1ρ∗1)]+dρdτ[a1+db1τ∗1cos(τ∗1ρ∗1)−dcos(τ∗1ρ∗1)+dτ∗1ρ∗1sin(τ∗1ρ∗1)]=ρ∗1[−db1cos(τ∗1ρ∗1)−dρ∗1sin(τ∗1ρ∗1)],=ρ∗1(ρ∗21+a2). | (4.10) |
Let R=a1+dτ∗1(b1cos(τ∗1ρ∗1)+ρ∗1sin(τ∗1ρ∗1))−dcos(τ∗1ρ∗1)
and S=−2ρ∗1+dτ∗1b1sin(τ∗1ρ∗1)−dsin(τ∗1ρ∗1)−dτ∗1ρ∗1cos(τ∗1ρ∗1).
Equation (4.10) can be expressed as
Rdσdτ+Sdρdτ=a1ρ∗21,−Sdσdτ+Rdρdτ=ρ∗1(ρ∗21+a2). | (4.11) |
Solving (4.11) for dσdτ, we get
dσdτ=Ra1ρ∗21−Sρ∗1(ρ∗21+a2)R2+S2,=1R2+S2[[a1+dτ∗1(b1cos(τ∗1ρ∗1)+ρ∗1sin(τ∗1ρ∗1))−dcos(τ∗1ρ∗1)]a1ρ∗21[−2ρ∗1−dτ∗1b1sin(τ∗1ρ∗1)−dsin(τ∗1ρ∗1)−dτ∗1ρ∗1cos(τ∗1ρ∗1)]ρ∗1(ρ∗21+a2)],=1R2+S2[ρ∗1(a1−τ∗1(ρ∗21+a2)−dcos(τ∗1ρ∗1))a1ρ∗1−ρ∗1(−2ρ∗1−τ∗1(a1ρ∗1)−dsin(τ∗1ρ∗1))(ρ∗21+a2)],=ρ∗1(a21ρ∗1+2ρ∗1(ρ∗1+a2)−d2ρ∗1)R2+S2=ρ∗21(2ρ∗21+(a21+2a2−d2))R2+S2. |
Using inequality (4.7), we obtain
dσdτ|τ=τ∗1=ρ∗21dφdξR2+S2>0. |
Figure 8 shows the Hopf-bifurcation at τ=τ∗1.
We now return to the full eco-epidemiological model with no time delay. Recall that the non-dimensionalized form of this is Eq (2.2):
dxdt=x(1−x−y)(x−θ)(η+1−η1+sαz)−βxyc+δy−axz,dydt=βxyc+δy−gyz−μy,dzdt=bxz+pyz−mz. |
Propsition: The trajectory of system (2.2) is bounded.
Proof. Let s=x+y+z. Differentiating along the solution of model (2.2), we get
dsdt=dxdt+dydt+dzdt,=x(1−x−y)(x−θ)(η+1−η1+sαz)−(a−b)xz−(g−p)yz−μy−mz. |
For any positive constant P, we have
dsdt+Ps≤x(x−θ)+Px+Py+Pz−mz, |
since a≥b and g≥p.
dsdt+Ps≤1+P−(m−P)z, |
as u1+u2<k for all time implies that x+y<1.
Now if m>P, then
dsdt+Ps≤(1+P). |
Let (1+P)=M, therefore we have
dsdt+Ps≤M. |
Using the theory of differential inequality for s(t), we get
0<s(x,y,z)≤MP(1−e−Pt)+s0e−Pt, |
where s0=(x0,y0,z0). It can be deduced that limt→∞sups≤MP, independently of the initial conditions.
Corollary: If ϵ>0, then the region
D={0≤x,0≤y,0≤z,x+y≤1,x+y+z≤MP+ϵ} |
is an invariant region for model (2.2).
Since model (2.2) deals with animals populations, it is necessary to show that the prey and predator population will remain positive for all time.
x(t)=x(0)exp{∫t0[(1−x−y)(x−θ)(η+1−η1+sαz)−βyc+δy−az]du},y(t)=y(0)exp{∫t0[βxc+δy−gz−μ]du},z(t)=z(0)exp{∫t0[bx+py−m]du}. |
Hence, all solutions (x(t),y(t),z(t)) will remain positive for all time with positive initial values, that is, (x(0),y(0),z(0))∈R3+.
Before analyzing the model (2.2), we define two threshold quantities:
(1) The infection reproduction number r0=βcμ. This is the product of the average infectious duration 1μ and βc, the number of secondary infections per unit time at the disease-free equilibrium in a prey population with no predators. If r0<1, disease will not invade a disease-free equilibrium in the absence of predators. If r0>1, disease will invade such a population.
(2) The predator reproduction number is rd=bm. This is the product of the predator life expectancy 1m and the rate b at which each predator reproduces in a prey population at equilibrium with no disease present. If rd<1, the predator cannot invade such a population, whereas if rd>1, the predator will invade such a population.
The possible equilibria for the system are:
(a) E0=(0,0,0) is the trivial equilibrium and it always exists. Here, the populations of susceptible prey, infected prey, and predator species go to extinction.
(b) The two axial equilibria, E1=(1,0,0) and Eθ=(θ,0,0).
(c) The planar equilibrium Ez=(1rd,0,¯z) where the infected prey is extinct. This exists when 1<rd<1θ. In this case, ¯z is the unique nonnegative root of the quadratic equation
a¯z2+(aα−η(1−1rd)(1rd−θ))¯z−α(1−1rd)(1rd−θ)=0. | (5.1) |
By Descartes' rule of signs, Eq (5.1) has a unique nonnegative root if, and only if,
0<(1−1rd)(1rd−θ), |
in which case 1<rd<1θ.
