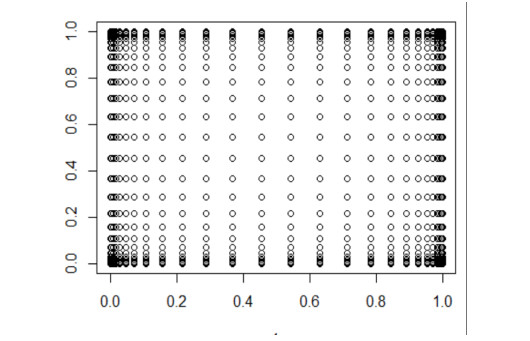
In this paper, we propose a beta kernel estimator to measure functional dependence (MFD). The MFD not only can measure the strength of linear or monotonic relationships, but it is also suitable for more complicated functional dependence. We derive the asymptotic distribution of the proposed estimator and then use several simulated examples to compare our estimator with the traditional measures. Our simulation results demonstrate that beta kernel provides high accuracy in estimation. A real data example is also given to illustrate one possible application of the new estimator.
Citation: Qingsong Shan, Qianning Liu. Nonparametric estimation of the measure of functional dependence[J]. AIMS Mathematics, 2021, 6(12): 13488-13502. doi: 10.3934/math.2021782
[1] | Gaosheng Liu, Yang Bai . Statistical inference in functional semiparametric spatial autoregressive model. AIMS Mathematics, 2021, 6(10): 10890-10906. doi: 10.3934/math.2021633 |
[2] | Zouaoui Chikr Elmezouar, Fatimah Alshahrani, Ibrahim M. Almanjahie, Salim Bouzebda, Zoulikha Kaid, Ali Laksaci . Strong consistency rate in functional single index expectile model for spatial data. AIMS Mathematics, 2024, 9(3): 5550-5581. doi: 10.3934/math.2024269 |
[3] | Oussama Bouanani, Salim Bouzebda . Limit theorems for local polynomial estimation of regression for functional dependent data. AIMS Mathematics, 2024, 9(9): 23651-23691. doi: 10.3934/math.20241150 |
[4] | H. M. Barakat, M. A. Alawady, I. A. Husseiny, M. Nagy, A. H. Mansi, M. O. Mohamed . Bivariate Epanechnikov-exponential distribution: statistical properties, reliability measures, and applications to computer science data. AIMS Mathematics, 2024, 9(11): 32299-32327. doi: 10.3934/math.20241550 |
[5] | Fatimah Alshahrani, Wahiba Bouabsa, Ibrahim M. Almanjahie, Mohammed Kadi Attouch . $ k $NN local linear estimation of the conditional density and mode for functional spatial high dimensional data. AIMS Mathematics, 2023, 8(7): 15844-15875. doi: 10.3934/math.2023809 |
[6] | Mashael A. Alshehri, Mohamed Kayid . Copulas generated by mixtures of weighted distributions. AIMS Mathematics, 2022, 7(5): 8953-8974. doi: 10.3934/math.2022499 |
[7] | Fatimah Alshahrani, Wahiba Bouabsa, Ibrahim M. Almanjahie, Mohammed Kadi Attouch . Robust kernel regression function with uncertain scale parameter for high dimensional ergodic data using $ k $-nearest neighbor estimation. AIMS Mathematics, 2023, 8(6): 13000-13023. doi: 10.3934/math.2023655 |
[8] | Xiao Zhang, Rongfang Yan . Stochastic comparisons of extreme order statistic from dependent and heterogeneous lower-truncated Weibull variables under Archimedean copula. AIMS Mathematics, 2022, 7(4): 6852-6875. doi: 10.3934/math.2022381 |
[9] | Yue Li, Yunyan Wang . Strong consistency of the nonparametric kernel estimator of the transition density for the second-order diffusion process. AIMS Mathematics, 2024, 9(7): 19015-19030. doi: 10.3934/math.2024925 |
[10] | Xueping Hu, Jingya Wang . A Berry-Ess$\acute{e}$n bound of wavelet estimation for a nonparametric regression model under linear process errors based on LNQD sequence. AIMS Mathematics, 2020, 5(6): 6985-6995. doi: 10.3934/math.2020448 |
In this paper, we propose a beta kernel estimator to measure functional dependence (MFD). The MFD not only can measure the strength of linear or monotonic relationships, but it is also suitable for more complicated functional dependence. We derive the asymptotic distribution of the proposed estimator and then use several simulated examples to compare our estimator with the traditional measures. Our simulation results demonstrate that beta kernel provides high accuracy in estimation. A real data example is also given to illustrate one possible application of the new estimator.
The study of association or dependence plays an important role in statistics. One of the important aspects of this is how to measure the strength of various associations among random variables. Among the measures of associations between random variables, Pearson's correlation coefficient, Spearman's ρ, and Kendall's τ are the most prominent ones. But they only measure linear or monotonic relationships, not suitable for a general nonlinear relationship. For example, when the relationship between two random variables is parabolic-shaped, none of the measures above will be applicable. Thus, a measure addressing this issue is desirable.
Two random variables are said to be mutually complete dependent (MCD) when they have mutual functional relationship. This concept was first introduced by Lancaster [1]. This is also known as the strongest dependence. In this relation, one variable is completely predictable of the other. Siburg and Stoimenov [2] constructed a measure of MCD for continuous random variables. Tasena and Dhompongsa [3] extended this measure to the multivariate case. In their papers, they proposed to measure the distance between two copulas by a modified Sobolev norm. For details about inner product and Sobolev norm on copula space, we refer to Darsow and Olsen [4].
