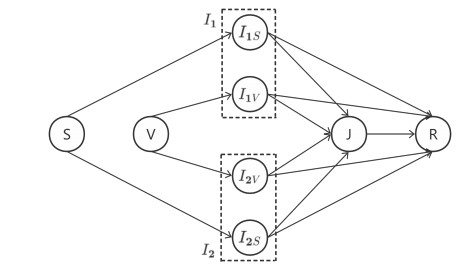
Toll-like receptors (TLRs) are essential defensive mediators implicated in immune diseases. Tight regulation of TLR function is indispensable to avoid the damaging effects of chronic signaling. Several endogenous molecules have emerged as negative regulators of TLR signaling. In this review, we highlighted the structure, regulation, and function of RP105 and A20 in negatively modulating TLR-dependent inflammatory diseases, and in fibrosis and potential therapeutic approaches.
Citation: Swarna Bale, John Varga, Swati Bhattacharyya. Role of RP105 and A20 in negative regulation of toll-like receptor activity in fibrosis: potential targets for therapeutic intervention[J]. AIMS Allergy and Immunology, 2021, 5(2): 102-126. doi: 10.3934/Allergy.2021009
[1] | Samitha Samaranayake, Axel Parmentier, Ethan Xuan, Alexandre Bayen . A mathematical framework for delay analysis in single source networks. Networks and Heterogeneous Media, 2017, 12(1): 113-145. doi: 10.3934/nhm.2017005 |
[2] | Mary Luz Mouronte, Rosa María Benito . Structural analysis and traffic flow in the transport networks of Madrid. Networks and Heterogeneous Media, 2015, 10(1): 127-148. doi: 10.3934/nhm.2015.10.127 |
[3] | Dirk Helbing, Jan Siegmeier, Stefan Lämmer . Self-organized network flows. Networks and Heterogeneous Media, 2007, 2(2): 193-210. doi: 10.3934/nhm.2007.2.193 |
[4] | Simone Göttlich, Elisa Iacomini, Thomas Jung . Properties of the LWR model with time delay. Networks and Heterogeneous Media, 2021, 16(1): 31-47. doi: 10.3934/nhm.2020032 |
[5] | Fabio Della Rossa, Carlo D’Angelo, Alfio Quarteroni . A distributed model of traffic flows on extended regions. Networks and Heterogeneous Media, 2010, 5(3): 525-544. doi: 10.3934/nhm.2010.5.525 |
[6] | Maya Briani, Emiliano Cristiani . An easy-to-use algorithm for simulating traffic flow on networks: Theoretical study. Networks and Heterogeneous Media, 2014, 9(3): 519-552. doi: 10.3934/nhm.2014.9.519 |
[7] | Tibye Saumtally, Jean-Patrick Lebacque, Habib Haj-Salem . A dynamical two-dimensional traffic model in an anisotropic network. Networks and Heterogeneous Media, 2013, 8(3): 663-684. doi: 10.3934/nhm.2013.8.663 |
[8] | Gabriella Bretti, Roberto Natalini, Benedetto Piccoli . Numerical approximations of a traffic flow model on networks. Networks and Heterogeneous Media, 2006, 1(1): 57-84. doi: 10.3934/nhm.2006.1.57 |
[9] | A. Marigo . Robustness of square networks. Networks and Heterogeneous Media, 2009, 4(3): 537-575. doi: 10.3934/nhm.2009.4.537 |
[10] | Ye Sun, Daniel B. Work . Error bounds for Kalman filters on traffic networks. Networks and Heterogeneous Media, 2018, 13(2): 261-295. doi: 10.3934/nhm.2018012 |
Toll-like receptors (TLRs) are essential defensive mediators implicated in immune diseases. Tight regulation of TLR function is indispensable to avoid the damaging effects of chronic signaling. Several endogenous molecules have emerged as negative regulators of TLR signaling. In this review, we highlighted the structure, regulation, and function of RP105 and A20 in negatively modulating TLR-dependent inflammatory diseases, and in fibrosis and potential therapeutic approaches.
Many infectious diseases, such as novel coronavirus pneumonia (COVID-19), Ebola and monkeypox, are caused by pathogen variants that can be spread among people and which endanger human life and threaten national security. With the increase of the convenience of modern logistics, some extinct diseases recur, and some re-surging epidemics emerge. Therefore, how to effectively curtail the disease spread has been a major concern of the whole modern society.
Vaccines can boost the production of competent antibodies by stimulating the immune system and lower the morbidity and mortality rates. Hence, vaccination plays a crucial role in preventing and controlling outbreaks of the diseases. Specific immunity induced by vaccination effectively fights against the invasion of viruses, bacteria and other pathogens. With the advancement of science and technology, more and more vaccines have been developed to effectively resist infectious diseases and radically eradicate the diseases, such as smallpox, diphtheria, varicella, etc.
Facing an emerging disease, it is sometimes possible to take a voluntary vaccination strategy to curb its prevalence. In this case, everyone is inclined to either get vaccinated or not. Therefore, the onset of an epidemic depends not only on the transmission mechanisms of an infectious disease, but also on the choices of potential vaccine recipients. For a bounded rationality, they will evaluate the payoff from vaccination, including the side effects of vaccines and the potential risk if they do not take the vaccine, to decide to get vaccinated or not [1]. A myriad of studies has shown that voluntary vaccination policies cannot radically eliminate the outbreak of an epidemic because of the behavior-disease-vaccination interactions, which may form a feedback loop [2,3,4,5]. When a disease breaks out and causes lots of deaths, most people would like to receive a vaccination for preventing the disease infection. Once the vaccination coverage reaches a certain level and produces herd immunity, the disease will be effectively controlled. As a consequence, the non-vaccinated have no motivation to get vaccinated and lead to the resurgence of the epidemic.
To further study how individuals' behaviors influence infectious diseases, researchers have employed the evolutionary game theory to set up co-evolutionary models of infectious diseases and human behaviors [6,7,8,9,10,11,12,13,14]. Indeed, such models provide useful tools to understand the effects of human behaviors on the development of an epidemic. For instance, Kuge and Tanimoto simultaneously proposed a susceptible-infected-recovered (SIR) epidemic model to consider the effects of innate immunity from the treatment and specific immunity from vaccination [15]. Alam et al. adopted an SIR epidemic model to find that imperfect immunization attenuates the rate of virus transmission and gives a second protection to the vaccinated even though they do not receive perfect vaccine protection [16]. Later, Alam coupled the vaccination evolutionary game and an SEIR/V model to study the effects of two control strategies: isolation and quarantine. It was found that the effects of isolation are better than those of quarantine [17]. Arefin et al, based on evolutionary game theory, proposed a two-strain model to evaluate the trade-off of the evolutionary mutation [18]. Kabir et al. combined the SIR model with unawareness and awareness to study the effect of information dissemination on preventing the transmission of infectious diseases [19]. Huang et al. proposed a mathematical model based on complex networks to investigate the co-evolution of epidemic transmission and individuals' behaviors [20].
Most infectious diseases are caused by a single pathogenic strain, but in reality, the disease may be caused by different variants of the pathogen, such as the variants of COVID-19 represented by more than 5 serotypes: alpha, beta, gamma, delta and omicron [21]. Influenza can be categorized by three serotypes: A, B and C. Such multi-strains interact with each other, and some trade-off mechanisms have been identified that result in the coexistence of pathogens in epidemic models. Coinfection is one such trade-off mechanism, and it is defined as a process of the single host infected by two or more pathogenic strains. Evidences has shown that coinfection is common in HIV (human immunodeficiency virus) infection, with combined infections with TB, hepatitis and malaria. Most importantly, co-infection by variants of a pathogen often leads to more mortality and brings a huge challenge to public health. Therefore, it is necessary to create appropriate approaches to evaluate the effects of coinfection. Many researchers have used infectious disease models to analyze the impacts of coinfection on epidemic spread. Gao et al. showed that coinfection leads to the coexistence of muti-strain mechanisms [22]. In [23], Elaiw et al. proposed a single-host SARS-CoV-2/HIV co-infection model, and they showed that weak CD4+ T cell immunity in co-infected patients can cause severe SARS-CoV-2 infections from a cellular perspective. Hezam et al. proposed the co-infection phenomenon between COVID-19 and cholera to evaluate the combinations of the best policies for curbing such two diseases [24]. Newman and Ferrario studied a co-infection model on a contact network and showed that the results on scale-free networks are in agreement with those results on large wired networks [25]. Osman and Makinde built a listeriosis and anthrax coinfection model, and they studied the effects on each strain and coinfected strain by varying exposure rates [26]. Martcheva and Pilyugin used an age-structured epidemic model to characterize the coinfection phenomena [27]. Through detailed analysis, they showed that coinfection produces rich dynamics, including backward bifurcation and Hopf bifurcation. Li et al. proposed a multiscale system taking into account for interplays of within and between systems [28]. On an intra-host scale, the two strains compete with each other, and the one with a larger basic reproduction number dominates. On a population scale, co-infection results in the coexistence of the two strains. Sanz et al. proposed a two-strain epidemic model with coinfection on complex networks [29].
In most of the reviewed existing epidemic models with coinfection, they have neglected the effects of individual vaccination behaviors on the evolution of the epidemic infection. In this paper, we couple the evolutionary vaccination behaviors and epidemic models to examine the effect of the co-infection mechanism on the development of an epidemic. The aim of this paper is to explore the co-evolution of flu infection and uptaking vaccination strategies of individuals.
