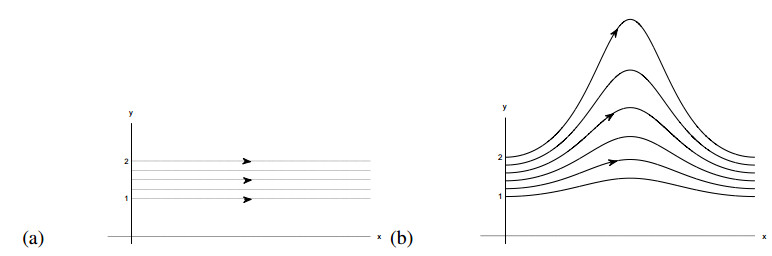
Citation: Guoqiao You. A novel quantity for identifying the repelling structures of continuous dynamical systems[J]. AIMS Mathematics, 2021, 6(4): 3378-3392. doi: 10.3934/math.2021202
[1] | Junsoo Lee, Wassim M. Haddad . On finite-time stability and stabilization of nonlinear hybrid dynamical systems. AIMS Mathematics, 2021, 6(6): 5535-5562. doi: 10.3934/math.2021328 |
[2] | Naseem Abbas, Amjad Hussain, Mohsen Bakouri, Thoraya N. Alharthi, Ilyas Khan . A study of dynamical features and novel soliton structures of complex-coupled Maccari's system. AIMS Mathematics, 2025, 10(2): 3025-3040. doi: 10.3934/math.2025141 |
[3] | Zhao Li, Shan Zhao . Bifurcation, chaotic behavior and solitary wave solutions for the Akbota equation. AIMS Mathematics, 2024, 9(8): 22590-22601. doi: 10.3934/math.20241100 |
[4] | Mingliang Zheng . Study on the symmetries and conserved quantities of flexible mechanical multibody dynamics. AIMS Mathematics, 2023, 8(11): 27969-27982. doi: 10.3934/math.20231430 |
[5] | Muhammad Bilal Riaz, Syeda Sarwat Kazmi, Adil Jhangeer, Jan Martinovic . Unveiling solitons and dynamic patterns for a (3+1)-dimensional model describing nonlinear wave motion. AIMS Mathematics, 2024, 9(8): 20390-20412. doi: 10.3934/math.2024992 |
[6] | Beibei Su, Liang Zhao, Liang Du, Qun Gu . Research on the ellipsoidal boundary of reachable sets of neutral systems with bounded disturbances and discrete time delays. AIMS Mathematics, 2024, 9(6): 16586-16604. doi: 10.3934/math.2024804 |
[7] | Minghung Lin, Yiyou Hou, Maryam A. Al-Towailb, Hassan Saberi-Nik . The global attractive sets and synchronization of a fractional-order complex dynamical system. AIMS Mathematics, 2023, 8(2): 3523-3541. doi: 10.3934/math.2023179 |
[8] | Andrew Calcan, Scott B. Lindstrom . The ADMM algorithm for audio signal recovery and performance modification with the dual Douglas-Rachford dynamical system. AIMS Mathematics, 2024, 9(6): 14640-14657. doi: 10.3934/math.2024712 |
[9] | Arunachalam Murali, Krishnan Muthunagai . Generation of Julia and Mandelbrot fractals for a generalized rational type mapping via viscosity approximation type iterative method extended with s-convexity. AIMS Mathematics, 2024, 9(8): 20221-20244. doi: 10.3934/math.2024985 |
[10] | Zhengqi Zhang, Huaiqin Wu . Cluster synchronization in finite/fixed time for semi-Markovian switching T-S fuzzy complex dynamical networks with discontinuous dynamic nodes. AIMS Mathematics, 2022, 7(7): 11942-11971. doi: 10.3934/math.2022666 |
In recent decades, people from various fields have been working on developing useful tools to analyze, visualize, and then extract important information in time-varying continuous dynamical systems, especially the fluid flows [1,2,4,5,11,16,17,19,20,21,23]. Mathematically, a dynamical system is modelled as an ordinary differential equation (ODE) given by
˙x(t)=u(x(t),t) | (1.1) |
with the initial condition x(t0)=x0 and a Lipschitz velocity field u:Rd×R→Rd. Among all, the Lagrangian coherent structure (LCS) is one of most useful and interesting approaches, which partitions the space-time domain into subregions based on certain quantity measured along with the passive tracer advected according to the associated dynamical system.
