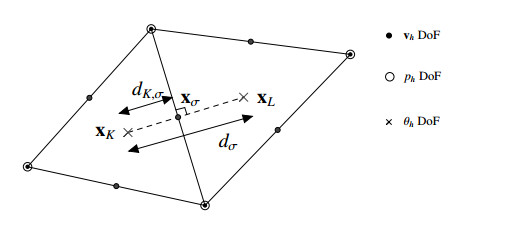
Citation: Yong Wang, Li-qun Gu. Biomedical diagnosis perspective of epigenetic detections using alpha-hemolysin nanopore[J]. AIMS Materials Science, 2015, 2(4): 448-472. doi: 10.3934/matersci.2015.4.448
[1] | Shengying Mu, Yanhui Zhou . An analysis of the isoparametric bilinear finite volume element method by applying the Simpson rule to quadrilateral meshes. AIMS Mathematics, 2023, 8(10): 22507-22537. doi: 10.3934/math.20231147 |
[2] | Zuliang Lu, Ruixiang Xu, Chunjuan Hou, Lu Xing . A priori error estimates of finite volume element method for bilinear parabolic optimal control problem. AIMS Mathematics, 2023, 8(8): 19374-19390. doi: 10.3934/math.2023988 |
[3] | Yuelong Tang . Error estimates of mixed finite elements combined with Crank-Nicolson scheme for parabolic control problems. AIMS Mathematics, 2023, 8(5): 12506-12519. doi: 10.3934/math.2023628 |
[4] | Zhichao Fang, Ruixia Du, Hong Li, Yang Liu . A two-grid mixed finite volume element method for nonlinear time fractional reaction-diffusion equations. AIMS Mathematics, 2022, 7(2): 1941-1970. doi: 10.3934/math.2022112 |
[5] | Yu Xu, Youjun Deng, Dong Wei . Numerical solution of forward and inverse problems of heat conduction in multi-layered media. AIMS Mathematics, 2025, 10(3): 6144-6167. doi: 10.3934/math.2025280 |
[6] | H. S. Alayachi, Mahmoud A. E. Abdelrahman, Kamel Mohamed . Finite-volume two-step scheme for solving the shear shallow water model. AIMS Mathematics, 2024, 9(8): 20118-20135. doi: 10.3934/math.2024980 |
[7] | Shuangbing Guo, Xiliang Lu, Zhiyue Zhang . Finite element method for an eigenvalue optimization problem of the Schrödinger operator. AIMS Mathematics, 2022, 7(4): 5049-5071. doi: 10.3934/math.2022281 |
[8] | Jie Liu, Zhaojie Zhou . Finite element approximation of time fractional optimal control problem with integral state constraint. AIMS Mathematics, 2021, 6(1): 979-997. doi: 10.3934/math.2021059 |
[9] | Zuliang Lu, Xiankui Wu, Fei Huang, Fei Cai, Chunjuan Hou, Yin Yang . Convergence and quasi-optimality based on an adaptive finite element method for the bilinear optimal control problem. AIMS Mathematics, 2021, 6(9): 9510-9535. doi: 10.3934/math.2021553 |
[10] | Weiwen Wan, Rong An . Convergence analysis of Euler and BDF2 grad-div stabilization methods for the time-dependent penetrative convection model. AIMS Mathematics, 2024, 9(1): 453-480. doi: 10.3934/math.2024025 |
Variable density and low Mach numbers flows have been widely investigated for the last decades. Indeed, they arise in plenty of physical phenomena in which the sound wave speed is much faster than the convective characteristics of the fluid : flows in high-temperature gas reactors, meteorological flows, combustion processes and many others. In this work, we are interested in a specific model derived from the usual low-Mach one for a calorically perfect gas, coming from an asymptotic expansion of the variables with respect to the Mach number $ \mathcal{M} $ in the Navier-Stokes compressible equations (see [34]). For the usual low-Mach model, the local-in-time existence of classical solutions in Sobolev spaces is established in [23]. As observed in [33], a small perturbation of a constant initial density provides a global existence of weak solutions in the two-dimensional case. The originality of the model considered here relies on the dynamic viscosity of the fluid being explicitly given as a function of the temperature, as introduced in [4] and generalized in [5]. In this recent work, the authors establish the global existence of weak solutions in the three-dimensional case with no smallness assumption on the initial velocity.
Thanks to a change of variables, we obtain a system in which the velocity field is divergence-free, leading in return to the presence of a non linear and so-called "Joule term" in the mass conservation equation expressed in term of the temperature. In [8], some theoretical results are obtained on the local-in-time existence of strong solutions in the three-dimensional case. We mention also [19] where the authors study the local and global existence in critical Besov spaces, assuming that the initial density is close to a constant and that the initial velocity is small enough. The formulation of this model is close to the so-called ghost effect system, considered in [30,32], where a thermal stress term is added to the right-hand-side of the momentum equation. The local well-posedness of classical solutions is established in [32] for 2D or 3D unbounded domains. A local well-posedness result for strong solutions is proved in [30], where the authors give also the existence and uniqueness of a global strong solution for the two-dimensional case.
From a numerical point of view, many authors compute flows at low-Mach number regime. We refer only to the so-called pressure-based methods, widely used to compute incompressible flows (see e.g. [1,2,20,29,31]), but there exists also the so-called density-based methods widely used to compute supersonic or transonic flows, and recently adapted in the case of low-Mach regime (see [27,28]). In previous contributions on incompressible variable density flows [6,9,10] or on low-Mach flows with large variations of temperature [7], a combined finite volume - finite element scheme was proposed. Based on a time splitting, this combined method allows to solve the mass conservation equation by a finite volume method, whereas the momentum equation associated with the divergence free constraint and the temperature one are solved by a finite element method. It allows, in particular, to preserve the constant density states and to ensure the discrete maximum principle. In the present work, following the same idea, the nonlinear temperature equation is solved by a finite volume method, whereas the velocity equation associated with the divergence free constraint is solved by a finite element one.
The main contribution of this paper is twofold. First, we prove a discrete maximum principle on the temperature (see Theorem 3.1), similarly to the solution behavior at the continuous level. Second, we establish a footbridge between the finite volume fluxes and the finite element velocity field (see subsection 3.4), to ensure the good consistency of the method.
The outline of the paper is the following. Section 2 briefly introduces the model derivation. Section 3 details the proposed combined finite volume - finite element scheme. The maximum principle is established (Theorem 3.1), some variants of the original scheme are proposed (subsection 3.3), and the link between the finite volume fluxes and the finite element velocity field is carefully explained (subsection 3.4). Finally, section 4 proposes several numerical tests to illustrate the obtained theoretical results, and to investigate the behavior of the scheme on a physical benchmark corresponding to the convection of the temperature in a cavity.
As already mentioned in the introduction, a low-Mach model is obtained by inserting the asymptotic expansions of the variables with respect to the Mach number $ \mathcal{M} $ in the Navier-Stokes compressible equations (see for example [2,20,34]). One of the characteristics of the process is to consider the asymptotic expansion of the pressure $ \pi $ with respect to $ \mathcal{M} $. Denoting $ \textbf{x} \in \mathbb{R}^d $ as the space variable and $ t \in \mathbb{R}^+_* $ as the time one, we write
$ π(x,t)=P(t)+M2q(x,t)+o(M2), $ |
where $ P $ is called the thermodynamic pressure and $ q $ the dynamic pressure. Here, we assume that $ P(t) = P_0 > 0 $ is constant for all $ t\geq0 $. The other variables considered here are the velocity $ \mathbf{u}(\textbf{x}, t) $, the density $ \rho(\textbf{x}, t) $ and the temperature $ \theta(\textbf{x}, t) $.
Let $ \Omega \subset \mathbb{R}^d $ be an open polygonal domain with a boundary $ \Gamma $ and $ T $ a positive real. The continuity, momentum, temperature and state equations in $ \mathcal{Q}_T = \Omega \times [0;T] $ for a calorically perfect gas are given by:
$ ∂tρ+∇⋅(ρu)=0, $ | (2.1a) |
$ [0.1cm]ρ∂tu+ρu⋅∇u+∇q−∇⋅(˜μ(2Du−23∇⋅uI))=ρg, $ | (2.1b) |
$ [0.1cm]∇⋅u=γ−1γP0∇⋅(κ∇θ), $ | (2.1c) |
$ [0.1cm]P0=Rρθ, $ | (2.1d) |
where $ \tilde{\mu} $ is the viscosity of the flow, $ \kappa $ is the heat conductivity which is assumed constant, $ R $ is the gas law constant and $ \gamma $ is the gas specific heat ratio. Here, $ {\mathbb{D}} \mathbf{u} = (\nabla \mathbf{u} + \nabla^T \mathbf{u})/2 $ denotes the deformation tensor, I the identity matrix and $ \mathbf{g}(\textbf{x}, t) $ the gravity field. In order to reduce the study to a system whose velocity is solenoidal, we define:
$ v=u−λ∇θ, $ |
where $ \lambda = \dfrac{(\gamma-1) \kappa}{\gamma \, P_0} > 0 $ is a fixed constant. In addition, the density is eliminated from the equations thanks to the state equation (2.1d). Following the idea introduced in [5] where a particular relation between the density and the viscosity in the combustion model was introduced, we assume moreover that
$ ˜μ(θ)=P0Rμ(θ), $ |
with
$ μ(θ)=−λlnθ, $ |
so that $ \mu(\theta) $ is strictly positive if and only if $ \theta \in (0;1) $. If we denote by $ p $ the modified pressure, defined by:
$ p = \frac{R}{P_0} q + \lambda^2 \Delta \theta - \frac{2 \, \lambda}{3} \mu(\theta) \; \Delta \theta, $ |
we consequently obtain (see [8] for all details):
$ ∂tθ+∇⋅(θv)+2λ|∇θ|2−λ∇⋅(θ∇θ)=0, $ | (2.2a) |
$ 1θ(∂tv+(v⋅∇)v)−∇⋅(μ(θ)Dv)+λθ(∇v−∇Tv)∇θ+∇p=1θg, $ | (2.2b) |
$ ∇⋅v=0. $ | (2.2c) |
The system (2.2) needs to be completed with suitable initial and boundary conditions. We set $ \overline{\Gamma} = \overline{\Gamma^D} \cup \overline{\Gamma^N} $, with $ \Gamma^D \cap \Gamma^N = \emptyset $. The initial conditions for the system (2.2) are given by:
$ θ(x,0)=θ0(x)∀x∈Ω,v(x,0)=v0(x)∀x∈Ω, $ |
with:
$ 0<m≤minx∈Ωθ0(x)≤maxx∈Ωθ0(x)≤M<1. $ |
The boundary conditions on the temperature and the velocity are given by:
$ ∇θ(x,t)⋅n=0,∀x∈ΓN,∀t∈[0;T],θ(x,t)=θD(x,t),∀x∈ΓD,∀t∈[0;T],v(x,t)=vD(x,t),∀x∈Γ,∀t∈[0;T]. $ |
The local existence of a regular solution to the problem (2.2) has been shown in [8] in the case of dimension $ d = 3 $, as well as the maximum principle for the temperature. Furthermore, the unique global strong solution can be proved in the case of dimension $ d = 2 $ following the proof of Theorem 1.5 in [30] and assuming that for any fixed $ \bar \theta > 0 $, there exists a small constant $ \delta(\bar \theta) > 0 $ such that
$ ‖θ0−ˉθ‖L∞(Ω)≤δ(ˉθ). $ | (2.3) |
In this paper, we will prove that there exists at least one solution to the discretized temperature equation, and that this solution verifies the maximum principle. The questions of unicity and convergence of the numerical scheme will be addressed in a further work.
The numerical scheme is based on a time splitting, allowing to solve the temperature equation with finite volumes, whereas the velocity equation is solved with finite elements, using the same strategy as in previous works [7,9].
Let $ \Delta t $ be the time step and $ t^n = n \, \Delta t $. Functions approximated at time $ t^n $ will be identified with superscript $ n $. Assuming that $ \theta^n $ and $ \mathbf{v}^n $ are known:
1. We first compute the new temperature $ \theta^{n+1} $ by solving the temperature equation (2.2a) using an Euler implicit scheme:
$ {θn+1−θnΔt+∇⋅(θn+1vn)+2λ|∇θn+1|2−λ∇⋅(θn+1∇θn+1)=0,∇θn+1(x)⋅n=0,∀x∈ΓN,θn+1(x)=θn+1D(x),∀x∈ΓD, $ | (3.1) |
with $ \theta_D^{n+1}(\textbf{x}) = \theta_D(\textbf{x}, t^{n+1}) $. The non-linearities of Equation (3.1) are treated thanks to Newton's method.
2. Then we compute the new velocity $ \mathbf{v}^{n+1} $ and the new pressure $ p^{n+1} $ by solving the momentum equation (2.2b)-(2.2c) by:
$ {1θn+1(vn+1−vnΔt+(vn⋅∇)vn+1)−∇⋅(μ(θn+1)Dvn+1)+λθn+1(∇vn+1−∇Tvn+1) ∇θn+1+∇pn+1=1θn+1g,∇⋅vn+1=0,vn+1(x)=vn+1D(x),∀x∈Γ. $ | (3.2) |
Here and as usual, the nonlinear term in the momentum equation (3.2) is linearized.