This paper is organized as follows: In Section 2, we design a game theoretical-compartmental epidemic model with coinfection. In Section 3, we study the dynamics of the model through a detailed analysis. Finally, in Section 4, we end with a brief discussion.
We assume that an outbreak of an epidemic happens at two distinct time scales. One is the local time scale (tlocal), and the other is the global time scale (tglobal). On the first time scale, the period goes through the onset to the end of the epidemic. On the last time scale, individuals taking vaccines dynamically evolves along with periodical outbreaks of the epidemic.
Similar to [18], we classify the total population into eight categories: S(tlocal) is the proportion of susceptible individuals at time t, V(tlocal) is the proportion of vaccinated individuals at time tlocal, I1S(tlocal) is the proportion of susceptible individuals infected by strain one at time tlocal, I1V(tlocal) is the proportion of vaccinated individuals infected by strain one at time tlocal, I2S(tlocal) is the proportion of susceptible individuals infected by strain two at time tlocal, I2V(tlocal) is the proportion of vaccinated individuals infected by strain two at time tlocal, J(tlocal) is the proportion of individuals infected by strain one and strain two at time tlocal, and R(tlocal) is the proportion of recovered individuals at time tlocal.
We assume that individuals initially infected by strain 1 can be re-infected by strain 2, and they move to the co-infection class (J(tlocal)). Similarly, individuals initially infected by strain 2 can be re-infected by strain 1, and they move to the co-infection class (J(tlocal)). Since the vaccine is not fully effective, we assume that its efficacy values for strain 1 and strain 2 are the coefficients e1 and e2. Susceptibles (S(tlocal)), vaccinated individuals (V(tlocal)), susceptibles infected by strain 2 (I2S(tlocal)) and vaccinated individuals infected by strain 2 (I2V(tlocal)) can become individuals infected by strain 1 at transmission rate β1. Susceptibles (S(tlocal)), vaccinated individuals (V(tlocal)), susceptibles infected by strain 1 (I1S(tlocal)) and vaccinated individuals infected by strain 1 (I1V(tlocal)) can become ones infected by strain 2 at a transmission rate β2. Individuals infected by strain 1 (I1S(tlocal), I1V(tlocal)) recover at rate γ1, and then they move to the recovery class (R(tlocal)). Individuals infected by strain 2 (I2S(tlocal), I2V(tlocal)) recover at a rate of γ2, and then they move to the recovery class (R(tlocal)). It is assumed that co-infected individuals (J(tlocal)) take a longer time to recover than individuals infected by one of the strains alone (γ3<min{γ1,γ2}). A schematic diagram of our model is presented in Figure 1. The framework of a two-strain epidemic model with coinfection takes the following form.
dS(tlocal)dt=−β1S(tlocal)(I1(tlocal)+J(tlocal))−β2S(tlocal)(I2(tlocal)+J(tlocal)),dV(tlocal)dt=−β1(V(tlocal)−e1V(x,0))(I1(tlocal)+J(tlocal))−β2(V(tlocal)−e2V(0))(I2(tlocal)+J(tlocal)),dI1S(tlocal)dt=β1S(tlocal)(I1(tlocal)+J(tlocal))−β2I1S(tlocal)(I2(tlocal)+J(tlocal))−γ1I1S(tlocal),dI1V(tlocal)dt=β1(V(tlocal)−e1V(0))(I1(tlocal)+J(tlocal))−β2I1V(tlocal)(I2(tlocal)+J(tlocal))−γ1I1V(tlocal),dI2S(tlocal)dt=β2S(tlocal)(I2(tlocal)+J(tlocal))−β1I2S(tlocal)(I1(tlocal)+J(tlocal))−γ2I2S(tlocal),dI2V(tlocal)dt=β2(V(tlocal)−e2V(0))(I2(tlocal)+J(tlocal))−β1I2V(tlocal)(I1(tlocal)+J(tlocal))−γ2I2V(tlocal),dJ(tlocal)dt=β2I1S(tlocal)(I2(tlocal)+J(tlocal))+β2I1V(tlocal)(I2(tlocal)+J(tlocal))+β1I2S(tlocal)(I1(tlocal)+J(tlocal))+β1I2V(tlocal)(I1(tlocal)+J(tlocal))−γ3J(tlocal),dR(tlocal)dt=γ1I1S(tlocal)+γ1I1V(tlocal)+γ2I2S(tlocal)+γ2I2V(tlocal)+γ3J(tlocal). | (2.1) |
Define
I1(tlocal)=I1S(tlocal)+I1V(tlocal),I2(tlocal)=I2S(tlocal)+I2V(tlocal). |
To satisfy the biological significance, all parameters are positive, and they are chosen from Table 1. The total population is given by
S(tlocal)+V(tlocal)+I1(tlocal)+I2(tlocal)+J(tlocal)+R(tlocal)=1. |
Parameters | Description | Value | Source |
β1 | The transmission rate for strain 1 | 0.5 day−1 | Assumed |
β2 | The transmission rate for strain 2 | 0.7 day−1 | Assumed |
γ1 | Recovery rate after infection by strain 1 | 1/11 day−1 | [30] |
γ2 | Recovery rate after infection by strain 2 | 1/9 day−1 | [30] |
γ3 | Recovery rate after infection by strain 1 and strain 2 | 1/14 day−1 | Assumed |
e1 | Effectiveness of vaccination at protecting against strain 1 | 0.61 | [31] |
e2 | Effectiveness of vaccination at protecting against strain 2 | 0.33 | [31] |
The initial values satisfy
S(0)=1−x>0,V(0)=x≥0,I1(0)=0,I2(0)=0,J(0)=0,R(0)=0. |
In fact, in model 2.1, strain 1 can be considered as influenza A (H1N1) virus, and strain 2 is associated with influenza A (H3N2) virus. As we know, an influenza quadrivalent vaccine can protect against four different influenza viruses. In addition, all people over 6 months of age need to get an annual flu shot for slowing down influenza transmission.
The final epidemic size is the proportion of the population that is infected at the end of the epidemic. Since all the infected individuals finally become the recovered ones at the end of the disease, the proportion of recovered individuals at the final stage of the epidemic ((tlocal=∞)) is the final size of the epidemic, denoted by R(∞). Similarly, we have the following.
● HV is a collective term referring to individuals who choose to get vaccinated before the outbreak, but they are not infected by any strain during the epidemic, represented by HV(∞).
● I1V is a collective term referring to individuals who choose to get vaccinated before the outbreak, and they are infected by strain 1 during the epidemic, represented by I1V(∞).
● I2V is a collective term referring to individuals who choose to get vaccinated before the outbreak, and they are infected by strain 2 during the epidemic, represented by I2V(∞).
● IV is another collective term referring to individuals who choose to get vaccinated before the outbreak, and they are co-infected by strains 1 and 2 during the epidemic, represented by IV(∞).
● SFR is a collective term referring to individuals who are not vaccinated before the outbreak, and they are not infected by any strain during the epidemic, represented by SFR(∞).
● FFR1 is a collective term referring to individuals who are not vaccinated before the outbreak, but they are infected by strain 1 during the epidemic, represented by FFR1(∞).
● FFR2 is a collective term referring to individuals who are not vaccinated before the outbreak, but they are infected by strain 2 during the epidemic, represented by FFR2(∞).
● FFR is a collective term referring to individuals who are not vaccinated before the outbreak, but they are infected by both strain 1 and strain 2 during the epidemic, represented by FFR(∞).
Based on the next generation matrix [32], the reproduction number associated with each strain is defined by
Ri=βi(1−eix)γi,i=1,2. |
Hence, the basic reproduction number is
R0=max{R1,R2}. |
When R0<1, system (2.1) has only a disease-free equilibrium (DFE), which means that neither strain colonizes the host, and the disease becomes extinct. When R1>1,R2<1, it follows from the competitive exclusion principle that strain 1 is dominant, and strain 2 is eliminated. Similarly, when R2>1,R1<1, strain 2 is dominant, and strain 1 is eradicated. When R1>1,R2>1, the two strains coexist, and the two strains both invade the host.
Although the extermination of smallpox served as a perfect example of completely eliminating a disease by vaccines, there are still many diseases that cannot be radically eradicated in a region. There is a paradox in immuno-epidemiology: While vaccination leads to herd immunity, individuals who have not been vaccinated are indirectly protected against the disease with no incentive to take vaccines, and they don't pay any costs. These are called free riders. From a personal payment perspective, free riders are not required to pay any costs associated with the epidemic.
Next, we analyze the payoffs of different types of people. The cost of the vaccinated from direct costs and side-effects is denoted by CV. The payoff of potential infection from the risk of death and suffering is denoted by CI. Thus, the relative payoff of vaccination is Cr=CV/CI. We assume that the payoff of the vaccinated is less than the that of the non-vaccinated, and 0<Cr≤1. Here, we divide the relative payoffs of the population into eight categories (see Table 2). We can obtain the average social payoff π, the average vaccinated payoff ⟨πV⟩, and the average unvaccinated payoff ⟨πNV⟩.