The most commonly used way to extract the LCS is based on the so-called finite time Lyapunov exponent (FTLE) [5,6,8,9,10,13,14,15,21]. It measures the rate of change in the distance between neighboring particles across a finite interval of time with an infinitesimal perturbation in the initial position. Define the flow map Φt0+Tt0:Rd→Rd as the mapping which takes the point x0 to the particle location at the final time t=t0+T, i.e Φt0+Tt0(x0)=x(t0+T) with x(t) satisfying (1.1). The FTLE can then be computed using the largest eigenvalue of the deformation matrix based on the Jacobian of this resulting flow map. For example, the FTLE σT0(x) from time t=0 to time t=T is computed as σT0(x)=1Tln√λmax(ΔT0(x)) where ΔT0(x)=(∇ΦT0(x))T(∇ΦT0(x)) is the deformation matrix and (⋅)T denotes the matrix transpose. Following the definition of Haller [5,7,8], one can see that the LCS is closely related to the ridges of the FTLE fields.
From the definition of the FTLE, however, one can see that the FTLE computed over a time interval, say [t0,t0+T], only concerns the particle positions at the initial time t=t0 and the terminal time t=t0+T. In other words, it does not care about where these particle trajectories travel at the intermediate times t∈(t0,t0+T). In this paper, we propose an alternative tool called the Lagrangian Averaged Repelling Rate (LARR), to measure the averaged repelling rate of each particular particle trajectory in the whole time interval. In particular, we trace the particle evolution and compute the trajectory integral of its maximum repelling rate against nearby trajectories within the whole time intervel [t0,t0+T]. It means that, the proposed LARR takes the repelling behavior of the trajectory at any intermediate time t∈(t0,t0+T) into account. A counterpart for measuring the attracting behaviors of particle trajectories can be similarly defined and discussed and we omit it in this paper.
Finally, we want to point out that compared to the traditional FTLE, the LARR actually measures the repelling properties of trajectories from a different perspective. People might make a choice depending on their need or the particular application. If they care about the ultimate growth rate of distances between particle trajectories at the single final time, then only the initial and final positions of these trajectories are concerned and the FTLE should be used. However, if they want to measure the averaged growth rate of distances between particle trajectories during the whole time interval, then LARR might be a better choice.
In this section, we first give two simple examples to illustrate that the FTLE values only depend on the initial and final locations of particle trajectories and then propose an alternative quantity called LARR. After that, the objectivity of the LARR is shown and an efficient Eulerian algorithm for computing the LARR is developed.
Before giving the definition of the LARR, we first consider two examples to show that the FTLE only focuses on the initial and final particle locations and thus might not be enough in measuring the averaged separation or repelling behaviors of particle trajectories. The first example is a constant velocity model given by u(x)=(1,0)T, where all particles travel with the constant speed 1 towards the positive x-axis as shown in Figure 1(a). It is easy to see that the deformation matrix is the second order identity matrix and hence the FTLE σ10(x) from t=0 to t=1 equals to zero at any point x in the phase space. Then we consider a little more complex dynamical system with the velocity field u(x)=(1,y2sin(2πt))T, from which we can obtain an analytical flow map ΦT0(x)=(x+T,πy/(π−ysin2(πT)))T. In particular, the flow map at T=1 is given by Φ10(x)=(x+1,y)T and the resulting FTLE is also zero at any point x. Figure 1(b) shows several trajectories within the time interval [0,1] of particles taking off from the points on the line segment with endpoints (0,1) and (0,2). In both subfigures, we can see that the distance between any two nearby trajectories at time t=1 does not change compared to their initial distance. As we said, the FTLE only cares about the particle states at the initial time t=0 and the terminal time t=1. As a result, it is not surprising that the FTLE σ10(x) constantly equals to zero at any point x in both examples. However, we can see from Figure 1(b) that those particle trajectories do not always keep a constant distance from each other during the evolution process in the intermediate time levels t∈[0,1]. On average, the upper trajectories have higher repelling rates than the lower ones.