From now, we fix $ d = 2 $, but our study can be easily extended to the three-dimensional case. The discretization in space is based on a triangulation $ \mathcal{T} $ of the domain $ \Omega \subset \mathbb{R}^2 $ (the elements of $ \mathcal{T} $ are called control volumes), a family $ \mathcal{E} $ of edges and a set $ \mathcal{P} = (\textbf{x}_K)_{K\in \mathcal{T}} $ of points of $ \Omega $ defining an admissible mesh in the sense of Definition 3.1 in [25]. We recall that the admissibility of $ \mathcal{T} $ implies that the straight line between two neighboring centers of cells $ \textbf{x}_K $ and $ \textbf{x}_L $ is orthogonal to the edge $ \sigma\in \mathcal{E} $ such that $ \overline{\sigma} = \overline{K} \cap \overline{L} $ (and which is noted $ \sigma = K|L $) in a point $ \textbf{x}_\sigma $ (see Figure 1). This condition is satisfied if all the angles of the triangles are less than $ \frac{\pi}{2} $.
The set of interior (resp. boundary) edges is denoted by $ \mathcal{E}^{\mathrm{int}} = \left\{ \sigma \in \mathcal{E} \; \ \sigma \not\subset \Gamma\right\} $ (resp. $ \mathcal{E}^{\mathrm{ext}} = \left\{ \sigma \in \mathcal{E} \; \ \sigma \subset \Gamma\right\} $). Among the outer edges, there are $ \mathcal{E}^N = \left\{ \sigma \in \mathcal{E} \; \ \sigma \subset \Gamma^N \right\} $ and $ \mathcal{E}^D = \left\{ \sigma \in \mathcal{E} \; \ \sigma \subset \Gamma^D \right\} $. For all $ K \in \mathcal{T} $, we denote by $ \mathcal{E}_K = \left\{ \sigma \in \mathcal{E} \; \ \sigma \subset \overline{K} \right\} $ the edges of $ K $, $ \mathcal{E}^{\mathrm{int}}_K = \mathcal{E}^{\mathrm{int}} \cap \mathcal{E}_K $, $ \mathcal{E}^{\mathrm{ext}}_K = \mathcal{E}^{\mathrm{ext}} \cap \mathcal{E}_K $, $ \mathcal{E}_K^N = \mathcal{E}_K^N\cap \mathcal{E}_K $ and $ \mathcal{E}_K^D = \mathcal{E}_K^D \cap \mathcal{E}_K $.
The measure of $ K \in \mathcal{T} $ is denoted by $ m_K $ and the length of $ \sigma $ by $ m_\sigma $. For $ \sigma \in \mathcal{E}^{\mathrm{int}} $ such that $ \sigma = K|L $, $ d_\sigma $ denotes the distance between $ \textbf{x}_K $ and $ \textbf{x}_L $ and $ d_{K, \sigma} $ the distance between $ \textbf{x}_K $ and $ \sigma $. For $ \sigma \in \mathcal{E}^{\mathrm{ext}}_K $, we note $ d_\sigma $ the distance between $ \textbf{x}_K $ and $ \sigma $. For $ \sigma \in \mathcal{E} $, The transmissibility coefficient is given by $ \tau_\sigma = \dfrac{m_\sigma}{d_\sigma} $. Finally, for $ \sigma \in \mathcal{E}_K $, we denote by $ \mathbf{n}_{K, \sigma} $ the exterior unit normal vector to $ \sigma $. The size of the mesh is given by:
$ h=maxK∈Tdiam(K). $ |
The piecewise constant temperature $ \theta_h $ is computed with a cell-centered finite volume method described in section 3.2, so that $ \theta_h \in \mathcal{X}(\mathcal{T}) $ with :
$ X(T)={θ∈L2(Ω) ; ∀K∈T ; θK=θ|K∈P0}. $ |
The velocity $ \mathbf{v}_h $ is discretized with $ \mathbb{P}_2 $-Lagrange finite elements, and the pressure $ p_h $ with $ \mathbb{P}_1 $-Lagrange finite elements, so that they satisfy the usual LBB stability condition. The Degrees of Freedom of each variable are shown in the Figure 1.
The computation of the velocity and pressure by finite elements is usual, and we refer to our previous work for details [7,9]. One of the original points of the present work is the computation of the temperature by finite volumes. Indeed, we aim to develop a numerical scheme that ensures the discrete maximum principle property, see section 3.2. Another point of interest is the link between the two numerical methods, see section 3.4. Indeed, from the velocity field that has been computed by finite elements, we have to determine fluxes through the interfaces of the control volumes, which will be used for the computation of the temperature.
Let $ \mathbf{v} $ be a given velocity field and $ \theta^n $ the previous approximate temperature, we aim at solving the temperature equation:
$ {θn+1−θnΔt+∇⋅(θn+1v)+2λ|∇θn+1|2−λ∇⋅(θn+1∇θn+1)=0,∀x∈Ω,∇θn+1(x)⋅n=0,∀x∈ΓN,θn+1(x)=θn+1D(x),∀x∈ΓD. $ | (3.3) |
Since we want to ensure maximum principle properties, we favor a finite volume method. The main difficulty comes from the term $ |\nabla \theta^{n+1}|^2 $, called the Joule term in the context of the electrical conductivity, see for example A. Bradji and R. Herbin [3] or the works from C. Chainais and her collaborators [13,14]. Indeed, the definition of a discrete gradient is not straightforward, since the finite volume solution is piecewise constant. We can thus refer to the work of R. Eymard and his collaborators [24,26] for some definitions of discrete gradients on an admissible mesh. Alternatively, K. Domelevo and P. Omnes [21], and C. Chainais [13], used a discrete gradient reconstruction following the idea from the paper of Y. Coudière, J.-P. Vila and P. Villedieu [18]. The principle of these schemes, valid on very general meshes, consists in the double resolution of the equations, on primal and dual meshes. Moreover in [22], J. Droniou and R. Eymard propose a scheme whose unknowns are the function, its gradient and flows. Therefore, the definition of a discrete gradient by mesh is intrinsic to the scheme.
The respect of the bounds, and in particular of the lower bound, is another difficulty related to the Joule term. Indeed, if we consider "close" models, like the equation of porous media, see for example the work of C. Cancès and C. Guichard [12], or the convection-diffusion equation involved in Khazhikhov-Smagulov models-type, see for example C. Calgaro, M. Ezzoug and E. Zahrouni [11], the maximum principle is obtained quite easily for one order schemes. Nevertheless, adding the positive term $ |\nabla \theta^{n+1}|^2 $ in the temperature equation prevents the scheme from directly obtaining the lower bound. Consequently, we will have to particularly pay attention to the discretization of this term.
We are looking for $ \theta_h^{n+1} = (\theta_K^{n+1})_{K\in \mathcal{T}} \in \mathcal{X}(\mathcal{T}) $ an approximated solution of $ \theta(t^{n+1}, .) $. The finite volume scheme is classically obtained by integrating equation (3.3) on a control volume $ K $, that is:
$ mKθn+1K−θnKΔt+∑σ∈EKvnK,σθn+1σ,++2λmKJK(θn+1h)+λ∑σ∈EKFn+1K,σ=0for all K∈T, $ | (3.4) |
with
$ vnK,σ=∫σv(x,tn)⋅nK,σdγ(x). $ | (3.5) |
Here, $ \theta_{\sigma, +}^{n+1} $ is defined for $ \sigma \in \mathcal{E}_K $ by:
$ θn+1σ,+={θn+1Kif vnK,σ≥0,θn+1K,σotherwise, $ | (3.6) |
with
$ θn+1K,σ={θn+1Lfor σ∈EintK such that σ=K|L,θn+1D(xσ)for σ∈EDK,θn+1Kfor σ∈ENK. $ |
The numerical flux $ F_{K, \sigma}^{n+1} $ is an approximation of the exact flux of the diffusive term through the edge $ \sigma $ and is given by:
$ Fn+1K,σ=τσθn+1σ(θn+1K−θn+1K,σ), $ | (3.7) |
where we define $ \theta_\sigma^{n+1} $, an approximation of $ \theta^{n+1} $ at $ \textbf{x}_\sigma $, by:
$ θn+1σ=max(θn+1K,θn+1K,σ). $ | (3.8) |
Concerning the Joule term, $ \mathcal{J}_K(\theta_h^{n+1}) $ is an approximation of $ \frac{1}{m_K} \int_K |\nabla \theta(t^{n+1}, \textbf{x})|^2 \mathrm{d} \textbf{x} $. We notice that $ |\nabla \theta|^2 = \nabla \cdot (\theta\, \nabla \theta)- \theta \, \Delta \theta $, and we propose the following definition:
$ JK(θn+1h)=1mK∑σ∈EKτσ¯θn+1σ(θn+1K,σ−θn+1K)−1mKθn+1K∑σ∈EKτσ(θn+1K,σ−θn+1K), $ |
where $ \overline{ \theta}_\sigma^{n+1} $ is another approximation of $ \theta^{n+1} $ at $ \textbf{x}_\sigma $ (this choice will be justified later) defined this time by:
$ ¯θn+1σ=θn+1K+θn+1K,σ2. $ | (3.9) |
Finally we obtain:
$ JK(θn+1h)=12mK∑σ∈EKτσ(θn+1K,σ−θn+1K)2. $ | (3.10) |
The rest of the section is devoted to the proof of the following result:
Theorem 3.1. We assume that
$ 0<m≤θ0K≤M,∀K∈T, $ | (3.11) |
and:
$ m≤θnD(xσ)≤M,∀σ∈ED,∀n=1,⋯,N. $ | (3.12) |
Then the scheme (3.4) admits at least one solution that satisfies:
$ m≤θnK≤M,∀K∈T,∀n=1,⋯,N. $ | (3.13) |
Proof. We start by studying the following intermediate scheme:
$ mKθn+1K−θnKΔt+∑σ∈EKvnK,σθn+1σ,++2λmKJK(θn+1h)+λ∑σ∈EK˜Fn+1K,σ=0for all K∈T, $ | (3.14) |
with the modified numerical flux
$ ˜Fn+1K,σ=τσ˜θn+1σ(θn+1K−θn+1K,σ), $ | (3.15) |
and
$ ˜θn+1σ=max(0,θn+1σ,θn+1σ−(min(θn+1K,θn+1K,σ)−m)),for σ∈EK. $ | (3.16) |
We underline that the definition (3.16) ensures that $ \tilde{ \theta}_\sigma^{n+1} \geq 0 $. With this choice in the scheme (3.14), the diffusion term has been a little "inflated" in order to compensate the Joule term and to increase the stability, as it will be seen later. Note moreover that if $ \theta_h^{n+1} $ is solution of (3.14) and satisfies (3.13), it is also solution of (3.4).
The schedule of proof is as follows. We first show in Lemma 3.2 that a solution of (3.14) satisfies the discrete maximum principle. Then, by an argument of topological degree, we prove the existence of a solution to (3.14) (see Lemma 3.3). As this solution satisfies the maximum principle, it is also a solution of (3.4) and this concludes the proof of Theorem 3.1.
Lemma 3.2. We assume that (3.11) and (3.12) are satisfied. Let $ \theta_h^{n+1} $ be a solution of (3.14). Then $ \theta_h^{n+1} $ satisfies (3.13).
Proof. For $ n = 0 $, the property (3.13) clearly holds because of (3.11). Suppose now that (3.13) holds at step $ n $ and assume that there exists $ K_M \in \mathcal{T} $ such that:
$ θn+1KM=maxK∈Tθn+1K>M. $ |
We write (3.14) for the control volume $ K_M $ and obtain:
$ ∑σ∈EKMvnKM,σθn+1σ,++λ∑σ∈EKMτσ(θn+1KM,σ−θn+1KM)2+λ∑σ∈EKMτσ˜θn+1σ(θn+1KM−θn+1KM,σ)=mKMθnKM−θn+1KMΔt. $ | (3.17) |
The right hand side of (3.17) is negative, whereas the left hand side is non-negative (indeed, the treatment of the first term is classical, see for instance [25], and the sign of other terms is obvious). We thus obtain a contradiction.
