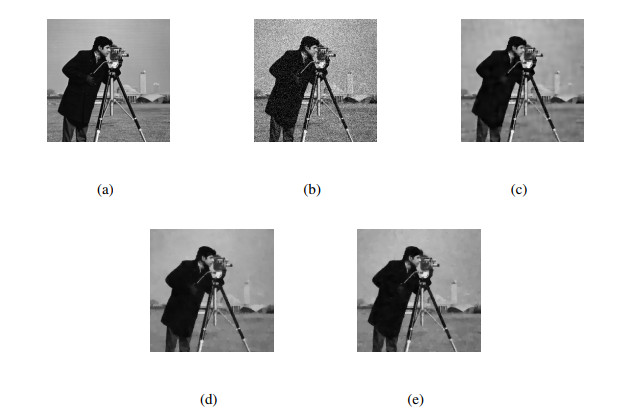
Noise is regarded as an unavoidable component of digital image acquisition. Hence, noise removal has been considered as one of the fundamental tasks in the field of image processing. Accordingly, excellent results have been achieved by using second-order models. However, these outcomes are affected by the staircase effect. To eliminate this anomaly and maintaining the balance of removing noise and preserving edges, a fourth-order model is proposed. The existence and uniqueness of the entropy solution for this model are established. Besides, to to verify the effectiveness of the model in noise removal, we carried out numerical experiment and presented our results. Indeed, the experimental results show that our model is superior to PM model and ROF model in terms of removing noise and preserving edges.
Citation: Abdelgader Siddig, Zhichang Guo, Zhenyu Zhou, Boying Wu. Entropy solutions for an adaptive fourth-order nonlinear degenerate problem for noise removal[J]. AIMS Mathematics, 2021, 6(4): 3974-3995. doi: 10.3934/math.2021236
[1] | Yazid Alhojilan, Islam Samir . Investigating stochastic solutions for fourth order dispersive NLSE with quantic nonlinearity. AIMS Mathematics, 2023, 8(7): 15201-15213. doi: 10.3934/math.2023776 |
[2] | Azadeh Ghanadian, Taher Lotfi . Approximate solution of nonlinear Black–Scholes equation via a fully discretized fourth-order method. AIMS Mathematics, 2020, 5(2): 879-893. doi: 10.3934/math.2020060 |
[3] | Donghong Zhao, Ruiying Huang, Li Feng . Proximity algorithms for the $ {\mathit{L}}^{1}{\mathit{L}}^{2}/{\mathit{T}\mathit{V}}^{\mathit{\alpha }} $ image denoising model. AIMS Mathematics, 2024, 9(6): 16643-16665. doi: 10.3934/math.2024807 |
[4] | Shiyv Wang, Xueqin Lv, Songyan He . The reproducing kernel method for nonlinear fourth-order BVPs. AIMS Mathematics, 2023, 8(11): 25371-25381. doi: 10.3934/math.20231294 |
[5] | Xing Zhang, Lei Liu, Yan-Jun Liu . Adaptive NN control based on Butterworth low-pass filter for quarter active suspension systems with actuator failure. AIMS Mathematics, 2021, 6(1): 754-771. doi: 10.3934/math.2021046 |
[6] | Lufeng Bai . A new approach for Cauchy noise removal. AIMS Mathematics, 2021, 6(9): 10296-10312. doi: 10.3934/math.2021596 |
[7] | Muhammad Asim Khan, Norma Alias, Umair Ali . A new fourth-order grouping iterative method for the time fractional sub-diffusion equation having a weak singularity at initial time. AIMS Mathematics, 2023, 8(6): 13725-13746. doi: 10.3934/math.2023697 |
[8] | Yanhong Zhang, Li Chen . Positive solution for a class of nonlinear fourth-order boundary value problem. AIMS Mathematics, 2023, 8(1): 1014-1021. doi: 10.3934/math.2023049 |
[9] | Dongdong Ruan, Xiaofeng Wang . On the convergence of a new fourth-order method for finding a zero of a derivative. AIMS Mathematics, 2024, 9(4): 10353-10362. doi: 10.3934/math.2024506 |
[10] | Khaled Kefi . Existence and multiplicity of triple weak solutions for a nonlinear elliptic problem with fourth-order operator and Hardy potential. AIMS Mathematics, 2024, 9(7): 17758-17773. doi: 10.3934/math.2024863 |
Noise is regarded as an unavoidable component of digital image acquisition. Hence, noise removal has been considered as one of the fundamental tasks in the field of image processing. Accordingly, excellent results have been achieved by using second-order models. However, these outcomes are affected by the staircase effect. To eliminate this anomaly and maintaining the balance of removing noise and preserving edges, a fourth-order model is proposed. The existence and uniqueness of the entropy solution for this model are established. Besides, to to verify the effectiveness of the model in noise removal, we carried out numerical experiment and presented our results. Indeed, the experimental results show that our model is superior to PM model and ROF model in terms of removing noise and preserving edges.
Noise is the most difficult task in the field of image processing and computer vision. In this work, we focus on removing additive Gaussian noise. The problem is formulated mathematically as: let u(x,y) be a digital image and u0(x,y) be its observation with random noise η(x,y). For (x,y)∈Ω s.t.
u0(x,y)=u(x,y)+η(x,y). | (1.1) |
The noise level is approximately known
‖u−u0‖2L2(Ω)=∫Ω(u−u0)2dx≈σ2. | (1.2) |
The goal of denoising is to filter out high frequency signals, while preserving the important features of the image such as edges. Therefore, we search for an image processing model which removes noise and offers better handling of edges.
During the last two decades, the method of partial differential equations (PDEs) for image processing has become a major research topic. A classical PDEs model named ROF (Rudin, Osher, Fatemi) model which was based on the total variation (TV), was first introduced by Rudin et al. [18]. The idea in the ROF model is to minimize the the total variation of the image u,
TV=minu∈BV(Ω)∩L2(Ω)∫Ω|∇u|dx+λ2∫Ω(u−u0)2dx. | (1.3) |
In fact, one of the main advantages of using ROF model for image restoration is that the discontinuities are allowed. However, the main drawback of denoising models based on the TV is that they tend to yield piecewise constant images, a phenomenon known as staircase effects. Strong [19], introduced an adaptive TV functional (TVα)
TVα=minu∈BV(Ω)∩L2(Ω)∫Ωα(x)|∇u(x)|dx+λ2∫Ω(u−u0)2dx, | (1.4) |
for spatially adaptive image restoration. The function α(x) is an edge detector to control the diffusion. The main idea of edge detector is that edges of an image are associated with location of high gradient in a slightly smooth version of the noisy image.