Inspired by the second example in Section 2.1, we here propose a novel tool to measure the averaged repelling rate along the whole particle trajectory under consideration. In particular, we have to consider the repelling rate of the trajectory at any time level τ∈[t0,t0+T], not only the initial and the terminal time levels. To measure the repelling rate of the particle trajectory x(t) at a particular time level τ∈[t0,t0+T], we consider another particle trajectory x∗(t) which is infinitesimally close to x(t) at time τ, i.e. |ξ(τ)|≪1, where ξ(t)≜x∗(t)−x(t) denotes the difference between the two trajectories at time t. According to (1.1), we have
dξ(t)dt|t=τ=u(x(τ)+ξ(τ),τ)−u(x(τ),τ)=∇u(x(τ),τ)ξ(τ)+G(x(τ),ξ(τ)) |
where G is the high order nonlinear terms of ξ(τ). Ignoring the nonlinear terms, we will simply have ˙ξ(τ)=∇u(x(τ),τ)ξ(τ).
Define the separation rate between x(t) and x∗(t) at time τ as V(τ)≜12ddt|ξ(t)|2|t=τ, then we have V(τ)=⟨ξ(t),˙ξ(t)⟩|t=τ=⟨ξ(τ),∇u(x(τ),τ)ξ(τ)⟩=⟨ξ(τ),S(x(τ),τ)ξ(τ)⟩ where S(x,t) is the rate-of-strain tensor given by S=(∇u+∇uT)/2. If V<0, the two trajectories get closer over time while V>0 indicates their separation. As a result, the separation rate between x(t) and x∗(t) at t=τ is bounded by V(τ)≤λmax(S(x(τ),τ))|e(τ)|2, where e(τ) aligns with the eigenvector associated with the largest eigenvalue of the rate-of-strain tensor λmax(S(x(τ),τ)). In other words, the maximum repelling rate of the trajectory x(t) at time t=τ against any nearby trajectory is actually measured by the quantity λmax(S(x(τ),τ)). The LARR of the trajectory x(t) within the time interval [t0,t0+T] is then defined as the integral
LARRt0+Tt0(x0)=1T∫t0+Tt0λmax(S(x(t),t))dt | (2.1) |
where x0=x(t0) is the initial location of x(t) at time t=t0. We can see that the proposed LARR concerns the repelling behavior of the trajectory at any intermediate time.
Now we get back to the two examples given in Section 2.1 and consider the corresponding LARR values. For the first example, the rate-of-strain tensor S(x,t) is constantly equal to zero and therefore LARRt0+Tt0(x)=0 for any x in the spatial domain, which indicates that no particle trajectory repels the nearby trajectories. This satisfies our expectation since all particle trajectories have kept their distance from each other during the whole time interval [t0,t0+T]. In the second example, the trajectory of the particle initially located at x0=(x0,y0) can be analytically given by x(t)=(x0+t,πy0/(π−y0sin2(πt)))T. The LARR of the trajectories within the time interval [0,1] starting from the line segment (0,1)→(0,2) is given by LARR10(x0,y0)=2lnππ−y0 with 1≤y0≤2, which indicates that the upper the trajectory, the larger the LARR. This result is very reasonable as shown in Figure 1(b), since the upper trajectories repel the nearby trajectories more during the evolution process. This example suggests that the LARR is more valid and reasonable in measuring the averaged repelling behaviors of the whole particle trajectory.
Since LARR is used to measure the intrinsic repelling behaviors of particle trajectories, a basic requirement is that it is objective, i.e. remains invariant under coordinate changes of the form
x=Q(t)˜x+b(t), | (2.2) |
where Q(t) is a time-varying orthogonal matrix and b(t) is a time-varying translation vector. The following theorem guarantees this objectivity of the LARR.
Theorem 2.1. The LARR defined as in (2.1) is invariant under any coordinate change given by (2.2).
Proof. According to the definition of the flow map, we have x(t)=Φtt0(x0). Plugging (2.2) into this formula gives Q(t)˜x(t)+b(t)=Φtt0(Q(t0)˜x0+b(t0)), or equivalently,
˜Φtt0(˜x0)=QT(t)[Φtt0(Q(t0)˜x0+b(t0))−b(t)], |
where we use ˜⋅ to denote the operations in the transformed framework. Differentiating this equation with respect to ˜x0 gives ˜∇˜Φtt0(˜x0)=QT(t)∇Φtt0(x0)Q(t0). Differentiating this formula with respect to time t and recalling that ddt∇Φtt0(x)=∇u(Φtt0(x),t)∇Φtt0(x), we have
˜∇˜u(˜x(t),t)=˙QT(t)Q(t)+QT(t)∇u(x(t),t)Q(t). |
Adding the transpose of both sides yields
2˜S(˜x(t),t)=˙QT(t)Q(t)+QT(t)˙Q(t)+2QT(t)S(x(t),t)Q(t)=d(QT(t)Q(t))/dt+2QT(t)S(x(t),t)Q(t)=2QT(t)S(x(t),t)Q(t). |
That is, ˜S(˜x(t),t) is similar to S(x(t),t) and thus λmax(˜S(˜x(t),t))=λmax(S(x(t),t)).
