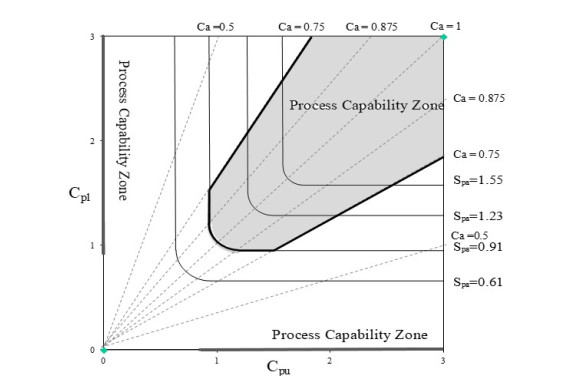
Citation: Chun-Min Yu, Kun-Tzu Yu, Kuen-Suan Chen. Entire product capability analysis chart with asymmetric tolerances index Spa[J]. Mathematical Biosciences and Engineering, 2020, 17(6): 7605-7620. doi: 10.3934/mbe.2020387
[1] | Jie Wang, Jian Wang, Jiafeng Cao . A heterogeneous parasitic-mutualistic model of mistletoes and birds on a periodically evolving domain. Mathematical Biosciences and Engineering, 2020, 17(6): 6678-6698. doi: 10.3934/mbe.2020347 |
[2] | Micaela Morettini, Christian Göbl, Alexandra Kautzky-Willer, Giovanni Pacini, Andrea Tura, Laura Burattini . Former gestational diabetes: Mathematical modeling of intravenous glucose tolerance test for the assessment of insulin clearance and its determinants. Mathematical Biosciences and Engineering, 2020, 17(2): 1604-1615. doi: 10.3934/mbe.2020084 |
[3] | Joseph Malinzi, Rachid Ouifki, Amina Eladdadi, Delfim F. M. Torres, K. A. Jane White . Enhancement of chemotherapy using oncolytic virotherapy: Mathematical and optimal control analysis. Mathematical Biosciences and Engineering, 2018, 15(6): 1435-1463. doi: 10.3934/mbe.2018066 |
[4] | Haolei Gu, Kedong Yin . Forecasting algae and shellfish carbon sink capability on fractional order accumulation grey model. Mathematical Biosciences and Engineering, 2022, 19(6): 5409-5427. doi: 10.3934/mbe.2022254 |
[5] | Wei Wang, Xiulan Lai . Global stability analysis of a viral infection model in a critical case. Mathematical Biosciences and Engineering, 2020, 17(2): 1442-1449. doi: 10.3934/mbe.2020074 |
[6] | Yijun Lou, Li Liu, Daozhou Gao . Modeling co-infection of Ixodes tick-borne pathogens. Mathematical Biosciences and Engineering, 2017, 14(5&6): 1301-1316. doi: 10.3934/mbe.2017067 |
[7] | Debao Guan, Yuqian Mei, Lijian Xu, Li Cai, Xiaoyu Luo, Hao Gao . Effects of dispersed fibres in myocardial mechanics, Part I: passive response. Mathematical Biosciences and Engineering, 2022, 19(4): 3972-3993. doi: 10.3934/mbe.2022183 |
[8] | Guozheng Yang, Lintao Liu, Xuehu Yan . A compressed secret image sharing method with shadow image verification capability. Mathematical Biosciences and Engineering, 2020, 17(4): 4295-4316. doi: 10.3934/mbe.2020237 |
[9] | Shuixian Yan, Sanling Yuan . Critical value in a SIR network model with heterogeneous infectiousness and susceptibility. Mathematical Biosciences and Engineering, 2020, 17(5): 5802-5811. doi: 10.3934/mbe.2020310 |
[10] | Chunyang Qin, Yuming Chen, Xia Wang . Global dynamics of a delayed diffusive virus infection model with cell-mediated immunity and cell-to-cell transmission. Mathematical Biosciences and Engineering, 2020, 17(5): 4678-4705. doi: 10.3934/mbe.2020257 |
The process capability analysis in the manufacturing process plays a critical role in the quality improvement program. When a process is statistically controlled, its capability can be evaluated to manufacture products based on specifications. By means of the process capability analysis, manufacturing managers are able to perceive process capability levels. Both process capability indices (PCIs) and process capability analysis charts frequently appear in the process capability analysis [1,2,3,4,5,6]. In the multi-process environment, many experts and scholars integrate different types of PCIs to accurately and reliably develop various analysis charts which can monitor and control process capabilities.
Singhal [7] introduced a graphical chart with the first-generation process capability index (yield-based index), the Smaller-the-Better (STB) capability index, and the Larger-the-Better (LTB) capability index. The yield-based index is the minimal value of the horizontal and vertical axes, which refines the previous chart to include capability zones, instead of the expected fallout contours. The processes can fall into different performance categories (or capability zones) which easily explain the state of each process and help factory managers improve the quality of each process. Nevertheless, the yield-based index has difficulty telling the difference between the off-target process and the on-target process. Pearn and Chen [9] introduced a modified PCAC combining the process capability indices of the 2nd and 3rd generations, attempting to point out the factors that result in process failure at centering the target. Not only the second-generation but also the third-generation process capability indices were selected because of their sensitivity to process centering. Furthermore, Chen et al. [10] noted that another important problem is how to adopt asymmetric tolerances to accurately evaluate the quality of bilateral quality characteristics. According to Chen et al. [11], a Process Capability Analysis Chart (PCAC) was employed to precisely assess the potential capability and performance of an entire product possessing STB, LTB, and Nominal-the-Best (NTB) characteristics using asymmetric tolerances. Reasonably, the index of asymmetric tolerances indicates the condition of a process when the asymmetric tolerances make the process deviate from the target.
Process yield is another criterion used in manufacturing to analyze process performance. The yield-based index has difficulty mirroring a specific process yield. Huang et al. [12] proposed yield index to take over the yield-based index. The yield index of the process offers a gauge of the process yield by reason of their one-to-one relations. Further, they also proposed an entire PCAC using the process yield index to precisely demonstrate the process performance of the backlight module. Shortly thereafter, Chen et al. [13] constructed a contour plot called the control chart of the process yield index with the multi-characteristic process capability analysis (MCPCA). The process yield index is computed as a function of the accuracy index and the precision index. The accuracy index refers to the departure rate while the precision index estimates the deviation related to the tolerances. MCPCA yields timely information so that the management is able to easily discern a product's quality level and has a clear picture about which parameters need to be targeted for quality improvement.