In the recent decades, to overcome the staircase effects that caused by second-order variational model, fourth-order PDEs have been introduced in image restoration, [1,2,3,4,9,10,12,14,16,20,22,24,25,26,27,28]. You-Kaveh [26] proposed the following functional
∫Ωf(|△u|)dxdy, | (1.5) |
where △ denote the Laplacian operator. Based on the gradient descent method, this second order functional yields a fourth-order PDE
ut=−△(g((△u)2)△u), | (1.6) |
where g(s)=k2/(k2+s2) and k is an image dependent parameter. This equation does avoid blocky piecewise constant solution. However, it produces speckles in the processed image [16]. Many other authors have considered image denoising models based on the minimizer of high-order functionals. Laysaker et al. [16] proposed the LLT model
∫Ω(|uxx|+|uyy|)dxdy, | (1.7) |
and
∫Ω√|uxx|2+|uxy|2+|uyx|2+|uyy|2dxdy, | (1.8) |
they try to minimize the TV of ∇u. Minimizing these two functionals is equivalent to solve the following PDEs respectively
ut=−(uxx∣uxx∣)xx−(uyy∣uyy∣)yy | (1.9) |
and
ut=−(uxx∣D2u∣)xx−(uxy∣D2u∣)yx−(uyx∣D2u∣)xy−(uyy∣D2u∣)yy, | (1.10) |
where ∣D2u∣=√|uxx|2+|uxy|2+|uyx|2+|uyy|2. These equations have proved to be the improved version of (1.6).
The theoretical analysis showed that fourth-order equations have advantages over second-order equations in some aspects. Fourth-order PDEs usually produce the smooth image of the observed image. This is believed to be a better approximation in smooth region. Therefore, the staircase effect is suggested to be reduced and the recovery image will look better. It is reasonable to conclude that fourth-order diffusion performs better than the second-order models in the aspect of the recovery of smooth regions.
In this paper, to address the problem of denoising images contaminated with additive noise, a fourth-order model is suggested. Using the gradient module of the image to design a speed controlling function. This function indicated where is the edge in the image, thus the new model can preserve edge in this region. The motivation for proposing this model is to overcome certain inconsistencies in second order models founded during the process of recovering smooth regions and better preservation of the fine details. The model is based on solving a nonlinear fourth order degenerate equation with the noisy image as its initial data. By use of Roth's method, we proved the existence and uniqueness of the entropy solution. Additionally, the numerical results demonstrate that the proposed model is superior to PM (Perona, Malik) [17] and ROF models.
The rest of this article is organized as follows. In Section 2, we give some preliminaries that we will use. Section 3 is devoted to the proposed model and the proofs of existence and uniqueness of its solution. The difference schemes are presented in Section 4. Numerical experiments are presented in Section 5 and the conclusion of this paper is given in Section 6.
In this section, we recall some necessary definitions and notations, [11,13,14]. We begin with some definitions of the space BV2, which consists of functions u∈W1,1(Ω) s.t ∇u∈BV(Ω), this space is also denoted by BH(Ω). To know more about space of bounded Hessian, we refer the reader to [4,5,8].
Definition 2.1. Let Ω⊆Rn be a bounded open domain with Lipschitz boundary. Let u∈L1(Ω). Then the BV2 semi-norm of u is characterized by
||D2u||=supϕ∈C20(Ω,Rn×n){∫Ωn∑i,j=1u∂j∂iϕijdx:|ϕ(x)|≤1,∀x∈Ω}<∞, | (2.1) |
where C20(Ω,X) is the space of functions from Ω to X, 2-times continuously differentiable with compact support and ϕ(x)is a vector valued function, with |ϕ(x)|=√∑ni,j=1(ϕij)2. Here we remark that the space BV2 equipped with ‖u‖BV2(Ω)=||D2u||+‖u‖L1(Ω) is a Banach space.
Definition 2.2. Suppose that Ω⊆Rn be a bounded open domain with Lipschitz boundary, u∈L1(Ω), and α(x)≥0 is continuous and real function. Then we define the weighted BV2 semi-norm of u as
||D2u||α=supϕ∈C20(Ω,Rn×n){∫Ωn∑i,j=1u∂j∂iϕijdx:|ϕ(x)|≤α,∀x∈Ω}<∞ | (2.2) |
In this section, we propose a fourth-order image denoising model, with some guidance from previous work [6,14,15,16,26,27]. There are some benefits of fourth-order models. On the one hand, it can remove high frequency oscillation more effectively than second-order models because the evaluation of the second-order becomes weak in the high frequency area. One the other hand, for the fourth-order model, there is flexibility in employing different functional behaviors in the formulation.
Consider the following boundary value problem
∂u∂t+ D2ij(α(x) D2iju|D2iju|)=0(x,t)∈ΩT=(0,T)×Ω, | (3.1) |
u(x,t)=0,(x,t)∈(0,T)×∂Ω, | (3.2) |
∂u∂→n=0,(x,t)∈(0,T)×∂Ω, | (3.3) |
u(x,0)=u0(x),x∈Ω, | (3.4) |
where α(x)=1√1+|Gσ∗∇u0|2, Gσ(x) is the Gaussian filter with parameter σ, u0(x) is the original image, Ω is bounded domain of R2 with appropriate smooth boundary, T>0 is fixed, →n denote the unit outward normal of the boundary ∂Ω.
The term α(x) is used to enhance edges. In fact, it controls the speed of the diffusion: in the smooth region where ∇u0 is small, the diffusion is strong. Near possible edges, however, where ∇u0 is large, the diffusion spread is low. The convolution with Gσ should smooth away any large oscillations of noise. Therefore, we can get the smooth image and further preserve the edges in a best way.
Definition 3.1. A measurable function u:ΩT→R is an entropy solution of (3.1)–(3.4) in ΩT if u∈C([0,T];L2(Ω))∩L∞(0,T;BV2(Ω)),∂u∂t∈L2(ΩT) and there exist z, such that αz∈L∞(ΩT) with ∥αz∥L∞(ΩT)≤1,ut+Dijαzij=0 in D′(ΩT) such that
∫Ω(u(t)−v)utdx≤∫Ωαz(t)⋅D2vdx−||D2u||α, | (3.5) |
for every v∈L∞(0,T;W2,10(Ω)).