(d) The planar equilibrium Ey=(¯x,¯y,0), where ¯x=1r0(c+δ¯y) and ¯y is the nonnegative root of the cubic equation
¯y3[δ3r20+δ2r0]+¯y2[−δ2r0+cδ2r20+2δ2r20−θδ2r0+2cδr0−θδ]+¯y[−2cδr0+θδ+3c2δr20−2cθδr0+c2r0−cθ+β]+[−c2r0+cθ+c3r20−c2θr0]=0. | (5.2) |
This equation can be written as γ1y3+γ2y2+γ3y+γ4=0, where
γ1=(δ3r20+δ2r0)>0,γ2=(δ2cr0+δr0)(1r0−θ)+(c+δr0)δr0c+(1r0−1)δ2r0c,γ3=δ(1r0−1)(1r0−θ)+(c+δr0)(1r0−θ)+δr0(1r0−1)+β,γ4=c(1r0−1)(1r0−θ). |
Note that γ4<0 if 1<r0<1θ.
(e) The interior equilibrium E∗=(x∗,y∗,z∗), where
x∗=1b[m−py∗],z∗=1g(c+δy∗)[β(m−py∗)b−μ(c+μδy∗)]. |
Note that
βm−μcbβp+μδb<mp. |
For this equilibrium to exist, we need
y∗<βm−μcbβp+μδb. |
y∗ is a positive root of the equation ξ1y4+ξ2y3+ξ3y2+ξ4y+ξ5=0, where ξ1, ξ2, ξ3, ξ4, and ξ5 are given in Appendix A.
It is simple to show that the stability matrix for the system (2.1) linearized about the equilibrium point is
(a11a12a13a21a22a23a31a32a33) | (5.3) |
wherea11=(η+1−η1+sαz)[(1−x−y)(x−θ)−x(x−θ)+x(1−x−y)]−βyc+δy−az,a12=−x(x−θ)(η+1−η1+sαz)−δβxy(c+δy)2+βxc+δy,a13=−x(1−x−y)(x−θ)α(1−η)(α+z)2−ax, a21=βyc+δy,a22=βxc+δy−δβxy(c+δy)2−az−μ, a23=−gy, a31=bz, a32=pz,a33=bx+py−m. |
E0 is locally asymptotically stable because all the corresponding eigenvalues are negative. The stability matrix for equilibrium E1=(1,0,0) is:
J1=(−1+θ−1+θ−βc−a0μ(r0−1)000m(rd−1)). |
The eigenvalues are λ1=−1+θ(<0), λ2=μ(r0−1) and λ3=m(rd−1), so E1 is locally asymptotically stable if r0<1 and rd<1, as shown in Figure 9.
The stability matrix for equilibrium Eθ=(θ,0,0) is:
Jθ=(θ(1−θ)−βθc−aθ0μθ(r0−1θ)000mθ(rd−1θ)). |
The eigenvalues are λ1=θ(1−θ), λ2=μθ(r0−1θ) and λ3=mθ(rd−1θ). Eθ is unstable since λ1=θ(1−θ)>0.
The stability matrix for Ez is:
Jz=(σ1σ2σ30βcrd−g¯z−μ0b¯zp¯z0), |
whereσ1=(η+1−η1+sα¯z)1rd(1−2rd+θ),σ2=−1rd(1rd−θ)(η+1−η1+sα¯z)−βcrd,σ3=−1rd(1−1rd)(1rd−θ)α(1−η)(α+¯z)2−ard. |
One eigenvalue is given by λ2=βcrd−g¯z−μ, which is negative if
2aβ−2acrdμ−cgrd{η(1−1rd)(1rd−θ)−aα}{+√[aα−η(1−1rd)(1rd−θ)]2+4aα(1−1rd)(1rd−θ) }<0. |
The other eigenvalues, λ1 and λ3, are roots of the characteristic equation given by
λ2−[(η+1−η1+sα¯z)1rd(1−2rd+θ)]λ+b¯z[1rd(1−1rd)(1rd−θ)α(1−η)(α+¯z)2+ard]=0. |
Clearly, the roots of above equation have negative real parts if
(η+1−η1+sα¯z)1rd(1−2rd+θ)<0, which implies that rd<21+θ. |
Therefore, the equilibrium Ez is locally asymptotically stable if
2aβ−2acrdμ−cgrd{η(1−1rd)(1rd−θ)−aα}{+√[aα−η(1−1rd)(1rd−θ)]2+4aα(1−1rd)(1rd−θ) }<0, |
and rd<21+θ. Figure 10 shows the local stability for the equilibrium point Ez.
The stability matrix for Ey is:
Jy=(τ1τ2τ3β¯yc+δ¯y−δβ¯x¯yc+δ¯y−g¯y00b¯x−p¯y−m), |
where τ1=¯x(1−2¯x−¯y−θ), τ2=−¯x(¯x−θ)−β¯xc(c+δ¯y)2 and τ3=¯x(1−¯x−¯y)(¯x−θ)(1−η)α−a¯x. One eigenvalue is given by λ3=b¯x−p¯y−m. The other eigenvalues, λ1 and λ2, are roots of the characteristic equation given by:
λ2−(K+N)λ+(KN−ML)=0. |
Here, K=τ1, L=τ2<0, M=β¯yc+δ¯y>0, and N=−δβ¯x¯y(c+δ¯y)2<0.
The roots of the characteristic equation have negative real parts if K+N<0 and (KN−ML)>0. Figure 11 shows the solution converges to the equilibrium point Ey=(¯x,¯y,0).
The stability matrix at the interior attractor is given by
J∗E=(ABCDEFGH0), | (5.4) |
where A=(η+1−η1+sαz∗)[x∗(1−2x∗−y∗+θ)], B=−x∗(x∗−θ)(η+1−η1+sαz∗)+δβx∗y∗(c+δy∗)2−βx∗c+δy∗<0, C=−x∗(1−x∗−y∗)(x∗−θ)α(1−η)(α+z∗)2−ax∗<0, D=βy∗c+δy∗>0, E=−δβx∗y∗(c+δy∗)2<0, F=−gy∗<0, G=bz∗>0, and H=pz∗>0.
The characteristic equation associated with this stability matrix is given by
λ3+a1λ2+a2λ+a3=0, | (5.5) |
where a1=tr[J∗E]=−(A+E),a2=AE−BD−FH−CG, and a3=−det[J∗E]=AHF−BGF−DHC+GEC. From the Routh-Hurwitz criteria, the equilibrium E∗ is locally asymptotically stable if, and only if, a1>0, a3>0, and a1a2>a3. Figure 12 demonstrates that the solution converges to the equilibrium point E∗=(x∗,y∗,z∗).