However, there exists a crucial problem that needs to be solved. That is, most approaches are untrustworthy because the point estimate of PCI sampling is a random variable without assessing any sampling errors. Several researchers have addressed this. Pearn et al. [14] developed the third-generation index PCAC. In this model, multiple samples of the control chart urge the distributions of the estimated third-generation index and accuracy index. Precise lower confidence bounds are tallied and plotted. Pearn and Wu [15] mentioned that several PCACs have so overlooked sampling errors that capability measures and groupings seem undependable. Therefore, they proposed a decent method to transform estimated indices into lower confidence bounds as well as plot the corresponding lower confidence bounds on PCAC. Chen and Chen [16] advanced a PCAC taking the interval estimates of these indices into account to accurately assess a product's characteristics. Chen et al. [17] came up with the process capability analysis chart. Besides, they proposed a new estimator of the second-generation index and discussed the features of the statistical estimation and hypothesis testing, in an effort to reliably evaluate the process capability. Chang et al. [18] not only considered the sample errors but also employed the mathematical programming, so that a statistical hypothesis testing model was developed to help manufacturing sections examine the outcome of their implemented improvements. Recently, Chen and Chen [19] developed an advanced PCAC by means of asymmetric tolerances, in order to appraise the process performance of T-Bar Ceiling Suspension System. Moreover, this method, considering the interval estimates of the PCIs, attempted to surmount the weakness of scrutiny or sampling errors of point estimates.
Reviewing the development described in aforementioned studies, each article proposed a refined tool or method which contributed valuable information that managers can use to implement quality improvement. Nonetheless, owing to rising requirements for products' quality, a single instrument cannot provide managers complete and reliable information. This is because several critical issues are difficult to be simultaneously taken into account in a model. These issues include capability evaluation of asymmetric process, process yield, process accuracy and loss, and the reliability of estimation. To provide comprehensive and reliable information managers can use, this study retains the merits of the past approaches and constructs a useful and dynamic approach via a Spa product capability analysis chart (Spa -PCAC). This model has four key points. First, the Spa -PCAC can widely represent multiple process capabilities with asymmetric tolerances consisting of STB, LTB, and NTB characteristics. A new index Spa is generated based on the index of process yield, which can provide a consistent and reasonable measure for processes with asymmetric tolerances. Second, considering sampling errors, this study derives interval estimations of the used indices to reliably assess the process capabilities. Third, based on the loss function defined in the Taguchi method, the index, Ca, is incorporated into the Spa -PCAC because it is adequate to assess the validity of the manufacturing process and process loss. Fourth, the quality-level of Six-Sigma and the connection with process yield are introduced in the process capability zone of Spa -PCAC to check if the process capabilities can reach the preset levels. Managers are able to adopt Spa -PCAC, a practical and easy-to-use tool, attempting to evaluate and enhance manufacturing processes constantly.
This study is divided into the following sections. In section 2, we not only begin with the definition of index Spa but also introduce how to construct the model of Spa -PCAC based on the required quality level. Next, in section 3, we derive the confidence interval of Spa from the mathematical programming. In section 4, we adopt a multi-process product containing seven quality characteristics as an example to illustrate Spa -PCAC. Lastly, in section 5, we summarize our results and provide the managers with reliable information in order to implement quality improvements.
Kane [20] proposed two process capability indices, Cpu and Cpl, to evaluate process capabilities of STB and LTB characteristics.
Cpu=USL−μ3σ,Cpl=μ−LSL3σ, | (1) |
In Eq (1), USL is the upper lower specification limit while LSL is the lower specification limit; μ is the process mean, and σ is the process standard deviation. In general conditions, one-to-one mathematical relationships prevail over the process yield and Cpu or Cpl, which is expressed as % Yield=Φ(3Cpi) for i=u,l.
Based on Chen et al. [11], in the process of NTB, index Ca is associated with the relative distance between the mean and the target. Ca is expressed as
Ca=1−max{μ−TDu,T−μDl}, | (2) |
where Du=USL−T, Dl=T−LSL and T is the target. Obviously, equally relative distances create the identical values for Ca. Index Ca can demonstrate process accuracy as well as process loss. In addition, Boyles [21] proposed index Spk, which accurately measures the process yield for the normal process with bilateral specifications, as expressed in the following equation:
Spk=13Φ−1{12Φ(USL−μσ)+12Φ(μ−LSLσ)}, | (3) |
where Φ is an accumulative density function of the standard normal distribution, N (0, 1), while Φ−1 is a reversed function of Φ. Actually,
USL−μσ=T+d−μσ=d−(μ−T)σ=dσ(1−μ−Td),μ−LSLσ=μ−(T−d)σ=d+(μ−T)σ=dσ(1+μ−Td), | (4) |
In Eq (4), d=(USL−LSL)/2 is the distance to the upper and lower limits, indicating half the length of the specification interval. Thus, Spk is rewritten as
Spk=13Φ−1{12Φ[dσ(1−μ−Td)]+12Φ[dσ(1+μ−Td)]}. | (5) |
Huang et al. [12] suggested a multi-process capability analysis chart (MPCAC) containing indices Cpu, Cpl, Ca, and Cps (Spk) as well as evaluated the integrated process capability of a multi-process product (backlight module) adopting STB, LTB, and NTB symmetric specifications respectively. On the contrary, as stated by Pearn and Shu [22], index Spk cannot provide consistent and reasonable measures for processes by means of asymmetric tolerances. This study proposes a generalization index, Spa, based on Spk, which can apply asymmetric tolerances to processes. The index, Spa, is expressed as follows:
Spa=13Φ−1{12Φ[(1−δ)θ]+12Φ[(1+δ)θ]}. | (6) |
Let θ=σdA,δ={μ−TDu,μ⩾Tμ−TDl,μ<T,when {μ=USL⇒δ=1μ=LSL⇒δ=−1LSL<μ<USL⇒−1<δ<1, | (7) |
where dA=min(Du,Dl). Therefore, when Dμ=Dl=d (symmetric case), Spa will decrease to the original index, Spk. For a fixed process standard deviation - σ, Spa drops more quickly as μ deviates from T and gets closer to the specification limit. On the other hand, it reduces slower when μ deviates from T and gets farther to the specification limit. The newly generalized Spa is supposed to acquire its maximum at μ=T (on-target process) no matter whether the specifications are symmetric or asymmetric. It can be seen that the newly generalized Spa is more suitable than the existing Spk to be adopted in an asymmetric case, providing greater accuracy to monitor process potential and performance.