Before investigating the existence and uniqueness of problem (3.1)–(3.4), let us consider the following approximate evaluation problem: for 1<p≤2 and u0p∈W2,p(Ω), we construct the following problem
∂up∂t+ D2ij(α(x)|D2ijup|p−2D2ijup)=0,(x,t)∈ΩT | (3.6) |
up(x,t)=0,(x,t)∈(0,T)×∂Ω, | (3.7) |
∂up∂→n=0,(x,t)∈(0,T)×∂Ω, | (3.8) |
up(x,0)=u0p(x),x∈Ω. | (3.9) |
Lemma 3.1. For any fixed p, 1<p≤2, the above problem (3.6)–(3.9) admits a weak solution up∈L∞(0,T;W2,p0(Ω))∩C([0,T];L2(Ω)) and ∂up∂t∈L2(ΩT) such that
limt→0+∥up(x,t)−u0p(x)∥L2(Ω)=0, | (3.10) |
and for any φ∈C∞0(ΩT) the following integral equality holds
∫T0∫Ω∂up∂tφ(x,t)dxdt+∫T0∫Ωα(x)| D2ijup|p−2 D2ijup⋅D2ijφ(x,t)dxdt=0, | (3.11) |
with
∥up∥L∞(0,T;W2,p0(Ω))+∥up∥L∞(0,T;L2(Ω))+‖∂up∂t‖L2(ΩT)≤C, | (3.12) |
where C is a constant independent of p.
Proof. We apply Rothe's method [23], to construct an approximate solution sequence. Divide the interval [0,T] into n equal segments and define h=Tn. For any j: 1≤j≤n, for any positive integer n and a function u(x,t), denote
un,jp(x)=up(x,jh),j=1,2,...,n. |
For fixed j, define the following functional on W2,p0(Ω)
E(w)=1p∫Ωα(x)|D2ijw|pdx+12h∫Ω(w−un,j−1p)2dx. | (3.13) |
The idea here is to prove that if un,j−1p is known and un,0p=u0p, then there is a minimizer for (3.13).
Let um∈W2,p0(Ω)∩L2(Ω) be a minimizing sequence for E. Since α is bounded below, then the sequence um is bounded in W2,p0(Ω) and L2(Ω). Therefore, there exists a subsequence umiof um and a function un,jp∈W2,p0(Ω)∩L2(Ω) such that as i→∞,
umi→un,jpweakly in W2,p0(Ω)andL2(Ω). | (3.14) |
From this and the weak lower semicontinuity of the norms, we get
E(un,jp)≤lim infi→∞E(umi)=infw∈W2,p0(Ω)∩L2(Ω)E(w). |
Then un,jp is the solution of the Euler equation corresponding to E(w)
D2ij(α(x)|D2ijun.jp|p−2D2ijun.jp)+1h(un.jp−un,j−1p)=0, | (3.15) |
which implies
1h∫Ω(un,jp−un,j−1p)η(x)dx+∫Ωα(x)|D2ijun,jp|p−2 D2ijun,jp⋅D2ijη(x)dx=0, | (3.16) |
for any η(x)∈C∞0(Ω).
Let χn,j(t) be the indicator function of [h(j−1),hj) and
λn,j(t)={th−(j−1),if t∈[h(j−1),hj),0,otherwise. |
We construct an approximation function as
unp(x,t)=n∑j=1χn,j(t)un,jp, withunp(x,0)=u0p(x) |
and
wnp(x,t)=n∑j=1χn,j(t)[un,j−1p(x)+λn,j(t)(un,jp(x)−un,j−1p(x))]. |
By (3.16), we have
∫Ω(∂wnp∂tη(x)+α(x)|D2ijunp∣p−2D2ijunp⋅D2ijη(x))dx=0, |
for every η(x)∈C∞0(Ω) a.e. t∈[0,T], which implies that
∫T0∫Ω(∂wnp∂tφ(x,t)+α(x)|D2ijunp|p−2D2ijunp⋅D2ijφ(x,t))dxdt=0, | (3.17) |
for every φ∈C∞0(ΩT).
Next, we obtain some estimates for unp(x,t) and wnp(x,t). Notice that, we choose η(x)∈W2,p0(Ω) as the test function in (3.16). Let η(x)=un,jp−un,j−1p, we have
1h∫Ω(un,jp−un,j−1p)2dx+∫Ωα(x)|D2ijun,jp|p−2 D2ijun,jp⋅D2ij(un,jp−un,j−1p)dx=0, |
1h∫Ω(un,jp−un,j−1p)2dx+∫Ωα(x)|D2ijun,jp|pdx=∫Ωα(x)|D2ijun,jp|p−2 D2ijun,jp⋅D2ijun,j−1pdx. |
Using Young's inequality, we have
1h∫Ω(un,jp−un,j−1p)2dx+∫Ωα(x)|D2ijun,jp|pdx≤p−1p∫Ωα(x)|D2ijun,jp|pdx+1p∫Ωα(x)|D2ijun,j−1p|pdx. |
That is
1h∫Ω(un,jp−un,j−1p)2dx+1p∫Ωα(x)|D2ijun,jp|pdx≤1p∫Ωα(x)|D2ijun,j−1p|pdx. | (3.18) |
For any m with 1≤m≤n, summing (3.18) for j from 1 to m
∫Ωα(x)|D2ijun,mp|pdx≤∫Ωα(x)|D2iju0p|pdx, |
which implies
sup0<t<T‖D2ijunp‖pW2,p0(Ω)≤C, | (3.19) |
where C is a constant independent of p,n.