π=−CrHV(∞)−(Cr+1)(I1V(∞)+I2V(∞))−(Cr+2)IV(∞)−(FFR1(∞)+FFR2(∞)+2FFR(∞)), | (2.2) |
⟨πV⟩=−(CrHV(∞)+(Cr+1)(I1V(∞)+I2V(∞))+(Cr+2)IV(∞))/x, | (2.3) |
⟨πNV⟩=−(FFR1(∞)+FFR2(∞)+2FFR(∞))/(1−x). | (2.4) |
State/Strategy | Healthy | Infected with strain 1 | Infected with strain 2 | Coinfected with strain 1 and strain 2 |
Vaccination (V) | πHV=−Cr | πI1V=−Cr−1 | πI2V=−Cr−1 | πIV=−Cr−2 |
Non-vaccination (NV) | πSFR=0 | πFFR1=−1 | πFFR2=−1 | πFFR=−2 |
Unlike adopting static game-theoretic strategies, we assume that the information about the epidemic is limited, which implies that at the end of each cycle of the epidemic, individuals update their strategies for the next period depending on their neighbors' payoffs. For the sake of convenience, we employ the individual-based risk assessment (IB-RA) strategy proposed by Fu et al. [33] and the strategy-based risk assessment (SB-RA) strategy proposed by Fukuda et al. [34] to derive the evolutionary game-theoretical model.
1) Individual-based risk assessment (IB-RA) model
To explore the role played by individual imitative behavior and group structure in vaccination, Fu et al. [33] proposed an evolutionary game approach. Individual i randomly selects one of his or her neighbours j to imitate, and he or her would like to imitate one who acquired higher benefits in the previous epidemic course. The probability of individual i adopting the strategy of individual j is assumed to be given in the Fermi type [26,27,28,29], which takes the form of
P(Si←Sj)=11+exp[−(πj−πi)/κ], | (2.5) |
where Si denotes the strategy adopted by individual i. πi denotes the payoff by individual i during the previous epidemic. κ denotes selection intensity. The larger the value of κ, the more likely the individual is to switch his behaviors. For example, the probability that a healthy vaccinator (HV) will imitate a successful free-rider (SFR) is formulated by
P(HV←SFR)=11+exp[−(0−(−Cr))/κ]. |
Other cases are shown in the appendix.
2) Strategy-based risk assessment (SB-RA) model
Fukuda et al. modified the uptaken IB-RA rule from the payoff of an individual to an average one. The transfer probability for individual i is taken in the form of
P(Si←Sj)=11+exp[−(⟨πj⟩−πi)/κ], | (2.6) |
where ⟨πj⟩ denotes the average payoff for individuals who adopt strategy Sj. For example, the probability that a healthy vaccinator (HV) imitates non-vaccinators is
P(HV←NV)=11+exp[−(⟨πNV⟩−(−Cr))/κ]. |
Similarly, other cases are shown in the appendix.
The vaccination dynamics corresponding to different imitative behaviors can be derived from the imitation probabilities mentioned above. The global dynamics of an individual behavior in the IB-RA framework at the global time scale (tglobal) is given by
dxdtglobal=HV(∞)⋅SFR(∞)⋅(P(SFR←HV)−P(HV←SFR))+HV(∞)⋅FFR1(∞)⋅(P(FFR1←HV)−P(HV←FFR1))+HV(∞)⋅FFR2(∞)⋅(P(FFR2←HV)−P(HV←FFR2))+HV(∞)⋅FFR(∞)⋅(P(FFR←HV)−P(HV←FFR))+I1V(∞)⋅SFR(∞)⋅(P(SFR←I1V)−P(I1V←SFR))+I1V(∞)⋅FFR1(∞)⋅(P(FFR1←I1V)−P(I1V←FFR1))+I1V(∞)⋅FFR2(∞)⋅(P(FFR2←I1V)−P(I1V←FFR2))+I1V(∞)⋅FFR(∞)⋅(P(FFR←I1V)−P(I1V←FFR))+I2V(∞)⋅SFR(∞)⋅(P(SFR←I2V)−P(I2V←SFR))+I2V(∞)⋅FFR1(∞)⋅(P(FFR1←I2V)−P(I2V←FFR1))+I2V(∞)⋅FFR2(∞)⋅(P(FFR2←I2V)−P(I2V←FFR2))+I2V(∞)⋅FFR(∞)⋅(P(FFR←I2V)−P(I2V←FFR))+IV(∞)⋅SFR(∞)⋅(P(SFR←IV)−P(IV←SFR))+IV(∞)⋅FFR1(∞)⋅(P(FFR1←IV)−P(IV←FFR1))+IV(∞)⋅FFR2(∞)⋅(P(FFR2←IV)−P(IV←FFR2))+IV(∞)⋅FFR(∞)⋅(P(FFR←IV)−P(IV←FFR)). | (2.7) |
The global dynamics of individual behavior in the SB-RA framework at the global time scale (tglobal) is taken in the form of
dxdtglobal=SFR(∞)⋅V(∞)⋅P(SFR←V)+FFR1(∞)⋅V(∞)⋅P(FFR1←V)+FFR2(∞)⋅V(∞)⋅P(FFR2←V)+FFR(∞)⋅V(∞)⋅P(FFR←V)−HV(∞)⋅NV(∞)⋅P(HV←NV)−I1V(∞)⋅NV(∞)⋅P(I1V←NV)−I2V(∞)⋅NV(∞)⋅P(I2V←NV)−IV(∞)⋅NV(∞)⋅P(IV←NV). | (2.8) |
Due to the complexity of the system, we have conducted numerical simulations to show the dynamics of the model. We focus on how the parameters affect the dynamics. Similar to [18], we first investigated the influence of vaccine effectiveness on the game-theoretic epidemic system in the frameworks of the IB-RA and SB-RA rules. Meanwhile, we investigated the effects of the transmission rate of each strain on the evolutionary game system by adopting such two rules.
The top and bottom panels of Figure 2 respectively show the influence of e1 and e2 on the reproduction number of Strain 1 (R1), the reproduction number of Strain 2 (R2), final epidemic size (FES), vaccination coverage (VC) and social average payment (ASP) in the frameworks of the IB-RA and SB-RA rules. In Figure 2, the horizontal coordinate represents the effectiveness of the vaccine against Strain 1 and the vertical coordinate indicates the effectiveness of the vaccine against Strain 2. First, we have noted that there exists a triangular region in the lower left corners of subplots of Figure 2. In such regions, the efficacy of vaccines is not high enough to curtail the two strains, a large number of people will be infected, and fewer people will take vaccines. As a consequence, it will cause a huge socioeconomic burden. With the increase of the vaccine effectiveness against Strain 1, the reproduction number associated with strain 1 is monotonically decreasing. Similarly, the reproduction number with respect to strain 2 monotonically decreases as e2 increases (see Figure 2b-*). This phenomenon suggests that improving the efficacy of immunity from vaccines is in favor of controlling the disease spread. From Figure 2c-*, 2d-* and 2e-*, we have concluded that the efficacies of vaccines associated with the two strains are equal and high, and this leads to the best response for curbing the disease transmission. Comparing the results by adopting the IB-RA and SB-RA strategies, we found that the SB-RA strategy is more effective in preventing the disease.
The top and bottom panels of Figure 3 show the effects of β1 and β2 on the reproduction number of strain 1 (R1), the reproduction number of strain 2 (R2), final size (FES), vaccine coverage (VC), and social average payment (ASP) corresponding to the IB-RA and SB-RA rules, respectively. In Figure 3, the horizontal coordinate represents the transmission rate of strain 1, and the vertical coordinate represents the transmission rate of strain 2.
In Figure 3a-*, the reproduction number associated with strain 1 monotonically increases as the rate of transmission of strain 1 increases. A familiar trend was observed for the reproduction number with respect to strain 2 in Figure 3b-*. Furthermore, the reproduction number associated with strain 2 is not only dependent on β2, but it is influenced by β2. Figure 3c-* displays the regions of disease-free equilibrium (DFE), the dominance of strain 1, the dominance of strain 2 and the coexistence area (see Figure 3c-*), which is substantially different when compared with the results of [18]. Comparing the results of the IB-RA and SB-RA updated rules, we have seen that that the epidemic, by adopting the SB-RA strategy, has a larger final size, a less vaccine coverage, and a greater economic burden on society.
This work aims to study the co-evolution of a two-strain epidemic with coinfection and the evolution of game-theoretical vaccination behaviors. We adopted the IB-RA and the SB-RA updated rules to uptake the individuals' vaccination strategies. Through numerical analysis, we have found that co-infection deeply aggravates the spread of disease, and the basic reproduction number is closely related with both β1 and β2. Similarly, those biomass, such as the epidemic final size, the average social payoff, etc. strongly depend on the efficacy of each strain ϵj(j=1,2). This is substantially different from those outcomes of traditional epidemic models with only a single strain. We have shown that higher vaccine effectiveness and lower transmission rate are encouraged in curbing infectious diseases under the IB-RA and SB-RA updated rules, which are consistent with those results in [18]. Comparing with those results, adopting SB-RA updated rules has a better effect on reducing the epidemic final size and lowering the payoff of society.
Due to the introduction of the coinfection mechanism, there exists four scenarios: the region of the eradication of the disease, the region of strain 1 dominating, the area of strain 2 dominating and the area of the coexistence. The closer the transmission rates of the two strains are, the heavier the social burden will be, even if a huge proportion of people would like to get vaccinated. All these findings are significant guidance for the control of epidemics with coinfection by two strains.