When the parameters go through specific critical values, a transcritical bifurcation and a Hopf-bifurcation occur around the equilibrium points.
According to Sotomayor's theorem for local bifurcation [31], if the following conditions are satisfied:
(1)wTfm(E1,m0)=0,
(2)wT(Dfm(E1,m0).υ)≠0,
(3)wTD2f(E1,m0)(υ,υ)≠0,
where w and υ are the left and right eigenvectors of fm(E1,m0), then the system (2.2) experiences a transcritical bifurcation at the equilibrium point E1=(1,0,0), when rd=1 (i.e m=b).
Theorem 4. If r0≠c, then the system (2.2) undergoes a transcritical bifurcation at the positive equilibrium E1=(1,0,0) when m=b.
Proof:
The linearized system around the equilibrium point E1 is:
J1=(−1+θ−1+θ−βc−a0μc(r0−c)000m(rd−1)). |
Now, λ3=0 at rd=1, (i.e, at m0=b). So
J(E1,m0)=(−1+θ−1+θ−βc−a0μc(r0−c)0000). |
Let us define υ=(υ1,υ2,υ3)T and w=(w1,w2,w3)T to be the right and left eigenvectors of λ3=0. Now solving J(E1,m0)υ=0 implies that υ=(aυ3−1+θ,0,υ3), and by solving JT(E1,m0)w=0, we get w=(0,0,w3) where υ3,w3 are any nonzero real numbers.
Now, if X=(x,y,z), system (2.2) can be rewritten in the following vector form:
˙X=f(X), | (5.6) |
wheref(X)=(x(1−x−y)(x−θ)(η+1−η1+sαz)−βxyc+δy−axzβxyc+δy−gyz−μybxz+pyz−mz). |
Taking the derivative of f(X) with respect to m, we get fm(E1,m0)=(000). Hence, wTfm(E1,m0)=0.
Next, taking the derivative of fm(X) with respect to X=(x,y,z)T, we get
Dfm(E1,m0)=(00000000−1). |
Therefore, we have wT(Dfm(E1,m0).υ)=−w3υ3≠0. Furthermore,
D2f(X)=(A1A2A3A4A5A6A7A8A9A10A11A12A13A14A15A16A17A18A19A20A21A22A23A24A25A26A27), |
where A1=(η+1−η1+sαz)[−2(x−θ)−2x], A2=(η+1−η1+sαz)[−(x−θ)−x]−βc(c+δy)2, A3=−α(1−η)(α+z)2[(1−x−y)(2x−θ)−x(x−θ)]−a, A4=(η+1−η1+sαz)[−(x−θ)−x]−βc(c+δy)2, A5=2βcδy(c+δy)3, A6=x(x−θ)α(1−η)(α+z)2, A7=α(1−η)(α+z)2[−(1−2x−y)(x−θ)−x(1−x−y)]−a, A8=x(x−θ)α(1−η)(α+z)2, A9=2x(1−x−y)(x−θ)α(1−η)(α+z)3, A10=0, A11=βc(c+δy)2, A12=0, A13=βcy(c+δy)2, A14=−2δcβx(c+δy)3, A15=−g, A16=0, A17=−g, A18=0, A19=0, A20=0, A21=b, A22=0, A23=0, A24=p, A25=b, A26=p, A27=0.
So, wTD2f(E1,m0)(υ,υ)=2bυ1υ3w3+2pυ2υ3w3≠0. Therefore, according to Sotomayor's theorem [31] for local bifurcation, system (2.2) has a transcritical bifurcation at steady-state E1=(1,0,0) when the parameter rd=1, (i.e, m=b) (Figure 13).
We observe that the system shows the Hopf-bifurcation for the equilibrium point Ez with respect to the parameter θ when θ=0.333335, (Ez=(0.666667,0,0.046177)), as shown in Figure 14 by using Matcont software for bifurcation. The blue color shows that the equilibrium is stable, while the red color shows the unstable equilibrium.
In addition, Figure 15 shows another Hopf-bifurcation for the equilibrium point Ez with respect to the parameter m when m=0.900001, (Ez=(0.600000,0,0.062795)).
Also, we observed the Hopf-bifurcation for the equilibrium point Ez with respect to the parameter b when b=1.666664, (Ez=(0.600001,0,0.062795)), as seen in Figure 16.
Figure 17 shows the Hopf-bifurcation for the equilibrium point Ey with respect to the parameter μ when μ=0.845893(Ey=(0.502786,0.075836,0)).
Also, we found the Hopf-bifurcation for the equilibrium point Ey with respect to the parameter δ when δ=0.101810(Ey=(0.556617,0.073434,0)), as shown in Figure 18.
We observe from Figure 19 that the population of uninfected prey declines as fear intensity rises, and after a certain level of fear, population of uninfected prey will not change due to habituation to stress. Increasing the fear parameter stabilizes the system. For an intermediate value of fear, the system becomes unstable, and after a certain level of fear, the system shows instability and the predator population goes extinct. As a result, the infected prey and the uninfected prey interact and this increases disease transmission, and, consequently, the infected prey increases.
Figure 20 shows the effect of the Allee threshold effect on uninfected prey, infected prey, and predator. It is clear that when the Allee threshold increases, the population of uninfected prey and predator decrease while the population of infected prey increases. When the Allee threshold exceeds 0.3, all populations go to extinction.