Summing (3.18) for j from 1 to n yield
1hn∑j=1∫Ω(un,jp−un,j−1p)2dx≤1p∫Ωα(x)|D2iju0p|pdx=C. | (3.20) |
By the definition of wnp(x,t), we have
∂wnp∂t=1hn∑j=1χn,j(t)(un,jp−un,j−1p). |
Thus
‖∂wnp∂t‖2L2(ΩT)=1h2n∑j=1h‖(un,jp−un,j−1p)‖2L2(Ω)≤C. | (3.21) |
By (3.19), we can obtain
sup0<t<T∫Ω| D2ijwnp|pdx=sup0<t<Tn∑j=1χn,j(t)∫Ω|(1−λn,j(t)) D2ijun,j−1p+λn,j(t) D2ijun,jp|pdx≤sup0<t<T‖ D2ijunp‖pW2,p0(Ω)≤C. | (3.22) |
Choosing η(x)=un,jp in (3.16), we get
1h∫Ω(un,jp−un,j−1p)un,jpdx+∫Ωα(x)|D2ijun,jp|p−2 D2ijun,jp⋅D2ijun,jpdx=0, |
which implies
1h∫Ω(un,jp−un,j−1p)un,jpdx+∫Ωα(x)|D2ijun,jp|pdx=0. |
By Young's inequality, we have
h∫Ωα(x)|D2ijun,jp|pdx+12∫Ω|un,jp|2dx≤12∫Ω|un,j−1p|2dx. | (3.23) |
Thus
∫Ω|un,jp|2dx≤∫Ω|un,j−1p|2dx. | (3.24) |
This implies
sup0<t<T∫Ω|unp|2dx≤∫Ω|u0p|2dx. | (3.25) |
Similar to the proof of (3.22), we also see
sup0<t<T∫Ω|wnp|2dx≤∫Ω|u0p|2dx. | (3.26) |
Choosing η(x)=un,j−1p in (3.16)
∫Ω(un,jp−un,j−1p)un,j−1pdx+h∫Ωα(x)|D2ijun,jp|p−2 D2ijun,jp⋅D2ijun,j−1pdx=0.′ |
Applying Hölder's inequality and the estimate (3.19), we have
∫Ω(un,j−1p−un,jp)un,j−1pdx≤Ch. |
By Young's inequality again yields
∫Ω|un,j−1p|2dx≤Ch+12∫Ω|un,j−1p|2dx+12∫Ω|un,jp|2dx. |
Thus
−Ch≤∫Ω|un,jp|2dx−∫Ω|un,j−1p|2dx. | (3.27) |
Define B(unp)=α(x)| D2ijunp|p−2 D2ijunp. Combining (3.19), (3.21), (3.22), (3.25) and (3.26), we conclude that there exist subsequences of unp,wnp,∂wnp∂t and B(unp), denoted by themselves such that, as n→∞
unp∗⇀up,inL∞(0,T;W2,p0(Ω)),wnp∗⇀wp,inL∞(0,T;W2,p0(Ω)),∂wnp∂t⇀∂wp∂t,inL2(ΩT),B(unp)∗⇀ζ,inL∞(0,T;Lp′(Ω)), | (3.28) |
holds for some up,wp,ζ. And we also have
‖wp‖pL∞(0,T;W2,p0(Ω))+‖∂wp∂t‖2L2(ΩT)+‖up‖pL∞(0,T;W2,p0(Ω))≤C. | (3.29) |
Then let n→∞ in (3.17),
∂wp∂t+ D2ijζ=0. | (3.30) |
Next, we show that up=wp. By the definition of up and wp, we have
wnp−unp=n∑j=1χn,j(t)(1−λn,j(t))(un,j−1p−un,jp), |
which combined with (3.20), leads to
‖wnp−unp‖2L2(ΩT)≤n∑j=1h‖un,jp−un,j−1p‖2L2(ΩT)≤Ch2→0,as h→0. | (3.31) |
Now, it remains to show that ζ=B(up). From (3.17) and the convergence sets (3.28), as n→∞, we can get
∫T0∫Ω∂up∂tφ(x,t)dxdt+∫T0∫Ωζ.D2ijφ(x,t)dxdt=0. | (3.32) |
For any g∈Lp(0,T,W2,p(Ω)) and for j from 1 to n, we can obtain, by the monotonicity condition, the inequality
∫Ω(B(un.jp)−B(g))(D2ijun.jp−D2ijg)dx≥0. | (3.33) |
Letting η=un.jp in (3.16), we obtain
1h∫Ω(un,jp−un,j−1p)un,jpdx+∫ΩB(un,jp)⋅D2ijun,jpdx=0. | (3.34) |
Applying Young's inequality on the first term of (3.34) together with the inequality (3.33) and integrating over ((j−1)h,jh), we get
12∫Ω[|un,jp|2−|un,j−1p|2]dx+∫jh(j−1)h∫ΩB(un,jp)⋅D2ijgdxdt+∫jh(j−1)h∫ΩB(g)(D2ijun.jp−D2ijg)dxdt≤0. | (3.35) |
Summing up (3.35) for j from 1 to n, we obtain
12∫Ω[|unp(T)|2−|u0p|2]dx+∫T0∫ΩB(unp)⋅D2ijgdxdt+∫T0∫ΩB(g)(D2ijunp−D2ijg)dxdt≤0. | (3.36) |
Recalling the convergence sets (3.28) and letting n→∞, (3.36) yields
12∫Ω[|up(T)|2−|u0p|2]dx+∫T0∫Ωζ⋅D2ijgdxdt+∫T0∫ΩB(g)(D2ijup−D2ijg)dxdt≤0. | (3.37) |
We can rewrite (3.37) in the form
∫T0∫Ω∂up∂tupdxdt+∫T0∫Ωζ⋅D2ijgdxdt+∫T0∫ΩB(g)(D2ijup−D2ijg)dxdt≤0. | (3.38) |
Letting φ=up in (3.30), we obtain
∫T0∫Ω∂up∂tupdxdt+∫T0∫Ωζ⋅D2ijupdxdt=0. | (3.39) |
Then, substituting (3.39) into (3.38) leads to
∫T0∫Ω(ζ−B(g))(D2ijup−D2ijg)dxdt≥0. | (3.40) |
Choose g=up−ks where k>0 and D2ijs∈L∞(0,T;W2,p(Ω)). We then have
∫T0∫Ω(ζ−B(up−ks))D2ijsdxdt≥0. | (3.41) |
Sending k→0, we obtain
∫T0∫Ω(ζ−B(up))D2ijsdxdt≥0,∀s∈L∞(0,T;W2,p(Ω)). | (3.42) |
Since s is arbitrary, we see that ζ=B(up).
Now, we prove (3.10), we let φ=up(x,t) and φ=up(x,t1) in (3.30), for 0≤t1≤t≤t2≤T, we obtain
∫Ω(u2p(x,t2)−u2p(x,t1))dx=−2∫t2t1∫Ωα(x)| D2ijup|pdxdt, |
and
∫Ωup(x,t2)up(x,t1)dx−∫Ωu2p(x,t1)dx=−∫t2t1∫Ωα(x)| D2ijup|p−2 D2ijup.D2ijup(x,t1)dxdt. |
Then
∫Ω|up(x,t2)−up(x,t1)|2dx=∫Ω(u2p(x,t2)−u2p(x,t1))dx+2∫Ω(u2p(x,t1)−up(x,t2)up(x,t1))dx,=−2∫t2t1∫Ωα(x)| D2ijup|pdxdt+2∫t2t1∫Ωα(x)| D2ijup|p−2 D2ijup.D2ijup(x,t1)dxdt. |
From the above equation, we deduce that
limt→0+∥up(x,t)−u0p(x)∥L2(Ω)=0, |
and the proof is completed.