Assume now that there exists $ K_m \in \mathcal{T} $ such that:
$ θn+1Km=minK∈Tθn+1K<m. $ | (3.18) |
We write (3.14) on $ K_m $:
$ ∑σ∈EKmvnKm,σθn+1σ,++λ∑σ∈EKmτσ(θn+1Km,σ−θn+1Km)2+λ∑σ∈EKmτσ˜θn+1σ(θn+1Km−θn+1Km,σ)=mKmθnKm−θn+1KmΔt. $ | (3.19) |
The right hand side of (3.19) is positive. Looking now at the left hand side, we notice that the first (see [25]) and third terms are non positive, whereas the second is non negative. Thus, to obtain a contradiction, we must reach a balance between the Joule term and the diffusive one. By noticing that:
$ ∑σ∈EKmτσ(θn+1Km,σ−θn+1Km)2+∑σ∈EKmτσ˜θn+1σ(θn+1Km−θn+1Km,σ)=∑σ∈EKmτσ(θn+1Km−θn+1Km,σ+˜θn+1σ)(θn+1Km−θn+1Km,σ), $ |
a sufficient condition to obtain the contradiction is to show that for all $ \sigma \in \mathcal{E}_K $, $ \theta_{K_m}^{n+1}- \theta_{K_m, \sigma}^{n+1}+\tilde{ \theta}_\sigma^{n+1}\geq 0 $. Indeed it holds, because of (3.18) and Definition (3.16) of $ \tilde{ \theta}_\sigma^{n+1} $, we have:
$ θn+1Km−θn+1Km,σ+˜θn+1σ=θn+1Km−θn+1Km,σ+θn+1Km,σ−(θn+1Km−m)=m≥0. $ | (3.20) |
Lemma 3.3. We assume that (3.11) and (3.12) are satisfied. Then the scheme (3.14) admits at least one solution.
Proof. Lemma 3.3 is proved thanks to a topological degree argument (see for instance [25]). Let $ \mu \in [0, 1] $, we define $ \theta_{h, \mu}^{n+1} = (\theta_{K, \mu}^{n+1})_{K\in \mathcal{T}} $ as the solution of the scheme: $ \forall K\in \mathcal{T} $,
$ mKθn+1K,μ−θnKΔt+∑σ∈EKvnK,σθn+1σ,+,μ+2μλmKJK(θn+1h)+μλ∑σ∈EK˜Fn+1K,σ,μ+2(1−μ)λmK¯JK(θn+1h,μ)+(1−μ)λ∑σ∈EK¯Fn+1K,σ,μ=0, $ | (3.21) |
where $ \theta_{\sigma, +, \mu}^{n+1} $ and $ \tilde{F}_{K, \sigma, \mu}^{n+1} $ are respectively defined by (3.6) and (3.15) by replacing $ \theta^{n+1}_K $ and $ \theta^{n+1}_L $ by $ \theta_{K, \mu}^{n+1} $ and $ \theta_{L, \mu}^{n+1} $; also $ \overline{F}_{K, \sigma, \mu}^{n+1} $ is defined by (3.22) and $ \overline{ \mathcal{J}}_{K}(\theta_{h, \mu}^{n+1}) $ by (3.23):
$ ¯Fn+1K,σ,μ=τσM(θn+1K,μ−θn+1K,σ,μ). $ | (3.22) |
$ ¯JK(θn+1h,μ)=12mK(M−m)∑σ∈EKτσ(θn+1K,σ,μ−θn+1K,μ). $ | (3.23) |
Then (3.21) is equivalent to:
$ mKθn+1K,μ−θnKΔt+∑σ∈EKvnK,σθn+1σ,+,μ+2μλmKJK(θn+1h,μ)+μλ∑σ∈EK˜Fn+1K,σ,μ+(1−μ)λm∑σ∈EKτσ(θn+1K,μ−θn+1K,σ,μ)=0, $ |
and consequently, we can show as in the proof of Lemma 3.2 that for all $ K\in \mathcal{T} $,
$ m≤θn+1K,μ≤M. $ | (3.24) |
We now define $ \mathcal{K} = [m-1;M+1]^{\# \mathcal{T}} $ a compact of $ \mathbb{R}^{\# \mathcal{T}} $, and $ \mathcal{H}\, :\, [0, 1]\times \mathbb{R}^{\# \mathcal{T}} \longrightarrow \mathbb{R}^{\# \mathcal{T}} $ by: $ \forall K \in \mathcal{T} $,
$ HK(μ,(θn+1K)K∈T)=mKθn+1K,μ−θnKΔt+∑σ∈EKvnK,σθn+1σ,+,μ+2μλmKJK(θn+1h)+μλ∑σ∈EK˜Fn+1K,σ,μ+2(1−μ)λmK¯JK(θn+1h,μ)+(1−μ)λ∑σ∈EK¯Fn+1K,σ,μ. $ |
Then, $ \mathcal{H} \in \mathcal{C}([0, 1]\times \mathcal{K}, \mathbb{R}^{\# \mathcal{T}}) $ and thanks to (3.24),
$ HK(μ,(θn+1K)K∈T)=0 $ |
admits no solution on $ \partial \mathcal{K} $. Consequently, its topological degree is independent of $ \mu $. As (3.21) admits a solution for $ \mu = 0 $ its topological degree is different from zero. We can now conclude that (3.21) has a solution for $ \mu = 1 $, and therefore (3.14) has at least one solution.
Lemma 3.2 and Lemma 3.3 conclude the proof of Theorem 3.1 since as already mentioned, if $ \theta_h^{n+1} $ is solution of (3.14) and satisfies (3.13), it is also solution of (3.4).
In this subsection, several variants of the scheme $ (3.4) $, denoted in the following $ (\mathcal{S} \mathcal{D}_\mathrm{max} \mathcal{J}_\mathrm{cen}) $, are presented. The scheme $ (\mathcal{S} \mathcal{D}_\mathrm{moy} \mathcal{J}_\mathrm{up}) $ (subsection 3.3.1) will also fulfill the discrete maximum principle without any condition, whereas the scheme $ (\mathcal{S} \mathcal{D}_\mathrm{moy} \mathcal{J}_\mathrm{cen}) $ (subsection 3.3.2) as well as $ (\mathcal{S} \mathcal{D}_\mathrm{max} \mathcal{J}_\mathrm{EGH}) $ and $ (\mathcal{S} \mathcal{D}_\mathrm{moy} \mathcal{J}_\mathrm{EGH}) $ (subsection 3.3.3) will fulfill the discrete maximum principle under some restrictions on the temperature bounds $ m $ and $ M $.
We propose here a first variant of $ (\mathcal{S} \mathcal{D}_\mathrm{max} \mathcal{J}_\mathrm{cen}) $, which also leads to an unconditionally maximum principle. The idea is to consider the scheme (3.4), but with two differences compared to $ (\mathcal{S} \mathcal{D}_\mathrm{max} \mathcal{J}_\mathrm{cen}) $. On the one hand, the diffusion term is centered, by defining now $ \theta_\sigma^{n+1} $ used in the definition of the diffusive flux $ F_{K, \sigma}^{n+1} $ in (3.7) by:
$ θn+1σ=θn+1K+θn+1K,σ2 $ | (3.25) |
instead of (3.8). On the other hand, an upwind in the Joule term gives us
$ JK(θn+1h)=1mK∑σ∈EKτσ((θn+1K−θn+1K,σ)+)2 $ | (3.26) |
instead of (3.10), where we use the notation $ a^+ = \max (0, a) $. In that case, the maximum principle occurs. Indeed, the proof of Theorem 3.1 can easily be adapted by noticing that $ \left((\theta_K^{n+1}- \theta_{K, \sigma}^{n+1})^+\right)^2 = 0 $ if $ \theta_K^{n+1}\leq \theta_{K, \sigma}^{n+1} $. Let us note that the combination of (3.8) for the diffusion flux definition associated to (3.26) for the Joule term flux definition would also lead to a scheme with an unconditional maximum principle. Nevertheless, this choice would generate a loss of accuracy compared with $ (\mathcal{S} \mathcal{D}_\mathrm{max} \mathcal{J}_\mathrm{cen}) $ and $ (\mathcal{S} \mathcal{D}_\mathrm{moy} \mathcal{J}_\mathrm{up}) $ which are both already unconditionally maximum-principle preserving. Since it is useless to upwind both the diffusion term and the Joule one, we did not considered it.
Remark 3.4. Note that we could also have considered the following definition in the diffusion term:
$ θn+1σ={dL,σθn+1K+dK,σθn+1Ldσ, for σ=K|L,θn+1K+θn+1D,σ2, for σ∈EDK. $ | (3.27) |
From the theoretical and numerical points of view, the cases (3.25) and (3.27) give similar results.
A quite natural question, in order to increase the accuracy of the approximation, is to investigate the behaviour of the scheme when both flux are centered. Namely, it would consist in considering (3.25) for the definition of the diffusive flux $ F_{K, \sigma}^{n+1} $ in (3.7), while using (3.10) for the Joule term flux definition. In that case, the upper bound can be obtained in the same way as in Lemma 3.2. Nevertheless, the obtention of the lower bound needs an additional assumption, so that the maximum principle can be ensured by a specific balance between the Joule term and the diffusion one. The definition of $ \tilde{ \theta}_\sigma^{n+1} $ in (3.16) has to be a little modified and given by :
$ \tilde{ \theta}_\sigma^{n+1} = \max \left(0, \theta_\sigma^{n+1}, \theta_\sigma^{n+1}-\, \frac32 \left(\min( \theta_K^{n+1}, \theta_{K, \sigma}^{n+1})-m\right)\right) \quad \text{for }\sigma \in \mathcal{E}_K, $ |
so that the positivity of $ \theta_{K_m}^{n+1}- \theta_{K_m, \sigma}^{n+1}+\tilde{ \theta}_\sigma^{n+1} $ (see (3.20)) is ensured provided that $ M \leq 3 m $. As it will be illustrated in the numerical test 4.1 below, such a condition is necessary. This can seem strange from the physical point of view. As we see in the proof, it is necessary for technical reasons, but could also be linked to the fact that the global solution in time of the continuous model is ensured if the initial datum is not too far from a constant state (see (2.3)). Anyway, even with this restriction in mind, $ (\mathcal{S} \mathcal{D}_\mathrm{moy} \mathcal{J}_\mathrm{cen}) $ could be used for cases where temperature variations are low, to expect a better accuracy of the solution compared to the one obtained by $ (\mathcal{S} \mathcal{D}_\mathrm{max} \mathcal{J}_\mathrm{cen}) $ or $ (\mathcal{S} \mathcal{D}_\mathrm{moy} \mathcal{J}_\mathrm{up}) $.
Finally, two other schemes are investigated, considering another way to define the piecewise discrete gradient in each control volume given by (see [26]) :
$ JK(θn+1h)=(1mK∑σ∈EKτσ(θn+1K−θn+1K,σ)(xσ−xK))2, $ | (3.28) |
while considering either (3.8) or (3.25) for the computation of $ \theta_\sigma^{n+1} $ arising in the definition of $ F_{K, \sigma}^{n+1} $ given by (3.7), and leading respectively to the schemes denoted $ (\mathcal{S} \mathcal{D}_\mathrm{max} \mathcal{J}_\mathrm{EGH}) $ and $ (\mathcal{S} \mathcal{D}_\mathrm{moy} \mathcal{J}_\mathrm{EGH}) $. Once again and in both cases, the maximum principle is ensured under a condition $ M \leq C(\mathcal{T}) \, m $ with $ C(\mathcal{T}) \in (1, 2) $, depending on geometrical characteristics of the mesh (see [17]).
In Section 4, we implement these different schemes and compare them on two main criteria: verification of the maximum principle and convergence rates. Table 1 summarizes the five considered schemes.
Diffusion term $ \theta_\sigma^{n+1}= $ |
|||
$ \dfrac{ \theta_K^{n+1}+ \theta_{K, \sigma}^{n+1}}{2} $ | $ \max(\theta_K^{n+1}, \theta_{K, \sigma}^{n+1}) $ | ||
Joule Term | $ \dfrac{1}{2\, m_K}\sum\limits_{\sigma \in \mathcal{E}_K}^{} \tau_\sigma (\theta_{K}^{n+1}- \theta_{K, \sigma}^{n+1})^2 $ | $ (\mathcal{S} \mathcal{D}_\mathrm{moy} \mathcal{J}_\mathrm{cen}) $ | $ (\mathcal{S} \mathcal{D}_\mathrm{max} \mathcal{J}_\mathrm{cen}) $ |
$ \mathcal{J}_K(\theta_ \mathcal{T}^{n+1})= $ | $ \dfrac{1}{m_K}\sum\limits_{\sigma \in \mathcal{E}_K}^{} \tau_\sigma \left((\theta_{K}^{n+1}- \theta_{K, \sigma}^{n+1})^+\right)^2 $ | $ (\mathcal{S} \mathcal{D}_\mathrm{moy} \mathcal{J}_\mathrm{up}) $ | $\Huge{ \times} $ |
$ \left(\dfrac{1}{m_K}\sum_{\sigma \in \mathcal{E}_K}^{} \tau_\sigma (\theta_K^{n+1}- \theta_{K, \sigma}^{n+1}) (\textbf{x}_\sigma- \textbf{x}_K)\right)^2 $ | $ (\mathcal{S} \mathcal{D}_\mathrm{moy} \mathcal{J}_\mathrm{EGH}) $ | $ (\mathcal{S} \mathcal{D}_\mathrm{max} \mathcal{J}_\mathrm{EGH}) $ |
The resolution by a finite element method of (3.2) gives us the velocity field $ \mathbf{v}_h^{n+1} $ which is $ \mathbb{P} _2 $ on each triangle $ K\in \mathcal{T} $. Let us denote by $ (\mathbf{v}_\sigma^{n+1})_{\sigma \in \mathcal{E}} $ the value of this velocity field at the center of edges. Thus, the local divergence constraint reads:
$ ∑σ∈EKmσvn+1σ⋅nK,σ=αK,∀K∈T. $ | (3.29) |
The sequence of reals $ (\alpha_K)_{K\in \mathcal{T}} $ is different from zero in general. Consequently, the velocity field $ (\mathbf{v}_\sigma^{n+1})_{\sigma \in \mathcal{E}} $ is not divergence-free in the Finite Volume sense, and can not be used for the resolution of the temperature equation. Here we can not follow the idea of [9], adapted in [7] for the projection method, which consists in defining a constant velocity per triangle. Indeed, the unknowns for the temperature are located at the center of the cells, and no more at the vertices of the mesh.