Recall the non-dimensionalized full eco-epidemiological model (2.4) with a time delay included:
dxdt=x(1−x−y)(x−θ)(η+1−η1+sαz)−βxyc+δy−axz,dydt=βxyc+δy−gyz−μy,dzdt=bx(t−τ)z(t−τ)+py(t−τ)z(t−τ)−mz. |
DefineA=∂f∂x=(η+1−η1+sαz)[−(x2−θx)+(1−x−y)(2x−θ)]−βyc+δy−az,B=∂f∂y=−x(x−θ)(η+1−η1+sαz)−βxc(c+δy)2<0,C=∂f∂z=−x(1−x−y)(x−θ)α(1−η)(α+z)2−ax<0, D=∂g∂x=βyc+δy>0,E=∂g∂y=βxc(c+δy)2−gz−μ and F=∂g∂z=−gy<0. |
Also define b¯x+p¯y=m. The characteristic equation for model (2.4) is
ξ3+P2ξ2+P1ξ+P0+e−ξτ(Q2ξ2+Q1ξ+Q0)=0, | (6.1) |
whereP0=(AE−BD)m,=[((η+1−η1+sαz)[−(x2−θx)+(1−x−y)(2x−θ)]−βyc+δy−az)×(βxc(c+δy)2−gz−μ)+(x(x−θ)(η+1−η1+sαz)+βxc(c+δy)2)(βyc+δy)]m,P1=AE−Am−BD−Em,=(η+1−η1+sαz)x(1−2x+θ−y)[βxc(c+δy)2−gz−μ−m]+[x(x−θ)(η+1−η1+sαz)+βxc(c+δy)2]βyc+δy+[−βxc(c+δy)2+gz+μ]m,P2=−A−E+m,=−(η+1−η1+sαz)[−(x2−θx)+(1−x−y)(2x−θ)]+βyc+δy+az−βxc(c+δy)2+gz+μ+m,Q0=−AEm+AFp¯z+BDm−BFb¯z−CDp¯z+CEb¯z,Q1=(A+E)m−Cb¯z−Fb¯z,=[(η+1−η1+sαz)x(1−2x+θ−y)+βxc(c+δy)2−gz−μ]m+[x(1−x−y)(x−θ)α(1−η)(α+z)2+ax]b¯z+gyb¯z,Q2=−m. |
If τ=0, then E∗ is asymptotically stable under certain conditions. When τ≠0, we take ξ=ι+iψ where ι,ψ∈R. Substituting the value of ξ into (6.1) and separating in terms of real and imaginary parts:
(ι3−3ιψ2)+P2(ι2−ψ2)+P1ι+P0=−e−ιτ[cos(ψτ)(Q2(ι2−ψ2))+(Q1ι+Q0)cos(ψτ)+sin(ψτ)(2Q2ιψ+Q1ψ)],(3ι2ψ−ψ3)+2P2ιψ+P1ψ=−e−ιτ[cos(ψτ)(2Q2ιψ+Q1ψ)−sin(ψτ)(Q2(ι2−ψ2))−(Q1ι+Q0)sin(ψτ)]. | (6.2) |
Let τ1=τ∗1 be such that ι(τ∗1)=0 and ψ(τ∗1)=ψ∗1, then (6.2) can be written as
−P2ψ∗21+P0=A1cos(ψ∗1τ∗1)−B1sin(ψ∗1τ∗1),−ψ∗31+P1ψ∗1=−B1cos(ψ∗1τ∗1)−A1sin(ψ∗1τ∗1), | (6.3) |
where A1=Q2ψ∗21−Q0 and B1=Q1ψ∗1. By squaring and adding, on simplification, it follows that
ψ∗61+ψ∗41(P22−2P1−Q22)+ψ∗21(−2P2P0+P21+2Q0Q2−Q21)+(P20−Q20)=0. | (6.4) |
Let ψ∗21=w. Also
φ(w)=w3+P∗2w2+P∗1w+P∗0=0, | (6.5) |
where P∗0=P20−Q20, P∗1=−2P2P0+P21+2Q0Q2−Q21, and P∗2=P22−2P1−Q22. Note that φ′(w)=3w2+2P∗2w+P∗1. The two roots of the equation φ′(w)=0 are: w1=−P∗2+√P∗22−3P∗13 and w1=−P∗2−√P∗22−3P∗13. The conditions for (6.5) to have positive roots are as follows:
(a) If P∗0<0, then (6.5) has at least one positive root.
(b) If P∗0≥0 and P∗22≤3P∗1, then (6.5) has no positive root.
(c) If P∗0≥0 and P∗22>3P∗1, then (6.5) has a positive root if, and only if, w1>0 and ϕ(w1)≤0.
Using the above conditions, we can say that if condition (b) is satisfied then the stability of E∗ will not change on increasing τ. If (6.5) has a positive root, then the stability of E∗ may change as τ changes. Let w1,w2, and w3 be three positive roots of (6.5), then (6.4) has positive roots ψ∗1=√wk, k=1,2,3. Using (6.3), we get:
cos(ψ∗1τ∗1)=A1(P0−P2ψ∗21−P0)+B1(−ψ∗31+P1ψ∗1)A21+B21, |
or τ∗1=1ψ∗1{cos−1(A1(P0−P2ψ∗21)−B1(−ψ∗31+P1ψ∗1))(A21+B21))}, | (6.6) |
where ψ∗1 is the last positive root of (6.4). To establish Hopf-bifurcation at τ=τ∗1, we need to show that
dιdτ|τ=τ∗1≠0. | (6.7) |
Differentiating (6.2) with respect to τ and setting ι=0 and ψ=ψ∗1, we get
dιdτ|τ=τ∗1[Ucos(τ∗1ψ∗1)+Vsin(τ∗1ψ∗1)+M]+dψdτ|τ=τ∗1[−Vcos(τ∗1ψ∗1)+Usin(τ∗1ψ∗1)−N]=−Xsin(τ∗1ψ∗1)−Ycos(τ∗1ψ∗1), |
dιdτ|τ=τ∗1[Vcos(τ∗1ψ∗1)−Usin(τ∗1ψ∗1)+N]+dψdτ|τ=τ∗1[Ucos(τ∗1ψ∗1)+Vsin(τ∗1ψ∗1)+M]=−Xcos(τ∗1ψ∗1)+Ysin(τ∗1ψ∗1), | (6.8) |
where U=Q2ψ∗21τ∗1−Q0τ∗1+Q1, V=2Q2ψ∗1−Q1ψ∗1τ∗1, M=−3ψ∗21+P1, N=2P2ψ∗1, X=Q2ψ∗31−Q0ψ∗1, and Y=Q1(ψ∗1)2. Finally, by solving dιdτ|τ=τ∗1 and dψdτ|τ=τ∗1, we have
dιdτ|τ=τ∗1=ψ∗21[3ψ∗41+2ψ∗21(P22−2P1−Q22)+(2Q2Q0+P21−Q21−2P2P0)]S21+S22, | (6.9) |
where S1=Ucos(τ∗1ψ∗1)+Vsin(τ∗1ψ∗1)+M, S2=Usin(τ∗1ψ∗1)−Vcos(τ∗1ψ∗1)−N, and S21+S22>0.