Theorem 3.1. If u0∈BV2(Ω) and u0=0,∂u∂n=0,x∈∂Ω in the sense of trace then the problem (3.1)–(3.4) admits one and only one entropy solution.
Proof. By Lemma 3.1, there exists up, which is a weak solution of the problem (3.6)–(3.9) and a constant C such that
∥up∥L∞(0,T;W2,p0(Ω))+∥up∥L∞(0,T;L2(Ω))+‖∂up∂t‖L2(ΩT)≤C. | (3.43) |
So, from (3.43), there exists a subsequence of up, denoted by itself and a function u∈L∞(0,T;BV2(Ω))∩C([0,T];L2(Ω)) with ∂u∂t∈L2(ΩT) such that, as p→1+,
up→u,in W1,1(Ω),with ||D2u||α≤lim infp→1+‖D2ijup‖Lp(Ω),a.e. t∈(0,T) |
and
∂up∂t⇀∂u∂t, weakly in L2(ΩT).
We also have up→u strongly in L2(ΩT)a.e. t∈(0,T) and
limt→0+‖u(x,t)−u0(x)‖L2(Ω)=0. |
Applying the method in [7], we next prove that α(x)|D2ijup|p−2 D2ijup is weakly relatively compact in L1(ΩT). Employing (3.43) and Hölder's inequality,
|∫T0∫Ωα(x)|D2ijup|p−2D2ijupdxdt|≤∫T0∫Ω∣α(x)∣∣D2ijup∣p−1dxdt≤Cp−1pmeas(ΩT)1p, |
where C is independent of p. Thus, {α(x)|D2ijup|p−2D2ijup} is bounded and equi-integrable in L1(ΩT) and is therefore weakly relatively compact in L1(ΩT). Thus we deduce that as p→1+,
{α(x)|D2ijup|p−2 D2ijup}⇀αz, weakly in L1(ΩT).
So we get by Lemma 3.1 and the fact that ∂up∂t⇀∂u∂t in L2(ΩT),
∫T0∫Ω∂u∂tφ(x,t)dxdt+∫T0∫Ωαz⋅D2ijφ(x,t)dxdt=0, | (3.44) |
for every φ(x,t)∈C∞0(ΩT) and ut+Dijαzij=0 in D′(ΩT).
Now, it remains to prove that ∥αz∥L∞(ΩT)≤1.
For any k>0, setting
Ap,k={(x,t)∈ΩT:∣D2ijup∣>k}, we have that
meas(Ap,k)≤Ckp, for every p>1,k>0. |
As above, there exists a function gk∈L1(ΩT) such that
α(x)|D2ijup|p−2 D2ijupχAp,k⇀gk, as p→1+ weakly in L1(ΩT),
where χAp,k is the indicator function of Ap,k. Now for any ϕ∈L∞(ΩT) with
‖ϕ‖L∞(ΩT)≤1, by the definition of χAp,k, we see that
|∫T0∫Ωα(x)|D2ijup|p−2 D2ijupϕχAp,kdxdt|≤Ck. |
Letting p→1+, we have
∫T0∫Ω∣gk∣dxdt≤Ck,for every k>0. | (3.45) |
Since we have that
|∫T0∫Ωα(x)|D2ijup|p−2 D2ijupχΩT/Ap,k|≤kp−1, for any p>1, |
letting p→1+, we obtain that α(x)∣D2ijup∣p−2 D2ijupχΩT/Ap,k weakly converges in L1(ΩT) to some function fk∈L1(ΩT) with ‖fk‖L∞(ΩT)≤1. Since, for any k>0, we may write αz=fk+gk with ‖fk‖L∞(ΩT)≤1 and gk satisfies (3.45), it is easily follows that ‖αz‖L∞(ΩT)≤1.
Next, we verify the solution definition inequality (3.5). For any vn∈C∞0(ΩT) and taking φ=(up−vn)ξ(t) in (3.11), we have
∫T0∫Ω∂up∂t(up−vn)ξ(t)dxdt=−∫T0∫Ωα(x)|D2ijup|p−2 D2ijup⋅D2ij((up−vn)ξ(t))dxdt. |
Letting p→1+,
∫T0∫Ω∂u∂t(u(t)−vn)ξ(t)dxdt≤∫T0∫Ωαz(t)⋅D2ijvnξ(t)dxdt−∫T0||D2u||αξ(t)dt. |
Then for any v∈L∞(0,T;W2,10(Ω)), letting n→∞,
∫T0∫Ω∂u∂t(u(t)−v)ξ(t)dxdt≤∫T0∫Ωαz(t)⋅D2ijvξ(t)dxdt−∫T0||D2u||αξ(t)dt. |
Since ξ(t) is arbitrary, we have
∫Ω∂u∂t(u(t)−v)dx≤∫Ωαz(t)⋅D2ijvdx−||D2u||α, |
for every v∈L∞(0,T;W2,10(Ω)) and a.e. on [0,T].
Finally, we prove the uniqueness of the entropy solution. Let u1,u2 both be entropy solution with data u10,u20. Then there exists αz1,αz2∈L∞(ΩT) such that
∫Ω∂u1∂t(u1−v)dx≤∫Ωαz1⋅D2ijvdx−||D2u1||α, | (3.46) |
and
∫Ω∂u2∂t(u2−v)dx≤∫Ωαz2⋅D2ijvdx−||D2u2||α, | (3.47) |
for every v∈L∞(0,T;W2,10(Ω)) and a.e. on [0,T]. Let u1n,u2n∈L∞(0,T;W2,p0(Ω)) be approximates functions, respectively, for u1 and u2, such that
limn→∞(‖D2iju1n‖L1(Ω)−||D2u1||α)=0,limn→∞∥u1n−u1∥L2(Ω)=0, |
and
limn→∞(‖D2iju2n‖L1(Ω)−||D2u2||α)=0,limn→∞∥u2n−u2∥L2(Ω)=0, |
a.e. on[0,T]. Taking v=u2n in (3.46) and v=u1n in (3.47), adding the two equations and rearranging the result, we obtain
∫Ω(u1−u2)(∂u1∂t−∂u2∂t)dx+∫Ω(u1−u1n)∂u2∂tdx+∫Ω(u2−u2n)∂u1∂t≤∫Ωαz1⋅D2iju2ndx−||D2u1||α+∫Ωαz2⋅D2iju1ndx−||D2u2||α. |
So integrating from 0 to t and letting n→∞, we get
∫Ω(u1−u2)2dx≤∫Ω(u10−u20)2dx. |
The proof is completed.