For $ \sigma \in \mathcal{E}^{\mathrm{int}} $, we define arbitrarly $ K_\sigma^+ $ and $ K_\sigma^- $ the two triangles such that $ \sigma = K_\sigma^+ |K_\sigma^- $. For $ \sigma \in \mathcal{E}^{\mathrm{ext}} $, we denote by $ K_\sigma^+ $ the triangle such that $ \sigma \subset \partial K_\sigma^+ $. Let $ \mathbf{n}_\sigma $ the unit normal vector to $ \sigma $ getting out of $ K_\sigma^+ $, see Figure 2.
We also define $ \forall K \in \mathcal{T} $ and $ \forall \sigma \in \mathcal{E}_K $:
$ εK,σ={1if K=K+σ,−1if K=K−σ. $ |
By denoting $ (f_\sigma) \in \mathbb{R}^{\# \mathcal{E}} $ the global numerical flux defined by:
$ fσ=mσvn+1σ⋅nσ,∀σ∈E, $ |
equation (3.29) can be written as:
$ ∑σ∈EKεK,σfσ=αK,∀K∈T. $ | (3.30) |
We now approximate $ (f_\sigma)_{\sigma \in \mathcal{E}} $ by $ (\tilde{f}_\sigma)_{\sigma \in \mathcal{E}} $ in order to obtain the local divergence-free constraint
$ ∑σ∈EKεK,σ˜fσ=0,∀K∈T. $ | (3.31) |
Then the fluxes $ (\tilde{f}_\sigma)_{\sigma \in \mathcal{E}} $ are used in the cell-centered Finite Volume scheme (3.4) for the computation of the temperature field, by setting:
$ v_{K, \sigma}^{n+1} = \varepsilon_{K, \sigma} \, \tilde{f}_\sigma. $ |
Practically, $ (\tilde{f}_\sigma)_{\sigma \in \mathcal{E}} $ is computed as an approximation of $ ({f}_\sigma)_{\sigma \in \mathcal{E}} $ in the discrete least-mean-squares sense, which fulfills the divergence-free constraint on each finite volume control. It consists in solving the global optimization problem given by:
$ (˜fσ)=argmin(˜hσ)∈R#E{12∑σ∈Eintwσ(fσ−˜hσ)2;∀K∈T,∑σ∈EKεK,σ˜hσ=0}, $ | (3.32) |
where $ (w_\sigma)_{\sigma\in \mathcal{E}} $ is a sequence of strictly positive weights, which can be defined for example by $ w_\sigma = 1 $ or $ w_\sigma = \frac{m_\sigma}{d_\sigma} $, $ \forall \sigma \in \mathcal{E} $. Numerically, we observed similar behaviors for both possibilities. The solution of the problem (3.32) is given by the following theorem:
Theorem 3.5. Let $ \mathbf{M} \in \mathbb{R}^{\# \mathcal{T} \times \# \mathcal{T}} $ the M-matrix defined $ \forall K, L\in \mathcal{T} $ by:
$ \begin{array}{l} \mathbf{M}_{KL} = \left\{ \begin{array}{ll} \sum\limits_{\sigma \in \mathcal{E}_K} \dfrac{1}{w_\sigma} & \mathit{\text{if}}~~ K = L, \\[0.2cm] -\dfrac{1}{w_\sigma} & \mathit{\text{si}}~~ \overline{K}\cap \overline{L} = \sigma, \\ 0 & \mathit{\text{otherwise, }}~~ \end{array} \right. \end{array} $ |
and $ A = (\alpha_K)_{K\in \mathcal{T}} \in \mathbb{R}^{\# \mathcal{T}} $. Let $ \Lambda = (\lambda_K)_{K\in \mathcal{T}} \in \mathbb{R}^{\# \mathcal{T}} $ be the solution of:
$ MΛ=A. $ | (3.33) |
Thus the solution of (3.32) can be defined $ \forall \sigma \in \mathcal{E} $ by:
$ ˜fσ=fσ−1wσ(λK+σ−λK−σ), $ | (3.34) |
where for all $ \sigma \in \mathcal{E}^{\mathrm{ext}} $ such that $ \sigma \subset \partial K $, we set $ \lambda_{K_\sigma^-} = 0 $.
Proof. With the following change of variables:
$ gσ=˜fσ−fσ, $ | (3.35) |
problem (3.32) writes:
$ (gσ)=argmin(hσ)∈R#E{12∑σ∈Ewσh2σ;∀K∈T,∑σ∈EKεK,σhσ+αK=0}. $ | (3.36) |
We define the lagrangian associated with (3.36) for $ (h_\sigma) \in \mathbb{R}^{\# \mathcal{E}} $ and $ (\mu_K) \in \mathbb{R}^{\# \mathcal{T}} $ by:
$ L((hσ),(μK))=12∑σ∈Ewσh2σ+∑K∈TμK(∑σ∈EKεK,σhσ+αK). $ |
We will show the existence of a saddle point of $ \mathcal{L} $. Its first argument will therefore be the solution of the problem (3.36), while the second one corresponds to the Lagrange multiplier associated with the constraints, see for example [16]. We recall that if $ \left((g_\sigma), (\lambda_K)\right) $ is a saddle point of $ \mathcal{L} $, then:
$ sup(μK)inf(hσ)L((hσ),(μK))=L((gσ),(λK))=inf(hσ)sup(μK)L((hσ),(μK)). $ |
We start with computing $ \mathcal{H}\left((\mu_K)\right) = \inf_{(h_\sigma)} \mathcal{L}\left((h_\sigma), (\mu _K)\right) $. We easily verify that $ (g_\sigma) $ (which depends on $ (\mu_K) $) defined by:
$ gσ=−1wσ(μK+σ−μK−σ),∀σ∈E $ | (3.37) |
is solution of
$ ∂L∂hσ((gσ),(μK))=0. $ |
We thus obtain:
$ H((μK))=12∑σ∈E1wσ(μK+σ−μK−σ)2+∑K∈TμK(∑σ∈EK−εK,σwσ(μK+σ−μK−σ)+αK)=−12∑σ∈E1wσ(μK+σ−μK−σ)2+∑K∈TμKαK=−12∑σ∈E1wσ(μK−μK,σ)2+∑K∈TμKαK, $ |
with the notation
$ μK,σ={μLif σ∈EintK,σ=K|L,0if σ∈EextK. $ | (3.38) |
We now compute $ (\lambda_K) = \underset{({\mu_K})}{\mathrm{argmax}} \ \mathcal{H}\left((\mu_K)\right) $ by solving:
$ ∂(−H)∂μK((λK))=0,∀K∈T, $ |
which is equivalent, applying the notation (3.38) to $ \lambda_{K, \sigma} $, to
$ ∑σ∈EK1wσ(λK−λK,σ)−αK=0. $ |
Thus, $ (\lambda_K) $ is obtained by solving the linear system (3.33). We therefore deduce the expression of $ (g_\sigma) $ thanks to (3.37) and then $ (\tilde{f}_\sigma) $ with (3.35).
We previously proved that the schemes $ (\mathcal{S} \mathcal{D}_\mathrm{max} \mathcal{J}_\mathrm{cen}) $ and $ (\mathcal{S} \mathcal{D}_\mathrm{moy} \mathcal{J}_\mathrm{up}) $ satisfy the maximum principle, without any restriction on $ m $ and $ M $. This first experiment illustrates that if $ M $ is too large compared to $ m $, the other schemes do not respect the maximum principle. In this perspective, we consider only the temperature equation (2.2a) and set:
$ v(x,t)=0,∀x∈Ω,∀t∈[0,T]. $ |
The initial temperature is defined on $ \Omega = [0;1]^2 $ by:
$ θ0(x)=1,∀x∈Ω. $ |
On all the boundaries, we impose Dirichlet conditions, see Figure 3. We denote by $ \Gamma_H = \{ 1\}\times (0, 3; 0, 7) $ and set $ \forall t \in [0;T] $:
$ θD(x,t)={1if x∈Γ∖ΓHMif x∈ΓH. $ |
We run the simulations for different values of $ M $ on a triangulation $ \mathcal{T} $ with mesh size $ h = 7.25 \cdot 10^{-2} $ and report the results in the Table 2. We find numerically the results shown previously. On the one hand, the schemes $ (\mathcal{S} \mathcal{D}_\mathrm{max} \mathcal{J}_\mathrm{cen}) $ and $ (\mathcal{S} \mathcal{D}_\mathrm{moy} \mathcal{J}_\mathrm{up}) $ allow us to obtain the maximum principle whatever the value of $ M $. On the other hand, the schemes $ (\mathcal{S} \mathcal{D}_\mathrm{moy} \mathcal{J}_\mathrm{cen}) $, $ (\mathcal{S} \mathcal{D}_\mathrm{moy} \mathcal{J}_\mathrm{EGH}) $ and $ (\mathcal{S} \mathcal{D}_\mathrm{max} \mathcal{J}_\mathrm{EGH}) $ do not give a solution that satisfies the maximum principle if $ M $ is too large.
![]() |
$ (\mathcal{S} \mathcal{D}_\mathrm{moy} \mathcal{J}_\mathrm{EGH}) $ | $ (\mathcal{S} \mathcal{D}_\mathrm{max} \mathcal{J}_\mathrm{EGH}) $ | $ (\mathcal{S} \mathcal{D}_\mathrm{moy} \mathcal{J}_\mathrm{cen}) $ | $ (\mathcal{S} \mathcal{D}_\mathrm{max} \mathcal{J}_\mathrm{cen}) $ | $ (\mathcal{S} \mathcal{D}_\mathrm{moy} \mathcal{J}_\mathrm{up}) $ |
2 | $ \checkmark $ | $ \checkmark $ | $ \checkmark $ | $ \checkmark $ | $ \checkmark $ |
3 | $ {\LARGE \times} $ | $ \checkmark $ | $ \checkmark $ | $ \checkmark $ | $ \checkmark $ |
4 | $ {\LARGE \times} $ | $ \checkmark $ | $ {\LARGE \times} $ | $ \checkmark $ | $ \checkmark $ |
10 | $ {\LARGE \times} $ | $ {\LARGE \times} $ | $ {\LARGE \times} $ | $ \checkmark $ | $ \checkmark $ |
100 | $ {\LARGE \times} $ | $ {\LARGE \times} $ | $ {\LARGE \times} $ | $ \checkmark $ | $ \checkmark $ |
In this benchmark, we want to investigate the accuracy of the scheme described in section 3.2, depending on the discretization of the diffusive and Joule terms. We consider the domain $ \Omega = [0;1]^2 $. The simulations will be performed until the time $ T = 0.1 $. The exact solution is defined for $ (x, y, t)\in \Omega \times [0;T] $ by:
$ θex(x,y,t)=1+t10λπ2(1,5+cosπx)(1,5+cosπy), $ |
with $ \lambda = 2 $. In (3.3), a source term is added accordingly. For the velocity, two cases are considered:
a) $ \mathbf{v} = \mathbf{0} $,
b) $ \mathbf{v} $ defined by
$ v(x,y)=1λπ2(−sin(πy)1,5+cos(πy)sin(πx)1,5+cos(πx)). $ | (4.1) |
Here, Dirichlet conditions are imposed on all the boundaries, so that $ \Gamma_D = \Gamma $. The temperature error in $ L^\infty (0, T; L^2 (\Omega)) $ is plotted in Figure 4 as a function of the mesh size $ h $, in log-log scale, for each scheme. We notice that without the convective term (case a)), the centered schemes $ (\mathcal{S} \mathcal{D}_\mathrm{moy} \mathcal{J}_\mathrm{cen}) $ and $ (\mathcal{S} \mathcal{D}_\mathrm{moy} \mathcal{J}_\mathrm{EGH}) $ converge at order two, whereas the others are only at order one. Thus, the upwind choice in the diffusion term (schemes $ (\mathcal{S} \mathcal{D}_\mathrm{max} \mathcal{J}_\mathrm{cen}) $ and $ (\mathcal{S} \mathcal{D}_\mathrm{max} \mathcal{J}_\mathrm{EGH}) $) or in the Joule term (scheme $ (\mathcal{S} \mathcal{D}_\mathrm{moy} \mathcal{J}_\mathrm{up}) $ degrades the rate of convergence to order one, but is necessary to obtain the maximum principle. Adding the convective term (case b)), the centered schemes $ (\mathcal{S} \mathcal{D}_\mathrm{moy} \mathcal{J}_\mathrm{cen}) $ and $ (\mathcal{S} \mathcal{D}_\mathrm{moy} \mathcal{J}_\mathrm{EGH}) $ converge at order 3/2. This decrease of convergence rate can be explained by the upwind treatment of the convective term in order to ensure the stability.