If ψ∗1 is the last positive root of (6.4), then using (6.5),
dφdw|w=ψ∗21>0. | (6.10) |
Hence,dιdτ|τ=τ∗1=ψ∗21dφdw|w=ψ∗21S21+S22>0. |
Figure 21 shows the Hopf-bifurcation of model (2.4) with respect to τ.
Let X(t)=¯X+u(t), Y(t)=¯Y+v(t), and Z(t)=¯Z+w(t). Linearizing the system (2.4) at the coexistence equilibrium E∗, we get:
dudt=[(η+1−η1+sαz)[−(x2−θx)+(1−x−y)(2x−θ)]−βyc+δy−az]u+[−x(x−θ)(η+1−η1+sαz)−βxc(c+δy)2]v+[−x(1−x−y)(x−θ)α(1−η)(α+z)2−ax]w,dvdt=(βyc+δy)u+(cβx(c+δy)2−gz−μ)v+(−g¯y)w, anddwdt=−mw+b¯zu(t−τ)+b¯zv(t−τ)+b¯yw(t−τ). | (6.11) |
Taking Laplace transforms for (6.11), we obtain
sL{u}−u(0)=[(η+1−η1+sα¯z)[−(¯x2−θ¯x)+(1−¯x−¯y)(2¯x−θ)]−β¯yc+δ¯y−a¯z]L{u}+[−¯x(¯x−θ)(η+1−η1+sα¯z)−β¯xc(c+δ¯y)2]L{v}+[−¯x(1−¯x−¯y)(¯x−θ)α(1−η)(α+¯z)2−a¯x]L{w},sL{v}−v(0)=β¯yc+δ¯yL{u}+(cβ¯x(c+δ¯y)2−g¯z−μ)L{v}−g¯yL{w},sL{w}−w(0)=−mL{w}+b¯zL{u(t−τ)}+b¯zL{v(t−τ)}+b¯yL{w(t−τ)}. | (6.12) |
Here,L{u(t−τ)}=∫0−τe−s(t1+τ)u(t1)dt1+∫∞0e−s(t1+τ)u(t1)dt1=L1e−sτ+e−sτL{u(t)}. |
Similarly, L{v(t−τ)}=L2e−sτ+e−sτL{v(t)}and L{w(t−τ)}=L3e−sτ+e−sτL{w(t)}, |
where L2=∫0−τe−s(t1)v(t1)dt1=∫0−τe−s(t)v(t)dt and L3=∫0−τe−s(t1)w(t1)dt1=∫0−τe−s(t)w(t)dt.
If L{u(t)},L{v(t)} and L{w(t)} have poles with positive real parts, then the inverse Laplace transformation of L{u(t)},L{v(t)}, and L{w(t)} will have terms which exponentially increase with time. Thus, E∗ will be locally asymptotically stable if, and only if, all poles of L{u(t)},L{v(t)}, and L{w(t)} have negative real parts. Using the Nyquist criterion E∗ will be asymptotically stable if the following two conditions are satisfied [33,34]:
ReG(iw0)=0, | (6.13) |
ImG(iw0)>0, | (6.14) |
where G(s)=s3+P2s2+P1s+P0+e−sτ(Q2s2+Q1s+Q0)=0 and w0 is the smallest positive root of (6.13). Equating the real and imaginary parts, we get
P2w20−P0=(Q0−Q2w20)cos(w0τ)+Q1w0sin(w0τ), | (6.15) |
−w30+P1w0>sin(w0τ)(Q0−Q2w20)−Q1w0cos(w0τ). | (6.16) |
To find the length of maximum delay so that stability is preserved, we write (6.15) and (6.16) as
P2w2−P0=(Q0−Q2w2)cos(wτ)+Q1wsin(wτ), | (6.17) |
−w3+P1w>sin(wτ)(Q0−Q2w2)−Q1wcos(wτ). | (6.18) |
Equation (6.17) and inequality (6.18) are the conditions for stability and both should be satisfied simultaneously. We first find an upper bound w+, independent of τ, such that (6.18) is valid for w0≤w≤w+. We will later use this inequality to determine a range of feasible values for τ∗.
Since ∣cos(wτ)∣≤1 and ∣sin(wτ)∣≤1, using these inequalities in (6.17),
P2w2≤ ∣Q0−Q2w2∣+∣Q1∣w+∣P0∣,and soP2w2≤ ∣Q0∣+∣Q2w2∣+∣Q1∣w+∣P0∣. | (6.19) |
So,(∣P2∣−∣Q2∣)w2−∣Q1∣w−(∣Q0∣+∣P0∣)≤0. | (6.20) |
Let w+ denote the maximum value of w satisfying (6.18),
w+=∣Q1∣+√Q21+4(∣P2∣−∣Q2∣)(∣Q0∣+∣P0∣)2(∣P2∣−∣Q2∣), | (6.21) |
where w+≥w0. From (6.18), we get
w2<(Q2w−Q0w)sin(wτ)+Q1cos(wτ)+P1. | (6.22) |
Since E∗ is locally asymptotically stable for τ=0, by assumption, the inequality (6.22) will continue to hold for sufficiently small τ and w=w0. Using (6.17) and (6.22), we get
P2[(Q2wQ0w)sin(wτ)+Q1cos(wτ)+P1]−P0≥ (Q0−Q2w2)cos(wτ)+Q1wsin(wτ), |
or(Q1w+P2Q0w−P2Q2w)sin(wτ)+(P2Q1−Q0+Q2w2)(1−cos(wτ))≤P2P1−P0+P2Q1−Q0+Q2w2, |
where sin(wτ)≤wτ and 1−cos(wτ)≤2sin2(wτ2)≤w2τ22. So
(Q1w+P2Q0w−P2Q2w)sin(wτ)+(P2Q1−Q0+Q2w2)(1−cos(wτ)) ≤|Q1w+P2Q0w−P2Q2w| wτ+|P2Q1−Q0+Q2w2|w2τ22,≤(∣Q1−P2Q2∣w2+∣P2Q0∣)τ+∣Q2w2+P2Q1−Q0∣w2τ22,≤(∣Q1−P2Q2∣w2++∣P2Q0∣)τ+∣Q2w2+P2Q1−Q0∣w2+τ22, |
since w0≤w≤w+.