Dette et al. [5] found that a simple modification of the measure of MCD can be used to measure the strength of functional dependence (MFD). Note that this functional dependence includes a wider range of dependence since it could be nonlinear or even nonmonotonic. The discrete form of MFD was given in Shan et al. [6]. Given the theoretical definition of MFD, the next question will be how to estimate it. Similar to the measure of MCD, MFD is also constructed based on copula. So a straightforward way of estimating MFD contains two steps. First, estimating the copula or its density. Second, using the estimated copula or its density to estimate the MFD. Generally in step one, there are two approaches to estimate the copula or its density, parametric way or nonparametric way. In the parametric way, one assumes a parametric model for copula, and estimates its parameters by the method of maximum likelihood (MLE). However, unlike distribution functions, the dependence structure, i.e., the copula, is usually hidden behind the data set, which makes the claim of having prior knowledge of copula family quite questionable. So in this paper, we will mainly consider the nonparametric way.
In this article, we propose estimating the MFD using the kernel method with the beta kernel. We introduce the new estimators and study their asymptotic properties in Section 3. Using Monte Carlo simulations, we investigate the finite sample performance of the proposed estimators relative to traditional measures of dependence. Our simulation results, reported in Section 4, show that the new estimators are accurate and stable with the choice of different parameters in a given model. In Section 5, a real data example is used to illustrate the new estimators. The paper is concluded by some remarks in Section 6.
This section introduces some notations and concepts that will be used in the remainder of the article. We will focus on bivariate continuous distributions. Consider an independent and identically distributed sample (X1,Y1),⋯,(Xn,Yn) of a bivariate random vector (X,Y) with joint distribution function H, and marginal distribution functions F and G, respectively. Then, by Sklar's Theorem [7], there exists a unique copula C:I2=[0,1]2→I=[0,1] such that
H(x,y)=C(F(x),G(y)). | (2.1) |
Therefore, the copula density is given by
c(F(x),G(y))=∂2∂x∂yC(F(x),G(y)). | (2.2) |
The measure of MCD is based on the norm of functions in the copula space C. The Sobolev norm for copula takes the following form
‖C‖2=∫10∫10[(∂C∂u)2+(∂C∂v)2]dudv. | (2.3) |
The above norm has the following properties.
Proposition 1. The Sobolev norm for copulas satisfies ‖C‖2∈[2/3,1] for all C∈C. Moreover, the following properties hold:
i. ‖C‖2=2/3 if and only if X and Y are independent.
ii. ‖C‖2=1 if and only if X and Y are MCD.
Inspired by this proposition, Siburg and Stoimenov [2] proposed the measure of MCD, which can be defined as follows.
Definition 1. Given two continuous random variables X,Y with copula C, we define
ρ(X,Y)=(3||C||2−2)1/2. | (2.4) |
ρ(X,Y) can be interpreted as a normalized Sobolev distance of C from the independent copula denoted by Π:
ρ(X,Y)=√3||C−P||=||C−Π||||Cm−Π||, |
where Cm is a MCD copula. A close look at the MCD shows that it can be decomposed into two opposite functional dependencies, i.e., Y is a function of X and X is a function of Y. Therefore, the measure of functional dependence (MFD) can be derived by modifying the measure for MCD, which is the main idea of Dette et al. [5]. Its discrete form was discussed in Shan et al. [6]. The construction of MFD is based on the following propositions.
Proposition 2. Let X and Y be two random variables with copula C. Then,
i.X and Y are independent if and only if ∂1CX,Y(u,v)=v for Lebesgue almost all (u,v)∈I2.
ii.Y is almost surely (a.s.) a Borel function of X if and only if ∂1CX,Y(u,v)∈{0,1} for Lebesgue almost all (u,v)∈I2.
Proposition 3. For any CX,Y∈C, we have ‖∂1CX,Y‖22∈[1/3,1/2], Moreover,
i.‖∂1CX,Y‖22=1/3 if and only if X and Y are independent.
ii.‖∂1CX,Y‖22=1/2 if and only if Y is a.s. a Borel function of X.
Notice that ‖∂1CX,Y‖ reaches its boundaries at two extreme cases. ‖∂2CX,Y‖ has similar propositions which we will not reproduce here. Using these definitions and propositions, we can define
ρ21(Y|X)=6∫10∫10(∂C∂u)2dudv−2, | (2.5) |
ρ22(X|Y)=6∫10∫10(∂C∂v)2dudv−2. | (2.6) |
This is a standardized form of ‖∂1CX,Y‖. Hence ρ1 inherits similar properties:
i. ρ1=0 if and only if X and Y are independent.
ii. ρ1=1 if and only if Y is a.s. a Borel function of X.
Similarly, ρ2=1 indicates X is a.s. a Borel function of Y. Those properties suggest that ρi(i=1,2) can be used to measure functional dependence. We can assess the strength of dependence from the magnitude of ρi.
The measures ρ1, ρ2 and ρ are all constructed based on copulas. They both can be stated in terms of copulas or copula densities. In other words, (2.5) can be written as
ρ21=6∫10∫10(∫v0c(u,y)dy)2dudv−2, | (3.1) |
and (2.6) can be written as
ρ22=6∫10∫10(∫u0c(x,v)dx)2dudv−2. | (3.2) |
Accordingly, there are two approaches to estimate the measures, through copula or its density. We will focus on the latter in this paper.
The estimation of the copula density has been discussed in many papers. For example, Kauermann et al. [8] estimated copula density with B-spline. Genest et al. [9] estimated copula density through wavelets. It is recognized that the estimation of copula density involves more technical difficulties than usual density estimation. One of the big issues in this respect is boundary bias. Several methods have been proposed to address this issue. Omelka et al. [10] suggested an improved version of mirror-reflection estimator. Charpentier et al. [11] suggested to use transformation estimator. Chen [12] suggested to use beta kernels whose support matches the support of copulas. Geenens et al. [13] used probit transformation to reduce boundary bias effect in kernel estimation of copula density. Majdara and Nooshabadi [14] provides a novel method in estimating copula density in high dimension space.