We thank the editor and the referees for their exceptionally helpful comments during the review process. This work is partially supported by the National Natural Science Foundation of China (No.11771017, No.61573016), the Shanxi Province Science foundation (20210302123454), and the Shanxi Province Science Foundation for Youths (No. 201901D211413).
All authors declare no conflicts of interest in this paper.
P(HV←SFR)=11+exp[−(0−(−Cr))/κ],P(HV←FFR1)=11+exp[−(−1−(−Cr))/κ],P(HV←FFR2)=11+exp[−(−1−(−Cr))/κ],P(HV←FFR)=11+exp[−(−2−(−Cr))/κ],P(I1V←SFR)=11+exp[−(0−(−Cr−1))/κ],P(I1V←FFR1)=11+exp[−(−1−(−Cr−1))/κ],P(I1V←FFR2)=11+exp[−(−1−(−Cr−1))/κ],P(I1V←FFR)=11+exp[−(−2−(−Cr−1))/κ],P(I2V←SFR)=11+exp[−(0−(−Cr−1))/κ],P(I2V←FFR1)=11+exp[−(−1−(−Cr−1))/κ],P(I2V←FFR2)=11+exp[−(−1−(−Cr−1))/κ],P(I2V←FFR)=11+exp[−(−2−(−Cr−1))/κ],P(IV←SFR)=11+exp[−(0−(−Cr−2))/κ],P(IV←FFR1)=11+exp[−(−1−(−Cr−2))/κ],P(IV←FFR2)=11+exp[−(−1−(−Cr−2))/κ],P(IV←FFR)=11+exp[−(−2−(−Cr−2))/κ],P(SFR←HV)=11+exp[−(−Cr−0)/κ],P(SFR←I1V)=11+exp[−(−Cr−1−0)/κ],P(SFR←I2V)=11+exp[−(−Cr−1−0)/κ],P(SFR←IV)=11+exp[−(−Cr−2−0)/κ],P(FFR1←HV)=11+exp[−(−Cr−(−1))/κ],P(FFR1←I1V)=11+exp[−(−Cr−1−(−1))/κ],P(FFR1←I2V)=11+exp[−(−Cr−1−(−1))/κ],P(FFR1←IV)=11+exp[−(−Cr−2−(−1))/κ],P(FFR2←HV)=11+exp[−(−Cr−(−1))/κ],P(FFR2←I1V)=11+exp[−(−Cr−1−(−1))/κ],P(FFR2←I2V)=11+exp[−(−Cr−1−(−1))/κ],P(FFR2←IV)=11+exp[−(−Cr−2−(−1))/κ],P(FFR←HV)=11+exp[−(−Cr−(−2))/κ],P(FFR←I1V)=11+exp[−(−Cr−1−(−2))/κ],P(FFR←I2V)=11+exp[−(−Cr−1−(−2))/κ],P(FFR←IV)=11+exp[−(−Cr−2−(−2))/κ]. |
P(HV←NV)=11+exp[−(⟨πNV⟩−(−Cr))/κ],P(I1V←NV)=11+exp[−(⟨πNV⟩−(−Cr−1))/κ],P(I2V←NV)=11+exp[−(⟨πNV⟩−(−Cr−1))/κ],P(IV←NV)=11+exp[−(⟨πNV⟩−(−Cr−2))/κ],P(SFR←V)=11+exp[−(⟨πV⟩−(0))/κ],P(FFR1←V)=11+exp[−(⟨πV⟩−(−1))/κ],P(FFR2←V)=11+exp[−(⟨πV⟩−(−1))/κ],P(FFR←V)=11+exp[−(⟨πV⟩−(−2))/κ]. |
[1] |
Medzhitov R, Preston-Hurlburt P, Janeway CA (1997) A human homologue of the Drosophila Toll protein signals activation of adaptive immunity. Nature 388: 394-397. doi: 10.1038/41131
![]() |
[2] |
Stoffels JMJ, Zhao C, Baron W (2013) Fibronectin in tissue regeneration: timely disassembly of the scaffold is necessary to complete the build. Cell Mol Life Sci 70: 4243-4253. doi: 10.1007/s00018-013-1350-0
![]() |
[3] | Bhattacharyya S, Wang W, Morales-Nebreda L, et al. (2016) Tenascin-C drives persistence of organ fibrosis. Nat Commun 7: 1-14. |
[4] |
Huang QQ, Sobkoviak R, Jockheck-Clark AR, et al. (2009) Heat shock protein 96 is elevated in rheumatoid arthritis and activates macrophages primarily via TLR2 signaling. J Immunol 182: 4965-4973. doi: 10.4049/jimmunol.0801563
![]() |
[5] |
Huang QQ, Pope RM (2010) Toll-like receptor signaling: a potential link among rheumatoid arthritis, systemic lupus, and atherosclerosis. J Leukocyte Biol 88: 253-262. doi: 10.1189/jlb.0310126
![]() |
[6] |
Vencken SF, Greene CM (2016) Toll-like receptors in cystic fibrosis: impact of dysfunctional microRNA on innate immune responses in the cystic fibrosis lung. J Innate Immun 8: 541-549. doi: 10.1159/000444687
![]() |
[7] |
Bhattacharyya S, Wang W, Qin W, et al. (2018) TLR4-dependent fibroblast activation drives persistent organ fibrosis in skin and lung. JCI Insight 3: e98850. doi: 10.1172/jci.insight.98850
![]() |
[8] |
Divanovic S, Trompette A, Atabani SF, et al. (2005) Inhibition of TLR-4/MD-2 signaling by RP105/MD-1. J Endotoxin Res 11: 363-368. doi: 10.1177/09680519050110061201
![]() |
[9] |
Gon Y, Asai Y, Hashimoto S, et al. (2004) A20 inhibits toll-like receptor 2- and 4-mediated interleukin-8 synthesis in airway epithelial cells. Am J Resp Cell Mol 31: 330-336. doi: 10.1165/rcmb.2003-0438OC
![]() |
[10] |
Peng J, Tao X, Li R, et al. (2015) Novel toll/IL-1 receptor homologous region adaptors act as negative regulators in amphioxus TLR signaling. J Immunol 195: 3110-3118. doi: 10.4049/jimmunol.1403003
![]() |
[11] |
Divanovic S, Trompette A, Petiniot LK, et al. (2007) Regulation of TLR4 signaling and the host interface with pathogens and danger: the role of RP105. J Leukocyte Biol 82: 265-271. doi: 10.1189/jlb.0107021
![]() |
[12] |
Zhao X, Chu Q, Cui J, et al. (2018) MicroRNA-19a as a negative regulator in TLR signaling pathway by direct targeting myeloid differentiation factor 88 in miiuy croaker. Dev Comp Immunol 87: 171-175. doi: 10.1016/j.dci.2018.06.009
![]() |
[13] |
Xu M, Li D, Yang C, et al. (2018) MicroRNA-34a inhibition of the TLR signaling pathway via CXCL10 suppresses breast cancer cell invasion and migration. Cell Physiol Biochem 46: 1286-1304. doi: 10.1159/000489111
![]() |
[14] | Xie J, Zhang L, Fan X, et al. (2019) MicroRNA-146a improves sepsis-induced cardiomyopathy by regulating the TLR-4/NF-κB signaling pathway. Exp Ther Med 18: 779-785. |
[15] |
Curtale G, Mirolo M, Renzi TA, et al. (2013) Negative regulation of Toll-like receptor 4 signaling by IL-10-dependent microRNA-146b. P Natl Acad Sci USA 110: 11499-11504. doi: 10.1073/pnas.1219852110
![]() |
[16] |
Jung WJ, Lee SY, Choi SI, et al. (2018) Toll-like receptor expression in pulmonary sensory neurons in the bleomycin-induced fibrosis model. PLoS One 13: e0193117. doi: 10.1371/journal.pone.0193117
![]() |
[17] |
Stärkel P, Schnabl B, Leclercq S, et al. (2019) Deficient IL-6/stat3 signaling, high TLR7, and type I interferons in early human alcoholic liver disease: A triad for liver damage and fibrosis. Hepatol Commun 3: 867-882. doi: 10.1002/hep4.1364
![]() |
[18] |
Castellano G, Stasi A, Franzin R, et al. (2019) LPS-binding protein modulates acute renal fibrosis by inducing pericyte-to-myofibroblast trans-differentiation through TLR-4 signaling. Int J Mol Sci 20: 3682. doi: 10.3390/ijms20153682
![]() |
[19] |
Cáceres FT, Gaspari TA, Samuel CS, et al. (2019) Serelaxin inhibits the profibrotic TGF-β1/IL-1β axis by targeting TLR-4 and the NLRP3 inflammasome in cardiac myofibroblasts. FASEB J 33: 14717-14733. doi: 10.1096/fj.201901079RR
![]() |
[20] | Liu AJ, Wu PC, Ciou JR, et al. (2021) Differential expression of Toll-like receptors 1 and 3 in patients with systemic lupus erythematosus and systemic sclerosis. Research Square In press. |
[21] |
Bhattacharyya S, Varga J (2015) Emerging roles of innate immune signaling and toll-like receptors in fibrosis and systemic sclerosis. Curr Rheumatol Rep 17: 2. doi: 10.1007/s11926-014-0474-z
![]() |
[22] |
Beutler B (2009) Microbe sensing, positive feedback loops, and the pathogenesis of inflammatory diseases. Immunol Rev 227: 248-263. doi: 10.1111/j.1600-065X.2008.00733.x
![]() |
[23] |
West AP, Koblansky AA, Ghosh S (2006) Recognition and signaling by toll-like receptors. Annu Rev Cell Dev Biol 22: 409-437. doi: 10.1146/annurev.cellbio.21.122303.115827
![]() |
[24] |
Kawai T, Akira S (2011) Toll-like receptors and their crosstalk with other innate receptors in infection and immunity. Immunity 34: 637-650. doi: 10.1016/j.immuni.2011.05.006
![]() |
[25] |
Kumar H, Kawai T, Akira S (2011) Pathogen recognition by the innate immune system. Int Rev Immunol 30: 16-34. doi: 10.3109/08830185.2010.529976
![]() |
[26] |
Howell J, Angus P, Gow P, et al. (2013) Toll-like receptors in hepatitis C infection: Implications for pathogenesis and treatment. J Gastroen Hepatol 28: 766-776. doi: 10.1111/jgh.12170
![]() |
[27] |