If(∣Q1−P2Q2∣w2++∣P2Q0∣)τ+∣Q2w2++P2Q1−Q0∣w2+τ22≤P2P1−P0+P2Q1−Q0+Q2w2+, |
then(Q1w++P2Q0w+−P2Q2w+)sin(w+τ)+(P2Q1−Q0+Q2w2+)(1−cos(w+τ))≤P2P1−P0+P2Q1−Q0+Q2w2+. |
Let ∣Q2w2++P2Q1−Q0∣w2+2=L1, ∣Q1−P2Q1∣w2++∣P2Q0∣=L2, and P2P1−P0+P2Q1−Q0+Q2w2+=L3. So, L1τ2+L2τ≤L3. Suppose τ∗ is the positive root of L1τ2+L2τ=L3, that is,
τ∗=−L2+√L22+4L1L32L1. |
Theorem 5. If 0<τ<τ∗ and the Nyquist criterion to the local asymptotic stability is satisfied, then τ∗ estimates the maximum delay length to preserve stability.
This paper has examined prey-predator models with the Allee effects and the cost of fear in prey reproduction, where the predator predates the prey with a Holling Type I functional response. We have investigated the dynamic behavior of the model mathematically, including the existence and stability of equilibria, the occurrence of Hopf-bifurcation around the coexistence equilibrium point, and the existence of a limit cycle that emerges from Hopf-bifurcation. We have described equilibria, the stabilities of the system, and the occurrence of Hopf-bifurcation. The coexistence equilibrium is locally asymptotically stable if twice the death rate of the predator is one unit higher than the Allee threshold value.
We have shown that as the fear level rises, the population of prey remains unaffected because, at N∗=d, the fear level has no effect. However, when the level of fear increases, the population of predators decreases. The existence of the coexistence equilibrium point E∗=(N∗,P∗) implies that θ<d<1. Since N∗=d, the Allee effect has no affect on the prey population, and the coexistence equilibrium E∗ is stable when it occurs for a small Allee effect. Furthermore, we have demonstrated that as the Allee threshold rises, the predator population rises. However, after certain values of the Allee threshold, both populations become unstable and then go extinct. If we take the death rate of the predator d as a bifurcation parameter, the system experiences Andronov-Hopf bifurcation. When the parameter d passes through the bifurcation value d0=1, our system experiences a transcritical bifurcation at steady state E1 according to Sotomayor's theorem [31]. The dynamics of the delayed system have been investigated and we have discussed the Hopf-bifurcation.
Finally, we have looked at an eco-epidemiological model that takes the cost of fear and the Allee effect into account in the prey population. We have examined a predator-prey model in which the prey is afflicted with a disease. The overall prey population is divided into two categories, susceptible prey and infected prey. When susceptible prey comes into contact with infected prey, it becomes infected. Infected prey is unable to procreate because it has the disease. The predator consumes both uninfected and infected prey, but infected prey is easy to capture whilst it takes more time to catch the uninfected prey. As a result, we have assumed that the consumption rate of infected prey is higher than that of uninfected prey. There are five biologically significant equilibria. We have discussed the stabilities of these equilibria. We have performed a numerical study to examine how the Allee effect and fear effect affect our system, and we have discovered that the population of uninfected prey and predator drops as the Allee threshold rises, whereas the population of infected prey rises. All populations become extinct when the Allee threshold reaches a specified value. We have demonstrated that when the fear intensity rises, the population of uninfected prey declines, and after a certain level of fear, there is no effect on the population of uninfected prey as the fear increases, and this is due to physiological effects when they get acclimatised to fear. The system becomes stable for an intermediate level of fear, and after a certain level of fear, the system exhibits instability because the predator population goes extinct, leaving only uninfected and infected prey, which increases disease transmission and leads to an increase in infected prey. We have found that the equilibria Ez and Ey have a Hopf-bifurcation under specific parametric conditions.
To make the model (2.1) more realistic, we have incorporated a time delay and Hopf-bifurcation occurs by taking the delay as the bifurcation parameter. Hopf-bifurcation will occur when the delay τ passes through a series of critical values. If 0<τ<τ∗ and the Nyquist criterion to the local asymptotic stability is true then τ∗ estimates the maximum delay length to preserve stability.
Hopf-bifurcation has helped us in finding the existence of a region of instability in the neighborhood of non-zero equilibrium, where prey and predator species will persist undergoing regular fluctuation. For the transcritical bifurcations a stable coexistence equilibrium E∗ combines with another equilibrium and disappears and the positive equilibrium E1 switches from instability to stability.
The authors declare they have not used Artificial Intelligence (AI) tools in the creation of this article.
Data sharing is not applicable to this article as no datasets were generated or analysed during the current study.
The authors declare that there is no conflict of interest.