In this section, assuming τ to be the time step size and h the space grid size, we discretize time and space as follows:
t=nτ,n=0,1,2,⋯,x=ih,i=0,1,2,⋯,I,y=jh,j=0,1,2,⋯,J, |
where Ih×Jh is the size of the original image. Let uni,j denote approximations of u(nτ,ih,jh). We define the discrete approximation:
△xuni,j=uni+1,j−2uni,j+uni−1,jh2,△yuni,j=uni,j+1−2uni,j+uni,j−1h2,△xyuni,j=uni+1,j+1+uni,j−uni,j+1−uni+1,jh2. |
The discrete explicit scheme of the problem can be written as
un+1i,j=uni,j−τ[△x(αij△xuni,j|△xuni,j|ϵ)+△y(αij△yuni,j|△yuni,j|ϵ)+△xy(αij△xyuni,j|△xyuni,j|ϵ)],αi,j=1√1+|Gσ∗∇u0(x)|2i,j,|⋅|ϵ=|⋅|+ϵ,ϵ>0,u0i,j=u0(ih,jh),0≤i≤I,0≤j≤J,uni,0=uni,1,un0,j=un1,j,unI,j=unI−1,j,uni,J=uni,J−1,uni,0=0,un0,j=0,unI,j=0,uni,J=0. |
Here the MATLAB function "conv2" is used to represent the two-dimensional discrete Convolution Transform of the matrix ui,j.
In this section, we demonstrate the performance of our model in denoising images involving Gaussian white noise. We applied difference equations discussed in section 4 and compared the results with the results of ROF model [18] and PM model [17]. We used step size τ=0.02, gride size h=1 and λ=0.
At the end of the denoising process, the peak signal to noise ratio (PSNR), mean absolute-deviation error (MAE) and structure similarity index measure (SSIM) values were recorded to measure the denoising performance. The values are given by the following formulas:
PSNR(u,u0)=10 log10IJ|maxu0−minu0|2‖u−u0‖2L2dB |
and
MAE(u,u0)=‖u−u0‖L1IJ, |
where |maxu0−minu0| gives the gray-scale range of the original image, u0 and u denote, respectively, the original image and the denoised image, I×J is the dimension of image.
SSIM, designed by Wang et al.[21], is a quality used to measure the similarity between any two images. Given any two images u and u0, SSIM is given by the formula
SSIM(u,u0)=L(u,u0)⋅C(u,u0)⋅R(u,u0). |
L(u,u0)=2μuμu0+k1μ2u+μ2u0+k1, compares the two images' mean luminance μu and μu0. The maximal value of L(u,u0)=1, if μu=μu0, C(u,u0)=2σuσu0+k2σ2u+σ2u0+k2, measures the closeness of contrast of the two images u and u0. Contrast is determined in terms of standard deviation, σ. Contrast comparison measure C(u,u0)=1 maximally if and only if σu=σu0; that is, when the images have equal contrast.
R(u,u0)=σuu0+k3σuσu0+k3, is a structure comparison measure which determines the correlation between the images u and u0, where σuu0 is covariance between u and u0. It attains maximal value of 1 if, structurally, the two images coincide, but its value is equal to zero when there is absolutely no structural coincidence. The quantities k1,k2 and k3 are small positive perturbations that avert the possibility of having zero denominators.
Two test images of "Cameraman" and "Peppers" are corrupted by white Gaussian noise with standard deviation (SD) of 30, (Figures 1 and 2). Tables 1 and 2, present the numerical results of restoration of Cameraman image, (Figure 1), and those of the Peppers image, (Figure 2). The comparisons are based on PSNR, MAE and SSIM. The proposed method shows the best performance with respect to PSNR, MAE and SSIM.
Algorithm | σ | PSNR | MAE | SSIM |
PM model | 30 | 27.98 | 7.06 | 0.8345 |
ROF model | 30 | 28.26 | 6.80 | 0.8359 |
Our Method | 30 | 28.66 | 6.44 | 0.8537 |
Algorithm | σ | PSNR | MAE | SSIM |
PM model | 20 | 28.89 | 5.83 | 0.8386 |
ROF model | 20 | 28.76 | 5.85 | 0.8397 |
Our Method | 20 | 29.04 | 5.62 | 0.8450 |
Our first example is Cameraman image, which is displayed in Figure 1a and 1b is its degraded version. Furthermore, Figure 1c, 1d and 1e, are portions of the recovered images with the proposed model, ROF model and PM model, respectively. It is clear that our method can overcome the staircase effect that caused by the second order method.
The second example is Peppers image, which is displayed in Figure 2a, its degraded version is showed in Figure 2b. Basically, Figure 2c, 2d and 2e, are portions of the recovered images by the proposed model, ROF model and PM model, respectively. It is evident that, our method yields good results in restoring image since it avoids the staircase effect that caused by the second order method while, at the same time, handle edges in a best way.
Similarly, the two test images are corrupted by white Gaussian noise with SD of 20, (Figures 3 and 4). Tables 3 and 4, present the numerical results of restoration of Cameraman image, (Figure 3), and those of the Peppers image, (Figure 4). The comparisons are based on PSNR, MAE and SSIM. Here again, the proposed method shows the best performance with respect to PSNR, MAE and SSIM. In Figure 3a and 3b we display Cameraman image and the noisy version. Figure 3c, 3d and 3e, are portions of the recovered images with the proposed model, ROF model and PM model, respectively. We display Peppers image and the degraded version in Figure 4a and 4b. Figure 4c, 4d and 4e, are portions of the recovered images with the proposed model, ROF model and PM model, respectively. Here also, the proposed model yields better results in denoising image while handling edges in a best way.