Dette et al. [5] discussed the asymptotic behavior of the estimation ρ1 based on symmetric kernels and Eq (2.5). In this paper, we will use the beta kernel in estimating ρ1 and ρ2. Beta kernel smoothing was considered by Harrell and Davis [15]. Chen [12], Chen [16] applied beta kernel smoothing in density estimation, and found that the beta estimator can reduce boundary bias and variance compared with local linear estimators for densities with finite support. Following the same idea, Charpentier et al. [11] proposed the beta kernel based estimator for copula density.
Let (X1,Y1),(X2,Y2),⋯(Xn,Yn) be a sample from HX,Y(x,y) with unknown marginals. Denote the copula corresponding to HX,Y(x,y) by C(u,v). We assume that both H and C are completely unknown. We start by the most convenient situation in which we assume that the copula C is twice differentiable. Let c denote the density of the copula. Usually copulas are estimated via pseudo-observations (ˆF(Xi),ˆG(Yi)), where ˆF and ˆG are the empirical distribution functions, i.e.,
U=ˆF(x)=1n+1n∑i=11(Xi≤x),andV=ˆG(y)=1n+1n∑i=11(Yi≤y), | (3.3) |
with 1(A) being the usual indicator function. The beta kernel based estimator of the copula density at point (u,v)∈[0,1]2 is
ˆch(u,v)=1nn∑i=1K(Ui,uh+1,1−uh+1)K(Vi,vh+1,1−vh+1), |
where K(⋅,α,β) is the density of the beta distribution with parameters α and β, i.e.,
K(x,α,β)=xα(1−x)βB(α,β),x∈[0,1], |
with B(α,β)=Γ(α+β)/Γ(α)Γ(β). For convenience, the same bandwidth is used in both kernels in ˆch(u,v). Charpentier et al. [11] claimed the asymptotic normality of ˆch(u,v)by showing that, for all (u,v)∈[0,1]2,
√nh[ˆch(u,v)−c(u,v)]L→N(0,σ(u,v)2), |
as nh→∞ and h→0, where "L→" means convergence in distribution. Nagler [17] provided detailed proof and gave the bias and variance of ˆch(u,v) in Proposition 4. He also discussed bandwidth selection for ˆch(u,v).
Proposition 4. Let c(u,v) be twice continuously differentiable on (0,1)2, and hn→0 and nhn→∞ as n→∞. Then, for all (u,v)∈(0,1)2,
Bias[ˆch(u,v)]=hn[(1−2u)cu(u,v)+(1−2v)cv(u,v)+12u(1−u)cuu(u,v)+12v(1−v)cvv(u,v)+o(hn), |
Var[ˆch(u,v)]=14nhhπc(u,v)√u(1−u)v(1−v)+o(1nhn). |
Note that there is a little difference between Proposition 4, which is on the interior of (0,1)2, and Charpentier's claim [11], which is on the whole [0,1]2. Since the definition of functional dependence is based on the integration of copula densities, which will not be affected by the boundaries. We will consider the whole [0,1]2 in the remainder.
Since all of the three measures, ρ1, ρ2 and ρ, are constructed in similar manner, we only take ρ1 as an example and show how to estimate it through estimating the copula density using the beta kernel. Let ℓ∞([0,1]2) be the space of the collection of all uniformly bounded real-valued functions defined on [0,1]2, equipped with the uniform metric m defined as
m(f1,f2)=supx∈[0,1]2|f1(x)−f2(x)|,f1,f2∈ℓ∞([0,1]2). | (3.4) |
Define ϕi: ℓ∞([0,1]2)→R, i=1,2, by
ϕ1:c(u,v)→∫10∫10(∫v0c(u,y)dy)2dudv, |
ϕ2:c(u,v)→∫10∫10(∫u0c(x,v)dx)2dudv. |
Then, the three measures, ρ21, ρ21 and ρ2, are functionals of c(⋅,⋅). So, it suffices to show that ϕ1 and ϕ2 are Hadamard differentiable.
Theorem 1. Let c(u,v) be twice continuously differentiable on [0,1]2, and h→0 and nh→∞ as n→∞. Then,
√nh(ˆρ21−ρ21)L→ϕ′1(N(0,σ2(u,v))), |
where ϕ′1(l(u,y))=∫10∫10(2∫v0c(u,y)dy∫v0l(u,y)dy)dudv.
Proof. ρ1, ρ2 can be represented as a map ϕ1, ϕ2: ℓ∞([0,1]2)→R([0,1]) via ρ1=ϕ1(c) and ρ2=ϕ2(c), respectively. The function space ℓ∞([0,1]2) is equipped with the uniform metric m. For all converging sequences tn→0 and ln→l such that c+tnln∈ℓ∞([0,1]2) for every n, we have
ϕ1(c+tnln)−ϕ1(c)tn=1tn∫10∫10[(∫v0(c(u,y)+tnln(u,y))dy)2−(∫v0(c(u,y))dy)2]dudv=∫10∫101tn(∫v0(c(u,y)+tnln(u,y))dy+∫v0c(u,y)dy)⋅(∫v0(c(u,y)+tnln(u,y))dy−∫v0c(u,y)dy)dudv=∫10∫10(∫v0(c(u,y)+tnln(u,y))dy+∫v0c(u,y)dy)⋅(∫v0(ln(u,y))dy)dudv. |
So, the Hadamard derivative of ϕ1 at c is
ϕ′1(h)=∫10∫10(2∫v0c(u,y)dy∫v0l(u,y)dy)dudv. |
Therefore, according to the Delta method [18],
ϕ′1(N(0,σ(u,v)2))=∫10∫10(2∫v0c(u,y)dy∫v0N(0,σ(u,y)2)dy)dudv. |
This completes the proof.