Since the LARR is a quantity defined along each particular particle trajectory, a natural way to compute it is using the Lagrangian ray tracing approach. In particular, one numerically solves the integral (2.1) and the ODE system (1.1) simultaneously from the initial time t=t0 to the terminal time t=t0+T. In this paper, we propose an efficient Eulerian-type algorithm to compute the LARR where only one single partial differential equation (PDE) needs to be solved. For simplicity, suppose [0,T] is the time interval of interest and the value of LARRT0(x) is required at each mesh point x. According to (2.1), we have LARRT0(x)=1T∫T0λmax(S(Φt0(x),t))dt. Then we define a real-valued function F(x,t):Ω×[0,T]→R as
F(x,t)≜∫Ttλmax(S(Φst(x),s))ds. | (2.3) |
with Ω the computational domain. Indeed, F(x,t) denotes the line integral of the maximal repelling rate along the particle trajectory starting from the location x at time t and terminating at time T. It is obvious that F(x,T)=0 and LARRT0(x)=F(x,0)/T for any x∈Ω. Furthermore, F(x,t) decreases along any particle trajectory since
DF(x,t)Dt=−λmax(S(Φtt(x),t))=−λmax(S(x),t)) |
where D(⋅)Dt denotes the material derivative, or equivalently,
∂F(x,t)∂t+(u⋅∇)F(x,t)=−λmax(S(x),t)). | (2.4) |
Compared to the Lagrangian approach, the proposed Eulerian approach is more efficient. In particular, our Eulerian approach only needs to solve one single PDE (2.4) using any well-developed high order numerical methods like WENO5-TVDRK2 [3,18,22] backward in time from t=T to t=0 with the terminal condition F(x,T)=0. The required LARR is then obtained by assigning LARRT0(x)=F(x,0)/T. In contrast, the Lagrangian approach propose to solve the integral (2.1) and the ODE system (1.1) simultaneously from the initial time t=0 to the terminal time t=T. That is, d+1 equations are solved together in the Lagrangian approach where d is the dimension of the underlying velocty field. Our Eulerian approach is even more efficient if the velocity data is only available at discrete mesh points. All computations are implemented only on the mesh in our Eulerian approach. As a result, we only need discrete velocity data at mesh points and no interpolation is required. For the Lagrangian approach, however, since x(t) is in general not a mesh point, integration of ODEs (1.1) will require interpolation on the discrete velocity data at each time step. Furthermore, the computation of the integral (2.1) involves the computation of the velocity gradient along each particle trajectory. One has to first solve the velocity gradient at mesh points by using the finite difference scheme upon the discrete velocity data and then use the interpolation scheme to obtain the velocity gradient data at off-grid points. All these extra interpolation routines could be quite time-consuming. Even worse, as mentioned in [24], one needs to use high-order numerical algorithms to implement the integration and interpolation, otherwise some small scale flow features might not be accurately extracted.
We give four examples to support our claims. The first three are analytical examples while the last one is a real data example.
This example is taken from [21] to describe a periodically varying double-gyre. The flow is modeled by the stream-function ψ(x,y,t)=Asin[πk(x,t)]sin(πy), where k(x,t)=a(t)x2+b(t)x with a(t)=ϵsin(ωt) and b(t)=1−2ϵsin(ωt). Here we set the parameters A=0.1 and ω=2π/10.