According to the MPCAC proposed by Huang et al. [12], this study selects index Spa to replace Spk as well as reconstructs a Spa product capability analysis chart (Spa -PCAC) to assess a manufacturing process, the secondary lines standing for the values of index Ca are outlined on the Spa -PCAC, in order to precisely control the process shifts and monitor the process loss. Third, the process capability zone is mapped with bold lines on Spa -PCAC following the required quality level of the manufacturing process. Fourth, the interval estimates of Cpu, Cpl, and Spa are drawn on Spa -PCAC. The process capabilities reach the required level when the estimates of the confidence intervals of the PCIs are situated in the capability zone of the process. It is simple to discriminate process performance from the sites plotted on the Spa -PCAC, as shown in Figure 1. Hence, the PCIs and Spa -PCAC not only strengthen the limitations of previous studies but also reliably evaluate and effectively monitor process capabilities.
Linderman et al. [23] and Chen et al. [24] suggested that Six-Sigma is considered as a decent technique of improvement and is employed by plenty of enterprises. The number of Sigma has been regarded as a tool used by some enterprises to measure process capability. Motorola set the standard that process variability should be above or below the mean 6σ. Furthermore, Motorola stated that the process devoured by disruptions would deviate the mean from the target as much as 1.5σ [25]. As a result, based on Eq (7), when the quality level reaches k-Sigma (kσ) (i.e., dA=kσ) and the process mean shifts at 1.5σ, then θ=1/1kk and |δ|=1.5/1.5kk. The PCIs, Cpu, Cpl, and Spa, are expressed as follows:
Cpu=USL−μ3σ=T+d−μ3σ=kσ−1.5σ3σ=k−1.53, |
Cpl=μ−LSL3σ=μ−(T−d)3σ=kσ−1.5σ3σ=k−1.53, |
Spa=13Φ−1{12Φ[(1−δ)θ]+12Φ[(1+δ)θ]}=13Φ−1{12Φ[(1−1.5/1.5kk)1/1kk]+12Φ[(1+1.5/1.5kk)1/1kk]} | (8) |
=13Φ−1{12Φ(k−1.5)+12Φ(k+1.5)}. |
This paper defines that the process quality reaches k-Sigma quality level, then Cpu ⩾ Cpu (k), Cpl ⩾ Cpl (k) and Spa ⩾ Spa (k). In other words, PCI (k) represents the lower limit value when the process quality reaches k-Sigma quality level. Table 1 displays the values of Cpu (k), Cpl (k), and Spa (k) for k = 3, 4, 5, 6.
Quality Level (k-Sigma) |
Spa (k) | Cpi (k) i = u, l |
Process Yield (%) |
3-Sigma | 0.61 | 0.50 | 93.319 |
4-Sigma | 0.91 | 0.83 | 99.361 |
5-Sigma | 1.23 | 1.17 | 99.977 |
6-Sigma | 1.55 | 1.50 | 99.999 |
Additionally, based on Eq (2), let Ca=1−1a, where 1/1aa=max{(μ−T)/(μ−T)DuDu,(T−μ)/(T−μ)DlDl}. According to Chen et al. [11], the values of μ for each Ca. are [T + (1/a)*Du] and [T − (1/a)*Dl]. The incline of the relevant secondary line is m1=(a+1)/=(a+1)(a−1)(a−1) as the mean is larger than the target (μ⩾T); the slope of the relevant secondary line is m2=(a−1)/(a−1)(a+1)(a+1) as the mean is smaller than the target (μ<T). Owing to m1×m2=1, secondary lines Y=m1X and Y=m2X are symmetrical with line Y=X, as shown in Figure 1. As we can see, Ca refers to process accuracy. In general, Ca cannot be too tiny because a smaller Ca represents that the mean deviates from the target, and that will incur a great amount of process loss. For NTB quality type, when the process loss is taken into consideration (e.g., Ca⩾1−1/1aa, and the quality level is required to reach k-Sigma, as shown in Table 1), we can define a set Sk and mark a capability zone with bold lines on Spa -PCAC as follows:
Nominal−the−Best: Sk={(X,Y)|Spa(X,Y)⩾Spa(k),a−1a+1⩽Y/X⩽a+1a−1,X⩾0,Y⩾0} | (9) |
Similarly, for the unilateral process, if the quality level reaches k-Sigma, the capability zones can be respectively plotted on the horizontal and vertical axes with bold lines according to the values of Cpu and Cpl, as depicted in Table 1. The capability zone of STB and LTB quality type can be show as following sets:
Smaller−the−Better: Su={(X,Y)|X⩾Cpu(k),Y=0} | (10) |
Larger−the−Better: Sl={(X,Y)|X=0,Y⩾Cpl(k)} | (11) |
As a result, the capability zones on Spa -PCAC (the gray zones in Figure 1) can help managers check whether all process capabilities can satisfy the required quality levels. Then, the process capability can be evaluated based on the intersection of the process capability interval estimation area of each quality characteristic and the capability zone. The evaluation rules are as follows:
(1) When the intersection of the interval estimation area of the index and the gray area in Figure 1 is a nonempty set, it means that the process capability of the quality characteristic has reached the quality level and the process capability needs to be maintained.
(2) When the intersection of the interval estimation area of the index and the gray area in Figure 1 is a nonempty set and the white zone is included, it means that the process capability of the quality characteristic cannot meet the quality requirements and needs to be improved.
According to the above evaluation rules, the industries can easily determine whether the process capability of all the quality characteristics of the whole product meets the quality level requirements.