The asymptotic distributions of ˆρ21 and ˆρ2 can be derived in exactly the same manner, so we omit their details.
In the following, we show that estimators of MFD through copula have the same asymptotic distributions as those established through copula density. As an example, let's check the asymptotic distribution of ˆρ2.
Mapping a copula density to an MFD can be decomposed into two steps as follows
cφ→Cψ→MFD. |
The first map is a double-integration that is linear and continuous, and thus, it is Hadamard-differentiable. We only need to check the second map.
Let D12([0,1]2) be the Sobolev space, D1,D2∈D12([0,1]2), and define the inner product
⟨D1,D2⟩=∫[0,1]2▽D1⋅▽D2dλ, |
where ▽ is gradient. The Sobolev norm induced by the inner product in D12([0,1]2) is
|D|2=⟨D,D⟩=∫[0,1]2[(∂D∂u)2+(∂D∂v)2]dudv, |
for D∈D12([0,1]2).
Let C⊂D12([0,1]2) be the copula space and C∈C is a copula. Define ψ:C↦R by ψ(C)=|C|2. Then, the derivative of ψ at C along D is:
limn→∞1tn(ψ(C+tnHn)−ψ(C))=limn→∞1tn(⟨C+tnHn,C+tnHn⟩−⟨C,C⟩)=limn→∞1tn(⟨C,C⟩+2⟨tnHn,C⟩+⟨tnHn,tnHn⟩−⟨C,C⟩)=limn→∞2⟨Hn,C⟩=2⟨H,C⟩. |
The last step follows from Theorem 2.3 in [19]. This result shows the convergence of ˆρ2, and straightforward calculations will show that it is consistent with the asymptotic distribution in Theorem 1.
In copula density estimation, Nagler [20] suggested using a grid that is equally spaced after a transformation by the inverse Gaussian cdf, which is shown in Figure 1. Our simulation results below show that evaluating copula density at a set of grid points in a similar pattern will improve the accuracy of estimators of MCD. To compare the impact of the choice of grid, we considered two copula families, the Gaussian copula with parameters 0,0.1,0.2,0.5,0.8,0.9 and the Gumbel copula with parameters 1,10/9,10/7,10/3,5,10. Two samples of sizes 200 and 1000 were taken from each copula, respectively. First, copula densities are estimated from each sample based on the KDEcopula package. Then, the estimated copula density was evaluated on two sets of grid points: the usual grid with equally spaced points and a normalized grid. From the discretized copula density, we calculate the estimate of MFD. Figures 2 and 3 show the mean absolute error (MAE) of estimators with sample size 200 and 1000 under 500 replication. In each case, we find the MAE of estimators based on an equally spaced grid, labeled "equal", are significantly higher than the MAE of the same estimators based on the transformed grid, labeled "norm".
In this section, we explore the finite sample performance of the proposed estimators using the mean squared error (MSE). To put all estimators on the same scale, we standardize MFD. In other words, we use ρ1, ρ2 and ρ. The corresponding estimators will be denoted by ˆρ1(Y∣X), ˆρ2(X∣Y) and ˆρ(X,Y). For two-dimensional density estimation, using cross-validation to choose the bandwidth is computationally expensive. Therefore, in all simulations, a rule-of-thumb bandwidth is used. More precisely, the bandwidth is selected based on the asymptotic mean integrated squared error (AMISE)-optimality with respect to the Frank copula. For further details on bandwidth selection in this context, we refer to Nagler [20]. In all simulations reported here, the integration is calculated over a grid of 30×30 points. For the choice of the grid, we adopt the method in Nagler [20]. That is, we apply the Gaussian cumulative distribution function to equally spaced 30 knots on a line segment [−3,3]. The final two-dimensional grid is shown in Figure 1. This choice takes into account the fact that copula densities usually have high fluctuation on the boundary and corners. Putting more evaluating points on those regions will reduce approximation errors.
In Tables 1 and 2, we present the simulated MSE of the estimators of ρ1 and ρ2 for samples size 50, 100 and 200 for Gaussian copula. These results are based on 1000 replications. Both copulas are generated by R package "copula". We find that our estimators have reasonable precision in all cases. As the sample size increases, MSE is getting smaller. And there is no significant difference in MSE for different θ values, which indicates our estimator is stable for the choice of θ's.