In this part, we compute the LARR field LARR100(x) using our Eulerian algorithm by assigning ϵ=0.3 and using only the velocity data at mesh points. The solution is shown in Figure 2(a) where Δx=Δy=1/256. In the implementation, we only need to solve the single PDE (2.4) backward from t=10 to t=0 with the terminal condition F(x,10)=0. To show the convergence of our Eulerian algorithm, we have shown the L1 errors of LARR100(x) using the red solid line in Figure 2(b) with Δx varying from 1/32 to 1/512 while keeping Δt/Δx fixed. As a comparison, the errors of the solutions of LARR100(x) computed using the Lagrangian approach are also shown using a blue dashed line in the same figure where the TVD-RK4 scheme is used for the integration of corresponding ODEs and the third order cubic spline method is used as the interpolation operator for solving the velocity and velocity gradient at off-grid points. As can be seen, our Eulerian approach is a little more accurate than the Lagrangian approach and our proposed Eulerian approach shows second order accuracy with respect to Δx. For the Lagrangian approach, since we have used high order numerical schemes to implement the integration, interpolation and finite difference, the second order accuracy can also be observed. However, once lower order interpolation schemes are used, the Lagrangian approach will no longer show second order accuracy. Regarding the computational efficiency, our Eulerian approach will behave much better than the traditional Lagrangian approach. The comparison is given in Table 1 which shows that the Lagrangian approach requires twice to three times the computational time of our Eulerian approach.
Δx | 1/32 | 1/64 | 1/128 | 1/256 | 1/512 |
Eulerian approach | 0.9s | 3.2s | 20.6s | 179.8s | 1453.1s |
Lagrangian approach | 1.5s | 8.5s | 51.2s | 502.3s | 3985.2s |
Now we look further into Figure 2(a), from which we can observe sharp structures which correspond to the initial locations of the trajectories with high repelling rates. In particular, we have marked on this figure point A=(1.15625,0.34375) which has a high LARR value and point B=(1,0.5) which has a low LARR value. From the definition of LARR, the particle trajectories starting from point A and B at time t=0 and terminating at time t=10 must repel the nearby trajectories with a high and low rate, respectively. In Figure 3(a), we have shown the evolution of the distances between the trajectories starting from point A and four neighboring mesh points during the time period [0,10]. A counterpart for point B is shown in Figure 3(b). To have a clearer comparison, in Figure 3(c) we have used a red (blue) line to show the temporal evolution of the maximum distance between the trajectories starting from point A (point B) and four neighboring mesh points. All the results support our claim that the higher LARR value suggests the more repelling particle trajectory.
Then we turn ϵ to 0.1 and compare our LARR field with the traditional FTLE field in detail. Figure 4(a) and (b) show the LARR field and the FTLE field, respectively. We can observe that the macroscopic topological structures of these two fields are similar. However, there do exist some differences between them, especially at the ridge locations. The FTLE field seems to have a sharper ridge and the FTLE values on the ridge have little difference. In contrast, the LARR values on its ridge have obvious differences, which implies that trajectories starting from the points on the LARR ridge have different levels of repelling properties. To have a better explanation, we first choose two points A1=(1.0430,0.1680) and A2=(1.0820,0.9219) on the FTLE ridge which have been marked using small black circles as shown in Figure 5. The LARR values at A1 and A2 are 0.8248 and 0.7127, respectively, i.e. LARR100(A1)=0.8248 and LARR100(A2)=0.7127. And the FTLE values are given by σ100(A1)=0.4988 and σ100(A2)=0.5604, respectively. Interestingly we have σ100(A1)<σ100(A2) but LARR100(A1)>LARR100(A2). As introduced in Section 1, the proposed LARR and the traditional FTLE measure the repelling properties of trajectories from different perspectives. The FTLE only concerns the particle positions at the initial time t=0 and the final time t=10 and does not care about where these particle trajectories have traveled at the intermediate times t∈(0,10). The proposed LARR, however, measures the averaged repelling rate of each particular particle trajectory against nearby trajectories in the whole time interval t∈[0,10]. It means that, the LARR traces the repelling behavior of the trajectory at any time t∈[0,10]. More intuitively, in Figure 6(a) we have shown the temporal evolution of the maximum distance between the trajectories starting from point Ai (i=1,2) and four neighboring mesh points during the time period [0,10]. We can see that the red line is beyond the blue line until about t=9.2 and then the blue line goes beyond the red line. This means that, in most of the time during t∈[0,10] or averagely, the trajectory starting from A1 is more repelling than that from A2. As a result, we have LARR100(A1)>LARR100(A2). In contrast, the FTLE does not care about the intermediate process and only focuses on the initial and final states. At the final time t=10, the blue line is beyond the red line. Therefore, it gives σ100(A1)<σ100(A2), although the blue line is under the red line in most of the time.