We will now validate the scheme $ (\mathcal{S} \mathcal{D}_\mathrm{max} \mathcal{J}_\mathrm{cen}) $ by coupling the temperature equation with the velocity one on the benchmark introduced in [1,15,29,31] and used in [7] (slightly adapted because of the choice of the model (2.2)). We consider a square cavity $ \Omega = [0, 1]^2 $ containing a calorifically perfect gas, see Figure 5. The gas is initially at rest with uniform temperature:
$ u0=0 and θ0=0.5. $ |
Note that the temperature has been scaled to be between 0 and 1. A temperature of $ \theta_h = \theta_0 (1+\varepsilon) $ (respectively $ \theta_c = \theta_0(1-\varepsilon) $) is imposed on the left (respectively right) wall with $ \varepsilon = 0.01 $ as in [29]. For this small temperature amplitude, the thermodynamic pressure can be approximated by a constant, where the author verifies numerically that $ P(T) = P_0 $ with an accuracy of $ 10^{-5} $). The horizontal walls are insulated. Denoting by $ \Gamma_N = [0, 1] \times \{0, 1\} $, we thus have:
$ ∇θ(⋅,t)⋅n|ΓN=0,∀t∈[0;T]. $ |
On all walls, the no-slip condition is imposed for the physical velocity $ \mathbf{u} $, which gives for the solenoidal one:
$ v(x,t)=0,∀x∈ΓN,∀t∈[0;T],v(x,t)=λ∇θ(x,t),∀x∈ΓD,∀t∈[0;T]. $ |
We set $ \lambda = 4, 76 \cdot 10^{-2} $, which corresponds to a Rayleigh number of $ 10^{-5} $, as it is the case in [31].
The time iterations are performed until the numerical steady state is reached, i.e. the relative errors for the solenoidal velocity and the temperature are less than $ 10^{-10} $. The steady state is obtained approximatively at $ T = 100 $, using for instance a mesh $ \mathcal T $ composed by 3584 triangles (corresponding to $ h = 1.8 \cdot10^{-2} $) and a time step $ \Delta t $ of the order of $ 10^{-2} $. We represent in Figure 6 the streamlines of the velocity field and the contour lines of the temperature at steady state. The solutions obtained are close to the ones given in Figures 4 and 5 of [29]. We also verify that the maximum principle for the temperature is always respected.
Note that even though we have to solve a global optimization problem in order to obtain the fluxes used in the finite volume scheme, its cost is negligible. Indeed, the matrix of the linear system (3.33) is assembled a single time before the time loop. On the contrary, some matrices in the finite element step must be assembled at each time step. Note moreover that the cost of Newton's iterations for the temperature equation resolution is also negligible. Indeed, only two or three iterations are necessary in order to obtain the solution with an accuracy of $ 10^{-5} $. With this choice, approximatively only 10% of the overall computation time is devoted to the resolution of the temperature equation. This ratio in the computation cost is quite the same when we consider more coarse or more fine meshes.
In this work we propose some finite volume schemes for the resolution of an unsteady convection-diffusion equation involving a Joule term. Several variants are investigated, depending on the way to discretize the diffusion term as well as the Joule one. A first main point is that two of these schemes verify the discrete maximum principle without any restriction on the data. Such schemes allow us to define a combined finite volume - finite element scheme for a low-Mach system, where the temperature is computed by the finite volume scheme whereas the velocity and pressure are approximated by a finite element one. The second point is that the finite volume velocity field, used for the convective term in the temperature equation, is computed from the finite element one by the resolution of a discrete least-mean-squares problem to ensure the local free divergence constraint. The numerical results illustrate the properties of the different schemes and confirm the relevance of the approach. The question of the convergence remains to be investigated, and will be addressed in a future work.
This work was supported in part by the Labex CEMPI (ANR-11-LABX-0007-01). The authors gratefully acknowledge Clément Cancès, Claire Chainais-Hillairet and Benoit Merlet for fruitful discussions about it.
The authors declare no conflicts of interest in this paper.
[1] |
Dupont C, Armant DR, Brenner CA (2009) Epigenetics: Definition, mechanisms and clinical perspective. Semin Reprod Med 27: 351-357. doi: 10.1055/s-0029-1237423
![]() |
[2] |
Dawson MA, Kouzarides T (2012) Cancer epigenetics: From mechanism to therapy. Cell 150: 12-27. doi: 10.1016/j.cell.2012.06.013
![]() |
[3] |
Esteller M (2008) Molecular origins of cancer: Epigenetics in cancer. New Engl J Med 358: 1148-1159+1096. doi: 10.1056/NEJMra072067
![]() |
[4] |
Laird PW (2005) Cancer epigenetics. Hum Mol Genet 14: R65-R76. doi: 10.1093/hmg/ddi113
![]() |
[5] |
Okugawa Y, Grady WM, Goel A (2015) Epigenetic Alterations in Colorectal Cancer: Emerging Biomarkers. Gastroenterology 149: 1204-1225e1212. doi: 10.1053/j.gastro.2015.07.011
![]() |
[6] | Deng D, Liu Z, Du Y (2010) Epigenetic Alterations as Cancer Diagnostic, Prognostic, and Predictive Biomarkers. Adv Genet 71: 126-176. |
[7] |
Herceg Z, Hainaut P (2007) Genetic and epigenetic alterations as biomarkers for cancer detection, diagnosis and prognosis. Mol Oncol 1: 26-41. doi: 10.1016/j.molonc.2007.01.004
![]() |
[8] |
Dekker C (2007) Solid-state nanopores. Nat Nanotechnol 2: 209-215. doi: 10.1038/nnano.2007.27
![]() |
[9] |
Keyser UF (2011) Controlling molecular transport through nanopores. J R Soc Interface 8: 1369-1378. doi: 10.1098/rsif.2011.0222
![]() |
[10] |
Siwy ZS, Howorka S (2010) Engineered voltage-responsive nanopores. Chem Soc Rev 39: 1115-1132. doi: 10.1039/B909105J
![]() |
[11] |
Garaj S, Hubbard W, Reina A, et al. (2010) Graphene as a subnanometre trans-electrode membrane. Nature 467: 190-193. doi: 10.1038/nature09379
![]() |
[12] |
Siwy ZS, Davenport M (2010) Nanopores: Graphene opens up to DNA. Nat Nanotechnol 5: 697-698. doi: 10.1038/nnano.2010.198
![]() |
[13] |
Tada K, Haruyama J, Yang HX, et al. (2011) Graphene magnet realized by hydrogenated graphene nanopore arrays. Appl Phys Lett 99: 183111. doi: 10.1063/1.3653286
![]() |
[14] |
Ding S, Gao C, Gu LQ (2009) Capturing single molecules of immunoglobulin and ricin with an aptamer-encoded glass nanopore. Anal Chem 81: 6649-6655. doi: 10.1021/ac9006705
![]() |
[15] |
Gu LQ, Shim JW (2010) Single molecule sensing by nanopores and nanopore devices. Analyst 135: 441-451. doi: 10.1039/B907735A
![]() |
[16] | Fertig N, Meyer C, Blick RH, et al. (2001) Microstructured glass chip for ion-channel electrophysiology. Phys Rev E Stat Nonlin Soft Matter Phys 64: 409011-409014. |
[17] |
Li J, Stein D, McMullan C, et al. (2001) Ion-beam sculpting at nanometre length scales. Nature 412: 166-169. doi: 10.1038/35084037
![]() |
[18] |
Storm AJ, Chen JH, Ling XS, et al. (2003) Fabrication of solid-state nanopores with single-nanometre precision. Nat Mater 2: 537-540. doi: 10.1038/nmat941
![]() |
[19] |
Kasianowicz JJ, Brandin E, Branton D, et al. (1996) Characterization of individual polynucleotide molecules using a membrane channel. Proc Natl Acad Sci U S A 93: 13770-13773. doi: 10.1073/pnas.93.24.13770
![]() |
[20] |
Laszlo AH, Derrington IM, Brinkerhoff H, et al. (2013) Detection and mapping of 5-methylcytosine and 5-hydroxymethylcytosine with nanopore MspA. Proc Natl Acad Sci U S A 110: 18904-18909. doi: 10.1073/pnas.1310240110
![]() |
[21] | Laszlo AH, Derrington IM, Ross BC, et al. (2014) Decoding long nanopore sequencing reads of natural DNA. Nat Biotechnol 32: 829-833. |
[22] |
Wang Y, Montana V, Grubišić V, et al. (2015) Nanopore sensing of botulinum toxin type B by discriminating an enzymatically cleaved peptide from a synaptic protein synaptobrevin 2 derivative. ACS Appl Mater Inter 7: 184-192. doi: 10.1021/am5056596
![]() |
[23] |
Liu J, Eren E, Vijayaraghavan J, et al. (2012) OccK channels from Pseudomonas aeruginosa exhibit diverse single-channel electrical signatures but conserved anion selectivity. Biochemistry 51: 2319-2330. doi: 10.1021/bi300066w
![]() |
[24] |
Mohammad MM, Iyer R, Howard KR, et al. (2012) Engineering a rigid protein tunnel for biomolecular detection. J Am Chem Soc 134: 9521-9531. doi: 10.1021/ja3043646
![]() |
[25] |
Cheneke BR, van den Berg B, Movileanu L (2015) Quasithermodynamic contributions to the fluctuations of a protein nanopore. ACS Chem Biol 10: 784-794. doi: 10.1021/cb5008025
![]() |
[26] |
Wang HY, Li Y, Qin LX, et al. (2013) Single-molecule DNA detection using a novel SP1 protein nanopore. Chem Commun (Camb) 49: 1741-1743. doi: 10.1039/c3cc38939a
![]() |
[27] |
Wang S, Haque F, Rychahou PG, et al. (2013) Engineered nanopore of phi29 dna-packaging motor for real-time detection of single colon cancer specific antibody in serum. ACS Nano 7: 9814-9822. doi: 10.1021/nn404435v
![]() |
[28] |
Wu D, Bi S, Zhang L, et al. (2014) Single-molecule study of proteins by biological nanopore sensors. Sensors (Basel) 14: 18211-18222. doi: 10.3390/s141018211
![]() |
[29] |
Venkatesan BM, Bashir R (2011) Nanopore sensors for nucleic acid analysis. Nat Nanotechnol 6: 615-624. doi: 10.1038/nnano.2011.129
![]() |
[30] |
Hall AR, Scott A, Rotem D, et al. (2010) Hybrid pore formation by directed insertion of +Ý-haemolysin into solid-state nanopores. Nat Nanotechnol 5: 874-877. doi: 10.1038/nnano.2010.237
![]() |
[31] |
Bell NA, Engst CR, Ablay M, et al. (2012) DNA origami nanopores. Nano Lett 12: 512-517. doi: 10.1021/nl204098n
![]() |
[32] |
Iqbal SM, Akin D, Bashir R (2007) Solid-state nanopore channels with DNA selectivity. Nat Nanotechnol 2: 243-248. doi: 10.1038/nnano.2007.78
![]() |
[33] |
Tinazli A, Tang J, Valiokas R, et al. (2005) High-affinity chelator thiols for switchable and oriented immobilization of histidine-tagged proteins: a generic platform for protein chip technologies. Chemistry 11: 5249-5259. doi: 10.1002/chem.200500154
![]() |
[34] |
Wei R, Pedone D, Zurner A, et al. (2010) Fabrication of metallized nanopores in silicon nitride membranes for single-molecule sensing. Small 6: 1406-1414. doi: 10.1002/smll.201000253
![]() |
[35] |
Yusko EC, Johnson JM, Majd S, et al. (2011) Controlling protein translocation through nanopores with bio-inspired fluid walls. Nat Nanotechnol 6: 253-260. doi: 10.1038/nnano.2011.12