n=50 | n=100 | n=200 | ||
θ=0 | ˆρ1(Y∣X) | 2.3×10−3 | 1.5×10−3 | 8.0×10−4 |
ˆρ2(X∣Y) | 2.3×10−3 | 1.5×10−3 | 7.3×10−3 | |
θ=0.3 | ˆρ1(Y∣X) | 6.1×10−3 | 3.8×10−3 | 2.2×10−3 |
ˆρ2(X∣Y) | 6.2×10−3 | 3.8×10−3 | 2.1×10−3 | |
θ=0.6 | ˆρ1(Y∣X) | 8.8×10−3 | 4.2×10−3 | 2.1×10−3 |
ˆρ2(X∣Y) | 8.9×10−3 | 4.1×10−3 | 2.1×10−3 | |
θ=0.9 | ˆρ1(Y∣X) | 2.5×10−3 | 1.1×10−3 | 4.9×10−4 |
ˆρ2(X∣Y) | 2.3×10−3 | 1.1×10−3 | 5.0×10−4 |
n=50 | n=100 | n=200 | ||
θ=0.2 | ˆρ1(Y∣X) | 3.7×10−3 | 2.3×10−3 | 1.8×10−3 |
ˆρ2(X∣Y) | 3.7×10−3 | 2.3×10−3 | 1.8×10−3 | |
θ=0.5 | ˆρ1(Y∣X) | 7.2×10−3 | 4.0×10−3 | 2.3×10−3 |
ˆρ2(X∣Y) | 7.2×10−3 | 4.1×10−3 | 2.3×10−3 | |
θ=1 | ˆρ1(Y∣X) | 9.3×10−3 | 4.9×10−3 | 2.6×10−3 |
ˆρ2(X∣Y) | 9.3×10−3 | 4.9×10−3 | 2.5×10−3 | |
θ=2 | ˆρ1(Y∣X) | 7.5×10−3 | 3.5×10−3 | 1.8×10−3 |
ˆρ2(X∣Y) | 7.4×10−3 | 3.5×10−3 | 1.9×10−3 | |
θ=5 | ˆρ1(Y∣X) | 3.1×10−3 | 1.2×10−3 | 6.1×10−4 |
ˆρ2(X∣Y) | 3.1×10−3 | 1.2×10−3 | 6.0×10−4 |
In the second part of the simulation, we will compare the performance of MFDs with other measures of dependence, e.g., linear correlation coefficient r, Spearman's ρ and Kendall's τ under several different types of relationships. We choose three different dependence structures: elliptical distributions, monotonic dependence and regressional dependence, represented by normal copula, cubic function and quadratic function, respectively.
The first example is a quadratic function. 500 data are generated from the following model,
Y=X2+ε, | (4.1) |
where ε∼N(0,σ) and σ=1, 5, and 10, respectively (see Figure 4). To obtain the copula data, we apply the empirical marginal distributions to the data, i.e., apply a rank transformation as shown in (3.3) to the data generated by the model (4.1). Then beta kernel estimation is applied to get the estimations of ρ1 and ρ2.
Obviously, neither Spearman's ρ nor Kendall's τ is suitable for this situation. The simulation results in Table 3 also showed that they are almost 0 in all cases. ˆρ(X,Y), on the other hand, is much higher than both Spearman's ρ and Kendall's τ, especially for small σ. This indicates that the type of dependence is functional, not monotonic. And the magnitude of ˆρ(X,Y) tells that the strength of dependence is getting weaker as σ increases. In a comparison of ˆρ1(Y∣X) and ˆρ2(X∣Y), we find that functional dependence is stronger in the Y to X direction than the other direction since ˆρ1(Y∣X) is higher. Again, as σ increases, the strength of dependence in this direction is also getting weaker.
ˆρ1(Y∣X) | ˆρ2(X∣Y) | ˆρ(X,Y) | Spearman's ρ | Kendall's τ | |
σ=1 | 0.51 | 0.25 | 0.40 | -0.01 | -0.02 |
σ=5 | 0.41 | 0.20 | 0.32 | -0.02 | -0.02 |
σ=10 | 0.27 | 0.13 | 0.21 | -0.01 | -0.01 |
To compare the performance of MFD with Kendall's τ and Spearman's ρ in monotonic dependence. 500 data are generated from the following model,
Y=X3+ε, | (4.2) |
where ε∼N(0,σ) and σ=1, 5 and 10. The scatter plot of model (4.2) is in Figure 5 and the simulation results are in Table 4. As shown in Table 4, the values of MFD has a similar decreasing pattern as the other two measures when σ increases. Indeed, a cubic function is one type of functional dependence, so MFDs are capable of measuring the strength of monotonic dependence. The values of ˆρ1(Y∣X) and ˆρ2(X∣Y), which measure the strength of functional dependence in two directions (Y to X and X to Y) separately, are close to each other, obviously this is because model (4.2) is symmetric.
ˆρ1(Y∣X) | ˆρ2(X∣Y) | ˆρ(X,Y) | Spearman's ρ | Kendall's τ | |
σ=1 | 0.89 | 0.89 | 0.89 | 0.82 | 0.95 |
σ=5 | 0.63 | 0.62 | 0.63 | 0.55 | 0.74 |
σ=10 | 0.42 | 0.41 | 0.41 | 0.35 | 0.50 |
Next, we take into account the Pearson's correlation coefficient. We take normal copulas as an example, which is
Cr(u,v)=∫Φ−1(u)−∞∫Φ−1(v)−∞12π√1−r2exp{−t2+s2−2rts2(1−r2)}dtds, | (4.3) |
with r=0.1,0.5 and 0.9. The scatter plots of Gaussian copulas are in Figure 6. Table 5 shows the simulation results. As expected, those measures show no significant difference in measuring the dependence of elliptical distributions.
ˆρ1(Y∣X) | ˆρ2(X∣Y) | ˆρ(X,Y) | Spearman's ρ | Kendall's τ | |
r=0.1 | 0.09 | 0.09 | 0.09 | 0.08 | 0.11 |
r=0.5 | 0.33 | 0.33 | 0.33 | 0.29 | 0.42 |
r=0.9 | 0.73 | 0.73 | 0.73 | 0.68 | 0.87 |
The comparison of MFDs with other measures in models 4.1–4.3 shows that MFDs have good adaptability for different types of relationships.