Liu G, Zhao Y (2007) Toll-like receptors and immune regulation: their direct and indirect modulation on regulatory CD4+ CD25+ T cells. Immunology 122: 149-156. doi: 10.1111/j.1365-2567.2007.02651.x
![]() |
[28] |
Yao C, Oh JH, Lee DH, et al. (2015) Toll-like receptor family members in skin fibroblasts are functional and have a higher expression compared to skin keratinocytes. Int J Mol Med 35: 1443-1450. doi: 10.3892/ijmm.2015.2146
![]() |
[29] |
Price AE, Shamardani K, Lugo KA, et al. (2018) A map of toll-like receptor expression in the intestinal epithelium reveals distinct spatial, cell type-specific, and temporal patterns. Immunity 49: 560-575. doi: 10.1016/j.immuni.2018.07.016
![]() |
[30] |
Yamamoto M, Sato S, Hemmi H, et al. (2003) TRAM is specifically involved in the Toll-like receptor 4-mediated MyD88-independent signaling pathway. Nat Immunol 4: 1144-1150. doi: 10.1038/ni986
![]() |
[31] |
Yamamoto M, Sato S, Hemmi H, et al. (2003) Role of adaptor TRIF in the MyD88-independent toll-like receptor signaling pathway. Science 301: 640-643. doi: 10.1126/science.1087262
![]() |
[32] |
Meier A, Alter G, Frahm N, et al. (2007) MyD88-dependent immune activation mediated by human immunodeficiency virus type 1-encoded Toll-like receptor ligands. J Virol 81: 8180-8191. doi: 10.1128/JVI.00421-07
![]() |
[33] |
Cronin JG, Turner ML, Goetze L, et al. (2012) Toll-like receptor 4 and MYD88-dependent signaling mechanisms of the innate immune system are essential for the response to lipopolysaccharide by epithelial and stromal cells of the bovine endometrium. Biol Reprod 86: 51. doi: 10.1095/biolreprod.111.092718
![]() |
[34] |
Kawagoe T, Sato S, Matsushita K, et al. (2008) Sequential control of Toll-like receptor–dependent responses by IRAK1 and IRAK2. Nat Immunol 9: 684. doi: 10.1038/ni.1606
![]() |
[35] |
Kawai T, Akira S (2007) Signaling to NF-κB by Toll-like receptors. Trends Mol Med 13: 460-469. doi: 10.1016/j.molmed.2007.09.002
![]() |
[36] |
Akira S, Takeda K (2004) Toll-like receptor signalling. Nat Rev Immunol 4: 499-511. doi: 10.1038/nri1391
![]() |
[37] |
Adhikari A, Xu M, Chen ZJ (2007) Ubiquitin-mediated activation of TAK1 and IKK. Oncogene 26: 3214-3226. doi: 10.1038/sj.onc.1210413
![]() |
[38] |
Chen F, Bhatia D, Chang Q, et al. (2006) Finding NEMO by K63-linked polyubiquitin chain. Cell Death Differ 13: 1835. doi: 10.1038/sj.cdd.4402014
![]() |
[39] |
Anwar MA, Basith S, Choi S (2013) Negative regulatory approaches to the attenuation of Toll-like receptor signaling. Exp Mol Med 45: e11-e11. doi: 10.1038/emm.2013.28
![]() |
[40] |
Bibeau-Poirier A, Servant MJ (2008) Roles of ubiquitination in pattern-recognition receptors and type I interferon receptor signaling. Cytokine 43: 359-367. doi: 10.1016/j.cyto.2008.07.012
![]() |
[41] |
Wang C, Chen T, Zhang J, et al. (2009) The E3 ubiquitin ligase Nrdp1 ‘preferentially’ promotes TLR-mediated production of type I interferon. Nat Immunol 10: 744-752. doi: 10.1038/ni.1742
![]() |
[42] |
Wang T, Chuang TH, Ronni T, et al. (2006) Flightless I homolog negatively modulates the TLR pathway. J Immunol 176: 1355-1362. doi: 10.4049/jimmunol.176.3.1355
![]() |
[43] |
Chen Z, Zhou R, Zhang Y, et al. (2020) β-arrestin 2 quenches TLR signaling to facilitate the immune evasion of EPEC. Gut Microbes 11: 1423-1437. doi: 10.1080/19490976.2020.1759490
![]() |
[44] |
Kobayashi K, Hernandez LD, Galán JE, et al. (2002) IRAK-M is a negative regulator of Toll-like receptor signaling. Cell 110: 191-202. doi: 10.1016/S0092-8674(02)00827-9
![]() |
[45] |
Al-Shaghdali K, Durante B, Hayward C, et al. (2019) Macrophage subsets exhibit distinct E. coli-LPS tolerisable cytokines associated with the negative regulators, IRAK-M and Tollip. PLoS One 14: e0214681. doi: 10.1371/journal.pone.0214681
![]() |
[46] |
Jiang S, Li X, Hess NJ, et al. (2016) TLR10 is a negative regulator of both MyD88-dependent and-independent TLR signaling. J Immunol 196: 3834-3841. doi: 10.4049/jimmunol.1502599
![]() |
[47] |
Olmos-Ortiz A, Déciga-García M, Preciado-Martínez E, et al. (2019) Prolactin decreases LPS-induced inflammatory cytokines by inhibiting TLR-4/NFκB signaling in the human placenta. Mol Hum Reprod 25: 660-667. doi: 10.1093/molehr/gaz038
![]() |
[48] |
Cao Y, Sun Y, Chang H, et al. (2019) The E3 ubiquitin ligase RNF 182 inhibits TLR-triggered cytokine production through promoting p65 ubiquitination and degradation. FEBS Lett 593: 3210-3219. doi: 10.1002/1873-3468.13583
![]() |
[49] |
Song Y, Li P, Qin L, et al. (2021) CUL4B negatively regulates Toll-like receptor-triggered proinflammatory responses by repressing Pten transcription. Cell Mol Immunol 18: 339-349. doi: 10.1038/s41423-019-0323-0
![]() |
[50] |
Lou Y, Han M, Liu H, et al. (2020) Essential roles of S100A10 in Toll-like receptor signaling and immunity to infection. Cell Mol Immunol 17: 1053-1062. doi: 10.1038/s41423-019-0278-1
![]() |
[51] | Hu YH, Wang Y, Wang F, et al. (2020) SPOP negatively regulates Toll-like receptor-induced inflammation by disrupting MyD88 self-association. Cell Mol Immunol In press. |
[52] |
Jiang G, Gong M, Song H, et al. (2020) Tob2 Inhibits TLR-Induced Inflammatory Responses by Association with TRAF6 and MyD88. J Immunol 205: 981-986. doi: 10.4049/jimmunol.2000057
![]() |
[53] | Miyake K, Yamashita Y, Ogata M, et al. (1995) RP105, a novel B cell surface molecule implicated in B cell activation, is a member of the leucine-rich repeat protein family. J Immunol 154: 3333-3340. |
[54] |
Divanovic S, Trompette A, Atabani SF, et al. (2005) Negative regulation of Toll-like receptor 4 signaling by the Toll-like receptor homolog RP105. Nat Immunol 6: 571-578. doi: 10.1038/ni1198
![]() |
[55] |
Kimoto M, Nagasawa K, Miyake K (2003) Role of TLR4/MD-2 and RP105/MD-1 in innate recognition of lipopolysaccharide. Scand J Infect Dis 35: 568-572. doi: 10.1080/00365540310015700
![]() |
[56] |
Ogata H, Su I, Miyake K, et al. (2000) The toll-like receptor protein RP105 regulates lipopolysaccharide signaling in B cells. J Exp Med 192: 23-30. doi: 10.1084/jem.192.1.23
![]() |
[57] |
Nagai Y, Shimazu R, Ogata H, et al. (2002) Requirement for MD-1 in cell surface expression of RP105/CD180 and B-cell responsiveness to lipopolysaccharide. Blood 99: 1699-1705. doi: 10.1182/blood.V99.5.1699
![]() |
[58] |
Miyake K, Yamashita Y, Hitoshi Y, et al. (1994) Murine B cell proliferation and protection from apoptosis with an antibody against a 105-kD molecule: unresponsiveness of X-linked immunodeficient B cells. J Exp Med 180: 1217-1224. doi: 10.1084/jem.180.4.1217
![]() |
[59] |
Chan VWF, Mecklenbräuker I, Su I, et al. (1998) The molecular mechanism of B cell activation by toll-like receptor protein RP-105. J Exp Med 188: 93-101. doi: 10.1084/jem.188.1.93
![]() |
[60] |
Grumont RJ, Rourke IJ, O'Reilly LA, et al. (1998) B lymphocytes differentially use the Rel and nuclear factor κB1 (NF-κB1) transcription factors to regulate cell cycle progression and apoptosis in quiescent and mitogen-activated cells. J Exp Med 187: 663-674. doi: 10.1084/jem.187.5.663
![]() |
[61] |
Lardenoye JHP, Delsing DJM, De Vries MR, et al. (2000) Accelerated atherosclerosis by placement of a perivascular cuff and a cholesterol-rich diet in ApoE*3Leiden transgenic mice. Circ Res 87: 248-253. doi: 10.1161/01.RES.87.3.248