![]() |
[36] | Wang D, Harrer S, Luan B, et al. (2014) Regulating the transport of DNA through Biofriendly Nanochannels in a thin solid membrane. Sci Rep 4: 3985. |
[37] | Branton D, Deamer DW, Marziali A, et al. (2008) The potential and challenges of nanopore sequencing. Nat Nanotechnol 26: 1146-1153. |
[38] |
Cherf GM, Lieberman KR, Rashid H, et al. (2012) Automated forward and reverse ratcheting of DNA in a nanopore at 5-A precision. Nat Biotechnol 30: 344-348. doi: 10.1038/nbt.2147
![]() |
[39] |
Kasianowicz JJ, Brandin E, Branton D, et al. (1996) Characterization of individual polynucleotide molecules using a membrane channel. Proc Natl Acad Sci U S A 93: 13770-13773. doi: 10.1073/pnas.93.24.13770
![]() |
[40] |
Manrao EA, Derrington IM, Laszlo AH, et al. (2012) Reading DNA at single-nucleotide resolution with a mutant MspA nanopore and phi29 DNA polymerase. Nat Biotechnol 30: 349-353. doi: 10.1038/nbt.2171
![]() |
[41] |
An N, Fleming AM, White HS, et al. (2012) Crown ether-electrolyte interactions permit nanopore detection of individual DNA abasic sites in single molecules. Proc Natl Acad Sci U S A 109: 11504-11509. doi: 10.1073/pnas.1201669109
![]() |
[42] |
Wallace EV, Stoddart D, Heron AJ, et al. (2010) Identification of epigenetic DNA modifications with a protein nanopore. Chem Commun(Camb) 46: 8195-8197. doi: 10.1039/c0cc02864a
![]() |
[43] |
Shim J, Kim Y, Humphreys GI, et al. (2015) Nanopore-based assay for detection of methylation in double-stranded DNA fragments. ACS Nano 9: 290-300. doi: 10.1021/nn5045596
![]() |
[44] |
Bayley H, Cremer PS (2001) Stochastic sensors inspired by biology. Nature 413: 226-230. doi: 10.1038/35093038
![]() |
[45] |
Gu LQ, Shim JW (2010) Single molecule sensing by nanopores and nanopore devices. Analyst 135: 441-451. doi: 10.1039/B907735A
![]() |
[46] |
Howorka S, Siwy Z (2009) Nanopore analytics: sensing of single molecules. Chem Soc Rev 38: 2360-2384. doi: 10.1039/b813796j
![]() |
[47] | Luan B, Peng H, Polonsky S, et al. (2010) Base-by-base ratcheting of single stranded DNA through a solid-state nanopore. Phys Rev Lett 104. |
[48] |
Asandei A, Chinappi M, Lee JK, et al. (2015) Placement of oppositely charged aminoacids at a polypeptide termini determines the voltage-controlled braking of polymer transport through nanometer-scale pores. Sci Rep 5: 10419. doi: 10.1038/srep10419
![]() |
[49] | Mereuta L, Roy M, Asandei A, et al. (2014) Slowing down single-molecule trafficking through a protein nanopore reveals intermediates for peptide translocation. Sci Rep 4: 3885. |
[50] |
Deamer D (2010) Nanopore analysis of nucleic acids bound to exonucleases and polymerases. Annu Rev Biophys 39: 79-90. doi: 10.1146/annurev.biophys.093008.131250
![]() |
[51] |
Olasagasti F, Lieberman KR, Benner S, et al. (2010) Replication of individual DNA molecules under electronic control using a protein nanopore. Nat Nanotechnol 5: 798-806. doi: 10.1038/nnano.2010.177
![]() |
[52] |
Chu J, Gonzalez-Lopez M, Cockroft SL, et al. (2010) Real-time monitoring of DNA polymerase function and stepwise single-nucleotide DNA strand translocation through a protein nanopore. Angew Chem Int Ed Engl 49: 10106-10109. doi: 10.1002/anie.201005460
![]() |
[53] |
Benner S, Chen RJ, Wilson NA, et al. (2007) Sequence-specific detection of individual DNA polymerase complexes in real time using a nanopore. Nat Nanotechnol 2: 718-724. doi: 10.1038/nnano.2007.344
![]() |
[54] |
Asandei A, Iftemi S, Mereuta L, et al. (2014) Probing of various physiologically relevant metals: Amyloid-β peptide interactions with a lipid membrane-immobilized protein nanopore. J Membrane Biol 247: 523-530. doi: 10.1007/s00232-014-9662-z
![]() |
[55] |
Asandei A, Schiopu I, Iftemi S, et al. (2013) Investigation of Cu2+ binding to human and rat amyloid fragments Aβ (1-16) with a protein nanopore. Langmuir 29: 15634-15642. doi: 10.1021/la403915t
![]() |
[56] |
Ying YL, Wang HY, Sutherland TC, et al. (2011) Monitoring of an ATP-binding aptamer and its conformational changes using an α-hemolysin nanopore. Small 7: 87-94. doi: 10.1002/smll.201001428
![]() |
[57] |
Clarke J, Wu HC, Jayasinghe L, et al. (2009) Continuous base identification for single-molecule nanopore DNA sequencing. Nat Nanotechnol 4: 265-270. doi: 10.1038/nnano.2009.12
![]() |
[58] |
Branton D, Deamer DW, Marziali A, et al. (2008) The potential and challenges of nanopore sequencing. Nat Biotechnol 26: 1146-1153. doi: 10.1038/nbt.1495
![]() |
[59] |
Bayley H (2006) Sequencing single molecules of DNA. Curr Opin Chem Biol 10: 628-637. doi: 10.1016/j.cbpa.2006.10.040
![]() |
[60] |
Bartel DP (2004) MicroRNAs: Genomics, Biogenesis, Mechanism, and Function. Cell 116: 281-297. doi: 10.1016/S0092-8674(04)00045-5
![]() |
[61] |
Carthew RW, Sontheimer EJ (2009) Origins and Mechanisms of miRNAs and siRNAs. Cell 136: 642-655. doi: 10.1016/j.cell.2009.01.035
![]() |
[62] |
Calin GA, Croce CM (2006) MicroRNA signatures in human cancers. Nat Rev Cancer 6: 857-866. doi: 10.1038/nrc1997
![]() |
[63] |
Chen X, Ba Y, Ma L, et al. (2008) Characterization of microRNAs in serum: A novel class of biomarkers for diagnosis of cancer and other diseases. Cell Res 18: 997-1006. doi: 10.1038/cr.2008.282
![]() |
[64] |
Mitchell PS, Parkin RK, Kroh EM, et al. (2008) Circulating microRNAs as stable blood-based markers for cancer detection. Proc Natl Acad Sci U S A 105: 10513-10518. doi: 10.1073/pnas.0804549105
![]() |
[65] |
Wang Y, Zheng D, Tan Q, et al. (2011) Nanopore-based detection of circulating microRNAs in lung cancer patients. Nat Nanotechnol 6: 668-674. doi: 10.1038/nnano.2011.147
![]() |
[66] |
Tian K, He Z, Wang Y, et al. (2013) Designing a polycationic probe for simultaneous enrichment and detection of microRNAs in a nanopore. ACS Nano 7: 3962-3969. doi: 10.1021/nn305789z
![]() |
[67] |
Zhang X, Wang Y, Fricke BL, et al. (2014) Programming nanopore ion flow for encoded multiplex microRNA detection. ACS Nano 8: 3444-3450. doi: 10.1021/nn406339n
![]() |
[68] |
Cao C, Ying YL, Gu Z, et al. (2014) Enhanced resolution of low molecular weight poly(ethylene glycol) in nanopore analysis. Anal Chem 86: 11946-11950. doi: 10.1021/ac504233s
![]() |
[69] |
Baral A, Kumar P, Pathak R, et al. (2013) Emerging trends in G-quadruplex biology-role in epigenetic and evolutionary events. Mol BioSyst 9: 1568-1575. doi: 10.1039/c3mb25492e
![]() |
[70] |
Frees S, Menendez C, Crum M, et al. (2014) QGRS-Conserve: A computational method for discovering evolutionarily conserved G-quadruplex motifs. Human Genomics 8: 8. doi: 10.1186/1479-7364-8-8
![]() |
[71] |
Paeschke K, Bochman ML, Daniela Garcia P, et al. (2013) Pif1 family helicases suppress genome instability at G-quadruplex motifs. Nature 497: 458-462. doi: 10.1038/nature12149
![]() |
[72] |
Qiu J, Wang M, Zhang Y, et al. (2015) Biological function and medicinal research significance of g-quadruplex interactive proteins. Curr Top Med Chem 15: 1971-1987. doi: 10.2174/1568026615666150515150803
![]() |
[73] |
Fleming AM, Burrows CJ (2013) G-quadruplex folds of the human telomere sequence alter the site reactivity and reaction pathway of guanine oxidation compared to duplex DNA. Chem Res Toxicol 26: 593-607. doi: 10.1021/tx400028y
![]() |
[74] |
An N, Fleming AM, Middleton EG, et al. (2014) Single-molecule investigation of G-quadruplex folds of the human telomere sequence in a protein nanocavity. Proc Natl Acad Sci U S A 111: 14325-14331. doi: 10.1073/pnas.1415944111
![]() |
[75] |
An N, Fleming AM, Burrows CJ (2013) Interactions of the human telomere sequence with the nanocavity of the α-hemolysin ion channel reveal structure-dependent electrical signatures for hybrid folds. J Am Chem Soc 135: 8562-8570. doi: 10.1021/ja400973m
![]() |
[76] |
Wolna AH, Fleming AM, Burrows CJ (2014) Single-molecule analysis of thymine dimer-containing G-quadruplexes formed from the human telomere sequence. Biochemistry 53: 7484-7493. doi: 10.1021/bi501072m
![]() |
[77] |
Shim JW, Gu LQ (2008) Encapsulating a single G-quadruplex aptamer in a protein nanocavity. J Phys Chem B 112: 8354-8360. doi: 10.1021/jp0775911
![]() |
[78] |
Shim JW, Tan Q, Gu LQ (2009) Single-molecule detection of folding and unfolding of the G-quadruplex aptamer in a nanopore nanocavity. Nucleic Acids Res 37: 972-982. doi: 10.1093/nar/gkn968
![]() |
[79] |
Shim J, Gu LQ (2012) Single-molecule investigation of G-quadruplex using a nanopore sensor. Methods 57: 40-46. doi: 10.1016/j.ymeth.2012.03.026
![]() |
[80] | Yang J, Xu ZP, Huang Y, et al. (2004) ATM and ATR: Sensing DNA damage. World J Gastroentero 10: 155-160. |
[81] |
Diculescu VC, Paquim AMC, Brett AMO (2005) Electrochemical DNA sensors for detection of DNA damage. Sensors 5: 377-393. doi: 10.3390/s5060377
![]() |
[82] |
Fojta M (2002) Electrochemical sensors for DNA interactions and damage. Electroanalysis 14: 1449-1463. doi: 10.1002/1521-4109(200211)14:21<1449::AID-ELAN1449>3.0.CO;2-Z
![]() |
[83] |
Paleček E, Bartošík M (2012) Electrochemistry of nucleic acids. Chem Rev 112: 3427-3481. doi: 10.1021/cr200303p
![]() |
[84] |
An N, Fleming AM, White HS, et al. (2015) Nanopore detection of 8-oxoguanine in the human telomere repeat sequence. ACS Nano 9: 4296-4307. doi: 10.1021/acsnano.5b00722
![]() |
[85] |
Boiteux S, Guillet M (2004) Abasic sites in DNA: Repair and biological consequences in Saccharomyces cerevisiae. DNA Repair 3: 1-12. doi: 10.1016/j.dnarep.2003.10.002
![]() |
[86] |
Wilson Iii DM, Barsky D (2001) The major human abasic endonuclease: Formation, consequences and repair of abasic lesions in DNA. Mutat Res-DNA Repair 485: 283-307. doi: 10.1016/S0921-8777(01)00063-5
![]() |
[87] | Reardon JT, Sancar A (2005) Nucleotide Excision Repair. In: Moldave K, editor. Progress in Nucleic Acid Research and Molecular Biology, 183-235. |
[88] |
Gates KS (2009) An overview of chemical processes that damage cellular DNA: Spontaneous hydrolysis, alkylation, and reactions with radicals. Chem Res Toxicol 22: 1747-1760. doi: 10.1021/tx900242k
![]() |
[89] |
Greco NJ, Tor Y (2005) Simple fluorescent pyrimidine analogues detect the presence of DNA abasic sites. J Am Chem Soc 127: 10784-10785. doi: 10.1021/ja052000a
![]() |
[90] |