The measurement of functional relationships has many possible applications ([21,22]). The Communities and Crime Data Set [23] contains community crime rate of 1994 communities with 123 possibly related variables. We will use functional dependence measure as a criteria for variable selection to choose the variables which have the most impact on community crime rate. We calculate the measures ρ, ρ1, and ρ2 given in Eqs (2.4)–(2.6), respectively by using beta kernel estimation for community crime rate and each of other variables. Variables with higher values of the measures have a greater impact on community crime rate. The Table 6 shows 9 variables with highest functional dependence measures and Table 7 gives the explanation of the abbreviations. Notice that the measures can detect strong non-linear relationships. As shown in Figure 7, two of the selected variables (PctIlleg and racePctWhite), showed clear nonlinear relations with the crime rate.
Variable | ˆρ1(Y∣X) | ˆρ2(X∣Y) | ˆρ(X,Y) | |
1 | PctKids2Par | 0.56 | 0.57 | 0.57 |
2 | PctIlleg | 0.53 | 0.54 | 0.54 |
3 | PctFam2Par | 0.53 | 0.54 | 0.53 |
4 | NumIlleg | 0.52 | 0.53 | 0.53 |
5 | PctTeen2Par | 0.49 | 0.49 | 0.49 |
6 | racePctWhite | 0.49 | 0.49 | 0.49 |
7 | FemalePctDiv | 0.49 | 0.49 | 0.49 |
8 | NumUnderPov | 0.48 | 0.48 | 0.48 |
9 | TotalPctDiv | 0.48 | 0.48 | 0.48 |
Variable | Attribute |
PctKids2Par | Percentage of kids in family housing with two parents |
PctIlleg | Percentage of kids born to never married |
PctFam2Par | Percentage of families (with kids) that are headed by two parents |
NumIlleg | Number of kids born to never married |
PctTeen2Par | Percent of kids age 12–17 in two parent households |
racePctWhite | Percentage of population that is caucasian |
FemalePctDiv | Percentage of females who are divorced |
NumUnderPov | Number of people under the poverty level |
TotalPctDiv | Percentage of population who are divorced |
This paper showed that, compared with Spearman's ρ or Kendall's τ, the measures of functional relationship could not only measure the strength of a relationship but also indicate the direction of a possible functional relationship. We provide a novel method to estimate the measures of functional relationships. The simulation results showed that they have fairly good accuracy.
Although MFD can quantify the strength of functional dependence, it doesn't suggest any specific form of the function. So one possible application of this measure is in variable selection. We use MFD to filter out the less correlated variables, then use parametric or nonparametric methods to construct a predicting model. In the community crime data example, we showed that MFD could detect nonlinear relationship, but as for how many variables should be retained, in other words, how to set up the threshold of MFD in variable selection is a question that needs to be discussed and may involve some subjective opinions. After the desired number of variables are chosen, people may use either parametric or non-parametric methods to set up the model.
This work was supported in part by the Education Department of Jiangxi Province under Grant GJJ190253 and Grant GJJ190259.
The authors have no conflicts of interest to declare.
[1] | H. O. Lancaster, Dependence, measures and indices of, In: Encyclopedia of statistical sciences, 1982. |
[2] |
K. F. Siburg, P. A. Stoimenov, A measure of mutual complete dependence, Metrika, 71 (2010), 239–251. doi: 10.1007/s00184-008-0229-9
![]() |
[3] |
S. Tasena, S. Dhompongsa, A measure of multivariate mutual complete dependence, Int. J. Approx. Reason., 54 (2013), 748–761. doi: 10.1016/j.ijar.2013.01.001
![]() |
[4] | W. F. Darsow, E. T. Olsen, Norms for copulas, Int. J. Math. Math. Sci., 18 (1995), 576296. |
[5] |
H. Dette, K. F. Siburg, P. A. Stoimenov, A copula-based non-parametric measure of regression dependence, Scand. J. Stat., 40 (2013), 21–41. doi: 10.1111/j.1467-9469.2011.00767.x
![]() |