As mentioned in the discussion of the literature section of this paper, some limitations of the existing approaches include (1) they are unreliable since sampling errors are neglected and (2) the approaches are only applicable in case of full inspection. Point estimate is prone to resulting in uncertain sampling errors, so that it may lead to the probability of misjudgment [26,27,28,29,30,31]. To overcome these limitations, the proposed Spa -PCAC takes into account the interval estimates of Spa, Cpu, and Cpl, which reliably measure a product with the NTB asymmetric tolerances, LTB characteristics, and STB characteristics. This can be explained as follows. The index Spa is viewed as a function of process parameters μ and σ denoted by Spa(μ,σ). We derive the confidence interval of Spa(μ,σ) via the following mathematical programming (MP):
{MaxSpa(μ,σ)s.t.a1⩽μ⩽a2b1⩽σ⩽b2for USpa,{MinSpa(μ,σ)s.t.a1⩽μ⩽a2b1⩽σ⩽b2for LSpa. | (12) |
The upper limit of confidence interval (denoted by USpa) and lower limit of confidence interval (denoted by LSpa) are computed separately in accordance with the maximum and minimum values of the objective function Spa(μ,σ) under the constraints a1⩽μ⩽a2 and b1⩽σ⩽b2. (a1,a2) and (b1,b2) are the confidence intervals of μ and σ, respectively. These two constraints compose the feasible solution area (FSA) of the MP model, that is, FSA(μ,σ)={a1⩽μ⩽a2,b1⩽σ⩽b2}. It is obvious to see that the maximum value of Spa(μ,σ) must be at the minimum value b1 of σ while the minimum value of Spa(μ,σ) must be at the maximum value b2. Spa(μ,σ) is re-expressed as follows based on the Eqs (6) and (7). Let Spa(μ,σ)=f(μ,σ) when μ⩾T, and Spa(μ,σ)=g(μ,σ) when μ<T.
Spa(μ,σ)={f(μ,σ)=13Φ−1{12Φ[dAσ(1−μ−TDu)]+12Φ[dAσ(1+μ−TDu)]},μ⩾Tg(μ,σ)=13Φ−1{12Φ[dAσ(1−μ−TDl)]+12Φ[dAσ(1+μ−TDl)]},μ<T. | (13) |
We can draw the figure of Spa(μ,σ) under the fixed σ, as shown in Figure 2.
In Figure 2, we can see the optimal solution of Spa(μ,σ) is dependent on the relative position between the interval (a1,a2) and the process target T. In order to clearly explain the relevance of the position between interval (a1,a2) and the process target T, this study classifies three cases as follows.
Case I: a1⩽T⩽a2 is composed of two situations:
(1) When μ is located in (T,a2) (i.e. T⩽μ⩽a2), we know Spa(μ,σ)=f(μ,σ). Therefore, the upper limit of confidence USpa=max{f(μ,b1)|T⩽μ⩽a2}. Since f(μ,b1) strictly decreases as μ increases, we can find USpa=f(T,b1). Similarly, we have LSpa=min{f(μ,b2)|T⩽μ⩽a2}=f(a2,b2).
(2) When μ is located in (a1,T) (i.e. a1⩽μ⩽T), we see that Spa(μ,σ)=g(μ,σ). Therefore, USpa=max{g(μ,b1)|a1⩽μ⩽T}. Since g(μ,b1) strictly increases as μ increases, we have USpa=g(T,b1). Similarly, the LSpa=min{g(μ,b2)|a1⩽μ⩽T}=g(a1,b2).
Case II: T<a1<a2
Spa(μ,σ) is equal to f(μ,σ) because of μ>T. We can find USpa=max{f(μ,b1)|T<a1⩽μ⩽a2}. f(μ,b1) strictly decreases as μ increases such that USpa=f(a1,b1). Similarly, we have LSpa=min{f(μ,b2)|T<a1⩽μ⩽a2}=f(a2,b2).
Case III: a1<a2<T
Spa(μ,σ) is equal to g(μ,σ) because of μ<T. We can find USpa=max{g(μ,b1)|a1⩽μ⩽a2<T}. g(μ,b1) strictly increases as μ increases such that USpa=g(a2,b1). Similarly, we obtain LSpa=min{g(μ,b2)|a1⩽μ⩽a2<T}=g(a1,b2).
The above MP model is calculated based on the sample data of the in-control process. In the short term, the XS control chart can be employed to monitor whether the process is stable; after the process is stable, the chart data can be controlled to conduct a process capability evaluation. In the long term, the stable process capability can be evaluated by simple random sampling, in order to solve the problem of cost and timeliness [32,33,34,35]. Let X1,…,Xn be a random sample obtained from an in-control process with the normal distribution. A natural estimate of Spa(μ,σ) may be received from replacing (1) the unrevealed process mean μ with the sample mean ˉX=(X1+X2+⋯+Xn)/(X1+X2+⋯+Xn)nn and (2) the unrevealed process standard deviation σ with the sample standard deviation S=[∑(Xi−ˉX)2/(Xi−ˉX)2(n−1)(n−1)]1/122, respectively. Hence, the MP model may be re-expressed as shown in Eqs (12) and (13):
{MaxSpa(ˉX,S)s.t.a1⩽ˉX⩽a2b1⩽S⩽b2for UˆSpa,{MinSpa(ˉX,S)s.t.a1⩽ˉX⩽a2b1⩽S⩽b2for LˆSpa. | (14) |
The estimates of upper and lower limits of confidence interval are denoted by LˆSpa and UˆSpa respectively.