Lucotti A, Tommasini M, Pezzoli D, et al. (2014) Molecular interactions of DNA with transfectants: A study based on infrared spectroscopy and quantum chemistry as aids to fluorescence spectroscopy and dynamic light scattering analyses. RSC Adv 4: 49620-49627. doi: 10.1039/C4RA08845J
![]() |
[91] |
Sun HB, Qian L, Yokota H (2001) Detection of abasic sites on individual DNA molecules using atomic force microscopy. Anal Chem 73: 2229-2232. doi: 10.1021/ac0013249
![]() |
[92] |
Bowler FR, Diaz-Mochon JJ, Swift MD, et al. (2010) DNA analysis by dynamic chemistry. Angew Chem Int Edit 49: 1809-1812. doi: 10.1002/anie.200905699
![]() |
[93] |
Atamna H, Cheung I, Ames BN (2000) A method for detecting abasic sites in living cells: Age-dependent changes in base excision repair. Proc Natl Acad Sci U S A 97: 686-691. doi: 10.1073/pnas.97.2.686
![]() |
[94] |
Kojima N, Takebayashi T, Mikami A, et al. (2009) Construction of highly reactive probes for abasic site detection by introduction of an aromatic and a guanidine residue into an aminooxy group. J Am Chem Soc 131: 13208-13209. doi: 10.1021/ja904767k
![]() |
[95] |
Zeglis BM, Boland JA, Barton JK (2008) Targeting abasic sites and single base bulges in DNA with metalloinsertors. J Am Chem Soc 130: 7530-7531. doi: 10.1021/ja801479y
![]() |
[96] |
Wang Y, Liu L, Wu C, et al. (2009) Direct detection and quantification of abasic sites for in vivo studies of DNA damage and repair. Nucl Med Biol 36: 975-983. doi: 10.1016/j.nucmedbio.2009.07.007
![]() |
[97] |
Hada M, Sutherland BM (2006) Spectrum of complex DNA damages depends on the incident radiation. Radiat Res 165: 223-230. doi: 10.1667/RR3498.1
![]() |
[98] |
An N, Fleming AM, White HS, et al. (2012) Crown ether-electrolyte interactions permit nanopore detection of individual DNA abasic sites in single molecules. Proc Natl Acad Sci U S A 109: 11504-11509. doi: 10.1073/pnas.1201669109
![]() |
[99] |
Jin Q, Fleming AM, Johnson RP, et al. (2013) Base-excision repair activity of uracil-DNA glycosylase monitored using the latch zone of α-hemolysin. J Am Chem Soc 135: 19347-19353. doi: 10.1021/ja410615d
![]() |
[100] |
Johnson RP, Fleming AM, Jin Q, et al. (2014) Temperature and electrolyte optimization of the α-hemolysin latch sensing zone for detection of base modification in double-stranded DNA. Biophys J 107: 924-931. doi: 10.1016/j.bpj.2014.07.006
![]() |
[101] | Lin YH, Tseng WL (2009) Highly sensitive and selective detection of silver ions and silver nanoparticles in aqueous solution using an oligonucleotide-based fluorogenic probe. Chem Commun (Camb): 6619-6621. |
[102] | Ono A, Cao S, Togashi H, et al. (2008) Specific interactions between silver(I) ions and cytosine-cytosine pairs in DNA duplexes. Chem Commun (Camb): 4825-4827. |
[103] |
Wen Y, Xing F, He S, et al. (2010) A graphene-based fluorescent nanoprobe for silver(I) ions detection by using graphene oxide and a silver-specific oligonucleotide. Chem Commun (Camb) 46: 2596-2598. doi: 10.1039/b924832c
![]() |
[104] |
Torigoe H, Okamoto I, Dairaku T, et al. (2012) Thermodynamic and structural properties of the specific binding between Ag(+) ion and C:C mismatched base pair in duplex DNA to form C-Ag-C metal-mediated base pair. Biochimie 94: 2431-2440. doi: 10.1016/j.biochi.2012.06.024
![]() |
[105] |
Miyake Y, Togashi H, Tashiro M, et al. (2006) MercuryII-mediated formation of thymine-HgII-thymine base pairs in DNA duplexes. J Am Chem Soc 128: 2172-2173. doi: 10.1021/ja056354d
![]() |
[106] |
Tanaka Y, Oda S, Yamaguchi H, et al. (2007) 15N-15N J-coupling across Hg(II): direct observation of Hg(II)-mediated T-T base pairs in a DNA duplex. J Am Chem Soc 129: 244-245. doi: 10.1021/ja065552h
![]() |
[107] |
Torigoe H, Ono A, Kozasa T (2010) Hg(II) ion specifically binds with T:T mismatched base pair in duplex DNA. Chemistry 16: 13218-13225. doi: 10.1002/chem.201001171
![]() |
[108] |
Wen S, Zeng T, Liu L, et al. (2011) Highly sensitive and selective DNA-based detection of mercury(II) with alpha-hemolysin nanopore. J Am Chem Soc 133: 18312-18317. doi: 10.1021/ja206983z
![]() |
[109] |
Ono A, Torigoe H, Tanaka Y, et al. (2011) Binding of metal ions by pyrimidine base pairs in DNA duplexes. Chem Soc Rev 40: 5855-5866. doi: 10.1039/c1cs15149e
![]() |
[110] |
Brena RM, Huang TH, Plass C (2006) Quantitative assessment of DNA methylation: Potential applications for disease diagnosis, classification, and prognosis in clinical settings. J Mol Med (Berl) 84: 365-377. doi: 10.1007/s00109-005-0034-0
![]() |
[111] |
Kriaucionis S, Heintz N (2009) The nuclear DNA base 5-hydroxymethylcytosine is present in Purkinje neurons and the brain. Science 324: 929-930. doi: 10.1126/science.1169786
![]() |
[112] |
Lister R, Pelizzola M, Dowen RH, et al. (2009) Human DNA methylomes at base resolution show widespread epigenomic differences. Nature 462: 315-322. doi: 10.1038/nature08514
![]() |
[113] | Li WW, Gong L, Bayley H (2013) Single-molecule detection of 5-hydroxymethylcytosine in DNA through chemical modification and nanopore analysis. Angew Chem Int Ed 52: 4350-4355. |
[114] | Shim J, Humphreys GI, Venkatesan BM, et al. (2013) Detection and quantification of methylation in DNA using solid-state nanopores. Sci Rep 3: 1389. |
[115] |
Wallace EV, Stoddart D, Heron AJ, et al. (2010) Identification of epigenetic DNA modifications with a protein nanopore. Chem Commun (Camb) 46: 8195-8197. doi: 10.1039/c0cc02864a
![]() |
[116] |
Wanunu M, Cohen-Karni D, Johnson RR, et al. (2011) Discrimination of methylcytosine from hydroxymethylcytosine in DNA molecules. J Am Chem Soc 133: 486-492. doi: 10.1021/ja107836t
![]() |
[117] |
Yu M, Hon GC, Szulwach KE, et al. (2012) Tet-assisted bisulfite sequencing of 5-hydroxymethylcytosine. Nat Protoc 7: 2159-2170. doi: 10.1038/nprot.2012.137
![]() |
[118] |
Yu M, Hon GC, Szulwach KE, et al. (2012) Base-resolution analysis of 5-hydroxymethylcytosine in the mammalian genome. Cell 149: 1368-1380. doi: 10.1016/j.cell.2012.04.027
![]() |
[119] |
Booth MJ, Branco MR, Ficz G, et al. (2012) Quantitative sequencing of 5-methylcytosine and 5-hydroxymethylcytosine at single-base resolution. Science 336: 934-937. doi: 10.1126/science.1220671
![]() |
[120] | Wang Y, Luan BQ, Yang Z, et al. (2014) Single Molecule Investigation of Ag+ Interactions with Single Cytosine-, Methylcytosine- and Hydroxymethylcytosine-Cytosine Mismatches in a Nanopore. Sci Rep 4: 5883. |
[121] |
Torigoe H, Miyakawa Y, Ono A, et al. (2011) Thermodynamic properties of the specific binding between Ag+ ions and C:C mismatched base pairs in duplex DNA. Nucleos Nucleot Nucl 30: 149-167. doi: 10.1080/15257770.2011.553210
![]() |
[122] |
Kistenmacher TJ, Rossi M, Marzilli LG (1979) Crystal and molecular structure of (nitrato)(1-methylcytosine)silver(I): An unusual cross-linked polymer containing a heavy metal and a modified nucleic acid constituent. Inorg Chem 18: 240-244. doi: 10.1021/ic50192a007
![]() |
[123] |
Urata H, Yamaguchi E, Nakamura Y, et al. (2011) Pyrimidine-pyrimidine base pairs stabilized by silver(I) ions. Chem Commun (Camb) 47: 941-943. doi: 10.1039/C0CC04091F
![]() |
[124] |
Wang Y, Ritzo B, Gu L-Q (2015) Silver (i) ions modulate the stability of DNA duplexes containing cytosine, methylcytosine and hydroxymethylcytosine at different salt concentrations. RSC Adv 5: 2655-2658. doi: 10.1039/C4RA14490B
![]() |
[125] |
Egger G, Liang G, Aparicio A, et al. (2004) Epigenetics in human disease and prospects for epigenetic therapy. Nature 429: 457-463. doi: 10.1038/nature02625
![]() |
[126] |
Bestor TH (2000) The DNA methyltransferases of mammals. Hum Mol Genet 9: 2395-2402. doi: 10.1093/hmg/9.16.2395
![]() |
[127] | Kang I, Wang Y, Reagan C, et al. (2013) Designing DNA interstrand lock for locus-specific methylation detection in a nanopore. Sci Rep 3: 2381. |
[128] |
Calin GA, Croce CM (2006) MicroRNA signatures in human cancers. Nat Rev Cancer 6: 857-866. doi: 10.1038/nrc1997
![]() |
[129] |
Vlassov VV, Laktionov PP, Rykova EY (2010) Circulating nucleic acids as a potential source for cancer biomarkers. Curr Mol Med 10: 142-165. doi: 10.2174/156652410790963295
![]() |
[130] |
Mitchell PS, Parkin RK, Kroh EM, et al. (2008) Circulating microRNAs as stable blood-based markers for cancer detection. Proc Natl Acad Sci U S A 105: 10513-10518. doi: 10.1073/pnas.0804549105
![]() |
[131] | Oremek GM, Sapoutzis N (2003) Pro-gastrin-releasing peptide (Pro-GRP), a tumor marker for small cell lung cancer. Anticancer Res 23: 895-898. |
[132] |
Molina R, Auge JM, Bosch X, et al. (2009) Usefulness of serum tumor markers, including progastrin-releasing peptide, in patients with lung cancer: correlation with histology. Tumour Biol 30: 121-129. doi: 10.1159/000224628
![]() |
[133] |
Albrethsen J, Moller CH, Olsen J, et al. (2006) Human neutrophil peptides 1, 2 and 3 are biochemical markers for metastatic colorectal cancer. Eur J Cancer 42: 3057-3064. doi: 10.1016/j.ejca.2006.05.039
![]() |
[134] |
Bassani-Sternberg M, Barnea E, Beer I, et al. (2010) Soluble plasma HLA peptidome as a potential source for cancer biomarkers. Proc Natl Acad Sci U S A 107: 18769-18776. doi: 10.1073/pnas.1008501107
![]() |
[135] |
Stella Tsai CS, Chen HC, Tung JN, et al. (2010) Serum cellular apoptosis susceptibility protein is a potential prognostic marker for metastatic colorectal cancer. Am J Pathol 176: 1619-1628. doi: 10.2353/ajpath.2010.090467
![]() |
[136] |
Stoeva SI, Lee JS, Smith JE, et al. (2006) Multiplexed detection of protein cancer markers with biobarcoded nanoparticle probes. J Am Chem Soc 128: 8378-8379. doi: 10.1021/ja0613106
![]() |
[137] |
Zheng W, He L (2010) Multiplexed detection of protein cancer markers on Au/Ag-barcoded nanorods using fluorescent-conjugated polymers. Anal Bioanal Chem 397: 2261-2270. doi: 10.1007/s00216-010-3834-1
![]() |
[138] | Jin H, Liu GL (2012) Fabrication and optical characterization of light trapping silicon nanopore and nanoscrew devices. Nanotechnology 23. |
[139] | Merchant CA, Drndić M (2012) Graphene nanopore devices for DNA sensing. In: Gracheva ME, editor. Methods in Molecular Biology, 211-226. |
[140] |
Park S, Lim J, Pak YE, et al. (2013) A solid state nanopore device for investigating the magnetic properties of magnetic nanoparticles. Sensors (Basel, Switzerland) 13: 6900-6909. doi: 10.3390/s130606900