We have also chosen another two points B1=(0.6563,0.9492) and B2=(1.0586,0.4766) on the ridge as marked using small black stars in Figure 5. The corresponding LARR values are LARR100(B1)=0.6617, LARR100(B2)=0.7664 and the FTLE values at the two points are almost the same given by σ100(B1)=0.5430, σ100(B2)=0.5441. Figure 6(b) gives the temporal evolution of the maximum distance between the trajectories starting from point Bi (i=1,2) and four neighboring mesh points within t∈[0,10]. We can see that the blue line is always beyond the red line but finally they are very close at t=10. This means that, the trajectory from B2 is more repelling than that from B1 during the whole time interval t∈[0,10] and surely LARR100(B2)>LARR100(B1). However, since they are very close at the final time t=10, we have σ100(B1)≈σ100(B2), although the blue line is always beyond the red line.
At the end of this example, we extend the use of the proposed LARR to identify the most attracting trajectories. Recalling that backward FTLE measures the level of compression [8,14], we here take a similar idea and propose to compute the backward LARR from the current time t=t0 back to an earlier time t=t0−T. Figure 7(a) shows the backward LARR field LARR020(x) from the current time t=20 back to t=0. The higher the LARR value, the more attracting the underlying particle trajectory. To show this, we have particularly marked two points C1=(0.9492,0.7109) and C2=(1.4727,0.4297) as shown in Figure 7(b) where the backward LARR values at C1 and C2 are high and low, respectively. In particular, we have LARR020(C1)=0.7899 and LARR020(C2)=0.0652. As a result, the particle trajectory reaching C1 at t=20 should be very attracting while the trajectory getting to C2 at t=20 can hardly exhibit attraction. This claim is verified in Figure 8 which shows some neighbouring trajectories near C1 and C2, respectively. In Figure 8(a) all the nearby trajectories get closer and closer to the trajectory reaching C1. In contrast, the trajectory arriving at C2 has not obviously attracted the nearby trajectories.
As the second example, we consider a model of Rayleigh-Bˊenard convection cells as introduced in [12]. The stream-function of this model is given by
ψ(x,y,t)=sin[π(x−g(t))]sin(πy). |
Following [12], we here use a quasi-periodic roll motion g(t)=0.3sin(4πt)+0.1sin(2t). The LARR10(x) field computed using our proposed algorithm is shown in Figure 9(a), with the mesh size Δx=Δy=1/256. As a comparison, we have also plotted the FTLE field σ10(x) in Figure 9(b). The two fields do exhibit some similarity to certain extent. However, like the double gyre flow in Section 3.1.2, we can observe some differences between them. For example, the FTLE values are very close at the two points A=(1.5117,0.1523) and B=(1.1836,0.5469) located on the FTLE ridge, which have been marked using small black circles in Figure 9(a), (b). In particular, we have σ10(A)=5.2240 and σ10(B)=5.2536. In contrast, the LARR values at the two points are LARR10(A)=5.8381<LARR10(B)=7.3802. In Figure 9(c) we have shown the temporal evolution of the maximum distance between the trajectories starting from point A and four neighboring mesh points within t∈[0,1], using the red line. A counterpart for point B is plotted using the blue line. We can see that the red line is always under the blue line and even far from the blue line during a subinterval of [0,1]. This indicates that averagely the particle trajectory starting from B is more repelling than that from A in the whole time period t∈[0,1]. There is no doubt that LARR10(B) should be greater than LARR10(A) since the LARR measures the averaged repelling rate of the particle trajectory against nearby trajectories in the whole time interval. However, as we can see from Figure 9(c), the red line almost catches the blue line at the final time t=1. As a result, the FTLE values at the two points are very close since the the FTLE only focuses on the final states of particle trajectories.
Then we take the spiral focus ridge for example to test our proposed Eulerian algorithm for computing the LARR and also the effectiveness of the LARR in revealing the repelling properties of particle trajectories. The example starts with a trivial field v(x,t)=(0,x)T and its flow map is given by (Φv)τ0(x)=(x,y+τx)T. Let
α(x,t)=(xcosγ−ysinγxsinγ+ycosγ) and β(x,t)=(xcos(−γ)−ysin(−γ)xsin(−γ)+ycos(−γ)) |
with γ=p0/(1+|x2+y2|). Then the velocity field w of the spiral focus ridge flow is constructed as
w(x,t)=(∇β)−1(x,t)⋅(v(β(x,t),t)−∂β∂t(x,t)). |
We set the parameter p0=12 and the computational domain as [−1,1]×[−1,1]. The LARR field LARR30(x) computed using the proposed algorithm is shown in Figure 10(a) with the mesh size Δx=Δy=1/128. We can see that the fine structures can be captured. Then we mark two points A=(0.5,−0.6953) and B=(0.3594,−0.6719) on Figure 10(a) with a high and low LARR value, respectively. The maximum distance between the trajectories starting from A and four neighboring mesh points is plotted using the red line in Figure 10(b). A counterpart for B is also shown using a blue line. As can be seen, the blue line is beyond the red line at the beginning. However, as time goes the red line rises and goes far beyond the blue line. As a result, the trajectory starting from point A is averagely more repelling than that from point B, which again verifies the effectiveness of the LARR.