![]() |
[62] |
Allen JL, Flick LM, Divanovic S, et al. (2012) Cutting edge: regulation of TLR4-driven B cell proliferation by RP105 is not B cell autonomous. J Immunol 188: 2065-2069. doi: 10.4049/jimmunol.1103282
![]() |
[63] |
Karper JC, Ewing MM, de Vries MR, et al. (2013) TLR accessory molecule RP105 (CD180) is involved in post-interventional vascular remodeling and soluble RP105 modulates neointima formation. PLoS One 8: e67923. doi: 10.1371/journal.pone.0067923
![]() |
[64] |
Karper JC, de Jager SCA, Ewing MM, et al. (2013) An unexpected intriguing effect of Toll-like receptor regulator RP105 (CD180) on atherosclerosis formation with alterations on B-cell activation. Arterioscl Throm Vas 33: 2810-2817. doi: 10.1161/ATVBAHA.113.301882
![]() |
[65] |
Wezel A, van der Velden D, Maassen JM, et al. (2015) RP105 deficiency attenuates early atherosclerosis via decreased monocyte influx in a CCR2 dependent manner. Atherosclerosis 238: 132-139. doi: 10.1016/j.atherosclerosis.2014.11.020
![]() |
[66] |
Wezel A, De Vries MR, Maassen JM, et al. (2016) Deficiency of the TLR4 analogue RP105 aggravates vein graft disease by inducing a pro-inflammatory response. Sci Rep 6: 1-13. doi: 10.1038/srep24248
![]() |
[67] |
Yang J, Zeng P, Yang J, et al. (2019) The Role of RP105 in cardiovascular disease through regulating TLR4 and PI3K signaling pathways. Curr Med Sci 39: 185-189. doi: 10.1007/s11596-019-2017-3
![]() |
[68] |
Yang J, Guo X, Yang J, et al. (2015) RP105 protects against apoptosis in ischemia/reperfusion-induced myocardial damage in rats by suppressing TLR4-mediated signaling pathways. Cell Physiol Biochem 36: 2137-2148. doi: 10.1159/000430180
![]() |
[69] |
Li X, Yang J, Yang J, et al. (2016) RP105 protects against myocardial ischemia–reperfusion injury via suppressing TLR4 signaling pathways in rat model. Exp Mol Pathol 100: 281-286. doi: 10.1016/j.yexmp.2015.12.016
![]() |
[70] |
Xiong X, Liu Y, Mei Y, et al. (2017) Novel protective role of myeloid differentiation 1 in pathological cardiac remodelling. Sci Rep 7: 1-13. doi: 10.1038/s41598-016-0028-x
![]() |
[71] |
Guo X, Jiang H, Yang J, et al. (2016) Radioprotective 105 kDa protein attenuates ischemia/reperfusion-induced myocardial apoptosis and autophagy by inhibiting the activation of the TLR4/NF-κB signaling pathway in rats. Int J Mol Med 38: 885-893. doi: 10.3892/ijmm.2016.2686
![]() |
[72] | Guo X, Jiang H, Chen J, et al. (2018) RP105 ameliorates hypoxia/reoxygenation injury in cardiac microvascular endothelial cells by suppressing TLR4/MAPKs/NF-κB signaling. Int J Mol Med 42: 505-513. |
[73] |
Yang Y, Yang J, Liu X, et al. (2018) Down-regulation of miR-327 alleviates ischemia/reperfusion-induced myocardial damage by targeting RP105. Cell Physiol Biochem 49: 1090-1104. doi: 10.1159/000493288
![]() |
[74] |
Qin Q, Cui L, Zhou Z, et al. (2019) Inhibition of microRNA-141-3p reduces hypoxia-induced apoptosis in H9c2 rat cardiomyocytes by activating the RP105-dependent PI3K/AKT signaling pathway. Med Sci Monit 25: 7016. doi: 10.12659/MSM.916361
![]() |
[75] | Sun Y, Liu L, Yuan J, et al. (2018) RP105 protects PC12 cells from oxygen–glucose deprivation/reoxygenation injury via activation of the PI3K/AKT signaling pathway. Int J Mol Med 41: 3081-3089. |
[76] |
Yu CH, Micaroni M, Puyskens A, et al. (2015) RP105 engages phosphatidylinositol 3-kinase p110δ to facilitate the trafficking and secretion of cytokines in macrophages during mycobacterial infection. J Immunol 195: 3890-3900. doi: 10.4049/jimmunol.1500017
![]() |
[77] |
Yazawa N, Fujimoto M, Sato S, et al. (2003) CD19 regulates innate immunity by the toll-like receptor RP105 signaling in B lymphocytes. Blood 102: 1374-1380. doi: 10.1182/blood-2002-11-3573
![]() |
[78] |
Honda Y, Yamagiwa S, Matsuda Y, et al. (2007) Altered expression of TLR homolog RP105 on monocytes hypersensitive to LPS in patients with primary biliary cirrhosis. J Hepatol 47: 404-411. doi: 10.1016/j.jhep.2007.03.012
![]() |
[79] |
Zhang Z, La Placa D, Nguyen T, et al. (2019) CEACAM1 regulates the IL-6 mediated fever response to LPS through the RP105 receptor in murine monocytes. BMC Immunol 20: 1-16. doi: 10.1186/s12865-018-0284-6
![]() |
[80] |
Koarada S, Tada Y, Ushiyama O, et al. (1999) B cells lacking RP105, a novel B cell antigen, in systemic lupus erythematosus. Arthritis Rheum 42: 2593-2600. doi: 10.1002/1529-0131(199912)42:12<2593::AID-ANR12>3.0.CO;2-G
![]() |
[81] |
Kikuchi Y, Koarada S, Tada Y, et al. (2001) Difference in B cell activation between dermatomyositis and polymyositis: analysis of the expression of RP105 on peripheral blood B cells. Ann Rheum Dis 60: 1137-1140. doi: 10.1136/ard.60.12.1137
![]() |
[82] |
Koarada S, Tada Y, Kikuchi Y, et al. (2001) CD180 (RP105) in rheumatic diseases. Rheumatology 40: 1315-1316. doi: 10.1093/rheumatology/40.11.1315
![]() |
[83] | Koarada S, Tada Y (2012) RP105-negative B cells in systemic lupus erythematosus. Clin Dev Immunol 2012: 1-5. |
[84] | Koarada S, Tada Y, Suematsu R, et al. (2012) Phenotyping of P105-negative B cell subsets in patients with systemic lupus erythematosus. Clin Dev Immunol 2012: 1-8. |
[85] |
Korganow AS, Knapp AM, Nehme-Schuster H, et al. (2010) Peripheral B cell abnormalities in patients with systemic lupus erythematosus in quiescent phase: decreased memory B cells and membrane CD19 expression. J Autoimmun 34: 426-434. doi: 10.1016/j.jaut.2009.11.002
![]() |
[86] |
Erdő-Bonyár S, Rapp J, Minier T, et al. (2019) Toll-Like receptor mediated activation of natural autoantibody producing b cell subpopulations in an autoimmune disease model. Int J Mol Sci 20: 6152. doi: 10.3390/ijms20246152
![]() |
[87] |
Harhaj EW, Dixit VM (2012) Regulation of NF-κB by deubiquitinases. Immunol Rev 246: 107-124. doi: 10.1111/j.1600-065X.2012.01100.x
![]() |
[88] |
Aksentijevich I, Zhou Q (2017) NF-κB pathway in autoinflammatory diseases: dysregulation of protein modifications by ubiquitin defines a new category of autoinflammatory diseases. Front Immunol 8: 399. doi: 10.3389/fimmu.2017.00399
![]() |
[89] |
Keusekotten K, Elliott PR, Glockner L, et al. (2013) OTULIN antagonizes LUBAC signaling by specifically hydrolyzing Met1-linked polyubiquitin. Cell 153: 1312-1326. doi: 10.1016/j.cell.2013.05.014
![]() |
[90] |
Dixit VM, Green S, Sarma V, et al. (1990) Tumor necrosis factor-alpha induction of novel gene products in human endothelial cells including a macrophage-specific chemotaxin. J Biol Chem 265: 2973-2978. doi: 10.1016/S0021-9258(19)39896-5
![]() |
[91] |
Kinsella S, Fichtner M, Watters O, et al. (2018) Increased A20-E3 ubiquitin ligase interactions in bid-deficient glia attenuate TLR3- and TLR4-induced inflammation. J Neuroinflammation 15: 1-12. doi: 10.1186/s12974-018-1143-3
![]() |
[92] |
Bhattacharyya S, Varga J (2018) Endogenous ligands of TLR4 promote unresolving tissue fibrosis: Implications for systemic sclerosis and its targeted therapy. Immunol Lett 195: 9-17. doi: 10.1016/j.imlet.2017.09.011
![]() |
[93] |