![]() |
[141] |
Wu GS, Zhang Y, Si W, et al. (2014) Integrated solid-state nanopore devices for third generation DNA sequencing. Sci China Technol Sc 57: 1925-1935. doi: 10.1007/s11431-014-5644-8
![]() |
[142] | Inoue K, Kawano R, Yasuga H, et al. Logic operation in DNA nano device: Electrical input/output through biological nanopore; 2013; Freiburg. Chemical and Biological Microsystems Society. 1881-1883. |
[143] |
Lim MC, Lee MH, Kim KB, et al. (2015) A mask-free passivation process for low noise nanopore devices. J Nanosci Nanotechno 15: 5971-5977. doi: 10.1166/jnn.2015.10500
![]() |
[144] |
Mulero R, Prabhu AS, Freedman KJ, et al. (2010) Nanopore-Based Devices for Bioanalytical Applications. J Assoc Lab Automat 15: 243-252. doi: 10.1016/j.jala.2010.01.009
![]() |
[145] |
Cockroft SL, Chu J, Amorin M, et al. (2008) A single-molecule nanopore device detects DNA polymerase activity with single-nucleotide resolution. J Am Chem Soc 130: 818-820. doi: 10.1021/ja077082c
![]() |
[146] |
Kawano R, Osaki T, Sasaki H, et al. (2010) A polymer-based nanopore-integrated microfluidic device for generating stable bilayer lipid membranes. Small 6: 2100-2104. doi: 10.1002/smll.201000997
![]() |
[147] |
Shim JW, Gu LQ (2007) Stochastic sensing on a modular chip containing a single-ion channel. Anal Chem 79: 2207-2213. doi: 10.1021/ac0614285
![]() |
[148] |
Kang XF, Cheley S, Rice-Ficht AC, et al. (2007) A storable encapsulated bilayer chip containing a single protein nanopore. J Am Chem Soc 129: 4701-4705. doi: 10.1021/ja068654g
![]() |
[149] |
Eisenstein M (2012) Oxford Nanopore announcement sets sequencing sector abuzz. Nat Biotechnol 30: 295-296. doi: 10.1038/nbt0412-295
![]() |
[150] |
Quick J, Quinlan AR, Loman NJ (2014) A reference bacterial genome dataset generated on the MinIONTM portable single-molecule nanopore sequencer. GigaScience 3: 22. doi: 10.1186/2047-217X-3-22
![]() |
[151] | Ashton PM, Nair S, Dallman T, et al. (2015) MinION nanopore sequencing identifies the position and structure of a bacterial antibiotic resistance island. Nat Biotechnol 33: 296-302. |
[152] | Madoui MA, Engelen S, Cruaud C, et al. (2015) Genome assembly using Nanopore-guided long and error-free DNA reads. BMC Genomics 16. |
[153] |
Loman NJ, Quick J, Simpson JT (2015) A complete bacterial genome assembled de novo using only nanopore sequencing data. Nat Methods 12: 733-735. doi: 10.1038/nmeth.3444
![]() |
[154] | Palshikar P, Sharma A, Chauhan CS, et al. (2013) Biophotonics: A novel approach biomedical diagnosis. Int J Pharm Sci Rev Res 21: 350-354. |
[155] | Liu A, Ye B (2013) Application of gold nanoparticles in biomedical researches and diagnosis. Clin Lab 59: 23-36. |
[156] |
Nahar J, Imam T, Tickle KS, et al. (2012) Computational intelligence for microarray data and biomedical image analysis for the early diagnosis of breast cancer. Expert Syst Appl 39: 12371-12377. doi: 10.1016/j.eswa.2012.04.045
![]() |
[157] |
Kang JW, Lue N, Kong CR, et al. (2011) Combined confocal Raman and quantitative phase microscopy system for biomedical diagnosis. Biomed Opt Express 2: 2484-2492. doi: 10.1364/BOE.2.002484
![]() |
[158] |
Chen KI, Li BR, Chen YT (2011) Silicon nanowire field-effect transistor-based biosensors for biomedical diagnosis and cellular recording investigation. Nano Today 6: 131-154. doi: 10.1016/j.nantod.2011.02.001
![]() |
[159] |
Balas C (2009) Review of biomedical optical imaging - A powerful, non-invasive, non-ionizing technology for improving in vivo diagnosis. Meas Sci Technol 20: 104020. doi: 10.1088/0957-0233/20/10/104020
![]() |
[160] |
Ellis DI, Goodacre R (2006) Metabolic fingerprinting in disease diagnosis: Biomedical applications of infrared and Raman spectroscopy. Analyst 131: 875-885. doi: 10.1039/b602376m
![]() |
[161] |
Bottiroli G, Croce AC (2004) Autofluorescence spectroscopy of cells and tissues as a tool for biomedical diagnosis. Photoch Photobio Sci 3: 189-210. doi: 10.1039/b310627f
![]() |
[162] | Urbanova M, Plzak J, Strnad H, et al. (2010) Circulating nucleic acids as a new diagnostic tool. Cell Mol Biol Lett 15: 242-259. |
[163] |
Liu KJ, Brock MV, Shih Ie M, et al. (2010) Decoding circulating nucleic acids in human serum using microfluidic single molecule spectroscopy. J Am Chem Soc 132: 5793-5798. doi: 10.1021/ja100342q
![]() |
[164] |
Thompson L, Turko I, Murad F (2006) Mass spectrometry-based relative quantification of human neutrophil peptides 1, 2, and 3 from biological samples. Mol Immunol 43: 1485-1489. doi: 10.1016/j.molimm.2005.08.001
![]() |
[165] |
Wanunu M, Dadosh T, Ray V, et al. (2010) Rapid electronic detection of probe-specific microRNAs using thin nanopore sensors. Nat Nanotechnol 5: 807-814. doi: 10.1038/nnano.2010.202
![]() |
[166] |
Squires A, Meller A (2013) DNA Capture and Translocation through Nanoscale Pores-a Fine Balance of Electrophoresis and Electroosmosis. Biophys J 105: 543-544. doi: 10.1016/j.bpj.2013.06.008
![]() |
[167] |
Wanunu M, Morrison W, Rabin Y, et al. (2010) Electrostatic focusing of unlabelled DNA into nanoscale pores using a salt gradient. Nat Nanotechnol 5: 160-165. doi: 10.1038/nnano.2009.379
![]() |
[168] |
Hatlo MM, Panja D, van Roij R (2011) Translocation of DNA molecules through nanopores with salt gradients: the role of osmotic flow. Phys Rev Lett 107: 068101. doi: 10.1103/PhysRevLett.107.068101
![]() |
[169] |
He Y, Tsutsui M, Scheicher RH, et al. (2013) Mechanism of How Salt-Gradient-Induced Charges Affect the Translocation of DNA Molecules through a Nanopore. Biophys J 105: 776-782. doi: 10.1016/j.bpj.2013.05.065
![]() |
[170] |
Chou T (2009) Enhancement of charged macromolecule capture by nanopores in a salt gradient. J Chem Phys 131: 034703. doi: 10.1063/1.3170952
![]() |
1. | Caterina Calgaro, Emmanuel Creusé, 2020, Chapter 37, 978-3-030-43650-6, 405, 10.1007/978-3-030-43651-3_37 | |
2. | Marianne Bessemoulin-Chatard, Claire Chainais-Hillairet, Hélène Mathis, 2020, Chapter 5, 978-3-030-43650-6, 75, 10.1007/978-3-030-43651-3_5 | |
3. | Marianne Bessemoulin-Chatard, Claire Chainais-Hillairet, Hélène Mathis, Analysis of numerical schemes for semiconductor energy-transport models, 2021, 168, 01689274, 143, 10.1016/j.apnum.2021.05.030 | |
4. | Caterina Calgaro, Clément Cancès, Emmanuel Creusé, Discrete Gagliardo–Nirenberg inequality and application to the finite volume approximation of a convection–diffusion equation with a Joule effect term, 2024, 44, 0272-4979, 2394, 10.1093/imanum/drad063 |
Diffusion term $ \theta_\sigma^{n+1}= $ |
|||
$ \dfrac{ \theta_K^{n+1}+ \theta_{K, \sigma}^{n+1}}{2} $ | $ \max(\theta_K^{n+1}, \theta_{K, \sigma}^{n+1}) $ | ||
Joule Term | $ \dfrac{1}{2\, m_K}\sum\limits_{\sigma \in \mathcal{E}_K}^{} \tau_\sigma (\theta_{K}^{n+1}- \theta_{K, \sigma}^{n+1})^2 $ | $ (\mathcal{S} \mathcal{D}_\mathrm{moy} \mathcal{J}_\mathrm{cen}) $ | $ (\mathcal{S} \mathcal{D}_\mathrm{max} \mathcal{J}_\mathrm{cen}) $ |
$ \mathcal{J}_K(\theta_ \mathcal{T}^{n+1})= $ | $ \dfrac{1}{m_K}\sum\limits_{\sigma \in \mathcal{E}_K}^{} \tau_\sigma \left((\theta_{K}^{n+1}- \theta_{K, \sigma}^{n+1})^+\right)^2 $ | $ (\mathcal{S} \mathcal{D}_\mathrm{moy} \mathcal{J}_\mathrm{up}) $ | $\Huge{ \times} $ |
$ \left(\dfrac{1}{m_K}\sum_{\sigma \in \mathcal{E}_K}^{} \tau_\sigma (\theta_K^{n+1}- \theta_{K, \sigma}^{n+1}) (\textbf{x}_\sigma- \textbf{x}_K)\right)^2 $ | $ (\mathcal{S} \mathcal{D}_\mathrm{moy} \mathcal{J}_\mathrm{EGH}) $ | $ (\mathcal{S} \mathcal{D}_\mathrm{max} \mathcal{J}_\mathrm{EGH}) $ |
![]() |
$ (\mathcal{S} \mathcal{D}_\mathrm{moy} \mathcal{J}_\mathrm{EGH}) $ | $ (\mathcal{S} \mathcal{D}_\mathrm{max} \mathcal{J}_\mathrm{EGH}) $ | $ (\mathcal{S} \mathcal{D}_\mathrm{moy} \mathcal{J}_\mathrm{cen}) $ | $ (\mathcal{S} \mathcal{D}_\mathrm{max} \mathcal{J}_\mathrm{cen}) $ | $ (\mathcal{S} \mathcal{D}_\mathrm{moy} \mathcal{J}_\mathrm{up}) $ |
2 | $ \checkmark $ | $ \checkmark $ | $ \checkmark $ | $ \checkmark $ | $ \checkmark $ |
3 | $ {\LARGE \times} $ | $ \checkmark $ | $ \checkmark $ | $ \checkmark $ | $ \checkmark $ |
4 | $ {\LARGE \times} $ | $ \checkmark $ | $ {\LARGE \times} $ | $ \checkmark $ | $ \checkmark $ |
10 | $ {\LARGE \times} $ | $ {\LARGE \times} $ | $ {\LARGE \times} $ | $ \checkmark $ | $ \checkmark $ |
100 | $ {\LARGE \times} $ | $ {\LARGE \times} $ | $ {\LARGE \times} $ | $ \checkmark $ | $ \checkmark $ |
Diffusion term $ \theta_\sigma^{n+1}= $ |
|||
$ \dfrac{ \theta_K^{n+1}+ \theta_{K, \sigma}^{n+1}}{2} $ | $ \max(\theta_K^{n+1}, \theta_{K, \sigma}^{n+1}) $ | ||
Joule Term | $ \dfrac{1}{2\, m_K}\sum\limits_{\sigma \in \mathcal{E}_K}^{} \tau_\sigma (\theta_{K}^{n+1}- \theta_{K, \sigma}^{n+1})^2 $ | $ (\mathcal{S} \mathcal{D}_\mathrm{moy} \mathcal{J}_\mathrm{cen}) $ | $ (\mathcal{S} \mathcal{D}_\mathrm{max} \mathcal{J}_\mathrm{cen}) $ |
$ \mathcal{J}_K(\theta_ \mathcal{T}^{n+1})= $ | $ \dfrac{1}{m_K}\sum\limits_{\sigma \in \mathcal{E}_K}^{} \tau_\sigma \left((\theta_{K}^{n+1}- \theta_{K, \sigma}^{n+1})^+\right)^2 $ | $ (\mathcal{S} \mathcal{D}_\mathrm{moy} \mathcal{J}_\mathrm{up}) $ | $\Huge{ \times} $ |
$ \left(\dfrac{1}{m_K}\sum_{\sigma \in \mathcal{E}_K}^{} \tau_\sigma (\theta_K^{n+1}- \theta_{K, \sigma}^{n+1}) (\textbf{x}_\sigma- \textbf{x}_K)\right)^2 $ | $ (\mathcal{S} \mathcal{D}_\mathrm{moy} \mathcal{J}_\mathrm{EGH}) $ | $ (\mathcal{S} \mathcal{D}_\mathrm{max} \mathcal{J}_\mathrm{EGH}) $ |
![]() |
$ (\mathcal{S} \mathcal{D}_\mathrm{moy} \mathcal{J}_\mathrm{EGH}) $ | $ (\mathcal{S} \mathcal{D}_\mathrm{max} \mathcal{J}_\mathrm{EGH}) $ | $ (\mathcal{S} \mathcal{D}_\mathrm{moy} \mathcal{J}_\mathrm{cen}) $ | $ (\mathcal{S} \mathcal{D}_\mathrm{max} \mathcal{J}_\mathrm{cen}) $ | $ (\mathcal{S} \mathcal{D}_\mathrm{moy} \mathcal{J}_\mathrm{up}) $ |
2 | $ \checkmark $ | $ \checkmark $ | $ \checkmark $ | $ \checkmark $ | $ \checkmark $ |
3 | $ {\LARGE \times} $ | $ \checkmark $ | $ \checkmark $ | $ \checkmark $ | $ \checkmark $ |
4 | $ {\LARGE \times} $ | $ \checkmark $ | $ {\LARGE \times} $ | $ \checkmark $ | $ \checkmark $ |
10 | $ {\LARGE \times} $ | $ {\LARGE \times} $ | $ {\LARGE \times} $ | $ \checkmark $ | $ \checkmark $ |
100 | $ {\LARGE \times} $ | $ {\LARGE \times} $ | $ {\LARGE \times} $ | $ \checkmark $ | $ \checkmark $ |