ξ1= pδg(p−b)(−αbδg+bδημ+βηp),ξ2= (p−b)(b2δ2ηgμθ+bβδηgpθ+2bcδηgμp+βcηgp2−αb2δ2g2θ)(−2αbcδg2p)+(2p−b)(αbδ2g2m−bδ2ηgmμ)+(2b−3p)(βδηgmp)+bβδηgp2−αb2δ2g2p,ξ3= aαb3δ2gμ+2αb3cδg2θ−αb3δ2g2θ−2αb2cδg2pθ+αb2δ2g2mθ−2b3cδηgμθ+b3δ2ηgμθ+2b2cδηgμpθ−b2δ2ηgmμθ+aαb2βδgp−ab3δ2μ2−αb3βδg2+αb2c2g2p−2αb2cδg2m−2αb2cδg2p+αb2δ2g2m−αbc2g2p2+4αbcδg2mp−αbδ2g2m2−b2βcηgpθ+b2βδηgmθ+b2βδηgpθ−b2c2ηgμp+2b2cδg2mp−αbδ2g2m2−b2βcηgpθ+b2βδηgmθ+b2βδηgpθ−b2c2ηgμp+2b2cδηgmμ+2b2cδηgμp−b2δ2ηgmμ+bβcηgp2θ−2bβδηgmpθ+bc2ηgμp2−4bcδηgmμp+bδ2ηgm2μ−2ab2βδμp+b3βδgμ+2bβcηgmp+bβcηgp2−bβδηgm2−2bβδηgmp−3βcηgmp2+3βδηgm2p−abβ2p2+b2β2gp,ξ4= 2aαb3cδgμ+αb3c2g2θ−2αb3cδg2θ−αb2c2g2pθ+2αb2cδg2mθ−b3c2ηgμθ+2b3cδηgμθ+b2c2ηgμpθ−2b2cδηgmμθ+aαb2βcgp−aαb2δgm−2ab3cδμ2−αb3βcg2−αb2c2g2m−αb2c2g2p+2αb2cδg2m+2αbc2g2mp−2αbcδg2m2+b2βcηgmθ+b2βcηgpθ−b2βδηgmθ+b2c2ηgmμ+b2c2ηgμp−2b2cδηgmμ−2bβcηgmpθ+bβδηgm2θ−2bc2ηgmμp+2bcδηgm2μ−2ab2βcμp+2ab2βδmμ+b3βcgμ−bβcηgm2−2bβcηgmp+bβδηgm2+3βcηgm2p−βδηgm3+2abβ2mp−b2β2gm,ξ5= aαb3c2gμ−αb3c2g2θ+αb2c2g2mθ+b3c2θθηgμθ−b2c2ηgmμθ−aαb2βcgm−ab3c2μ2+αb2c2g2m−αbc2g2m2−b2βcηgmθ−b2c2ηgmμ+bβcηgm2θ+bc2ηgm2μ+2ab2βcmμ+bβcηgm2−βcηgm3−abβ2m2,ξ5= (1−bm)(αb2c2g2θm+bβcηgm2θ+bc2ηηgm2μ−b2c2ηgmμθ)(−αbc2g2m2−βcηgm3)+aαb3c2gμ−aαb2βcgm−ab3c2μ2+2ab2βcmμ−abβ2m2. |
[1] |
H. Ylönen, Weasels Mustela nivalis suppress reproduction in cyclic bank voles Clethrionomys glareolus, Oikos, 55 (1989), 138–140. https://doi.org/10.2307/3565886 doi: 10.2307/3565886
![]() |
[2] |
S. L. Lima, Stress and decision-making under the risk of predation: recent developments from behavioral, reproductive, and ecological perspectives, Adv. Stud. Behav., 27 (1998), 215–290. https://doi.org/10.1016/S0065-3454(08)60366-6 doi: 10.1016/S0065-3454(08)60366-6
![]() |
[3] |
L. Y. Zanette, A. F. White, M. C. Allen, M. Clinchy, Perceived predation risk reduces the number of offspring songbirds produce per year, Science, 334 (2011), 1398–1401. https://doi.org/10.1126/science.1210908 doi: 10.1126/science.1210908
![]() |
[4] | H. Ylönen, B. Jedzrejewska, W. Jedrzejewski, J. Heikilla, Antipredatory behaviour of Clethrionomys voles–'David and Goliath' arms race, Ann. Zool. Fenn., 29 (1992), 207–216. |
[5] |
W. Cresswell, Predation in bird populations, J. Ornithol., 152 (2011), 251–263. https://doi.org/10.1007/s10336-010-0638-1 doi: 10.1007/s10336-010-0638-1
![]() |
[6] |
E. L. Preisser, D. I. Bolnick, The many faces of fear: comparing the pathways and impacts of nonconsumptive predators effects on prey populations, PLoS One, 3 (2008), e2465. https://doi.org/10.1371/journal.pone.0002465 doi: 10.1371/journal.pone.0002465
![]() |
[7] |
H. Ronkainen, H. Ylönen, Behaviour of cyclic bank voles under risk of mustelid predation: do females avoid copulations?, Oecologia, 97 (1994), 377–381. https://doi.org/10.1007/BF00317328 doi: 10.1007/BF00317328
![]() |
[8] |
H. Ylönen, Vole cycles and anti predatory behaviour, Trends Ecol. Evol., 9 (1994), 426–430. https://doi.org/10.1016/0169-5347(94)90125-2 doi: 10.1016/0169-5347(94)90125-2
![]() |
[9] |
H. Ylönen, H. Ronkainen, Breeding suppression in the bank vole as anti predatory adaptation in a predictable environment, Evol. Ecol., 8 (1994), 658–666. https://doi.org/10.1007/BF01237848 doi: 10.1007/BF01237848
![]() |
[10] |
A. Kumar, B. Dubey, Modeling the effect of fear in a prey-predator system with prey refuge and gestation delay, Int. J. Bifurcat. Chaos, 29 (2019), 1950195. https://doi.org/10.1142/S0218127419501955 doi: 10.1142/S0218127419501955
![]() |
[11] |
M. Das, G. P. Samanta, Prey-predator fractional order model with fear effect and group defense, Int. J. Dynam. Control, 9 (2021), 334–349. https://doi.org/10.1007/s40435-020-00626-x doi: 10.1007/s40435-020-00626-x
![]() |
[12] |
X. Wang, L. Zanette, X. Zou, Modelling the fear effect in predator-prey interactions, J. Math. Biol., 73 (2016), 1179–1204. https://doi.org/10.1007/s00285-016-0989-1 doi: 10.1007/s00285-016-0989-1
![]() |
[13] |
X. Wang, X. Zou, Modeling the fear effect in predator-prey interactions with adaptive avoidance of predators, Bull. Math. Biol., 79 (2017), 1325–1359. https://doi.org/10.1007/s11538-017-0287-0 doi: 10.1007/s11538-017-0287-0
![]() |
[14] |
S. Mondal, A. Maiti, G. P. Samanta, Effects of fear and additional food in a delayed predator-prey model, Biophys. Rev. Lett., 13 (2018), 157–177. https://doi.org/10.1142/S1793048018500091 doi: 10.1142/S1793048018500091
![]() |
[15] |
H. Zhang, Y. Cai, S. Fu, W. Wang, Impact of the fear effect in a prey-predator model incorporating a prey refuge, Appl. Math. Comput., 356 (2019), 328–337. https://doi.org/10.1016/j.amc.2019.03.034 doi: 10.1016/j.amc.2019.03.034