Algorithm | σ | PSNR | MAE | SSIM |
PM model | 20 | 29.86 | 5.70 | 0.8727 |
ROF model | 20 | 29.90 | 5.64 | 0.8741 |
Our Method | 20 | 30.48 | 5.18 | 0.8795 |
Image | Algorithm | σ | PSNR | MAE | SSIM |
YK | 30 | 26.08 | 8.62 | 0.7275 | |
LLT | 30 | 27.10 | 8.11 | 0.7554 | |
Lena | Ours | 30 | 27.46 | 7.11 | 0.8078 |
YK | 20 | 27.73 | 6.97 | 0.8082 | |
LLT | 20 | 29.18 | 6.39 | 0.8211 | |
Ours | 20 | 29.20 | 5.89 | 0.8421 | |
YK | 30 | 25.80 | 9.31 | 0.7053 | |
LLT | 30 | 26.83 | 8.69 | 0.7258 | |
Barbara | Ours | 30 | 27.07 | 8.00 | 0.7520 |
YK | 20 | 27.13 | 7.98 | 0.7584 | |
LLT | 20 | 28.28 | 7.29 | 0.7794 | |
Ours | 20 | 28.46 | 6.79 | 0.7945 |
Not surprisingly, although the edges are preserved, the staircase effect is visible for the second order models, and there are some speckles in the processed images, with an example given in Figure 2. Comparing the images processed by our model and the original images, we can observed that, the differences are insignificant. The edges are preserved and no speckles appear in the processed images.
Finally, to illustrate the superiority of the proposed model over other related fourth-order models, we compared our results with YK model [26] and LLT model [16]. Barbara and Lena images have been corrupted by white Gaussian noise with SD of 30 (Figures 5 and 6) and SD of 20 (Figures 7 and 8). Numerical results for the images are tabulated in Table 4. Besides getting better outcomes, as evident from the results (see Figures 5 and 6), the model tackles the speckles caused by YK model at the same time.
In Figures 6 and 8, the results of Lena Image have been displayed. In Figure 6, the test image Lena and its noisy version degraded by Gaussian noise with SD of 30 are shown in the sections (a) and (b), sections (c) to (e) are the results of the YK model, LLT model and the proposed one. Similarly, in Figure 8, the test image Lena and its noisy version degraded by Gaussian noise with SD of 20 are shown with the same order described above. The last image in section (e) of Figures 6 and 8 are the results of our suggested filter in which the extent of the denoising performance is noticeably better than competitor filter.
In this article, we proposed a fourth-order image denoising model. The model was based on solving a fourth order partial differential equation by defining its corresponding functional. We proved, by use of Rothe's method, the existence and uniqueness of the entropy solution of the equation. Compared with the well known ROF and PM models, numerical results showed that our model perform better image recovery and can overcome staircase effects.
The authors declare that they have no conflict of interests whatsoever and do approve the publication of this paper.
[1] | T. Barbu, PDE-based restoration model using nonlinear second and fourth order diffusions, Proc. Romanian Acad. Ser. A, 16 (2015), 138–146. |
[2] | T. Barbu, I. Munteanu, A nonlinear fourth-order diffusion-based model for image denoising and restoration, Proc. Romanian Acad. Ser. A, 18 (2017), 108–115. |
[3] |
C. Brito-Loeza, K. Chen, V. Uc-Cetina, Image denoising using the gaussian curvature of the image surface, Numer. Meth. Part. Differ. Equ., 32 (2016), 1066–1089. doi: 10.1002/num.22042
![]() |
[4] |
L. Deng, H. Zhu, Z. Yang, Y. Li, Hessian matrix-based fourth-order anisotropic diffusion filter for image denoising, Opt. Laser Technol., 110 (2019), 184–190. doi: 10.1016/j.optlastec.2018.08.043
![]() |
[5] |
I. Fonseca, G. Leoni, J. Malỳ, R. Paroni, A note on meyers' theorem in Wk,1, Trans. Am. Math. Soc., 354 (2002), 3723–3741. doi: 10.1090/S0002-9947-02-03027-1
![]() |
[6] |
P. Guidotti, K. Longo, Well-posedness for a class of fourth order diffusions for image processing, Nonlinear Differ. Equ. Appl. NoDEA, 18 (2011), 407–425. doi: 10.1007/s00030-011-0101-x
![]() |
[7] |
Z. Guo, J. Yin, Q. Liu, On a reaction-diffusion system applied to image decomposition and restoration, Math. Comput. Modell., 53 (2011), 1336–1350. doi: 10.1016/j.mcm.2010.12.031
![]() |
[8] |
W. Hinterberger, O. Scherzer, Variational methods on the space of functions of bounded hessian for convexification and denoising, Computing, 76 (2006), 109–133. doi: 10.1007/s00607-005-0119-1
![]() |
[9] | A. Laghrib, A. Chakib, A. Hadri, A. Hakim, A nonlinear fourth-order pde for multi-frame image super-resolution enhancement, Discrete Cont. Dyn. Syst.-B, 25 (2020), 415. |
[10] |
A. Laghrib, A. Hadri, A. Hakim, An edge preserving high-order pde for multiframe image super-resolution, J. Franklin Inst., 356 (2019), 5834–5857. doi: 10.1016/j.jfranklin.2019.02.032
![]() |
[11] |
F. Li, C. Shen, J. Fan, C. Shen, Image restoration combining a total variational filter and a fourth-order filter, J. Vis. Commun. Image R., 18 (2007), 322–330. doi: 10.1016/j.jvcir.2007.04.005
![]() |
[12] |
G. Liu, T. Z. Huang, J. Liu, High-order TVL1-based images restoration and spatially adapted regularization parameter selection, Comput. Math. Appl., 67 (2014), 2015–2026. doi: 10.1016/j.camwa.2014.04.008