Spa(ˉX,S)={f′(ˉX,S)=13Φ−1{12Φ[dAS(1−ˉX−TDu)]+12Φ[dAS(1+ˉX−TDu)]},ˉX⩾Tg′(ˉX,S)=13Φ−1{12Φ[dAS(1−ˉX−TDl)]+12Φ[dAS(1+ˉX−TDl)]},ˉX<T. | (15) |
For a normal process, the (1−α/2)% confidence interval estimator of process μ is (ˉX−tα/α44(n−1)⋅S/S√n√n,ˉX+tα/α44(n−1)⋅S/S√n√n) and (1−α/2)% confidence interval estimator of process σ is (√(n−1)S2/(n−1)S2χ2α/α44(n−1)χ2α/α44(n−1),√(n−1)S2/(n−1)S2χ21−α/1−α44(n−1)χ21−α/1−α44(n−1)), where t(n−1) is distributed as a Student's t-distribution with n−1 degrees of freedom while χ2(n−1) is distributed as a chi-square distribution with n−1 degrees of freedom. We can derive the (1−α)% confidence interval estimator of the FSA(μ,σ) according to the Cartesian product:
FSA(μ,σ)={[ˆa1⩽μ⩽ˆa2]∩[ˆb1⩽σ⩽ˆb2]} ={[ˉX−tα/α44(n−1)⋅S√n⩽μ⩽ˉX+tα/α44(n−1)⋅S√n]∩[√(n−1)S2χ2α/α44(n−1)⩽σ⩽√(n−1)S2χ21−α/α44(n−1)]}. | (16) |
That is, the estimators of (a1,a2) and (b1,b2) are expressed as
∵P{[ˉX−tα/α44(n−1)⋅S√n⩽μ⩽ˉX+tα/α44(n−1)⋅S√n]∩[√(n−1)S2χ2α/α44(n−1)⩽σ⩽√(n−1)S2χ21−α/α44(n−1)]} ⩾1−P{[ˉX+tα/α44(n−1)⋅S√n<μ]∪[ˉX−tα/α44(n−1)⋅S√n>μ]} −P{[√(n−1)S2χ21−α/α44(n−1)<σ]∪[√(n−1)S2χ2α/α44(n−1)>σ]} =1−α2−α2=1−α. | (17) |
They result in
(ˆa1,ˆa2)=(ˉX−tα/α44(n−1)⋅S/S√n√n,ˉX+tα/α44(n−1)⋅S/S√n√n), (ˆb1,ˆb2)=(√(n−1)S2/(n−1)S2χ2α/α44(n−1)χ2α/α44(n−1),√(n−1)S2/(n−1)S2χ21−α/1−α44(n−1)χ21−α/1−α44(n−1)). | (18) |
Further, we can obtain the estimates of upper and lower limits of confidence interval, LˆSpa and UˆSpa, by following the analyses described in cases I~III. The solutions of the MP model based on the sample data are illustrated in Table 2.
Solutions | Case I.(1) | Case I.(2) | Case II | Case III |
ˆa1⩽T⩽ˉX⩽ˆa2 | ˆa1⩽ˉX⩽T⩽ˆa2 | T<ˆa1⩽ˉX⩽ˆa2 | ˆa1⩽ˉX⩽ˆa2<T | |
LˆSpa | f′(ˆa2,ˆb2) | g′(ˆa1,ˆb2) | f′(ˆa2,ˆb2) | g′(ˆa1,ˆb2) |
UˆSpa | f′(T,ˆb1) | g′(T,ˆb1) | f′(ˆa1,ˆb1) | g′(ˆa2,ˆb1) |
For unilateral process specifications, indices Cpu and Cpl are applied to assess process capability. The 100(1−α)% lower and upper confidence limits, Cl and Cu for Cpu and Cpl, satisfy P(Cl⩽Cpi⩽Cu)=1−α, for i∈{l,u}. That is,
P(Cl⩽Cpi)=1−α2,P(Cu⩽Cpi)=α2,for i∈{l,u}. | (19) |
Based on Chou et al. [36] as well as Pearn and Chen [37], the uniformly minimum-variance unbiased estimators (UMVUEs) for indices Cpu and Cpl are viewed as ˜Cpu and ˜Cpl respectively. The estimators are displayed as follows:
˜Cpu=bf⋅ˆCpu, and ˜Cpl=bf⋅ˆCpl, | (20) |
And
ˆCpu=USL−ˉX3S,ˆCpl=ˉX−LSL3S, | (21) |
where bf=[2/f]1/122Γ[f/2]/Γ[(f−1)/2], and f=n−1 is regarded as a correction factor. Estimator 3√n˜Cpi/bf for i∈{μ,l} is distributed as non-center t distribution t′(n−1;δ) with n−1 degrees of freedom and non-center parameter δ=3√nCpi. The 100(1−α)% lower and upper confidence limits are seen below:
Cl=bf3√nt′1−α/1−α22(n−1;3√n˜Cpi),Cu=bf3√nt′α/α22(n−1;3√n˜Cpi),for i∈{l,u}. | (22) |
To illustrate the application of Spa -PCAC, this study takes a multi-process product with seven quality characteristics as an example. Assume the sample data of each quality characteristic is obtained from an independent process with normal distribution. Under the condition that α-risk is 0.05 and the sample size is 30, process specifications and confidence intervals of capability indices for seven process characteristics are presented in Table 3.
Quality Characteristics | LSL | T | USL | ˉX | S | (ˆa1,ˆa2) | (ˆb1,ˆb2) | (LˆSpa,UˆSpa) | (Cl,Cu) | ˆCa |
N1 | 1.140 | 1.146 | 1.150 | 1.146 | 0.001 | (1.145, 1.146) | (0.001, 0.001) | (0.875, 1.724) | 0.917 | |
N2 | 3.400 | 3.500 | 3.600 | 3.510 | 0.020 | (3.500, 3.520) | (0.015, 0.028) | (1.022, 2.159) | 0.900 | |
N3 | 51.000 | 52.000 | 53.000 | 51.700 | 0.350 | (51.530, 51.870) | (0.270, 0.492) | (0.489, 1.136) | 0.700 | |
L1 | 7.500 | 8.000 | 0.120 | (1.031, 1.792) | ||||||
L2 | 6.500 | 7.800 | 0.500 | (0.626, 1.137) | ||||||
S1 | 8.000 | 6.000 | 1.000 | (0.467, 0.890) | ||||||
S2 | 30.000 | 25.000 | 1.400 | (0.878, 1.542) |
The required quality level of the product should be at least 4-Sigma and the process yield should be no less than 99.38%. Based on Eqs (9)-(11) with 4-sigma quality level, the capability zone can be show as follows:
Nominal-the-Best : Sk= {(X,Y)|Spa(X,Y)⩾0.91,35⩽Y/X⩽53,X⩾0,Y⩾0}
Smaller-the-Better : Su={(X,Y)|X⩾0.83,Y=0}
Larger-the-Better : Sl={(X,Y)|X=0,Y⩾0.83}
As listed in Table 1, the corresponding indices are ˆSpa=0.91, ˆCpu = 0.83, and ˆCpl=0.83. Meanwhile, both process accuracy and process loss are considered. Index Ca is required to achieve 0.75. Thus, the bold lines of the capable zones and the confidence intervals of corresponding indices can be plotted on the Spa -PCAC, as shown in Figure 3.