![]() |
[16] |
J. Wang, Y. Cai, S. Fu, W. Wang, The effect of the fear factor on the dynamics of a predator-prey model incorporating the prey refuge, Chaos Interdiscip. J. Nonlinear Sci., 29 (2019), 083109. https://doi.org/10.1063/1.5111121 doi: 10.1063/1.5111121
![]() |
[17] |
D. Duan, B. Niu, J. Wei, Hopf-hopf bifurcation and chaotic attractors in a delayed diffusive predator-prey model with fear effect, Chaos Soliton. Fract., 123 (2019), 206–216. https://doi.org/10.1016/j.chaos.2019.04.012 doi: 10.1016/j.chaos.2019.04.012
![]() |
[18] |
N. Pal, S. Samanta, J. Chattopadhyay, Revisited Hastings and Powell model with omnivory and predator switching, Chaos Soliton. Fract., 66 (2014), 58–73. https://doi.org/10.1016/j.chaos.2014.05.003 doi: 10.1016/j.chaos.2014.05.003
![]() |
[19] |
S. Pal, N. Pal, S. Samanta, J. Chattopadhyay, Fear effect in prey and hunting cooperation among predators in a Leslie-Gower model, Math. Biosci. Eng., 16 (2019), 5146–5179. https://doi.org/10.3934/mbe.2019258 doi: 10.3934/mbe.2019258
![]() |
[20] |
A. Das, G. P. Samanta, Modeling the fear effect on a stochastic prey-predator system with additional food for the predator, J. Phys. A: Math. Theor., 51 (2018), 465601. https://doi.org/10.1088/1751-8121/aae4c6 doi: 10.1088/1751-8121/aae4c6
![]() |
[21] |
P. Panday, N. Pal, S. Samanta, J. Chattopadhyay, Stability and bifurcation analysis of a three-species food chain model with fear, Int. J. Bifurcat. Chaos, 28 (2018), 1850009. https://doi.org/10.1142/S0218127418500098 doi: 10.1142/S0218127418500098
![]() |
[22] |
A. Sha, S. Samanta, M. Martcheva, J. Chattopadhyay, Backward bifurcation, oscillations and chaos in an eco-epidemiological model with fear effect, J. Biol. Dynam., 13 (2019), 301–327. https://doi.org/10.1080/17513758.2019.1593525 doi: 10.1080/17513758.2019.1593525
![]() |
[23] |
S. Samaddar, M. Dhar, P. Bhattacharya, Effect of fear on prey-predator dynamics: Exploring the role of prey refuge and additional food, Chaos Interdiscip. J. Nonlinear Sci., 30 (2020), 063129. https://doi.org/10.1063/5.0006968 doi: 10.1063/5.0006968
![]() |
[24] |
V. Kumar, N. Kumari, Stability and bifurcation analysis of Hassell-Varley prey-predator system with fear effect, Int. J. Appl. Comput. Math., 6 (2020), 150. https://doi.org/10.1007/s40819-020-00899-y doi: 10.1007/s40819-020-00899-y
![]() |
[25] |
Y. Huang, Z. Zhu, Z. Li, Modeling the Allee effect and fear effect in predator-prey system incorporating a prey refuge, Adv. Differ. Equations, 2020 (2020), 321. https://doi.org/10.1186/s13662-020-02727-5 doi: 10.1186/s13662-020-02727-5
![]() |
[26] |
K. Sarkar, S. Khajanchi, Impact of fear effect on the growth of prey in a predator-prey interaction model, Ecol. Compl., 42 (2020), 100826. https://doi.org/10.1016/j.ecocom.2020.100826 doi: 10.1016/j.ecocom.2020.100826
![]() |
[27] |
W. M. Liu, H. W. Hethcote, S. A. Levin, Dynamical behavior of epidemiological models with nonlinear incidence rates, J. Math. Biology, 25 (1987), 359–380. https://doi.org/10.1007/BF00277162 doi: 10.1007/BF00277162
![]() |
[28] | R. O. Peterson, R. E. Page, Wolf Density as a Predictor of Predation Rate, Swedish Wildlife Research, Sweden, 1987. |
[29] |
Q. J. A. Khan, E. Balakrishnan, G. C. Wake, Analysis of a predator-prey system with predator switching, Bull. Math. Biol., 66 (2004), 109–123. https://doi.org/10.1016/j.bulm.2003.08.005 doi: 10.1016/j.bulm.2003.08.005
![]() |
[30] |
P. K. Tiwari, K. A. N. A. Amri, S. Samanta, Q. J. A. Khan, J. Chattopadhyay, A systematic study of autonomous and nonautonomous predator-prey models with combined effects of fear, migration and switching, Nonlinear Dyn., 103 (2021), 2125–2162. https://doi.org/10.1007/s11071-021-06210-y doi: 10.1007/s11071-021-06210-y
![]() |
[31] | L. Perko, Differential Equations and Dynamical Systems, Springer, New York, 2001. |
[32] | S. N. Chow, J. K. Hale, Methods of bifurcation theory, Springer-Verlag, New York, 1982. |
[33] |
L. H. Erbe, H. I. Freedman, V. S. H. Rao, Three-species food-chain models with mutual interference and time delays, Math. Biosci., 80 (1986), 57–80. https://doi.org/10.1016/0025-5564(86)90067-2 doi: 10.1016/0025-5564(86)90067-2
![]() |
[34] | V. Geetha, S. Balamuralitharan, Hopf bifurcation analysis of nonlinear HIV infection model and the effect of delayed immune response with drug therapies, Bound. Value Probl., 2020 (2020). https://doi.org/10.1186/s13661-020-01410-8 |