![]() |
[13] |
Q. Liu, Z. Yao, Y. Ke, Entropy solutions for a fourth-order nonlinear degenerate problem for noise removal, Nonlinear Anal., 67 (2007), 1908–1918. doi: 10.1016/j.na.2006.08.016
![]() |
[14] |
X. Liu, L. Huang, Z. Guo, Adaptive fourth-order partial differential equation filter for image denoising, Appl. Math. Lett., 24 (2011), 1282–1288. doi: 10.1016/j.aml.2011.01.028
![]() |
[15] |
X. Liu, C. H. Lai, K. Pericleous, A fourth-order partial differential equation denoising model with an adaptive relaxation method, Int. J. Comput. Math., 92 (2015), 608–622. doi: 10.1080/00207160.2014.904854
![]() |
[16] |
M. Lysaker, A. Lundervold, X. C. Tai, Noise removal using fourth-order partial differential equation with applications to medical magnetic resonance images in space and time, IEEE Trans. Image Proc., 12 (2003), 1579–1590. doi: 10.1109/TIP.2003.819229
![]() |
[17] |
P. Perona, J. Malik, Scale-space and edge detection using anisotropic diffusion, IEEE Trans. Pattern Anal. Mach. Intell., 12 (1990), 629–639. doi: 10.1109/34.56205
![]() |
[18] |
L. I. Rudin, S. Osher, E. Fatemi, Nonlinear total variation based noise removal algorithms, Phys. D: Nonlinear Phenom., 60 (1992), 259–268. doi: 10.1016/0167-2789(92)90242-F
![]() |
[19] | D. M. Strong, Adaptive total variation minimizing image restoration, Department of Mathematics, University of California, Los Angeles, 1997. |
[20] |
L. Sun, K. Chen, A new iterative algorithm for mean curvature-based variational image denoising, BIT Numer. Math., 54 (2014), 523–553. doi: 10.1007/s10543-013-0448-y
![]() |
[21] |
Z. Wang, A. C. Bovik, H. R. Sheikh, E. P. Simoncelli, Image quality assessment: From error visibility to structural similarity, IEEE Trans. Image Proc., 13 (2004), 600–612. doi: 10.1109/TIP.2003.819861
![]() |
[22] | Y. Wen, J. Sun, Z. Guo, A new anisotropic fourth-order diffusion equation model based on image feature for image denoising, CAM report, 2020. |
[23] | Z. Wu, J. Yin, C. Wang, Elliptic & parabolic equations, World Scientific Publishing Company Incorporated, 2006. |
[24] |
X. Y. Liu, C. H. Lai, K. A. Pericleous, A fourth-order partial differential equation denoiding model with an adaptive relaxation method, Int. J. Comut. Math., 92 (2015), 608–622. doi: 10.1080/00207160.2014.904854
![]() |
[25] | Q. Yang, Image denoising combining the P-M model and the LLT model, J. Comput. Commun., 3 (2015), 22–30. |
[26] |
Y. L. You, M. Kaveh, Fourth-order partial differential equations for noise removal, IEEE Trans. Image Proc., 9 (2000), 1723–1730. doi: 10.1109/83.869184
![]() |
[27] |
X. Zhang, W. Ye, An adaptive fourth-order partial differential equation for image denoising, Comput. Math. Appl., 74 (2017), 2529–2545. doi: 10.1016/j.camwa.2017.07.036
![]() |
[28] |
W. Zhu, T. Chan, Image denoising using mean curvature of image surface, SIAM J. Imaging Sci., 5 (2012), 1–32. doi: 10.1137/110822268
![]() |
1. | Ahmed Nokrane, Nour Eddine Alaa, Fatima Aqel, Fourth-order nonlinear degenerate problem for image decomposition, 2023, 4, 2662-2963, 10.1007/s42985-023-00251-1 |
Algorithm | σ | PSNR | MAE | SSIM |
PM model | 30 | 27.98 | 7.06 | 0.8345 |
ROF model | 30 | 28.26 | 6.80 | 0.8359 |
Our Method | 30 | 28.66 | 6.44 | 0.8537 |
Algorithm | σ | PSNR | MAE | SSIM |
PM model | 20 | 28.89 | 5.83 | 0.8386 |
ROF model | 20 | 28.76 | 5.85 | 0.8397 |
Our Method | 20 | 29.04 | 5.62 | 0.8450 |
Algorithm | σ | PSNR | MAE | SSIM |
PM model | 20 | 29.86 | 5.70 | 0.8727 |
ROF model | 20 | 29.90 | 5.64 | 0.8741 |
Our Method | 20 | 30.48 | 5.18 | 0.8795 |
Image | Algorithm | σ | PSNR | MAE | SSIM |
YK | 30 | 26.08 | 8.62 | 0.7275 | |
LLT | 30 | 27.10 | 8.11 | 0.7554 | |
Lena | Ours | 30 | 27.46 | 7.11 | 0.8078 |
YK | 20 | 27.73 | 6.97 | 0.8082 | |
LLT | 20 | 29.18 | 6.39 | 0.8211 | |
Ours | 20 | 29.20 | 5.89 | 0.8421 | |
YK | 30 | 25.80 | 9.31 | 0.7053 | |
LLT | 30 | 26.83 | 8.69 | 0.7258 | |
Barbara | Ours | 30 | 27.07 | 8.00 | 0.7520 |
YK | 20 | 27.13 | 7.98 | 0.7584 | |
LLT | 20 | 28.28 | 7.29 | 0.7794 | |
Ours | 20 | 28.46 | 6.79 | 0.7945 |
Algorithm | σ | PSNR | MAE | SSIM |
PM model | 30 | 27.98 | 7.06 | 0.8345 |
ROF model | 30 | 28.26 | 6.80 | 0.8359 |
Our Method | 30 | 28.66 | 6.44 | 0.8537 |
Algorithm | σ | PSNR | MAE | SSIM |
PM model | 20 | 28.89 | 5.83 | 0.8386 |
ROF model | 20 | 28.76 | 5.85 | 0.8397 |
Our Method | 20 | 29.04 | 5.62 | 0.8450 |
Algorithm | σ | PSNR | MAE | SSIM |
PM model | 20 | 29.86 | 5.70 | 0.8727 |
ROF model | 20 | 29.90 | 5.64 | 0.8741 |
Our Method | 20 | 30.48 | 5.18 | 0.8795 |
Image | Algorithm | σ | PSNR | MAE | SSIM |
YK | 30 | 26.08 | 8.62 | 0.7275 | |
LLT | 30 | 27.10 | 8.11 | 0.7554 | |
Lena | Ours | 30 | 27.46 | 7.11 | 0.8078 |
YK | 20 | 27.73 | 6.97 | 0.8082 | |
LLT | 20 | 29.18 | 6.39 | 0.8211 | |
Ours | 20 | 29.20 | 5.89 | 0.8421 | |
YK | 30 | 25.80 | 9.31 | 0.7053 | |
LLT | 30 | 26.83 | 8.69 | 0.7258 | |
Barbara | Ours | 30 | 27.07 | 8.00 | 0.7520 |
YK | 20 | 27.13 | 7.98 | 0.7584 | |
LLT | 20 | 28.28 | 7.29 | 0.7794 | |
Ours | 20 | 28.46 | 6.79 | 0.7945 |