Based on the evaluation rules and considering the above seven quality characteristics of the multi-process product, the process capabilities are illustrated as follows:
(1) Quality characteristics N1, N2, and N3 belong to the NTB type with bilateral specifications. The 95% confidence interval of N2 is situated in the capability zone. The process capability of quality characteristic N2 is capable. N1 and N3 are not entirely situated in the process capability zone, which indicates that the process capabilities of N1 and N3 do not reach the 4-Sigma requirement. Thus, it is necessary to take action to improve their process quality, but N3 in particular should identify all assignable causes to increase the quality level. That can be done by moving the mean of the process to its target and decreasing its variation.
(2) Quality characteristics S1 and S2 fall into the STB type with unilateral specifications. Quality characteristic S2 reaches the required 4-Sigma level, whereas the confidence interval of S1 is not situated in the capability zone on the X-axis. Therefore, it is necessary to take action to uplift the process capabilities.
(3) Quality characteristics L1 and L2 are categorized into the LTB type with unilateral specifications. The confidence interval of L2 is not situated on the bold line of the capability zone on the Y-Axis. To enhance the process quality, it is necessary to reduce the variation of the process or deviate the mean from the lower specification limit. As for quality characteristic L1, it currently meets the 4-Sigma requirement, but it should be continuously monitored.
To monitor the process capabilities of a multi-process product, managers have to obtain sufficient and reliable information in order to implement quality improvements. This study attempts to improve several insufficiencies to enable managers to make such informed decisions. First, this study reconstructed an efficient method via the Spa -PCAC, which not only represented multiple process capabilities with asymmetric bilateral specifications by PCIs Spa and Ca but also used PCIs Cpu and Cpl to assess multiple process capabilities categorized into the STB and LTB types displayed on the horizontal and vertical axes respectively. This study selected index Spa, superior to index Spk, especially when evaluating process capabilities with asymmetric bilateral specifications. Second, as stated by Cheng [26] as well as Pearn and Chen [37], a large degree of uncertainty or risk needs to be taken into account regarding capability assessments for sampling errors. To reliably evaluate the quality of an entire product consisting of multiple characteristics, the proposed Spa -PCAC considered the interval estimates of the used indices. Third, based on the loss function stated in the Taguchi method [38,39,40,41,42], it can be learned that as long as the mean is closer to the target, the quality will be the better and the loss will be less. Index Ca was incorporated into the Spa -PCAC because it is adequate to assess the accuracy of the manufacturing process. Lastly, the quality-level of Six-Sigma and the connection with process yield were introduced in the capability zone of Spa -PCAC to check whether the process capabilities could reach the preset level. The Spa -PCAC explained the multi-characteristics process capabilities and distinguished the process accuracy from the locations of the interval estimates of PCIs. Furthermore, with respect to the unsatisfactory processes, actions for quality improvement were taken to enhance the entire process capabilities. Consequently, our proposed model is a valuable tool for managers to improve their manufacturing processes. Finally, when the process distribution is abnormal, the model proposed in this study is not applicable. This is the research limitation of this paper. The confidence interval derived by the mathematical programming method in this article is easier than the traditional confidence interval, so it is more suitable for the industry which emphasizes timeliness. As to how much higher than 100(1−α)% it should be, it is a more complicated issue. It is recommended to refer to the method proposed Chen et al. [34] for discussion. This study will list it as a future research topic.
This work was supported in part by the Ministry of Science and Technology, Taiwan, under grant number MOST 108-2622-E-167-008-CC3.
All authors declare no conflicts of interest in this paper.
[1] |
K. P. Lin, C. M. Yu, K. S. Chen, Production data analysis system using novel process capability indices-based circular economy, Ind. Manage. Data Syst., 119 (2019), 1655-1668. doi: 10.1108/IMDS-03-2019-0166
![]() |
[2] |
K. S. Chen, C. M. Yu, T. H. Hsu, S. R. Cai, K. C. Chiou, A model for evaluating the performance of the bearing manufacturing process, Appl. Sci., 9 (2019), 3105. doi: 10.3390/app9153105
![]() |
[3] | K. S. Chen, H. T. Chen, T. C. Chang, The construction and application of Six Sigma quality indices, Int. J. Prod. Res., 55 (2017), 2365-2384. |