To test the effectiveness of the LARR in measuring the repelling behaviors of particle trajectories and also the robustness of our proposed Eulerian algorithm, finally we consider a more challenging case, which is the Ocean Surface Current Analyses Real-time (OSCAR) data. In [25], we have used this dataset to compute the FTLE field and the ISLE field. Here we compute the LARR field of this dataset. As in [25], we also choose an ocean region near the Line Islands as our computational region, which is enclosed by S17∘ (17 degrees South) to N8∘ (8 degrees North) latitude and E180∘ (180 degrees East) to E230∘ longitude. We have re-processed the dataset to have a finer resolution of 0.25 days in the temporal direction and 1/12∘ in each spatial direction. The velocity data of the ocean surface current within the first 50 days in year 2015 is used and then the corresponding LARR field LARR500(x) is computed using our Eulerian algorithm. Figure 11(a) shows the solution of LARR500(x) from which we can observe fine structures with high LARR values. Again we have marked two points A=(E215.25∘,S1.25∘) and B=(E217.5∘,N2.25∘) on this figure which have a high LARR value and a low LARR value, respectively. From the definition of LARR, the particle trajectories starting from point A and B at time t=0 and terminating at time t=50 must repel the nearby trajectories with a high and low rate, respectively. In Figure 11(b) we have used a red (blue) line to show the temporal evolution of the maximum distance between the trajectories starting from point A (point B) and four neighboring mesh points, which also indicates that the higher LARR value does suggest the more repelling particle trajectory.
In this study, we have proposed a novel quantity called LARR to measure the averaged repelling rate along each particle trajectory against nearby trajectories. The LARR equally treats the repelling behavior of the trajectory during the whole time period, unlike the traditional FTLE which only concerns the initial and the terminal states of particle trajectories. The LARR is shown to be framework-independent and we have also proposed a simple yet efficient Eulerian algorithm to compute it. All the analytical and real data examples have shown the effectiveness of the proposed LARR in measuring the repelling behaviors of particle trajectories and the accuracy and efficiency of our proposed Eulerian algorithm. We believe that the LARR should be closely related to the LCS or even an alternative to LCS might be developed based on the LARR, which will be further subjects of future research.
We declare that we do not have any commercial or associative interest that represents a conflict of interest in connection with this work.
[1] |
E. J. Candès, L. Ying, Fast geodesics computation with the phase flow method, J. Comput. Phys., 220 (2006), 6–18. doi: 10.1016/j.jcp.2006.07.032
![]() |
[2] |
R. Ding, J. Li, Nonlinear finite-time Lyapunov exponent and predictability, Physics Letters A, 364 (2007), 396–400. doi: 10.1016/j.physleta.2006.11.094
![]() |
[3] |
S. Gottlieb, C. W. Shu, Total variation diminishing Runge-Kutta schemes, Math. Comput., 67 (1998), 73–85. doi: 10.1090/S0025-5718-98-00913-2
![]() |
[4] |
M. A. Green, C. W. Rowley, A. J. Smiths, Using hyperbolic Lagrangian coherent structures to investigate vortices in biospired fluid flows, Chaos, 20 (2010), 017510. doi: 10.1063/1.3270045
![]() |
[5] |
G. Haller, Distinguished material surfaces and coherent structures in Three-Dimensional fluid flows, Physica D, 149 (2001), 248–277. doi: 10.1016/S0167-2789(00)00199-8
![]() |