Feng H, Pyykkö I, Zou J (2016) Involvement of ubiquitin-editing protein A20 in modulating inflammation in rat cochlea associated with silver nanoparticle-induced CD68 upregulation and TLR4 activation. Nanoscale Res Lett 11: 1-13. doi: 10.1186/s11671-015-1209-4
![]() |
[94] |
Krikos A, Laherty CD, Dixit VM (1992) Transcriptional activation of the tumor necrosis factor alpha-inducible zinc finger protein, A20, is mediated by kappa B elements. J Biol Chem 267: 17971-17976. doi: 10.1016/S0021-9258(19)37138-8
![]() |
[95] |
Opipari AW, Boguski MS, Dixit VM (1990) The A20 cDNA induced by tumor necrosis factor alpha encodes a novel type of zinc finger protein. J Biol Chem 265: 14705-14708. doi: 10.1016/S0021-9258(18)77165-2
![]() |
[96] |
Catrysse L, Vereecke L, Beyaert R, et al. (2014) A20 in inflammation and autoimmunity. Trends Immunol 35: 22-31. doi: 10.1016/j.it.2013.10.005
![]() |
[97] |
Lee EG, Boone DL, Chai S, et al. (2000) Failure to regulate TNF-induced NF-κB and cell death responses in A20-deficient mice. Science 289: 2350-2354. doi: 10.1126/science.289.5488.2350
![]() |
[98] |
Lu TT, Onizawa M, Hammer GE, et al. (2013) Dimerization and ubiquitin mediated recruitment of A20, a complex deubiquitinating enzyme. Immunity 38: 896-905. doi: 10.1016/j.immuni.2013.03.008
![]() |
[99] |
Wertz IE, O'rourke KM, Zhou H, et al. (2004) De-ubiquitination and ubiquitin ligase domains of A20 downregulate NF-κB signalling. Nature 430: 694-699. doi: 10.1038/nature02794
![]() |
[100] |
Wertz IE, Newton K, Seshasayee D, et al. (2015) Phosphorylation and linear ubiquitin direct A20 inhibition of inflammation. Nature 528: 370-375. doi: 10.1038/nature16165
![]() |
[101] |
Mauro C, Pacifico F, Lavorgna A, et al. (2006) ABIN-1 binds to NEMO/IKKγ and co-operates with A20 in inhibiting NF-κB. J Biol Chem 281: 18482-18488. doi: 10.1074/jbc.M601502200
![]() |
[102] | Ha H, Han D, Choi Y (2009) TRAF-mediated TNFR-family signaling. Curr Protoc Immunol 87: 11. |
[103] |
Heyninck K, Beyaert R (1999) The cytokine-inducible zinc finger protein A20 inhibits IL-1-induced NF-κB activation at the level of TRAF6. FEBS Lett 442: 147-150. doi: 10.1016/S0014-5793(98)01645-7
![]() |
[104] |
De A, Dainichi T, Rathinam CV, et al. (2014) The deubiquitinase activity of A 20 is dispensable for NF-κ B signaling. EMBO Rep 15: 775-783. doi: 10.15252/embr.201338305
![]() |
[105] |
Boone DL, Turer EE, Lee EG, et al. (2004) The ubiquitin-modifying enzyme A20 is required for termination of Toll-like receptor responses. Nat Immunol 5: 1052-1060. doi: 10.1038/ni1110
![]() |
[106] |
Harhaj EW, Dixit VM (2011) Deubiquitinases in the regulation of NF-κB signaling. Cell Res 21: 22-39. doi: 10.1038/cr.2010.166
![]() |
[107] | Lin FT, Lin VY, Lin VTG, et al. (2016) TRIP6 antagonizes the recruitment of A20 and CYLD to TRAF6 to promote the LPA2 receptor-mediated TRAF6 activation. Cell Discovery 2: 1-22. |
[108] |
Saitoh T, Yamamoto M, Miyagishi M, et al. (2005) A20 is a negative regulator of IFN regulatory factor 3 signaling. J Immunol 174: 1507-1512. doi: 10.4049/jimmunol.174.3.1507
![]() |
[109] |
Feoktistova M, Makarov R, Brenji S, et al. (2020) A20 Promotes Ripoptosome Formation and TNF-Induced Apoptosis via cIAPs Regulation and NIK Stabilization in Keratinocytes. Cells 9: 351. doi: 10.3390/cells9020351
![]() |
[110] |
Li L, Huang B, Song S, et al. (2017) A20 functions as mediator in TNFα-induced injury of human umbilical vein endothelial cells through TAK1-dependent MAPK/eNOS pathway. Oncotarget 8: 65230. doi: 10.18632/oncotarget.18191
![]() |
[111] |
Li Y, Mooney EC, Holden SE, et al. (2019) A20 orchestrates inflammatory response in the oral mucosa through restraining NF-κB activity. J Immunol 202: 2044-2056. doi: 10.4049/jimmunol.1801286
![]() |
[112] |
Li Y, Mooney EC, Xia XJ, et al. (2020) A20 restricts inflammatory response and desensitizes gingival keratinocytes to apoptosis. Front Immunol 11: 365. doi: 10.3389/fimmu.2020.00365
![]() |
[113] |
Martens A, Priem D, Hoste E, et al. (2020) Two distinct ubiquitin-binding motifs in A20 mediate its anti-inflammatory and cell-protective activities. Nat Immunol 21: 381-387. doi: 10.1038/s41590-020-0621-9
![]() |
[114] |
Soni D, Wang DM, Regmi SC, et al. (2018) Deubiquitinase function of A20 maintains and repairs endothelial barrier after lung vascular injury. Cell Death Discovery 4: 1-14. doi: 10.1038/s41420-018-0056-3
![]() |
[115] |
Mohebiany AN, Ramphal NS, Karram K, et al. (2020) Microglial A20 protects the brain from CD8 T-cell-mediated immunopathology. Cell Rep 30: 1585-1597. doi: 10.1016/j.celrep.2019.12.097
![]() |
[116] |
Ma A, Malynn BA (2012) A20: linking a complex regulator of ubiquitylation to immunity and human disease. Nat Rev Immunol 12: 774-785. doi: 10.1038/nri3313
![]() |
[117] |
Malynn BA, Ma A (2019) A20: a multifunctional tool for regulating immunity and preventing disease. Cell Immunol 340: 103914. doi: 10.1016/j.cellimm.2019.04.002
![]() |
[118] |
Hövelmeyer N, Reissig S, Thi Xuan N, et al. (2011) A20 deficiency in B cells enhances B-cell proliferation and results in the development of autoantibodies. Eur J Immunol 41: 595-601. doi: 10.1002/eji.201041313
![]() |
[119] |
Tavares RM, Turer EE, Liu CL, et al. (2010) The ubiquitin modifying enzyme A20 restricts B cell survival and prevents autoimmunity. Immunity 33: 181-191. doi: 10.1016/j.immuni.2010.07.017
![]() |
[120] |
Chu Y, Vahl JC, Kumar D, et al. (2011) B cells lacking the tumor suppressor TNFAIP3/A20 display impaired differentiation and hyperactivation and cause inflammation and autoimmunity in aged mice. Blood 117: 2227-2236. doi: 10.1182/blood-2010-09-306019
![]() |
[121] |
Hammer GE, Turer EE, Taylor KE, et al. (2011) Expression of A20 by dendritic cells preserves immune homeostasis and prevents colitis and spondyloarthritis. Nat Immunol 12: 1184-1193. doi: 10.1038/ni.2135
![]() |
[122] |
Matmati M, Jacques P, Maelfait J, et al. (2011) A20 (TNFAIP3) deficiency in myeloid cells triggers erosive polyarthritis resembling rheumatoid arthritis. Nat Genet 43: 908-912. doi: 10.1038/ng.874
![]() |
[123] |
Vereecke L, Sze M, Guire CM, et al. (2010) Enterocyte-specific A20 deficiency sensitizes to tumor necrosis factor-induced toxicity and experimental colitis. J Exp Med 207: 1513-1523. doi: 10.1084/jem.20092474
![]() |
[124] |
Mooney EC, Sahingur SE (2021) The ubiquitin system and A20: implications in health and disease. J Dent Res 100: 10-20. doi: 10.1177/0022034520949486
![]() |
[125] |
Razani B, Whang MI, Kim FS, et al. (2020) Non-catalytic ubiquitin binding by A20 prevents psoriatic arthritis–like disease and inflammation. Nat Immunol 21: 422-433. doi: 10.1038/s41590-020-0634-4
![]() |
[126] |
Yoon CI, Ahn SG, Bae SJ, et al. (2019) High A20 expression negatively impacts survival in patients with breast cancer. PLoS One 14: e0221721. doi: 10.1371/journal.pone.0221721
![]() |
[127] |
Lee YH, Song GG (2012) Associations between TNFAIP3 gene polymorphisms and systemic lupus erythematosus: a meta-analysis. Genet Test Mol Biomarkers 16: 1105-1110. doi: 10.1089/gtmb.2012.0096
![]() |
[128] |
Korman BD, Criswell LA (2015) Recent advances in the genetics of systemic sclerosis: toward biological and clinical significance. Curr Rheumatol Rep 17: 1-11. doi: 10.1007/s11926-014-0484-x
![]() |
[129] |