[4] |
K. S. Chen, K. T. Yu, S. H. Sheu, Process capability monitoring chart with an application in the silicon-filler manufacturing process, Int. J. Prod. Econ., 103 (2006), 565-571. doi: 10.1016/j.ijpe.2005.11.004
![]() |
[5] |
M. H. Shu, K. S. Chen, Estimating process capability indices based on subsamples for asymmetric tolerancess, Commun. Stat. Theory Methods, 34 (2005), 485-505. doi: 10.1081/STA-200045863
![]() |
[6] |
J. Xu, C. Peng, Parametric bootstrap process capability index control charts for both mean and dispersion, Commun. Stat. Simul. Comput., 48 (2019), 2936-2954. doi: 10.1080/03610918.2018.1471505
![]() |
[7] |
S. C. Singhal, A new chart for analyzing multi-process performance, Qual. Eng., 2 (1990), 379-390. doi: 10.1080/08982119008962734
![]() |
[8] | S. C. Singhal, Multi-process performance analysis chat (MPPAC) with capability zones, Qual. Eng., 4 (1991), 75-81. |
[9] |
W. L. Pearn, K. S. Chen, Multi-process performance analysis: a case study, Qual. Eng., 10 (1997), 1-8. doi: 10.1080/08982119708919102
![]() |
[10] |
K. S. Chen, K. T. Yu, S. H. Sheu, Process capability monitoring chart with an application in the silicon-filler manufacturing process, Int. J. Prod. Econ., 103 (2006), 565-571. doi: 10.1016/j.ijpe.2005.11.004
![]() |
[11] |
K. S. Chen, M. L. Huang, R. K. Li, Process capability analysis for an entire product, Int. J. Prod. Res., 39 (2001), 4077-4087. doi: 10.1080/00207540110073082
![]() |
[12] |
M. L. Huang, K. S. Chen, Y. H. Hung, Integrated process capability analysis with an application in backlight module, Microelectron. Reliab., 42 (2002), 2009-2014. doi: 10.1016/S0026-2714(02)00126-9
![]() |
[13] |
K. S. Chen, W. L. Pearn, P. C. Lin, Capability measures for processes with multiple characteristics, Quality Reliab. Eng. Int., 19 (2003), 101-110. doi: 10.1002/qre.513
![]() |
[14] |
W. L. Pearn, M. H. Shu, B. M. Hsu, Monitoring manufacturing quality for multiple Li-BPIC processes based on capability index Cpmk, Int. J. Prod. Res., 43 (2005), 2493-2512. doi: 10.1080/00207540500045741
![]() |
[15] |
W. L. Pearn, C. W. Wu, Production quality and yield assurance for processes with multiple independent characteristics, Eur. J. Oper. Res., 173 (2006), 637-647. doi: 10.1016/j.ejor.2005.02.050
![]() |
[16] |
H. T. Chen, K. S. Chen, Advanced multi-process performance analysis chart for an entire product with joint confidence regions, Int. J. Prod. Res., 45 (2007), 2141-2159. doi: 10.1080/00207540600677658
![]() |
[17] |
K. S. Chen, M. L. Huang, Y. H. Hung, Process capability analysis chart with the application of Cpm, Int. J. Prod. Res., 46 (2008), 4483-4499. doi: 10.1080/00207540600806422
![]() |
[18] |
T. C. Chang, K. J. Wang, K. S. Chen, Capability performance analysis for processes with multiple characteristics using accuracy and precision, Proc. Inst. Mech. Eng., Part B, 228 (2014), 766-776. doi: 10.1177/0954405413508118
![]() |
[19] |
H. T. Chen, K. S. Chen, Assessing the assembly quality of a T-bar ceiling suspension by using an advanced multi-process performance analysis chart with asymmetric tolerance, Eur. J. Ind. Eng., 10 (2016), 264-283. doi: 10.1504/EJIE.2016.075857
![]() |
[20] | V. E. Kane, Process capability indices, J. Qual. Technol., 18 (1986), 41-52. |
[21] |
R. A. Boyles, Process capability with asymmetric tolerances, Commun. Stat. Simul. Comput., 23 (1994), 615-643. doi: 10.1080/03610919408813190
![]() |
[22] |
W. L. Pearn, M. H. Shu, Measuring manufacturing capability based on lower confidence bounds of Cpmk applied to current transmitter process, Int. J. Adv. Manuf. Technol., 23 (2004), 116-125. doi: 10.1007/s00170-003-1693-z
![]() |
[23] |
K. Linderman, R. G. Schroeder, S. Zaheer, A. S. Choo, Six-Sigma: A goal-theoretic perspective, J. Oper. Manage., 21 (2003), 193-203. doi: 10.1016/S0272-6963(02)00087-6
![]() |
[24] |
K. S. Chen, L. Y. Ouyang, C. H. Hsu, C. C. Wu, The communion bridge to Six-Sigma and process capability indices, Qual. Quant., 43 (2009), 463-469. doi: 10.1007/s11135-007-9123-1
![]() |
[25] | Y. C. Hsu, W. L. Pearn, P. C. Wu, Capability adjustment for gamma processes with mean shift consideration in implementing Six-Sigma program, Eur. J. Oper. Res., 191 (2008), 517-529. |
[26] |
S. W. Cheng, Practical implementation of the process capability indices, Qual. Eng., 7 (1994), 239-259. doi: 10.1080/08982119408918781
![]() |
[27] |
K. S. Chen, Fuzzy testing decision-making model for intelligent manufacturing process with Taguchi capability index, J. Intell. Fuzzy Syst., 38 (2020), 2129-2139. doi: 10.3233/JIFS-190865
![]() |
[28] |
K. S. Chen, Two-tailed Buckley fuzzy testing for operating performance index, J. Comput. Appl. Math., 361 (2019), 55-63. doi: 10.1016/j.cam.2019.04.019
![]() |
[29] | K. S. Chen, T. C. Chang, Fuzzy testing model for the lifetime performance of products under consideration with exponential distribution, Ann. Oper. Res., 2020 (2020), 1-12. |