[6] |
Q. S. Shan, T. Wongyang, T. H. Wang, S. Tasena, A measure of mutual complete dependence in discrete variables through subcopula, Int. J. Approx. Reason., 65 (2015), 11–23. doi: 10.1016/j.ijar.2015.04.005
![]() |
[7] | M. Sklar, Fonctions de répartition à n dimensions et leurs marges, Publ. inst. statist. univ. Paris, 8 (1959), 229–231. |
[8] |
G. Kauermann, C. Schellhase, D. Ruppert, Flexible copula density estimation with penalized hierarchical b-splines, Scand. J. Stat., 40 (2013), 685–705. doi: 10.1111/sjos.12018
![]() |
[9] |
C. Genest, E. Masiello, K. Tribouley, Estimating copula densities through wavelets, Insur. Math. Econ., 44 (2009), 170–181. doi: 10.1016/j.insmatheco.2008.07.006
![]() |
[10] | M. Omelka, I. Gijbels, N. Veraverbeke, Improved kernel estimation of copulas: weak convergence and goodness-of-fit testing, Ann. Statist., 37 (2009), 3023–3058. |
[11] | A. Charpentier, J. D. Fermanian, O. Scaillet, The estimation of copulas: Theory and practice, Copulas: From theory to application in finance, 2007, 35–60. |
[12] |
S. X. Chen, Beta kernel estimators for density functions, Comput. Statist. Data Anal., 31 (1999), 131–145. doi: 10.1016/S0167-9473(99)00010-9
![]() |
[13] | G. Geenens, A. Charpentier, D. Paindaveine, Probit transformation for nonparametric kernel estimation of the copula density, Bernoulli, 23 (2017), 1848–1873. |
[14] | A. Majdara, S. Nooshabadi, Nonparametric density estimation using copula transform, bayesian sequential partitioning, and diffusion-based kernel estimator, IEEE T. Knowl. Data En., 32 (2019), 821–826. |
[15] |
F. E. Harrell, C. E. Davis, A new distribution-free quantile estimator, Biometrika, 69 (1982), 635–640. doi: 10.1093/biomet/69.3.635
![]() |
[16] | S. X. Chen, Beta kernel smoothers for regression curves, Stat. Sinica, 10 (2000), 73–91. |
[17] | T. Nagler, Kernel methods for vine copula estimation, München: Universi at Munchen, 2014. |
[18] | A. W. Van Der Vaart, J. A. Wellner, Weak convergence and empirical processes, Springer, 1996. |
[19] | W. F. Darsow, B. Nguyen, E. T. Olsen, Copulas and markov processes, Illinois J. Math., 36 (1992), 600–642. |
[20] | T. Nagler, kdecopula: An R package for the kernel estimation of copula densities, 2016, arXiv: 1603.04229. |
[21] |
X. Han, Z. L. Wang, M. Xie, Y. H. He, Y. Li, W. Z. Wang, Remaining useful life prediction and predictive maintenance strategies for multi-state manufacturing systems considering functional dependence, Reliab. Eng. Syst. Safe., 210 (2021), 107560. doi: 10.1016/j.ress.2021.107560
![]() |
[22] | Y. H. He, Z. X. Chen, Y. X. Zhao, X. Han, D. Zhou, Mission reliability evaluation for fuzzy multistate manufacturing system based on an extended stochastic flow network, IEEE T. Reliab., 69 (2019), 1239–1253. |
[23] | D. Dua, C. Graff, UCI machine learning repository, Irvine, CA: University of California, school of information and computer acience. Available from: https://archive.ics.uci.edu/ml/index.php. |
n=50 | n=100 | n=200 | ||
θ=0 | ˆρ1(Y∣X) | 2.3×10−3 | 1.5×10−3 | 8.0×10−4 |
ˆρ2(X∣Y) | 2.3×10−3 | 1.5×10−3 | 7.3×10−3 | |
θ=0.3 | ˆρ1(Y∣X) | 6.1×10−3 | 3.8×10−3 | 2.2×10−3 |
ˆρ2(X∣Y) | 6.2×10−3 | 3.8×10−3 | 2.1×10−3 | |
θ=0.6 | ˆρ1(Y∣X) | 8.8×10−3 | 4.2×10−3 | 2.1×10−3 |
ˆρ2(X∣Y) | 8.9×10−3 | 4.1×10−3 | 2.1×10−3 | |
θ=0.9 | ˆρ1(Y∣X) | 2.5×10−3 | 1.1×10−3 | 4.9×10−4 |
ˆρ2(X∣Y) | 2.3×10−3 | 1.1×10−3 | 5.0×10−4 |
n=50 | n=100 | n=200 | ||
θ=0.2 | ˆρ1(Y∣X) | 3.7×10−3 | 2.3×10−3 | 1.8×10−3 |
ˆρ2(X∣Y) | 3.7×10−3 | 2.3×10−3 | 1.8×10−3 | |
θ=0.5 | ˆρ1(Y∣X) | 7.2×10−3 | 4.0×10−3 | 2.3×10−3 |
ˆρ2(X∣Y) | 7.2×10−3 | 4.1×10−3 | 2.3×10−3 | |
θ=1 | ˆρ1(Y∣X) | 9.3×10−3 | 4.9×10−3 | 2.6×10−3 |
ˆρ2(X∣Y) | 9.3×10−3 | 4.9×10−3 | 2.5×10−3 | |
θ=2 | ˆρ1(Y∣X) | 7.5×10−3 | 3.5×10−3 | 1.8×10−3 |
ˆρ2(X∣Y) | 7.4×10−3 | 3.5×10−3 | 1.9×10−3 | |
θ=5 | ˆρ1(Y∣X) | 3.1×10−3 | 1.2×10−3 | 6.1×10−4 |
ˆρ2(X∣Y) | 3.1×10−3 | 1.2×10−3 | 6.0×10−4 |
ˆρ1(Y∣X) | ˆρ2(X∣Y) | ˆρ(X,Y) | Spearman's ρ | Kendall's τ | |