Mayes MD, Bossini-Castillo L, Gorlova O, et al. (2014) Immunochip analysis identifies multiple susceptibility loci for systemic sclerosis. Am J Hum Genet 94: 47-61. doi: 10.1016/j.ajhg.2013.12.002
![]() |
[130] |
Martin JE, Assassi S, Diaz-Gallo LM, et al. (2013) A systemic sclerosis and systemic lupus erythematosus pan-meta-GWAS reveals new shared susceptibility loci. Hum Mol Genet 22: 4021-4029. doi: 10.1093/hmg/ddt248
![]() |
[131] |
Dieude P, Guedj M, Wipff J, et al. (2010) Association of the TNFAIP3 rs5029939 variant with systemic sclerosis in the European Caucasian population. Ann Rheum Dis 69: 1958-1964. doi: 10.1136/ard.2009.127928
![]() |
[132] |
Koumakis E, Giraud M, Dieudé P, et al. (2013) SAT0013 Candidate gene study in systemic sclerosis identifies a rare and functional variant of TNFAIP3 locus as a risk factor for individual polyautoimmunity. Ann Rheum Dis 71: 475-475. doi: 10.1136/annrheumdis-2012-eular.1670
![]() |
[133] |
Liu Y, Ye Z, Li X, et al. (2017) Genetic and functional associations with decreased anti-inflammatory tumor necrosis factor alpha induced protein 3 in macrophages from subjects with axial spondyloarthritis. Front Immunol 8: 860. doi: 10.3389/fimmu.2017.00860
![]() |
[134] |
Sun YY, Fan YC, Wang N, et al. (2015) Increased A20 mRNA level in peripheral blood mononuclear cells is associated with immune phases of patients with chronic hepatitis B. Medicine 94: e2428. doi: 10.1097/MD.0000000000002428
![]() |
[135] |
Xu H, Wang L, Zheng P, et al. (2017) Elevated serum A20 is associated with severity of chronic hepatitis B and A20 inhibits NF-κB-mediated inflammatory response. Oncotarget 8: 38914. doi: 10.18632/oncotarget.17153
![]() |
[136] |
Hung YY, Lin CC, Kang HY, et al. (2017) TNFAIP3, a negative regulator of the TLR signaling pathway, is a potential predictive biomarker of response to antidepressant treatment in major depressive disorder. Brain Behav Immun 59: 265-272. doi: 10.1016/j.bbi.2016.09.014
![]() |
[137] |
Huang H, Tang QZ, Wang AB, et al. (2010) Tumor suppressor A20 protects against cardiac hypertrophy and fibrosis by blocking transforming growth factor-β-activated kinase 1-dependent signaling. Hypertension 56: 232-239. doi: 10.1161/HYPERTENSIONAHA.110.149963
![]() |
[138] |
Xu W, Wang C, Liang M, et al. (2018) A20 prevents obesity-induced development of cardiac dysfunction. J Mol Med 96: 159-172. doi: 10.1007/s00109-017-1608-3
![]() |
[139] | Jung SM, Lee JH, Park J, et al. (2013) Smad6 inhibits non-canonical TGF-β1 signalling by recruiting the deubiquitinase A20 to TRAF6. Nat Commun 4: 1-16. |
[140] |
Liu S, Lv X, Liu C, et al. (2019) Targeting degradation of the transcription factor C/EBPβ reduces lung fibrosis by restoring activity of the ubiquitin-editing enzyme A20 in macrophages. Immunity 51: 522-534. doi: 10.1016/j.immuni.2019.06.014
![]() |
[141] |
Wang X, Ai L, Xu Q, et al. (2017) A20 attenuates liver fibrosis in NAFLD and inhibits inflammation responses. Inflammation 40: 840-848. doi: 10.1007/s10753-017-0528-2
![]() |
[142] | Kelly C, Reihill J, Malconsom B, et al. (2013) Defective A20 signalling in CF: Anti-inflammatory action of gibberellins. Eur Respir J 42: 2106. |
[143] |
Malcomson B, Wilson H, Veglia E, et al. (2016) Connectivity mapping (ssCMap) to predict A20-inducing drugs and their antiinflammatory action in cystic fibrosis. P Natl Acad Sci USA 113: E3725-E3734. doi: 10.1073/pnas.1520289113
![]() |
[144] |
Bhattacharyya S, Wang W, Graham LVD, et al. (2016) A20 suppresses canonical Smad-dependent fibroblast activation: novel function for an endogenous inflammatory modulator. Arthritis Res Ther 18: 1-10. doi: 10.1186/s13075-016-1118-7
![]() |
[145] |
Hand LE, Usan P, Cooper GJS, et al. (2015) Adiponectin induces A20 expression in adipose tissue to confer metabolic benefit. Diabetes 64: 128-136. doi: 10.2337/db13-1835
![]() |
[146] |
Carrion AM, Link WA, Ledo F, et al. (1999) DREAM is a Ca2+-regulated transcriptional repressor. Nature 398: 80-84. doi: 10.1038/18044
![]() |
[147] |
Cebolla B, Fernández-Pérez A, Perea G, et al. (2008) DREAM mediates cAMP-dependent, Ca2+-induced stimulation of GFAP gene expression and regulates cortical astrogliogenesis. J Neurosci 28: 6703-6713. doi: 10.1523/JNEUROSCI.0215-08.2008
![]() |
[148] |
Savignac M, Mellström B, Bébin AG, et al. (2010) Increased B cell proliferation and reduced Ig production in DREAM transgenic mice. J Immunol 185: 7527-7536. doi: 10.4049/jimmunol.1000152
![]() |
[149] |
Tiruppathi C, Soni D, Wang DM, et al. (2014) The transcription factor DREAM represses the deubiquitinase A20 and mediates inflammation. Nat Immunol 15: 239-247. doi: 10.1038/ni.2823
![]() |
[150] |
Naranjo JR, Zhang H, Villar D, et al. (2016) Activating transcription factor 6 derepression mediates neuroprotection in Huntington disease. J Clin Invest 126: 627-638. doi: 10.1172/JCI82670
![]() |
[151] |
Lopez-Hurtado A, Peraza DA, Cercos P, et al. (2019) Targeting the neuronal calcium sensor DReAM with small-molecules for Huntington's disease treatment. Sci Rep 9: 1-16. doi: 10.1038/s41598-019-43677-7
![]() |
[152] |
Bhattacharyya S, Wang W, Tamaki Z, et al. (2018) Pharmacological inhibition of toll-like receptor-4 signaling by TAK242 prevents and induces regression of experimental organ fibrosis. Front Immunol 9: 2434. doi: 10.3389/fimmu.2018.02434
![]() |
[153] |
Bhattacharyya S, Wang W, Graham LVD, et al. (2016) A20 suppresses canonical Smad-dependent fibroblast activation: novel function for an endogenous inflammatory modulator. Arthritis Res Ther 18: 1-10. doi: 10.1186/s13075-016-1118-7
![]() |
Parameters | Description | Value | Source |
β1 | The transmission rate for strain 1 | 0.5 day−1 | Assumed |
β2 | The transmission rate for strain 2 | 0.7 day−1 | Assumed |
γ1 | Recovery rate after infection by strain 1 | 1/11 day−1 | [30] |
γ2 | Recovery rate after infection by strain 2 | 1/9 day−1 | [30] |
γ3 | Recovery rate after infection by strain 1 and strain 2 | 1/14 day−1 | Assumed |
e1 | Effectiveness of vaccination at protecting against strain 1 | 0.61 | [31] |
e2 | Effectiveness of vaccination at protecting against strain 2 | 0.33 | [31] |
State/Strategy | Healthy | Infected with strain 1 | Infected with strain 2 | Coinfected with strain 1 and strain 2 |
Vaccination (V) | πHV=−Cr | πI1V=−Cr−1 | πI2V=−Cr−1 | πIV=−Cr−2 |
Non-vaccination (NV) | πSFR=0 | πFFR1=−1 | πFFR2=−1 | πFFR=−2 |
Parameters | Description | Value | Source |
β1 | The transmission rate for strain 1 | 0.5 day−1 | Assumed |
β2 | The transmission rate for strain 2 | 0.7 day−1 | Assumed |
γ1 | Recovery rate after infection by strain 1 | 1/11 day−1 | [30] |
γ2 | Recovery rate after infection by strain 2 | 1/9 day−1 | [30] |
γ3 | Recovery rate after infection by strain 1 and strain 2 | 1/14 day−1 | Assumed |
e1 | Effectiveness of vaccination at protecting against strain 1 | 0.61 | [31] |
e2 | Effectiveness of vaccination at protecting against strain 2 | 0.33 | [31] |
State/Strategy | Healthy | Infected with strain 1 | Infected with strain 2 | Coinfected with strain 1 and strain 2 |
Vaccination (V) | πHV=−Cr | πI1V=−Cr−1 | πI2V=−Cr−1 | πIV=−Cr−2 |
Non-vaccination (NV) | πSFR=0 | πFFR1=−1 | πFFR2=−1 | πFFR=−2 |