[30] | K. S. Chen, C. H. Wang, K. H. Tan, Developing a fuzzy green supplier selection model using Six Sigma quality indices. Int. J. Prod. Econ., 212 (2019), 1-7. |
[31] |
K. S. Chen, C. M. Yu, Fuzzy test model for performance evaluation matrix of service operating systems, Comput. Ind. Eng., 140 (2020), 106240. doi: 10.1016/j.cie.2019.106240
![]() |
[32] | D. C. Montgomery, Introduction to Statistical Quality Control, 7th ed., Wiley, New York, 2012. |
[33] |
K. T. Yu, S. H. Sheu, K. S. Chen, The evaluation of process capability for a machining center, Int. J. Adv. Manuf. Technol., 33 (2007), 505-510. doi: 10.1007/s00170-006-0481-y
![]() |
[34] |
K. S. Chen, H. T. Chen, T. C. Chang, The construction and application of Six Sigma quality indices, Int. J. Prod. Res., 55(2017), 2365-2384. doi: 10.1080/00207543.2016.1246763
![]() |
[35] | K. S. Chen, K. J. Wang, T. C. Chang, A novel approach to deriving the lower confidence limit of indices Cpu, Cpl, and Cpk in assessing process capability, Int. J. Prod. Res., 55 (2017), 4963-4981. |
[36] |
Y. M. Chou, D. B. Owen, Lower confidence limits on process capability indices, J. Qual. Technol., 22 (1990), 223-229. doi: 10.1080/00224065.1990.11979242
![]() |
[37] |
W. L. Pearn, K. S. Chen, One-sided capability indices Cpu and Cpl: decision making with sample information, Int. J. Qual. Reliab. Manage., 19 (2002), 221-245. doi: 10.1108/02656710210421544
![]() |
[38] | A. V. Feigenbaum, Quality control: principles, practice and administration; an industrial management tool for improving product quality and design and for reducing operating costs and losses, McGraw-Hill industrial organization and management series, New York, McGraw-Hill, 1945. |
[39] | A. V. Feigenbaum, Total Quality Control, McGraw-Hill, New York, 1961. |
[40] | G. Taguchi, S. Konishi, Taguchi Methods, Orthogonal Arrays and Linear Graphs: Tools for Quality, American Supplier Institute, 1987. |
[41] | R. A. Boyles, The Taguchi capability index, J. Qual. Technol., 23 (1991), 17-26. |
[42] | K. S. Chen, T. C. Chang, Construction and fuzzy hypothesis testing of Taguchi Six Sigma quality index, Int. J. Prod. Res., 58 (2020), 3110-3125. |
1. | Chun-Min Yu, Chih-Feng Wu, Kuen-Suan Chen, Chang-Hsien Hsu, Fuzzy Quality Evaluation Model Constructed by Process Quality Index, 2021, 11, 2076-3417, 11262, 10.3390/app112311262 | |
2. | Chiao-Tzu Huang, Kuei-Kuei Lai, Process Quality Evaluation Model with Taguchi Cost Loss Index, 2021, 11, 2076-3417, 10182, 10.3390/app112110182 | |
3. | Kuen-Suan Chen, Chang-Hsien Hsu, Kuo-Ching Chiou, Product quality evaluation by confidence intervals of process yield index, 2022, 12, 2045-2322, 10.1038/s41598-022-14595-y | |
4. | Kuen-Suan Chen, Tsung-Hua Hsieh, Chun-Min Yu, Kai-Chao Yao, Smaller-the-better-type six sigma product index, 2023, 13, 2045-2322, 10.1038/s41598-023-44721-3 |
Quality Level (k-Sigma) |
Spa (k) | Cpi (k) i = u, l |
Process Yield (%) |
3-Sigma | 0.61 | 0.50 | 93.319 |
4-Sigma | 0.91 | 0.83 | 99.361 |
5-Sigma | 1.23 | 1.17 | 99.977 |
6-Sigma | 1.55 | 1.50 | 99.999 |
Solutions | Case I.(1) | Case I.(2) | Case II | Case III |
ˆa1⩽T⩽ˉX⩽ˆa2 | ˆa1⩽ˉX⩽T⩽ˆa2 | T<ˆa1⩽ˉX⩽ˆa2 | ˆa1⩽ˉX⩽ˆa2<T | |
LˆSpa | f′(ˆa2,ˆb2) | g′(ˆa1,ˆb2) | f′(ˆa2,ˆb2) | g′(ˆa1,ˆb2) |
UˆSpa | f′(T,ˆb1) | g′(T,ˆb1) | f′(ˆa1,ˆb1) | g′(ˆa2,ˆb1) |
Quality Characteristics | LSL | T | USL | ˉX | S | (ˆa1,ˆa2) | (ˆb1,ˆb2) | (LˆSpa,UˆSpa) | (Cl,Cu) | ˆCa |
N1 | 1.140 | 1.146 | 1.150 | 1.146 | 0.001 | (1.145, 1.146) | (0.001, 0.001) | (0.875, 1.724) | 0.917 | |
N2 | 3.400 | 3.500 | 3.600 | 3.510 | 0.020 | (3.500, 3.520) | (0.015, 0.028) | (1.022, 2.159) | 0.900 | |
N3 | 51.000 | 52.000 | 53.000 | 51.700 | 0.350 | (51.530, 51.870) | (0.270, 0.492) | (0.489, 1.136) | 0.700 | |
L1 | 7.500 | 8.000 | 0.120 | (1.031, 1.792) | ||||||
L2 | 6.500 | 7.800 | 0.500 | (0.626, 1.137) | ||||||
S1 | 8.000 | 6.000 | 1.000 | (0.467, 0.890) | ||||||
S2 | 30.000 | 25.000 | 1.400 | (0.878, 1.542) |
Quality Level (k-Sigma) |
Spa (k) | Cpi (k) i = u, l |
Process Yield (%) |
3-Sigma | 0.61 | 0.50 | 93.319 |
4-Sigma | 0.91 | 0.83 | 99.361 |
5-Sigma | 1.23 | 1.17 | 99.977 |
6-Sigma | 1.55 | 1.50 | 99.999 |
Solutions | Case I.(1) | Case I.(2) | Case II | Case III |
ˆa1⩽T⩽ˉX⩽ˆa2 | ˆa1⩽ˉX⩽T⩽ˆa2 | T<ˆa1⩽ˉX⩽ˆa2 | ˆa1⩽ˉX⩽ˆa2<T | |
LˆSpa | f′(ˆa2,ˆb2) | g′(ˆa1,ˆb2) | f′(ˆa2,ˆb2) | g′(ˆa1,ˆb2) |
UˆSpa | f′(T,ˆb1) | g′(T,ˆb1) | f′(ˆa1,ˆb1) | g′(ˆa2,ˆb1) |
Quality Characteristics | LSL | T | USL | ˉX | S | (ˆa1,ˆa2) | (ˆb1,ˆb2) | (LˆSpa,UˆSpa) | (Cl,Cu) | ˆCa |
N1 | 1.140 | 1.146 | 1.150 | 1.146 | 0.001 | (1.145, 1.146) | (0.001, 0.001) | (0.875, 1.724) | 0.917 | |
N2 | 3.400 | 3.500 | 3.600 | 3.510 | 0.020 | (3.500, 3.520) | (0.015, 0.028) | (1.022, 2.159) | 0.900 | |
N3 | 51.000 | 52.000 | 53.000 | 51.700 | 0.350 | (51.530, 51.870) | (0.270, 0.492) | (0.489, 1.136) | 0.700 | |
L1 | 7.500 | 8.000 | 0.120 | (1.031, 1.792) | ||||||
L2 | 6.500 | 7.800 | 0.500 | (0.626, 1.137) | ||||||
S1 | 8.000 | 6.000 | 1.000 | (0.467, 0.890) | ||||||
S2 | 30.000 | 25.000 | 1.400 | (0.878, 1.542) |