[6] | G. Haller, Lagrangian structures and the rate of Strain in a partition of Two-Dimensional turbulence, Phys. Fluids A, 13 (2001), 3368–3385. |
[7] |
G. Haller, Lagrangian coherent structures from approximate velocity data, Physics Fluid, 14 (2002), 1851–1861. doi: 10.1063/1.1477449
![]() |
[8] |
G. Haller, A variational theory of hyperbolic Lagrangian coherent structure, Physica D, 240 (2011), 574–598. doi: 10.1016/j.physd.2010.11.010
![]() |
[9] |
G. Haller, G. Yuan, Lagrangian coherent structures and mixing in Two-Dimensional turbulence, Physica D, 147 (2000), 352–370. doi: 10.1016/S0167-2789(00)00142-1
![]() |
[10] |
D. Karrasch, G. Haller, Do finite-size Lynapunov exponents detect coherent structures? Chaos, 23 (2013), 043126. doi: 10.1063/1.4837075
![]() |
[11] | F. Lekien, N. Leonard, Dynamically consistent Lagrangian coherent structures, Experimental Chaos: 8-th Experimental Chaos Conference, 2004,132–139. |
[12] |
F. Lekien, S. D. Ross, The computation of finite-time Lyapunov exponents on unstructured meshes and for non-Euclidean manifolds, Chaos, 20 (2010), 017505. doi: 10.1063/1.3278516
![]() |
[13] |
F. Lekien, S. C. Shadden, J. E. Marsden, Lagrangian coherent structures in n-dimensional systems, J. Math. Phys., 48 (2007), 065404. doi: 10.1063/1.2740025
![]() |
[14] |
S. Leung, An Eulerian approach for computing the finite time Lyapunov exponent, J. Comput. Phys., 230 (2011), 3500–3524. doi: 10.1016/j.jcp.2011.01.046
![]() |
[15] |
S. Leung, A backward phase flow method for the finite time Lyapunov exponent, Chaos, 23 (2013), 043132. doi: 10.1063/1.4847175
![]() |
[16] |
S. Leung, J. Qian, R. Burridge, Eulerian Gaussian Beams for high frequency wave propagation, Geophysics, 72 (2007), SM61–SM76. doi: 10.1190/1.2752136
![]() |
[17] |
D. Lipinski, K. Mohseni, Flow structures and fluid transport for the hydromedusae Sarsia tubulosa and Aequorea victoria, J. Exp. Biology, 212 (2009), 2436–2447. doi: 10.1242/jeb.026740
![]() |
[18] |
X. D. Liu, S. J. Osher, T. Chan, Weighted essentially NonOscillatory schemes, J. Comput. Phys., 115 (1994), 200–212. doi: 10.1006/jcph.1994.1187
![]() |
[19] |
S. Lukens, X. Yang, L. Fauci, Using Lagrangian coherent structures to analyze fluid mixing by cillia, Chaos, 20 (2010), 017511. doi: 10.1063/1.3271340
![]() |
[20] |
T. Sapsis, G. Haller, Inertial particle dynamics in a hurricane, J. Atmos. Sci., 66 (2009), 2481–2492. doi: 10.1175/2009JAS2865.1
![]() |
[21] |
S. C. Shadden, F. Lekien, J. E. Marsden, Definition and properties of Lagrangian coherent structures from finite-time Lyapunov exponents in two-dimensional aperiodic flows, Physica D, 212 (2005), 271–304. doi: 10.1016/j.physd.2005.10.007
![]() |
[22] | C. W. Shu, Essentially Non-Oscillatory and weighted essentially Non-Oscillatory schemes for hyperbolic conservation laws, NASA Langley Research Center, 1997. |
[23] |
W. Tang, T. Peacock, Lagrangian coherent structures and internal wave attractors, Chaos, 20 (2010), 017508. doi: 10.1063/1.3273054
![]() |
[24] |
F. Wang, D. Zhao, L. Deng, S. Li, An accurate vortex feature extraction method for Lagrangian vortex visualization on high-order flow field data, J. Visualization, 20 (2017), 729–742. doi: 10.1007/s12650-017-0421-y
![]() |
[25] |
G. You, T. Wong, S. Leung, Eulerian methods for visualizing continuous dynamical systems using Lyapunov exponents, SIAM J. Sci. Comput., 39 (2017), A415–A437. doi: 10.1137/16M1066890
![]() |
Δx | 1/32 | 1/64 | 1/128 | 1/256 | 1/512 |
Eulerian approach | 0.9s | 3.2s | 20.6s | 179.8s | 1453.1s |
Lagrangian approach | 1.5s | 8.5s | 51.2s | 502.3s | 3985.2s |