σ=1 | 0.51 | 0.25 | 0.40 | -0.01 | -0.02 |
σ=5 | 0.41 | 0.20 | 0.32 | -0.02 | -0.02 |
σ=10 | 0.27 | 0.13 | 0.21 | -0.01 | -0.01 |
ˆρ1(Y∣X) | ˆρ2(X∣Y) | ˆρ(X,Y) | Spearman's ρ | Kendall's τ | |
σ=1 | 0.89 | 0.89 | 0.89 | 0.82 | 0.95 |
σ=5 | 0.63 | 0.62 | 0.63 | 0.55 | 0.74 |
σ=10 | 0.42 | 0.41 | 0.41 | 0.35 | 0.50 |
ˆρ1(Y∣X) | ˆρ2(X∣Y) | ˆρ(X,Y) | Spearman's ρ | Kendall's τ | |
r=0.1 | 0.09 | 0.09 | 0.09 | 0.08 | 0.11 |
r=0.5 | 0.33 | 0.33 | 0.33 | 0.29 | 0.42 |
r=0.9 | 0.73 | 0.73 | 0.73 | 0.68 | 0.87 |
Variable | ˆρ1(Y∣X) | ˆρ2(X∣Y) | ˆρ(X,Y) | |
1 | PctKids2Par | 0.56 | 0.57 | 0.57 |
2 | PctIlleg | 0.53 | 0.54 | 0.54 |
3 | PctFam2Par | 0.53 | 0.54 | 0.53 |
4 | NumIlleg | 0.52 | 0.53 | 0.53 |
5 | PctTeen2Par | 0.49 | 0.49 | 0.49 |
6 | racePctWhite | 0.49 | 0.49 | 0.49 |
7 | FemalePctDiv | 0.49 | 0.49 | 0.49 |
8 | NumUnderPov | 0.48 | 0.48 | 0.48 |
9 | TotalPctDiv | 0.48 | 0.48 | 0.48 |
Variable | Attribute |
PctKids2Par | Percentage of kids in family housing with two parents |
PctIlleg | Percentage of kids born to never married |
PctFam2Par | Percentage of families (with kids) that are headed by two parents |
NumIlleg | Number of kids born to never married |
PctTeen2Par | Percent of kids age 12–17 in two parent households |
racePctWhite | Percentage of population that is caucasian |
FemalePctDiv | Percentage of females who are divorced |
NumUnderPov | Number of people under the poverty level |
TotalPctDiv | Percentage of population who are divorced |
n=50 | n=100 | n=200 | ||
θ=0 | ˆρ1(Y∣X) | 2.3×10−3 | 1.5×10−3 | 8.0×10−4 |
ˆρ2(X∣Y) | 2.3×10−3 | 1.5×10−3 | 7.3×10−3 | |
θ=0.3 | ˆρ1(Y∣X) | 6.1×10−3 | 3.8×10−3 | 2.2×10−3 |
ˆρ2(X∣Y) | 6.2×10−3 | 3.8×10−3 | 2.1×10−3 | |
θ=0.6 | ˆρ1(Y∣X) | 8.8×10−3 | 4.2×10−3 | 2.1×10−3 |
ˆρ2(X∣Y) | 8.9×10−3 | 4.1×10−3 | 2.1×10−3 | |
θ=0.9 | ˆρ1(Y∣X) | 2.5×10−3 | 1.1×10−3 | 4.9×10−4 |
ˆρ2(X∣Y) | 2.3×10−3 | 1.1×10−3 | 5.0×10−4 |
n=50 | n=100 | n=200 | ||
θ=0.2 | ˆρ1(Y∣X) | 3.7×10−3 | 2.3×10−3 | 1.8×10−3 |
ˆρ2(X∣Y) | 3.7×10−3 | 2.3×10−3 | 1.8×10−3 | |
θ=0.5 | ˆρ1(Y∣X) | 7.2×10−3 | 4.0×10−3 | 2.3×10−3 |
ˆρ2(X∣Y) | 7.2×10−3 | 4.1×10−3 | 2.3×10−3 | |
θ=1 | ˆρ1(Y∣X) | 9.3×10−3 | 4.9×10−3 | 2.6×10−3 |
ˆρ2(X∣Y) | 9.3×10−3 | 4.9×10−3 | 2.5×10−3 | |
θ=2 | ˆρ1(Y∣X) | 7.5×10−3 | 3.5×10−3 | 1.8×10−3 |
ˆρ2(X∣Y) | 7.4×10−3 | 3.5×10−3 | 1.9×10−3 | |
θ=5 | ˆρ1(Y∣X) | 3.1×10−3 | 1.2×10−3 | 6.1×10−4 |
ˆρ2(X∣Y) | 3.1×10−3 | 1.2×10−3 | 6.0×10−4 |
ˆρ1(Y∣X) | ˆρ2(X∣Y) | ˆρ(X,Y) | Spearman's ρ | Kendall's τ | |
σ=1 | 0.51 | 0.25 | 0.40 | -0.01 | -0.02 |
σ=5 | 0.41 | 0.20 | 0.32 | -0.02 | -0.02 |
σ=10 | 0.27 | 0.13 | 0.21 | -0.01 | -0.01 |
ˆρ1(Y∣X) | ˆρ2(X∣Y) | ˆρ(X,Y) | Spearman's ρ | Kendall's τ | |
σ=1 | 0.89 | 0.89 | 0.89 | 0.82 | 0.95 |
σ=5 | 0.63 | 0.62 | 0.63 | 0.55 | 0.74 |
σ=10 | 0.42 | 0.41 | 0.41 | 0.35 | 0.50 |
ˆρ1(Y∣X) | ˆρ2(X∣Y) | ˆρ(X,Y) | Spearman's ρ | Kendall's τ | |
r=0.1 | 0.09 | 0.09 | 0.09 | 0.08 | 0.11 |
r=0.5 | 0.33 | 0.33 | 0.33 | 0.29 | 0.42 |
r=0.9 | 0.73 | 0.73 | 0.73 | 0.68 | 0.87 |
Variable | ˆρ1(Y∣X) | ˆρ2(X∣Y) | ˆρ(X,Y) | |
1 | PctKids2Par | 0.56 | 0.57 | 0.57 |
2 | PctIlleg | 0.53 | 0.54 | 0.54 |
3 | PctFam2Par | 0.53 | 0.54 | 0.53 |
4 | NumIlleg | 0.52 | 0.53 | 0.53 |
5 | PctTeen2Par | 0.49 | 0.49 | 0.49 |
6 | racePctWhite | 0.49 | 0.49 | 0.49 |
7 | FemalePctDiv | 0.49 | 0.49 | 0.49 |
8 | NumUnderPov | 0.48 | 0.48 | 0.48 |
9 | TotalPctDiv | 0.48 | 0.48 | 0.48 |
Variable | Attribute |
PctKids2Par | Percentage of kids in family housing with two parents |
PctIlleg | Percentage of kids born to never married |
PctFam2Par | Percentage of families (with kids) that are headed by two parents |
NumIlleg | Number of kids born to never married |
PctTeen2Par | Percent of kids age 12–17 in two parent households |
racePctWhite | Percentage of population that is caucasian |
FemalePctDiv | Percentage of females who are divorced |
NumUnderPov | Number of people under the poverty level |
TotalPctDiv | Percentage of population who are divorced |